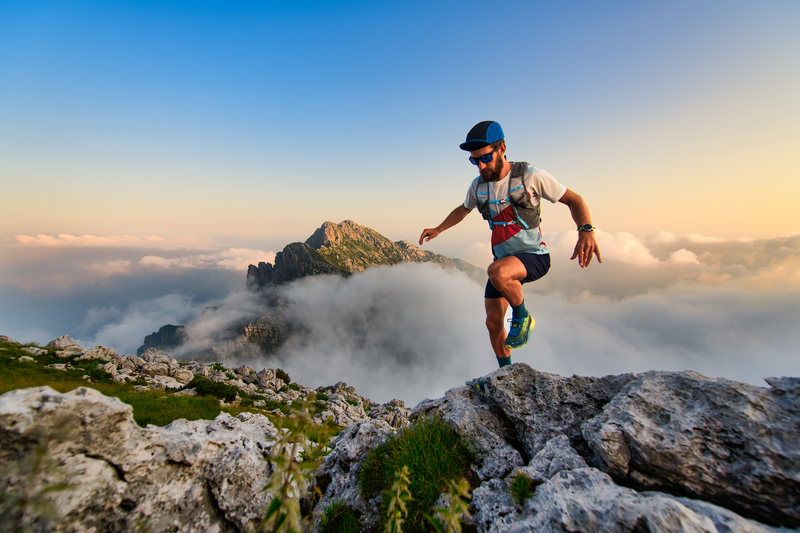
94% of researchers rate our articles as excellent or good
Learn more about the work of our research integrity team to safeguard the quality of each article we publish.
Find out more
ORIGINAL RESEARCH article
Front. Mar. Sci. , 09 August 2022
Sec. Marine Fisheries, Aquaculture and Living Resources
Volume 9 - 2022 | https://doi.org/10.3389/fmars.2022.923205
Climate change is exerting unprecedented effects on the habitats of marine mammals. Common minke whales (Balaenoptera acutorostrata) have suffered immense harm from commercial whaling, and the recovery of this species is likely threatened by climate change. To better manage and conserve this species, it is important to predict its current habitat distribution and the potential change under future climate change scenarios. Such predictions are typically generated by species distribution models (SDMs), which construct a correlation between species occurrence data and its habitat environmental variables. SDMs are commonly constructed at the species level, assuming a homogenous response of the species to climatic variables across their entire geographic range. Spatially segregated populations from the same species inhabit distinct environments and gradually adapt to the local conditions, resulting in niche differentiation among populations. Species-level SDMs that ignore the effects of local adaptation mask differences in population responses to climate change and might present an unrealistic picture of potential species distributions. Based on morphological and genetic evidence, the common minke whale was divided into three populations: the North Atlantic population (NAP), Southern Hemisphere population (SHP) and North Pacific population (NPP); these populations inhabit isolated geographic areas with distinct environmental conditions. We quantified the realized niches of these populations and found evidence of significant ecological niche differentiation. We then constructed SDMs at the species and population levels and compared the predictions from these two types of models under different climate change scenarios. Both types of models projected similar change trends in species range, with a contraction of future suitable habitats for the NAP and SHP and an expansion for the NPP. However, the magnitudes of this change differed; the population-level model projected more optimistic results for the SHP and NAP, indicating less habitat loss. This study highlighted the importance of considering local adaptation when estimating the impact of climate change on species habitat suitability. These spatiotemporal predictions provide essential knowledge for designing climate-adaptive conservation and management strategies, such as the delimitation of mobile marine protected areas (MPAs).
The growth and reproduction of marine organisms depend on special niche spaces. However, anthropogenic-induced climate change has resulted in a series of changes in the marine environment, such as increases in water temperature, ocean acidification, and hypoxia (Cheung et al., 2013; Breitburg et al., 2018; Baringer et al., 2020). Therefore, changes in the marine environment conditions may change the current distribution patterns of species and affect the function and service of marine ecosystems. Many studies have found poleward shifts in marine species to trace suitable niches in response to a warming climate (e.g., Hastings et al., 2020; Murcia et al., 2020; Abe et al., 2021). Cetaceans, top predators at a high trophic level, can directly affect the marine ecosystem by predation (Bowen, 1997). The predation of these species induced costly changes to their prey in the abundance and spatiotemporal patterns. Thus, the removal of these apex predators, such as by commercial whaling, will greatly impact community structure (Kiszka et al., 2015). Additionally, cetaceans transport nutrients from deep waters to the surface by releasing fecal plumes rich in iron and nitrogen, promoting the growth of phytoplankton (Smith et al., 2013; Weelden et al., 2021). Thus, cetaceans play an irreplaceable role in the cycling systems of the marine ecosystem; climate-induced changes in the distribution of these species will have cascading effects (Roman et al., 2014; Cartagena-Matos et al., 2021).
The common minke whale (Balaenoptera acutorostrata) is the most common baleen whale globally. And it is widely distributed throughout most tropical, temperate and polar regions. As an omnivorous species, its prey changes with area, mainly including Japanese anchovy, Pacific saury, Pacific anchovy, krill and small fishes (Tamura and Fujise, 2002; Mitani et al., 2006; Pastene et al., 2007; Song and Zhang, 2014). Because of its small body size, this species did not attract more attention in earlier commercial whaling, but it became the main hunted species when the other large whale species were severely depleted and hard to locate (IWC, 2021). Common minke whales are likely recovering from the intense whaling era after the promulgation of control measures on whaling (Tulloch et al., 2018). However, climate change, which has resulted in unprecedented habitat loss and fragmentation for whales and other marine mammals, may imperil the recovery of the common minke whale (Lambert et al., 2014; Perrin et al., 2018; Tulloch et al., 2018; Weelden et al., 2021). In 2021, China revised the Lists of ‘Wild Animals under Special State Protection’ and upgraded protection level of the common minke whale from second-class protection (wildlife identified as in small numbers) to first-class protection (wildlife identified as in rare or endangered), given the decline of this species in recent years (Liu et al., 2022). Although common minke whales face strong anthropogenic and climate stresses, few studies have investigated the alteration in their habitat in the face of changed environments (Boer, 2010; Glover et al., 2010; Lee et al., 2017; Risch et al., 2019). Hence, it is urgent to estimate the climate impacts on the continued habitat suitability of common minke whales to inform the long-term conservation and management decisions for this species.
Species distribution models (SDMs) describe the relationships between georeferenced species distribution data (e.g., presence, abundance) and simultaneously available environmental predictors and have been extensively used in estimating the habitat suitability of species (e.g., Robinson et al., 2017; Zhang et al., 2020; Fu et al., 2021; Soultan et al., 2022). Constructed SDMs can be applied to predict the future range of species when the environmental predictors can be projected by global climate models under the different assumptions of representative concentration pathway (RCP) emission scenarios (Moss et al., 2010). SDMs are commonly constructed at the species level under the assumption of “niche conservatism” (e.g., Dormann, 2007; Araújo and Peterson, 2012; Chen et al., 2021; Fu et al., 2021; Zhang et al., 2021). This assumption suggests that the individuals belonging to the same species have a similar niche space and demonstrate a consistent response to climate change across the species’ entire distributional range. The spatially segregated populations from the same species living in ecologically distinct environmental conditions over a long evolutionary history will gradually adapt to the local conditions, and this local adaptation is likely to result in niche divergence among populations. The genetic and phenotypic heterogeneity caused by local adaptation has been verified in some species by phylogeographic analysis (Razgour et al., 2019; Wang et al., 2020; Milmann et al., 2021; Peñalver-Alcázar et al., 2021; Zhang et al., 2021). Consequently, pooling data at the species level to construct global SDMs may mask the local differential responses to climate change and produce unreliable predictive results. More recently, an increasing number of studies realized the importance of considering genetic isolation or local adaptation in building SDMs and found that population-level SDMs produced more realistic range predictions for species (e.g., Lecocq et al., 2015; Hällfors et al., 2016; Smith et al., 2017; DeMarche et al., 2019; Peñalver-Alcázar et al., 2021; Zhang et al., 2021). Climate-induced changes in ocean conditions have been proven to impact the habitats of several whale species (Smith et al., 1986; Salvadeo et al., 2013; Lambert et al., 2014; Lee et al., 2017). However, no further studies were found at higher taxonomic resolutions below the species level to estimate the climate impacts on whale species.
