- 1Earth Sciences Department, University of Toronto, Toronto, ON, Canada
- 2Chemical and Physical Sciences Department, University of Toronto Mississauga, Mississauga, ON, Canada
- 3Geography, Geomatics and Environment, University of Toronto Mississauga, Mississauga, ON, Canada
- 4Laboratory of Tree-Ring Research, College of Science, University of Arizona, Tucson, AZ, United States
- 5GEOMAR Helmholtz Centre for Ocean Research Kiel, Kiel, Germany
- 6Institut für Geowissenschaften, Christian-Albrechts-Universität zu Kiel, Kiel, Germany
- 7Bodega Marine Laboratory, Coastal and Marine Sciences Institute, University of California, Davis, Davis, CA, United States
- 8Department of Earth and Planetary Science, University of California, Davis, Davis, CA, United States
- 9Department of Earth Sciences, University of Geneva, Geneva, Switzerland
Paleoclimate and paleoenvironmental reconstructions from increment-yielding archives strongly depend on precise age models. Like bivalves, corals, trees, and speleothems, the coralline alga Clathromorphum compactum produces annual growth increments and shows considerable promise as an environmental archive for arctic and subarctic regions. Though their growth increment widths correlate with temperature and sea ice cover in high Arctic regions, existing timeseries have not been crossdated. In fact, previous studies have shown a lack of inter-sample correlation in non-crossdated timeseries suggesting possible age model dating errors. Here, we use dendrochronology crossdating techniques and COFECHA software to ensure and validate synchrony between C. compactum timeseries (<141 years) from three specimens collected near Beechey Island, Nunavut, Canada. Results showed that non-crossdated timeseries constructed by four coralline red algae researchers using annual increments of the same C. compactum samples were highly variable and showcase the likelihood of dating errors in non-crossdated timeseries. Crossdating improved inter-series correlations, and correlations to sea ice-related records, suggesting that at least three crossdated timeseries are required to isolate paleoclimate signals. Our findings suggest that future reconstructions with C. compactum should employ crossdating techniques to reduce dating errors and allow for more precise climate reconstructions.
Lay Abstract: Long-term environmental records provide a critical baseline to examine how humans have impacted Earth’s natural climate. An important piece to consider is sea ice’s role in natural climate variability because its brightness limits warming by reflecting solar irradiation back to space. However, instrumental records of sea ice rarely extend beyond the early satellite era (late 1970s), limiting our understanding of how sea ice affects natural climate variability in the preindustrial era. A lack of historical baseline prompted the development of sea ice proxies, including the long-lived marine alga, Clathromorphum compactum. Similar to tree-rings, C. compactum produces a new mineralized layer each year, and layer thicknesses have been shown to respond to sea ice cover, making them useful to record long-term sea ice variability. However, a recent study showed that records had replicability problems, maybe due to dating mistakes. Our study applies tree-ring dating methods (dendrochronology) to match annual algal growth layers across algal specimens. Results showed that these new methods reduced dating errors, allowing for more precise past sea ice cover reconstructions.
1 Introduction
1.1 Dating Methods for Biogenic Environmental Proxies
Sclerochronology – the study of physical and chemical variability in the incremental structures of invertebrates, fish otoliths and non-geniculate coralline red algae – plays an important role in the field of paleo-environmental reconstructions due to the significant environmental controls on chemical variations, growth rates and on the cyclicity of growth band formation (Prendergast et al., 2017). Growth increment (or growth band) widths often have a strong relationship with one environmental parameter, but in many cases growth mechanisms are complex due to various controls on growth (Weymouth, 1922; Ansell, 1968; Schöne et al., 2003; Witbaard et al., 2003; Hippler et al., 2009; Butler et al., 2010; Ballesta-Artero et al., 2017). When growth increments are strongly related to a single environmental variable, this variable is often the dominant variable changing interannually. For example, growth increments from the coralline red alga Clathromorphum compactum have been shown to strongly correlate with sea ice cover but only in high Arctic regions were sea ice is likely to be the dominant interannual variable (Leclerc et al., 2021).
When validated by multiple samples overlapping in age, sclerochronological timeseries can be used to reconstruct paleo-environmental variables going back centuries (Butler et al., 2009; Butler et al., 2013; DeLong et al., 2014; Reynolds et al., 2016; Reynolds et al., 2017). To properly judge rates of change and natural ranges of variability to include in model simulations, proxy dating must be reliable. In the case of individual, live-collected samples, the date of collection is known and can be used as an initial anchor point to count back in time. However, measurement timeseries that are dated by increment count alone have inherent temporal uncertainty due to locally absent or unresolvable (e.g., extremely narrow) growth increments that can be heterogeneous between samples and within different cross-sections of individual samples. For instance, corals, which typically rely on a single core sample, are reported to have ± 1 to 5 years of uncertainty per century (Swart et al., 1996; Felis et al., 2000; DeLong et al., 2007; Shen et al., 2008; DeLong et al., 2013). These uncertainties are due to complex and sometimes irregular structural growth across the coral colony (DeLong et al., 2013). Errors can propagate back through time, which even at a rate of 1-5% can complicate reconstructions of interannual and decadal climate variability such as the El Nino-Southern Oscillation (DeLong et al., 2013; Black et al., 2016). Temporal measurement errors in individual sample timeseries, however, can be effectively identified and corrected by crossdating. Crossdating is a hypothesis-driven method first established in dendrochronology – the study of tree rings (Glock, 1937; Fritts, 1976) – to scrutinize and validate age models of individual samples by comparing measurement timeseries (e.g., growth or other geochemical variables) with those of other independently validated samples from a given species and collection site, or to a master chronology (i.e., the averaged timeseries of several crossdated samples also from the same species and site). The crossdating method is an iterative and retrospective process that seeks to identify synchronous patterns in width, anatomy, or geochemical data among multiple samples, information that is used to diagnose dating errors (i.e., missed, false or locally absent increments) (Fritts, 1976). Accordingly, crossdating has increasingly been used in scherochronology, matching widths of growth increments among live-collected individuals with dead-collected samples to create chronologies that extend farther back in time (Butler et al., 2013).
Radiometric dating is often used to verify absolute dating of long-term crossdated chronologies (Linge et al., 2008; Butler et al., 2009; DeLong et al., 2013; Marali and Schöne, 2015; Reynolds et al., 2016). However, radiometric dating techniques (i.e., accelerator mass spectrometry radiocarbon (14C) or uranium-thorium (230Th) dating) should not be used exclusively without prior crossdating. This is due to error margins and time-averaging of multiple decades resulting from collecting carbonate material from multiple thin increments needed to fulfill analytical weight requirements; 10-15 years of growth in slow growing organisms (DeLong et al., 2013; Rasher et al., 2020). However, in cases where sample replication is high, growth coherence is strong between individual samples, and overlap among samples spans multiple decades, the chances of spurious crossdating, as can be a concern with dead-collected individuals, is very low and additional verification by radiometric dating is less critical.
1.2 Benefits and Limitations of Crossdating
Crossdating is critical to extract environmental signals within proxy data. In the event of dating errors, owing to locally absent or false increments, patterns among samples become offset relative to one another. Then when these misaligned samples are averaged, the final chronology and underlying environmental signals are muted (Black et al., 2016). Crossdating ensures that all samples are correctly aligned in time before a mean chronology is calculated, so that the underlying environmental signal can be isolated and studied. To ensure annual resolution, crossdating focuses on interannual timescales, comparing each increment to its immediate neighbors. Crossdating methods identify synchrony by comparing interannual patterns of relatively wide or narrow increments (or relatively high or low values in the case of geochemical indicators). If an increment is missed or falsely added, the pattern in that individual will appear offset relative to the pattern in the other samples, indicating that an error has occurred. Crossdating is first and foremost a visual technique, but dating should also be statistically verified using software tools like COFECHA (Holmes, 1983). These programs attempt to statistically mimic the process of visual crossdating by removing long-term trends (low-frequency variability) to isolate the interannual variability, and cross-correlate among individuals to quantify synchrony. Long-term trends, as could be induced by the 20th century decline of sea ice or increase in glacial runoff (Hetzinger et al., 2019; Hetzinger et al., 2021), or ontogenetic trends, increase the chances of spurious correlations and are therefore eliminated (i.e., detrended). COFECHA flags unusually insignificant correlations as possible errors, prompting the analyst to visually reinvestigate the original samples and/or geochemical data to determine if a true dating error occurred. Critically, all data must be visually crossdated before statistical verification, since the statistical analysis assesses the correlation between individual samples and the mean dating series defined by all other specimens. If the quality of the mean dating series is lessened by crossdating errors present in individual series, the power of the analysis to detect errors will also be compromised.
Once data have been crossdated, ontogenetic low-frequency trends (if present), as are commonly observed in tree ring growth records, can be detrended using data-adaptive curve fitting methods, a process called standardization (Fritts, 1976). Mathematically, the standardized series are typically calculated as the ratio of observed growth over expected growth (i.e., the ontogenetic growth trend), which renders the standardized timeseries unitless with a stationary mean value and variance (Cook, 1987). In tree-ring studies, the standardized timeseries are often then averaged by individual to attenuate intra-sample noise (i.e., in cases where more than one radii has been measured from an given sample) and then by site to create a mean site chronology which attenuates inter-sample noise and enhances the common signal (Fritts, 1976). In turn, well-crossdated chronologies can be used to hindcast past environmental variability, feedbacks and mechanisms, and the frequency of extreme events (Butler et al., 2013; DeLong et al., 2014).
While crossdating can be used to build robust annually resolved timeseries, it becomes impractical or impossible if the data (e.g., sample images or instrumental output) are of low quality or if there is no synchrony among samples. In addition, there will always be some degree of asynchrony among samples, and the challenge of crossdating is identifying the underlying synchronous patterns through this individual-level “noise.” Indeed, non-synchronous increment patterns that could affect some individuals but not all can result from: 1) pollution (Turekian et al., 1982); 2) extreme variation in water salinity, temperature and turbidity over fine spatial scales (Epplé et al., 2006); 3) disturbed habitats (Stott et al., 2010); 4) injury (Fritts, 1976); and; 5) disease or predation (Marali and Schöne, 2015). If no environmental variable affects growth, as could occur at sites with very favorable growing conditions, then growth will not be synchronous and crossdating will be impossible. Likewise, increments must be clearly formed and samples well prepared to delineate increment boundaries and identify synchronous growth patterns. Finally, individuals must be sufficiently long-lived that patterns can be robustly matched among individuals (Black et al., 2016).
1.3 Application of Crossdating to Coralline Red Algae
Historical climate records are rare in remote regions, such as the Arctic, and climate monitoring stations are sparsely distributed. Therefore, reliable highly resolved baseline environmental data required to understand Arctic environments of the past can only be provided by proxy records. The arctic and subarctic coralline red algae species, Clathromorphum compactum, has been used to produce multi-century, annually resolved proxy timeseries of temperature, sea ice, runoff, primary productivity, and multi-decadal climate oscillations (Halfar et al., 2008; Halfar et al., 2011; Hetzinger et al., 2011; Halfar et al., 2013; Chan et al., 2017; Hou et al., 2018; Hetzinger et al., 2019; Hetzinger et al., 2021; Leclerc et al., 2021). This species’ high-magnesium calcium carbonate skeleton consists of annual calcified growth increments produced at a rate relative to surrounding sea surface temperature (SST) and sunlight access (Williams et al., 2018a) (Figure 1). When continuous magnesium to calcium ratios (Mg/Ca) are measured either with laser ablation inductively coupled mass spectrometry (LA-ICP-MS) or electron microprobe, the annual cyclicity of Mg/Ca ratios can be graphically observed. Cycles of Mg/Ca are produced by the alga preferentially incorporating magnesium during the warm season (summer), and less so in the cold season (winter), enabling their use to date specimens and growth increments (e.g., Caragnano et al., 2017). This dating methodology enabled the use of Clathromorphum compactum growth increments and sub-annual sampling of isotopes and trace elements to further understandings of temperate and Arctic environments.
