- 1Naval Information Warfare Center Pacific, San Diego, CA, United States
- 2National Marine Mammal Foundation, San Diego, CA, United States
- 3Centre for Research into Ecological and Environmental Modelling, University of St Andrews, St Andrews, United Kingdom
North Pacific minke whale (Balaenoptera acutorostrata) boing calls are commonly detected in Hawaiian waters. When producing boing vocalizations, minke whales seem to be in one of two calling behavioral states. Most often minke whales produce boings with inter-call intervals of several minutes, but sometimes minke whales call rapidly with inter-call intervals of less than a minute. Since minke whales are difficult to detect visually, cue-rate-based density estimation using passive acoustic monitoring has been proposed. However, the variables that influence cue rate or calling rate are poorly understood in most whales, including minke whales. We collected passive acoustic recordings from 47 bottom-mounted hydrophones at the Pacific Missile Range Facility’s instrumented range off the coast of Kauaʻi, Hawaiʻi to test the hypothesis that minke whales call more rapidly when closer in proximity to other calling conspecifics. A total of 599 days of data were recorded between August 2012 and July 2017 and were automatically post-processed to detect, classify, and localize calls. Localized calls were grouped into tracks and manually validated, resulting in 509 individual tracks composed of 36,033 calls within a 16 x 39 km focal study area. Tracked minke whales exhibited a strong bimodal call rate with means of one call every 6.85 min (σ= 2.54 min) and 0.63 min (σ= 0.36 min). We ran hidden Markov models to quantify the relationship between call rate and the distance to the nearest calling conspecific. Overall, the probability of the higher call rate occurring increased as the distance to the nearest conspecific decreased, and the probability of the lower call rate occurring increased as the distance to the nearest conspecific increased. We also examined individual track data and found that minke whales may also exhibit other responses (i.e. increased speed, changes in heading, and cessation of calling) when calling conspecifics are nearby. These findings provide new information about minke whale calling behavior in what is likely a breeding area.
1 Introduction
North Pacific minke whale (Balaenoptera acutorostrata) boing calls are commonly detected in Hawaiian waters from fall to spring, but the animals that produce them have rarely been sighted and little is known about their behavior in this area. The minke whale boing call was first detailed by Wenz (1964) using recordings from submarines operating off the coast of San Diego, California and in Hawaiian waters and also from two bottom-mounted hydrophones off the coast of Kāneʻohe, Hawaiʻi. Although it was suspected that the boing was produced by a whale species (Thompson and Friedl, 1982), it took about 40 years until the source of the boing was determined to be from a minke whale (Rankin and Barlow, 2005). Minke whales are difficult to detect visually, so passive acoustic monitoring (PAM) is an important methodology for learning about the behavior and life history of this species.
Thompson and Friedl (1982) recorded boing sounds from two bottom-mounted hydrophones off the coast of Oʻahu. They observed two calling states with distinct inter-call intervals (ICIs). They proposed that the whales produced calls with ICIs of approximately 30 s when in acoustic contact with another calling whale, and 6 min when not in acoustic contact with another calling whale, but this study was limited by a low sample size and the inability to localize calls and measure distances between individuals (Thompson and Friedl, 1982). The significance of the boing call is currently unknown. However, since humpback whales (Megaptera novaeangliae) in Hawaiian waters use song for breeding purposes (Tyack, 1981) and have a similar seasonal and spatial overlap with minke whales, past researchers have suggested that the minke whale boing is also a mating call (Oswald et al., 2011; Martin et al., 2015), but this hypothesis is yet to be tested. Learning more about the calling rate of whales in different contexts can help us better understand the function of calls in whale behavior.
The central North Pacific boing call produced by minke whales in Hawaiian waters (as opposed to the eastern North Pacific boing call, which has a slightly different pulse repetition rate and call duration) has an onset transient component followed by a long call (mean duration of 2.6 sec) with both frequency and amplitude modulation (Rankin and Barlow, 2005). The 1,350 to 1,440 Hz band contains the majority of energy from the boing call with 115 Hz pulse repetition rate harmonic bands (Wenz, 1964; Rankin and Barlow, 2005; Martin et al., 2015). The peak frequency in this band has been termed the dominant signal component (DSC) (Martin, 2009), and has been identified as a feature to attribute calls to individual whales in some situations (Martin et al., 2013).
The life history of minke whales that spend time in Hawaiian waters is unknown, but acoustic detections and visual sightings in other areas can give clues about their migratory patterns. In the Chukchi Sea, Delarue et al. (2012) detected a small number of central North Pacific boings in the early fall (n=44), and boings resembling the eastern North Pacific type in the late summer and fall (n=10). In the North Pacific, pulse trains and downsweep calls have been documented in the presence of minke whales during the summer in Cormorant Channel, British Columbia, Canada which is likely a feeding area (Nikolich and Towers, 2020). Minke whales sighted off Vancouver Island and central British Columbia often have scars characteristic of cookiecutter sharks (Isistius brasiliensis) which are only in tropical and subtropical waters (Towers et al., 2013). Based on these observations and acoustic detections, the minke whales detected in Hawaiian waters probably migrate north to arctic or subarctic waters to feed, similar to the migration patterns of other large baleen whales.
In addition to helping us understand whale behavior, calling rate, or cue rate, is a necessary variable for PAM cue-rate-based density estimation (Marques et al., 2009). Visual surveys are currently the primary method to estimate marine mammal abundance, but minke whales are difficult to see due to their small size, minimal surface cues, and tendency to be alone, and so it is very challenging to estimate their abundance precisely (Zerbini et al., 2006). In addition, the visual ship-based surveys for marine mammals in Hawaiian waters are usually conducted during summer and fall, and since minke whales are rarely visually or acoustically detected during this time, no total abundance estimate exists for minke whales in this region (Carretta et al., 2014). Quantifying the minke whale cue rate and the variables that influence it may make it possible to estimate the abundance of minke whales using PAM for applications when tracking individuals is not possible.
In this paper, we investigate the hypothesis proposed by Thompson and Friedl (1982) that minke whales call more rapidly when closer to other calling minke whales. We recorded and localized boing calls off of Kauaʻi, Hawaiʻi between fall and spring for 5 years, and spatio-temporally grouped these localized calls into acoustic tracks. Using these tracks, we quantified individual minke whale ICIs and modeled ICI as a function of distance to the nearest tracked calling conspecific. This paper summarizes the calling behavior of minke whales using 36,033 boing calls from 509 tracks.
2 Methods
2.1 Study area and data description
The Pacific Missile Range Facility (PMRF) is a U.S. Navy training and testing area northwest of the island of Kauaʻi, Hawaiʻi. For this analysis, we analyzed recordings from 47 bottom-mounted hydrophones spread over a grid approximately 20 km to the east-west and 58 km to the north-south (Figure 1) to detect, classify, and localize minke whale boing calls (Figure 2) from data collected between August 2012 and July 2017. This period from 2012 to 2017 was chosen because the broadband hydrophone array configuration was consistent during this time. As detailed by Martin et al. (2015), the depths of the hydrophones ranged from 650 m to 4,700 m and had a suitable frequency response to detect calls from minke whales. A custom personal computer-based recorder described by Martin et al. (2013) and updated with a second 32-channel analog-to-digital converter was used to record a total of 62 hydrophones and two inter-range instrumentation group time code channels. Full bandwidth data were collected at a 96 kHz sample rate with 16-bit samples. Starting in August 2014, the collection of additional long-term recordings at a 6 kHz sample rate increased the recording effort to monitor low-frequency whale calls, including the minke whale boing call. Recordings at the 96 kHz sample rate typically had maximum durations of 1.9 days of continuous data, while recordings at the 6 kHz sample rate had maximum durations of 10.9 days. The recording limit was a function of sample rate, bit depth, the number of channels recorded, and a maximum hard disk drive capacity of 2 terabytes supported by the recorder. No recordings during known U.S. Navy training or testing activities were included in the analysis. This work only analyzed tracks from unclassified recordings. Of the focal tracks analyzed, two started within 24 hours after U.S. Navy training and testing activities ended. Based on previous work by Durbach et al. (2021), between 24 hours and 4 days after the last sonar transmission, the kinematic behavior of minke whales on PMRF is similar to baseline behavior.