Based on geographical origin and morphological and genetic differences, the common minke whale was divided into three populations: the North Atlantic population (B. a. acutorostrata; abbreviated NAP), the North Pacific population (B. a. scammoni; NPP), and the dwarf minke whale population (abbreviated SHP according to its geographical origin), which is an unnamed population that occurs almost exclusively in the Southern Hemisphere (Rice, 1998; Milmann et al., 2021). Although the extent of distribution overlap and genetic exchange among the populations cannot be confirmed, the three populations have inhabited distinct ecological environments over their long-term evolutionary histories, and local adaptation is likely to exist (Pastene et al., 2007; Kishida, 2017). In this study, we first quantified the realized niche (the part of the fundamental niche occupied by species in reality) and compared the niche differentiation of the three populations. We then established SDMs at the population and species levels to predict the potential distribution of common minke whales and estimated the vulnerability of this species to climate change. The aim of this study was to address the following hypotheses: (1) the three populations occupy significantly different niche spaces, which results in differential responses to climate change; (2) population-level models, accounting for local adaptation, produce more reliable predictive results than do corresponding species-level models. Our results are expected to provide essential knowledge for designing climate-adaptive conservation and management strategies at more precise taxonomic unit.
Common minke whales are found in all ocean basins, with the dwarf minke whale population limited to the Southern Hemisphere (IWC, 2021). Given the known distribution ranges of the three populations, our study areas were limited to 100°W-105°E, 0°-84°N for the NAP, 105°-260°E, 0°-84°N for the NPP and 180°W-180°E, 0°-79°S for the SHP. To better show the predictive results, we converted the coordinate range from -180°W-180°E to 0°-360°E to present the predictive maps of the NPP. Occurrence records of the common minke whale were obtained from the two public databases: Global Biodiversity Information Facility, Ocean Biogeographic Information System (Table 1), and published articles (Rosel et al., 2016; Kavanagh et al., 2018; Milmann et al., 2018; Wang, et al., 2020). The occurrence records spanned from 1990 to 2014. Sampling was not evenly distributed and its clustering may overrepresent the environmental conditions (Kramer-Schadt et al., 2013). To minimize sampling bias, we spatially thinned the occurrence data using the R package spThin and retained one occurrence point per grid of 5 × 5 arc minutes (i.e., 9.2×9.2 km), consistent with the spatial resolution of the environmental predictors (Aiello-Lammens et al., 2015). After this data-filtering process, a total of 6936 records were retained to build the SDM at the species level (hereafter “species model”), of which 5783 records belonged to the NAP used to build the SDM at the population level (hereafter “NAP model”), 597 records belonged to the NPP used to build the NPP model, and 687 records belonged to the SHP used to build the SHP model (Figure 1). The available data reflected the presence of the common minke whale, whereas most SDMs require information on species absence (Sánchez-Mercado and Ferrer-Paris, 2010; Singer et al., 2017). Due to the lack of true-absence records, we randomly simulated the same number of pseudo-absence records with that of presence records in the environment conditions where the common minke whale did not occur using the pseudoAbsences function in the R package MOPA (Iturbide, 2017). Thus, the binary presence/absence (0/1) data were used as the response variable to build the SDMs.
Table 1 Sample size, time range and source of the initially selected common minke whale occurrence data.
Figure 1 Map of study regions and occurrence records of the common minke whale. Red dots, orange dots and blue dots indicate the occurrence records of the North Atlantic population (NAP), the North Pacific population (NPP) and the Southern Hemisphere population (SHP), respectively.
Based on the literatures on species-environment associations and considering data availability, we selected nine environmental variables as potential predictors of occurrence of common minke whales (Table 2). Cetacean occurrence in subarctic waters is primarily driven by sea ice cover and prey occurrence (Brower et al., 2018). Correspondingly, ice thickness was selected as a predictive factor. Additionally, Todd and Williamson (2022) found that dissolved oxygen and chlorophyll-a concentrations significantly influenced cetacean occurrence; thus, these two factors were included. Hydrographic conditions such as mixing, upwelling, and frontogenesis normally promote high primary productivity, which leads to higher concentrations of prey, attracting more common minke whales to forage in a given area. Therefore, primary productivity and current velocity were selected as predictors (Lee et al., 2017). In the coastal waters, salinity, water temperature, water depth and distance to shore influence the abundance of cetaceans; these environmental variables generally serve as proxies for other variables, which directly influence whale occurrence (Tetley et al., 2008; Robinson et al., 2009; Víkingsson et al., 2015).
Table 2 The nine environmental variables initially selected for this study and their units, spatial resolution, temporal resolution and sources.
We also assessed the collinearity of the predictors to prevent undue influences on the results. Two or more variables with a Pearson correlation coefficient greater than 0.7 were considered to be collinear and only one of these variables was retained for the model analysis (Dormann et al., 2013; Schickele et al., 2020; Zhang et al., 2021). After this process, current velocity, salinity, water temperature, water depth, distance to shore, chlorophyll concentration and ice thickness were retained. The present and future environmental data were downloaded from online datasets with a spatial resolution of 5×5 arc minutes (i.e., 9.2×9.2 km); these datasets included the Bio-ORACLE v2.1 dataset (http://www.bio-oracle.org) (Assis et al., 2018) for current velocity, salinity, water temperature, chlorophyll concentration and ice thickness, and the Global Marine Environment Datasets (http://gmed.auckland.ac.nz) (Basher et al., 2018) for water depth and distance to shore. The average values of environmental variables during 2000-2014 were taken to represent the present environmental conditions. BIO-ORACLE provides future environmental projections using three atmospheric-ocean general circulation models (AOGCMs) under four representative concentration pathway (RCP) emission scenarios (RCP 2.6, RCP 4.5, RCP 6.0 and RCP 8.5). To reduce the uncertainties of the environmental projections, we used the average values of the projected results from the three AOGAMs to represent the future environmental conditions. RCP 2.6 indicates an optimistic emission level with good control of greenhouse gas concentrations; RCP45 and RCP60 represent the moderate emission level; and RCP 8.5 indicates a pessimistic condition with uncontrolled (i.e., business-as-usual) emissions. Increased greenhouse gas emissions would result in larger increases in water temperature and larger decreases in salinity, chlorophyll concentration and ice thickness in the future (Table 3). Here, for more comparability, we used two representative concentration path scenarios (RCP 2.6 and RCP 8.5) for future distribution prediction in the periods of the 2050s (2040–2050) and 2100s (2090–2100). Water depth and distance to shore were assumed not to change over time (Zhang et al., 2021).
Table 3 Current and future (i.e., 2050s and 2100s) environmental conditions under two representative concentration pathway (RCP) emission scenarios.
The occupied niche space of each population can be quantified by calculating a n-dimensional ecological hypervolume based on the environmental conditions where the population occurred. To reduce the dimension of niche space, we first conducted a principal component analysis (PCA) using the R package FactoMineR (Le et al., 2008) on all environmental predictor variables and retained the first four principal components, as they accounted for more than 80% of the total variance (Supplementary Figure S1). We then calculated the four-dimensional hypervolume based on the retained principal components using the R package hypervolume (Blonder et al., 2021). The volume of hypervolume (an unitless measure) can indicate the size of niche space occupied by the population. The ecological niche differentiation between populations can be assessed by overlapping the hypervolume of each population using the R package BAT (Cardoso et al., 2021). The total niche differentiation (βTotal) ranges from 0 (completely consistent niche space) to 1 (completely different niche space) and can be decomposed into two components: niche shift, corresponding to the replacement of space between the hypervolumes occupied by two populations, and niche contraction/expansion, indicating the net differences between the amount of space encompassed by each hypervolume (Carvalho and Cardoso, 2020; Mammola and Cardoso, 2020). We used the R package ecospat (Broennimann et ., 2021) to conduct pairwise niche similarity tests, and a P value > 0.05 indicated that the niches occupied by two populations were not more similar than expected by chance, implying no significant climatic niche conservatism.
