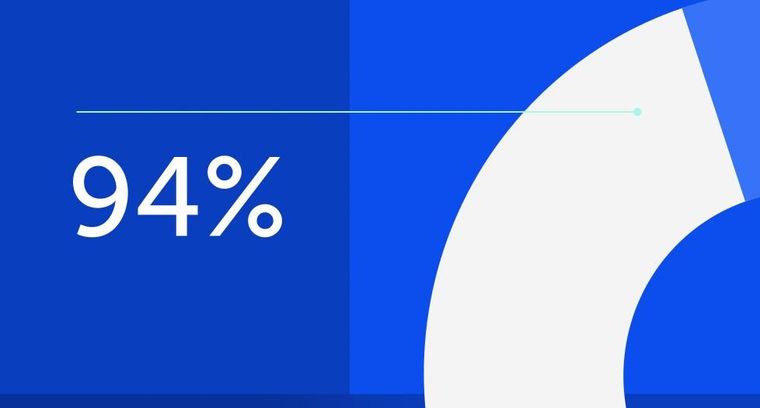
94% of researchers rate our articles as excellent or good
Learn more about the work of our research integrity team to safeguard the quality of each article we publish.
Find out more
ORIGINAL RESEARCH article
Front. Mar. Sci., 26 July 2022
Sec. Marine Megafauna
Volume 9 - 2022 | https://doi.org/10.3389/fmars.2022.938033
Wind patterns shape migratory pathways and detours of many procellariiform bird species that seasonally migrate between hemispheres. These seabirds are long-lived, and the period of immaturity is presumed to be a time of development and learning the environment, specifically how to use wind to their advantage. We assess how wind encountered by individual Great Shearwaters (Ardenna gravis) varies along the migration journey and compare responses between presumed mature and immature birds (early and late, respectively) in southbound migration and mature birds in northbound migration. We analyze modeled Argos locations from 71 individual tracks of migratory Great Shearwaters with concurrent U (East/West) and V (North/South) wind components. Migration in seabirds is well studied, but there is limited quantitative work measuring individual birds directly interacting with wind and their associated changes in flight behavior during migration. We show that Great Shearwaters made optimal use of winds, and that different age groups made decisions that exposed them to different wind constraints. Overall, Great Shearwaters derived positive responses from wind under most conditions and did not rely on a drifting strategy, which would be suggested if wind effect (difference between ground and airspeed) was predominantly positive during migrations. Instead, they appeared to use a compensating strategy to achieve an acceptable course and speed. The difference we observed in migration phenologies suggests that by migrating later, immature birds might travel the path of least resistance and experience flight conditions that are less risky furthering their ability to withstand a variety of wind conditions encountered later in life as done by adults, which migrate earlier and are subject to more variable flight conditions. We conclude that like other procellariiforms, a longer period of sexual maturity is required to enhance flight performance and mediate energy expenditure through experiential learning and increased fitness.
The adaptive behavior of bird flight relative to wind has important implications for migration, orientation, foraging, and energetics (Enri et al., 2005; Shamoun-Baranes et al., 2007). Winds influence the transport economy of birds and the selection of favorable tailwinds for migratory flights is of overriding importance for optimal migration performance (Liechti and Bruderer, 1998). Long-distance migrants cross or circumvent large barriers such as oceans or deserts with ramifications (e.g., wind selectivity, stop-overs, detours, predation) for navigation, energetics, and survival. Optimal use of wind is obtained by selecting trajectories that avoid headwinds and minimize the potential negative effects of wind on energy expenditure associated with geographic barriers (Alerstam, 2011; Gill et al., 2014). Focusing on spatial and temporal variability and ways in which migration is correlated with environmental conditions can further our understanding of the scope of flexibility in migratory behavior (Vardanis et al., 2011).
Many species of the order Procellariiformes (albatrosses, petrels, shearwaters, storm petrels) are inter-hemispheric migrants and have evolved on the high seas and their associated wind patterns (Warham, 1996). The optimal path for several species of shearwaters migrating across the Equator between breeding and wintering areas, is not necessarily the shortest path (Schaffer et al., 2006; Dell’Ariccia et al., 2018). These birds rather often fly longer distances along corridors where winds are more favorable, and flight requires less energy (Felicisimo et al., 2008; González-Solis et al., 2009). When cruising with favorable tail or side winds, Wandering Albatrosses (Diomedea exulans) achieve high flight speeds while expending little more energy than birds resting on land (Weimerskirch et al., 2000). Whether it is while foraging or migrating, petrel and shearwater movements clearly benefit from favorable wind strength and direction (Schaffer et al., 2006; Adams and Flora, 2010; Ventura et al., 2020), yet intensification of storm activities may increase seabird mortality by forcing them off migratory routes or causing them to spend too much energy just to survive in rough seas (Tavares et al., 2019).
Investigating the link between seabird movements and wind conditions over vast expanses of the open ocean requires knowledge of a bird’s location, and its travel direction relative to wind direction and magnitude (e.g., Adams and Flora, 2010). Satellite-tracking devices such as platform terminal transmitters (PTTs) mounted on birds provide fine-scale (km range) data on location, time, and travel direction that can be used to infer a bird’s behavioral state (e.g., area-restricted search or transit) (Powers et al., 2017). These data can be combined with satellite-derived data for location-specific wind direction and magnitude (e.g., Illan et al., 2017), thereby allowing calculation of times and areas in which flight is wind-aided. During long-distance migration periods, these data can also be used to calculate where birds need to compensate for changing wind conditions to reach destinations, such as a nesting site or foraging areas.
In most procellariform species, juveniles leave their natal grounds independently from their parents, and thus explore and learn the environment on their own (de Grissac et al., 2016). There are innate aspects of juvenile movements that correspond to those of adults (Sergio et al., 2014), but timing, movement behavior, or route choices often differ (Thiers et al., 2014). These birds are long-lived with varying periods of immaturity before they return to nesting colonies to breed: 5 – 9 years for shearwaters (Bradley et al., 1999; Fletcher et al., 2013; Campioni et al., 2019), and 3 – 12 years for albatrosses (Porter and Colson, 1987; Crespin et al., 2006; Orben et al., 2018). This period of immaturity in Wandering Albatross is related to experiential learning about spatial, behavioral, and resource constraints (Riotte-Lambert and Weimerskirch, 2013). Likewise, Cory’s Shearwaters (Calonectris diomedea) show remarkable age-related differences in migration timing, trajectory, and oceanographic conditions experienced (Péron and Grémillet, 2013). Given their longevity, the extended period of immaturity in procellariiforms is a time when individuals must learn to navigate within their changing environment while responding adequately to the phenology of changing seasons (Campioni et al., 2019). Such developmental learning is essential to survive and then recruit to the breeding population.Foraging and home ranges of Great Shearwaters (Ardenna gravis) have been examined in the S Atlantic (Ronconi et al., 2018; Schoombie et al., 2018) and western N Atlantic (Brown, 1986; Powers et al., 2017; Powers et al., 2020), but we understand little about their interhemispheric migrations between breeding and wintering areas except for earlier accounts summarized by Cramp and Simmons (1977). The goal of this work is to describe Great Shearwater migration and investigate migration trajectories in relation to age, orientation, and spatial interactions with wind. Previous studies in the western N Atlantic found that older Great Shearwaters tend to migrate earlier than juveniles (Powers et al., 2017; Ronconi et al., 2018; Powers et al., 2020). Moreover, the longer period of immaturity in procellariiforms is thought to be reflective of developmental learning, as suggested by existing differences in migration strategies between younger birds and adults at age of breeding (Orben et al., 2018; Campioni et al., 2019). Thus, we predict that early migrants (adults) are confronted with different wind conditions during the southbound migration over the Equator than late migrants (immature birds) (Felicisimo et al., 2008).