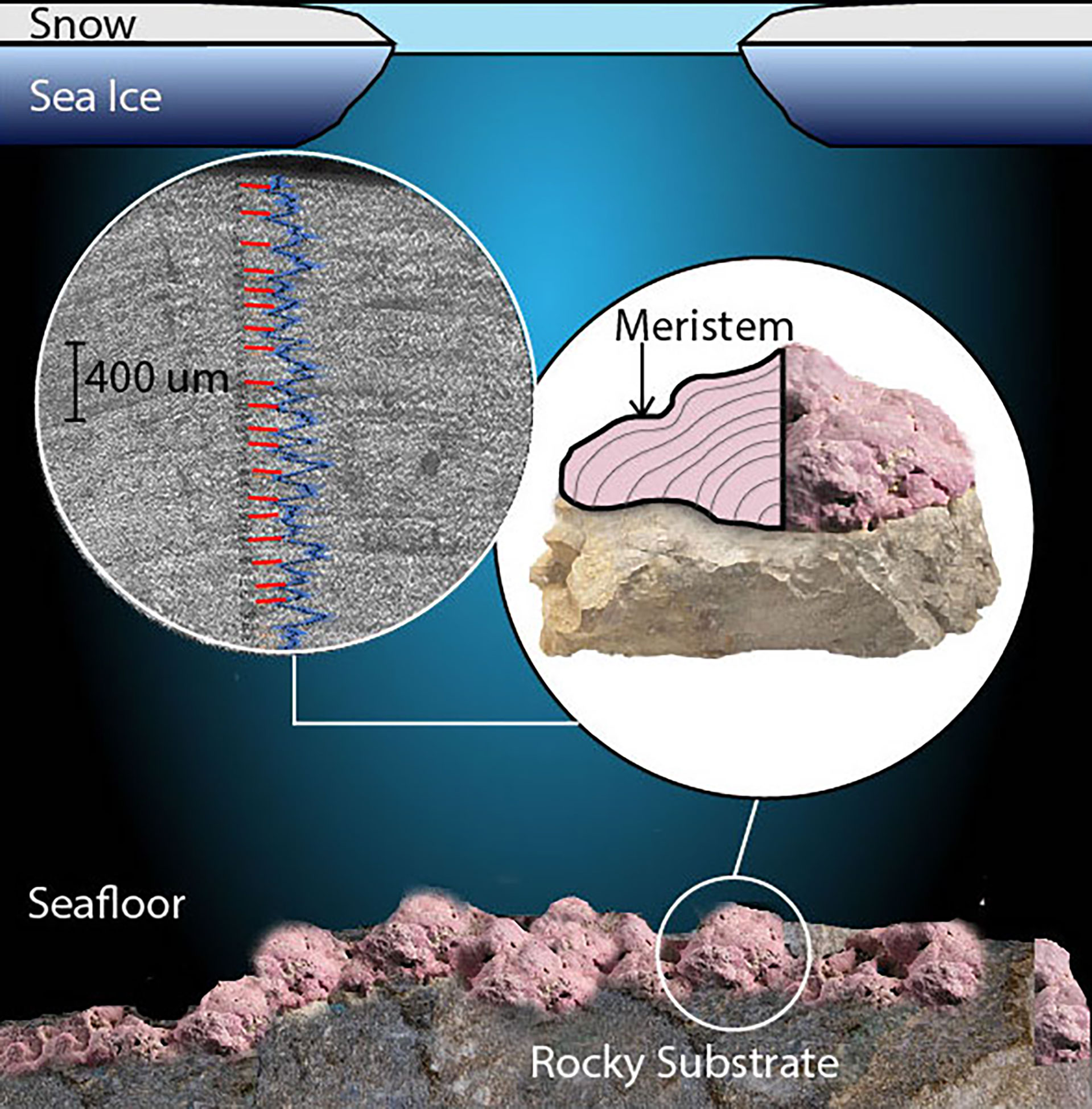
Figure 1 Most of Clathromorphum compactum’s growth occurs during open water conditions in summer months, while dark sea ice covered winter conditions only allow limited growth producing bands of smaller heavily calcified (dark grey) cells. Colonies develop on rocky surfaces at 10-30 m depths. Continuous Mg/Ca measurement transect (blue), along axis of growth superimposed onto high-resolution sample image. Annual Mg/Ca minima shown with red line markers. Modified from Leclerc et al. (2021).
Many previous coralline algae proxy studies have relied on an individual sample per site (Halfar et al., 2013; Fietzke et al., 2015; Chan et al., 2017; Hou et al., 2018). In cases where multiple samples were involved, annual dates (i.e., age models) of staggered samples were determined by what placement produced the best cross-correlation with segments overlapping in 14C or 230Th ages (e.g., (Kamenos, 2010; Williams et al., 2017). However, formal crossdating of growth increments has not yet been applied (Black et al., 2019). Alternatively, a common method involves identifying winter Mg/Ca values (i.e., annual Mg/Ca minima) (Figure 1) as anchor points for annual Mg/Ca cycles, validated by the presence of a visual increment in high-resolution images, and counting the annual cycles back in time (Figure 1). Often multiple overlapping measurements are necessary to avoid the reproductive cavities (i.e., conceptacles) and ensure intra-sample replicability (Figure 2). Finally, researchers average annual width and/or trace element values between samples to produce a non-crossdated mean dating series (e.g., Hetzinger et al., 2019). In instances where climate records are available, climate records can be used to justify final increment widths. Though there are justifications for this technique (Stoica et al., 2000), it remains controversial. It can elicit subjective age model determinations based on what fits better with environmental records especially when not corroborated by other samples. Furthermore, without crossdating, one cannot be sure that growth or geochemical data are truly being driven by a shared environmental signal required for paleo-environmental reconstructions.
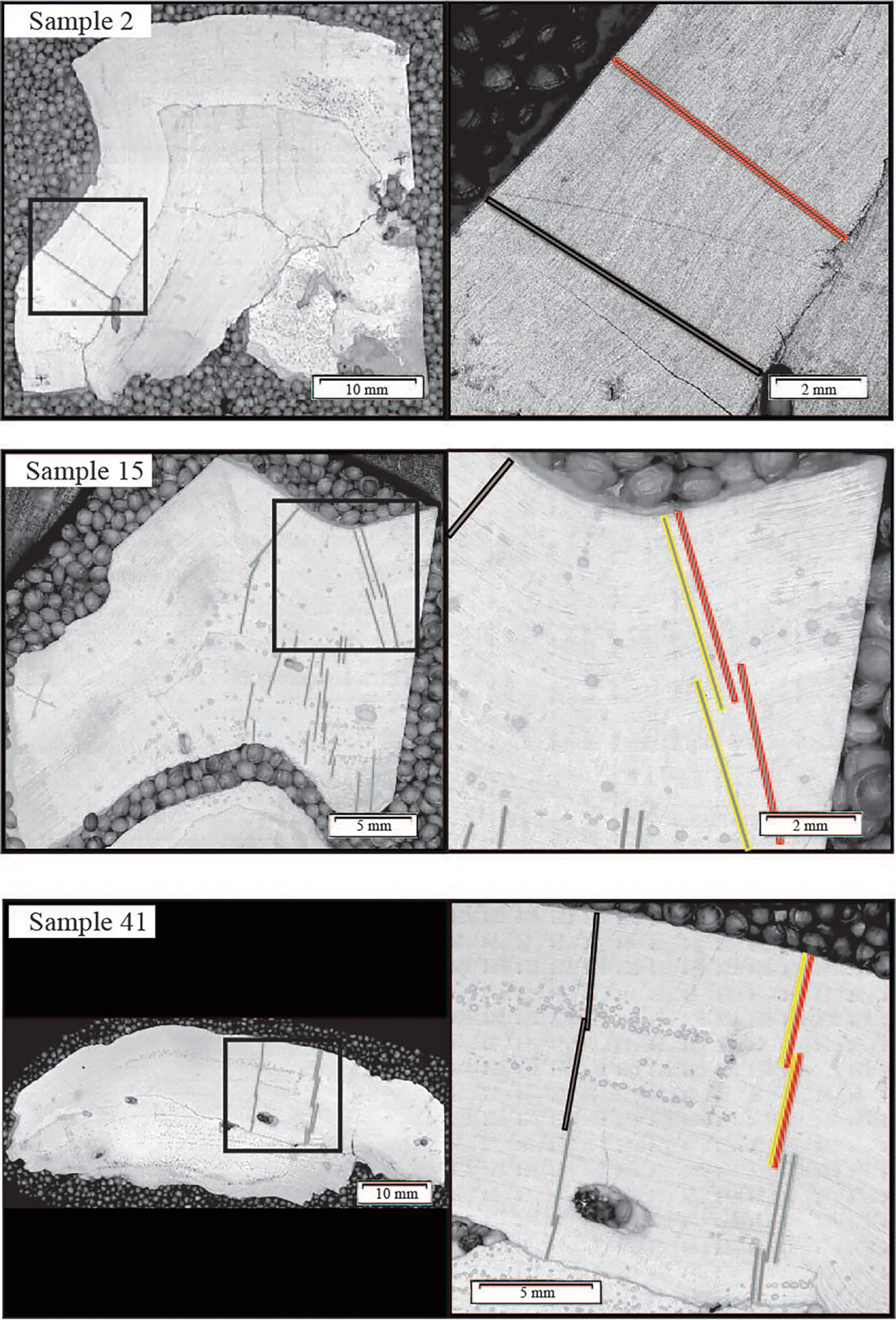
Figure 2 High-resolution sample image and laser transects used in this crossdating study. Transect 1 (Black); Transect 2 (Red); transect after bleaching the sample (Yellow). Boxes in images on the left are magnified in images on the right. Laser measurement paths for samples 2 and 15 end at growth unconformity. Non-highlighted laser measurement paths for sample 41 not included in this study as no other samples could provide corresponding increments that could be used for crossdating.
There is conflicting evidence for whether researchers can use individual algal samples without crossdating for paleo-climatic studies. A study on monthly resolved Mg/Ca ratios of non-crossdated C. compactum samples in the Gulf of Maine found high reproducibility (Hetzinger et al., 2018). Another study near the French islands of St. Pierre and Miquelon used stains and visual identification of non-crossdated C. compactum growth increments and showed high intra- and inter-sample replicability of Mg/Ca and Ba/Ca (Siebert et al., 2020). However, the latter study also showed insignificant inter-sample correlations of growth increment widths, stating that potentially chiton predation pressure and other variables can have a larger impact on inter-sample growth variability. This may be more common in warmer subarctic sites due to the relationship between warmer temperatures and predation (Adey and Steneck, 2001; Williams et al., 2018a; Leclerc et al., 2021). Further, the Siebert et al. (2020) and Hetzinger et al. (2018) studies downsampled Mg/Ca ratio data to a 12-value/year resolution that follows a cyclical pattern every year. Hence, such a timeseries suffers from autocorrelation and inevitably correlates even in the presence of dating errors. Therefore, these findings are insufficient to demonstrate that Mg/Ca data were synchronous between specimens without crossdating. On the other hand, high arctic sites have produced algal growth increments that correspond strongly to sea ice conditions (Leclerc et al., 2021), suggesting that growth is being controlled by a common variable and that growth should be synchronous. Nevertheless, low inter-sample replicability has been found at many high arctic sites (Hetzinger et al., 2019; Leclerc et al., 2021). This may be due to the narrower increments produced in the high Arctic, which may be more likely to be missed and cause dating errors, as has been demonstrated in other biogenic proxies (Black et al., 2016). While accurate identification of growth bands and Mg/Ca cycles depend on experience and sample quality (Black et al., 2016), unconvincing and non-synchronous Mg/Ca and growth increment records of C. compactum support the importance of crossdating methodologies for coralline algal records.
This study compares the replicability of C. compactum annual growth increments before and after crossdating for three samples from Beechey Island, Nunavut, Canada (Figure 3). For the non-crossdated samples, we first report the findings of an inter-observer study to demonstrate the embedded subjectivity in non-crossdated age models. We then determine which preliminary dating methods best prepare timeseries for crossdating (i.e., increment identification or annual Mg/Ca cycles corroborated by visual increments). We report intra- and inter-sample replicability before and after crossdating, in addition to a brief comparison to instrumental and historical sea ice-related records. Results demonstrate that crossdating improves inter- and intra-sample replicability and increases correlations to sea ice records.
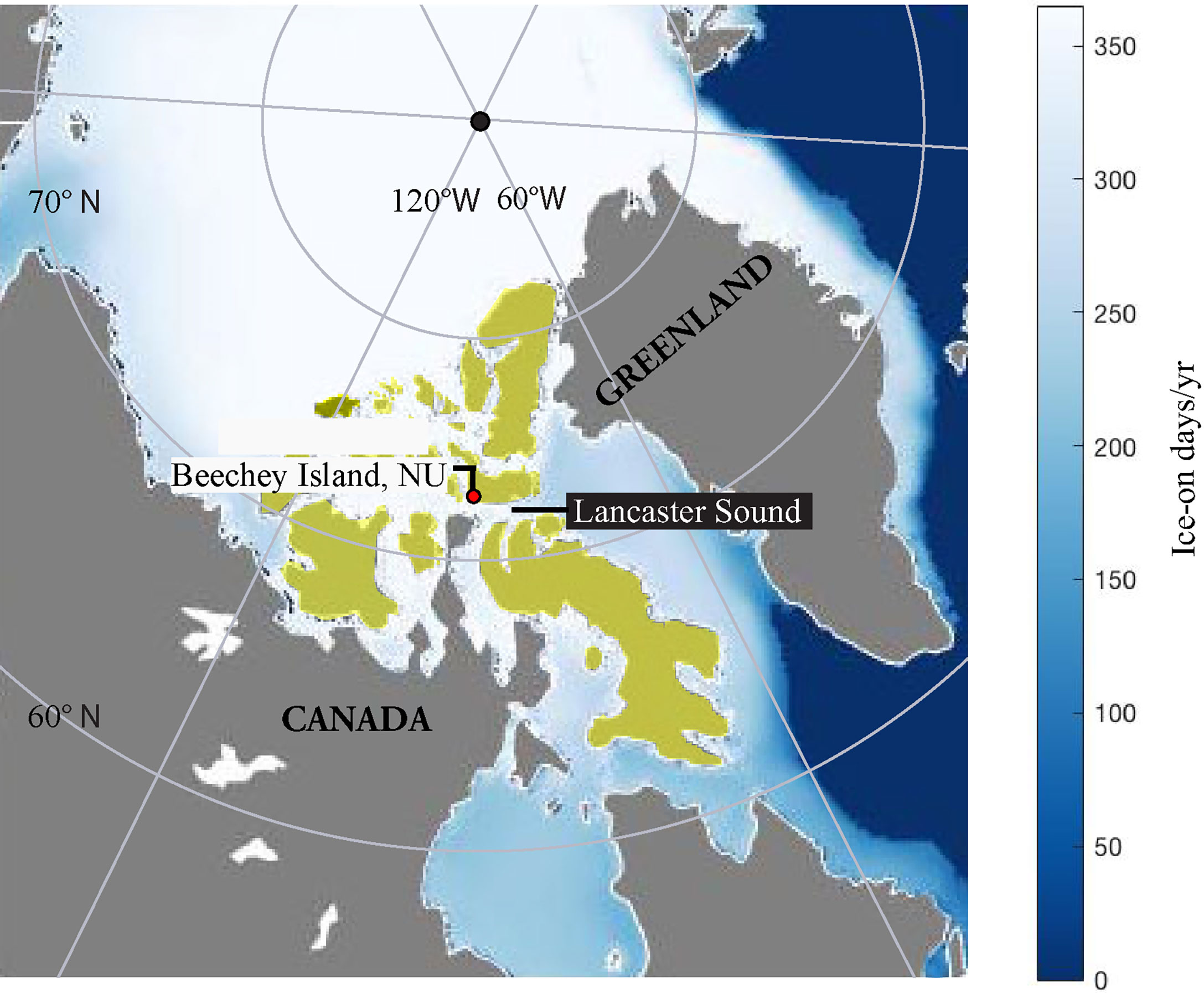
Figure 3 Map of Clathromorphum compactum sampling site (Beechey Island; 74°42’54.46”N 91°47’29.35”W) used for this study. Highlighted yellow region comprises Canadian Arctic Archipelago. Gradient of blue indicates duration of ice cover (ice-on days: <15% SIC) per year (average between 1979-2015). Data sourced from NSIDC daily SIC dataset (25 km2 resolution).