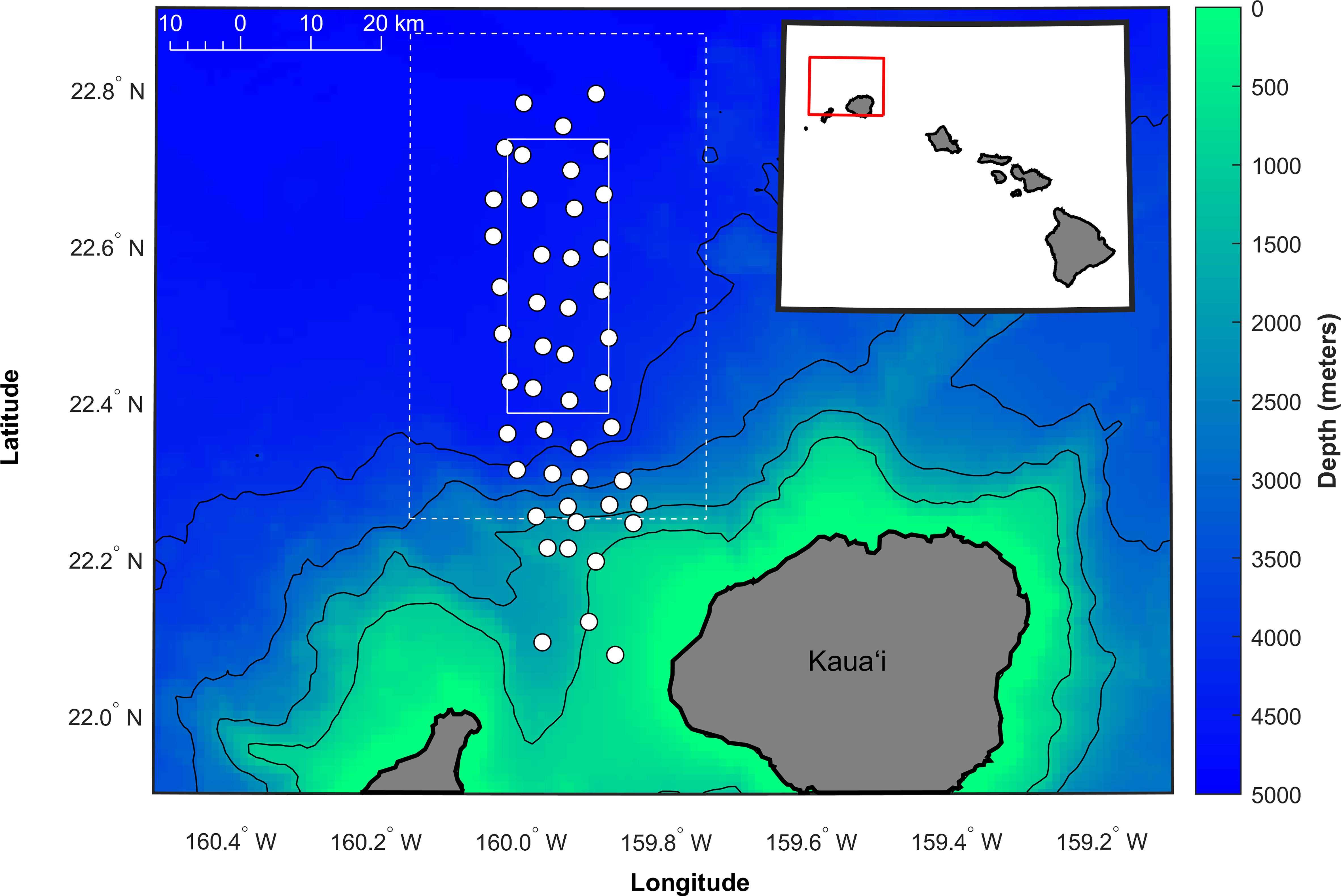
Figure 1 Map of the approximate locations of the 47 hydrophones used in this analysis (white circles) at the Pacific Missile Range Facility (PMRF) off Kauaʻi, Hawaiʻi, as shown by the red box in the inset map. The focal study area of the array extends approximately 16 km to the east-west and 39 km to the north-south (boundaries shown by a solid white box). The 15 km search area outside the focal area is shown with a dashed white box. The depth contours are every 1,000 m (1 km spatial resolution, Hawaii Mapping Research Group, The School of Ocean and Earth Science and Technology, the University of Hawaiʻi at Mānoa, http://www.soest.hawaii.edu/hmrg/multibeam/bathymetry.php).
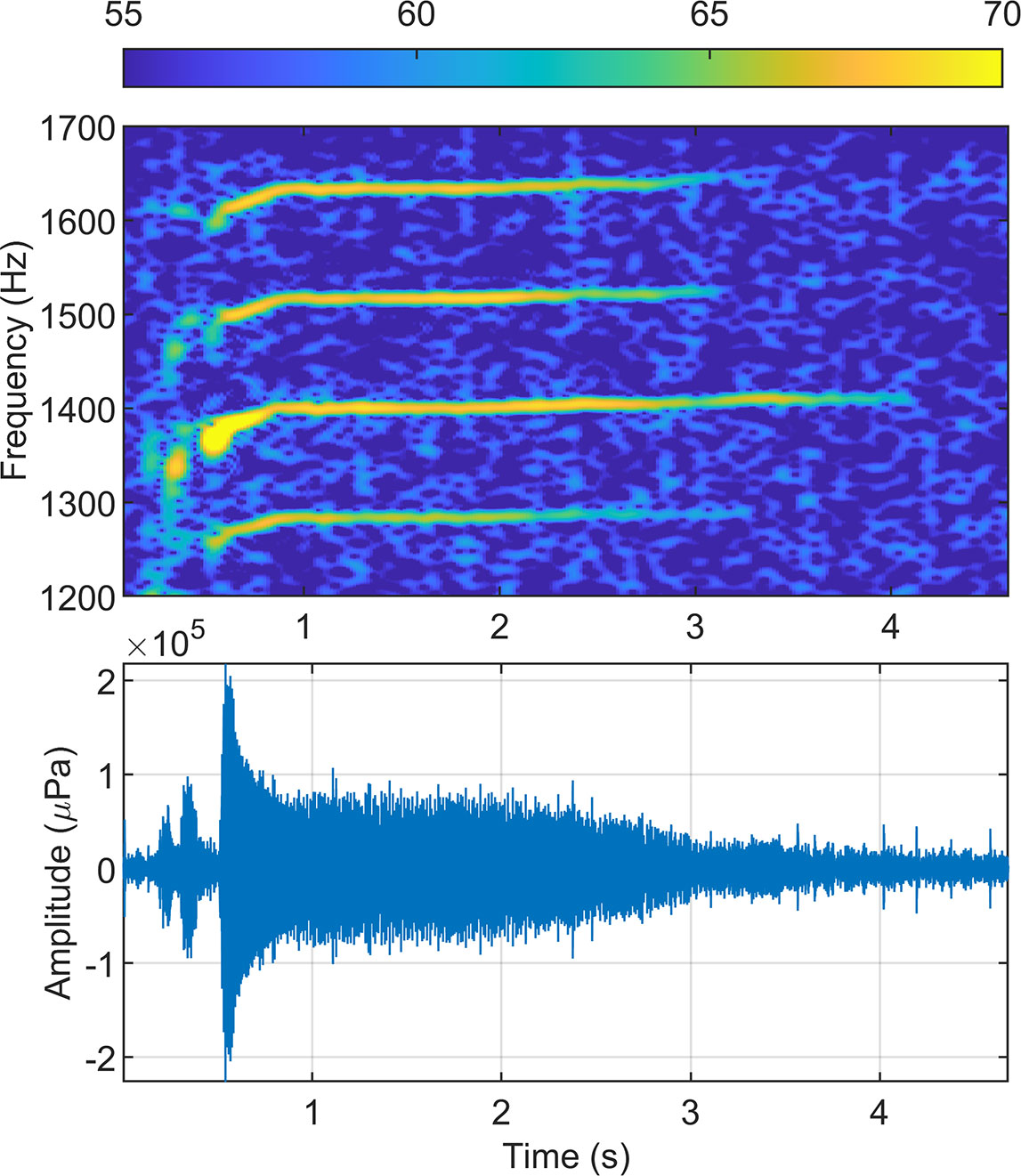
Figure 2 Example minke whale boing spectrogram (upper) and time series (lower) recorded at the Pacific Missile Range Facility (PMRF). Color in the spectrogram represents received sound pressure level in dB re 1µPa.
In order to investigate the impact of conspecifics on minke whale calling rate, we had to ensure that if a minke whale was vocalizing within a certain distance from another whale, both whales would be detected, localized, and tracked in all expected noise conditions. Based on the geometry of the PMRF array and the probability of detection of minke whale calls as a function of location, we designated an inner rectangular focal area where, if a whale called from a distance of 15 km or less from the focal area boundaries, we would be able to detect, localize, and track it. In other words, we could detect nearest calling conspecifics for whales tracked within the focal area out to 15 km. The focal area extended approximately 16 km to the east-west and 39 km to the north-south (indicated by the solid white box in Figure 1) with approximate latitude boundaries of 22.38°N and 22.74°N, and longitude boundaries of 160.02°W and 159.87°W. The 15 km search area was smaller than that defined by Harris et al. (2019) which conservatively encompassed the area where minke whale calls could be accurately detected, localized, and tracked based on expected noise conditions, source levels, and transmission properties of the boing call.
2.2 Post-processing and validation
The automated minke whale boing call detection and classification algorithms developed to process recordings collected at the PMRF instrumented range have been previously described (Mellinger et al., 2011; Martin et al., 2013; Martin et al., 2015) and are briefly reiterated here. The central North Pacific minke whale boing call detection and classification process operated in multiple stages. The first stage detected the near-continuous wave portion of the call by measuring if the energy in the detection band (1,320–1,450 Hz) exceeded a long-term spectral average noise estimate in the detection band for more than 0.8 sec. The second stage computed a spectral correlation to locate the onset time of the frequency modulated portion of call, and was used as the measured time of arrival (TOA) in the model-based localizer (discussed in the next paragraph). The third stage reprocessed the raw time series data with a longer fast Fourier transform (FFT) length to produce an FFT bin resolution of less than 1 Hz. The average power in the 1,350–1,440 Hz range over the duration of the signal was calculated and compared to the peak power in the same frequency and time range. The resulting value was termed a quasi-signal-to-noise ratio (QSNR) since the average power in the 1,350–1,440 Hz range also included the peak signal. If the QSNR exceeded a user-defined value of 2.0, the signal was classified as a boing call detection, and a high resolution measurement of the DSC was made. To our knowledge, the central North Pacific boing call is the only call associated with minke whales that has been recorded on PMRF.
