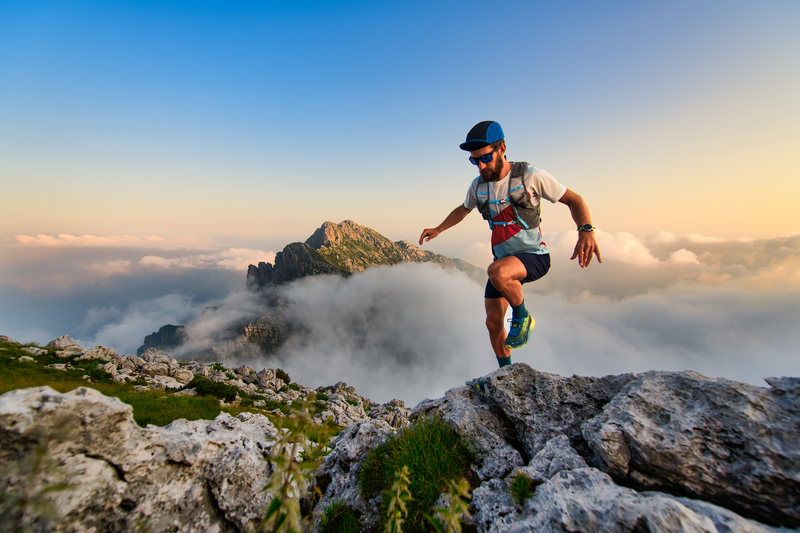
95% of researchers rate our articles as excellent or good
Learn more about the work of our research integrity team to safeguard the quality of each article we publish.
Find out more
ORIGINAL RESEARCH article
Front. Mar. Sci. , 29 July 2022
Sec. Marine Biotechnology and Bioproducts
Volume 9 - 2022 | https://doi.org/10.3389/fmars.2022.914197
This article is part of the Research Topic Sustainable Production of Marine Natural Products - From Discovery to Application View all 9 articles
Many marine sponges harbor dense communities of microbes that aid in the chemical defense of these nonmotile hosts. Metabolites that comprise this chemical arsenal can have pharmaceutically-relevant activities such as antibacterial, antiviral, antifungal and anticancer properties. Previous investigation of the Caribbean giant barrel sponge Xestospongia muta revealed a microbial community including novel Actinobacteria, a phylum well known for its production of antibiotic compounds. This novel assemblage was investigated for its ability to produce compounds that inhibit M. tuberculosis by using a bioinformatics approach. Microbial extracts were tested for their ability to inhibit growth of M. tb and genomes of the 11 strains that showed anti-M. tb activity including Micrococcus (n=2), Micromonospora (n=4), Streptomyces (n=3), and Brevibacterium spp. (n=2) were sequenced by using Illumina MiSeq. Three assembly algorithms/pipelines (SPAdes, A5-miseq and Shovill) were compared for their ability to construct contigs with minimal gaps to maximize the probability of identifying complete biosynthetic gene clusters (BGCs) present in the genomes. Although A5-miseq and Shovill usually assembled raw reads into the fewest contigs, after necessary post-assembly filtering, SPAdes generally produced the most complete genomes with the fewest contigs. This study revealed the strengths and weaknesses of the different assemblers based on their ease of use and ability to be manipulated based on output format. None of the assembly methods handle contamination well and high-quality DNA is a prerequisite. BGCs of compounds with known anti-TB activity were identified in all Micromonospora and Streptomyces strains (genomes > 5 Mb), while no such BGCs were identified in Micrococcus or Brevibacterium strains (genomes < 5 Mb). The majority of the putative BGCs identified were located on contig edges, emphasizing the inability of short-read assemblers to resolve repeat regions and supporting the need for long-read sequencing to fully resolve BGCs.
Marine sponges are found in all parts of the ocean, ranging from warm, shallow tropical waters to polar waters and even the deep ocean (Hooper and van Soest, 2002; Hentschel et al., 2006). Similarly, actinomycetes are found in a wide range of terrestrial, freshwater and marine environments (van der Meij et al., 2017). Marine sponges can harbor a huge amount and remarkable diversity of microbial symbionts both intracellularly and extracellularly within the mesohyl matrix (Vacelet, 1975; Vacelet and Donadey, 1977; Hentschel et al., 2002). Additionally, there is evidence that sponges actively select for the presence of species-specific symbiotic microbial communities (Taylor et al., 2004; Hentschel et al., 2006; Taylor et al., 2007; Lee et al., 2010; Montalvo and Hill, 2011). Although a minimal core community has been identified to exist among sponges of various species and habitats, the majority of the microbial diversity present within a given sponge host is species-specific (sponge-specific or monophyletic), and generally distinct from the microbial diversity present in the surrounding seawater as evidenced by both culture-dependent and independent studies (Wilkinson, 1978; Webster and Hill, 2001; Hentschel et al., 2002; Taylor et al., 2004; Taylor et al., 2005; Hill et al., 2006; Taylor et al., 2007; Lee et al., 2010; Montalvo and Hill, 2011; Schmitt et al., 2012). Furthermore, studies assessing the intra- and interspecies variability of these microbial communities over distant geographic locations and temporal changes have found striking stability in at least a subpopulation of these communities (Taylor et al., 2004; Webster et al., 2004; Taylor et al., 2005; Montalvo and Hill, 2011; Schmitt et al., 2012). Depending on the density of symbiotic bacteria harbored by sponges, they can be classified as being either of low microbial abundance (LMA) or high microbial abundance (HMA, aka ‘bacteriosponge’) (Vacelet and Donadey, 1977; Reiswig, 1981; Hentschel et al., 2003) LMA sponges have a similar bacterial density to that of the surrounding seawater (105-106 bacteria per gram of sponge wet weight), while HMA sponges can harbor a density of 108-1010 bacteria per gram of sponge wet weight (Reiswig, 1981; Hentschel et al., 2003). As these bacterial members constitute such a significant portion of their host by volume, it is not surprising that they play a critical role in nutrient cycling, of which actinomycetes contribute to nitrogen and phosphorus cycling as well as decomposition of organic material (Goodfellow and Williams, 1983; Hentschel et al., 2006; Sabarathnam et al., 2010; Weigel and Erwin, 2017; Zhang et al., 2019). The largest known sponge species, fittingly named the giant barrel sponge, Xestospongia muta (Schmidt 1870) is an HMA sponge commonly found in coral reef communities in the Caribbean (Hentschel et al., 2006; McMurray et al., 2008). Xestospongia muta has been reported to reach up to 2 m in height in the natural environment (ranging from Florida to Brazil) and is believed to be long-lived, with some individuals estimated to be anywhere from 100 to 1,000 years old (van Soest, 1980; Hechtel, 1983; Kerr and Kelly-Borges, 1994; Montalvo et al., 2005; Hentschel et al., 2006; McMurray et al., 2008). Previous analysis by Montalvo et al. (2005) found Actinobacteria to dominate the microbial community composition of X. muta individuals isolated from the Florida Keys and identified a novel assemblage of actinobacteria isolates. Given that actinomycetes are such a prominent source of antibiotics, this novel assemblage was investigated for its potential to produce compounds with antimycobacterial activity with a primary focus on inhibition of M.tb.
Specifically considering the possibility that marine actinomycetes will synthesize antimycobacterial compounds, research suggests this likelihood is based on more than just random chance due to sheer abundance of actinomycetes harbored within host tissue. In a recent study, 11 Mycobacterium species, together with an antimycobacterial Salinispora species, were isolated from the sponge Amphimedon queenslandica (Izumi et al., 2010). Several Salinispora species, including the strain isolated in the 2020 investigation, are capable of synthesizing rifamycins, a group of antibiotics that includes one of the top-line anti-TB drugs, rifampicin (Kim et al., 2006; Wilson et al., 2010). The authors of the study hypothesize that production of antimycobacterial compounds by marine actinomycetes may function in competition between the cohabiting sponge symbionts. Furthermore, several anti-TB compounds have already been isolated from marine sponges and associated actinomycetes. In addition to marine-sponge derived rifamycin-producing Salinispora strains (Kim et al., 2006), macfarlandins (anti-TB diterpenoids) were isolated from a Samoan Chelonaplysilla sponge (de Oliveira et al., 2020), and haliclonadiamines derivatives (antimycobacterial alkaloids) were isolated from an Okinawan Haliclona sponge species (Abdjul et al., 2018). It is important to note that only crude Haliclona and Chelonaplysilla extracts were tested for growth inhibition, and thus, the possibility cannot be ruled out that the isolated antimycobacterial compounds actually derive from associated microbes. Interestingly, the first marketable antibiotic isolated from an actinomycete (streptomycin) effectively inhibited M.tb (Schatz et al., 1944; Woodruff, 2014). Unfortunately, as is the case with many antibiotics, resistance to this drug developed soon after it became available (Pyle, 1947). In 2020, almost 10 million people became infected with M.tb, 1.5 million of whom died as a result of infection (World Health Organization, 2021). In the previous year, approximately 500,000 cases developed rifampicin-resistant infections, 78% of which further evolved into multi-drug resistant TB (World Health Organization, 2020). The current status of TB clearly demands an urgent need for novel antibiotics to treat the disease.
After screening microbial extracts, a bioinformatics approach was undertaken to identify the genomic pathway(s) most probably associated with any compounds responsible for observed anti-TB activity, as well as to assess the full biosynthetic potential of interesting strains. These compounds, known as natural products, can be classified into various categories based on their chemical structure, the most common being polyketides (PKs), nonribosomal peptides (NRPs), ribosomally synthesized and post-translationally modified peptides (RiPPs), terpenoids and alkaloids (Medema et al., 2015; Hug et al., 2020). Directly studying the genes responsible for synthesizing these natural products enables assessment of the full biosynthetic potential of specific microbes despite the inability to culture the bacteria or lack of production of a particular product in the laboratory. This powerful approach is achievable by virtue of the fact that several classes of natural products (PKs, NRPs) are encoded by genes laid out in a recognizable pattern collectively known as a biosynthetic gene cluster (BGC) (Martín & Liras, 1989). The assembly-line nature of their synthesis facilitates their detection through genome-mining efforts (Van Lanen & Shen, 2006; Zerikly & Challis, 2009). Quick detection of BGCs by computational methods enables rapid dereplication, avoiding the unfortunate but common occurrence of reisolating an already discovered compound (Woodruff & McDaniel, 1958; Baltz, 2005). Furthermore, assessment of the putative BGC repertoire of a genome enables prioritization of strains for further analysis (Zhang et al., 2017; Ward & Allenby, 2018).