Great Shearwaters (n = 128) were tracked with PTTs deployed at three sites in the western N Atlantic during their non-breeding season, and while breeding in the Tristan da Cunha archipelago (Inaccessible and Gough Islands) from the S Atlantic (Table 1). The locations and techniques used to capture and deploy these PTTs are described in Powers et al. (2017); Ronconi et al. (2018), and Powers et al. (2020). PTTs used for birds in New Brunswick, Maine, and Tristan da Cunha were battery-only Kiwi Sat 202 PTT from SirTrack Wildlife Tracking Solutions (30 g). Solar PTT-100 tags from Microwave Telemetry (15 g) were used in Massachusetts. Duty cycles for tags deployed in Massachusetts were 24-hr [on]; tags used in Maine and New Brunswick were set at 8-hr [on] and 16-hr [off]; tags deployed on breeding birds in Tristan da Cunha were set at 8-h [on] and 42-h [off]. Argos locations were obtained from the time of their release until the transmitters stopped working. No device information was available when transmissions ended. Sex was identified genetically from blood or feather samples provided (see Acknowledgements).
Table 1 Breakdown by location, years, mean PTT1 longevity, and total number of Great Shearwaters tagged, and number of individuals used in this study.
To improve the confidence of Argos location estimates and evenness of sampling intervals along chronologically ordered tracks (Reid et al., 2014; Jodice et al., 2016), we fitted a Bayesian switching state-space model (SSSM) to all tracking data using the ‘bsam’ (v 1.1.3) R package (Jonsen et al., 2005; Jonsen et al., 2013). While a standard state-space model provides improved location estimates and evenness of sampling, the “switching” part of the model provides an estimated state behavior expressed as a coefficient (b) on a scale between 1 and 2, representing transitory or area-restricted search (ARS) behaviors, respectively (Jonsen et al., 2013; Powers et al., 2017). Estimated locations were derived using the hierarchical first difference correlated random walk with switching method (hDCRWS), where all individuals were modeled collectively by year tagged. The modeling approach used a Markov chain Monte Carlo method with 10,000 iterations (thinned by every 10th record) after a burn-in of 40,000 iterations to eliminate effects of initial values. The model was run with a 6-h time-step to match the time resolution of derived wind data.
Since the behavior estimate (b) is two-state but on a continuous scale and we used PTTs with differing duty cycles, we applied a confidence interval around each behavior: b >= 1.75 for ARS and b<= 1.25 for transit (Silva et al., 2013; Powers et al., 2017; Ronconi et al., 2018). We considered migrating behavior as a category on its own given the bird’s intent is clearly distinct during this time of their annual cycle (i.e., achieving migration). Start of migration was determined using a combination of two days of consistent transit behavior combined with continual unidirectional change in latitude or longitude, as two days distinguished migration from short-term transits between foraging sites during non-migratory periods. Migration ended when there was no further unidirectional change in latitude or longitude combined with mostly ARS behavior over two days. Finally, we eliminated any individual that did not start or show migratory behavior, leaving 71 birds for further analysis (Table 1). Most excluded birds came from the SW Gulf of Maine, which comprised mostly younger birds and PTTs were deployed 1.5 months earlier (Powers et al., 2020) than those in the other parts of the Gulf of Maine, which were mostly older birds (Powers et al., 2017; Ronconi et al., 2018).
Wind vector data was obtained from the North American Regional Reanalysis dataset via the ‘RNCEP’ R package (Kemp et al., 2012) with a spatial resolution of 2.5° x 2.5°, and a temporal resolution of 6 h. We extracted the U (East/West) and V (North/South) wind components at each predicted SSSM location for each bird (n = 71) as measured at the surface. Shearwaters typically fly within 2 m of the ocean’s surface (Pennycuick, 1982). Interpolation in time and space was used so the resulting wind components were adjusted using a trilinear interpolation in latitude, longitude, and time (Kemp et al., 2012). Following Shamoun-Baranes et al. (2007), using the SSSM locations and data on wind, we calculated three orthogonal vectors essential in the study of bird flight in relation to wind. A vector (velocity) is made of two components namely its magnitude (speed), and its direction.
We first determined ground speed (m·s-1) and track bearing (°), the magnitude and direction components of the ground vector (Vg). The ground vector is associated with the observed trajectory of the bird. We then calculated the two components of the wind vector (Vw): the wind speed, the square root of the sum of squares of the east- and northward components (U and V) (Shamoun-Baranes et al., 2007); and the wind direction, defined as the direction toward which the wind is blowing. Lastly, we calculated the airspeed vector (Va) and its components, namely airspeed and true heading of the bird’s displacement relative to the airflow (see Shamoun-Baranes et al., 2007). This vector can be understood as the original bird displacement if the bird was moving in no wind conditions. Thus, on a perfectly still day Va= Vg. Accordingly, the airspeed vector (Va) is equal to the difference between the ground vector (Vg) and the wind vector (Vw). The airspeed vector also represents the energy expended in given wind conditions (Shamoun-Baranes et al., 2007; Kogure et al., 2016). We calculated wind support (Vs), which represents how ground speed in the direction of flight is influenced by wind. In other words, wind support is the resulting wind speed experienced by the bird in its direction of travel. Wind support is calculated as:
where θ is the angle between the wind vector (Vw) and the ground vector (Vg). Wind support can be negative (headwind) or positive (tailwind). We considered relative wind support (relVs), a metric spanning from -1 to 1 that quantifies the use of wind by birds independently of the wind speed, with -1 meaning the bird flies directly into the wind, and +1 the bird flies exactly in the direction of the wind. We also calculated wind effect (We), which is the difference between ground speed and airspeed (i.e., the effect wind has on the flight speed whether it is negative or positive). Table 2 lists and describes each of these wind/flight metrics.
Table 2 List and definition of wind/flight metrics apparent to Great Shearwaters at each predicted switching state-space model location-time.
Migration periods were separated by flight direction: southbound and northbound. We defined migratory pathways by calculating kernel density utilization distributions of migrating birds using the ‘adehabitatHR’ package (Calenge, 2006) in R (R Core Team, 2020). Based on migration start-date, we separated southbound migrants into two groups: early (mid-August to late-September) and late (October to December), which we attributed to age (Figure 1; Powers et al., 2020). Since we only tagged known breeding birds on nesting islands for the northbound migration, only a single age group (adult) was described.
Figure 1 Histogram of starting dates for Great Shearwaters by calendar week showing bimodal peaks by early (n = 42) and late (n = 20) phases of the southbound migration. ‘early’ peak is 4-Sep and occurred in week 36, and ‘late’ peak is 16-Nov and occurred in week 46.
We examined the statistical significance of selected comparisons of wind/flight metrics using Spearman’s Rank Correlation Test and used the correlation coefficient (rho) to assess how well the relationship between the selected variables could be described using a monotonic function. Based on literature and logic, we expected correlations between selected wind analysis parameters; Vg and Vs should be positively correlated as ground speed is expected to increase with positive wind support (Safi et al., 2013; Kogure et al., 2016; Krietsch et al., 2020), and We and Vashould be negatively correlated as a bird experiencing negative wind effect is expected to expend more energy to continue flight (Shamoun-Baranes et al., 2007).