The model-based localization algorithm for data processing was previously described by Martin et al. (2015) and is briefly described here. To localize calls, we compared the measured time difference of arrivals (TDOAs) of each call across the hydrophones with the modeled TDOAs. The onset times for automatic detections on multiple hydrophones were the measured TOAs, and were subtracted from each other to calculate measured TDOAs. Modeled TDOAs were calculated from theoretical source locations. An iterative spatial gridding process minimized the weighted least square error between measured and modeled TDOAs to spatially locate a call. The least square error was weighted by order of TOA with earlier arrivals weighted more than later arrivals, and was also normalized by the number of hydrophones in the localization solution. Only localizations with a weighted least square error value between measured and modeled TDOAs of less than or equal to 0.075 sec were used in this analysis. In addition, the DSCs of the first four detections for a candidate localization were required to be within 5 Hz to initiate grouping of detections. Currently, ground-truth whale positions to determine localization accuracy do not exist. However, Martin et al. (2015) noted that surface ship hull-mounted mid-frequency active sonar transmissions within the hydrophone array at PMRF that were localized with the same algorithm were typically within 50 m of ship positional data. The accuracy with which the start of a signal is detected influences the accuracy of localization. Despite mid-frequency active sonar transmissions being a higher frequency than minke whale boings, the start of both signals have an onset transient with a sharp rise time that is detected well.
An automatic tracking algorithm (previously described by Klay et al. (2015) and used by Harris et al. (2019); Helble et al. (2020b); Durbach et al. (2021) to track minke whales) spatially and temporally grouped localized calls into individual tracks by recursively examining distance and time between successive candidate localizations. The following user-defined values were tuned for tracking minke whales: a track had to be composed of 12 or more localizations, a localization solution required automatic detections from 8 or more hydrophones, the distance between localizations had to be less than or equal to 0.06 decimal degrees in both latitude and longitude (approximately 6.7 km), and the maximum time allowed between any two localizations before terminating a track was set to 40 min. Gaps between recordings could vary from minutes to weeks. In this study, we did not group calls into tracks across individual recordings.
For every localized call, we searched for the closest localized call from another track within the past 10, 20, and 30 min. If at least one other vocalizing minke whale was present during that time window and within the 15 km search area from the focal study area, we calculated the straight-line horizontal distance between the focal track’s call and the neighboring track’s calls. The distance to the nearest calling conspecific or “nearest neighbor” was the distance to the closest localized call from another track during that time window. For statistical analysis, nearest neighbor distances were assigned to categories of 0–5 km, 5–10 km, 10–15 km, or 15+ km. If there were no other tracks within the search area and time window, then the nearest neighbor for the focal track was assigned to the 15+ km category given that there were additional whales beyond 15 km. Grouping nearest neighbor distances into categories allowed us to keep observations from all tracks, including those that did not have a detectable nearest neighbor, without assuming an arbitrary large value for its nearest neighbor distance. The 5 km distance bins were small enough to see changes in calling behavior across distances, but large enough to have large sample sizes and small confidence intervals for each category. We only searched for nearest neighbors within the same recording as that containing the focal track. We tested multiple nearest neighbor time windows (10, 20, and 30 min) to account for variability in call timing and location (e.g. if an animal skipped calls or if some calls from the nearest neighbor were outside of the study area). Since the maximum time between subsequent calls before terminating a track was 40 min, we did not test window sizes larger than 30 min to avoid potentially declaring a whale as its own nearest neighbor.
We manually validated all tracks to ensure that a track was generated by an individual whale since spurious tracks could be generated under certain conditions. Specifically, a single track could be classified as two or more tracks in close proximity to each other when the ICI decreased or the number of co-occurring tracks in the study area increased. During the validation process, it was important to inspect and rectify potential duplicate tracks by removing or combining them since they could produce artificial minimum distances between conspecifics (i.e. a whale could be its own nearest neighbor). An interactive program was used to systematically examine the movement, DSC, and ICI of tracks in close proximity to each other. We combined tracks if the overall track path appeared to be a single animal which was evidenced by a relatively stable DSC and a call rate distribution that fell within the expected bimodal call rate distribution. Alternatively, we removed a track if it did not satisfy these criteria and appeared to be produced by multiple animals. No tracks were split since parsing calls from an individual track to multiple individuals is difficult and subjective without other data from tags or visual sightings. The validation process reduced the total number of focal tracks by 15.7%. Using unmodified tracks has the advantage of adding no subjectivity from the analyst, but a fraction of these automatic tracks are likely spurious or split from the same animal. To test the sensitivity of the results to the validation process and ensure call rate results were not biased by combining or removing tracks, both validated and unvalidated tracks were run through the same statistical analyses and the overall trend of the results were similar. From this analysis we determined that any subjectivity introduced from the validation process was minimal and using validated tracks for the statistical analyses provided the most accurate information on minke whale calling behavior and distances to conspecifics.
In addition to validating how calls were grouped into tracks, we also quantified the performance of the entire detection, classification, localization, and tracking process in a way similar to Helble et al. (2020a). We randomly selected 5% of all tracks from the full range of seasons and years. The corresponding raw acoustic data during the times of these tracks from four hydrophones centered and evenly spaced in the study area were imported into Raven Pro (Center for Conservation Bioacoustics, 2019) to generate spectrograms. The TOAs for tracked calls were also imported and colored boxes were overlaid on the signals in the spectrogram, with each color associated with an individual whale track. Sequential calls from the same individual had similar delay patterns across the four hydrophones. If an unmarked call recorded on the four channels had the same delay pattern as other calls along a known track, the call was counted as a missed localization. A missed localization means a call along a track should have been detected, localized, and tracked, but was missed or excluded in one of the automated stages. If detections coincidentally arrived at the correct delay patterns to warrant a localization but did not appear to be a minke boing or if boings were detected but suspected to be from multiple minke sources (based on the signal characteristics and/or source level), they were marked as false localizations. Any missed or false localizations were noted from each of these tracks. In reality, there was no evidence that false localizations occurred along a track, which is not surprising because calls from different whales would need to arrive in near-perfect delay sequences on 8 or more hydrophones to be considered a valid localization. Missed localizations did occasionally occur, and so a “stress test” dataset was created to determine how sensitive subsequent analyses were to missed localizations. To test this sensitivity, 30% of all calls along each track were randomly deleted, and this decimated dataset was also used to see if any changes in the calling behavior statistical analysis could be mistakenly skewed by any missed localizations. In practice, the average missed localization rate was nearly an order of magnitude smaller than 30%, and so the stress test marked a worse-case scenario for any missed localizations to skew the behavioral statistical analysis.
2.3 Changes in calling behavior statistical analysis
We used hidden Markov models (HMMs) to quantify the relationship between calling rate and distance to the closest calling conspecific. For each localized call, we measured the time difference between this call and the next call in the track and saved this value as the ICI. Only tracks or segments of tracks that were within the focal area were modeled in the HMMs. For tracks with multiple segments within the focal area, we only kept the longest segment. This segment was required to have at least 4 localizations within the focal area.
Since minke whales in Hawaiʻi have two calling states, a rapid and nominal calling state, and we hypothesized that the likelihood of being in each is affected by the distance to the closest calling conspecific, HMMs were appropriate for modeling. We modeled the minke whale ICI as HMMs that were functions of distance to the nearest calling neighbor. The ICI was modeled as a gamma distribution with initial values of 5 min for State 1 (representing the nominal calling rate) and 0.5 min for State 2 (representing the rapid calling rate) with standard deviations of 1 min for both. These initial values were chosen based on the observed distribution of data and the calling rates reported by Thompson and Friedl (1982), but the results presented were not sensitive to these initial values as initial values ±25% were tested and the best models did not change. We used the Akaike information criterion (AIC) (Akaike, 1974) to determine which nearest neighbor window size was best (10 min, 20 min, or 30 min). Once the best model was selected, we used the Viterbi algorithm to group each localized call into one of the two calling states (Langrock et al., 2012; McClintock and Michelot, 2018). To calculate the amount of time spent in each state, we added up the ICIs for the calls assigned to each state. HMM analyses for this study were conducted in R (v 4.0.3, R Core Team, 2020) using the momentuHMM package (McClintock and Michelot, 2018).
3 Results
Between August 2012 and July 2017, 599 days of data were recorded on each hydrophone. During this time, 2,245 individual minke whale tracks were observed and contained 223,732 calls. Of these tracks, 509 were located within the focal area at least part of the time and so were included as “focal tracks”. The subset of focal tracks contained 36,033 localized calls. Although we recorded throughout the year, minke whale boing vocalizations were only present between October and May (Figure 3). The increase in recording effort from collecting long-term data at the 6 kHz sample rate (initiated in August 2014) is evident from the lower cyan bars in Figure 3 starting in September 2014.