The GC-rich nature of actinomycete genomes makes sequencing and assembly very challenging (Benjamini and Speed, 2012; Rajwani et al., 2021). To optimize the success of this genome mining strategy, three assembly pipelines were compared for their particular ability to efficiently assemble reads and minimize gaps in actinomycete genomes. Based on successful strategies described in the literature, the following pipelines were evaluated: SPAdes (SPAdes, RRID : SCR_000131) (Bankevich et al., 2012), Shovill (shovill, RRID : SCR_017077) (Seemann, 2020) and A5-miSeq (A5-miseq, RRID : SCR_012148) (Tritt et al., 2012; Coil et al., 2015) (Klein et al., 2016; Koenigsaecker et al., 2016; Schorn et al., 2016; Durrell et al., 2017; Egidi et al., 2017; Kincheloe et al., 2017; Bellassi et al., 2020; Blackwell et al., 2021; Soldatou et al., 2021; Tarlachkov et al., 2021). All three pipelines assemble raw Illumina data with slightly different processing steps. SPAdes involves four major stages: assembly graph construction, k-mer adjustment, paired assembly graph construction, and contig construction (Bankevich et al., 2012). The Shovill pipeline utilizes SPAdes genome assembler to assemble reads but involves modified pre- and post-assembly steps, and is optimized for smaller genomes (Seemann, 2020). The A5-miSeq workflow consists of five steps: read cleaning, contig assembly, crude scaffolding, mis-assembly correction, and final scaffolding. Similar to SPAdes, all steps are automated, but what makes this pipeline unique is the fact that all parameters are fixed, without the option to adjust (Coil et al., 2015). The following programs were employed post-assembly to detect putative BGCs: antiSMASH (antibiotics & Secondary Metabolite Analysis Shell) (Blin et al., 2019) and NP.Searcher (Li et al., 2009). The most comprehensive of these programs, antiSMASH, can detect biosynthetic genes associated with more than 20 natural product classes including PKs, NRPs, terpenes, and bacteriocins (Medema et al., 2011; Medema and Fischbach, 2015). The antiSMASH program uses Hidden Markov Models (HMMs) via HMMer3 (Hmmer, RRID : SCR_005305) to detect possible clusters through alignment of the translated nucleotide sequence with proteins or protein domains determined to be exclusively present in particular BGCs and maintains an extensive database of known BGCs to facilitate comparative cluster analysis. NP.Searcher utilizes BLAST to rapidly scan genomes and determine substrate specificities of adenylation and acyltransferase domains in nonribosomal peptide synthetases (NRPSs) and polyketide synthases (PKSs), respectively. This program also conveniently provides the Simplified Molecular Input Line Entry Specification (SMILES) output of expected products and links results with programs that predict the associated 2D and 3D chemical structures of the natural product (Li et al., 2009).
Strains were previously isolated from X. muta samples collected at a depth of 20 m by SCUBA at Conch Reef, Key Large, Florida in July 2001 and June 2004 (latitude 24°56.82’ N, longitude 80°27.40’ W). Strains were isolated and stored as described by Montalvo et al. (2005). Cryovials of isolates were plated out on both International Streptomyces Project 2 (ISP2) agar (BD-Difco™, Franklin Lakes, NJ, USA) supplemented with 20% salt (granular sodium chloride - J.T. Baker, Phillipsburg, NJ, USA) and Reasoner’s 2A Agar (R2A) (BD - Difco™, Franklin Lakes, NJ, USA). Plates were incubated at 30°C until growth of individual colonies could be observed. Two individual colonies per plate were then transferred to the corresponding medium from which they were initially isolated – 100 mL of ISP2 or Reasoner’s 2A broth (R2B) (EZ-Media - Microbiology International, Frederick, MD, USA) – in 250-mL baffled flasks to provide good aeration, and incubated at 30°C with shaking at 150 rpm for a minimum of two weeks, until cultures appeared dense. ISP2 liquid medium was prepared using yeast extract (BD-Bacto™, Franklin Lakes, NJ, USA), dextrose (Fisher Scientific, Hampton, NH, USA), malt extract (MP Bio, Santa Ana, CA, USA) and 20% salt (granular sodium chloride - J.T. Baker, Phillipsburg, NJ, USA).
Mycobacterium tuberculosis H37Ra, Mycobacterium marinum ATCC 927 and Mycobacterium smegmatis MC2 155 were all plated from cryovials onto Middlebrook M7H10 agar (Sigma-Aldrich, St. Louis, MO, USA) supplemented with 10% OADC (oleic acid (Sigma-Aldrich, St. Louis, MO, USA), bovine serum albumin fraction V (Roche – Sigma-Aldrich, St. Louis, MO, USA), dextrose (Fisher Scientific, Hampton, NH, USA), catalase (Sigma, St. Louis, MO, USA), sodium chloride (enzyme grade - Fisher Scientific, Hampton, NH, USA) and subsequently cultured in Middlebrook M7H9 liquid medium (BD-Difco™, Franklin Lakes, NJ, USA) supplemented with 10% ADC (bovine serum albumin fraction V, dextrose, catalase, sodium chloride) and 250 µL Tween 80 (Amresco, Solon, OH, USA). M. marinum ATCC 927 and M. smegmatis MC2 155 were incubated at 30°C with shaking at 150 rpm and M. tuberculosis H37Ra was incubated at 37°C with shaking at 150 rpm.
After dense growth was evident in actinobacterial cultures, the cultures were extracted with HPLC-plus grade ethyl acetate (EtOAc) (Sigma-Aldrich, St. Louis, MO, USA). A 1:1 volume of EtOAc was added to each culture and incubated overnight at 30°C with shaking at 150 rpm. The organic phases were dried by rotary evaporation, and the final samples were dried in GC vials using a Savant SpeedVac® PLUS SC210A. The aqueous phases were discarded.
Extracts were dissolved in dimethyl sulfoxide (DMSO) and applied to 6 mm Whatman filter discs at a concentration of 25 µg/10 µL and 250 µg/10 µL to establish a dose response. Discs were applied to plates inoculated with M. tuberculosis H37Ra, M. marinum ATCC 927 and M. smegmatis MC2 155 at exponential phase. A disc inoculated with 10 µL of DMSO was used as a negative control. All extracts were tested at both concentrations in duplicate. Plates of M. marinum ATCC 927 and M. smegmatis MC2 155 were incubated for several days at 30°C and M. tuberculosis H37Ra at 37°C until dense lawn growth and inhibition zones could be observed, usually at two weeks. Resulting zones of inhibition were measured under an illuminated colony counter.
Strains were assigned taxonomic classifications after initial isolation, which were confirmed at the time of this study on the basis of partial 16S rRNA gene sequence analysis. DNA was extracted using the UltraClean® Microbial DNA Isolation Kit (MO Bio Laboratories Inc., Carlsbad, CA, USA). Genomic DNA was quantified using a Nanodrop 2000 Spectrophotometer (Thermo Scientific, Waltham, MA, USA). Degenerate primer 27F 5’-AGAGTTTGATCMTGGCTCAG-3’ (Hyman et al., 2005) and 1492R 5’-CGGTTACCTTGTTACGACTT-3’ (Sasoh et al., 2006) were used to amplify 16S rRNA gene fragments, and polymerase chain reaction (PCR) amplification was performed on a PTC-200 Peltier Thermal Cycler (MJ Research, St. Bruno, QC, CA). The PCR reaction mix consisted of 12.5 µL JumpStart™ REDTaq® ReadyMix™ Reaction Mix (Sigma-Aldrich, St. Louis, MO, USA), at least 15 ng of DNA template, 1 µL each of 27F and 1492R (10 µM stock) and sterile deionized water for a final volume of 25 µL. The PCR was programmed to the following protocol: 31 cycles of denaturation at 95°C for 1 min 30 sec, annealing at 55°C for 1 min 30 sec, and elongation at 72°C for 1 min 30 sec, followed by a final extension step at 72°C for 7 min. PCR products were separated on a 2% agarose gel to confirm amplification and purified with ExoSAP-IT™ or ExoSAP-IT™ Express PCR Product Cleanup Reagent (ThermoFisher Scientific, Waltham, MA, USA). Forward and reverse Sanger sequences were trimmed and assembled using CLC Main Workbench 7 (CLC Main Workbench, RRID : SCR_000354), and the resulting consensus sequence was compared against the NCBI database with BLASTN (BLASTN, RRID : SCR_001598) to identify the strain. Sequence errors were corrected manually by visual inspection of chromatograms.
For genomic sequencing, DNA was sequenced by using a MiSeq sequencer (Illumina) with the MiSeq version 2.4.0.4 Reagent Kit. The Nextera DNA Flex Library Prep Kit (100 ng DNA) was used to prepare the sequencing libraries for Brevibacterium sp. strain XM4083 and Micromonospora sp. strain XM-20-01 (300 cycles each), while the Nextera XT Library Prep Kit (1 ng DNA) was used to prepare sequencing libraries for the remaining nine strains (2 x 250 bp paired-end reads for a total of 500 cycles).
Assembly was performed using three de novo methods: 1) reads were trimmed using Trimmomatic version 0.30 (Trimmomatic, RRID : SCR_011848) (Bolger et al., 2014) and assembled using SPAdes version 3.14.1 (Bankevich et al., 2012); 2) reads were assembled using A5-miseq version 20160825 assembly pipeline (Coil et al., 2015), and 3) reads were assembled using Shovill version 1.1.0 (unpublished, available at https://github.com/tseemann/shovill), which includes an optional step to trim adaptors (Trimmomatic version 0.39). Initial assembly statistics were evaluated with QUAST (QUAST, RRID : SCR_001228) (Gurevich et al., 2013). Contigs were then filtered primarily based on coverage, followed by match identity after comparison with the Nucleotide BLAST database. If a contig did not return a BLASTN hit (Zhang et al., 2000), blastx (BLASTX, RRID : SCR_001653) (Altschul et al., 1997) was performed and the contig was retained if it returned a hit to a protein identified from the expected genus with substantial query coverage and percent identity. Resulting contigs were also aligned to contigs of other genomes sequenced in the same Illumina MiSeq run to identify and remove spillover reads or cross contamination. Manual filtering was performed to remove any additional contigs with dubious coverage (determined cut-off value varied per assembly). PATRIC version 3.5.41 (Pathosystems Resource Integration Center, RRID : SCR_004154) was used to perform genome annotation and to calculate post-filtering statistics (Brettin et al., 2015; Davis et al., 2020). The final assemblies were validated by evaluating contamination and completeness values, calculated using CheckM version 1.0.18 app through KBase (kbase.us) (CheckM, RRID : SCR_016646) (Parks et al., 2015). Scaffolding was performed with MeDuSa version 1.6 (MeDuSa, RRID : SCR_022058) (Bosi et al., 2015). Genomes were scaffolded by comparison to all available complete or nearly complete genome sequences in the NCBI BLAST database that aligned to the trimmed 16S rRNA gene sequence of the particular strain. If the trimmed 16S rRNA gene sequence did not return any hits to complete genome sequences, the trimmed forward or reverse read was analyzed by BLASTN, and complete genome sequences were selected from the resulting list for scaffolding. Default parameters were used for all software packages. However, for Trimmomatic, a slightly modified script was used that was more sensitive for adapters and also included a sliding window of four bases to scan the reads and remove bases when the average quality per base was below 15. BGCs were identified using the following algorithms: antiSMASH version 5.0 (Blin et al., 2019) in relaxed mode and NP.Searcher (Li et al., 2009). For a schematic overview of this analysis, see Figure 1.