To examine the relationship between flight metrics and latitude during migrations we used generalized additive mixed models (GAMM) including individual as a random effect. For southbound migrants, we tested for differences between early and late migrants, and thus added migrant group into the GAMM as a fixed term and as the “by” argument within the smooth term (Wood, 2017). We then compared a model with the fixed term migrant group to a model without fixed term using a likelihood ratio test to assess whether migrant group explained differences in the relationship between flight metrics and latitude. For each GAMM model, we limited the number of knots to five to ease interpretation of results. For each migration, we present the trend in metrics along latitudes, but also present the smooth function obtained from the final GAMM to describe the effect of latitude on the flight metrics.
To explore migration path decisions and orientation, we divided southbound and northbound migrations into five and four areas based on the geography of the migration trajectory and where there were principal changes in wind patterns. The boundary limits of each geographic area are shown in Figures 2 and 3. The areas are ordered from start to finish of migration. The southbound migration starts on wintering areas in the western N Atlantic and tracks birds going across the Equator into the S Atlantic. The northbound migration tracks birds from the Patagonian Shelf back into the western N Atlantic after the breeding season. We considered ground track bearing, true heading, and wind direction. The circular values (angles) did not follow the von Misses distribution (all; p< 0.05), and the concentration parameter (measure of dispersion and uniformity of distribution of angles) differed between areas-migrant groups (southbound), or areas (northbound). Thus, we used Watson-Wheeler non-parametric test to examine if either the mean or variance differed between groups compared. We also examined if migration duration and distance differed by migration groups using analysis of variance (ANOVA).
Figure 2 Utilization distribution areas at 50% and 25% densities (yellow and red polygons) are shown for (A) early (n = 42) and (C) late (n = 20) southbound migrations of Great Shearwaters with locations of each predicted location (blue dots). Relative wind support (relVs) along individual trajectories is shown for (B) early and (D) late migrants. For relative wind support, a value of -1 means perfect headwinds and a value of +1 means perfect tailwinds. The Atlantic Ocean was broken into five geographic areas (1S – 5S) from north to south based primarily on latitude. Four-pointed stars denote tagging areas within the Gulf of Maine in the N Atltantic and hexagonal stars denote breeding colonies on Inaccessible and Gough Islands in Tristan da Cunha in the S Atlantic.
Figure 3 (A) Utilization distribution areas at 50% and 25% densities (yellow and red polygons) of the northbound migration of Great Shearwaters (n = 10) with locations of each predicted location (blue dots). (B) Relative wind support (relVs) along individual trajectories. For relative wind support, a value of -1 means perfect headwinds and a value of +1 means perfect tailwinds. The Atlantic Ocean was broken into four geographic areas (1N – 4N) based on latitude from south to north. Four-pointed stars denote tagging areas within the Gulf of Maine in the N Atltantic and hexagonal stars denote breeding colonies on Inaccessible and Gough Islands in Tristan da Cunha in the S Atlantic.
Using the entire dataset of 41,722 SSSM locations obtained from 71 birds, 7,472 locations (18%) were birds in migration (6,570 southbound and 902 northbound). We corroborated the expected correlations for the three migration groups (S – early, S – late, N) between wind support (Vs) and ground speed (Vg), and airspeed (Va) and wind effect (Table 3). As expected, Vgwas positively aided by Vsduring migration (Table 3). The overall magnitude of wind effect on Vawas negatively correlated as birds would increase Vawhen facing headwinds, but it nevertheless resulted in reduced Vg. Hence, the effect of wind on flight in terms of speed achieved for energy expended was negative, on average.
Table 3 Spearman’s rank correlation coefficients (rho) by migration direction (North or South) and age group (‘early’ or ‘late’) for 71 Great Shearwaters for selected statistically significant wind interactions.
We examined the southbound migration of Great Shearwaters based on datasets from 62 individuals tagged in the Gulf of Maine off the NE United States and Bay of Fundy off New Brunswick, Canada. One bird tagged while breeding on Inaccessible Island in the middle of the S Atlantic was evaluated in both directions. Another bird tagged in the SW Gulf of Maine was determined to be a female using a feather sample and went directly to Inaccessible Island maintaining the schedule expected of a breeding female (Cuthbert, 2005; Ronconi et al., 2010; Ronconi et al., 2018). Only two of the birds tracked southbound were confirmed breeding adults. However, most early migrants (83%) but zero late migrants later visited the breeding colonies. Starting dates of the southbound migration showed a bimodal distribution (Figure 1); an ‘early’ peak on 4 September followed by a ‘late’ peak on 16 November (Table 4). Following Powers et al. (2020), early migrants were believed to be older (subadults and adults), and late migrants were younger (primarily juveniles). PTTs for 86% of early migrants lasted long enough to record the end of migration as opposed to only 35% of the late migrants. SirTrack PTTs deployed in W Gulf of Maine and Bay of Fundy lasted for 112 – 129 days (Table 1) and were deployed in late August to mid-September on mostly early migrants. By comparison, Microwave Telemetry PTTs used in the SW Gulf of Maine lasted 101 days (Table 1) and were deployed in mid-July on mostly late migrants, which did not migrate until November. The southbound migration took 27 – 32 days to complete. Although early migrants took on average 5 days less to complete it, and consistently traveled over a longer distance and at a higher rate of speed than late migrants, these differences were not significant (Table 4; duration: t = -1.36, df = 6.5, p = 0.2189; distance: t = 1.05, df = 8.0, p = 0.323).
The post-breeding northward migration of Great Shearwaters was based on 10 individuals, nine tagged on Inaccessible Island and one on Gough Island. The flight from the Patagonian Shelf off Argentina started on 26 May, was shorter, and took less time to complete than the southbound migration (Table 4). Although the overall distance traveled north was 1622 km shorter than going south, the distance covered per day, and velocity of Northbound Migrants were nearly equivalent to that of early migrants going south (Table 4). Only 4 of 10 birds were tracked all the way to their non-breeding areas. Six PTTs stopped transmitting between NE Brazil and west to Tobago and Trinidad, or north to Puerto Rico along with islands of the Lesser Antilles. The dates of their last locations ranged from 18-June to 11-July; these PTTs may have stopped transmitting due to tag failure as the average duration of all PTTs deployed from Tristan da Cunha was 202 days (Table 1), which occurred on 19 June. One-way ANOVA showed no significant difference in means of the three migrant groups for migration duration and distance (duration: F = 3.16, df = (2, 44), p = 0.052; distance: F = 3.12, df = (2, 44), p = 0.054).
Table 4 Migration statistics by direction (North or South) and group (early or late) for 71 Great Shearwaters.
Migration tracks for selected individual birds from early and late migrant groups are shown in Figure 4 and illustrate the dynamic changes and subsequent responses between ground track bearing, true heading, and wind direction and wind effect by modeled location during the flight. The distribution of locations during migration for early and late phases are separately shown with their 25% and 50% kernel density utilization distributions with the five defined geographic regions (area 1S – 5S) of the Atlantic Ocean (Figures 2A, C). The distributions and means for southbound migrants’ ground track bearing, true heading, and associated wind direction differed amongst geographic regions and migrant groups (all Watson-Wheeler test; W > 201.66, p< 0.001; Figure 5A; Supplementary Tables 1, 2). Despite highly variable winds, the overall path of the southern migration was well defined in area 1S as both early and late migrants maintained easterly trajectories from waters near Atlantic Canada north of 40° N. In area 2S, the path became less defined for early migrants as they broadly changed direction to the south after passing east of 40° W (Figure 2A), while late migrants continued to move in a more defined path towards the coast of N Africa (Figure 2C). Both groups generally used SE true headings to achieve an effective southerly trajectory under a mostly favorable SW blowing wind.