2 Methods
2.1 Collection, Preparation, and Geochemical Analysis
Clathromorphum compactum crusts were collected in 2016 via SCUBA (19-20 meters depth) at a site near Beechey Island in Lancaster Sound, Nunavut, Canada (Figure 3). The three samples used throughout this study (identified throughout as 2, 15, and 41; Figure 3) were prepared into polished thick sections exposing a cross-section of annual growth increments that were then digitally imaged with an Olympus VS-BX reflected light microscope and automated stage using Geo.TS software (Olympus Soft Imaging Systems) and automatically stitched into a photo mosaic. Images were used to digitize laser ablation paths along the growth axis on the Geo.TS software, which were then transferred to a NWR 193 UC laser ablation inductively coupled plasma mass spectrometer (LA-ICP-MS) system coupled with an Agilent 7900 quadrupole mass spectrometer at the University of Toronto’s Earth Science Centre. Measurements of Mg/Ca were obtained by conducting continuous laser ablation line scans at 5 μm/sec speed, a slit size of 10 by 70 μm and 10 Hz pulse rate (for more details see Leclerc et al., 2021). In all samples, Mg/Ca outliers appeared as Mg/Ca spikes when plotted. Outliers were removed outside the lower and upper quartiles of Mg/Ca data (first quartile minus 2 standard deviations and third quartile plus 2 standard deviations, respectively).
For intra-specimen replicability purposes, two laser ablation transects were measured for each sample. Visual increments were difficult to identify on two of the samples (15 and 41) and Mg/Ca cycles from the first two transects running through the entire sample did not resemble one another (Supplementary Figures 1.2a, 1.3a). Accordingly, we applied a previously established bleaching protocol (see Supplement Materials in (Fietzke et al., 2015) to the above two samples to remove organics and test if bleaching would improve the identification of Mg/Ca cycles. Annual Mg/Ca cycles were clearer and visual increments were more visible after bleaching for these samples. Bleached samples 15 and 41 were re-imaged and re-analyzed with LA-ICP-MS following the previously outlined methods, and accordingly, had a total of three measured transects. For our purposes, the bleached samples’ Mg/Ca output was helpful as the calibrated Mg/Ca cycles were synchronous with one of the non-bleached transects and could be used for crossdating. We therefore removed one transect from each sample with low resemblance to the average of the other two transects that had high replicability between them for the rest of analysis (see Supplementary Text 2.1, Supplementary Figures 1.2, 1.3). Low resemblance may be related to growth disturbances from predators (Adey et al., 2013) or C. compactum’s hemispherical shape that allows for distinct areas of the sample to have different access to sunlight (Adey, 1965). As an additional note, Mg/Ca cycles were clearer and visual increments were more visible after bleaching for these samples.
2.2 Inter-Observer Study
In 2019, four of the co-authors (hereafter named observers), who are all experienced in coralline red algae age model construction, were asked to provide age models for two C. compactum samples (2 and 15) to determine the variability of ages assigned to each transect without crossdating. They were provided detailed instructions and calibrated Mg/Ca ratios taken along two transects of samples 2 and 15. The first step of instructions asked them to identify perceived annual Mg/Ca minima. They were allowed to look at Mg/Ca ratios from both transects to determine minima. Second, observers were provided Mg/Ca ratios superimposed on top of high-resolution microscopic images of the samples’ increments and asked to make determinations on the correct position of minima (i.e., a Mg/Ca-visual method). Finally, as a third and last step, observers were given regional satellite sea ice concentration records (1979-2015; 75 km2 resolution) to compare with their age models from the previous step. They were allowed to refine their age models based on this data and the assumption that Mg/Ca ratios and increment widths negatively correlated with sea ice concentrations (SIC), as this variable had been shown to have a strong correlation with growth increments and Mg/Ca at this site (Leclerc et al., 2021). Based on the “confirmation” of cycles by SIC data, this changed their interpretation of other cycles that date prior to the satellite era (pre-1979). Minima were counted for each transect and compared between observers.
2.3 Visual and Mg/Ca-Visual Methods for Intra-Sample Comparison
Two methods used to assess intra-sample coherence were initially tested prior to crossdating (between-sample) to evaluate which was most efficient and effective at producing low intra-sample variability of timeseries. These methods were evaluated for all three specimens (samples 2, 15, 41). We then also evaluated which method, produced the best starting point for inter-sample crossdating by running timeseries through COFECHA. The first method, visual, consisted of matching growth increment widths identified in high-resolution microscopic images of samples from transects (see section 2.1). The second method, Mg/Ca-visual, involved cross-matching growth increments measured based on the distance between two sequential Mg/Ca minima identified through annual LA-ICP-MS-gathered Mg/Ca ratio cycles from the two transects. Increments identified by Mg/Ca cycles were then also cross-referenced to visual growth increments. Finally, for each method, a single timeseries for each sample was produced by averaging annual growth increment timeseries from both transects. For inter-specimen comparison, growth increments were standardized into unitless anomalies by subtracting each increment width measurement by the sample average and dividing the difference by the standard deviation.
2.4 Crossdating
Pointer years (Schweingruber et al., 1990a) – years with particularly thick or narrow increments, or particularly high or low Mg/Ca annual maxima – were identified in each transect of samples 2, 15, and 41. Subsequently, measurement timeseries were cross-matched first within and then between samples. Once these prominent pointer years had been identified, more subtle patterns of synchrony were compared to further refine crossdating. Both annual growth increment widths and Mg/Ca maxima were used for crossdating. This process allowed for the identification of false, locally absent and missed rings (see Supplementary Text 2.2 for lexicon for dendrochronology terms and definitions). After all specimens were crossdated and statistically validated (see section 2.5), the original annual growth increment timeseries (standardized as anomalies) were averaged to reduce individual noise and isolate the climate signal (Fritts, 1976; Schweingruber et al., 1990b).
2.5 Crossdating Validation and Assessment
COFECHA dendrochronology software provided statistical verification of the crossdating among measurement timeseries. Inter-series correlations were calculated for increment widths and Mg/Ca annual maxima, respectively. Prior to calculating these statistics, COFECHA removes low-frequency variability from each measurement timeseries to isolate high-frequency variability suitable for correlation analysis (Holmes, 1983). It also identifies outlier measurements in the output that lie in the outer tails of the distribution of all rings to help identify possible measurement errors due to the misidentification of Mg/Ca minima. Unlike tree rings and bivalves (e.g., Marali and Schöne, 2015), the coralline alga C. compactum does not experience ontogenetic decrease in growth, as it retains stable growth solely driven by environmental variability (Halfar et al., 2007). However, detrending was used here to remove low-frequency variation caused by multi-decadal and centennial environmental trends such as the 20th century reduction of sea ice (Hetzinger et al., 2021). For detrending, a 32-year cubic spline with a 50% frequency response (default setting in COFECHA) was applied to annual growth increment measurements for each of the transects, and growth increments or Mg/Ca values were divided by the value predicted by the spline to yield dimensionless indices. Any remaining autocorrelation was removed using low-order autoregressive models to ensure that all standardized measurement time series were serially independent and thus meet the assumptions of correlation analysis (Holmes, 1983). Correlations between each standardized set of measurements and the average of all others were broken into 50-year segments overlapping by 25 years. If a dating error had occurred, correlations would drop noticeably in the years preceding the error. Thus, dividing measurements into segments provides a way to narrow the location of dating errors in the dataset. Non-significant correlations (p > 0.01) flagged by COFECHA as possible dating errors (e.g., locally absent or false ring) were manually re-evaluated using the sample images and Mg/Ca measurement series and corrected (e.g., by adding or removing a ring boundary) where a dating error could be confirmed.
2.6 Master Chronology and Two-Sample Master Chronologies
Once all three samples’ (i.e., 2, 15, and 41) chronologies (n=6 transects) were crossdated and validated with COFECHA, raw growth increment and calibrated annual Mg/Ca maxima for each transect (n=2 transects per sample) were individually averaged to produce samples averages (n=3 sample averages). Sample averages were converted into anomalies (see section 2.1) and averaged between samples to produce a Master chronology (Figures 8, 9).
The strength of crossdating was assessed with 49-year running correlations (Figures 6, 7). Each detrended sample timeseries was evaluated in comparison to the average of the two other crossdated specimens (identified as two-sample master chronology). For example, crossdated timeseries of sample 2 was correlated to the two-sample master chronology of samples 15 and 41 in Figures 6D, 7D.
2.7 Sea Ice-Related Records
Non-crossdated timeseries and the crossdated chronology were compared to different sea ice-related records. Records used include: 1) Atlantic Multi-decadal Oscillation (AMO) 10-year running mean smoothed from the Kaplan SST V2, calculated at NOAA PSL1 (http://www.psl.noaa.gov/data/timeseries/AMO/); 2) Annual sea ice concentrations (SIC) for summer averages (May-Oct) were extracted from the gridded National Snow & Ice Data Center (NSIDC) Sea ice Concentration Data Set (Version 3) (https://nsidc.org/data/g02202; (Peng et al., 2013; Meier et al., 2017) for a 75 km2 area around the Beechey Island algal collection site (for more information on procedure see (Leclerc et al., 2021); 3) Long-term annual summer (May-Oct) sea ice concentration were extracted from the HadISST record (1° resolution) from KNMI Climate Explorer tool (https://climexp.knmi.nl) and; 4) Winter (Jan-Apr) Labrador Sea Ice Export record was extracted? from Hill and Jones (1990).
3 Results
3.1 Inter-Observer Study
When given the same data (high-resolution sample images and Mg/Ca ratios), individual observers provided estimates of differing timeseries lengths. The range of years identified by each observer diminished with each step of age model construction (Table 1). After reviewing calibrated Mg/Ca ratios, the difference in record length reported among observers was 18-62 years; equivalent to 8-29% of the entire record length. This difference reduced to 15-22 years after adding the visual interpretation of increments in high-resolution images, accounting for 7-14% of the entire record length. Finally, after refining age models based on regional satellite sea ice concentration records, the difference in length was further reduced to 8-19 years, corresponding to 5-9% of the entire length of records. The results of the inter-observer study highlight that a degree of subjectivity is imbedded in non-crossdated age model interpretation.
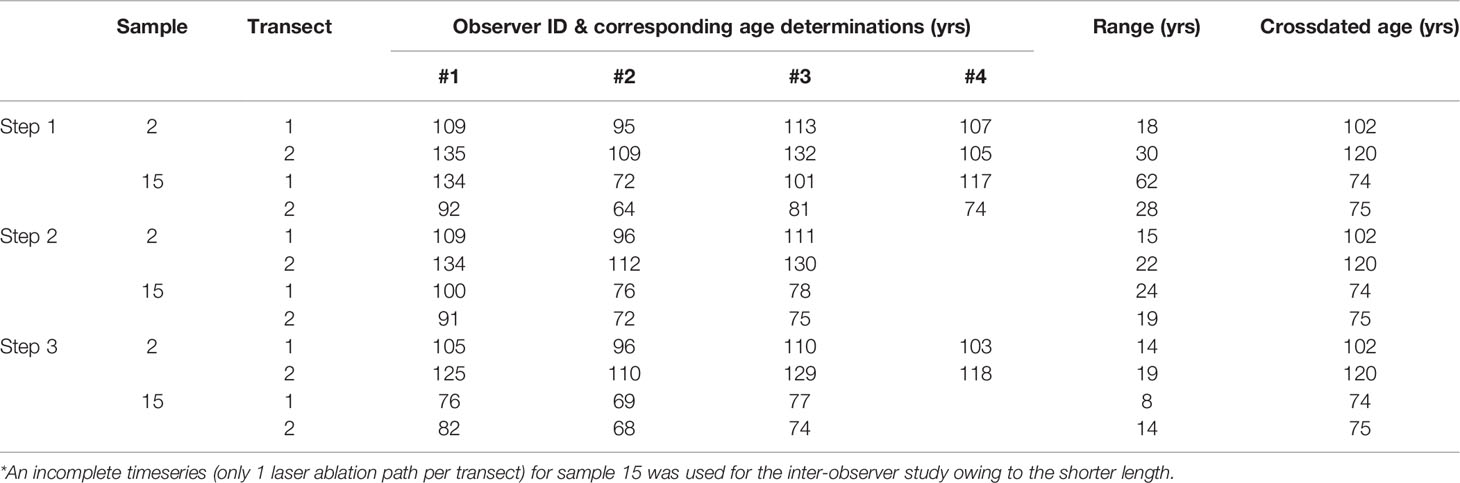
Table 1 Results of inter-observer study reporting number of years identified in each transect after each step outlined in methods section 2.2.