Figure 1 Schematic overview of research pipeline: microbiology procedures and experiments to identify strains producing extracts with inhibitory activity against M. tb and comparative bioinformatic analysis of three de novo assembly pipelines with evaluation parameters to optimize assembly completeness for genome mining. Note: Some symbols sourced from Integration and Application Network (ian.umces.edu/media-library).
In addition to 16S rRNA gene sequence analysis from Sanger sequencing data, the housekeeping genes recA and gyrB were identified from PATRIC annotation of assembled Illumina MiSeq data and aligned with CLC Main Workbench 7 (CLC Main Workbench, RRID : SCR_000354). These genes were chosen based on studies supporting their use as supplemental (or even better than 16S rRNA in some cases) phylogenetic markers for the classification of related bacterial strains (Rossi et al., 2006; Liu et al., 2012; Zhang et al., 2019). Pairwise genome comparison using all three assemblies (SPAdes, A5-miseq, Shovill) per genome was performed by calculating average nucleotide identity (ANI) based on BLAST and MUMmer (MUMmer, RRID : SCR_018171) with JSpecies Web Server (JSpeciesWS) (JSpeciesWS, RRID : SCR_022059) (Kurtz et al., 2004; Goris et al., 2007; Richter et al., 2016). Genome dot plots were created using D-Genies web application (D-GENIES, RRID : SCR_018967) aligned with Minimap2 version 2.24 (Minimap2, RRID : SCR_018550) (Cabanettes and Klopp, 2018).
From the original collection of 101 novel actinomycetes previously isolated from X. muta, 58 strains were recovered from storage and grew on either on ISP2 or R2A medium. Of these, 11 strains were found to produce extracts that consistently inhibit the growth of M. tuberculosis H37Ra (Table 1). Despite the fact that the 16S rRNA gene sequences of several strains returned BLASTN hits with 100% identity to other sequences in the database, these strains are individually described throughout this study, because even very closely related strains can produce different bioactive compounds (Antony-Babu et al., 2017). The extracts of four of the 11 isolates were found to have more wide-ranging activity and consistently inhibited the growth of all three mycobacteria tested: M. tuberculosis H37Ra, M. smegmatis MC2 155 and M. marinum ATCC 927. M. smegmatis MC2 155 and M. marinum ATCC 927 were used as preliminary indicators for anti-TB activity, as they are both closely related to M. tuberculosis and grow much more rapidly, facilitating more rapid screening. Additionally, M. marinum ATCC 927 is a known pathogen that rarely infects humans but causes a “tuberculosis-like illness in fish” (Akram and Aboobacker, 2021). In every case, extracts tested at 250 µg/10 µL DMSO were shown to produce a zone of inhibition greater than when tested at 25 µg/10 µL DMSO, confirming a dose-dependent response (Supplementary Table 1).
Table 1 Strain identification of active extracts, nearest well-identified BLAST hit and observed bioactivity.
Genome assembly for each strain was performed using paired-end reads with three separate assembly pipelines: (1) Trimmomatic and SPAdes, (2) A5-miseq, and (3) Shovill. In each case, default parameters were used in assembly. As Trimmomatic is merely a trimming function performed pre-assembly, the first pipeline will be hereafter referred to as SPAdes-assembled. It is important to note that there is a slight difference in the way that QUAST and PATRIC calculate assembly statistics. The pre-filtering assembly statistics (generated by QUAST) only consider contigs of at least 500 bp when calculating GC content and N50 values, while PATRIC generates these two statistics for the final post-filtered assembly by considering all contigs, irrespective of length. Furthermore, pre- and post-filtering sequence coverage values actually refer to average k-mer coverage for SPAdes and Shovill assemblies. Although Shovill is based on SPAdes, this pipeline varies in that it estimates coverage depth by calculating the ratio of total reads over genome size and automatically downsamples fastq files to a depth of 150x. In addition, Shovill removes any contigs below a coverage of 2x by default. For A5-miseq assemblies, pre-filtering coverage values are calculated from the average coverage of reads included in the final assembly after quality control and error correction. Because A5-miseq does not provide individual coverage values for assembled contigs, it is not possible to calculate a coverage depth of the assembly post-filtering. These inherent differences in the three pipelines result in highly variable coverage values between assemblies in some cases.
Prefiltering. The two Micrococcus strains’ assemblies were the smallest genomes analyzed in this set, with Micrococcus sp. strain XM4230A and Micrococcus sp. strain XM4230B having genomes of approximately 2.5 to 3.1 Mb, regardless of assembly method employed (Table 2, Figure 2). Shovill assembled raw data into the fewest initial contigs for strain XM4230A (63) while A5-miseq assembled raw data into the fewest initial contigs for strain XM4230B (121) (Figure 3). For strain XM4230A, the genome was determined to be between 2.6 and 3.1 Mb and have a GC content of 72.7%. For strain XM4230B, the genome size was much more consistent, with all assembly methods yielding a genome of 2.5-2.6 Mb with a GC content of 72.6-72.8%. Shovill yielded the highest N50 value for strain XM4230A (287,979) while SPAdes generated contigs with the highest N50 value for strain XM4230B (76,897) (Figure 4). Raw read files for strain XM4230A were substantially smaller (~300 Mb each) compared to those of all other genomes analyzed (usually ~1 Gb each).
Figure 4 N50 values calculated for genome assemblies pre- and post-filtering for contamination and spillover. Note: the pre-filtering N50 value for XM-20-01 assembled with SPAdes is 1,606 and assembled with A5-miseq is 22,545.
Post-filtering. Substantial removal of contaminant Micromonospora contigs was necessary for strain XM4230A when assembled with SPAdes (95% of total contigs (985/1,038) removed) and A5-miseq (73% of total contigs (116/158) removed). Ultimately, A5-miseq generated the best assembly for strain XM4230A and yielded the fewest contigs (42) (Figure 3), although the Shovill assembly retained a slightly higher N50 value (287,979 v. 222,030) (Figure 4). The final GC content of strain XM4230A was 72.8% in every assembly. After removal of contaminant contigs [44% of total contigs (63/143)] and filtering out low coverage contigs, SPAdes yielded the best assembly for strain XM4230B with fewest contigs (80) and a final GC content of 72.8% (Figure 3). The N50 values did not change for any genomes of strain XM4230B post-filtering.
Scaffolding. Based on BLAST hits aligning to the trimmed and error-corrected 16S rRNA gene sequences for the Micrococcus isolates, strain XM4230A was compared to two complete reference genomes, and strain XM4230B was compared to three complete reference genomes for scaffolding. Strain XM4230A assembled into seven to 12 scaffolds, with A5-assembled data yielding the best output. Strain XM4230B assembled into eight to 12 scaffolds, with A5-miseq-assembled contigs yielding the fewest scaffolds.
Prefiltering. Three of the four Micromonospora strains had very similar assemblies, with each genome assembling to approximately 6.7 Mb, irrespective of assembly method used (Table 3, Figure 2). In the case of Micromonospora sp. strain XM-20-01, significant fungal contamination was evidenced by the large total genome length initially assembled by SPAdes (33.0 Mb) and A5-miseq (27.0 Mb) and the reduced GC content (<60%) compared to other actinomycetes (Figure 2). This possibility was confirmed by BLAST hit comparison of contigs against the NCBI nt database. For three of the four strains (Micromonospora spp. strain XM-20-01, strain R42003, and strain R42004), Shovill assembled the genomes into the fewest contigs pre-filtering (Figure 3). For Micromonospora sp. strain R42106, SPAdes yielded the fewest contigs before filtering (Figure 3). For every strain except XM-20-01, all three assemblers yielded pre-filtered genomes with a GC content of 72.91 or 72.92%. N50 values were consistently highest for SPAdes assemblies, except for strain XM-20-01, for which Shovill provided the largest N50 value (110,598) (Figure 4).
Post-filtering. After comparing assembled contigs against the BLAST nt database, filtering for coverage and spillover contamination, SPAdes assemblies consistently yielded the fewest contigs (171-291), except for strain XM-20-01, for which Shovill yielded almost 10% fewer contigs (154) than it did with SPAdes or A5-miseq (Figure 3). Every assembly method for every Micromonospora strain produced a final genome size of 6.7 Mb with a final GC content of 72.9% (Figure 2). Filtering procedures did not significantly alter genome size, except for in the case of Micromonospora sp. strain XM-20-01, which was heavily contaminated with fungal contigs. After removal of all contaminant contigs, the genome of strain XM-20-01 was 6.7 Mb with a GC content of approximately 72.9%, in line with the other three strains (Figure 2). The N50 values did not change post-filtering for strains R42003, R42004, and R42106, since the contigs removed were small and had little impact on the overall assembly length (Figure 4). However, after removing all contaminant contigs from the XM-20-01 genome, the N50 value rose from less than 2,000 to over 90,000 bps for SPAdes and A5-miseq assemblies (Figure 4).
Scaffolding. Based on BLASTN identity of the trimmed reverse partial 16S rRNA gene sequences, Micromonospora sp. strain XM-20-01 contigs were scaffolded by comparison to two other complete genome sequences, resulting in 23 to 30 final scaffolds, with the fewest resulting when using the final SPAdes-assembled contigs. Strain R42003 ultimately assembled into six to eight contigs when compared to five reference genomes, with the SPAdes-assembled data yielding the fewest contigs again. In the case of strains R42004 and R42106, A5-miseq-assembled contigs yielded the fewest scaffolds with MeDuSa when each compared against three complete reference genomes, with the respective ranges being 13 to 21 scaffolds for strain R42004 and four to 15 scaffolds for strain R42016.
Prefiltering. All three software packages assembled strain XM4083 into a genome of approximately 4.0 Mb with a GC content of 68.02%, and strain R8603A2 into a genome of approximately 3.3 Mb with a GC content of 70.4% (Table 4, Figure 2). A5-miseq yielded by far the fewest contigs for strain XM4083 prefiltering (16), and Shovill yielded significantly fewer contigs for strain R8603A2 (66) than A5-miseq (79) or SPAdes (220) (Figure 3). N50 values for strain XM4083 were highly variable depending on the assembly method employed, and A5-miseq yielded the largest N50 value (801,351) (Figure 4). For strain R8603A2, N50 values were much more consistent regardless of assembly method, and Shovill yielded the largest N50 value (267,419) (Figure 4).
Post-filtering. Regardless of assembly method employed, the final genome size of strain XM4083 was approximately 4.0 Mb with a GC content of 68.0%, and the final genome size of strain R8603A2 was approximately 3.3 Mb with a GC content of 70.5% (Figure 2). Significant removal of contigs was necessary for the SPAdes assemblies of both strains (83% of total contigs (84/101) for strain XM4083 and 71% of total contigs (157/220) for strain R8603A2) (Figure 3). Post-filtering, A5-miseq still yielded the fewest contigs for strain XM4083 (16) although SPAdes was not very far off, yielding 17 (Figure 3). The final contig count for strain R8603A2 was very similar for all three assembly methods, and SPAdes now produced the fewest contigs (63) (Figure 3). N50 values did not change from the original assemblies (Figure 4).
