- 1State Key Laboratory of Water Environment Simulation & School of Environment, Beijing Normal University, Beijing, China
- 2Yellow River Estuary Wetland Ecosystem Observation and Research Station, Ministry of Education, Shandong, China
- 3Tang Scholar, Beijing Normal University, Beijing, China
- 4Research and Development Center for Watershed Environmental Eco-Engineering, Beijing Normal University, Zhuhai, China
- 5Horn Point Laboratory, University of Maryland Center for Environmental Science, Cambridge, MD, United States
As an aggressive invasive salt marsh plant, Spartina alterniflora has been found to invade along tidal channel networks and threaten native salt marsh ecosystems. Previous studies have established patterning correlations between S. alterniflora invasion and tidal channel functions (drainage efficiency). However, a systematic analysis of S. alterniflora invasion in relation to functional and geometric features of tidal channel networks is still lacking. In this study, we extracted tidal channel networks from remote sensing images of the Yellow River Delta, China, and performed numerical experiments to examine S. alterniflora invasion patterns with tidal channel networks with varying drainage efficiency and geometric nuances. An existing vegetation dynamics model was adapted to incorporate hydrochorous seed dispersal and salinity buffer zone as the primary mechanisms of tidal channels to facilitate vegetation colonization and was further coupled with Delft3D. We analyzed the correlation of the simulated S. alterniflora area with a comprehensive set of tidal channel functional and geometric metrics across different spatial scales. Our results confirmed that watersheds with higher drainage efficiency (larger tidal channel density (TCD) and geometric efficiency (GE), smaller overmarsh path length (OPL)) attained larger S. alterniflora area. Given a similar drainage efficiency, tidal channel networks with greater geometric mean bifurcation ratio enhanced S. alterniflora invasion. On a local scale, channel order dictated local drainage efficiency (spatially-varying TCDo) and further influenced S. alterniflora area. The observed patterns were further verified in principle by two real cases in the Yellow River Delta. Finally, in viewing the efficacy of all metrics tested and further considering their computational costs, we proposed a holistic metric framework consisting of global metrics including TCD and geometric mean bifurcation ratio and local metric including spatially-varying TCDo, to assess how tidal channel network mediates S. alterniflora invasion in particular and salt marsh vegetation expansion in general in marsh-channel systems.
Introduction
Salt marshes are one of the most valuable ecosystems located between land and ocean, which provide a variety of crucial ecosystem functions such as habitat provision, biodiversity maintenance and carbon fixation (Barbier et al., 2011). However, invasive plants, such as Spartina alterniflora, have been found to invade salt marsh ecosystems worldwide, causing severe ecological and societal impacts (Ning et al., 2021a). Over the past decades, the typical invasive salt marsh plant S. alterniflora, a perennial rhizomatous grass native to the East Coast of North America, has been invading rapidly to estuarine and coastal salt marshes in other parts of the world (Zheng et al., 2018). Because of its high water-logging and salinity tolerance, S. alterniflora was first introduced to China in 1979 and subsequently widely transplanted on mudflats along the coast of China for erosion control and dike protection (An et al., 2007). Due to its ability in both sexual reproduction through seed dispersal and asexual reproduction through the clonal spread, S. alterniflora has gradually become one of the dominant species in low marshes along the coast of China (Xiao et al., 2011; Zhu et al., 2014), causing great damage to the structure and functioning of the local ecosystems (Li et al., 2009). Recent field observations have shown that S. alterniflora invades into middle and high marshes primarily through tidal channel networks (Tyler and Zieman, 1999; Schwarz et al., 2016; Qi et al., 2017; Ning et al., 2020; Sun et al., 2020) (Figure 1), which is in line with previous findings that marsh vegetation distribution is strongly affected by tidal channel network (Lathrop et al., 2003; Elsey-Quirk and Leck, 2021; Ning et al., 2021b). Therefore, an in-depth understanding of the role of tidal channel network on S. alterniflora landward invasion in the marsh‐channel system is imperative for invasion prediction and control.