Figure 4 Examples of flight paths for three individual Great Shearwaters as representatives of each migrant group analyzed (southbound – early, southbound – late, northbound). Trajectories are colored according to wind effect and illustrate dynamic changes and subsequent responses to wind by individual during migration with the magnitude (length of arrow) and direction of each vector at each state-space modeled location. Four-pointed stars denote tagging areas in the Gulf of Maine within the N Atltantic and hexagonal stars denote breeding colonies on Inaccessible and Gough Islands in Tristan da Cunha in the S Atlantic.
Figure 5 Rose diagrams showing the density distribution in 15° bins of the different direction vectors in relation to one another for (A) southbound migrants (early and late phases) and (B) Northbound Migrants by geographic regions (Figure 2). Wind bearing is depicted blowing towards as this is how it relates to the other vectors for the calculation of flight metrics. Area shaded on the vertical axis reflects greater probability of occurrence in that bin.
In area 3S while approaching the Equator and the doldrums region, all flight paths were southerly in direction across a wide range of longitude east of 40° W. Early migrants had both a southerly ground track bearing and true heading, and faced northerly blowing headwinds in that area; their flight paths coalesced as their speeds slowed approaching the Equator (Figure 2A). This convergence occurred south of the Equator for late migrants (Figure 2C). Late migrants used primarily a SE true heading to maintain their southerly ground track under more favorable WSW blowing tailwinds (Figure 5A). The median week for early migrants to pass through area 3S was 17 – 23 September, whereas late migrants passed through it more than two months later between 1 – 7 December. In area 3S almost half of the early migrants experienced a northerly blowing headwind (circular mean ± s.d. 347° ± 1.4°; Supplementary Table 1), which resulted in negative relative wind support in that region (Figure 2B). Conversely, most late migrants had positive relative wind support in that same area (Figure 2D) due to the presence of SW blowing tailwinds (Figure 5A). The overall strength of the wind vector in this area was light (5 – 6 m·s-1) for both groups.After passing the Equator off the coast of Brazil in area 4S, flight paths for both migrant groups became spatially unified on the outer continental shelf where both groups experienced a similar W - NW blowing wind and used SSE true heading (Figure 5A) to achieve an overall displacement to the SSW along the coast of Brazil (Figure 2). South of 20° S in area 5S, there was a divergence in migration trajectories for early migrants: most went to the Patagonian Shelf, while others moved east continuing across the S Atlantic before their direct transit flight stopped and foraging started (Figure 2A). All late migrants went only to the Patagonian Shelf (Figure 2C). SW blowing winds dominated in this area, and ground track bearings and true headings for all late migrants, and those early migrants going to the Patagonian Shelf aligned with wind direction (Figure 5A). The remaining early migrants that continued toward the nesting islands in the Tristan da Cunha archipelago used a wider range of true headings seeking favorable wind support to take them there (Figures 2A, B).
The overall path of the northern migration was well defined from the start around 40° S north to 20° N as shown in an example individual flight track for a northbound migrant (Figure 4). All birds moved along the edge of the continental shelf until reaching the Equator (Figure 3). The distribution of angles and their means differed amongst areas for ground track bearing, true heading, and wind direction (all Watson-Wheeler test; W > 478.92, p< 0.001; Figure 5B, Supplementary Tables 1, 2). At the start of migration in area 1N, birds leveraged a steady tailwind blowing N keeping a NE true heading to achieve NNE ground track bearing. By area 2N winds shifted direction to the NW but birds maintained an E to NE true heading to achieve N ground track to the Equator. At the Equator, their flight paths were consolidated longitudinally and most dense at the northeast corner of Brazil as they started to move into the N Atlantic in area 3N. There, winds were light approaching the Equator but began to increase in magnitude, while wind direction progressively changed to blowing WSW as they passed the Equator (Figures 4, 5B). Birds that completed the migration responded by heading NE to maintain a displacement to the NW towards the Caribbean Sea (Figure 3). North of 20° N wind direction became variable but birds modified their true heading to maintain a northerly displacement (Figures 3B, 4). Two birds stopped migrating on the Scotian Shelf (62.9° W, 42.2° N and 57.3° W, 46.4° N), one on the Grand Banks off Newfoundland (45.7° W, 45.0° N), and the other at the North Atlantic Current and Evlanov Seamount (NACES) MPA in the mid North Atlantic to the northwest of the Azores (47.7° W, 35.0° N).
For southbound migrants, group (early vs late) explained variation in ground speed (Vg), airspeed (Va), wind support (Vs), and wind effect smoothed by latitude (LRT, p< 0.001). Overall, late migrants traveled more slowly in the N Atlantic before passing the Equator (Figure 6A). In that region, latitude negatively affected airspeed (decreased Va) for early migrants and positively affected airspeed (increased Va) for late migrants (Figure 6A). Although Vgvariation was more pronounced in early migrants, both groups initially increased Vgat higher latitudes before slowing down on their approach to the Equator (Figure 6A). South of the Equator the effect of latitude on Vgbecame negative and no longer explained ground speed especially for early migrants. The negative effect of latitude was noted only past the Equator for late migrants (Figure 7A). Accordingly, for both groups, passing the Equator and the doldrums region was associated with increased flight effort (greater Va), and decreased wind support (Vs). Again, both effects were slightly more pronounced for early migrants (Figure 7A). Wind support varied at greater amplitude for early migrants overall compared to late migrants, and late migrants achieved greater Vsthan early migrants throughout their migration (Figure 6A). In the S Atlantic, the effect of latitude on Varelaxed especially for late migrants (Figure 7A), and both groups found more wind support at those southern latitudes (Figure 6A). It was not until reaching the S Atlantic that both migrant groups experienced a positive wind effect (i.e., Vgexceeding Va (Figure 6A). This shift in wind effect contrasted with the beginning of their southbound migration (> 25° N), where the wind had a highly negative effect on flight (Figure 7A) (i.e., birds’ airspeed was higher than their achieved ground speed) (Figure 6A). The wind effect relaxed when they started approaching 25° N and found a desirable southbound course near the African continent, particularly for early migrants (Figure 7A).
Figure 6 Flight metrics across latitude during (A) southbound migration for ‘early‘ and ‘late’ migrants, and (B) during northbound migration for Great Shearwaters tracked with platform terminal transmitter devices. Wind speed (Vw) is presented for reference.
Figure 7 Smoothed functions obtained from the generalized-additive mixed models (GAMM) fit for each metric according to latitude during (A) southbound migration for early and late migrants, and (B) during northbound migration. 0 on the y-axis corresponds to the scaled mean, such as the closer the smooth is to zero the more it indicates that latitude is not explaining variation in the metrics’ response; on either side of 0 latitude either explained a positive or negative response. Vg - Ground speed, Va - Airspeed, Vs - wind support, We - wind effect.
The effect of latitude on Vgwas positive as Great Shearwaters began migrating north from the Patagonian Shelf (Figure 7B). However, like the southbound migration, Vs and Vgstarted to decrease as birds approached the Equator (Figure 6B). At the Equator itself, the effect of latitude on Vs was null, and not until ca 40° N did that effect become positive (Figure 7B). Farther north, Great Shearwaters augmented ground speed with more airspeed while experiencing a constant negative effect of wind. They traveled slower (Vg) than energy deployed (Va) even though they managed to find mostly tailwinds (Vs) throughout the northbound migration (Figure 6B). Despite finding tailwinds to improve flight efficiency, the magnitude of that wind support remained small (< 5 m·s-1), and much energy was still needed to achieve a desired course and speed.