Scaffolding. Trimmed and error-corrected 16S rRNA gene sequences were compared against the NCBI BLAST nr/nt database to determine closely-related complete genomes to use as references for scaffolding both Brevibacterium isolates. Brevibacterium sp. strain XM4083 was scaffolded by comparison to six Brevibacterium reference genomes, and yielded six to 12 final scaffolds, with the SPAdes assembly producing the fewest final scaffolds. Strain R8603A2 was compared to two complete reference genomes and yielded 12 to 14 final scaffolds. Both SPAdes- and A5-miseq-assembled contigs yielded 12 final scaffolds.
Prefiltering. The genome size of strain XM4011 varied between approximately 5.9 and 7.5 Mb depending on assembler, although GC content was more consistent, ranging between 72.5 and 72.8% (Table 5, Figure 2). This discrepancy in genome size despite consistency in GC content was indicative of contamination with another actinomycete strain. In fact, a large amount of Micromonospora reads were found to contaminate the genome of strain XM4011. The genome sizes and GC contents of strains XM83C (~ 6.8 Mb and 72.23% GC) and XM4193 (~ 6.1 Mb and 72.0% GC) were much more consistent irrespective of software package (Table 5). Despite these relatively similar genome sizes, contig count varied widely for each genome depending on assembly method (Figure 3). Shovill produced the fewest contigs for strain XM4011 (749), and A5-miseq produced the fewest contigs for strain XM83C (805) and strain XM4193 (55). For all three Streptomyces genomes, SPAdes consistently yielded the highest N50 value (Figure 4).
Post-filtering. Significant contaminant contig removal was necessary for the SPAdes [87% of total contigs (2,842/3,265)] and A5-miseq [56% of total contigs (712/1,268)] assemblies of strain XM4011. The final genome size of strain XM4011 was approximately 5.9 Mb with a GC content of approximately 73% for all assemblers (Figure 2). All three software packages assembled strain XM83C into a genome of approximately 6.8 Mb with a final GC content of 72.2% (Figure 2). Strain XM4193 assembled into a final genome of approximately 6.1 Mb with a GC content of 72.0% with all three methods (Figure 2). Post-filtering of the SPAdes assembly for strain XM4193 required significant removal of contigs [77% of total contigs (83/108)] (Figure 3). Ultimately, SPAdes yielded the fewest contigs as well as the highest N50 values in the final genomes for all three Streptomyces strains (Figures 3, 4).
Scaffolding. Streptomyces sp. strain XM4011 was scaffolded by comparison against the only available closely-related reference genome (Streptomyces harbinensis strain NA02264), found by aligning the trimmed forward 16S rRNA gene fragment of strain XM4011 against the NCBI BLAST nr/nt database. The final genome consisted of seven to 27 scaffolds depending on which assembler data was used, with SPAdes contigs resulting in the fewest scaffolds. Strain XM83C was compared against six reference genomes and yielded 187 to 202 scaffolds, with A5-miseq producing the best final assembly. Unfortunately, Shovill-assembled data for strain XM83C could not be scaffolded with MeDuSa. The exact reason for this failure to scaffold is unknown, as multiple rounds were tested with various sets of reference genomes (one to six), although it should be mentioned that this contig set had the highest number of contigs post-filtering (943), the most for any of the data sets analyzed in this study. Strain XM4193 was compared against five reference genomes and yielded 11 to 20 scaffolds, with SPAdes-assembled data resulting in the fewest scaffolds.
Genomes assembled with all three software packages were analyzed with antiSMASH to identify potential BGCs encoded (Table 6). In general, only BGCs with at least 40% similarity to a known cluster were further considered, although several clusters with less than 40% similarity were still scrutinized if they were identified in other assemblies of the same genome with greater similarity. The majority of BGCs identified were classified as type I PKS, type III PKS, NRPS, terpene, or were characterized as “other”. Many type I PKS and type III PKS were associated with hybrid NRPS clusters. There was no difference in putative BGCs detected between the three assembly methods employed for Micrococcus sp. strain XM4230A, Micrococcus sp. strain XM4230B, Brevibacterium sp. strain XM4083 and Brevibacterium sp. strain R8603A2. Only one putative BGC encoding a carotenoid was identified for both Micrococcus strains and Brevibacterium sp. strain R8603A2. No BGCs with known anti-TB activity were detected in any assemblies of any strains of Micrococcus and Brevibacterium spp. Slight variations were detected among the results for all Streptomyces and Micromonospora strains depending on assembly method employed, although Micromonospora sp. strain XM-20-01 uniquely had the most variability in putative BGCs identified between different assemblies. All Streptomyces strains had the largest number of putative BGCs identified by antiSMASH (four to nine depending on the assembly), with Streptomyces sp. strain XM4193 consistently identifying the most putative clusters, as well as clusters with 100% similarity to known clusters. For all Micrococcus and Brevibacterium sp. analyzed, no BGCs with known anti-TB activity were detected. For all Streptomyces and Micromonospora strains, at least one compound with known anti-TB activity was detected. Inter-assembly BGC analysis with NP.Searcher also yielded very similar cluster detections for all actinomycete genomes analyzed (Table 7).
Table 6 Putative BGCs identified by antiSMASH for actinomycete strains based on assembly method employed.
The highly similar assembly statistics calculated for both Micrococcus isolates as well as the high similarity observed among all Micromonospora strains raised the possibility that these strains are very closely related to each other. It is important to note that although the actinomycetes in the original collection were derived from multiple X.muta samples, both Micrococcus strains were isolated from the same sponge sample, and all four Micromonospora strains were isolated from the same sponge sample. Both Micrococcus spp. strains XM4230A and XM4230B assembled to genomes of approximately 2.5 Mb with a GC content of approximately 72.8% and had identical partial 16S rRNA gene sequences (1353 bp). All four Micromonospora genomes were approximately 6.7 Mb with a GC content of 72.9%. For these four strains, the partial 16S rRNA gene fragments (1355 bp) sequenced with Sanger were identical. As an additional check on identity, the sequences of the housekeeping genes recA and gyrB as annotated by PATRIC were compared. Micrococcus spp. XM4230A and XM4230B had identical sequences for both recA and gyrB. All four Micromonospora spp. strains (XM-20-01, R42003, R42004, R42106) had identical recA and gyrB sequences. ANI values were calculated in pairwise-fashion for all Micromonospora strains. Irrespective of which final genome assembly was used for comparison, all Micromonospora strain comparisons had ANI values > 99.9%, well exceeding the species delineation threshold of 95% (results not shown). Similarly, Micromonospora sp. strain XM4230A had an ANI value > 99.9% compared to strain XM4230B (results not shown). An ANI value of 95% ± 0.5% corresponds to the DNA-DNA hybridization (DDH) species cutoff value of 70% (Goris et al., 2007). As a final proxy measure of identity and similarity, genome dot plots were performed to visualize the alignment of whole genomes of Micrococcus strains in pairwise fashion (Supplementary Figure 1). Similar results were observed for comparisons using all three versions of assembled genomes (only genomes assembled with the same software package were compared to each other for consistency), so only SPAdes-assembled genomes are presented to avoid redundancy. The same plots are presented for all Micromonospora strains as well (Supplementary Figures 2-7). Genome dot plots showed that the two Micrococcus strains and the four Micromonospora strains were highly similar but not identical.
The primary objective of this study was to determine the most efficient method of genome assembly with 250 base paired-end reads that could be applied to the challenging GC-rich actinomycetes with a wide range of genome sizes from various genera. Previous comparative studies on genome assemblers consistently identified SPAdes as generally producing the best assemblies of bacterial genomes, and thus this assembler was used as the focal point in this study, to be compared to more recently developed assembly algorithms (Magoc et al., 2013; Acuña-Amador et al., 2018). Although all three methods are fairly similar, they employ slightly different steps that affect their final output. SPAdes, and thus Shovill, assemble contigs using multi-sized de Bruijn graphs, while A5-miseq contig assembly is performed with the more recently developed IDBA-UD algorithm (IDBA-UD, RRID : SCR_011912) (Peng et al., 2012; Coil et al., 2015). The de Bruijn graph approach still serves as the base of the IDBA-UD algorithm, although it employs a different method for error correction of k-mers based on coverage depth (Bankevich et al., 2012; Peng et al., 2012). SPAdes uses default k-mer sizes of 21, 33, 55, 77, 99 and 127, while Shovill sets the default k-mer sizes for assembly with SPAdes to 31, 55, 79, 103, and 127. A5-miseq and Shovill both work exclusively for paired end Illumina data, while SPAdes has vastly more capabilities, including the ability to support unpaired reads, as well as hybrid assemblies with long read sequencing data. When using SPAdes, users must also be sure to perform an initial trimming step with another software package, such as Trimmomatic, to remove adapters before assembling raw reads. Shovill addresses this issue by incorporating Trimmomatic into its pipeline, albeit with predetermined settings that cannot be manually edited. This makes it very simple to use for the coding novice, but is less desirable for any cases where it is would be advantageous to modify the script. A5-miseq also prescreens raw reads for adapters with Trimmomatic before assembly, and features the option to provide an alternative adapter file if necessary. The developers of the original A5/A5-miseq pipelines assert that the main advantage of their software is the ability to produce quality genomes without any prior knowledge of the genome under assembly or parameter tuning, making this pipeline an enticing option for those with a limited bioinformatics background (Tritt et al., 2012).
Considering the initial assembly before filtering, either A5-miseq or Shovill always provided the fewest contigs per genome, except for Micromonospora sp. strain R42106. In four of the 11 genomes analyzed in this study, A5-miseq provided the fewest contigs pre-filtering, including for one Micrococcus genome and at least one representative for both Streptomyces and Brevibacterium. Shovill also yielded the fewest contigs for six of the 11 genomes analyzed, including for one Micrococcus genome, one Streptomyces genome, one Brevibacterium genome, and all but one Micromonospora genome. This is due to the final steps in the Shovill pipeline, in which minor assembly errors are corrected and any contigs deemed too short, with insufficient coverage (< 2x) or homopolymers, are removed. In many cases, no post-filtering was required of Shovill-assembled contigs. Only in one case was substantial filtering of contigs required post-assembly with Shovill. For Micromonospora sp. strain XM-20-01 data, which was heavily contaminated with fungal DNA, 85% of contigs were removed. A5-miseq also removed regions of mis-assembly, albeit most genomes assembled with this software still required contig filtering post-assembly. Interestingly, no post-processing was necessary for the good quality Micromonospora data (all but strain XM-20-01). On the other hand, SPAdes retained low coverage contigs in its output file, which must later be removed. Because the SPAdes contigs.fasta output file labels each “node” with a k-mer coverage value, identification of poor coverage contigs and subsequent removal is fairly straightforward. Unfortunately, A5-miseq does not provide a coverage value for individual contigs, and it is therefore more difficult to discern short but legitimate contigs from contaminants or erroneous sequences. In this case, judgement calls on filtering are dependent on match identity by comparison to the NCBI BLAST database. Further complicating this process is the unique feature of the A5-miseq assembly in which ambiguous nucleotide codes are included in the output contigs file, which results in underestimates in alignment scores with database entries. This did not significantly disrupt post-filtering for most genomes with good quality data, but in the case of Streptomyces sp. strain XM4011, lack of coverage values and ambiguous nucleotide codes increased the time required to complete filtering and removal. Thus, one should ensure that the DNA sequenced is high quality and devoid of contamination prior to sequencing and assembly. A similar conclusion was drawn by previous studies in which assembly algorithm performance was compared on various data sets and data quality was found to have a greater impact than the particular assembler on the final assembly (Salzberg et al., 2012).