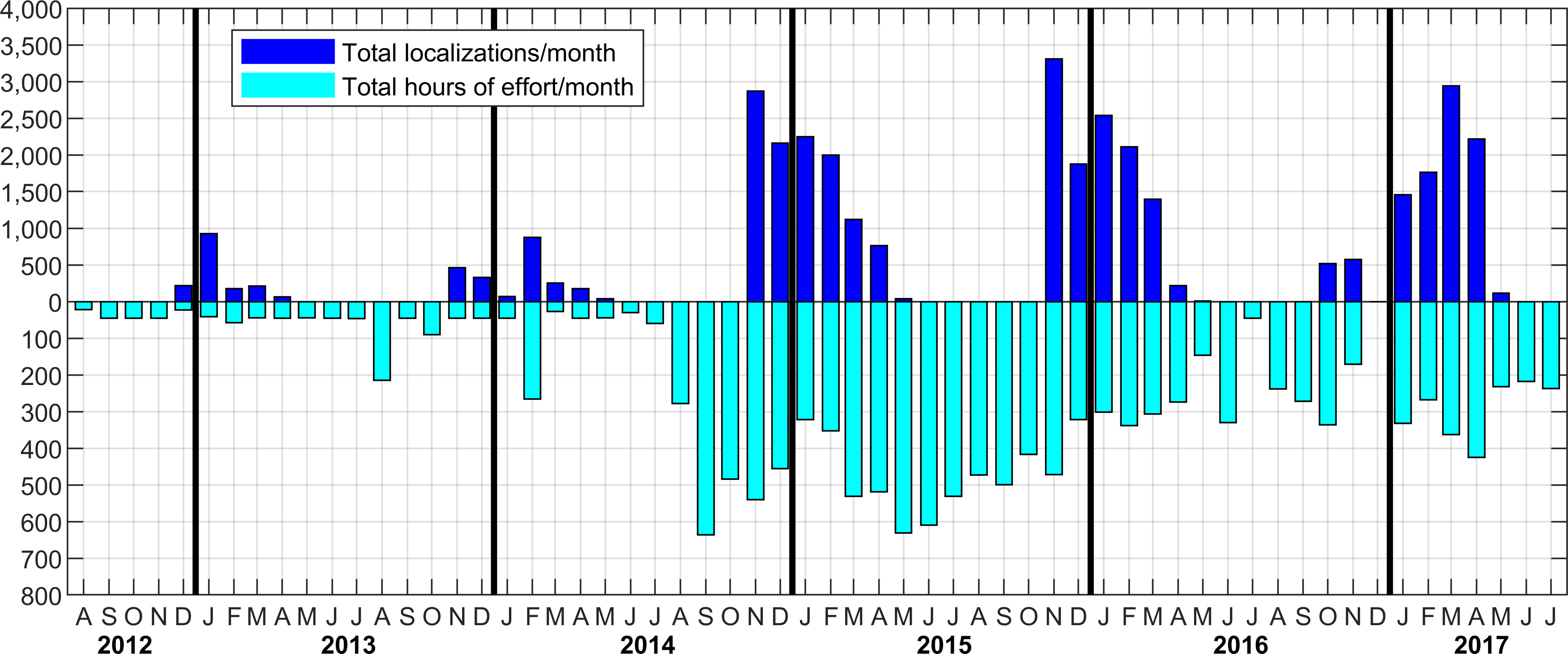
Figure 3 The number of localized minke whale calls that were tracked within the focal area per month (upper blue bars), and hours of recording effort per month (lower cyan bars) shown as a function of time from August 2012–July 2017.
Manual review of 5% of the 509 focal tracks (26 tracks) resulted in a mean missed localization rate of 4.6%. We noted that missed calls were almost always detected on 8 or more hydrophones, but were sometimes discarded by the automated localization process because the least square error value exceeded the user-defined threshold. No false localizations were noted in the manual review process. Relaxing the least square error threshold would result in fewer missed localizations, but would come at the expense of false localizations that can confuse the tracking algorithm. The 4.6% missed localization rate was acceptable and did not affect the outcome of determining the behavioral state, as demonstrated by the stress test dataset (details presented with the modeling results).
Minke whale boing ICIs followed a bimodal distribution (gray bars, Figure 4). Two calling states based on ICI were identified by the HMM and their probability density functions followed the observed ICI distribution well (blue and teal curves, Figure 4). Whales in State 1 had an mean ICI of approximately 6.85 min and whales in State 2 had an mean ICI of approximately 0.63 min (Table 1).
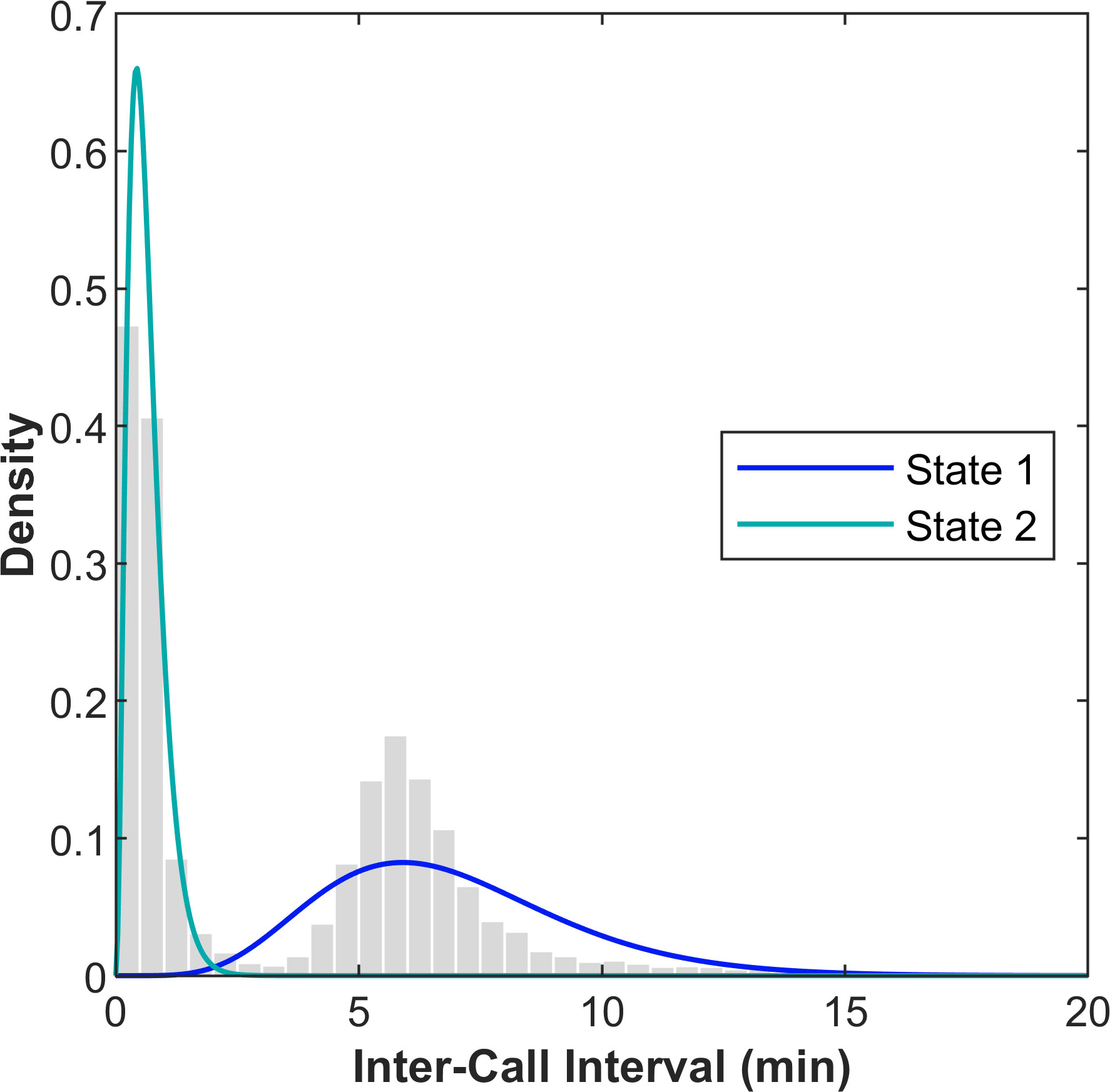
Figure 4 Distribution of observed minke whale boing inter-call intervals from 509 focal tracks at PMRF. The gray histogram shows the observed values normalized by the area of each bar, the blue curve shows the probability density function of State 1, and the teal curve shows the probability density function of State 2. These calling states were estimated using a hidden Markov model with distances grouped into 5 km bins and nearest neighbor determined using a 30 min window.
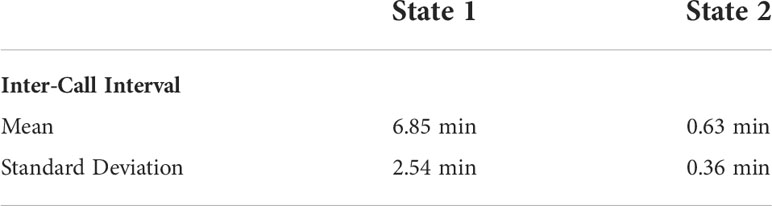
Table 1 Inter-call interval distribution parameters for hidden Markov model states modeling minke whale calling behavior as a function of distance to the nearest calling conspecific within the past 30 min.
We compared three different time windows to search for the nearest calling conspecific. The 30 min time window performed the best, followed by 20 min, and 10 min, which all performed better than the null model that tested the hypothesis that minke whale inter-call interval was not a function of distance to the nearest neighbor (Table 2). Because the inter-call interval changed by an order of magnitude between State 1 and State 2, these results were not sensitive to occasional missed localizations along a track. In the stress test dataset, 30% of calls were randomly dropped from a track, but the model ranking and stationary state probability trend remained the same (although the ICIs for the two states increased, as expected since there were fewer calls).
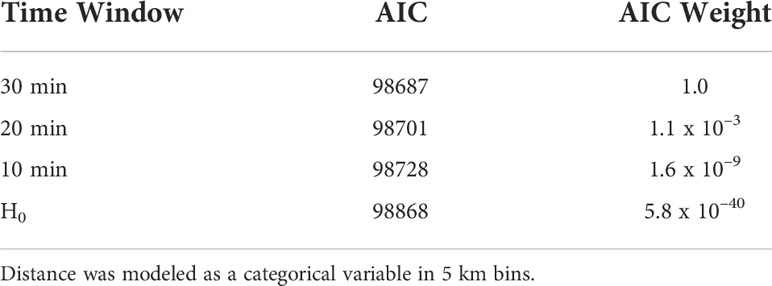
Table 2 Comparison of models using three different time windows to search for the nearest calling conspecific ranked by the Akaike information criterion (AIC) and AIC weights (Akaike, 1974; Burnham and Anderson, 2002) H0 represents the null model that calling rate is not a function of nearest calling conspecific.