Based on the presence/pseudo-absence records and present environmental data, we developed SMDs to evaluate the species-environment relationships. The SDMs were developed based on the R package biomod2 (Thuiller et al., 2021), and this package includes ten modeling algorithms: surface range envelope (SRE), generalized linear model (GLM), generalized additive model (GAM), classification tree analysis (CTA), generalized boosting model (GBM), multiple adaptive regression splines (MARS), flexible discriminant analysis (FDA), artificial neural network (ANN), random forest (RF), and maximum entropy (Maxent). The predictive performances of these models were assessed by a fivefold cross-validation method. This method split the original data at a ratio of 80%: 20% randomly, of which 80% of the data were used to train the model, and 20% of the data were used to validate the model (Guisan et al., 2017). Then, true skill statistics (TSS) and the area under the receiver operating characteristic curve (AUC) were calculated to estimate the predictive accuracy. These ten models were developed based on different statistical theories and may output differential predictive results. To integrate the respective advantages and reduce the uncertainty of a single model, we constructed weighted average ensemble models. The model with an AUC greater than 0.8 and a TSS greater than 0.7 was considered to have a high predictive accuracy and low uncertainty (Allouche et al., 2006; Guisan et al., 2017; Luo et al., 2017), and was selected to construct the ensemble models. In addition to the AUC and TSS, we calculated the continuous Boyce index (CBI) to assess the predictive performance of ensemble models, as this index has been proven to be more reliable than the traditional AUC and TSS indices, especially for presence-only data (Chardon et al., 2020; Zhang et al., 2021). The ensemble models were constructed at the species level (species model) and population level (NAP model, NPP model and SHP model).
Based on the constructed SDMs, we plotted the response curve of species with each environmental variable, presenting the variation in species occurrence probability with the environmental gradient. The importance of each predictor was estimated by a randomly permutated method (Guisan et al., 2017). This method calculated the correlation coefficient between the prediction using all the predictor variables and the prediction using only the estimated variable, which was permutated randomly. A high correlation between the two predictions indicates the low importance of the variable being estimated. The ensemble models constructed at the species and population levels were used to predict the potential distributions of the whole species and the three populations (NAP, NPP and SHP) under the current and future (2050s, 2100s) climate scenarios under RCP 2.6 and RCP 8.5, respectively. We produced continuous habitat suitability maps based on the direct outputs from the ensemble models. For a better interpretation of habitat suitability, we converted the continuous prediction into binary values by using automatically generated thresholds that maximized the TSS values of the ensemble model (Liu et al., 2013).
The volume of four-dimensional hypervolume for the NAP was 113.20, that for the NPP was 310.27 and that for the SHP was 2709.51. The paired comparison of hypervolumes indicated that the niche differentiation between populations was very large. The niche differentiation between the SHP and the NAP or NPP was mostly caused by contraction/expansion (>95%), while niche shifts contributed only marginally (<5%). The niche differentiation between the NAP and NPP was mainly due to contraction/expansion (>70%), while the niche shift contributed a small portion (<30%) (Table 4). The general niche comparison among the three populations showed that niche differentiation was mainly present along PCA1 (Figure 2A) which was mainly explained by water depth, distance to shore, and chlorophyll concentration (Supplementary Figure S2). The paired niche comparison showed that the niche differentiation between the SHP and NAP was mainly along PCA1 (Figure 2B1) which was mainly explained by water depth, distance to shore, chlorophyll concentration (Supplementary Figure S2); the niche differentiation between the SHP and NPP was mainly along PCA1 (Figure 2B2) which was mainly explained by distance to shore, water depth, ice thickness and chlorophyll concentration (Supplementary Figure S2), while the niche differentiation between the NAP and NPP was mainly presented along PCA3 (Figure 2B3) which was mainly explained by current velocity, salinity, water depth and chlorophyll concentration (Supplementary Figure S2). The pairwise niche similarity tests showed the P value for SHP vs. NPP is 0.2108, for SHP vs. NAP is 0.1169, and for NPP vs. NAP is 0.1039. All the P-values greater than 0.05 indicated no significant climatic niche conservatism.
Table 4 Total niche differentiation (βTotal) between populations and the proportion of niche shift and niche contraction/expansion.
Figure 2 The four-dimensional hypervolumes for the Southern Hemisphere population (SHP), North Atlantic population (NAP) and North Pacific population (NPP). The overlapping hypervolumes for the three populations (A), SHP and NAP (B1), SHP and NPP (B2) and NAP and NPP (B3).
Based on the TSS and AUC values, nine of the ten single models excluding the SRE were selected to construct the weighted ensemble model at the species and population levels (Figure 3). The higher values of AUC, TSS and CBI for all four ensemble models indicated high predictive performance (Table 5).
Figure 3 Predictive ability of the ten modeling algorithms estimated by the true skill statistics (TSS) and the area under the receiver operating characteristic curve (AUC) built at the population level (A) and species level (B). The black horizontal lines indicate the cutoff values of the AUC (0.8) and TSS (0.7) of the single model used to build the ensemble model.
Table 5 Mean values of the true skill statistics (TSS), the area under the receiver operating characteristic curve (AUC) and the continuous Boyce index (CBI) for the ensemble models built at the species level (species model) and populations level (SHP model, NAP model and NPP model).
The species model indicated that water depth and temperature were the two most influential variables that shaped the global distribution of the common minke whale (Figure 4A). This model indicated that the common minke whale preferred to live with water temperature between -5-20°C (Figure 5A) and water depth between 0-2000 m (Figure 5B). The population-level models indicated that the predominant factors affecting the potential distribution differed among the populations. Ice thickness and distance to shore were the two most important factors affecting the distribution of the SHP (Figure 4B). The occurrence probability of this population decreased with distance to shore and was high when ice thickness between 0-1 m (Figure 6A). The distribution of the NAP was mainly driven by water temperature and ice thickness (Figure 4B). This population preferred to live with water temperature below 20°C and ice thickness between 0-0.5 m (Figure 6B). Chlorophyll concentration and distance to shore were the two most important factors affecting the distribution of the NPP (Figure 4B). This population preferred to live in the nearshore waters where distance to shore not exceeding 800 m. Its occurrence probability increased with chlorophyll concentration and gradually stabilized when chlorophyll concentration over 0.8 mg·m-3 (Figure 6C).
Figure 4 The importance of environmental variables in driving the whole-species distribution based on the species-level model (A) and the population distributions based on the population-level model (B). Cv, current velocity; Sal, salinity; T, temperature; Depth, water depth; Dshore, distance to shore; Chl, chlorophyll concentration; It, ice thickness.
Figure 5 The response curves of the common minke whale occurrence probability against water temperature (A) and water depth (B) based on the species-level model.
Figure 6 The response curves of population occurrence probability against the two most important driving factors for the Southern Hemisphere population (A1, A2), the North Atlantic population (B1, B2), and the North Pacific population (C1, C2) based on the population-level model.