This study highlights the range of wind conditions encountered by Great Shearwaters during their southbound and northbound migrations and provides insight as to how individuals manage their flights in response to changing winds during migration. The expected correlation between flight metrics supported the relationships with latitude that we found for Vs and Vg, and Va and We. Wind support was significantly positive during both southbound and northbound migrations except in equatorial areas. Wind effect was typically negative during migration and airspeed was dynamically adjusted in response as compensation.
The southbound migration of Great Shearwaters occurred in two phases (early and late), reflecting the maturity of the birds. Early migrants (older sub-adults and adults) started migration more than two months earlier, traveled further on average and at higher speeds, and took less time to complete migration than late migrants (younger birds). Such dissimilarity in departure date from the non-breeding area also occurs in Cory’s Shearwaters, with adult breeders departing more than two months before immature birds (Campioni et al., 2019). The slower pace traveled by late migrants suggests that younger birds are still improving their flight skills. Nonetheless, the southbound path undertaken by early and late migrants was similar except for a divergence south of 20° S where some early migrants went east from Brazil toward the nesting colonies in the Tristan da Cunha archipelago, but with the other early migrants ultimately visiting the nesting islands later. Whereas all late migrants tracked directly south to the Patagonian Shelf and did not visit the breeding colonies.Late migrants took a more consistent trajectory and true heading while crossing the N Atlantic towards N Africa. There, Great Shearwaters found a suitable tailwind to take them south. However, the area where they veered south was considerably more variable for early migrants (Figure 2), perhaps due to higher variability in wind experienced compared to late migrants, which had more consistent SE blowing tailwinds while crossing the Atlantic to the east. Similarly, during the southbound equatorial crossing in September, early migrants slowed down as they encountered headwinds during the West African monsoon in the Atlantic Intertropical Convergence Zone (ITCZ). These seasonal winds emanating from the west occur over a wide range of longitudes and north blowing winds are characteristic of this convergence zone (Grodsky et al., 2003) and have been shown as barriers to other migratory seabirds (Felicisimo et al., 2008). Even though late migrants also faced diminishing winds in the equatorial Atlantic, they maintained a consistent S ground track across the Equator using a SW true heading because westerlies from the W African monsoon season had mostly dissipated by November, two months later than the passage of early migrants. Late migrants might benefit from winds that are more favorable during their migration by initiating migration later thus gaining knowledge about migration route at potentially lower cost. Campioni et al. (2019) tracked multiple age classes of Cory’s Shearwaters and observed that departure dates from non-breeding areas, and migration distance traveled decreased as birds approached breeding age likely due to improved navigational abilities. Similarly, immature Great Shearwaters require time to learn and improve navigation and spatial recognition of the migration route. The dichotomy we observed in migration phenologies also suggests that by migrating earlier, immatures might travel the path of least resistance, experiencing flight conditions that are less risky in terms of survival compared to early migrants.In the absence of favorable tailwinds, crossing the equatorial region during both southbound and northbound migrations appeared to be a decisive challenge for shearwaters leading them to increase energy expenditures. Reliance on compensatory flight to move across the equatorial region was stressed by the positive effect of those latitudes on airspeed (Va), which resulted in the strongest negative wind effect. However, during the southbound migration, the magnitude of those effects was lower for late migrants (younger birds). Moreover, migrating birds showed a widespread lack of wind support (Supplementary Figure 1) as they mostly experienced headwinds or a lack of any wind near the Equator. Again, this effect was not as pronounced for late migrants (younger birds), which experienced positive wind support on average throughout their southbound migration (Figures 2, 3).
The difference in phenology between the migrant groups may have evolved due to the necessity of breeding birds to be at the nesting islands by mid-September; their arrival is highly synchronized and occurs in just a few days (Cuthbert, 2005). Similarly, as part of the maturation process, pre-breeders must learn to reach the colony in time for courtship as mating happens from late September to mid-October before birds leave the colonies during a pre-egg laying exodus (Cuthbert, 2005). Thus, a shortened migration time and an earlier departure date from non-breeding areas might serve to improve future fitness of individuals in search of a new mate and burrow in preparation for their first breeding attempt. The southbound migration took 27 – 32 days. Accordingly, pre-breeders and breeding birds must leave the wintering areas by mid-August to be at the breeding colonies in time. Cory’s Shearwaters have been observed delaying their southern migration from the western N Atlantic across the Equator until the monsoon breaks down (Felicisimo et al., 2008). However, given Great Shearwater breeding phenology, starting migration earlier would not prevent their negative interaction with West African monsoons, which start in July (Grodsky et al., 2003). Thus, early migrants (pre-breeders and breeders) must face the prevailing headwinds blowing at that time of year to get across the doldrums and the Equator during a required time window. Such spatial and temporal constraints are often typical of seasonal movements (e.g., migrations: Dell’Ariccia et al., 2018), and during central-place foraging for breeding seabirds (Hedd and Gales, 2005; Magalhães et al., 2008; Boyd et al., 2014). The cost of getting across the doldrums and equatorial regions as efficiently as possible must be warranted by increased fitness through a successful mating or breeding attempt. Immature Great Shearwaters are not yet on an annual schedule to breed, and thus might choose an energetically easier path by leaving later. Younger birds may also be slower in acquiring reserves to migrate (Delord et al., 2021), and/or have no selective advantage of migrating earlier to compete with adults at foraging areas in the S Atlantic. Differential foraging strategies and segregation in trophic niches exist between breeders and immatures in procellariids (Riotte-Lambert and Weimerskirch, 2013; Campioni et al., 2016). These differences support competition avoidance mechanisms through either progressive developmental learning of the constraints of central place foraging (Riotte-Lambert and Weimerskirch, 2013), or developmental shift in prey selection (Campioni et al., 2016).
Great Shearwaters started their northbound migration off Argentina under optimal conditions until approaching the Equator off NE Brazil. At this point birds were exposed to SW blowing winds of greater velocity than either their ground or air speeds (Figure 6B), but they adjusted to a NE true bearing under stress and maintained a NW ground track (Figure 4). Manx Shearwaters use this area as a stop-over to either refuel (forage) or delay migration due to adverse weather (Guilford et al., 2009). Adult Sooty Shearwaters breeding in the Falkland Islands also migrate north off NE Brazil en route to non-breeding areas in the N Atlantic (Hedd et al., 2012). Going northbound from the Equator, wind support remained low, and much energy was still needed to keep the desired course as birds experienced a mixture of wind directions.
The implications of climate change for seabirds are a broad topic that has been discussed in general terms (Grémillet and Boulinier, 2009; Chambers et al., 2011; Quillfeldt and Masello, 2013; Orgeret et al., 2021), but this study provides insights to future research on mechanisms of seabird migrations that may be affected by changes in wind patterns due to global warming. As global warming progresses (Stocker et al., 2013), headwinds and tailwinds encountered by migrant terrestrial birds breeding in North America and traveling to the Caribbean or South America are projected to remain relatively unchanged, as the need for these species to counter or drift under the influence of strong crosswinds may be diminished (La Sorte and Fink, 2017). However, the strength of the prevailing westerly winds encountered early in their migration is projected to weaken substantially (La Sorte and Fink, 2017). Great Shearwaters (early and late migrants) leverage westerly winds during the early part of the southbound migration to cross the Atlantic before heading south, but early migrants are slowed by headwinds resulting from the West African monsoons to cross the Equator. Diminished westerly winds from global warming would likely reduce ground speed during their southbound migration, but a reduction in headwinds encountered during the Equatorial crossing would be beneficial by reducing energy demands for increased airspeed.