The probability of a minke whale being in State 1 and calling at a nominal rate increased as its distance to the nearest calling conspecific increased and the probability of a minke whale being in State 2 and calling at a rapid rate increased as its distance to the nearest calling conspecific decreased (Figure 5). Since each observation is a localized call, the plotted probabilities are the probability of a call being in a given state and not the length of time that a whale is in a state (Figure 5 upper). When a whale is calling rapidly, more localizations are available for a given time than when a whale is calling nominally. Of the 36,033 localized calls that were part of focal tracks, 49% were categorized as being in State 1 and 51% were categorized as being in State 2. However, these tracked whales spent a total of 84.7 days calling nominally (State 1) and 8.0 days calling rapidly (State 2), which is equal to 91% of the time in State 1 and 9% of the time in State 2. Therefore, regardless of the distance to the closest calling conspecific, at any given time, minke whales are most likely in State 1 (Figure 5 lower). Calling rapidly has a higher probability when whales are closer together, but it is never more likely than calling nominally.
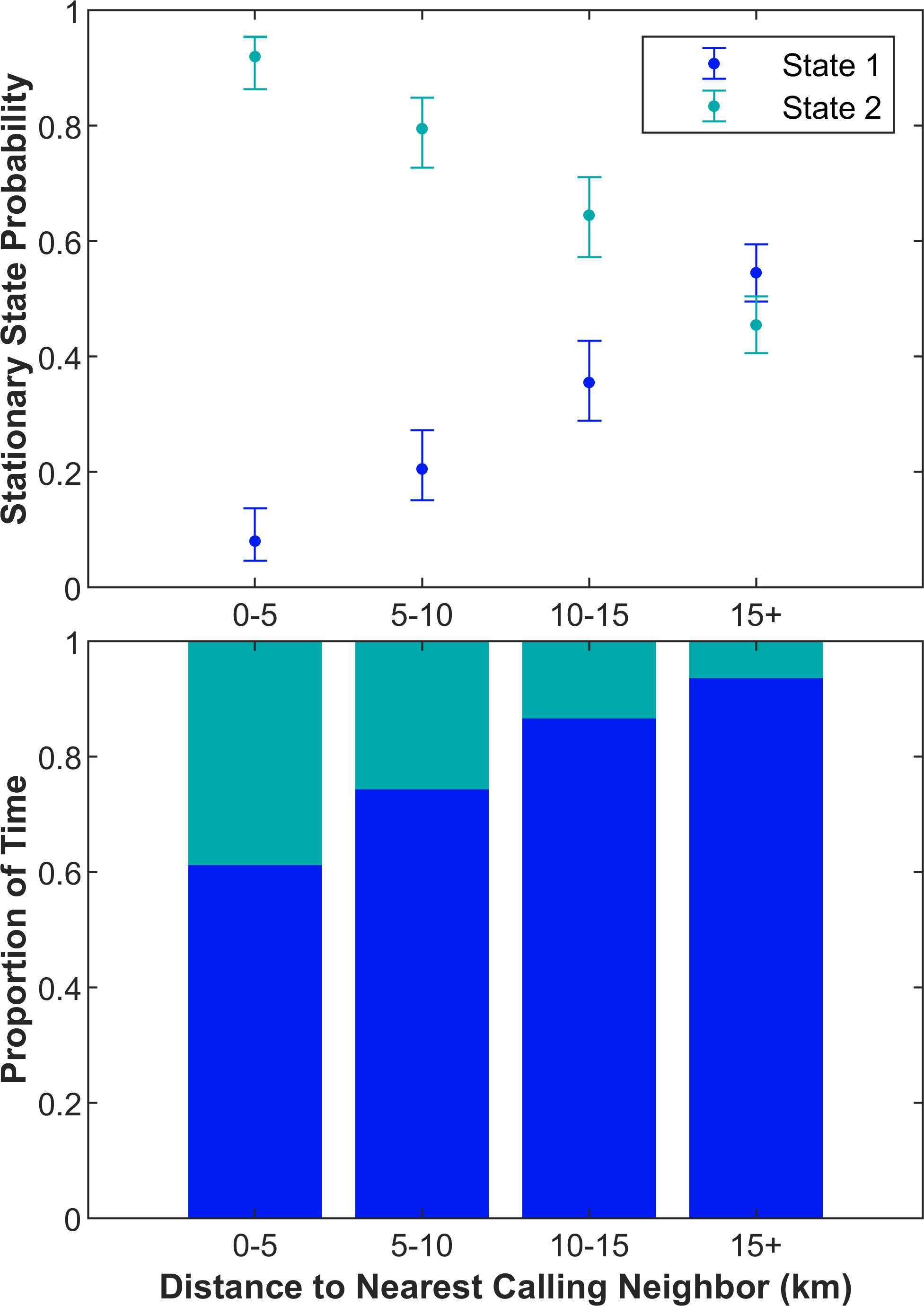
Figure 5 The probability of a minke whale call being a part of State 1 or 2 as a function of its distance to the nearest calling minke whale (upper plot) and the proportion of time in each of the states (lower plot). The blue and teal points show the stationary state probabilities of State 1 and State 2, respectively. The error bounds show the 95% confidence intervals. The blue and teal bars show the proportion of time that the focal animals were calling in State 1 and 2, respectively. The nearest neighbor distance is binned in 5 km intervals. The distances to the nearest neighbor are the distances to the closest calling whale within the past 30 min.
We selected two interactions between calling minke whales to illustrate these behaviors with individual whales. The first example occurred on 7 Feb 2017 (Figure 6). The minke whale that generated the primary track was calling entirely in State 1 and changed its heading and speed, and ultimately ceased calling during an encounter with a whale that generated the nearest track and was mainly in State 2. At the start of the primary track it was steadily calling and moving east-southeast, and had a distant nearest neighbor that was outside the search area. The whale that generated the track labeled as the “nearest track” in Figure 6 started calling at 18:31 UTC, almost an hour and 15 min after the start of the primary track. When the nearest track started calling it was 11.8 km away from the primary track and it quickly switched into State 2 and traveled southeast. At about the same time, the primary track turned to a south-southeast heading and increased its median speed before it stopped calling. While both animals were calling, the distance between the primary track and the nearest track varied between 10.8–11.8 km.
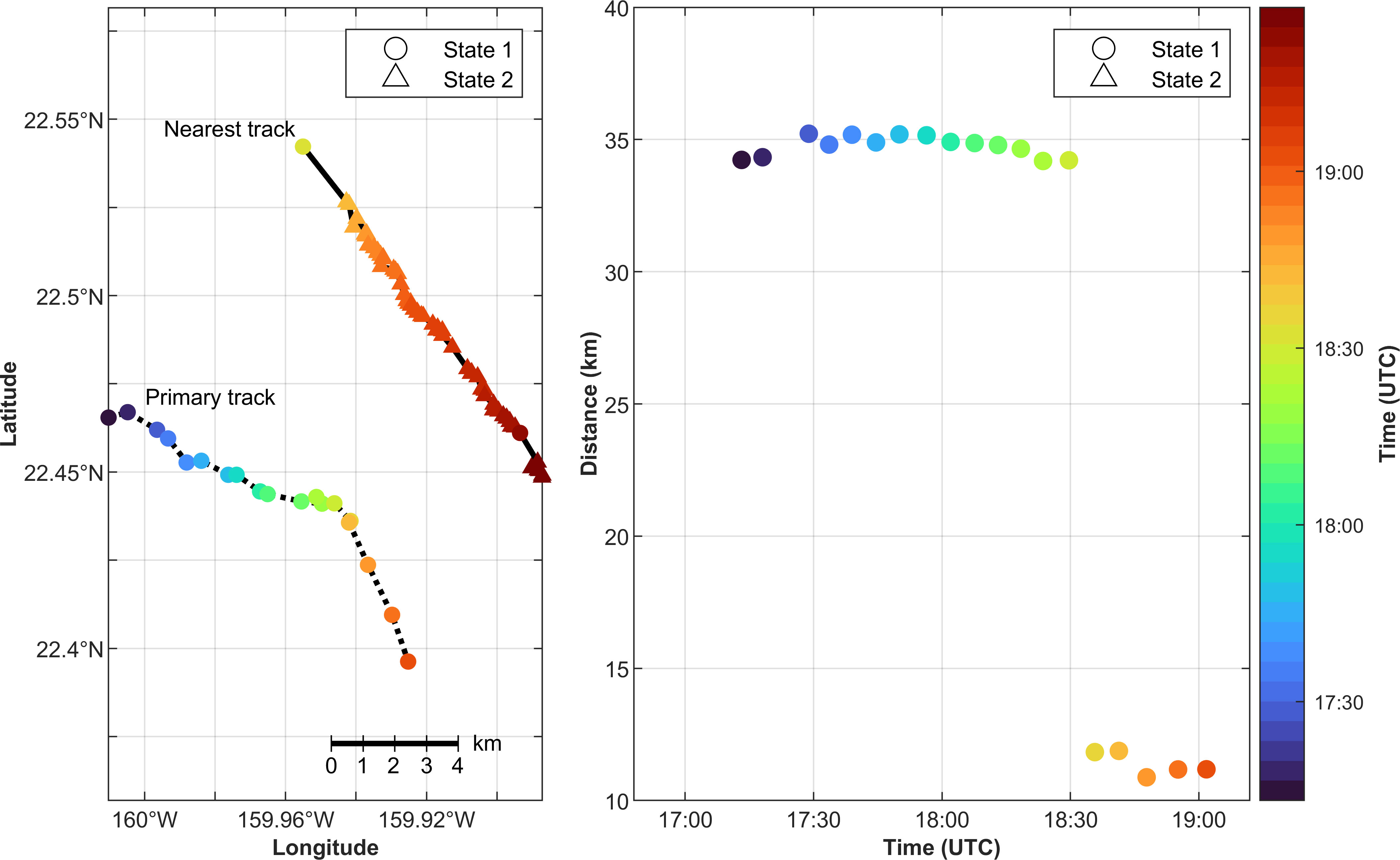
Figure 6 Example minke whale boing tracks at PMRF on 7 Feb 2017. The left panel shows a map of the interaction between two whales. Marker color indicates time and shape indicates calling state, with circles representing the nominal calling state (State 1) and triangles representing the rapid calling state (State 2). The dotted and solid lines connect the localized calls for the primary and nearest track, respectively. The right panel shows the distance to the primary track’s nearest neighbor. Time and calling state are again indicated by color and shape of the markers, respectively. Note that the nearest track between 17:00 and 18:30 UTC was outside of the 15 km search area and is a different track than the “nearest track” depicted in the map. This earlier track is not depicted in the left panel.