For every genome assembled in this study, SPAdes-assembled contigs required the most filtering post-assembly. However, the ability to modify pipeline options and the fact that this assembler maintains a coverage depth for each contig assembled makes these data much easier to manipulate and correct. Although A5-miseq normally requires minimal post-filtering, the inability to identify coverage depth of individual contigs makes filtering post-assembly more complicated and uncertain. Furthermore, A5-miseq is the only assembler among the three tested that adds strings of Ns into the assembly during scaffolding. These ambiguous sequences inflate the total genome size and, similar to the ambiguous nucleotide codes, they make it more difficult to accurately align assembled contigs with sequences in the BLAST database. Shovill provides individual coverage depth values for each contig assembled, enabling easier manipulation of post-assembly results. Despite the fact that no post-assembly filtering was normally needed for Shovill-assembled genomes, this software package rarely produced the best assembly in terms of final contig count and N50 value. It is interesting to note that for the “good quality” Micromonospora data (all but strain XM-20-01), A5-miseq assemblies required absolutely no post-assembly filtering, and Shovill assemblies required minimal to no filtering (only one contig was removed from the Shovill assembly for genome Micromonospora sp. strain R42003). However, based on the results, no correlations between genome assembler performance and bacterial genome size/actinomycete genus were observed. The most consistent observation was that in eight of the 11 genomes analyzed, SPAdes ultimately produced the best genome assembly when evaluated with contigs and N50 value as metrics. Only in the case of Brevibacterium sp. strain R8603A2 did SPAdes yield the fewest final contigs (best assembly) while Shovill-assembled data retained the slightly higher N50 value. N50 values varied for certain genomes by assembler employed but were not affected by filtering procedures. The exception is Micromonospora sp. strain XM-20-01, which was heavily contaminated with fungal contigs. Ultimately, this highlights the importance of the manual post-filtering required for SPAdes-assembled data, the main step distinguishing SPAdes from Shovill.
In theory, both A5-miseq and Shovill require no additional processing of raw data pre- or post-assembly, but as evident from this study, that is not always the case. Even if very high quality pure DNA is extracted and used for sequencing, contamination in not uncommon from the sequencing process itself. For instance, in several cases, contigs aligning to the blue crab Callinectes sapidus genome and barley Hordeum vulgare, both organisms known to be sequenced by the same sequencing laboratory, were identified among contigs assembled by A5-miseq that had to subsequently be removed from the final assembly. Contaminant identification was not consistent among assemblers. In some instances, what appeared to be spillover contigs from other actinomycetes sequenced in the same Illumina MiSeq run were detected. These spillover contigs were most easily detected through SPAdes-generated assemblies, often marked by short contig length (usually 1000 bp or less) and low coverage (< 2x). This contamination became more difficult to detect when trying to determine spillover contigs among genomes of the same genus. Multiple Micromonospora strains were sequenced in the same run, so to determine which Micromonospora isolate small contigs likely belonged to, they were aligned to all contigs assembled from all Micromonospora genomes sequenced at the same time. If a contig aligned to the assembly of another Micromonospora strain with high percent similarity (at least 90%) and to a node with significantly higher coverage, it was considered a spillover contig and removed. This decision was justified based on the fact that this process ensured that the ambiguous sequence would be retained in at least one other Micromonospora assembly, guaranteeing that it would not be overlooked during BGC analysis. Spillover contigs were virtually absent from Shovill assemblies, due to this assembler’s aforementioned post-processing filtering step.
One caveat of the conclusions in this study is that no complete reference genomes were available for any of these strains, as they are all novel isolates. Previous comparative analyses have used reference genomes of closely related species to evaluate assembly correctness, but they acknowledge that true differences existing between the sequenced genome and reference may be considered errors by this method (Magoc et al., 2013). Therefore, assemblies were not compared to any closely-related genomes. Mis-joins including relocations, translocations, inversions, as well as indels and unnecessarily duplicated or compressed repeats could not be identified as a result. Of course, long-read technology remains the superior method for complete and accurate genome assembly.
Despite the deeper coverage provided with sequencing short reads, it is not always possible to resolve repeat regions (often longer than the maximum read length) in a fragmented final assembly, as evidenced by the data presented in this study. In bacteria, an estimated 5-10% of the genome consists of genomic repeats (Hofnung and Shapiro, 1999; Parkhill et al., 2000; Shapiro and Von Sternberg, 2005). Further assembly of contigs into scaffolds was attempted using the web interface for MeDuSa. In every case, MeDuSa was able to use genomes of closely related strains to join contigs. It should be noted that in repeated scaffolding attempts on a particular assembly with comparison to the same set of reference genomes, the final results varied slightly. When a gap was determined to be present between two contigs, a string of 100 Ns was inserted between them. Therefore, repeated scaffolding on the same genome resulted in slightly different scaffold counts every time, with a genome size varying by 100n bases, where n is difference in amount of N strings inserted. This scaffolding technique is advantageous based on the fact that it enables better understanding of how the contigs are linked together, but still leaves an unknown regarding accurate genome size. The gap regions were often flanked by repeat sequences, confirming the universally poor performance of assemblers in reconstructing repeat regions from short reads.
The frequency with which repeat regions were observed to flank assembly breaks was reflected in the tendency of the BGCs identified by antiSMASH to be located on contig edges. Overall, no major differences were observed in the BGCs identified for a particular genome between the different assembly methods. Still, in 22 of the 33 assemblies analyzed, at least 50% of all the BGCs identified (including those below the 40% similarity cutoff threshold) fell on contig edges. In the Shovill assembly of Streptomyces sp. strain XM83C, 100% of the 33 putative BGCs identified were located on contig edges. For every assembly of every Micromonospora strain analyzed, at least 50% of the BGCs identified were located on contig edges, including the BGCs for ECO-02301, alkyl-O-dihydrogeranyl-methoxyhydroquinone, oligomycin, methymicin, diazepinomicin, and griseochelin. This explains why in some cases a BGC was identified above the 40% cutoff threshold in one assembly but below the cutoff in another (ex. alkyl-O-dihydrogeranyl-methoxyhydroquinone and ECO-02301). It is likely that Micromonospora sp. strain XM-20-01 also contains the diazaquinomycin H/J cluster, as opposed to diazepinomicin, since the latter BGC falls on a contig edge and they share genes. Due to the high percent similarity with the diazaquinomycin H/J cluster, it is very likely that a chemical analogue is responsible for the anti-TB activity observed for all Micromonospora extracts. Despite the fact that antiSMASH did not detect 6% of the cluster, the possibility cannot be ruled out that the BGC domains are arranged differently in these genomes so that the entire cluster does not fall on one single contig (modular arrangement), and that these strains do in fact contain the entire BGC for diazaquinomycin H/J. Likewise, it is possible that the Streptomyces sp. strain XM4011 genome contains the BGCs with greater % similarity than reported to the known anti-TB compounds valinomycin (40%) and ecumicin (52%), both of which are located on contig edges and thus possibly unresolved. Desferrioxamine B putatively identified by antiSMASH with 66% similarity is also on a contig edge and may in fact be the true compound effecting growth inhibition of M. tb. The complete BGC for desferrioxamine E was identified in every genome assembly of Streptomyces sp. strain XM4193 and is the only compound with known anti-TB activity identified for this strain. However it cannot be ruled out that this strain produces another novel compound with anti-TB activity that was not detected by antiSMASH. For Brevibacterium sp. strain XM4083, no BGCs were reported to be located on contig edges in the A5-miseq or Shovill assemblies, and only one putative BGC fell on a contig edge in the SPAdes assembly, although for a cluster that did not meet the cutoff threshold (no % similarity was provided).
Since antiSMASH did not identify any BGCs in any of the Micrococcus or Brevibacterium genomes related to BCGs previously reported to encode compounds that inhibit M.tb, it is likely that these strains produce novel compounds with anti-mycobacterial activity (or at least compounds not in the MIBiG database). Although mainly studied for their antioxidant and anticancer properties, there are at least three known carotenoids with anti-TB activity. One compound, fucoxanthin, is a marine-derived orange xanthophyll produced by both brown seaweed and diatoms rather than a bacterium (Peng et al., 2011; Šudomová et al., 2019). Fucoxanthin is highly abundant in the marine environment and is estimated to contribute more than 10% of total carotenoid production (Liaaen-Jensen, 1978; Viera et al., 2018). The other two carotenoids, flexirubin [a yellow-orange pigment isolated from Flavobacterium sp. Ant342 (F-YOP)] and violacein [a violet purple pigment isolated from Janthinobacterium sp. Ant5-2 (J-PVP)], originate from a freshwater lake in Antarctica (Mojib et al., 2010). Violacein, isolated from bacteria including Chromobacterium violaceum, has shown antimycobacterial activity (de Souza et al., 1999; Durán & Menck, 2001; Mojib et al., 2010). Fucoxanthin targets M. tb by interfering with cell wall biosynthesis (Šudomová et al., 2019). The mechanism of action of flexirubin and violacein remain undocumented, although one study notes that the antibacterial mechanism of violacein against methicillin-resistant Staphylococcus aureus (MRSA) differs from that of other common antibiotics (Choi et al., 2015; Choi et al., 2021). Furthermore, studies show that fatty acid-carotenoid complexes isolated from the microalga Chlorella vulgaris, composed of oleic acid or lineolic acid and various carotenoids including canthaxanthin, neoxanthin, cryptoxanthin and echinenone, can act as potent therapeutic agents against multi-drug resistant strains of M. tb (Kumar et al., 2020). Essential genes for the biosynthesis of fucoxanthin were not detected in any Micrococcus or Brevibacterium genomes by any genome mining tools nor by manual investigation of the PATRIC annotation output. The BGCs for violacein and flexirubin are in the MIBiG database, and neither were detected by antiSMASH. Further genomic analysis with long-read sequencing technology for all of these genomes with unresolved BGCs, as well as chemical analysis of extracts would provide much more critical information and is necessary to determine exactly what compounds these strains are producing that could inhibit M. tb.