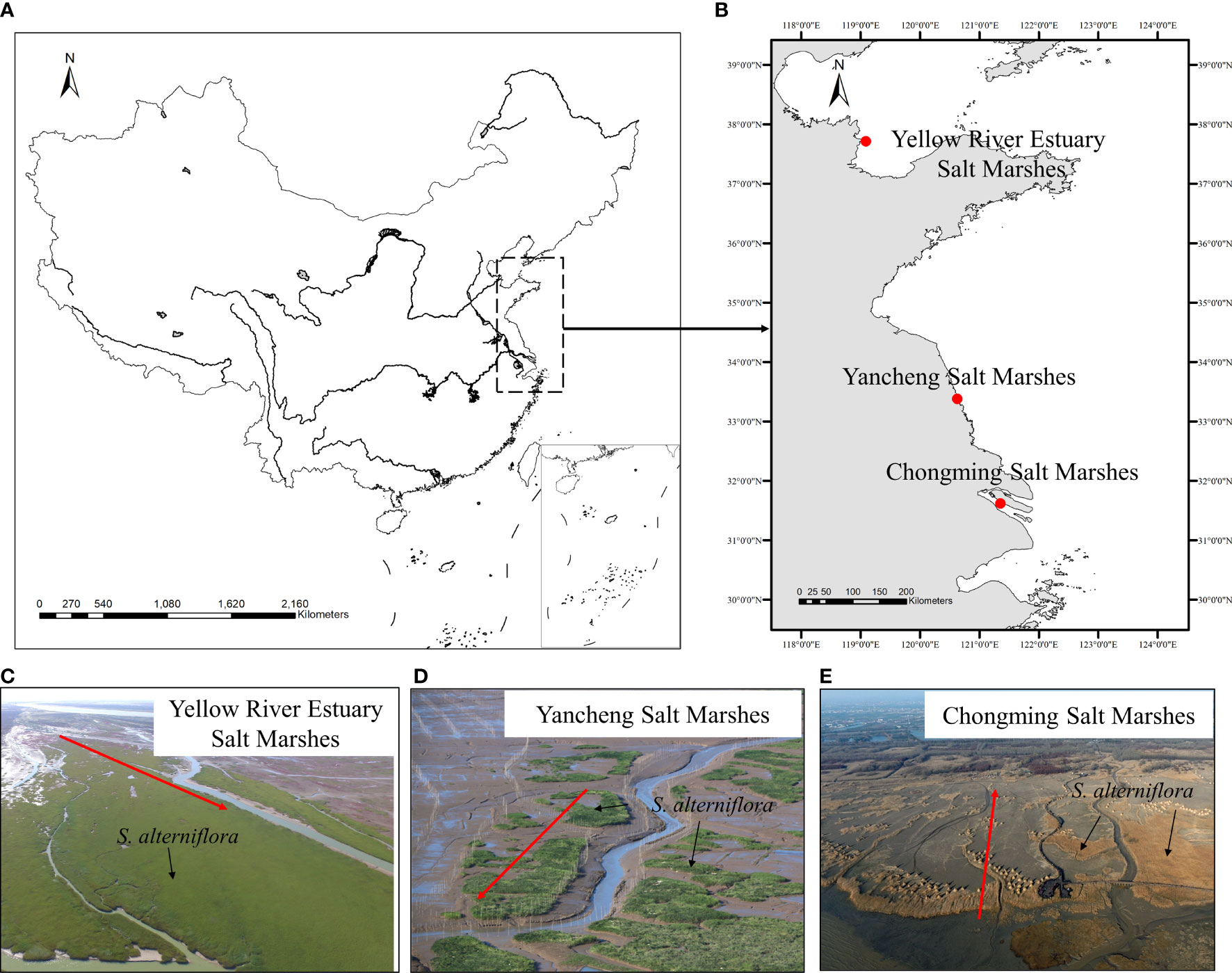
Figure 1 Examples of Spartina alterniflora landward invasion along with tidal channel networks in China. (A) Map of China; (B) Locations of example invasion sites; (C) Yellow River Estuary Salt Marshes. Photo credit: Z.H. Ning and X. Ma; (D) Yancheng Salt Marshes (Source: http://img.itc.cn/photo/olbZcUsvYoA); (E) Chongming Salt marshes (Source: http://photocdn.sohu.com/20160107/Img433760555.jpg). The red arrow represents the direction of invasion.
As a basic geomorphic feature of the marsh‐channel system, tidal channel network controls hydrodynamics, transport of sediment and biota in and between salt marshes (Kearney and Fagherazzi, 2016; Taramelli et al., 2018). The hydrochorous seed dispersal, i.e., water-mediated seed dispersal, is an important biota transport process mainly driven by tidal currents and a primary factor influencing plant community development in a marsh‐channel system (Chang et al., 2007; Shi et al., 2019). During periodic high tides that inundate the marsh platform, seeds fallen from their parent plants drift with the tidal current, and areas closer to tidal channels tend to trap more seeds, especially in middle and high marshes (Leck, 2003; Chang et al., 2007; Hopfensperger et al., 2009; Crawford et al., 2015; Shi et al., 2019). Moreover, periodic high tides flush and lower soil salinity near the channels, providing a salinity buffer zone for germination of retained seeds and establishment of seedlings (Silvestri et al., 2005; Ning et al., 2021b). The resulted plant patches along the tidal channel gradually merge into the contiguous community and expand landward (Sun et al., 2020; Wang et al., 2021). Therefore, tidal channel networks facilitate vegetation expansion primarily through these two mechanisms in marsh-channel systems. Both mechanisms are further dictated by the functions of the tidal channel network, which can be quantified by its drainage efficiency and the associated metrics, such as unchanneled flow lengths (UFL), tidal channel density (TCD), overmarsh path length (OPL) and geometric efficiency (GE) (Marani et al., 2003; Chirol et al., 2018).