3.2 Intra- and Inter-Sample Replicability of Growth Increments Prior to Crossdating
Inter-series correlations were positive and statistically significant within samples, indicating strong transect agreement for both methods (visual and Mg/Ca-visual) (Table 2). Inter-series correlations were much lower when calculated among samples for both methods. However, the Mg/Ca-visual method produced generally higher intra- and inter-sample inter-series correlations. (Table 2 and Figure 4) Further, the correlation between satellite Sea Ice Concentrations (SIC) and the visual mean chronology was less strong than that between SIC and the Mg/Ca-visual mean chronology (Table 2). Accordingly, the subsequent crossdating process was continued solely with the Mg/Ca-visual chronologies.
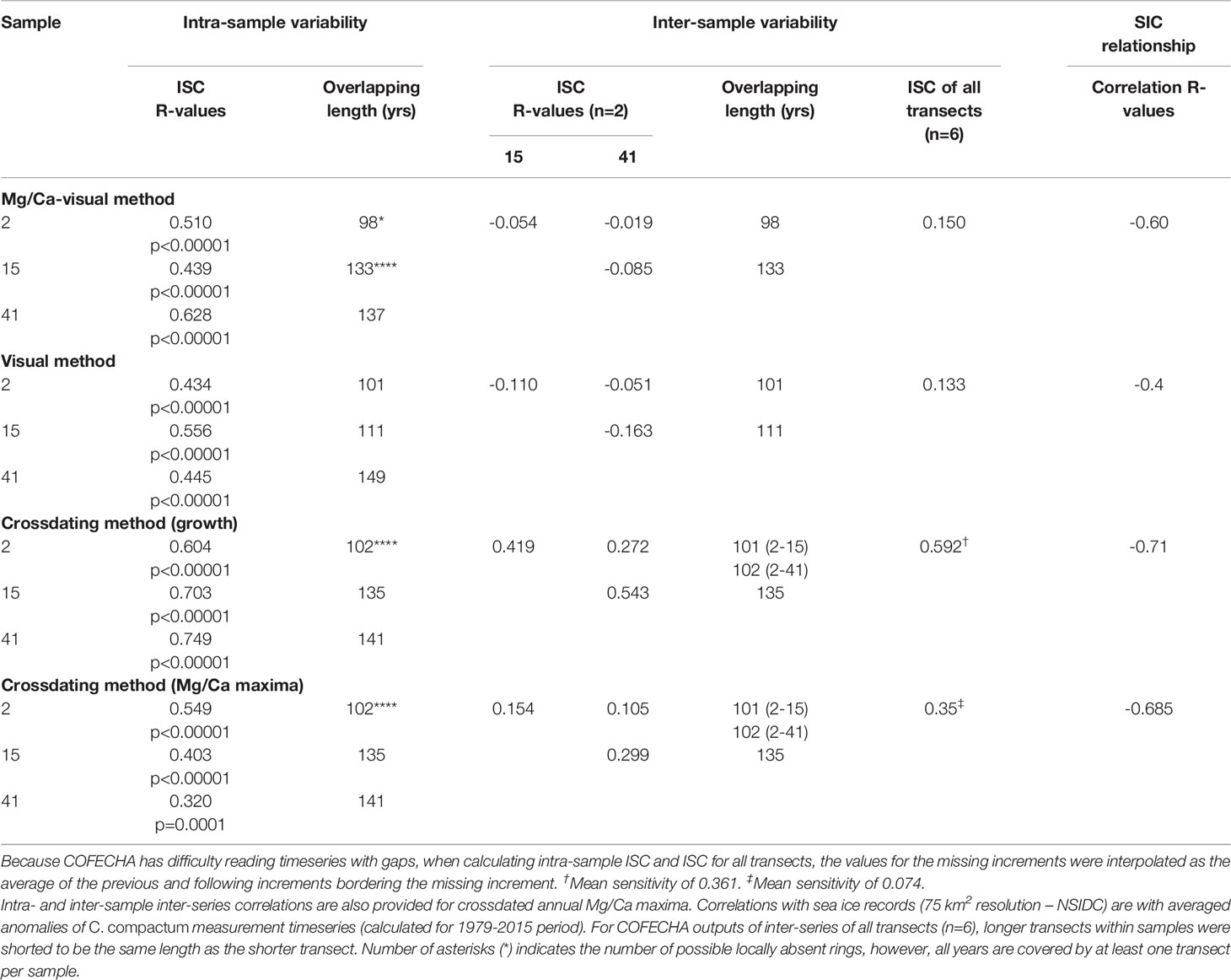
Table 2 Comparison of intra- and inter-sample COFECHA calculated inter-series correlation (i.e., ISC: 50-year windows) of annual growth increment widths.
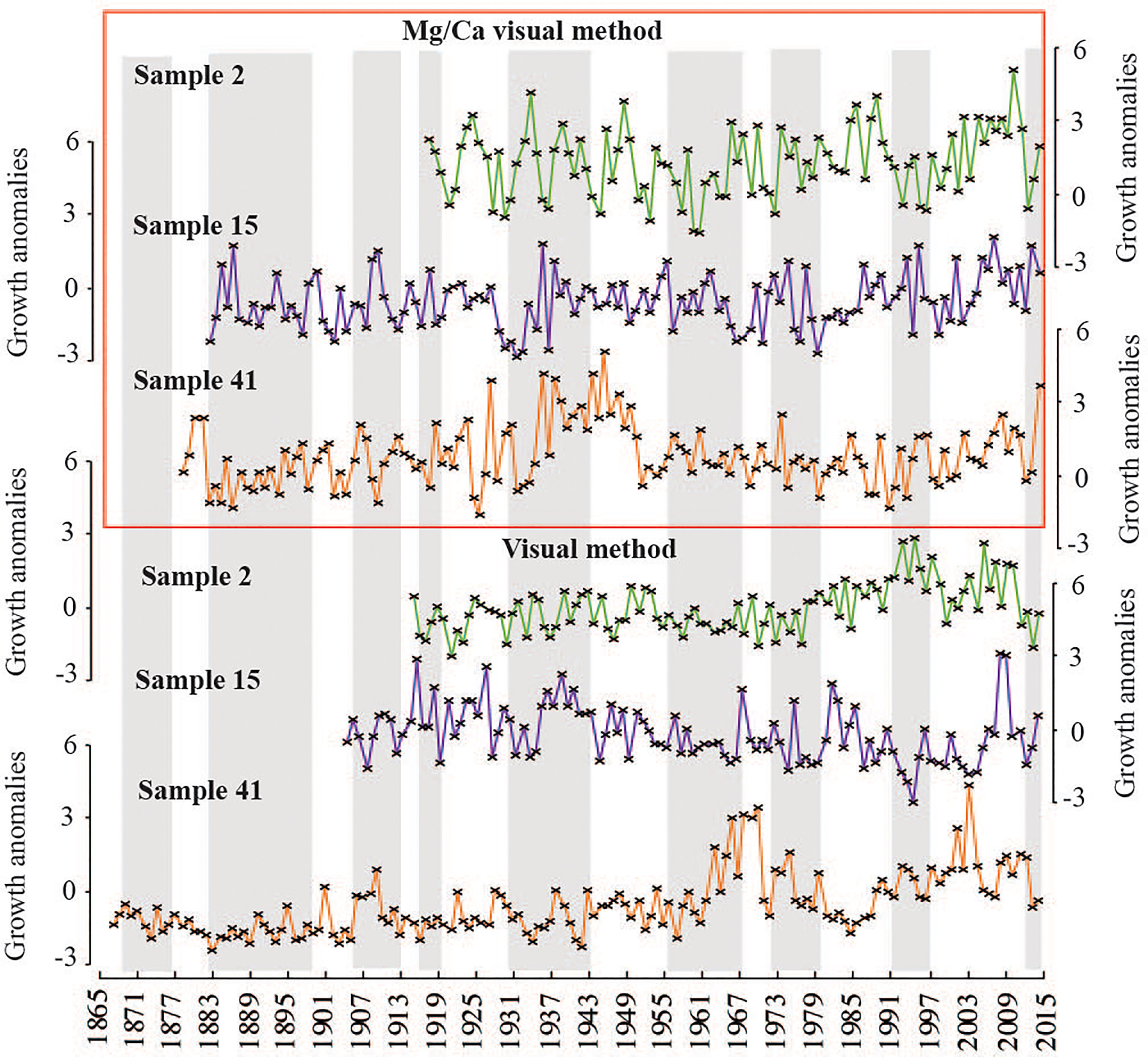
Figure 4 Growth anomalies of non-crossdated annual growth increments for all samples. Only sample averages shown here. All yielded significant intra-sample correlations (Table 2). Timeseries developed with Mg/Ca-visual methods (top panel – red box) and visual methods (lower panel) shown.
3.3 After Crossdating
Intra-sample and inter-sample inter-series correlations remained positive and significant throughout the length of the growth increment record (p<0.05; 49-year windows) and were consistently higher compared to non-crossdated timeseries (Table 2; Figures 5, 6). Each specimen correlated significantly to the two-sample master chronologies (the average of the two other samples) (Figures 6D–F). However, steep drops in inter-sample correlation were observed around 1946 (window: 1921-1970) and 1931 (window 1917-1956), suggesting two brief periods of reduced growth synchrony among samples (Figures 6E–G). Annual Mg/Ca maxima contain much more individual-level variability than increment widths and yielded lower inter-series correlations (Table 2; Figures 7D–F). The positive inter-series correlations (Table 2), while weak, hint at a common environmental signal affecting interannual variability, but more samples may be required to isolate that common Mg/Ca signal (Figures 7A–C). Further, detrended Mg/Ca maxima and increment widths (averages between transects) significantly correlate to one another within each sample (2: r=0.55, p<0.00001; 15: r=0.45, p<0.00001; and 41: r=0.60, p<0.00001), showing that some environmental drivers of growth and Mg/Ca variability are shared.
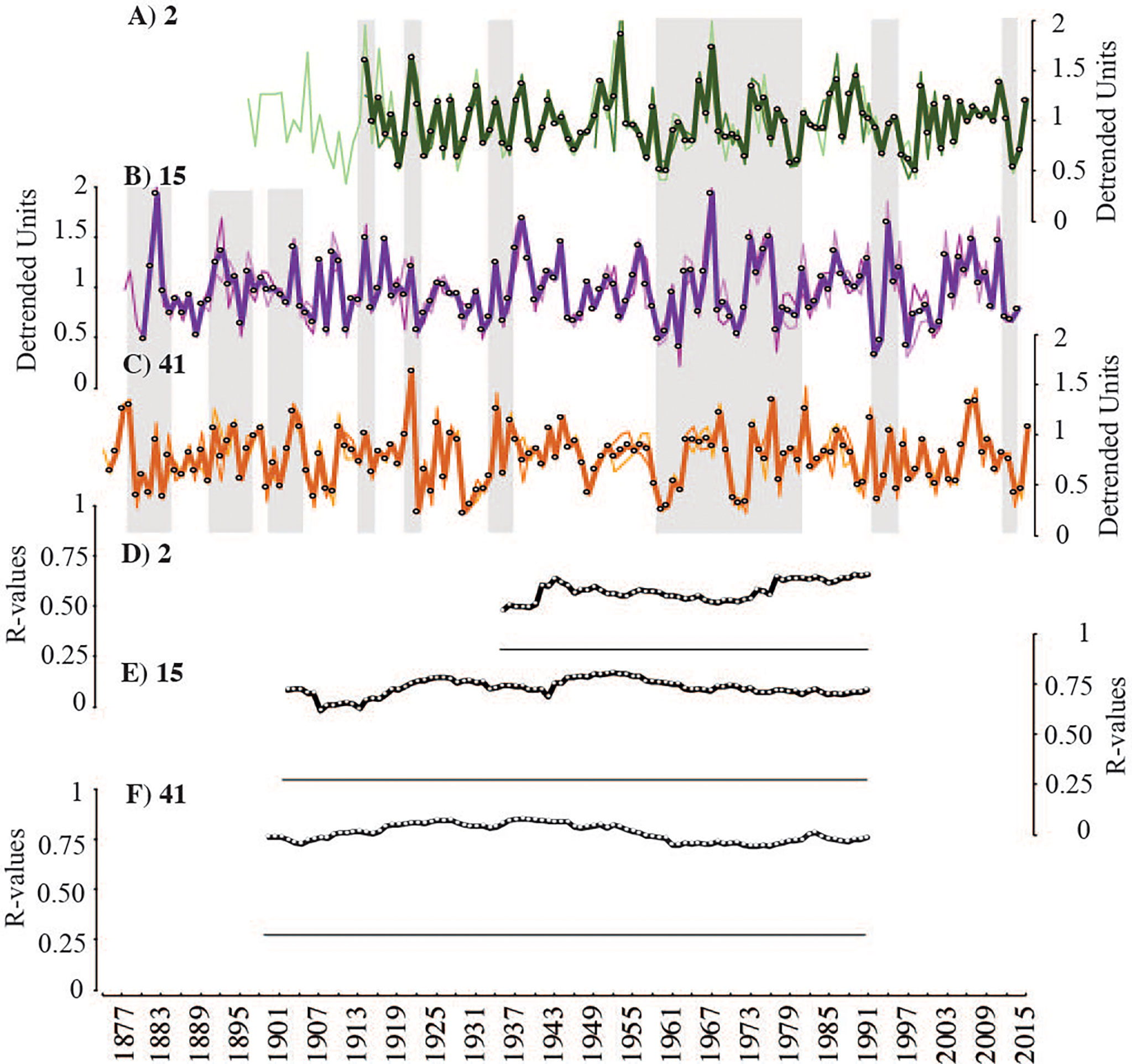
Figure 5 All crossdated growth increment data presented as detrended growth timeseries (A–C). Data from individual transects are plotted as thin lighter lines and sample averages as thicker darker lines. Intra-sample replicability presented as plotted R-values of running correlations (49-year windows) (D–F). Flat black lines represent threshold of significance (p < 0.05). All samples have significant intra-sample correlations throughout timeseries lengths.