Under the current environmental conditions, the SHP model predicted the potential distribution of the SHP mainly located along the shoreline of the Antarctic continent, including the northern coast of Australia, the Gulf of Carpentaria and both sides of the Mozambique Channel. The SHP model predicted more potential habitat (35,052,556 km2) for the SHP than did the prediction from the species model (12,998,842 km2). The NAP model predicted that the potential distribution of the NAP extended to the northeast sea area from the coastal waters of Canada, while in addition to these areas, the species model predicted extra suitable habitat along the southwest coast of Greenland. The species model presented more optimistic results with more suitable habitat (19,995,777 km2) than the NAP model (10,049,561 km2). The NPP model predicted that the potential distribution of the NPP was mainly located in the coastal waters of China, Japan, America, Russia and Canada, and this model presented more suitable habitat (12,844,797 km2), particularly in the Sea of Japan, Bering Strait and Chukchi Sea, than that (5,816,799 km2) of the species model (Figure 7).
Figure 7 The predicted potential distribution under the current environmental conditions based on models built at the species level (A1, B1) and population level (A2, B2). The continuous habitat projections (A1, A2) were converted into binary (0/1) projections by using automatically generated thresholds that maximized the TSS values of the ensemble models. The red lines in A2 and B2 represent the boundaries among the three populations.
Species- and population-level SDMs both predicted habitat contraction for the SHP and NAP and habitat expansion for the NPP, while the changed magnitude of habitats depended on the climate change scenarios. The pessimistic scenario with uncontrolled greenhouse gas emissions (RCP 8.5) resulted in a large variation in the suitable range (Table 6). Generally, the species-level model, compared with the population-level model, presented more pessimistic results for the SHP and NAP, with a greater loss of potential suitable habitat, and more optimistic results for the NPP, with a larger extension of suitable habitat. We presented the habitat change in the three populations projected by species-level and population-level models under RCP 8.5 in the 2100s for explanation. Species-level and population-level models predicted differential impacts of climate change on the potentially suitable habitat (Figure 8). Both the species model and the SHP model predicted that the SHP gained a large amount of suitable area in Port Argentina, Gulf of Carpentaria and in the waters off Antarctica, while it lost many suitable areas, mainly in the offshore areas of the Antarctic continent (Figures 8A1, B1). Both the species model and the NAP model predicted the loss of suitable habitats for the NAP under RCP 8.5 in the 2100s, while the degree of loss was different between the models, with 47.3% and 26.6% losses predicted by each, respectively (Table 6). The species model predicted that the NAP would gain large areas of suitable habitat in Hudson Bay (Figure 8A2), while the NAP model predicted a marginal extension of suitable habitat to the southern part of this bay (Figure 8B2). The species model and NPP model predicted increases in suitable habitat for the NPP by 23.5% and 30.1%, respectively, under RCP 8.5 in the 2100s. The species model predicted the complete disappearance of potential habitat for the NPP in the Yellow and Bohai Seas of China (Figure 8A3), while the results from the NPP model were relatively optimistic, with a small part of these areas still suitable for the NPP in the future (Figure 8B3). Both models predicted the expansion of suitable habitat across the Bering Strait to the Arctic Ocean and a loss of habitat in the southern waters (Figure 8A3, B3).
Table 6 Predicted size of changes (%) in species range based on population-level (species-level) models under the representative concentration pathways (RCPs) 2.6 and 8.5 at the middle (2050s) and end (2100s) of the 21st century.
Figure 8 Change in future habitat suitability projected by the species-level model (A1–A3) and population-level model (B1–B3) for the Southern Hemisphere population (SHP), the North Atlantic population (NAP) and the North Pacific population (NPP) of the common minke whale respectively in the 2100s under the representative concentration pathway (RCP) 8.5. The category “Loss” represents areas projected to be suitable under current climatic conditions but unsuitable under future climatic conditions; “Stable” represents areas projected to be suitable under both current and future climatic conditions; and “Gain” represents areas projected to be unsuitable under current climatic conditions but suitable under future climatic conditions.
This study quantified the realized niche of the three populations of common minke whales and found that the niche space of the SHP was much larger than that of the NAP and NPP. The wider distribution of the SHP in the Southern Hemisphere, in contrast with the relatively aggregated distributions of the NAP and NPP, was probably the reason for the larger climatic range of this population. Significant niche differentiation among the three populations suggested by the paired niche similarity tests, combined with recent studies on partial sequences of mitochondrial and nuclear DNA of common minke whales (Ramirez-Flores et al., 2019), suggested the violation of “niche conservatism” and the existence of local adaptation. The SDMs constructed at the population level presented differential responses of the three populations to climatic predictors, which further verified the adaptations of these populations to their respective environmental conditions.
The results from the four-dimensional hypervolume indicated that there was no significant climatic niche conservatism. This implied that the three populations responded to environmental gradients differently and confirmed the importance to develop SDMs below the species level. This local adaptation may be reflected by morphological and genetic differences (Rice, 1998). For instance, the SHP of common minke whales possesses a distinct white flipper mark and has a smaller body size than the NAP and NPP; additionally, the NPP has a relatively short rostrum and a different pattern of white bands on the flipper than the NAP. In this study, though the species- and population-level SDMs projected a similar change trend in the species range, with a contraction of suitable habitat for the NAP and SHP and an expansion of suitable habitat for the NPP under future climate change scenarios, but the range change magnitudes projected by the two types of models were different. The population-level model outputted more optimistic results for the SHP and NAP with less suitable habitat loss. This finding is consistent with several published studies, which suggested that adaptive genetic variation within a species can buffer the species’ vulnerability to climate change (Oney et al., 2013; Ikeda et al., 2017; Razgour et al., 2019; Zhang et al., 2021).
The three populations of common minke whales inhabit areas with distinct environmental conditions, resulting in niche differentiation and implying that climate-induced environmental changes will exert different effects on the populations. Of the three populations, our model results indicated that the NAP is the most vulnerable to climate change. The continuing rise in water temperature may directly impact the distribution of the NAP, e.g., through energy budgets and thermoregulation (Park et al., 2015; Heide-Jørgensen et al., 2020); however, the indirect effects, i.e., through influences on prey distribution (Tetley et al., 2008), may be more prominent. The prey of this species is diverse, ranging from euphausiids to several fish species, and depends on season and location (Víkingsson et al., 2015). Edwards et al. (2021) found that warming of the North Atlantic drives decreases in krill abundance with no associated range shift. Fossheim et al. (2015) found that climate change and warming induced borealization of fish communities in the Arctic. Temperature warming-induced habitat loss and range shift of prey are likely the key to habitat loss of the NAP. In contrast, ice thickness is the most important factor for the distribution of the SHP, but the effect may be indirect, through its influence on prey distribution. Antarctic krill (Euphausia superba) is the main prey of the SHP. However, sea ice extent has the predominant influence on the survival of Antarctic krill larvae in the South Ocean and the loss of winter sea ice results in declines in krill recruitment (Flores et al., 2012; Piñones and Fedorov, 2016). The abundance decrease and range shift of prey exert parallel influences on the distribution of its predators. For the SHP, the considerable loss of suitable habitat in offshore waters and gain of suitable habitat in the nearshore areas of Antarctica are probably caused by the change of distribution and abundance of prey. The larger loss and smaller gain of suitable habitat implies that climate change will negatively impact this population. Chlorophyll concentration was the most important factor for the distribution of the NPP and the waters with a high chlorophyll concentration attracted more prey to accumulate (Tetley et al., 2008), resulting in the high occurrence of the NPP to these areas. Climate change-induced decreases of chlorophyll concentration will alter the distribution patters of the NPP in order to trace for the suitable feeding grounds. The current distributed locations of the NPP facilitate it crossing the Bering Sea to colonize new habitats in the Arctic Ocean, indicating future habitat expansion. Previous studies have detected poleward shifts in many terrestrial and marine species in response to climate change (e.g., Hällfors et al., 2016; Zhang et al., 2020; Abe et al., 2021; Fu et al., 2021), consistent with the findings of this study.