Summarily, even when facing poor or less favorable conditions as noted by overall low wind effect along all migration paths, Great Shearwaters managed to find positive wind support, especially for late migrants traveling south, and during northbound migration. Given the common migration trajectory undertaken by the population tracked, the capacity of individuals to achieve the general desired course even when the effect of wind on the energy deployed to achieve a given speed is negative, indicates that Great Shearwaters do not solely rely on a drifting strategy while migrating. Great Shearwaters are constantly compensating to achieve a favorable course, using wind support when available. In the absence of wind support, they augment their voyage performance by working harder by increasing airspeed (i.e., compensating to achieve the desirable trajectory). Although migration routes may have evolved to maximize the benefit from average wind conditions, younger birds may be less adept at using the wind than breeding birds. These early years require improvement in their ability to withstand a variety of wind conditions, and likely in part explain the difference observed in migration strategies between the two groups. While constrained by the imminence of the breeding season, early migrants’ flight throughout migration was subject to conditions that were more variable, highlighting the importance for Great Shearwaters to gain the ability required to face a range of conditions and ultimately maximize fitness.
The datasets analyzed for this study will be made available on request to the corresponding author. Some tracking datasets in original format are available by request through the Seabird Tracking Database (http://seabirdtracking.org/ dataset IDs 662, 663, and 665).
The animal study was reviewed and approved by all applicable international, national, and institutional guidelines for the care and use of animals were followed. Permits to capture, handle, and tag birds were obtained from the US Department of the Interior (permit #21963). Permits to handle birds were obtained from the Massachusetts Division of Fisheries and Wildlife (permit #202-17SCB). Permits to capture and tag birds in Canada were obtained through the Canadian Wildlife Service (SC2429, SC2483, SC2543, SC2599, SC2655) and the Bird Banding Office (10,480-S). Animal Ethics approvals were obtained through the University of North Carolina Wilmington (protocols #2005-003 and #2007-007) and Dalhousie University (#09-041).
KP, IP, RR and DW designed the study. All authors collected the data. KP and IP analyzed the data. KP wrote the initial manuscript draft. All authors edited and approved the final version.
Funding was provided by the Volgenau Foundation, Bureau of Ocean Energy Management, Stellwagen Bank National Marine Sanctuary, National Marine Sanctuary Foundation, Mudge Foundation, Boston University Marine Program, and Pew Charitable Trusts. Funding for the Grand Manan and Tristan da Cunha portions of the study was provided by Environmental Damages Fund (Environment Canada), New Brunswick Wildlife Trust Fund, National Geographic Society, Ocean Fund, Canadian Wildlife Service, James L. Baillie Memorial Fund (Bird Studies Canada), US Fish and Wildlife Service, BirdLife International, North Star Science & Technology, and the Grand Manan Whale and Seabird Research Station. RAR was supported by a scholarship from the Natural Sciences and Engineering Research Council of Canada (NSERC) and the Killam Trusts, Dalhousie University.
The authors declare that the research was conducted in the absence of any commercial or financial relationships that could be construed as a potential conflict of interest.
All claims expressed in this article are solely those of the authors and do not necessarily represent those of their affiliated organizations, or those of the publisher, the editors and the reviewers. Any product that may be evaluated in this article, or claim that may be made by its manufacturer, is not guaranteed or endorsed by the publisher.
We thank L. Kaufman and students from the Boston University Marine Program and crew of NOAA R/V Auk for assistance in collecting shearwater data off Massachusetts. All applicable international, national, and institutional guidelines for the care and use of animals were followed. Genetic analyses of blood and feather samples were done by Animal Genetics, Inc., 1336 Timberlane Rd., Tallahassee, FL 32312-1766, USA. Permits to capture, handle, and tag birds were obtained from the US Department of the Interior (permit #21963). Permits to handle birds were obtained from the Massachusetts Division of Fisheries and Wildlife (permit #202-17SCB).
We thank the Administrator and Island Council of Tristan for permission to visit and work on Inaccessible Island. Logistical support in the South Atlantic was supplied by the South African National Antarctic Programme (SANAP), and Tristan’s Conservation Department; we especially thank N. Glass, and Captain C. October and the crew of the Edinburgh. Logistical support in the Bay of Fundy was supplied by the Grand Manan Whale and Seabird Research Station (GMWSRS), and we especially thank H. Koopman, L. Murison, Z. Swaim, H. Lane, C. McKinstry, A. Neimanis and the many field assistants that worked at GMWSRS. Permits to capture and tag birds were obtained through the Canadian Wildlife Service (SC2429, SC2483, SC2543, SC2599, SC2655) and the Bird Banding Office (10480-S). Animal Ethics approvals were obtained through the University of North Carolina Wilmington (protocols #2005-003 and #2007-007) and Dalhousie University (#09-041).
The Supplementary Material for this article can be found online at: https://www.frontiersin.org/articles/10.3389/fmars.2022.938033/full#supplementary-material
Adams J., Flora S. (2010). Correlating Seabird Movements With Ocean Winds: Linking Satellite Telemetry With Ocean Scatterometry. Mar. Biol. 157, 915–929. doi: 10.1007/s00227-009-1367-y
Alerstam T. (2011). Optimal Bird Migration Revisited. J. Ornithol 152, 5–23. doi: 10.1007/s10336-011-0694-1
Boyd C., Punt A. E., Weimerskirch H., Bertand S. (2014). Movement Models Provide Insights Into Variation in the Foraging Effort of Central Place Foragers. Ecol. Model. 286, 13–25. doi: 10.1016/j.ecolmodel.2014.03.015
Bradley J. S., Gunn B. M., Skira I. J., Meathrel C. E., Wooler R. D. (1999). Age-Dependent Prospecting and Recruitment to a Breeding Colony of Short-Tailed Shearwaters Puffinus Tenuirostris. Ibis 141, 277–285. doi: 10.1111/j.1474-919X.1999.tb07550.x
Brown R. G. B. (1986). Revised Atlas of Eastern Canadian Seabirds (Dartmouth NS: Canadian Wildlife Service, Bedford Institute of Oceanography), 111 pp.
Calenge C. (2006). The Package “Adehabitat” for R Software: A Tool for the Analysis of Space and Habitat Use by Animal. Ecol. Model. 197, 516–519. doi: 10.1016/j.ecolmodel.2006.03.017
Campioni L., Dias M. P., Granadeiro J. P., Catry P. (2019). An Ontogenetic Perspective on Migratory Strategy of a Long-Lived Pelagic Seabird: Timings and Destinations Change Progressively During Maturation. J. Anim. Ecol. 89, 29–43. doi: 10.1111/1365-2656.13044
Campioni L., Granadeiro J. P., Catry P. (2016). Niche Segregation Between Immature and Adult Seabirds: Does Progressive Maturation Play a Role? Behav. Ecol. 27, 426–433. doi: 10.1093/beheco/arv167
Chambers L. E., Devney C. A., Congdon B. C., Dunlop N., Woehler E. J., Dann P. (2011). Observed and Predicted Effects of Climate Change on Australian Seabirds. Emu 111, 235–251. doi: 10.1071/MU10033
Cramp S., Simmons K. E. L. (Eds.) (1977). Handbook of the Birds of Europe, the Middle East, and North Africa. Vol. I: Ostrich to Ducks (Oxford (UK): Oxford University Press), 722 pp.