Although the genomes of Micrococcus sp. strains XM4230A and XM4230B were almost identical and the four Micromonospora genomes were almost identical, all these genomes were retained and carefully analyzed in this study. Similar to the results reported by Antony-Babu et al. (2017), the antiSMASH results were not identical among the Micromonospora assemblies, possible indicating that they retain different metabolic profiles. Assembling the genomes of what we now know to be highly similar strains with the three assemblers served to validate algorithm correctness. The highly similar results obtained for assembly statistics and BGC identification confirm that each assembly method employed was fairly precise, but point out slight differences in contig assembly, as reflected by antiSMASH results.
This is the first study to compare the effectiveness of various short-read de novo bacterial genome assemblers specifically for actinomycete strains with very high GC content. Although a side-by-side comparison of SPAdes and A5-miseq, among other assemblers, was performed by Acuña-Amador et al. (2018), no studies specifically assess their performance in assembling genomes of high-GC content bacteria, such as the marine actinomycetes that are of great interest for natural products discovery. Past studies have observed that regions of high GC-bias, (either GC-rich or GC-poor) tend to have low coverage of reads, which in turn contributes to assembly breaks and reduces assembly completeness (Chen et al., 2013; Browne et al., 2020). This issue was observed for all assemblies analyzed in the previous study. A recently developed assembly algorithm (dnaasm) claims to properly assemble regions of tandem repeats and maintain the ability to restore repetitive regions of the genome covered by only a single read (Kuśmirek and Nowak, 2018). The dnaasm algorithm uses the relative frequency of reads to reconstruct tandem repeats. Unfortunately, the varied read coverage characteristic of genomes with high GC content means that this method is likely to be insufficient to completely resolve assembly breaks in actinomycete genomes. We conclude that when only short-read sequencing data is available for genomes with high GC content, employing SPAdes with a pre-assembly trimming step and post-assembly manual filtering ultimately yielded the most complete assemblies for BGC analysis.
The datasets presented in this study can be found in online repositories at https://www.ncbi.nlm.nih.gov/genbank/. The accession numbers are as follows: Streptomyces sp. XM4193 (JALLGK000000000), Streptomyces sp. XM83C (JALLGL000000000), Streptomyces sp. XM4011 (JALLGM000000000), Brevibacterium sp. R8603A2 (JALLGN000000000), Brevibacterium sp. XM4083 (VFYR00000000), Micromonospora sp. XM-20-01 (VFYQ00000000), Micromonospora sp. R42106 (JALLGO000000000), Micromonospora sp. R42004 (JALLGP000000000), Micromonospora sp. R42003 (JALLGQ000000000), Micrococcus sp. XM4230B (JALLGR000000000), Micrococcus sp. XM4230A (JALLGS000000000) (also provided in Supplementary data sheet (https://www.frontiersin.org/articles/10.3389/fmars.2022.914197/full#supplementary-material).
DT: experimental procedures, data analysis, writing. TB: bioinformatics support and troubleshooting, review, and editing. RH: conceptualization of microbiology methodology, supervision, review, and editing. All authors contributed to the article and approved the submitted version.
This work was supported by funding from the NIST-IMET Graduate Fellowship in Environmental Biotechnology, the University of Maryland College Park College of Mathematical and Natural Sciences Dean’s Fellowship, Ratcliffe Environmental Entrepreneur Fellowship, the Chateaubriand Fellowship STEM, and the American Association of University Women’s American Dissertation Fellowship.
We are grateful to the late Dr. Mark Shirtliff for providing the strain of Mycobacterium tuberculosis used in this study and we thank the BioAnalytical Services Laboratory at the Institute of Marine and Environmental Technology for performing sequencing.
The authors declare that the research was conducted in the absence of any commercial or financial relationships that could be construed as a potential conflict of interest.
All claims expressed in this article are solely those of the authors and do not necessarily represent those of their affiliated organizations, or those of the publisher, the editors and the reviewers. Any product that may be evaluated in this article, or claim that may be made by its manufacturer, is not guaranteed or endorsed by the publisher.
The Supplementary Material for this article can be found online at: https://www.frontiersin.org/articles/10.3389/fmars.2022.914197/full#supplementary-material
Abdjul D. B., Yagi A., Yamazaki H., Kirikoshi R., Takahashi O., Namikoshi M., et al. (2018). Anti-mycobacterial haliclonadiamine alkaloids from the okinawan marine sponge haliclona sp. collected at iriomote island. Phytochem. Lett. 26, 130–133. doi: 10.1016/j.phytol.2018.05.028
Acuña-Amador L., Primot A., Cadieu E., Roulet A., Barloy-Hubler F. (2018). Genomic repeats, misassembly and reannotation: A case study with long-read resequencing of porphyromonas gingivalis reference strains. BMC Genomics 19 (1), 64. doi: 10.1186/s12864-017-4429-4
Akram S., Aboobacker S. (2021). “Mycobacterium marinum,” in StatPearls (Treasure Island, FL: StatPearls Publishing). doi: 10.1016/S1294-5501(05)80179-7
Altschul S. F., Madden T. L., Schäffer A. A., Zhang J., Zhang Z., Miller W., et al. (1997). Gapped BLAST and PSI-BLAST: A new generation of protein database search programs. Nucleic Acids Res. 25 (17), 3389–3402. doi: 10.1093/NAR/25.17.3389
Antony-Babu S., Stien D., Eparvier V., Parrot D., Tomasi S., Suzuki M. T. (2017). Multiple streptomyces species with distinct secondary metabolomes have identical 16S rRNA gene sequences. Sci. Rep. 7 (1), 1–8. doi: 10.1038/s41598-017-11363-1
Baltz R. H. (2005). Antibiotic discovery from actinomycetes: Will a renaissance follow the decline and fall? SIM. News 55, 186–196.
Bankevich A., Nurk S., Antipov D., Gurevich A. A., Dvorkin M., Kulikov A. S., et al. (2012). SPAdes: A new genome assembly algorithm and its applications to single-cell sequencing. J. Comput. Biol. 19 (5), 455. doi: 10.1089/CMB.2012.0021
Bellassi P., Cappa F., Fontana A., Morelli L. (2020). Phenotypic and genotypic investigation of two representative strains of microbacterium species isolated from micro-filtered milk: Growth capacity and spoilage-potential assessment. Front. Microbiol. 11. doi: 10.3389/FMICB.2020.554178/FULL
Benjamini Y., Speed T. P. (2012). Summarizing and correcting the GC content bias in high-throughput sequencing. Nucleic Acids Res. 40 (10), e72–e72. doi: 10.1093/NAR/GKS001
Blackwell G. A., Hunt M., Malone K. M., Lima L., Horesh G., Alako B. T. F., et al. (2021). Exploring bacterial diversity via a curated and searchable snapshot of archived DNA sequences. PloS Biol. 19 (11), e3001421. doi: 10.1371/journal.pbio.3001421
Blin K., Shaw S., Steinke K., Villebro R., Ziemert N., Lee S. Y., et al. (2019). AntiSMASH 5.0: Updates to the secondary metabolite genome mining pipeline. Nucleic Acids Res. 47 (W1), W81–W87. doi: 10.1093/nar/gkz310
Brettin T., Davis J. J., Disz T., Edwards R. A., Gerdes S., Olsen G. J, et al (2015). RASTtk: A modular and extensible implementation of the RAST algorithm for building custom annotation pipelines and annotating batches of genomes. Scientific Reports 5, 8365. doi: 10.1038/SREP08365
Bolger A. M., Lohse M., Usadel B. (2014). Trimmomatic: a flexible trimmer for illumina sequence data. Bioinformatics 30 (15), 2114–2120. doi: 10.1093/BIOINFORMATICS/BTU170
Bosi E., Donati B., Galardini M., Brunetti S., Sagot M. F., Lió P., et al. (2015). MeDuSa: A multi-draft based scaffolder. Bioinformatics 31 (15), 2443–2451. doi: 10.1093/bioinformatics/btv171
Browne P. D., Nielsen T. K., Kot W., Aggerholm A., Gilbert M. T. P., Puetz L., et al. (2020). GC bias affects genomic and metagenomic reconstructions, underrepresenting GC-poor organisms. GigaScience 9 (2), giaa008. doi: 10.1093/gigascience/giaa008
Cabanettes F., Klopp C. (2018). D-GENIES: Dot plot large genomes in an interactive, efficient and simple way. PeerJ 6, e4958. doi: 10.7717/peerj.4958
Chen Y. C., Liu T., Yu C. H., Chiang T. Y., Hwang C. C. (2013). Effects of GC bias in next-generation-sequencing data on de novo genome assembly. PloS One 8 (4), 62856. doi: 10.1371/JOURNAL.PONE.0062856
Choi S. Y., Kim S., Lyuck S., Kim S. B., Mitchell R. J. (2015). High-level production of violacein by the newly isolated duganella violaceinigra str. NI28 and its impact on staphylococcus aureus. Sci. Rep. 5, 15598. doi: 10.1038/SREP15598
Choi S. Y., Lim S., Yoon K., Lee J. I., Mitchell R. J. (2021). Biotechnological activities and applications of bacterial pigments violacein and prodigiosin. J. Biol. Eng. 15 (1), 1–16. doi: 10.1186/S13036-021-00262-9
Coil D., Jospin G., Darling A. E. (2015). A5-miseq: An updated pipeline to assemble microbial genomes from illumina MiSeq data. Bioinformatics 31 (4), 587–589. doi: 10.1093/bioinformatics/btu661
Davis J. J., Wattam A. R., Aziz R. K., Brettin T., Butler R., Butler R. M., et al. (2020). The PATRIC bioinformatics resource center: expanding data and analysis capabilities. Nucleic Acids Res. 48 (D1), D606–D612. doi: 10.1093/NAR/GKZ943
de Oliveira J. A. M., Williams D. E., Bonnett S., Johnson J., Parish T., Andersen R. J. (2020). Diterpenoids isolated from the Samoan marine sponge chelonaplysilla sp. inhibit mycobacterium tuberculosis growth. J. Antibiot. 73 (8), 568–573. doi: 10.1038/s41429-020-0315-4
de Souza A., Aily D., Sato D., Durán N. (1999). Atividade da violaceína in vitro sobre o mycobacterium turbeculosis H37RA. Rev. Do. Instituto Adolfo Lutz 58 (1), 59–62. doi: 10.53393/RIAL.1999.V58.36676
Durán N., Menck C. F. M. (2001). Chromobacterium violaceum: A review of pharmacological and industiral perspectives. Crit. Rev. Microbiol. 27 (3), 201–222. doi: 10.1080/20014091096747
Durrell K., Prins A., Le Roes-Hill M. (2017). Draft genome sequence of gordonia lacunae BS2T. Genome Announcements 5 (40), 959–976. doi: 10.1128/GENOMEA.00959-17
Egidi E., Wood J. L., Fox E. M., Liu W., Franks A. E. (2017). Draft genome sequence of leifsonia sp. strain NCR5, a rhizobacterium isolated from cadmium-contaminated soil. Genome Announcements 5 (23), e00520-17. doi: 10.1128/GENOMEA.00520-17
Goodfellow M., Williams S. T. (1983). Ecology of actinomycetes. Annu. Rev. Microbiol. 37, 189–216. doi: 10.1146/annurev.mi.37.100183.001201
Goris J., Konstantinidis K. T., Klappenbach J. A., Coenye T., Vandamme P., Tiedje J. M. (2007). DNA-DNA Hybridization values and their relationship to whole-genome sequence similarities. Int. J. Systemat. Evolution. Microbiol. 57 (1), 81–91. doi: 10.1099/IJS.0.64483-0/CITE/REFWORKS
Gurevich A., Saveliev V., Vyahhi N., Tesler G. (2013). QUAST: quality assessment tool for genome assemblies. Bioinformatics 29 (8), 1072–1075. doi: 10.1093/BIOINFORMATICS/BTT086
Hechtel G. J. (1983). New species of marine demospongiae from Brazil. Iheringia. Série Zoologia. 63, 59–89.