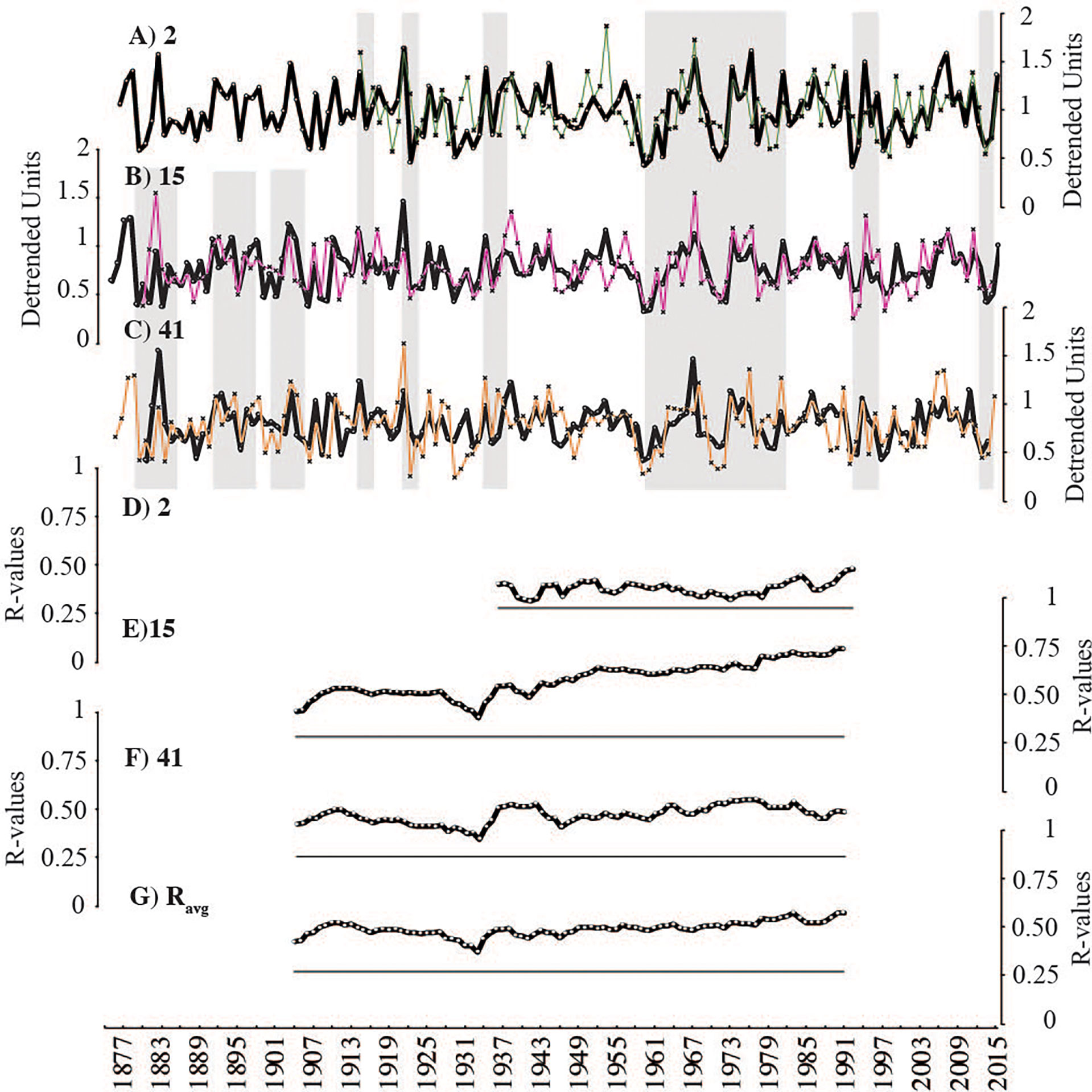
Figure 6 Individual sample timeseries (thin coloured lines A–C) compared to average of all other sample-mean series (black). Grey vertical bars highlight periods of strong coherence. Running correlations between samples presented as plotted R-values of running correlations (49-year windows) (D–F). Flat black lines represent threshold of significance (p < 0.05). Average inter-series correlation between all specimens (Ravg) plotted in lowest panel (G).
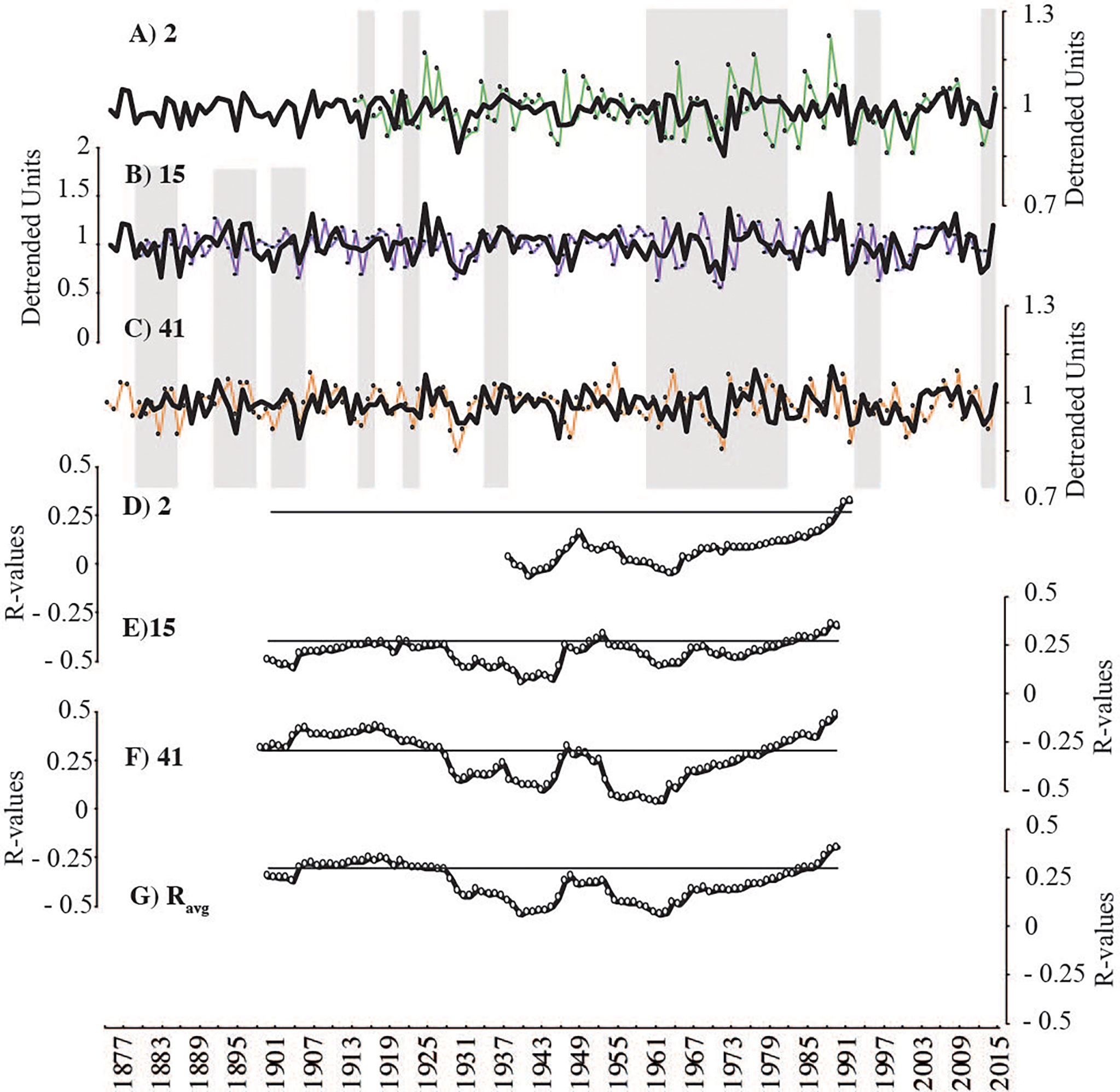
Figure 7 Individual detrended crossdated chronologies for annual Mg/Ca maxima (thin coloured lines A–C) compared to the averaged timeseries of the other two crossdated specimens (i.e., two-sample master chronology: black). Grey vertical bars show highlighted periods of synchrony. Running correlations between samples presented as plotted R-values of running correlations (49-year windows) (D–F). Flat black lines represent threshold of significance (p < 0.05). Average inter-series correlation between all specimens (Ravg) plotted in lowest panel (G).
Correlation coefficients between sample averages and summer sea ice concentrations were significant for only 2 specimens prior to crossdating (Figure 8; left panel), but significant for all 3 specimens following crossdating. Crossdating also produced a stronger correlation between sea ice concentrations and the master chronology (Figure 8D). Notably, crossdating improved correlations between individual timeseries and SIC for all samples except sample 2, where correlations remained similar (non-crossdated: r = -0.63; crossdated: r= -0.62). Averaging two samples slightly improved correlations to SICSUMMER. Average correlation of sea ice concentrations correlated with individual timeseries: r = -0.591; and correlated with the averaged timeseries of two specimens: r = -0.599. The highest correlation between the average of two samples and SIC was achieved with the average of sample 2 and 41 (r = -0.701) but was still marginally lower than the correlation coefficient yielded with three combined sample averages (r = -0.716).
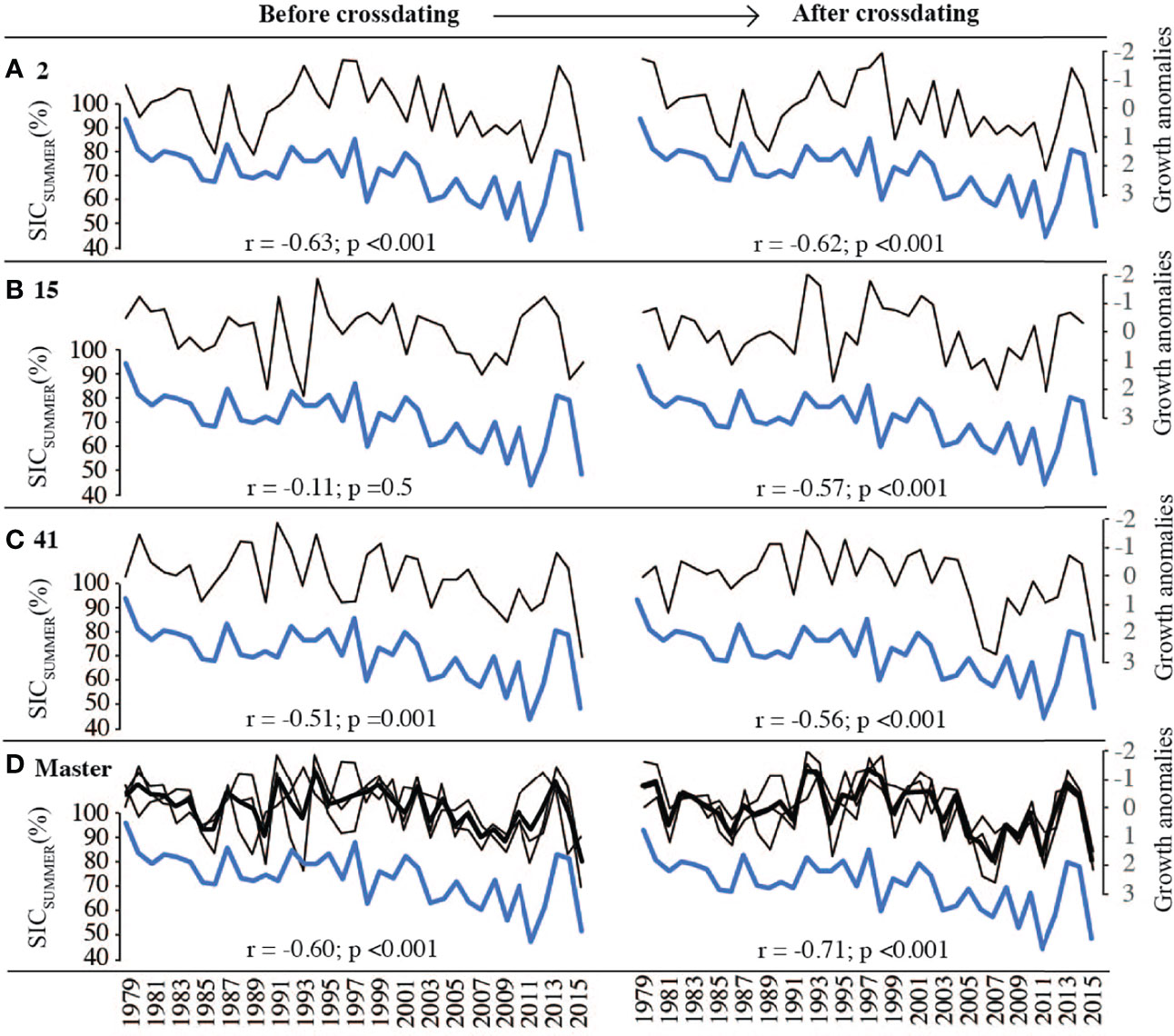
Figure 8 Relationship between regional summer sea ice concentrations (SIC; blue) and standardized algal growth anomalies (averaged transects for each sample; A–C black) before (left) and after (right) crossdating. Growth anomalies inversed on y-axis due to hypothesized negative relationship between growth and sea ice cover. Master chronology of all averaged timeseries presented in black (D). All correlation coefficients between individual timeseries/master chronology and SIC reported as R-values.