Although our study provides important data for designing climate-adaptive conservation strategies, it has some limitations. First, we used multiyear averages of environmental factors to represent current and future climate conditions, making it difficult to explore more fine-grained (e.g., annual and monthly) environmental changes in the distribution of the common minke whale. For instance, the timing of sea ice formation and retreat may strongly influence the distribution of prey and thus indirectly affect the potential distribution of common minke whales. Similarly, short-term climate forcing, especially for El Niño and La Niña which break these normal conditions, may have great impact on the distribution of cetaceans (Benson et al., 2002). Failing to account for these environmental changes at a more fine-grained time scale will affect the predictive accuracy of SDMs. Therefore, our results can only represent general change trends in the distribution of the common minke whale in the face of climate change, over large temporal and spatial scales. Second, the time frame for Bio-Oracle layers (2000–2014) was misaligned with a small proportion of the occurrence records used to train our models (with 26% of records predating 2000). This disparity may bias species-environment associations and result in underestimates of the impacts of climate change on habitat suitability. Third, though the flexibility in the common minke whale’s diet, several studies have proved the decline of its prey induced by climate change, which is likely the direct threats to the recovery of these whales (e.g., Tulloch et al., 2018; Edwards et al., 2021). With the integrated considerations of environmental change and prey declines induced by climate change in the future work, more pessimistic conditions are probable for the common minke whale. Fourth, we must realize that dispersal ability is an important factor affecting the potential distribution of the species. The common minke whale may not have the ability to reach all the suitable areas because of the presence of some barriers such as temperature gradients. Our study probably overestimates the potential distribution of common minke whales, especially for the NPP, under the assumption of an unlimited dispersal ability.
Though facing unprecedented anthropogenic and climate change stresses, the common minke whale has attracted little conservation or management attention. Tulloch et al. (2018) investigated the impacts of climate on populations of baleen whales and their prey in the Southern Ocean and concluded that the future recovery of baleen whales will be imperiled by climate change. Weelden et al. (2021) presented a review of the literature on the impacts of climate change on cetacean distribution, habitat and migration and highlighted the significant research gaps regarding this topic. Therefore, it is urgent to estimate the climate impacts on the habitat suitability of common minke whales for designing climate-adaptive conservation and management strategies. Overall, the three populations showed a poleward shift in response to a warming climate. However, the NAP and SHP are more vulnerable than the NPP to climate impacts, as indicated by the limited area available for colonization when they lose too much of their range in offshore waters. Therefore, these two populations deserve more conservative attention in the face of climate stresses.
Marine protected areas (MPAs) have been proven to be an effective in situ tool to protect endangered species and maintain ecosystem services (Wang and Li, 2021). The Mexican government built the world’s largest whale MPA network, with a total area of 3,000,000 km2, encompassing all the waters in the Pacific Ocean, the Atlantic Ocean and the Caribbean Sea within the country’s exclusive economic zone, with the aim of protecting 39 whale species living in these waters legally (Villalobos and Mansilla, 2018). However, climate-induced marine environment changes in MPAs may result in inadaptability of the protected species and reduce the effectiveness of MPAs (Bruno et al., 2018). For instance, Hunt et al. (2020) estimated the potential distribution of Australian humpback dolphins within an established MPA in Australia and found a negative impact of climate change on protective effectiveness. Mobile MPAs, whose boundaries can shift across space and time, have been suggested to safeguard the dynamic habitats and migratory species in a changing ocean (Maxwell et al., 2020). Davies et al. (2017) argued that explicit consideration of future range shifts and contractions of species is essential for the effective establishment of large MPAs. Climate change-induced range shifts called for the need to build mobile MAPs for the common minke whale. Our spatiotemporal predictions of suitable habitats provided essential knowledge for planning the location, size and boundary of mobile MPAs.
The current and future potential distributions of this species are mainly located in coastal waters, so controlling anthropogenic environmental pollution and reducing fishing pressure on these waters are also vital for conserving this species. Given the vulnerability of the SHP and NAP to climate change, we recommended stricter whaling restrictions, such as the prohibition of commercial whaling and reduction in whales captured for scientific studies, in these two populations. Additionally, the new suitable areas colonized by the NAP in the coastal waters of Norway and Sweden and by the SHP in the Weddell Sea deserve more conservation attention in the future. For instance, closed fishing season and area are alternative conservation measures in consideration of the influences of prey on the whale distribution. Despite the resilience of the NPP to climate change, this population will lose a large amount of suitable area in the nearshore areas of the Yellow Sea and Bohai Sea in the future. The ecological system of these areas is recovering from the intense historical anthropogenic stresses due to the recent promulgations of more eco-friendly strategies in China. The finer predictions at the local scale are expected to provide more oriented conservation and management strategies for the common minke whale in the coastal waters of China.
Finally, we must emphasize that MPAs and other protective measures recommended by us can only protect the current and future suitable habitats of whales from anthropogenic disturbance. However, the suitable habitats of SHP and NAP decreased with the increase of greenhouse gas concentration. With uncontrolled (i.e., business-as-usual) emissions over a long period of time, these two populations will be gradually driven to extinction. Hence, decreasing anthropogenic-induced greenhouse gas emission is the ultimate solution for the sustainability of these populations.
This study represented the first step to estimate the climate impacts on the potential distribution of the common minke whale. The significant differentiation of niche space and different responses to climatic predictors among the three populations signified the violation of the assumption of “niche conservatism” and highlighted the importance of constructing SDMs that integrate local adaptation. The spatiotemporal predictions of habitat suitability can provide essential knowledge for designing climate-adaptive conservation and management strategies, such as the delimitation of mobile MPAs. Further research will focus on the probabilities of estimating habitat suitability with the integrated consideration of the climate-induced environment and prey variations.
The original contributions presented in the study are included in the article/Supplementary Material. Further inquiries can be directed to the corresponding author.
Ethical review and approval was not required for the animal study because We used the data downloaded from the online datasets and published papers to conduct our study and did not carry out the field work.
BS conceived and designed the experiments, performed the experiments, analyzed the data, prepared figures and tables, authored drafts of the paper and approved the final draft. LZ and CL conceived and designed the experiments, reviewed drafts of the paper and approved the final draft. FS, ZL and JT provided partial data and approve the final draft. All authors contributed to the article and approved the submitted version.
We are grateful to the data provider of the Global Biodiversity Information Facility (GBIF), Ocean Biogeographic Information System (OBIS) and Bio-ORACLE v2.1 datasets. This work was funded by the National Key R&D Program of China (2020YFD0901205).
The authors declare that the research was conducted in the absence of any commercial or financial relationships that could be construed as a potential conflict of interest.
All claims expressed in this article are solely those of the authors and do not necessarily represent those of their affiliated organizations, or those of the publisher, the editors and the reviewers. Any product that may be evaluated in this article, or claim that may be made by its manufacturer, is not guaranteed or endorsed by the publisher.
The Supplementary Material for this article can be found online at: https://www.frontiersin.org/articles/10.3389/fmars.2022.923205/full#supplementary-material
Abe H., Suzuki H., Kitano Y. F., Kumagai N. H., Mitsui S., Yamano H. (2021). Climate-induced species range shift and local adaptation strategies in a temperate marine protected area, ashizuri-uwakai national park, Shikoku island, western Japan. Ocean Coast. Manage. 210, 105744. doi: 10.1016/j.ocecoaman.2021.105744
Aiello-Lammens M. E., Boria R. A., Radosavljevic A., Vilela B., Anderson R. P. (2015). spThin: an r package for spatial thinning of species occurrence records for use in ecological niche models. Ecography 38, 541–545. doi: 10.1111/ecog.01132
Allouche O., Tsoar A., Kadmon R. (2006). Assessing the accuracy of species distribution models: prevalence, kappa and the true skill statistic (TSS): Assessing the accuracy of distribution models. J. Appl. Ecol. 43, 1223–1232. doi: 10.1111/j.1365-2664.2006
Araújo M. B., Peterson A. T. (2012). Uses and misuses of bioclimatic envelope modeling. Ecology 93, 1527–1539. doi: 10.1890/11-1930.1
Assis J., Tyberghein L., Bosch S., Verbruggen H., Serrão E. A., De Clerck O., et al. (2018). Bio-ORACLE v2.0: Extending marine data layers for bioclimatic modelling. Glob. Ecol. Biogeogr. 27, 277–284. doi: 10.1111/geb.12693
Baringer M., Bif M. B., Boyer T., Bushinsky S. M., Carter B. R., Cetinić I., et al. (2020). Global oceans. Bull. Amer. Meteorol. Soc. 101, S129–S184. doi: 10.1175/BAMS-D-20-0105.1
Basher Z., Bowden D. A., Costello M. J. (2018) Global marine environment dataset (GMED) (World Wide Web electronic publication). Available at: http://gmed.auckland.ac.nz (Accessed October 30, 2021).