The second example of an interaction between two calling minke whales occurred on 8 Nov 2013 (Figure 7). During this encounter, the minke whale that generated the primary track switched to a rapid calling behavioral state when the whale that generated the nearest track and was also calling rapidly headed towards it. At the start of these tracks, the primary track was traveling southwest and the nearest track was traveling northeast and then north, with the distance between the two tracks decreasing. The primary track switched to calling mainly in State 2 at 6:27 UTC (indicated by arrow in Figure 7) when the whales were about 6.9 km apart. At the same time, the nearest track’s heading became more northeast, a reciprocal heading of the primary track. Before the tracks intersected, the whales paused calling for 36 min (primary track) and 15 min (nearest track). The nearest track started calling again first and was traveling northwest. When the primary track started calling it was about 2.7 km southwest of the nearest track and milled in that area while still calling in State 2. The nearest track then turned and headed south towards the primary track. The whale generating the primary track ceased calling when the nearest track was 900 m away.
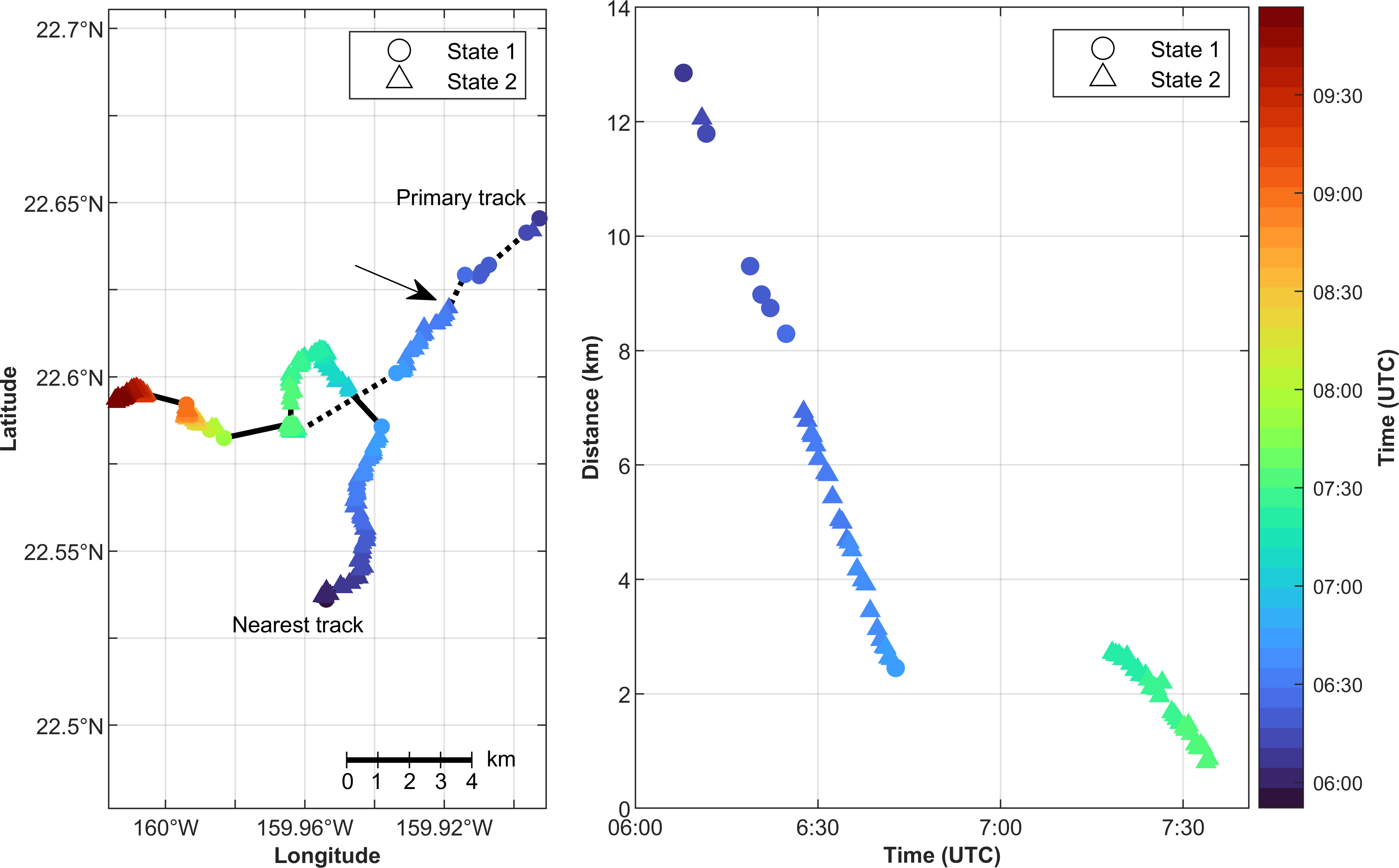
Figure 7 Example minke whale boing tracks at PMRF on 8 Nov 2013. The left panel shows a map of the interaction between two whales. Marker color indicates time and shape indicates calling state, with circles representing the nominal calling state (State 1) and triangles representing the rapid calling state (State 2). The arrow indicates when the primary track’s state changed from mainly State 1 to mainly State 2. The dotted and solid lines connect the localized calls for the primary and nearest track, respectively. The right panel shows the distance to the primary track’s nearest neighbor. The time and calling state are again indicated by the color and shape of the markers, respectively.
4 Discussion
Minke whales were more likely to call at an increased calling rate when in close proximity to another calling minke whale. They increased their calling rate by an order of magnitude between State 1 and State 2. Converting the ICIs reported in the Results section, the mean calling rate for State 1 was 8.7 calls/whale/hour and the mean calling rate for State 2 was 95.2 calls/whale/hour. The overall mean calling rate for minke whales on PMRF was 15.7 calls/whales/hour. Since calling rate is dependent on distance to the nearest neighbor, calling rate would be expected to be higher in areas where minke whale density is higher and lower in areas where minke whale density is lower.
The findings in this paper add complication to PAM cue-rate-based density estimation since calling rate is needed and is dependent on the number of animals in an area. In contrast, using PAM tracks of localized calls for a census-type density estimation (counting the number of tracks) is less complicated than density estimation methods using only calling rate, but is only available in places where the number and spacing of hydrophones allow for tracking (requirements for tracking are discussed in detail in Nosal, 2013; Helble et al., 2015). For researchers that are unable to localize and track calls, this work provides information on minke whale calling dynamics that are important to consider when studying calling behavior and density estimation. Although we present a cue rate for minke whale boing calls in this paper, this rate should be applied with caution to density estimation equations because we also found that calling rate is density dependent. In addition, this calling rate is based only on regularly vocalizing animals and we do not know the proportion of silent minke whales. Due to the complicated use of vocalizations by mysticetes, deploying multiple hydrophones to track animals may allow for more accurate PAM-based density estimation. Then the “cue” would be a track and the “cue rate” would be related to the probability of tracking a whale, which could be estimated with passive acoustic tagging studies. However, these tagging studies might show that the probability of acoustically tracking whales is also density dependent, which was shown with humpback whales migrating off the coast of Australia by Noad et al. (2017). Even so, deploying multiple hydrophones to localize and track whales is likely more stable for density estimation because it is easier to define a study area and tracking is less impacted by changes in calling rate.
Martin et al. (2015) hypothesized that the minke whale boing call is a mating call. Since minke whales increase their calling rate when in close proximity to other calling minke whales, we are hypothesizing that the boing call also communicates territoriality when the calling rate increases. Minke whales might call slowly to advertise their position and keep space between other vocalizing whales. When another whale gets too close, they begin to call rapidly, possibly as a sign of aggression. Territoriality is expressed acoustically in many other animal taxa. For example, territorial male Galápagos sea lions (Zalophus wollebaeki) and greater sac-winged bats (Saccopteryx bilineata) both increased their vocalization rates as the density of conspecifics around them increased (Kunc and Wolf, 2008; Eckenweber and Knörnschild, 2013). Other species including male green frogs (Rana clamitans) and ring-billed gulls (Larus delawarensis) use a specific call type to signal aggression and produced those calls when intruders entered their territory (Wells, 1978; Southern, 1981).
The minke whale boing call might have a dual purpose of being a mating call and a territorial call. Other species also use the same calls for both aggression and mating. Anurans, birds, and even fish species have been observed to produce the same calls while defending their territory from an intruder as they would if they were trying to attract a mate, although their calling rate may help signify the intent in these different scenarios (e.g. Wiewandt, 1969; Wingfield, 1994; Borgia and Coleman, 2000; Vasconcelos et al., 2010; Baker et al., 2012). However, aggression and mating may not be independent events. Species that use vocalizations to simultaneously deter competitors and attract mates include male midwife toads (Alytes obstetricans and Alytes cisternasii), red deer (Cervus elaphus), and fallow bucks (Dama dama) (McComb, 1991; Bosch and Márquez, 1996; McElligott and Hayden, 1999). These previous studies have used the proximity of non-calling conspecifics (specifically female conspecifics) to help determine the significance of calls, but in our study, we only know the positions of vocal whales and do not know how non-vocal whales are behaving and whether they are attracted or repelled from the boing calls.