The master chronology was downsampled to a 10-year running mean to test correlation to AMO; a smoothed 10-year resolution record; and showed a significant correlation of r=0.4 (n=130 years, p< 0.00001; Figure 9). At an annual resolution, correlations with winter Labrador Sea Ice Export (LSIE) and HadISST sea ice concentration records were significant but low (p<0.01) (Figure 9). However, when downsampled to a 5-year running mean, correlations became much more significant (p<0.00001). Notably, correlations with LSIE were strongest in the early record, especially if a 1-year lag was imposed to LSIE) and less so after 1958.
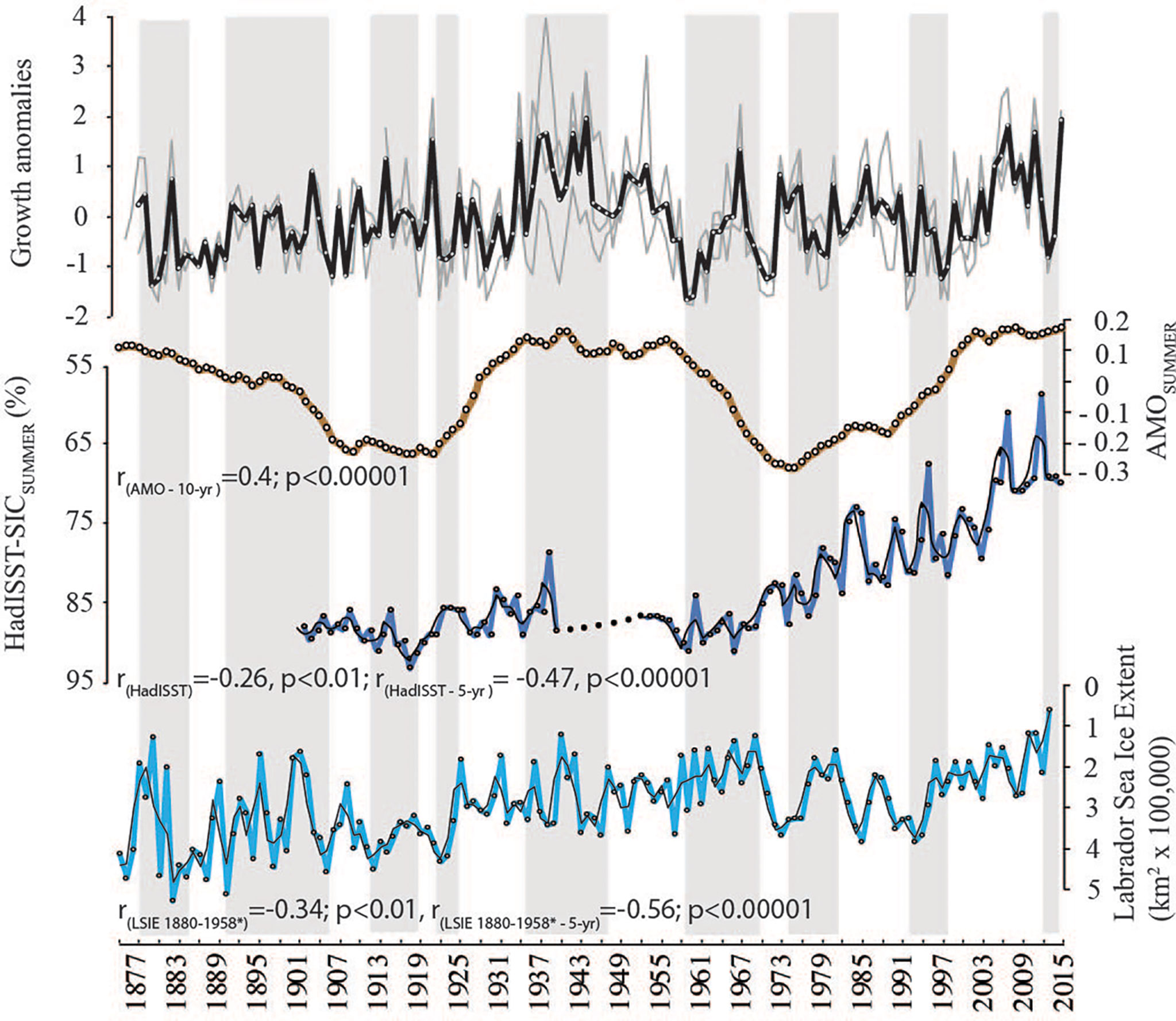
Figure 9 Relationship between crossdated master chronology (thick black line) and long-term sea ice-related records. Algal growth increments standardized as anomalies. Individual algal sample averages (grey); AMO: (May-October; brown); HadISST (data gathered from KNMI Climate Explorer 1° spatial resolution; 79-80°N to 91-92° W); dark blue, missing years shown in dotted black line); Labrador Sea Ice Extent (i.e., LSIE; Jan-Apr average; *1-year lag (Hill and Jones, 1990). Light black line in LSIE and HadISST records are 2-year running means.
4 Discussion
Our inter-observer study showed that non-crossdating methods are prone to integrating dating errors. Observers given the same high-resolution sample images and Mg/Ca ratios produced records with varying lengths for samples 2 and 15 between ±5 years/century and ±9.3 years/century, respectively. Further, comparison of two non-crossdating age model methods (i.e., Mg/Ca-visual, or visual methods) showed that both methods had a degree of replicability within samples, but correlations between algal specimens were weak and not statistically significant. A previous study found significant correlation between growth increments and satellite sea ice concentrations at 7 arctic sites (Leclerc et al., 2021), suggesting a common driver of growth and that crossdating methods may therefore be helpful to reduce inter-sample variability. The aforementioned study produced short chronologies (n = 37 years), that likely suffered from some age model misinterpretation, which was addressed by down-sampling data to a 5-year average resolution to reduce the effect of misidentified or incorrectly measured increments. However, smoothing filters dampen interannual patterns of growth variability, add autocorrelation, and thus hamper the ability to produce annually resolved timeseries, limiting their usefulness for climate model calibration and reconstruction. A considerable concern for confident and accurate dating of longer algal timeseries is that errors rates propagate with time. When producing multi-century timeseries, an error rate of ± 5 years/century could compound to a total error of ±10 years after 200 years, and ±30 years after 600 years. Crossdated timeseries are not limited by propagating errors, have greater intra- and inter-sample replicability, and produce master chronologies that better isolate the climate signal, especially at interannual timescales.
Crossdating was also helpful in diagnosing locally absent years and lags, and in interpreting reasons for lower intra-sample replicability. Several locally absent years were identified in the crossdated timeseries of sample 2. Sample 2 does not have any gaps in the averaged timeseries from its two transects, but crossdating of Mg/Ca cycles against other samples showed that both transects had locally absent year(s), some of which were present in one transect and not the other (Supplementary Figure 1.1). Furthermore, sample 15 had a locally absent increment for the most recent year of the record (2015), and therefore the entire timeseries was ‘lagged’ by one year. This 1-year lag was caused by a growth unconformity (i.e., growth gaps) mistaken initially for the meristem, made clear when crossdating and by superimposing Mg/Ca cycles over the high-resolution image. Accordingly, lags between coralline algae records and environmental variables in previous studies using one sample per site or without crossdating, may be spurious due to a missed or falsely added ring (e.g., (Hou et al., 2018; Hetzinger et al., 2019; Leclerc et al., 2021). Additionally, we found noticeably lower running correlation values for transects within sample 2, and excluded measurement timeseries in samples 15 and 41 (see section 2.1, Figure 3). We excluded these two measurement timeseries from subsequent crossdating analyses due to their poor coherence to the broader sample set. Like the transects from sample 2, removed transects were spatially further apart than the two transects retained for crossdating, had more neighboring conceptacles and bioerosion, followed a slightly different growth axis, and thus may have had disparate access to sunlight perhaps due to irregular shading from macroalgae overgrowth (Williams et al., 2018b). Accordingly, crossdating was able to identify: 1) transects with non-synchronized growth, likely due to transects that tracked periods of irregular or interrupted growth patterns, to eliminate from the master chronology; 2) locally absent increments difficult to interpret when only working with one sample, and; 3) periods of lower intra-sample covariability.
Our findings mirror results of other crossdated proxies that showed that non-crossdated mean dating series with interpretation errors correlated less strongly with environmental variables than crossdated chronologies (Black et al., 2016). Our crossdated and pre-crossdated age models were not refined, biased or forced to correlate to environmental data. On the contrary, a published non-crossdated mean dating series (n = 37 years) of the same Beechey samples presented slightly higher correlations to SIC (Leclerc et al., 2021: r = -0.74); r = -0.716: this study) however, low inter-series correlations demonstrated that growth was not synchronized between samples likely due to age model construction being biased towards satellite SIC records. The results of our study showed that high and significant relationships between growth and SIC can still be achieved without validating age models through environmental record comparison.
The Beechey Island master chronology shows a correlation (decadal resolution) with Atlantic Multi-decadal Oscillation Index a climate pattern that controls sea surface temperature and sea ice variability (Miles et al., 2014). This correlation is particularly strong in the mid-record when the positive phase of AMO is equivalent to warmer temperatures, longer growing seasons, and thus, larger growth increments (Figure 9). Our results are similar to previous findings that showed that growth increments (Halfar et al., 2011) and Ba/Ca ratios (Chan et al., 2017) in C. compactum samples collected from the coast of Newfoundland and the Gulf of St. Lawrence also correlated to AMO. Furthermore, the crossdated Beechey Island record had significant correlations to the HadISST sea ice concentration record and the early sections of the historical Labrador Sea Ice Export record (1880-1958 at a 1-year lag). The loss of correlation in recent decades may be due to the rapid decline of Labrador’s sea ice extent compared to the Canadian Arctic Archipelago’s, which has had a slower decline (Cavalieri and Parkinson, 2012) (Figure 9). These findings suggest long-term algal growth responds to seasonal sea ice conditions, and limited dating errors of the Beechey Island crossdated master chronology, as propagating dating errors would significantly skew correlations.
An important consideration is that the number of replicates crossdated here is relatively low. For tree-ring crossdating, typically at least 2 replicate cores from 20 trees (40 cores total) are crossdated to ensure that all locally absent and false rings are identified (Fritts, 1976). For C. compactum-based crossdating, the high analytical and acquisition costs and low yields of high-quality specimens from collection sites (i.e., samples with uninterrupted regular growth layers, spared of predator grazing, and with a low concentration of conceptacles) are significant limitations to acquiring a large sample size for crossdating. However, if effective crossdating can be achieved with a few analyzed algal samples, this approach represents a great opportunity to improve the quality and reliability of algal paleo-environmental records. Furthermore, in the case of the oldest sample (sample 41), the earliest increments did not overlap in time with any of the other samples, and only had intra-sample replicates. Accordingly, these un-crossdated early sections should cautiously be used for paleo-environmental reconstruction. While a high-quality older specimen is highly valuable for reconstruction purposes, it is also rarer, and unlikely to have external replicates. We recognize this to be a limitation of all long-term chronologies.
Inter-series correlations between C. compactum growth timeseries (n=6) are comparable to those attained from other crossdated biogenic proxies but remain lower than timeseries from some trees, fish otoliths, and bivalves (Tables 2, 3). A possible reason for C. compactum’s lower replicability is its very narrow increments (20-30 μm) formed in the high arctic during years with a lot of sea ice. Most modifications to chronologies during the crossdating process were made on ambiguous sections of growth or Mg/Ca cycles and tended to be thinner increments easily missed, a feature that may be common among all C. compactum chronologies in the high Arctic. We see a similar issue with Pacific geoduck bivalves that are systematically underaged due to narrow increments difficult to identify during slow growing periods, especially in older specimens (Black et al., 2008b). Therefore, it is important to acknowledge that narrow increments from high arctic C. compactum specimens may introduce some uncertainty to records.