Hentschel U., Fieseler L., Wehrl M., Gernert C., Steinert M., Hacker J., et al. (2003). Microbial diversity of marine sponges. Prog. Mol. subcel. Biol. 37, 59–88. doi: 10.1007/978-3-642-55519-0_3
Hentschel U., Hopke J., Horn M., Friedrich A. B., Wagner M., Hacker J., et al. (2002). Molecular evidence for a uniform microbial community in sponges from different oceans. Appl. Environ. Microbiol. 68 (9), 4431–4440. doi: 10.1128/AEM.68.9.4431-4440.2002
Hentschel U., Usher K. M., Taylor M. W. (2006). Marine sponges as microbial fermenters. FEMS Microbiol. Ecol. 55 (2), 167–177. doi: 10.1111/j.1574-6941.2005.00046.x
Hill M., Hill A., Lopez N., Harriott O. (2006). Sponge-specific bacterial symbionts in the Caribbean sponge, chondrilla nucula (Demospongiae, chondrosida). Mar. Biol. 148 (6), 1221–1230. doi: 10.1007/s00227-005-0164-5
Hofnung M., Shapiro J. A. (1999). Introduction. Res. Microbiol. 150 (9-10), 577–578. doi: 10.1016/s0923-2508(99)00133-3
Hooper J. N. A., van Soest R. W. M. (2002). “Systema porifera. a guide to the classification of sponges,” in Systema porifera: A guide to the classification of sponges. Eds. Hooper J. N. A., van Soest R. W. M. (Dordrecht: Kluwer Academic/Plenum Publishers), pp. 1–7. doi: 10.1007/978-1-4615-0747-5_1
Hug J. J., Krug D., Müller R. (2020). Bacteria as genetically programmable producers of bioactive natural products. Nat. Rev. Chem. 4 (4), 172–193. doi: 10.1038/s41570-020-0176-1
Hyman R. W., Fukushima M., Diamond L., Kumm J., Giudice L. C., Davis R. W. (2005). Microbes on the human vaginal epithelium. Proc. Natl. Acad. Sci. United States America 102 (22), 7952–7957. doi: 10.1073/pnas.0503236102
Izumi H., Gauthier M. E. A., Degnan B. M., Ng Y. K., Hewavitharana A. K., Shaw P. N., et al. (2010). Diversity of mycobacterium species from marine sponges and their sensitivity to antagonism by sponge-derived rifamycin-synthesizing actinobacterium in the genus salinispora. FEMS Microbiol. Lett. 313 (1), 33–40. doi: 10.1111/j.1574-6968.2010.02118.x
Kerr R. G., Kelly-Borges M. (1994). “Biochemical and morphological heterogeneity in the Caribbean sponge xestospongia muta (Petrosida: Petrosiidae),” in Sponges in time and space Biology, Chemistry, Paleontology: proceedings of the 4th International Porifera Congress, Amsterdam, Netherlands. Eds. van Soest R. W. M., van Kempen T. M. G., Braekman J. C. (Rotterdam; Brookfield, VT: Balkema), 65–73.
Kim T. K., Hewavitharana A. K., Shaw P. N., Fuerst J. A. (2006). Discovery of a new source of rifamycin antibiotics in marine sponge actinobacteria by phylogenetic prediction. Appl. Environ. Microbiol. 72 (3), 2118–2125. doi: 10.1128/AEM.72.3.2118-2125.2006
Kincheloe G. N., Eisen J. A., Coil D. A. (2017). Draft genome sequence of arthrobacter sp. strain UCD-GKA (Phylum actinobacteria). Genome Announcements 5 (6), e01599-16. doi: 10.1128/GENOMEA.01599-16
Klein B. A., Lemon K. P., Faller L. L., Jospin G., Eisen J. A., Coil D. A. (2016). Draft genome sequence of curtobacterium sp. strain UCD-KPL2560 (Phylum actinobacteria) (4(5: Genome Announcements). doi: 10.1128/GENOMEA.01040-16
Koenigsaecker T. M., Eisen J. A., Coil D. A. (2016). Draft genome sequence of gordonia sp. strain UCD-TK1 (Phylum Actinobacteria). Genome Announcements 4 (5), 1121–1137. doi: 10.1128/GENOMEA.01121-16
Kuśmirek W., Nowak R. (2018). De novo assembly of bacterial genomes with repetitive DNA regions by dnaasm application. BMC Bioinf. 19 (1), 1–10. doi: 10.1186/S12859-018-2281-4/TABLES/6
Kumar T. S., Josephine A., Sreelatha T., Azger Dusthackeer V. N., Mahizhaveni B., Dharani G., et al. (2020). Fatty acids-carotenoid complex: An effective anti-TB agent from the chlorella growth factor-extracted spent biomass of chlorella vulgaris. J. Ethnopharmacol. 249, 112392. doi: 10.1016/J.JEP.2019.112392
Kurtz S., Phillippy A., Delcher A. L., Smoot M., Shumway M., Antonescu C., et al. (2004). Versatile and open software for comparing large genomes. Genome Biol. 5 (2), 1–9. doi: 10.1186/GB-2004-5-2-R12/FIGURES/3
Lee O. O., Wang Y., Yang J., Lafi F. F., Al-Suwailem A., Qian P.-Y. (2010). Pyrosequencing reveals highly diverse and species-specific microbial communities in sponges from the red Sea. ISME J. 5 (4), 650–664. doi: 10.1038/ismej.2010.165
Liaaen-Jensen S. (1978). “Marine carotenoids,” in Marine natural products. chemical and biological perspectives, vol. 2 . Ed. Scheuer P. J. (Academic Press), 1–73. Academic Press,:New York
Liu W., Li L., Khan M. A., Zhu F. (2012). Popular molecular markers in bacteria. Mol. Genet. Microbiol. Virol. 27 (3), 103–107. doi: 10.3103/S0891416812030056
Li M. H. T., Ung P. M. U., Zajkowski J., Garneau-Tsodikova S., Sherman D. H. (2009). Automated genome mining for natural products. BMC Bioinf. 10, 185. doi: 10.1186/1471-2105-10-185
Magoc T., Pabinger S., Canzar S., Liu X., Su Q., Puiu D., et al. (2013). GAGE-b: An evaluation of genome assemblers for bacterial organisms. Bioinformatics 29 (14), 1718. doi: 10.1093/BIOINFORMATICS/BTT273
Martín J. F., Liras P. (1989). Organization and expression of genes involved in the biosynthesis of antibiotics and other secondary metabolites. Annu. Rev. Microbiol. 43, 173–206. doi: 10.1146/annurev.mi.43.100189.001133
McMurray S. E., Blum J. E., Pawlik J. R. (2008). Redwood of the reef: Growth and age of the giant barrel sponge xestospongia muta in the Florida keys. Mar. Biol. 155 (2), 159–171. doi: 10.1007/S00227-008-1014-Z
Medema M. H., Blin K., Cimermancic P., De Jager V., Zakrzewski P., Fischbach M. A., et al. (2011). antiSMASH: Rapid identification, annotation and analysis of secondary metabolite biosynthesis gene clusters in bacterial and fungal genome sequences. Nucleic Acids Res. 39, W339-46. doi: 10.1093/NAR/GKR466
Medema M. H., Fischbach M. A. (2015). Computational approaches to natural product discovery. Nat. Chem. Biol. 11 (9), 639. doi: 10.1038/NCHEMBIO.1884
Medema M. H., Kottmann R., Yilmaz P., Cummings M., Biggins J. B., Blin K., et al. (2015). Minimum information about a biosynthetic gene cluster. Nat. Chem. Biol. 11 (9), 625–631. doi: 10.1038/nchembio.1890
Mojib N., Philpott R., Huang J. P., Niederweis M., Bej A. K. (2010). Antimycobacterial activity in vitro of pigments isolated from Antarctic bacteria. Antonie Van Leeuwenhoek 98 (4), 531–540. doi: 10.1007/s10482-010-9470-0
Montalvo N. F., Hill R. T. (2011). Sponge-associated bacteria are strictly maintained in two closely related but geographically distant sponge hosts. Appl. Environ. Microbiol. 77 (20), 7207–7216. doi: 10.1128/AEM.05285-11
Montalvo N. F., Mohamed N. M., Enticknap J. J., Hill R. T. (2005). Novel actinobacteria from marine sponges. Antonie van Leeuwenhoek Int. J. Gen. Mol. Microbiol. 87 (1), 29–36. doi: 10.1007/s10482-004-6536-x
Parkhill J., Achtman M., James K. D., Bentley S. D., Churcher C., Klee S. R., et al. (2000). Complete DNA sequence of a serogroup a strain of neisseria meningitidis Z2491. Nature 404 (6777), 502–506. doi: 10.1038/35006655
Parks D. H., Imelfort M., Skennerton C. T., Hugenholtz P., Tyson G.W (2015). Assessing the quality of microbial genomes recovered from isolates, single cells, and metagenomes. Genome Research 25 (7), 1043–1055. doi: 10.1101/gr.186072.114
Peng Y., Leung H. C. M., Yiu S. M., Chin F. Y. L. (2012). IDBA-UD: A de novo assembler for single-cell and metagenomic sequencing data with highly uneven depth. Bioinformatics 28 (11), 1420–1428. doi: 10.1093/BIOINFORMATICS/BTS174
Peng J., Yuan J. P., Wu C. F., Wang J. H. (2011). Fucoxanthin, a marine carotenoid present in brown seaweeds and diatoms: Metabolism and bioactivities relevant to human health. Mar. Drugs 9 (10), 1806. doi: 10.3390/MD9101806
Pyle M. M. (1947). “Relative numbers of resistant tubercle bacilli in sputa of patients before and during treatment with streptomycin,” in Proceedings of the Staff Meetings of the Mayo Clinic, Vol. 22. 465–473.