Crespin L., Harris M. P., Lebreton J. D., Fredericksen M., Wanless S. (2006). Recruitment to a Seabird Population Depends on Environmental Factors and on Population Size. J. Anim. Ecol. 75, 228–238. doi: 10.1111/j.1365-2656.2006.01035.x
Cuthbert R. J. (2005). Breeding Biology, Chick Growth and Provisioning of Great Shearwaters (Puffinus Gravis) at Gough Island, South Atlantic Ocean. Emu 105, 305–310. doi: 10.1071/MU05036
de Grissac S., Borger L., Guitteaud A., Weimerskirch H. (2016). Contrasting Movement Strategies Among Juvenile Albatrosses and Petrels. Sci. Rep. 6, 26103. doi: 10.1038/srep26103
Dell’Ariccia G., Benhamou S., Dias M. P., Granadeiro J. P., Sudre J., Catry P., et al. (2018). Flexible Migratory Choices of Cory’s Shearwaters are Not Driven by Shifts in Prevailing Air Currents. Sci. Rep. 8, 3376. doi: 10.1038/s41598-018-21608-2
Delord K., Weimerskirch H., Barbraud C. (2021). The Challenges of Independence: Ontogeny of at-Sea Behaviour in a Long-Lived Seabird. bioRxiv2021.1023.465439. doi: 10.1101/2021.10.23.465439
Enri B., Liechti F., Bruderer B. (2005). The Role of Wind in Passerine Autumn Migration Between Europe and Africa. Behav. Ecol. 16, 732–740. doi: 10.093/beheco/ari046
Felicisimo Á.M., Munoz J., González-Solis J. (2008). Ocean Surface Winds Drive Dynamics of Transoceanic Aerial Movements. PLos One 3 (8), e2928. doi: 10.1371/journal.pone.0002928
Fletcher D., Moller H., Clucas R., Bragg C., Scott D., Scofield P., et al. (2013). Age at First Return to the Breeding Colony and Juvenile Survival of Sooty Shearwaters. Condor 115 (3), 465–476. doi: 10.1525/cond.2013.110151
Gill R. E. Jr, Douglas D. C., Handel C. M., Tibbitts T. L., Hufford G., Piersma T. (2014). Hemispheric-Scale Wind Selection Facilitates Bar-Tailed Godwit Circum-Migration of the Pacific. J. Anim. Behav. 90, 117–130. doi: 10.1016/j.anbehav.2014.01.020
González-Solis J., Felicisimo Á., Fox J. W., Afanasyev V., Kolbeinsson Y., Munoz J. (2009). Influence of Sea Surface Winds on Shearwater Migration Detours. Mar. Ecol. Prog. Ser. 391, 221–230. doi: 10.3354/meps08128
Grémillet D., Boulinier T. (2009). Spatial Ecology and Conservation of Seabirds Facing Global Climate Change: A Review. Mar. Ecol. Prog. Ser. 391, 121–137. doi: 10.3354/meps08212
Grodsky S. M., Carton J. A., Nigam S. (2003). Near Surface Westerly Wind Jet in the Atlantic ICTZ. Geophys Res. Lett. 30 (19). doi: 10.1029/2003GL017867
Guilford T., Meade J., Willis J., Phillips R. A., Boyle D., Roberts S., et al. (2009). Migration and Stopover in a Small Pelagic Seabird, the Manx Shearwater Puffinus Puffinus: Insights From Machine Learning. Proc. R Soc. B 276, 1215–1223. doi: 10.1098/rsbp.2008.1577
Hedd A., Gales R. (2005). Breeding and Overwintering Ecology of Shy Albatrosses in Southern Australia: Year-Round Patterns of Colony Attendance and Foraging-Trip Durations. Condor 107, 375–387. doi: 10.1093/condor/107.2.375
Hedd A., Montevecchi W. A., Otley H., Phillips R. A., Fifield D. A. (2012). Trans-Equatorial Migration and Habitat Use by Sooty Shearwaters Puffinus Griseus From the South Atlantic During the Nonbreeding Season. Mar. Ecol. Prog. Ser. 449, 277–290. doi: 10.3354/meps09538
Illan J. G., Wang G., Cunningham F. L., King D. T. (2017). Seasonal Effects of Wind Conditions on Migration Patterns of Soaring American White Pelican. PLos One 12 (10), e0186948. doi: 10.1371/journal.pone.0186948
Jodice P. G. R., Ronconi R. A., Rupp E., Wallace G. E., Satgé Y. (2016). First Satellite Tracks of the Endangered Black-Capped Petrel. Endanger Species Res. 29, 23–33. doi: 10.3354/esr00697
Jonsen I. D., Basson M., Bestley S., Bravington M. V., Patterson T. A., Pedersen M. W., et al. (2013). State-Space Models for Bio-Loggers: A Methodological Road Map. Deep Sea Res. Part II 88, 34–46. doi: 10.1016/j.dsr2.2012.07.008
Jonsen I. D., Flemming J. M., Myers R. A. (2005). Robust State-Space Modeling of Animal Movement Data. Ecology 86, 2874–2880. doi: 10.1890/04-1852
Kemp M., van Loon E., Shamoun-Baranes J., Bouten W. (2012). RNCEP: Global Weather and Climate Data at Your Fingertips. Methods Ecol. Evol. 3, 65–70. doi: 10.1111/j.2041-210X.2011.00138.x
Kogure Y., Sato K., Watanuki Y., Waneless S., Daunt F. (2016). European Shags Optimize Their Flight Behavior According to Wind Conditions. J. Exp. Biol. 219, 311–318. doi: 10.1242/jeb.131441
Krietsch J., Valcu M., Kempenaers B. (2020). Wind Conditions Influence Breeding Season Movements in a Nomadic Polygynous Shorebird. Proc. R Soc. B 287, 20192789. doi: 10.1098/rspb.2019.2789
La Sorte F. A.,, Fink D. (2017). Projected Changes in Prevailing Winds for Transatlantic Migratory Birds Under Global Warming. J. Anim. Ecol. 86, 273–284. doi: 10.1111/1365-2656.12624
Liechti F., Bruderer B. (1998). The Relevance of Wind for Optimal Migration Theory. J. Avian Biol. 29, 561–568. doi: 10.2307/3677176
Magalhães M. C., Santos R. S., Hamer K. C. (2008). Dual-Foraging of Cory’s Shearwaters in the Azores: Feeding Locations, Behaviour at Sea and Implications for Food Provisioning of Chicks. Mar. Ecol. Prog. Ser. 359, 283–293. doi: 10.3354/m3pw07340
Orben R. A., O’Connor A. J., Suryan R. M., Ozaki K., Sato F., Deguchi T. (2018). Ontogenetic Changes in at-Sea Distributions of Immature Short-Tailed Albatrosses Phaoebastria Albatrus. Endang Species Res. 35, 23–27. doi: 10.3354/esr00864
Orgeret F., Thiebault A., Kovacs K. M., Lydersen C., Hindell M. A., Thompson S. A., et al. (2021). Climate Change Impacts on Seabirds and Marine Mammals: The Importance of Study Duration, Thermal Tolerance and Generation Time. Ecol. Lett. 5), 218–239. doi: 10.111/ele.13920
Pennycuick C. J. (1982). The Flight of Petrels and Albatrosses (Procellariiformes), Observed in South Georgia and its Vicinity. Philos. Trans. R Soc. B Biol. Sci. 300 (1098), 75–106. doi: 10.1098/rstb.1982.0158
Péron C., Grémillet D. (2013). Tracking Through Life Stages: Adult, Immature and Juvenile Autumn Migration in a Long-Lived Seabird. PLos One 8 (8), e72713. doi: 10.1371/journal.pone.0072713
Porter J. M., Colson J. C. (1987). Long-Term Changes in Recruitment to the Breeding Group, and the Quality of Recruits at a Kittiwake Rissa Tridactyla Colony. J. Anim. Ecol. 56, 675–689. doi: 10.2307/5076
Powers K. D., Wiley D. N., Allyn A. J., Welch L., Ronconi R. A. (2017). Movements and Foraging Habitats of Great Shearwaters Puffinus Gravis in the Gulf of Maine. Mar. Ecol. Prog. Ser. 574, 211–226. doi: 10.3354/meps12168
Powers K. D., Wiley D. N., Robuck A. R., Olson Z. H., Welch L. J., Thompson M. A., et al. (2020). Spatiotemporal Characterization of non-Breeding Great Shearwaters Ardenna Gravis Within Their Wintering Range. Mar. Ornithol 48, 215–229.