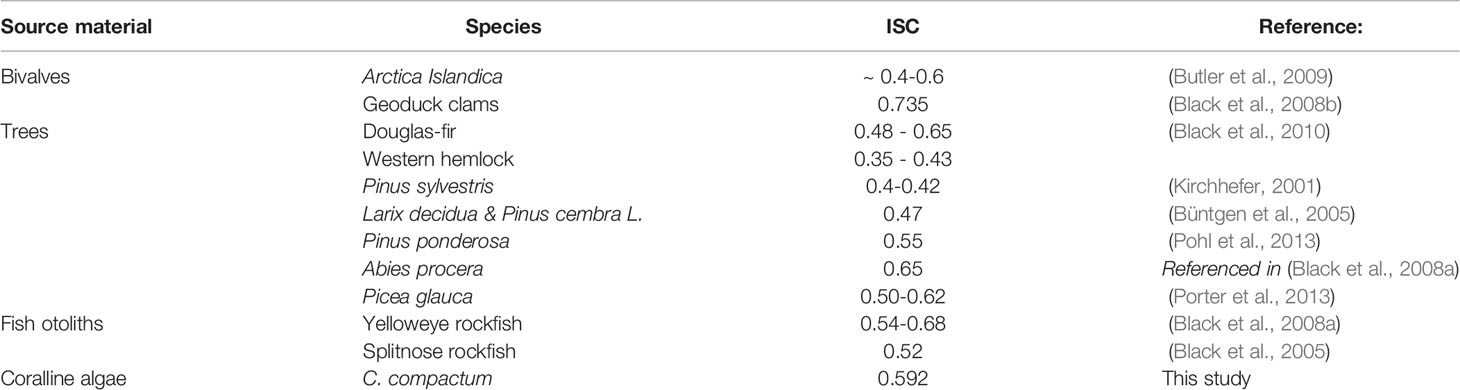
Table 3 Inter-series correlations (ISC) of inter-sample growth replicability compared between other crossdated biogenic proxies.
4.1 Outlooks and Recommendations
As a summarizing recommendation for future studies, crossdating should continue to be incorporated so algal growth data can accurately be used to reconstruct past sea ice conditions and other environmental variables. Doing so will increase accuracy and reliability of algal chronologies and enhance signal-to-noise ratio, enabling well-dated paleo-environmental reconstructions. For annual algal records of carbon isotopes (δ13C), magnesium to calcium ratios (Mg/Ca), barium to calcium ratios (Ba/Ca), and annual algal growth increments, crossdating at least two C. compactum specimens can reduce dating errors. In southern regions of the species distribution, where individual growth is more variable, Mg/Ca ratios may be more suitable for crossdating if more than three specimens are analyzed. If a single specimen produces visibly distinct growth boundaries and Mg/Ca cycles, while others are less defined, the clear specimens can be utilized as the base for the iterative crossdating process. When interpreting poorly defined growth increments and Mg/Ca cycles, analysts can crossdate sections before and after poorly defined increments against the master chronology to determine the presence of any missing or false rings. Analysts can also detrend data to extract intra- and inter-sample variability and reduce the potentially spurious effect of long-term trends on correlations. Once samples are crossdated, original or normalized growth increment widths (i.e., anomalies) can be averaged, and this master chronology used to assess the relationship with environmental variables. Given the difficulty of visually identifying narrow increments in some samples, optical improvements would be another significant methodological advancement. The future application of visual increment enhancements, such as Mutvei solution staining (Siebert et al., 2020) applied to high arctic specimens in conjunction with crossdating, could be considered for future study.
5 Conclusion
Joining a growing body of sclerochronologists (Helama et al., 2006; Black et al., 2016), we advocate for crossdating in age model construction of biogenic increment-yielding paleo-environmental archives. This proof-of-concept study reveals human bias in non-crossdated C. compactum timeseries and highlights the value of crossdating. Here we demonstrate that crossdating improves intra- and inter-sample replicability and enhances the relationship between algal growth and sea ice records. Growth synchrony between samples, and strong correlations to regional sea ice conditions highlights the strong and shared environmental control of sea ice on algal growth. While the application of crossdating methods to algal data is a lengthy process, it is also necessary to form a network of well-dated proxy records in order to understand the spatial and temporal variability of sea ice behavior, which can also be incorporated into modelling predictions.
Data Availability Statement
The original contributions presented in the study are included in the article/Supplementary Material. Further inquiries can be directed to the corresponding author.
Author Contributions
Data Curation: NL and AT. Formal Analysis: NL, JH, SH, and MZ. Funding acquisition: JH and NL. Methodology: NL, TP and BB. Project administration: NL and JH. Supervision: JH. Writing: NL. Review and editing: NL, JH, SH, TP, MZ and BB. All authors contributed to the article and approved the submitted version.
Funding
NSERC-Discovery (1303409; J.H.); NSERC-CGS-D (CGSD3-518838 – 2018; NL).
Conflict of Interest
The authors declare that the research was conducted in the absence of any commercial or financial relationships that could be construed as a potential conflict of interest.
Publisher’s Note
All claims expressed in this article are solely those of the authors and do not necessarily represent those of their affiliated organizations, or those of the publisher, the editors and the reviewers. Any product that may be evaluated in this article, or claim that may be made by its manufacturer, is not guaranteed or endorsed by the publisher.
Acknowledgments
We acknowledge the various institutions, individuals, and agencies that have supported this work. The University of Toronto Earth Sciences Department and the Earth Sciences Lab at the University of Toronto Mississauga for space and resources. Current and Alumni climate geology research group members: Alessio Scurci, Minoli Dias, and Phoebe Chan for support and problem solving. Dr. Max Friesen and Dr. Sarah Finkelstein for feedback, insights and questions. Reviewers for encouraging praise and constructive feedback to make this article accessible to multi-disciplinary researchers. NSERC for continued funding. Annelotte van der Linden for preliminary data processing.
Supplementary Material
The Supplementary Material for this article can be found online at: https://www.frontiersin.org/articles/10.3389/fmars.2022.923088/full#supplementary-material
References
Adey W. (1965). The Genus Clathromorphum (Corallinaceae) in the Gulf of Maine. Hydrobiologia 26 (3–4), 539–573. doi: 10.1007/BF00045545
Adey W. H., Halfar J., Williams B. (2013). The Coralline Genus Clathromorphum Foslie Emend. Adey: Biological, Physiological, and Ecological Factors Controlling Carbonate Production in an Arctic-Subarctic Climate Archive (Washington DC: Smithsonian Institution Scholarly Press).
Adey W. H., Steneck R. S. (2001). Thermogeography Over Time Creates Biogeographic Regions: A Temperature/Space/Time-Integrated Model and an Abundance-Weighted Test for Benthic Marine Algae. J. Phycol. 37 (5), 677–698. doi: 10.1046/j.1529-8817.2001.00176.x
Ansell A. D. (1968). The Rate of Growth of the Hard Clam Mercenaria Mercenarial) Throughout the Geographical Range. ICES J. Mar. Sci. 31 (3), 364–409. doi: 10.1093/icesjms/31.3.364
Ballesta-Artero I., Witbaard R., Carroll M. L., van der Meer J. (2017). Environmental Factors Regulating Gaping Activity of the Bivalve Arctica Islandica in Northern Norway. Mar. Biol. 164 (5), 1–15. doi: 10.1007/s00227-017-3144-7
Black B. A., Andersson C., Butler P. G., Carroll M. L., DeLong K. L., Reynolds D. J., et al. (2019). The Revolution of Crossdating in Marine Palaeoecology and Palaeoclimatology. Biol. Lett. 15 (1), 20180665. doi: 10.1098/rsbl.2018.0665
Black B. A., Boehlert G. W., Yoklavich M. M. (2005). Using Tree-Ring Crossdating Techniques to Validate Annual Growth Increments in Long-Lived Fishes. Can. J. Fish. Aquat. Sci. 62 (10), 2277–2284. doi: 10.1139/F05-142
Black B. A., Boehlert G. W., Yoklavich M. M. (2008a). Establishing Climate-Growth Relationships for Yelloweye Rockfish (Sebastes Ruberrimus) in the Northeast Pacific Using a Dendrochronological Approach. Fish. Oceanogr. 17 (5), 368–379. doi: 10.1111/j.1365-2419.2008.00484.x
Black B. A., Gillespie D. C., MacLellan S. E., Hand C. M. (2008b). Establishing Highly Accurate Production-Age Data Using the Tree-Ring Technique of Crossdating: A Case Study for Pacific Geoduck (Panopea Abrupta). Can. J. Fish. Aquat. Sci. 65 (12), 2572–2578. doi: 10.1139/F08-158
Black B. A., Griffin D., van der Sleen P., Wanamaker A. D., Speer J. H., Frank D. C., et al. (2016). The Value of Crossdating to Retain High-Frequency Variability, Climate Signals, and Extreme Events in Environmental Proxies. Glob. Change Biol. 22 (7), 2582–2595. doi: 10.1111/gcb.13256
Black B. A., Shaw D. C., Stone J. K. (2010). Impacts of Swiss Needle Cast on Overstory Douglas-Fir Forests of the Western Oregon Coast Range. For. Ecol. Manage. 259 (8), 1673–1680. doi: 10.1016/J.FORECO.2010.01.047
Büntgen U., Esper J., Frank D. C., Nicolussi K., Schmidhalter M. (2005). A 1052-Year Tree-Ring Proxy for Alpine Summer Temperatures. Clim. Dynam. 25 (2–3), 141–153. doi: 10.1007/s00382-005-0028-1
Butler P. G., Richardson C. A., Scourse J. D., Wanamaker A. D., Shammon T. M., Bennell J. D. (2010). Marine Climate in the Irish Sea: Analysis of a 489-Year Marine Master Chronology Derived From Growth Increments in the Shell of the Clam Arctica Islandica. Quaternary Sci. Rev. 29 (13–14), 1614–1632. doi: 10.1016/j.quascirev.2009.07.010
Butler P. G., Scourse J. D., Richardson C. A., Wanamaker A. D., Bryant C. L., Bennell J. D. (2009). Continuous Marine Radiocarbon Reservoir Calibration and the 13C Suess Effect in the Irish Sea: Results From the First Multi-Centennial Shell-Based Marine Master Chronology. Earth Planet. Sci. Lett. 279, 230–241(3–4). doi: 10.1016/j.epsl.2008.12.043
Butler P. G., Wanamaker A. D., Scourse J. D., Richardson C. A., Reynolds D. J. (2013). Variability of Marine Climate on the North Icelandic Shelf in a 1357-Year Proxy Archive Based on Growth Increments in the Bivalve Arctica Islandica. Palaeogeogr. Palaeoclimatol. Palaeoecol. 373, 141–151. doi: 10.1016/j.palaeo.2012.01.016
Caragnano A., Basso D., Storz D., Jacob D. E., Ragazzola F., Benzoni F., et al. (2017). Elemental Variability in the Coralline Alga Lithophyllum Yemenense as an Archive of Past Climate in the Gulf of Aden (NW Indian Ocean). J. Phycol. 53, 381–395. doi: 10.1111/JPY.12509
Cavalieri D. J., Parkinson C. L. (2012). Arctic Sea Ice Variability and Trends 1979-2010. Cryosphere 6 (4), 881–889. doi: 10.5194/tc-6-881-2012
Chan P., Halfar J., Adey W., Hetzinger S., Zack T., Moore G. W. K., et al. (2017). Multicentennial Record of Labrador Sea Primary Productivity and Sea-Ice Variability Archived in Coralline Algal Barium. Nat. Commun. 8 (1), 1–10. doi: 10.1038/ncomms15543
Cook E. R. (1987). The Decomposition of Tree-Ring Series for Environmental Studies. Tree-Ring Bull. 47, 37–59.
DeLong K. L., Flannery J. A., Poore R. Z., Quinn T. M., Maupin C. R., Lin K., et al. (2014). A Reconstruction of Sea Surface Temperature Variability in the Southeastern Gulf of Mexico From 1734 to 2008 C.E. Using Cross-Dated Sr/Ca Records From the Coral Siderastrea Siderea. Paleoceanography 29, 403–422. doi: 10.1002/2013PA002524
DeLong K. L., Quinn T. M., Taylor F. W. (2007). Reconstructing Twentieth-Century Sea Surface Temperature Variability in the Southwest Pacific: A Replication Study Using Multiple Coral Sr/Ca Records From New Caledonia. Paleoceanography 22 (4), 1–18. doi: 10.1029/2007PA001444
DeLong K. L., Quinn T. M., Taylor F. W., Shen C. C., Lin K. (2013). Improving Coral-Base Paleoclimate Reconstructions by Replicating 350 Years of Coral Sr/Ca Variations. Palaeogeogr. Palaeoclimatol. Palaeoecol. 373, 6–24. doi: 10.1016/j.palaeo.2012.08.019
Epplé V. M., Brey T., Witbaard R., Kuhnert H., Pätzold J. (2006). Sclerochronological Records of Arctica Islandica From the Inner German Bight. Holocene 16 (5), 763–769. doi: 10.1191/0959683606hl970rr
Felis T., Pätzold J., Loya Y., Fine M., Nawar A. H., Wefer G. (2000). A Coral Oxygen Isotope Record From the Northern Red Sea Documenting NAO, ENSO, and North Pacific Teleconnections on Middle East Climate Variability Since the Year 1750. Paleoceanography 15 (6), 679–694. doi: 10.1029/1999PA000477
Fietzke J., Ragazzola F., Halfar J., Dietze H., Foster L. C., Hansteen T. H., et al. (2015). Century-Scale Trends and Seasonality in pH and Temperature for Shallow Zones of the Bering Sea. Proc. Natl. Acad. Sci. U. S. A. 112 (10), 2960–2965. doi: 10.1002/dac.3707
Glock W. S. (1937). Principles and Methods of Tree-Ring Analysis (Washington, DC: Carnegie Institution of Washington).