Benson S. R., Croll D. A., Marinovic B. B., Chavez F. P., Harvey J. T. (2002). Changes in the cetacean assemblage of a coastal upwelling ecosystem during El niño 1997–98 and la niña 1999. Prog. Oceanogr. 54, 279–291. doi: 10.1016/S0079-6611(02)00054-X
Blonder B., Morrow C. B., Harris D. J., Brown S., Butruille G., Laini A., et al. (2021) Hypervolume: high dimensional geometry and set operations, projection, and inference using kernel density estimation, support vector machines, and convex hulls. r package version 3.0.0. Available at: https://CRAN.R-project.org/package=hypervolume.
Boer M. N. (2010). Spring distribution and density of minke whale Balaenoptera acutorostrata along an offshore bank in the central north Sea. Mar. Ecol. Prog. Ser. 408, 265–274. doi: 10.3354/meps08598
Bowen W. D. (1997). Role of marine mammals in aquatic ecosystems. Mar. Ecol. Prog. Ser. 158, 267–274. doi: 10.3354/meps158267
Breitburg D., Levin L. A., Oschlies A., Grégoire M., Chavez F. P., Conley D. J., et al. (2018). Declining oxygen in the global ocean and coastal waters. Science 359, eaam7240. doi: 10.1126/science.aam7240
Broennimann O., Cola V. D., Guisan A. (2021) Ecospat: spatial ecology miscellaneous methods. r package version 3.2. Available at: https://CRAN.R-project.org/package=ecospat.
Brower A. A., Clarke J. T., Ferguson M. C. (2018). Increased sightings of subArctic cetaceans in the eastern chukchi Sea 2008–2016: population recovery, response to climate change, or increased survey effort? Polar Biol. 41, 1033–1039. doi: 10.1007/s00300-018-2257-x
Bruno J. F., Bates A. E., Cacciapaglia C., Pike E. P., Amstrup S. C., Hooidonk R. V., et al. (2018). Climate change threatens the world’s marine protected areas. Nat. Clim Change 8, 499–503. doi: 10.1038/s41558-018-0149-2
Cardoso P., Mammola S., Rigal F., Carvalho J. (2021) BAT: biodiversity assessment tools. r package version 2.7.1. Available at: https://CRAN.R-project.org/package=BAT.
Cartagena-Matos B., Lugué K., Fonseca P., Marques T. A., Prieto R., Alves F. (2021). Trends in cetacean research in the Eastern north Atlantic. Mam Rev. 51, 436–453. doi: 10.1111/mam.12238
Carvalho J. C., Cardoso P. (2020). Decomposing the causes for niche differentiation between species using hypervolumes. Front. Ecol. Evol. 8. doi: 10.3389/fevo.2020.00243
Chardon N. I., Pironon S., Peterson M. L., Doak D. F. (2020). Incorporating intraspecific variation into species distribution models improves distribution predictions, but cannot predict species traits for a wide-spread plant species. Ecography 43, 60–74. doi: 10.1111/ecog.04630
Chen Y., Shan X., Ovando D., Yang T., Dai F., Jin X. (2021). Predicting current and future global distribution of black rockfish (Sebastes schlegelii) under changing climate. Ecol. Indic. 128, 107799. doi: 10.1016/j.ecolind.2021.107799
Cheung W. W. L., Watson R., Pauly D. (2013). Signature of ocean warming in global fisheries catch. Nature 497, 365–368. doi: 10.1038/nature12156
Davies T. E., Maxwell S. M., Kaschner K., Garilao C., Ban N. C. (2017). Large Marine protected areas represent biodiversity now and under climate change. Sci. Rep. 7, 9569. doi: 10.1038/s41598-017-08758-5
DeMarche M. L., Doak D. F., Morris W. F. (2019). Incorporating local adaptation into forecasts of species’ distribution and abundance under climate change. Glob. Change Biol. 25, 775–793. doi: 10.1111/gcb.14562
Dormann C. F. (2007). Promising the future? global change projections of species distributions. Basic Appl. Ecol. 8, 387–397. doi: 10.1016/j.baae.2006.11.001
Dormann C. F., Elith J., Bacher S., Buchmann C., Carl G., Carré G., et al. (2013). Collinearity: a review of methods to deal with it and a simulation study evaluating their performance. Ecography 36, 027–046. doi: 10.1111/j.1600-0587.2012.07348.x
Edwards M., Hélaouët P., Goberville E., Lindley A., Tarling G. A., Burrows M. T., et al. (2021). North Atlantic warming over six decades drives decreases in krill abundance with no associated range shift. Commun. Biol. 4, 644. doi: 10.1038/s42003-021-02159-1
Flores H., Atkinson A., Kawaguchi S., Krafft B., Milinevsky G., Nicol S., et al. (2012). Impact of climate change on Antarctic krill. Mar. Ecol.-Prog. Ser. 458, 1–19. doi: 10.3354/meps09831
Fossheim M., Primicerio R., Johannesen E., Ingvaldsen R. B., Aschan M. M., Dolgov A. V. (2015). Recent warming leads to a rapid borealization of fish communities in the Arctic. Nat. Clim. Change 5, 673–677. doi: 10.1038/NCLIMATE2647
Fu J., Zhao L., Liu C., Sun B. (2021). Estimating the impact of climate change on the potential distribution of IndoPacific humpback dolphins with species distribution model. PeerJ 9, e12001. doi: 10.7717/peerj.12001
Glover K. A., Kanda N., Haug T., Pastene L. A., Øien N., Goto M., et al. (2010). Migration of Antarctic minke whales to the Arctic. PLoS One 5, e15197. doi: 10.1371/journal.pone.0015197
Guisan A., Thuiller W., Zimmermann N. E. (2017). Habitat suitability and distribution models: With applications in r. J. Wildl. Manage. 84, 1212–1213. doi: 10.1002/jwmg.21868
Hällfors M. H., Liao J., Dzurisin J., Grundel R., Hyvärinen M., Towle K., et al. (2016). Addressing potential local adaptation in species distribution models: implications for conservation under climate change. Ecol. Appl. 26, 1154–1169. doi: 10.1890/15-0926
Hastings R. A., Rutterford L. A., Freer J. J., Collins R. A., Simpson S. D., Genner M. J. (2020). Climate change drives poleward increases and equatorward declines in marine species. Curr. Biol. 30, 1572–1577.e2. doi: 10.3354/meps158267
Heide-Jørgensen M. P., Blackwell S. B., Williams T. M., Sinding M. H. S., Skovrind M., Tervo O. M., et al. (2020). Some like it cold: Temperature-dependent habitat selection by narwhals. Ecol. Evol. 10, 8073–8090. doi: 10.1002/ece3.6464
Hunt T. N., Allen S. J., Bejder L., Parra G. J. (2020). Identifying priority habitat for conservation and management of Australian humpback dolphins within a marine protected area. Sci. Rep. 10, 14366. doi: 10.1038/s41598-020-69863-6
Ikeda D. H., Max T. L., Allan G. J., Lau M. K., Shuster S. M., Whitham T. G. (2017). Genetically informed ecological niche models improve climate change predictions. Glob. Change Biol. 23, 164–176. doi: 10.1111/gcb.13470
Iturbide M. (2017) Mopa: Species distribution modeling with pseudo-absences. r package version 1.0.0. Available at: https://CRAN.R-project.org/package=mopa.