Even if vocalizations are associated with mating behavior, their purpose may not be to attract mates. Sometimes other humpback whale males have approached singers, but singers have also been observed to sing when alone and when escorting mother-calf pairs (e.g. Darling and Bérubé, 2001; Smith et al., 2008). Male-male interactions were not always agonistic and Darling and Bérubé (2001) suggested that they might help in social ordering. Similar to minke whales, singing humpbacks might also change their singing behavior when in close proximity to another singing male and often stop singing if the approach gets very close, regardless of whether or not the other male is singing (Darling and Bérubé, 2001; Smith et al., 2008; Cholewiak et al., 2018). Our understanding of baleen whale behavior has advanced more slowly than other taxa due to their vast communication space and difficulties with visual observations, but studies like these can help to increase our knowledge of these protected species.
Although the seasonality of the minke whale boing in Hawaiian waters is similar to that of male songs from humpback whales and fin whales (Balaenoptera physalus) (e.g. Guazzo et al., 2020; Helble et al., 2020a), no biopsies have been collected from calling minke whales to provide evidence of their sex (unlike with humpback and fin whales, e.g. Darling and Bérubé, 2001; Croll et al., 2002; Smith et al., 2008). In other taxa, both males and females have been observed producing territorial calls, so our hypothesis that the minke whale call is used to signal aggression does not also imply the sex of the calling animals. For example, both male and female little blue penguins (Eudyptula minor) defended nest sites by vocalizing and/or moving toward intruders and these vocalizations may help them to signal their aggression in the low-visibility nighttime conditions when they are most active (Waas, 1991). Minke whales also live in low-visibility conditions, so vocalizations are more effective than visual signals to communicate a message over long distances. Since territoriality and aggression are associated with mating in many taxa, it is possible that the minke whale boing functions as a mating call when emitted at the lower calling rate and as a territorial or aggressive call at the higher calling rate.
An alternative hypothesis for the minke whale boing call is that this call is a social call not used for mating, and whales are increasing their calling rate to better communicate location and maintain cohesion with another nearby minke whale. The rarity of boing calls reported at higher latitudes during the summer and fall (Delarue et al., 2012) in potential feeding areas suggests that if the boing call is a social call, it seems to be used primarily in tropical waters from fall to spring. More information about the sex of calling minke whales and the reaction of conspecifics to the boing call would help differentiate between hypotheses.
Minke whale vocal activity is likely influenced by other factors not tested in this analysis. If the boing call is part of mating behavior, then calling behavior may be influenced by hormone levels and time of year (proposed for humpback whales by Clark and Clapham, 2004; Vu et al., 2012; Vu et al., 2014). The time of day can also affect whale behavior, including vocalization behavior (observed in gray whales (Eschrichtius robustus) by Guazzo et al., 2017). In addition, background noise level might affect the vocalization rate (Helble et al., 2020b; Guazzo et al., 2020). Minke whales might call more if noise levels are higher due to other calling conspecifics in the area or they might call more out of a defensive posture due to reduced communication space. Conversely, minke whales might call less if the communication space is reduced and they cannot hear nearby conspecifics (various responses to increased noise level are reviewed in Brumm and Zollinger, 2011). These variables should be tested with future research.
Ideally, similar studies should be performed in other locations to assess how minke whales and other species vocalize as a function of density. However, very few hydrophone set-ups currently available would be able to replicate the study described in this paper. PMRF is a unique area in that we were able to guarantee detection and localization of calling minke whales within a large (over 3,100 km2) search area. A large search area is required to be able to test for behavioral responses based on distance to a conspecific when conspecifics are spread out on the order of multiple kilometers.
In conclusion, minke whales producing boing calls in Hawaiian waters are more likely to call at a rapid rate when they are closer to other vocalizing conspecifics. Many questions still remain about the function of these boing calls, but the unique hydrophone array set-up at PMRF combined with the statistical tools applied here allowed for the analysis of more than 500 minke whale tracks containing tens of thousands of calls. Since the calling rate is dependent on the distance to the nearest neighbor, the calling rate would be expected to be higher in areas where the density of minke whales is higher and lower in areas where the density of minke whales is lower, adding complication to density estimation using passive acoustic monitoring. Despite this added difficulty when estimating abundance, these findings provide new information about minke whale calling behavior on what is probably a breeding area.
Data availability statement
The datasets presented in this study can be found in online repositories. The names of the repository/repositories and accession number(s) can be found below: https://seamap.env.duke.edu/dataset/2190.
Ethics statement
Ethical review and approval was not required for the animal study because this study was conducted using passive acoustic monitoring of wild animals with underwater microphones (i.e. hydrophones) and has no impact on animals’ lifecycle.
Author contributions
CM and SM formulated the idea for this study and CM performed the initial analysis. CM, SM, BM, and EH collected the data. GA and BM programmed the detection, classification, and localization algorithms. GA managed and post-processed the data. SM developed the initial tracking algorithm and CM, GA, and SM tuned the tracking algorithm for minke whale boings. ID assisted with the HMM statistical analysis. CM, RG, and TH validated and analyzed the data and wrote the draft manuscript. All authors discussed the results and contributed to the final manuscript.
Funding
This research was supported by Commander, U.S. Pacific Fleet (Code N465JR, AwardNumber N0007020WR0EP8F) and tool development utilized for this analysis was supported by the U.S. Navy’s Living Marine Resources Program (Award Number N0002520WR0141R).
Acknowledgments
The authors thank the personnel at PMRF, especially Eliseo Boloson, Mike Dick, Jim Hager, Robin Higuchi, Bryson Kurokawa, Jon Winsley, and Jeffrey Yates, for obtaining recordings of acoustic data, as without the acoustic data this type of analysis would not be possible.
Conflict of interest
The authors declare that the research was conducted in the absence of any commercial or financial relationships that could be construed as a potential conflict of interest.
Publisher’s note
All claims expressed in this article are solely those of the authors and do not necessarily represent those of their affiliated organizations, or those of the publisher, the editors and the reviewers. Any product that may be evaluated in this article, or claim that may be made by its manufacturer, is not guaranteed or endorsed by the publisher.
References
Akaike H. (1974). A new look at the statistical model identification. IEEE Trans. Automatic Control 19, 716–723. doi: 10.1109/tac.1974.1100705
Baker T. M., Wilson D. R., Mennill D. J. (2012). Vocal signals predict attack during aggressive interactions in black-capped chickadees. Anim. Behav. 84, 965–974. doi: 10.1016/j.anbehav.2012.07.022
Borgia G., Coleman S. W. (2000). Co-Option of male courtship signals from aggressive display in bowerbirds. Proc. R Soc. Lond B Biol. Sci. 267, 1735–1740. doi: 10.1098/rspb.2000.1203
Bosch J., Márquez R. (1996). Acoustic competition in male midwife toads Alytes obstetricans and Alytes cisternasii: response to neighbor size and calling rate. implications for female choice. Ethology 102, 841–855. doi: 10.1111/j.1439-0310.1996.tb01205.x
Brumm H., Zollinger S. A. (2011). The evolution of the Lombard effect: 100 years of psychoacoustic research. Behaviour 148, 1173–1198. doi: 10.1163/000579511x605759
Burnham K. P., Anderson D. R. (2002). Model selection and multimodal inference. 2 edn (New York: Springer-Verlag). doi: 10.1007/b97636
Carretta J. V., Oleson E. M., Weller D. W., Lang A. R., Forney K. A., Baker J., et al. (2014). U.S. Pacific marine mammal stock assessments: 2013. NOAA Technical Memorandum, NOAA-TM-NMFS-SWFSC-532. (Department of Commerce).
Center for Conservation Bioacoustics (2019). Raven Pro: Interactive sound analysis software (Version 1.6.1) (Ithaca, NY: The Cornell Lab of Ornithology). Available at: http://ravensoundsoftware.com/.
Cholewiak D. M., Cerchio S., Jacobsen J. K., Urbán-R. J., Clark C. W. (2018). Songbird dynamics under the sea: acoustic interactions between humpback whales suggest song mediates male interactions. R. Soc Open Sci. 5, 171298. doi: 10.1098/rsos.171298
Clark C. W., Clapham P. J. (2004). Acoustic monitoring on a humpback whale (Megaptera novaeangliae) feeding ground shows continual singing into late spring. Proc. R Soc. Lond B Biol. Sci. 271, 1051–1057. doi: 10.1098/rspb.2004.2699
Croll D. A., Clark C. W., Acevedo A., Tershy B., Flores S., Gedamke J., et al. (2002). Only male fin whales sing loud songs. Nature 417, 809. doi: 10.1038/417809a
Darling J. D., Bérubé M. (2001). Interactions of singing humpback whales with other males. Mar. Mamm. Sci. 17, 570–584. doi: 10.1111/j.1748-7692.2001.tb01005.x
Delarue J., Martin B., Hannay D. (2012). Minke whale boing sound detections in the northeastern chukchi Sea. Mar. Mamm. Sci. 29, E333–E341. doi: 10.1111/j.1748-7692.2012.00611.x
Durbach I. N., Harris C. M., Martin C., Helble T. A., Henderson E. E., Ierley G., et al. (2021). Changes in the movement and calling behaviour of minke whales (Balaenoptera acutorostrata) in response to Navy training. Front. Mar. Sci. 8. doi: 10.3389/fmars.2021.660122
Eckenweber M., Knörnschild M. (2013). Social influences on territorial signaling in male greater sac-winged bats. Behav. Ecol. Sociobiol. 67, 639–648. doi: 10.1007/s00265-013-1483-z
Guazzo R. A., Helble T. A., Alongi G. C., Durbach I. N., Martin C. R., Martin S. W., et al. (2020). Lombard Effect: humpback whale song source levels increase as ambient ocean noise levels increase. J. Acoust. Soc Am. 148, 542–555. doi: 10.1121/10.0001669
Guazzo R. A., Helble T. A., D’Spain G. L., Weller D. W., Wiggins S. M., Hildebrand J. A. (2017). Migratory behavior of eastern north Pacific gray whales tracked using a hydrophone array. PloS One 12, e0185585. doi: 10.1371/journal.pone.0185585
Harris C. M., Martin S. W., Martin C., Helble T. A., Henderson E. E., Paxton C. G. M., et al. (2019). Changes in the spatial distribution of acoustically derived minke whale (Balaenoptera acutorostrata) tracks in response to Navy training. Aquat. Mamm. 45, 661–674. doi: 10.1578/am.45.6.2019.661
Helble T. A., Guazzo R. A., Alongi G. C., Martin C. R., Martin S. W., Henderson E. E. (2020a). Fin whale song patterns shift over time in the central north Pacific. Front. Mar. Sci. 7. doi: 10.3389/fmars.2020.587110
Helble T. A., Guazzo R. A., Martin C. R., Durbach I. N., Alongi G. C., Martin S. W., et al. (2020b). Lombard Effect: minke whale call source levels vary with natural variations in ocean noise. J. Acoust. Soc Am. 147, 698–712. doi: 10.1121/10.0000596
Helble T. A., Ierley G. R., D’Spain G. L., Martin S. W. (2015). Automated acoustic localization and call association for vocalizing humpback whales on the navy’s Pacific missile range facility. J. Acoust. Soc Am. 137, 11–21. doi: 10.1121/1.4904505
Klay J., Mellinger D. K., Moretti D. J., Martin S. W., Roch M. A. (2015). Advanced methods for passive acoustic detection, classification, and localization of marine mammals. Tech. rep., Office of Naval Research, Arlington, VA. Last accessed 22 January 2020. Available at: https://www.onr.navy.mil/reports/FY15/mbklay.pdf.