In addition, geometric features such as bifurcation, channel sinuosity and channel orders are also found to influence the functions of channel network and vegetation distribution (Sanderson et al., 2000; Kim et al., 2013b; Mou et al., 2021). Amongst the major geometric features, bifurcation refers to the splitting of a channel into two separate channels (Kaspar, 2019). It affects hydrological and biogeochemical processes in and between marshes and ultimately affects vegetation distribution (Mou et al., 2021). Geometric mean bifurcation ratio, as a metric of bifurcation, is widely used to reflect the level of development of tidal channel networks and characterize the global geometry of a network system (Li et al., 2019; Mou et al., 2021). In contrast, both channel sinuosity and order are measured on a single channel and thus indicate geometric features at the local scale, which have also been found to influence vegetation patterns near channel margins (Sanderson et al., 2001; Kim et al., 2013b).
Recently, the patterning relationship between tidal channel networks and vegetation distribution at the landscape scale has been studied using remote sensing imagery. Specifically, Sun et al. (2020) observed that tidal channels with higher drainage efficiency (smaller OPL) attained larger S. alterniflora area, and the high-order channels were the main pathways for S. alterniflora invasion at the south bank of the Yellow River Estuary, China. Similarly, Liu et al. (2020) reported that in the northern Liaodong Bay, an efficient channel network (smaller UFL and larger TCD) alleviated abiotic stresses, i.e., soil moisture and salinity stress, and led to a larger Suaeda salsa area, and the extent of vegetation die-off increased with increasing lateral distance from tidal channels. Furthermore, several studies reported that vegetation area and abundance increased approximately linearly with channel size, which was roughly correlated with channel order (Sanderson et al., 2000; Fan et al., 2020). Moreover, an inverse logarithmic relationship between channel sinuosity and vegetation patch size at the local scale was found by Taramelli et al. (2018).
To sum up, previous studies have established the correlations of drainage efficiency and geometric features of tidal channel network with S. alterniflora invasion in particular and vegetation expansion in general (Taramelli et al., 2018; Fan et al., 2020; Liu et al., 2020; Sun et al., 2020). A tidal channel network with high drainage efficiency tends to provide a relatively larger suitable area for vegetation colonization (Kearney and Fagherazzi, 2016; Sun et al., 2020). Moreover, geometric features such as channel bifurcation and sinuosity, further mediate vegetation distribution (Sun et al., 2020). Although the existing studies including those mentioned above provide valuable insight into the effects of tidal channel networks on vegetation expansion, they are largely qualitative and concern only a limited number of metrics, and systematic analysis in relation to the functional and geometric features of tidal channel networks is still lacking. The latter can improve our understanding of the underlying mechanism and enhance our assessment and predictive capability for S. alterniflora invasion control.
As an attempt to fill the gap, we performed carefully designed numerical experiments using process-based models to mechanistically test the hypothesis that a higher drainage efficiency network obtains a larger S. alterniflora invasion area in a marsh-channel system. Moreover, we tested a secondary hypothesis that given the same drainage efficiency, the additional geometric nuances also make a difference for vegetation distribution. We adapted an existing S. alterniflora dynamics model to incorporate a new hydrochorous seeds dispersal module. Tidal channel networks with varying drainage efficiency were schematized from remote sensing images of the Yellow River Delta (YRD), China as an exemplary site where S. alterniflora invasion follows tidal channel networks (Sun et al., 2020). Here, we assumed that tidal channel geomorphology was in an equilibrium state and also neglected the vegetation feedback on tidal channel evolution, as vegetation mainly has stabilizing effects on pre-existing channel geomorphology (Schwarz et al., 2014; Taramelli et al., 2018). The simulated hydro- and morpho-dynamic results of these schematized tidal channel networks from Delft3D (Lesser et al., 2004) were used to drive the vegetation dynamics model to simulate a landward invasion of S. alterniflora. Statistical correlations between the simulated vegetation area and a comprehensive set of functional and geometric metrics were analyzed over the local and watershed scales. The simulated spatial patterns of S. alterniflora invasion and their evolution dynamics were verified against two real cases in YRD as well. Finally, a holistic metric framework was proposed to assess how the tidal channel network affects S. alterniflora invasion in particular and salt marsh vegetation expansion in general. According to our hypothesis, the following specific questions are addressed: (1) How does S. alterniflora distribution pattern relate to functional features of tidal channel networks as quantified by various drainage efficiency metrics? (2) Under a similar drainage efficiency, will S. alterniflora invasion be sensitive to channel geometric features, such as channel sinuosity and bifurcation? Answering the above questions can help advance our understanding of how tidal channel network mediates S. alterniflora invasion, and also assist in designing effective control strategies to prevent exotic species invasion in marsh-channel systems.