IWC (2021). International whaling commission. Available online at:https://www.iwc.int/en. [Accessed December 28, 2021]
Kavanagh A. S., Kett G., Richardson N., Rogan E., Jessopp M. J. (2018). High latitude winter sightings of common minke whale calves (Balaenoptera acutorostrata) in the northeast Atlantic. Mar. Biodivers. Rec. 11, 22. doi: 10.1186/s41200-018-0157-y
Kishida T. (2017). Population history of Antarctic and common minke whales inferred from individual whole-genome sequences. Mar. Mamm. Sci. 33, 645–652. doi: 10.1111/mms.12369
Kiszka J. J., Heithaus M. R., Wirsing A. J. (2015). Behavioural drivers of the ecological roles and importance of marine mammals. Mar. Ecol.-Prog. Ser. 523, 267–281. doi: 10.3354/meps11180
Kramer-Schadt S., Niedballa J., Pilgrim J. D., Schröder B., Lindenborn J., Reinfelder V., et al. (2013). The importance of correcting for sampling bias in MaxEnt species distribution models. Divers. Distrib. 19, 1366–1379. doi: 10.1111/ddi.12096
Lambert E., Pierce G. J., Hall K., Brereton T., Dunn T. E., Wall D., et al. (2014). Cetacean range and climate in the eastern north Atlantic: future predictions and implications for conservation. Glob. Change Biol. 20, 1782–1793. doi: 10.1111/gcb.12560
Lecocq T., Rasmont P., Harpke A., Schweiger O. (2015). Improving international trade regulation by considering intraspecific variation for invasion risk assessment of commercially traded species: The bombus terrestris case. Conserv. Lett. 9, 281–289. doi: 10.1111/conl.12215
Lee D., An Y. R., Park K. J., Kim H. W., Lee D., Joo H. T., et al. (2017). Spatial distribution of common minke whale (Balaenoptera acutorostrata) as an indication of a biological hotspot in the East Sea. Deep-Sea Res. Part II-Top. Stud. Oceanogr. 143, 91–99. doi: 10.1016/j.dsr2.2017.06.005
Le S., Josse J., Husson F. (2008). FactoMineR: An r package for multivariate analysis. J. Stat. Software 25, 1–18. doi: 10.18637/jss.v025.i01
Liu M. M., Lin M. L., Li S. H. (2022). Species diversity and spatiotemporal patterns based on cetacean stranding records in China 1950-2018. Sci. Total Environ. 822, 153651. doi: 10.1016/j.scitotenv.2022.153651
Liu C., White M., Newell G. (2013). Selecting thresholds for the prediction of species occurrence with presence-only data. J. Biogeogr. 40, 778–789. doi: 10.1111/jbi.12058
Luo M., Wang H., Lyu Z. (2017). Evaluating the performance of species distribution models Biomod2 and MaxEnt using the giant panda distribution data. Chin. J. Appl. Ecol. 28, 4001–4006. doi: 10.13287/j.1001-9332.201712.011
Mammola S., Cardoso P. (2020). Functional diversity metrics using kernel density n -dimensional hypervolumes. Methods Ecol. Evol. 11, 986–995. doi: 10.1111/2041-210X.13424
Maxwell S. M., Gjerde K. M., Conners M. G., Crowder L. B. (2020). Mobile protected areas for biodiversity on the high seas. Science 367, 252–254, aaz9327. doi: 10.1126/science
Milmann L., Machado R., Sucunza F., de Oliveira L. R., dos Santos R. A., Di Beneditto A. P. M., et al. (2018). New trophic link and potential feeding area of dwarf minke whale (Balaenoptera acutorostrata subsp.) in mid latitude waters of the southwestern Atlantic ocean. Mammalia 83, 49–52. doi: 10.1515/mammalia-2017-0127
Milmann L., Taguchi M., Siciliano S., Baumgarten J. E., Oliveira L. R., Valiati V. H., et al. (2021). New genetic evidences for distinct populations of the common minke whale (Balaenoptera acutorostrata) in the southern hemisphere. Polar Biol. 44, 1575–1589. doi: 10.1007/s00300-021-02897-2
Mitani Y., Bando T., Takai N., Sakamoto W. (2006). Patterns of stable carbon and nitrogen isotopes in the baleen of common minke whale Balaenoptera acutorostrata from the western north pacific. Fish. Sci. 72, 69–76. doi: 10.1111/j.1444-2906.2006.01118.x
Moss R. H., Edmonds J. A., Hibbard K. A., Manning M. R., Rose S. K., van Vuuren D. P., et al. (2010). The next generation of scenarios for climate change research and assessment. Nature 463, 747–756. doi: 10.1038/nature08823
Murcia S., Riul P., Mendez F., Rodriguez J. P., Rosenfeld S., Ojeda J., et al. (2020). Predicting distributional shifts of commercially important seaweed species in the subantarctic tip of south America under future environmental changes. J. Appl. Phycol. 32, 2105–2114. doi: 10.1007/s10811-020-02084-6
Oney B., Reineking B., O’Neill G., Kreyling J. (2013). Intraspecific variation buffers projected climate change impacts on pinus contorta. Ecol. Evol. 3, 437–449. doi: 10.1002/ece3.426
Park J. Y., An Y.-R., Kanda N., An C.-M., An H. S., Kang J.-H., et al. (2015). Cetaceans evolution: insights from the genome sequences of common minke whales. BMC Genomics 16, 13. doi: 10.1186/s12864-015-1213-1
Pastene L. A., Goto M., Kanda N., Zerbini A. N., Kerem D., Watanabe K., et al. (2007). Radiation and speciation of pelagic organisms during periods of global warming: the case of the common minke whale, Balaenoptera acutorostrata. Mol. Ecol. 16, 1481–1495. doi: 10.1111/j.1365-294X.2007.03244
Peñalver-Alcázar M., Jiménez-Valverde A., Aragón P. (2021). Niche differentiation between deeply divergent phylogenetic lineages of an endemic newt: implications for species distribution models. Zoology 144, 125852. doi: 10.1016/j.zool.2020.125852
Perrin W. F., Mallette S. D., Brownell R. L. (2018). “Minke whales: Balaenoptera acutorostrata and b. bonaerensis,” in The encyclopedia of marine mammals. Eds. Würsig B., Thewissen J. G. M., Kovacs K. M. (FL: Academic Press), 608–613.