Kunc H. P., Wolf J. B. W. (2008). Seasonal changes of vocal rates and their relation to territorial status in male galápagos sea lions (Zalophus wollebaeki). Ethology 114, 381–388. doi: 10.1111/j.1439-0310.2008.01484.x
Langrock R., King R., Matthiopoulos J., Thomas L., Fortin D., Morales J. M. (2012). Flexible and practical modeling of animal telemetry data: hidden Markov models and extensions. Ecology 93, 2336–2342. doi: 10.1890/11-2241.1
Marques T. A., Thomas L., Ward J., DiMarzio N., Tyack P. L. (2009). Estimating cetacean population density using fixed passive acoustic sensors: an example with Blainville’s beaked whales. J. Acoust. Soc Am. 125, 1982–1994. doi: 10.1121/1.3089590
Martin S. W. (2009). The ecology and acoustic behavior of wintering minke whales in the Hawaiian and Pacific islands. techreport N0001409WX20964 (San Diego, CA: SPAWAR Systems Center Pacific).
Martin S. W., Marques T. A., Thomas L., Morrissey R. P., Jarvis S., DiMarzio N., et al. (2013). Estimating minke whale (Balaenoptera acutorostrata) boing sound density using passive acoustic sensors. Mar. Mamm. Sci. 29, 142–158. doi: 10.1111/j.1748-7692.2011.00561.x
Martin S. W., Martin C. R., Matsuyama B. M., Henderson E. E. (2015). Minke whales (Balaenoptera acutorostrata) respond to Navy training. J. Acoust. Soc Am. 137, 2533–2541. doi: 10.1121/1.4919319
McClintock B. T., Michelot T. (2018). momentuHMM: R package for generalized hidden Markov models of animal movement. Methods Ecol. Evol. 9, 1518–1530. doi: 10.1111/2041-210x.12995
McComb K. E. (1991). Female choice for high roaring rates in red deer, Cervus elaphus. Anim. Behav. 41, 79–88. doi: 10.1016/s0003-3472(05)80504-4
McElligott A. G., Hayden T. J. (1999). Context-related vocalization rates of fallow bucks, Dama dama. Anim. Behav. 58, 1095–1104. doi: 10.1006/anbe.1999.1237
Mellinger D. K., Martin S. W., Morrissey R. P., Thomas L., Yosco J. J. (2011). A method for detecting whistles, moans, and other frequency contour sounds. J. Acoust. Soc Am. 129, 4055–4061. doi: 10.1121/1.3531926
Nikolich K., Towers J. R. (2020). Vocalizations of common minke whales (Balaenoptera acutorostrata) in an eastern north Pacific feeding ground. Bioacoustics 29, 97–108. doi: 10.1080/09524622.2018.1555716
Noad M. J., Dunlop R. A., Mack A. K. (2017). Changes in humpback whale singing behavior with abundance: implications for the development of acoustic surveys of cetaceans. J. Acoust. Soc Am. 142, 1611–1618. doi: 10.1121/1.5001502
Nosal E.-M. (2013). Methods for tracking multiple marine mammals with wide-baseline passive acoustic arrays. J. Acoust. Soc Am. 134, 2383–2392. doi: 10.1121/1.4816549
Oswald J. N., Au W. W. L., Duennebier F. (2011). Minke whale (Balaenoptera acutorostrata) boings detected at the station ALOHA cabled observatory. J. Acoust. Soc Am. 129, 3353–3360. doi: 10.1121/1.3575555
Rankin S., Barlow J. (2005). Source of the north Pacific “boing” sound attributed to minke whales. J. Acoust. Soc Am. 118, 3346–3351. doi: 10.1121/1.2046747
R Core Team (2020). R: A language and environment for statistical computing (Vienna, Austria: R Foundation for Statistical Computing).
Smith J. N., Goldizen A. W., Dunlop R. A., Noad M. J. (2008). Songs of male humpback whales, Megaptera novaeangliae, are involved in intersexual interactions. Anim. Behav. 76, 467–477. doi: 10.1016/j.anbehav.2008.02.013
Southern L. K. (1981). Sex-related differences in territorial aggression by ring-billed gulls. Auk 98, 179–181. http://www.jstor.org/stable/4085622
Thompson P. O., Friedl W. A. (1982). A long term study of low frequency sounds from several species of whales off Oahu, Hawaii. Cetology 45, 1–19.
Towers J. R., McMillan C. J., Malleson M., Hildering J., Ford J. K. B., Ellis G. M. (2013). Seasonal movements and ecological markers as evidence for migration of common minke whales photo-identified in the eastern north Pacific. J. Cetacean Res. Manage. 13, 221–229.
Tyack P. (1981). Interactions between singing Hawaiian humpback whales and conspecifics nearby. Behav. Ecol. Sociobiol. 8, 105–116. doi: 10.1007/BF00300822
Vasconcelos R. O., Simões J. M., Almada V. C., Fonseca P. J., Amorim M. C. P. (2010). Vocal behavior during territorial intrusions in the Lusitanian toadfish: boatwhistles also function as territorial ‘keep-out’ signals. Ethology 116, 155–165. doi: 10.1111/j.1439-0310.2009.01722.x
Vu E. T., Clark C., Catelani K., Kellar N. M., Calambokidis J. (2014). Seasonal blubber testosterone concentrations of male humpback whales (Megaptera novaeangliae). Mar. Mamm. Sci. 31, 1258–1264. doi: 10.1111/mms.12191
Vu E. T., Risch D., Clark C. W., Gaylord S., Hatch L. T., Thompson M. A., et al. (2012). Humpback whale song occurs extensively on feeding grounds in the western north Atlantic ocean. Aquat. Biol. 14, 175–183. doi: 10.3354/ab00390
Waas J. R. (1991). The risks and benefits of signalling aggressive motivation: a study of cave-dwelling little blue penguins. Behav. Ecol. Sociobiol. 29, 139–146. doi: 10.1007/bf00166489
Wells K. D. (1978). Territoriality in the green frog (Rana clamitans): vocalizations and agonistic behaviour. Anim. Behav. 26, 1051–1063. doi: 10.1016/0003-3472(78)90094-5
Wenz G. M. (1964). Curious noises and the sonic environment in the ocean. Mar. Bio-acoustics 1, 101–119.
Wiewandt T. A. (1969). Vocalization, aggressive behavior, and territoriality in the bullfrog, Rana catesbeiana. Copeia 1969, 276. doi: 10.2307/1442074
Wingfield J. C. (1994). Control of territorial aggression in a changing environment. Psychoneuroendocrinology 19, 709–721. doi: 10.1016/0306-4530(94)90052-3
Keywords: minke whale (Balaenoptera acutorostrata), inter-call interval (ICI), calling rate, cue rate, bioacoustics, passive acoustic monitoring, animal behavior, marine ecology
Citation: Martin CR, Guazzo RA, Helble TA, Alongi GC, Durbach IN, Martin SW, Matsuyama BM and Henderson EE (2022) North Pacific minke whales call rapidly when calling conspecifics are nearby. Front. Mar. Sci. 9:897298. doi: 10.3389/fmars.2022.897298
Received: 16 March 2022; Accepted: 28 July 2022;
Published: 22 August 2022.
Edited by:
Ana M. M. Sequeira, University of Western Australia, AustraliaReviewed by:
Denise Risch, Scottish Association For Marine Science, United KingdomDavid Moretti, Naval Undersea Warfare Center (NUWC), United States
Copyright © 2022 Martin, Guazzo, Helble, Alongi, Durbach, Martin, Matsuyama and Henderson. This is an open-access article distributed under the terms of the Creative Commons Attribution License (CC BY). The use, distribution or reproduction in other forums is permitted, provided the original author(s) and the copyright owner(s) are credited and that the original publication in this journal is cited, in accordance with accepted academic practice. No use, distribution or reproduction is permitted which does not comply with these terms.
*Correspondence: Cameron R. Martin, cameron.r.martin9.civ@us.navy.mil