Methods and Model Description
Tidal Channel Scenarios
We firstly explored how drainage efficiency, which was characteristic of functional features of tidal channel network and commonly measured by TCD, affected S. alterniflora invasion. Specifically, we extracted the tidal channel networks from remote sensing images of the YRD and set them as the initial geomorphology similar to Finotello et al. (2019). We selected the YRD as it is an exemplary site where a landward invasion of S. alterniflora followed the well-developed tidal channel networks as the main pathways (Fan et al., 2020; Sun et al., 2020). Considering the development stage of the tidal channel networks, we selected Landsat images of the YRD from the years 2010, 2014, 2016 and 2020. All images (see Table 1) were selected at low tides and cloud cover to facilitate distinguishing tidal channel networks, and the selected images were further processed for radiometric calibration and FLAASH atmospheric correction in ENVI 5.3 (Sun et al., 2020). Afterward, we merged the panchromatic band with multispectral bands through Gram–Schmidt pan sharpening to improve image resolution and conducted geometric correction. After processing all images, the tidal channel networks were extracted using the Normalized Difference Water Index (McFeeters, 1996) and further refined by visual interpretation. Previous studies suggested that interpretation of lower-order channels (2nd order and below) was laborious and usually led to artifacts that did not reflect the actual structure of the channel network in question (Kearney and Fagherazzi, 2016). Further considering that S. alterniflora invasion mainly follows high order channel as the main pathways (Sun et al., 2020), we only extracted channels above second order from the images. Lastly, we compared the channel networks extracted from different-year images, and the well-developed ones with minor changes in their geomorphology after S. alterniflora invasion were finally retained to be used for subsequent numerical experiments.
Our model domain included three closed land boundaries and one open seaward boundary (see Sec. 2.2). Because of the boundary configuration, the model domain, where the initial elevation is above 0 m, can be considered as a single watershed with a fixed area. Under such assumption, the tidal channel density was calculated by tidal channel length divided by the prescribed watershed area, which ranged from 0.0014 m-1 to 0.0064 m-1. In our simulation scenarios, the tidal channel networks varied from linear dendritic to dendritic patterns with increasing tidal channel density (Hughes, 2012). We divided them into three classes with high, medium and low TCDs, respectively, based on natural breaks classification (Jenks, 1967) of their respective TCD values (Figure 2). The natural breakpoints were 0.0027 m-1 and 0.0039 m-1. In each class, three-channel networks with the same or similar TCD value were selected as replicates. Once selected the channel networks with the same or similar TCD value, we gave priority to the ones with different geometric mean bifurcation ratio.