Quillfeldt P., Masello J. F. (2013). Impacts of Climate Change and Potential Effects of Climate Change on South American Seabirds – a Review. Mar. Biol. Res. 9 (4), 337–357. doi: 10.1080/17451000.2012.756982
R Development Core Team (2020). R: A Language and Environment for Statistical Computing (Vienna, Austria: The R Foundation for Statistical Computing).
Reid T. A., Ronconi R. A., Cuthbert R. J., Ryan P. G. (2014). The Summer Foraging Ranges of Adult Spectacled Petrels Procellaria Conspicillata. Antarct Sci. 26, 23–32. doi: 10.1017/S0954102013000266
Riotte-Lambert L., Weimerskirch H. (2013). Do Naïve Juvenile Seabirds Forage Differently From Adults? Proc. R Soc. B 80, 20131434. doi: 10.1098/rspb.2013.1434
Ronconi R. A., Ryan P. G., Ropert-Coudert Y. (2010). Diving of Great Shearwaters (Puffinus Gravis) in Cold and Warm Regions of the South Atlantic Ocean. PLos One 5 (11), e15508. doi: 10.1371/journal.pone.0015508
Ronconi R. A., Schoombie S., Westgate A. J., Wong S. N. P., Koopman H. N., Ryan P. G. (2018). Effects of Age, Sex, Colony, and Breeding Phase on Marine Space Use by Great Shearwaters Ardenna Gravis in the South Atlantic. Mar. Biol. 165, 58. doi: 10.1007/s00227-018-3299-x
Safi K., Kranstauber B., Weinzierl R., Griffin L., Rees E.C., Cabot D., et al. (2013). Flying With the Wind: Scale of Dependency of Speed and Direction Measurements in Modelling Wind Support in Avian Flight. Mov Ecol. 1, 1–13. doi: 10.1186/2051-3933-1-4
Schoombie S., Dilley B. J., Davies D., Ryan P. G. (2018). The Foraging Range of Great Shearwaters (Ardenna Gravis) Breeding on Gough Island. Polar. Biol.41, 2451–2458. doi: 10.1007/s00300-018-2381-7
Sergio F., Tauferna A., De Stephanis R., Jimenez L. L., Blas J., Tavecchia G., et al. (2014). Individual Improvements and Selective Mortality Shape Lifelong Migratory Performance. Nature 515, 410–413. doi: 10.1038/nature13696
Schaffer S. A., Tremblay Y., Weimerskirch H., Scott D., Thompson D. R., Sagar P. M., et al. (2006). Migratory Shearwaters Integrate Oceanic Resources Across the Pacific Ocean in an Endless Summer. P Natl. Acad. Sci-Biol 03 (34), 12799–12802. doi: 10.1073/pnas.0603715103
Shamoun-Baranes J., van Loon E., Liechti F., Bouten W. (2007). Analyzing the Effect of Wind on Flight: Pitfalls and Solutions. J. Exp. Biol. 210, 82–90. doi: 10.1242/jeb.02612
Silva M. A., Prieto R., Jonsen I., Baumgartner M. F., Santos R. S. (2013). North Atlantic Blue and Fin Whales Suspend Their Spring Migration to Forage in Middle Latitudes: Building Up Energy Reserves for the Journey? PLos One 8 (10), e76507. doi: 10.1371/journal.pone.007650
Stocker T.F., Qin D., Plattner G.-K, Tignor M.M.B., Allen S.K., Boschung J., et al. (2013). “Climate Change 2013: The Physical Science Basis,” in Contribution of Working Group I to the Fifth Assessment Report of the Intergovernmental Panel on Climate Change. Eds. Stocker T. F., Qin D., Plattner G.-K., et al (Cambridge, UK/New York, NY, USA: Cambridge University Press), 1535.
Tavares D. C., Moura J. F., Merico A., Siciliano S. (2019). Mortality of Seabirds Migrating Across the Tropical Atlantic in Relation to Oceanographic Processes. Anim. Conserv. 23, 307–319. doi: 10.1111/acv.12539
Thiers L., Delord K., Barbraud C., Phillips R. A., Pinaud D., Weimerskirch H. (2014). Foraging Zones of the Two Sibling Species of Giant Petrels in the Indian Ocean Throughout the Annual Life Cycle: Implication for Their Conservation. Mar. Ecol. Prog. Ser. 499, 233–248. doi: 10.3354/meps10620
Vardanis Y., Klaassen R. H. G., Strandberg R., Alerstam T. (2011). Individuality in Bird Migration: Routes and Timing. Biol. Lett. 7, 502–505. doi: 10.1098/rsbl.2010.1180
Ventura F., Granadeiro J. P., Padget O., Catry P. (2020). Gadfly Petrels Use Knowledge of the Windscape, Not Memorized Foraging Patches, to Optimize Foraging Trips on Ocean-Wide Scales. Proc. R Soc. B 287, 20191775. doi: 10.1098/rspb.2019.1775
Warham J. (1996). The Behaviour, Population Biology and Physiology of the Petrels (London (UK): Harcourt Brace & Company).
Weimerskirch H., Guionnet T., Marin J., Shaffer S. A., Costa D. P. (2000). Fast and Fuel Efficient? Optimal Use of Wind by Albatrosses. Proc. R Soc. B 267, 1869–1874. doi: 10.1098/rspb.2000.1223
Keywords: great shearwater, migration, wind influence, platform terminal transmitter, RNCEP library, developmental learning, telemetry
Citation: Powers KD, Pratte I, Ronconi RA, Wong SNP, Ryan PG, Welch LJ, Silva TL, Hatch KA, Westgate AJ and Wiley DN (2022) Age-Related Interactions with Wind During Migration Support the Hypothesis of Developmental Learning in a Migrating Long-Lived Seabird. Front. Mar. Sci. 9:938033. doi: 10.3389/fmars.2022.938033
Received: 10 May 2022; Accepted: 24 June 2022;
Published: 26 July 2022.
Edited by:
Guillermo Luna-Jorquera, Universidad Católica del Norte, ChileReviewed by:
Frank A. La Sorte, Cornell University, United StatesCopyright © 2022 Powers, Pratte, Ronconi, Wong, Ryan, Welch, Silva, Hatch, Westgate and Wiley. This is an open-access article distributed under the terms of the Creative Commons Attribution License (CC BY). The use, distribution or reproduction in other forums is permitted, provided the original author(s) and the copyright owner(s) are credited and that the original publication in this journal is cited, in accordance with accepted academic practice. No use, distribution or reproduction is permitted which does not comply with these terms.
*Correspondence: Kevin D. Powers, a2Rwb3dlcnMyNEBnbWFpbC5jb20=
Disclaimer: All claims expressed in this article are solely those of the authors and do not necessarily represent those of their affiliated organizations, or those of the publisher, the editors and the reviewers. Any product that may be evaluated in this article or claim that may be made by its manufacturer is not guaranteed or endorsed by the publisher.
Research integrity at Frontiers
Learn more about the work of our research integrity team to safeguard the quality of each article we publish.