Piñones A., Fedorov A. V. (2016). Projected changes of Antarctic krill habitat by the end of the 21st century. Geophys. Res. Lett. 43, 8580–8589. doi: 10.1002/2016GL069656
Ramirez-Flores O., Birtles A., Pazmino D., Zenger K. R., Van-Herwerden L. (2019). Dwarf minke whales from the south pacific share a matrilineal lineage distinct from Balaenoptera acutorostrata and B. a. scammoni. Aust. Mammal. 41, 231–240. doi: 10.1071/AM18015
Razgour O., Forester B., Taggart J. B., Bekaert M., Juste J., Ibáñez C., et al. (2019). Considering adaptive genetic variation in climate change vulnerability assessment reduces species range loss projections. Proc. Natl. Acad. Sci. U. S. A. 116, 10418–10423. doi: 10.1073/pnas.1820663116
Risch D., Norris T., Curnock M., Friedlaender A. (2019). Common and Antarctic minke whales: conservation status and future research directions. Front. Mar. Sci. 6. doi: 10.3389/fmars.2019.00247
Robinson N. M., Nelson W. A., Costello M. J., Sutherland J. E., Lundquist C. J. (2017). A systematic review of marine-based species distribution models (SDMs) with recommendations for best practice. Front. Mar. Sci. 4. doi: 10.3389/fmars.2017.00421
Robinson K. P., Tetley M. J., Mitchelson-Jacob E. J. (2009). The distribution and habitat preference of coastally occurring minke whales (Balaenoptera acutorostrata) in the outer southern Moray firth, northeast Scotland. J. Coast. Conserv. 13, 39–48. doi: 10.1007/s11852-009-0050-2
Roman J., Estes J. A., Morissette L., Smith C., Costa D., McCarthy J., et al. (2014). Whales as marine ecosystem engineers. Front. Ecol. Environ. 12, 377–385. doi: 10.1890/130220
Rosel P. E., Wilcox L. A., Monteiro C., Tumlin M. C. (2016). First record of Antarctic minke whale, balaenoptera bonaerensis, in the northern gulf of Mexico. Mar. Biodivers. Rec. 9, 63. doi: 10.1186/s41200-016-0058-x
Salvadeo C., Lluch-Cota S., Maravilla-Chávez M., Álvarez-Castañeda S., Mercuri M., Ortega-Rubio A. (2013). Impact of climate change on sustainable management of gray whale (Eschrichtius robustus) populations: Whale-watching and conservation. Arch. Biol. Sci. 65, 997–1005. doi: 10.2298/ABS1303997S
Sánchez-Mercado A. Y., Ferrer-Paris J. R. (2010). Mapping species distributions: Spatial inference and prediction (Cambridge, UK: Cambridge University Press).
Schickele A., Leroy B., Beaugrand G., Goberville E., Hattab T., Francour P., et al. (2020). Modelling European small pelagic fish distribution: Methodological insights. Ecol. Model. 416, 108902. doi: 10.1016/j.ecolmodel.2019.108902
Singer A., Millat G., Staneva J., Kröncke I. (2017). Modelling benthic macrofauna and seagrass distribution patterns in a north Sea tidal basin in response to 2050 climatic and environmental scenarios. Estuar. Coast. Shelf Sci. 188, 99–108. doi: 10.1016/j.ecss.2017.02.003
Smith A. B., Alsdurf J., Knapp M., Baer S. G., Johnson L. C. (2017). Phenotypic distribution models corroborate species distribution models: A shift in the role and prevalence of a dominant prairie grass in response to climate change. Glob. Change Biol. 23, 4365–4375. doi: 10.1111/gcb.13666
Smith R. C., Dustan P., Au D., Baker K. S., Dunlap E. A. (1986). Distribution of cetaceans and sea-surface chlorophyll concentrations in the California current. Mar. Biol. 91, 385–402. doi: 10.1007/BF00428633
Smith L. V., McMinn A., Martin A., Nicol S., Bowie A. R., Lannuzel D. (2013). Preliminary investigation into the stimulation of phytoplankton photophysiology and growth by whale faeces. J. Exp. Mar. Biol. Ecol. 446, 1–9. doi: 10.1016/j.jembe.2013.04.010
Song K. J., Zhang C. I. (2014). Stomach contents of bycaught minke whales (Balaenoptera acutorostrata) in Korean waters. Mar. Biol. Res. 10, 400–406. doi: 10.1080/17451000.2013.814787
Soultan A., Pavón-Jordán D., Bradter U., Sandercock B. K., Hochachka W. M., Johnston A., et al. (2022). The future distribution of wetland birds breeding in Europe validated against observed changes in distribution. Environ. Res. Lett. 17, 024025. doi: 10.1088/1748-9326/ac4ebe
Tamura T., Fujise Y. (2002). Geographical and seasonal changes of prey species of minke whales in the northwestern pacific. ICES J. Mar. Sci. 59, 516–528. doi: 10.1006/jmsc.2002.1199
Tetley M. J., Mitchelson-Jacob E. G., Robinson K. P. (2008). The summer distribution of coastal minke whales (Balaenoptera acutorostrata) in the southern outer Moray firth, NE Scotland, in relation to co-occurring mesoscale oceanographic features. Remote Sens. Environ. 112, 3449–3454. doi: 10.1016/j.rse.2007.10.015
Thuiller W., Georges D., Gueguen M., Engler R., Breiner F. (2021) biomod2: ensemble platform for species distribution modeling. r package version 3.5.1. Available at: https://CRAN.R-project.org/package=biomod2.
Todd V. L. G., Williamson L. D. (2022). Cetacean distribution in relation to oceanographic features at the kerguelen plateau. Polar Biol. 45, 113–126. doi: 10.1007/s00300-021-02977-3
Tulloch V. J. D., Plagányi E. E., Brown C., Richardson A. J., Matear R. (2018). Future recovery of baleen whales is imperiled by climate change. Glob. Change Biol. 25, 1263–1281. doi: 10.1111/gcb.14573
Víkingsson G. A., Pike D. G., Valdimarsson H., Schleimer A., Gunnlaugsson T., Silva T., et al. (2015). Distribution, abundance, and feeding ecology of baleen whales in icelandic waters: have recent environmental changes had an effect? Front. Ecol. Evol. 3. doi: 10.3389/fevo.2015.00006
Villalobos M. M., Mansilla S. P. (2018). Marine mammals of Mexico: Richness patterns, protected areas, and conservation trends. Estuar. Coast. Shelf S. 208, 153–160. doi: 10.1016/j.ecss.2018.05.002
Wang W., Li J. S. (2021). In-situ conservation of biodiversity in China: advances and prospects. Biodiv Sci. 29, 133–149. doi: 10.17520/biods.2020070
Wang Z., Tian J., Lei L., Du J., Liu W., Han J., et al. (2020). Species identification and morphological measurements of a pregnant minke whale (Balaenoptera acutorostrata acutorostrata). Acta Theriol Sin. 40, 122–128. doi: 10.16829/j.slxb.150376
Weelden C. V., Towers J. R., Bosker T. (2021). Impacts of climate change on cetacean distribution, habitat and migration. Clim. Change Ecol. 1, 100009. doi: 10.1016/j.ecochg.2021.100009
Zhang Z., Kass J. M., Mammola S., Koizumi I., Li X., Tanaka K., et al. (2021). Lineage-level distribution models lead to more realistic climate change predictions for a threatened crayfish. Divers. Distrib. 27, 684–695. doi: 10.1111/ddi.13225
Keywords: balaenoptera acutorostrata, climate change, species distribution models, habitat suitability, local adaptation
Citation: Sun B, Zhao L, Shao F, Lu Z, Tian J and Liu C (2022) Estimating the impacts of climate change on the habitat suitability of common minke whales integrating local adaptation. Front. Mar. Sci. 9:923205. doi: 10.3389/fmars.2022.923205
Received: 19 April 2022; Accepted: 19 July 2022;
Published: 09 August 2022.
Edited by:
Oscar Sosa-Nishizaki, Center for Scientific Research and Higher Education in Ensenada (CICESE), MexicoReviewed by:
Anna Maria Addamo, Joint Research Centre (JRC), ItalyCopyright © 2022 Sun, Zhao, Shao, Lu, Tian and Liu. This is an open-access article distributed under the terms of the Creative Commons Attribution License (CC BY). The use, distribution or reproduction in other forums is permitted, provided the original author(s) and the copyright owner(s) are credited and that the original publication in this journal is cited, in accordance with accepted academic practice. No use, distribution or reproduction is permitted which does not comply with these terms.
*Correspondence: Changdong Liu, Y2hhbmdkb25nQG91Yy5lZHUuY24=
Disclaimer: All claims expressed in this article are solely those of the authors and do not necessarily represent those of their affiliated organizations, or those of the publisher, the editors and the reviewers. Any product that may be evaluated in this article or claim that may be made by its manufacturer is not guaranteed or endorsed by the publisher.
Research integrity at Frontiers
Learn more about the work of our research integrity team to safeguard the quality of each article we publish.