Halfar J., Adey W. H., Kronz A., Hetzinger S., Edinger E., Fitzhugh W. W. (2013). Arctic Sea-Ice Decline Archived by Multicentury Annual-Resolution Record From Crustose Coralline Algal Proxy. Proc. Natl. Acad. Sci. U. S. A. 110 (49), 19737–19741. doi: 10.1073/pnas.1313775110
Halfar J., Hetzinger S., Adey W., Zack T., Gamboa G., Kunz B., et al. (2011). Coralline Algal Growth-Increment Widths Archive North Atlantic Climate Variability. Palaeogeogr. Palaeoclimatol. Palaeoecol. 302, 71–80. doi: 10.1016/j.palaeo.2010.04.009
Halfar J., Steneck R. S., Joachimski M., Kronz A., Wanamaker A. D. (2008). Coralline Red Algae as High-Resolution Climate Recorders. Geology 36 (6), 463–466. doi: 10.1130/G24635A.1
Halfar J., Steneck R., Schöne B., Moore G. W. K., Joachimski M., Kronz A., et al. (2007). Coralline Alga Reveals First Marine Record of Subarctic North Pacific Climate Change. Geophys. Res. Lett. 34 (7), 1–5. doi: 10.1029/2006GL028811
Helama S., Schöne B. R., Black B. A., Dunca E. (2006). Constructing Long-Term Proxy Series for Aquatic Environments With Absolute Dating Control Using a Sclerochronological Approach: Introduction and Advanced Applications. Mar. Freshw. Res. 57, 591–599. doi: 10.1071/MF05176
Hetzinger S., Halfar J., Kronz A., Simon K., Adey W. H., Steneck R. S. (2018). Reproducibility of Clathromorphum Compactum Coralline Algal Mg/Ca Ratios and Comparison to High-Resolution Sea Surface Temperature Data. Geochim. Cosmochim. Ac. 220, 96–109. doi: 10.1016/j.gca.2017.09.044
Hetzinger S., Halfar J., Zack T., Gamboa G., Jacob D. E., Kunz B. E., et al. (2011). High-Resolution Analysis of Trace Elements in Crustose Coralline Algae From the North Atlantic and North Pacific by Laser Ablation ICP-MS. Palaeogeogr. Palaeoclimatol. Palaeoecol. 302 (1), 81–94. doi: 10.1016/j.palaeo.2010.06.004
Hetzinger S., Halfar J., Zajacz Z., Möller M., Wisshak M. (2021). Late Twentieth Century Increase in Northern Spitsbergen (Svalbard) Glacier-Derived Runoff Tracked by Coralline Algal Ba/Ca Ratios. Clim. Dynam. 56 (9), 3295–3303. doi: 10.1007/s00382-021-05642-x
Hetzinger S., Halfar J., Zajacz Z., Wisshak M. (2019). Early Start of 20th-Century Arctic Sea-Ice Decline Recorded in Svalbard Coralline Algae. Geology 47 (10), 963–967. doi: 10.1130/G46507.1
Hill B. T., Jones S. J. (1990). The Newfoundland Ice Extent and the Solar Cycle From 1860 to 1988. J. Geophys. Res. 95 (C4), 5385. doi: 10.1029/JC095iC04p05385
Hippler D., Buhl D., Witbaard R., Richter D. K., Immenhauser A. (2009). Towards a Better Understanding of Magnesium-Isotope Ratios From Marine Skeletal Carbonates. Geochim. Cosmochim. Ac. 73, 6134–6146. doi: 10.1016/j.gca.2009.07.031
Holmes R. L. (1983). Computer-Assisted Quality Control in Tree-Ring Dating and Measurement. Tree-Ring Bull. 43, 69–78.
Hou A., Halfar J., Adey W., Wortmann U. G., Zajacz Z., Tsay A., et al. (2018). Long-Lived Coralline Alga Records Multidecadal Variability in Labrador Sea Carbon Isotopes. Chem. Geol. 526, 93–100. doi: 10.1016/j.chemgeo.2018.02.026
Kamenos N. A. (2010). North Atlantic Summers Have Warmed More Than Winters Since 1353, and the Response of Marine Zooplankton. Proc. Natl. Acad. Sci. U. S. A. 107 (52), 22442–22447. doi: 10.1073/pnas.1006141107
Kirchhefer A. J. (2001). Reconstruction of Summer Temperatures From Tree-Rings of Scots Pine (Pinus Sylvestris L.) in Coastal Northern Norway. Holocene 11 (1), 41–52. doi: 10.1191/095968301670181592
Leclerc N., Halfar J., Hetzinger S., Chan P. T. W., Adey W., Tsay A., et al. (2021). Suitability of the Coralline Alga Clathromorphum Compactum as an Arctic Archive for Past Sea Ice Cover. Paleoceanogr. Paleoclimatol 37 (1), 1–19. doi: 10.1029/2021PA004286
Linge H., Lauritzen S., Mangerud J., Kamenos N. A., Gherardi J. (2008). Assessing the Use of U – Th Methods to Determine the Age of Cold-Water Calcareous Algae. Quat. Geochronol. 3 (1–2), 76–88. doi: 10.1016/j.quageo.2007.09.003
Marali S., Schöne B. R. (2015). Oceanographic Control on Shell Growth of Arctica Islandica (Bivalvia) in Surface Waters of Northeast Iceland — Implications for Paleoclimate Reconstructions. Palaeogeogr. Palaeoclimatol. Palaeoecol. 420, 138–149. doi: 10.1016/j.palaeo.2014.12.016
Meier W. N., Fetterer F., Savoie M., Mallory S., Duerr R., Stroeve J. (2017). NOAA/NSIDC Climate Data Record of Passive Microwave Sea Ice Concentration. doi: 10.7265/N59P2ZTG
Miles M. W., Divine D. V., Furevik T., Jansen E., Moros M., Ogilvie A. E. J. (2014). A Signal of Persistent Atlantic Multidecadal Variability in Arctic Sea Ice. Geophys. Res. Lett. 41, 463–469. doi: 10.1002/2013GL058084
Peng G., Meier W. N., Scott D. J., Savoie M. H. (2013). A Long-Term and Reproducible Passive Microwave Sea Ice Concentration Data Record for Climate Studies and Monitoring. Earth Syst. Sci. Data 5 (2), 311–318. doi: 10.5194/essd-5-311-2013
Pohl K. A., Hadley K. S., Arabas K. B. (2013). A 545-Year Drought Reconstruction for Central Oregon. Phys. Geogr. 23 (4), 302–320. doi: 10.2747/0272-3646.23.4.302
Porter T. J., Pisaric M. F. J., Kokelj S. V., deMontigny P. (2013). A Ring-Width-Based Reconstruction of June-July Minimum Temperatures Since AD1245 From White Spruce Stands in the Mackenzie Delta Region, Northwestern Canada. Quaternary Res. 80 (2), 167–179. doi: 10.1016/j.yqres.2013.05.004
Prendergast A. L., Versteegh E. A. A., Schöne B. R. (2017). New Research on the Development of High-Resolution Palaeoenvironmental Proxies From Geochemical Properties of Biogenic Carbonates. Palaeogeogr. Palaeoclimatol. Palaeoecol. 484, 1–6. doi: 10.1016/j.palaeo.2017.05.032
Rasher D. B., Steneck R. S., Halfar J., Kroeker K. J., Ries J. B., Tinker M. T., et al. (2020). Keystone Predators Govern the Pathway and Pace of Climate Impacts in a Subarctic Marine Ecosystem. Science 369 (6509), 1351–1355. doi: 10.1126/science.aav7515
Reynolds D. J., Richardson C. A., Scourse J. D., Butler P. G., Hollyman P., Román-González A., et al. (2017). Reconstructing North Atlantic Marine Climate Variability Using an Absolutely-Dated Sclerochronological Network. Palaeogeogr. Palaeoclimatol. Palaeoecol. 465, 333–346. doi: 10.1016/j.palaeo.2016.08.006
Reynolds D. J., Scourse J. D., Halloran P. R., Nederbragt A. J., Wanamaker A. D., Butler P. G., et al. (2016). Annually Resolved North Atlantic Marine Climate Over the Last Millennium. Nat. Commun. 7 (1), 1–11. doi: 10.1038/ncomms13502
Schöne B. R., Tanabe K., Dettman D. L., Sato S. (2003). Environmental Controls on Shell Growth Rates and δ18o of the Shallow-Marine Bivalve Mollusk Phacosoma Japonicum in Japan. Mar. Biol. 142 (3), 473–485. doi: 10.1007/s00227-002-0970-y
Schweingruber F. H., Eckstein D., Serre-Bachet F., Bräker O. U. (1990a). Identification, Presentation and Interpretation of Event Years and Pointer Years in Dendrochronology. Dendrochronologia 8, 9–38.
Schweingruber F. H., Kairiukstis L., Shiyatov S. (1990b). “Sample Selection”, in Methods of Dendrochronology: Applications in the Environmental Sciences. Eds. Cook E. R., Kairiukstis L. A. (Dordrecht: Kluwer Academic Publishers), 23–35.
Shen C. C., Li K. S., Sieh K., Natawidjaja D., Cheng H., Wang X., et al. (2008). Variation of Initial 230Th/232Th and Limits of High Precision U-Th Dating of Shallow-Water Corals. Geochim. Cosmochim. Ac. 72 (17), 4201–4223. doi: 10.1016/j.gca.2008.06.011
Siebert V., Poitevin P., Chauvaud L., Schöne B. R., Lazure P., Thébault J. (2020). Using Growth and Geochemical Composition of Clathromorphum Compactum to Track Multiscale North Atlantic Hydro-Climate Variability. Palaeogeogr. Palaeoclimatol. Palaeoecol. 562, 110097. doi: 10.1016/j.palaeo.2020.110097
Stoica P., Larsson E. G., Sorelius J. (2000). Analysis of Mussel Growth Data. Hydrobiologia 438 (1), 245–249. doi: 10.1023/A:1004138422421
Stott K. J., Austin W. E. N., Sayer M. D. J., Weidman C. R., Cage A. G., Wilson R. J. S. (2010). The Potential of Arctica Islandica Growth Records to Reconstruct Coastal Climate in North West Scotland, Uk. Quaternary Sci. Rev. 29 (13–14), 1602–1613. doi: 10.1016/j.quascirev.2009.06.016
Swart P. K., Healy G. F., Dodge R. E., Kramer P., Hudson J. H., Halley R. B., et al. (1996). The Stable Oxygen and Carbon Isotopic Record From a Coral Growing in Florida Bay: A 160 Year Record of Climatic and Anthropogenic Influence. Palaeogeogr. Palaeoclimatol. Palaeoecol. 123 (1–4), 219–237. doi: 10.1016/0031-0182(95)00078-X
Turekian K. K., Cochran J. K., Nozaki Y., Thompson I., Jones D. S. (1982). Determination of Shell Deposition Rates of Arctica Islandica From the New York Bight Using Natural 228 Ra and 228 Th and Bomb-Produced 14 C1. Limnol. Oceanogr. 27 (4), 737–741. doi: 10.4319/lo.1982.27.4.0737
Weymouth F. W. (1922). Fish Bulletin No. 7 The Life-History and Growth of the Pismo Clam (Tivela Stultorum Mawe).
Williams S., Adey W. H., Halfar J., Kronz A., Gagnon P., Bélanger D., et al. (2018a). Effects of Light and Temperature on Mg Uptake, Growth, and Calcification in the Proxy Climate Archive Clathromorphum Compactum. Biogeosciences 15 (19), 5745–5759. doi: 10.5194/bg-15-5745-2018
Williams B., Halfar J., Delong K. L., Smith E., Steneck R., Lebednik P. A., et al. (2017). North Pacific Twentieth Century Decadal-Scale Variability is Unique for the Past 342 Years. Geophys. Res. Lett. 44 (8), 3761–3769. doi: 10.1002/2017GL073138
Williams S., Halfar J., Zack T., Blicher M., Juul-pedersen T. (2018b). Comparison of Climate Signals Obtained From Encrusting and Free-Living Rhodolith Coralline Algae. Chem. Geol. 476, 418–428. doi: 10.1016/j.chemgeo.2017.11.038
Keywords: Coralline red algae, Sea ice proxy, crossdating, sclerochronology, growth increments, dendrochronology, Mg/Ca ratios, LA-ICP-MS.
Citation: Leclerc N, Halfar J, Porter TJ, Black BA, Hetzinger S, Zulian M and Tsay A (2022) Utility of Dendrochronology Crossdating Methods in the Development of Arctic Coralline Red Algae Clathromorphum compactum Growth Increment Chronology for Sea Ice Cover Reconstruction. Front. Mar. Sci. 9:923088. doi: 10.3389/fmars.2022.923088
Received: 18 April 2022; Accepted: 10 May 2022;
Published: 09 June 2022.
Edited by:
Nadine Schubert, University of AlgarvePortugalReviewed by:
Ana Cristina Rebelo, Instituto Hidrográfico, PortugalFederica Ragazzola, Stazione Zoologica Anton Dohrn Napoli, Italy
Copyright © 2022 Leclerc, Halfar, Porter, Black, Hetzinger, Zulian and Tsay. This is an open-access article distributed under the terms of the Creative Commons Attribution License (CC BY). The use, distribution or reproduction in other forums is permitted, provided the original author(s) and the copyright owner(s) are credited and that the original publication in this journal is cited, in accordance with accepted academic practice. No use, distribution or reproduction is permitted which does not comply with these terms.
*Correspondence: Natasha Leclerc, bmF0YXNoYS5sZWNsZXJjQG1haWwudXRvcm9udG8uY2E=