Rajwani R., Ohlemacher S. I., Zhao G., Liu H.-B., Bewley C. A. (2021). Genome-guided discovery of natural products through multiplexed low-coverage whole-genome sequencing of soil actinomycetes on Oxford nanopore flongle. MSystems 6 (6), e0102021. doi: 10.1128/msystems.01020-21
Reiswig H. M. (1981). Partial carbon and energy budgets of the bacteriosponge verohgia fistularis (Porifera: Demospongiae) in Barbados. Mar. Ecol. 2 (4), 273–293. doi: 10.1111/J.1439-0485.1981.TB00271.X
Richter M., Rosselló-Móra R., Oliver Glöckner F., Peplies J. (2016). JSpeciesWS: A web server for prokaryotic species circumscription based on pairwise genome comparison. Bioinformatics 32 (6), 929–931. doi: 10.1093/BIOINFORMATICS/BTV681
Rossi F., Dellaglio F., Torriani S. (2006). Evaluation of recA gene as a phylogenetic marker in the classification of dairy propionibacteria. Systemat. Appl. Microbiol. 29 (6), 463–469. doi: 10.1016/J.SYAPM.2006.01.001
Sabarathnam B., Manilal A., Sujith S., Kiran G. S., Selvin J., Thomas A., et al. (2010). Role of sponge associated actinomycetes in the marine phosphorus biogeochemical cycles. American-Eurasian J. Agric. Environmental. Sci. 8 (3), 253–256.
Salzberg S. L., Phillippy A. M., Zimin A., Puiu D., Magoc T., Koren S., et al. (2012). GAGE: A critical evaluation of genome assemblies and assembly algorithms. Genome Res. 22 (3), 557. doi: 10.1101/GR.131383.111
Sasoh M., Masai E., Ishibashi S., Hara H., Kamimura N., Miyauchi K., et al. (2006). Characterization of the terephthalate degradation genes of comamonas sp. strain E6. Appl. Environ. Microbiol. 72 (3), 1825–1832. doi: 10.1128/AEM.72.3.1825-1832.2006
Schatz A., Bugie E., Waksman S. A. (1944). Streptomycin, a substance exhibiting antibiotic activity against gram-positive and gram-negative bacteria. Proc. Soc. Exp. Biol. Med. 55 (1), 66–69. doi: 10.3181/00379727-55-14461
Schmitt S., Tsai P., Bell J., Fromont J., Ilan M., Lindquist N., et al. (2012). Assessing the complex sponge microbiota: Core, variable and species-specific bacterial communities in marine sponges. ISME J. 6 (3), 564–576. doi: 10.1038/ismej.2011.116
Schorn M. A., Alanjary M. M., Aguinaldo K., Korobeynikov A., Podell S., Patin N., et al. (2016). Sequencing rare marine actinomycete genomes reveals high density of unique natural product biosynthetic gene clusters. Microbiology 162 (12), 2075. doi: 10.1099/MIC.0.000386
Seemann T. (2020) Shovill. Available at: https://github.com/tseemann/shovill.
Shapiro J. A., Von Sternberg R. (2005). Why repetitive DNA is essential to genome function. Biol. Rev. Cambridge Philos. Soc. 80 (2), 227–250. doi: 10.1017/S1464793104006657
Soldatou S., Eldjárn G. H., Ramsay A., van der Hooft J. J. J., Hughes A. H., Rogers S., et al. (2021). Comparative metabologenomics analysis of polar actinomycetes. Mar. Drugs 19 (2), 103. doi: 10.3390/MD19020103
Šudomová M., Shariati M. A., Echeverría J., Berindan-Neagoe I., Nabavi S. M., Hassan S. T. S. (2019). A microbiological, toxicological, and biochemical study of the effects of fucoxanthin, a marine carotenoid, on mycobacterium tuberculosis and the enzymes implicated in its cell wall: A link between mycobacterial infection and autoimmune diseases. Mar. Drugs 17 (11), 641. doi: 10.3390/MD17110641
Tarlachkov S. V., Starodumova I. P., Dorofeeva L. V., Prisyazhnaya N. V., Roubtsova T. V., Chizhov V. N., et al. (2021). Draft genome sequences of 28 actinobacteria of the family microbacteriaceae associated with nematode-infected plants. Microbiol. Res. Announcements 10 (9), e01400-20. doi: 10.1128/MRA.01400-20
Taylor M. W., Radax R., Steger D., Wagner M. (2007). Sponge-associated microorganisms: Evolution, ecology, and biotechnological potential. Microbiol. Mol. Biol. Rev. 71 (2), 295–347. doi: 10.1128/mmbr.00040-06
Taylor M. W., Schupp P. J., Dahllöf I., Kjelleberg S., Steinberg P. D. (2004). Host specificity in marine sponge-associated bacteria, and potential implications for marine microbial diversity. Environ. Microbiol. 6 (2), 121–130. doi: 10.1046/j.1462-2920.2003.00545.x
Taylor M. W., Schupp P. J., De Nys R., Kjelleberg S., Steinberg P. D. (2005). Biogeography of bacteria associated with the marine sponge cymbastela concentrica. Environ. Microbiol. 7 (3), 419–433. doi: 10.1111/j.1462-2920.2004.00711.x
Tritt A., Eisen J. A., Facciotti M. T., Darling A. E. (2012). An integrated pipeline for de novo assembly of microbial genomes. PloS One 7 (9), e42304. doi: 10.1371/JOURNAL.PONE.0042304
Vacelet J. (1975). Étude en microscopie électronique de l’association entre bactéries et spongiaires du genre Verongia (Dictyoceratida). J. Microsc. Biol. Cell. 23, 271–88.
Vacelet J., Donadey C. (1977). Electron microscope study of the association between some sponges and bacteria. J. Exp. Mar. Biol. Ecol. 30 (3), 301–314. doi: 10.1016/0022-0981(77)90038-7
van der Meij A., Worsley S. F., Hutchings M. I., van Wezel G. P. (2017). Chemical ecology of antibiotic production by actinomycetes. FEMS Microbiol. Rev. 41 (3), 392–416. doi: 10.1093/femsre/fux005
Van Lanen S. G., Shen B. (2006). Microbial genomics for the improvement of natural product discovery. Curr. Opin. Microbiol. 9 (3), 252–260. doi: 10.1016/j.mib.2006.04.002
van Soest R. W. M. (1980). “Marine sponges from curaçao and other Caribbean localities part II. haplosclerida,” in Studies on the fauna of curaçao and other Caribbean islands, vol. 62, 1–173.
Viera I., Pérez-Gálvez A., Roca M. (2018). Bioaccessibility of marine carotenoids. Mar. Drugs 16 (10), 397. doi: 10.3390/MD16100397
Ward A. C., Allenby N. E. (2018). Genome mining for the search and discovery of bioactive compounds: the streptomyces paradigm. FEMS Microbiol. Lett. 365 (24), fny240. doi: 10.1093/FEMSLE/FNY240
Webster N. S., Hill R. T. (2001). The culturable microbial community of the great barrier reef sponge rhopaloeides odorabile is dominated by an α-proteobacterium. Mar. Biol. 138 (4), 843–851. doi: 10.1007/S002270000503
Webster N. S., Negri A. P., Munro M. M. H. G., Battershill C. N. (2004). Diverse microbial communities inhabit Antarctic sponges. Environ. Microbiol. 6 (3), 288–300. doi: 10.1111/j.1462-2920.2004.00570.x
Weigel B. L., Erwin P. M. (2017). Effects of reciprocal transplantation on the microbiome and putative nitrogen cycling functions of the intertidal sponge, hymeniacidon heliophila. Sci. Rep. 7 (1), 1–12. doi: 10.1038/srep43247
Wilkinson C. R. (1978). Microbial associations in sponges. II. numerical analysis of sponge and water bacterial populations. Mar. Biol. 49 (2), 169–176. doi: 10.1007/BF00387116
Wilson M. C., Gulder T. A. M., Mahmud T., Moore B. S. (2010). Shared biosynthesis of the saliniketals and rifamycins in salinispora arenicola is controlled by the sare1259-encoded cytochrome P450. J. Am. Chem. Soc. 132 (36), 12757–12765. doi: 10.1021/ja105891a
Woodruff H. B. (2014). Selman a. waksman, winner of the 1952 Nobel prize for physiology or medicine. Appl. Environ. Microbiol. 80 (1), 2. doi: 10.1128/AEM.01143-13
Woodruff H. B., McDaniel L. E. (1958). “The antibiotic approach,” in The strategy of chemistry. Eds. Cohen S. T., Rowatt R. (Cambridge: University Press), 29–48.
World Health Organization. (2020). Global tuberculosis report 2020 (Geneva: World Health Organization). Available at: https://apps.who.int/iris/handle/10665/336069.
World Health Organization (2021). Global tuberculosis report 2021 (Geneva: World Health Organization). Available at: https://apps.who.int/iris/handle/10665/346387.
Zerikly M., Challis G. L. (2009). Strategies for the discovery of new natural products by genome mining. ChemBioChem 10 (4), 625–633. doi: 10.1002/cbic.200800389
Zhang F., Jonas L., Lin H., Hill R. T. (2019). Microbially mediated nutrient cycles in marine sponges. FEMS Microbiol. Ecol. 95 (11), fiz155. doi: 10.1093/femsec/fiz155
Zhang C., Li X., Yin L., Liu C., Zou H., Wu Z., et al. (2019). Analysis of the complete genome sequence of brevibacterium frigoritolerans ZB201705 isolated from drought- and salt-stressed rhizosphere soil of maize. Ann. Microbiol. 69 (13), 1489–1496. doi: 10.1007/s13213-019-01532-0
Zhang M. M., Qiao Y., Ang E. L., Zhao H. (2017). Using natural products for drug discovery: The impact of the genomics era. Expert Opin. Drug Discovery 12 (5), 475. doi: 10.1080/17460441.2017.1303478
Keywords: Micrococcus, Micromonospora, Streptomyces, Brevibacterium, anti-mycobacterial activity, marine
Citation: Tizabi D, Bachvaroff T and Hill RT (2022) Comparative analysis of assembly algorithms to optimize biosynthetic gene cluster identification in novel marine actinomycete genomes. Front. Mar. Sci. 9:914197. doi: 10.3389/fmars.2022.914197
Received: 06 April 2022; Accepted: 30 June 2022;
Published: 29 July 2022.
Edited by:
Wolfram Brück, University of Applied Sciences and Arts of Western Switzerland, SwitzerlandReviewed by:
Zhiyong Li, Shanghai Jiao Tong University, ChinaCopyright © 2022 Tizabi, Bachvaroff and Hill. This is an open-access article distributed under the terms of the Creative Commons Attribution License (CC BY). The use, distribution or reproduction in other forums is permitted, provided the original author(s) and the copyright owner(s) are credited and that the original publication in this journal is cited, in accordance with accepted academic practice. No use, distribution or reproduction is permitted which does not comply with these terms.
*Correspondence: Russell T. Hill, aGlsbEB1bWNlcy5lZHU=
Disclaimer: All claims expressed in this article are solely those of the authors and do not necessarily represent those of their affiliated organizations, or those of the publisher, the editors and the reviewers. Any product that may be evaluated in this article or claim that may be made by its manufacturer is not guaranteed or endorsed by the publisher.
Research integrity at Frontiers
Learn more about the work of our research integrity team to safeguard the quality of each article we publish.