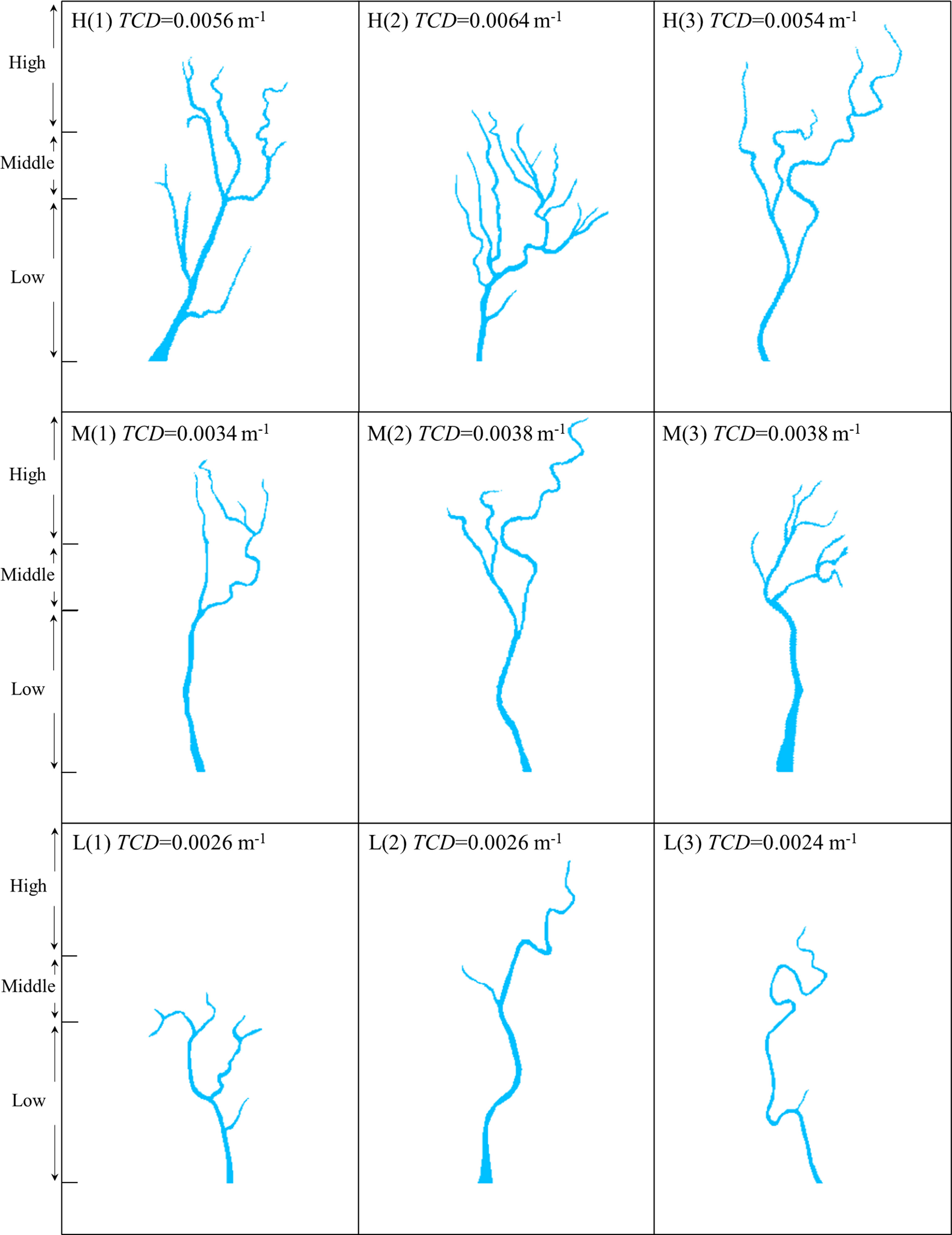
Figure 2 The geometries of tidal channel networks used in our numerical experiments. (H) High tidal channel density; (M) Middle tidal channel density; (L) Low tidal channel density. The marsh platform was divided into low, middle and high marshes based on elevation.
Model Description and Setting
In this study, we used Delft3D, which is a process-based numerical model that solves hydrodynamics, sediment transport and morphodynamics in a coupled fashion, to simulate hydro- and morpho-dynamics of the schematized tidal channel networks (Lesser et al., 2004). Based on a previously developed S. alterniflora dynamics model (Zheng et al., 2022), we further incorporated a hydrochorous seed dispersal module to account for seed dispersal in tidal channel networks. The upgraded S. alterniflora dynamics model was coupled with the Delft3D model to explore how the tidal channel network mediates S. alterniflora landward invasion.
Delft3D Model Setting
A rectangular watershed-scale computational domain (3,000 m × 1,500 m) was set up. As suggested by Best et al. (2018), a grid size of 10 m×10 m is sufficient for channel description. Recent studies have shown that the width of high order channel in YRD ranged from hundreds of meters in low marsh to several meters in high marsh (Sun et al., 2020; Wu et al., 2020). Considering the smaller channel size in high marshes, we adopted a grid size of 5 m×5 m. The initial bed elevation increased from -2 m at the seaward boundary to 2 m at the landward boundary over a cross-shore distance of 3,000 m. This elevation gradient covers low, middle and high marshes (Wang et al., 2018). Here, we divided marsh platforms into low, middle and high marshes with different elevations based on vegetation type, tidal range and elevation in the YRD (see Figure 3C) (Wang et al., 2018; Xie et al., 2019; Ning et al., 2021b). The marshes above 1.27 m elevations were considered as high marshes, while marshes below 0.9 m were low marshes, and marshes with elevation in between were identified as middle marshes. The seaward boundary was prescribed as an open boundary and the rest were impermeable. An example of the model setting and domain is shown in Figure 3A.
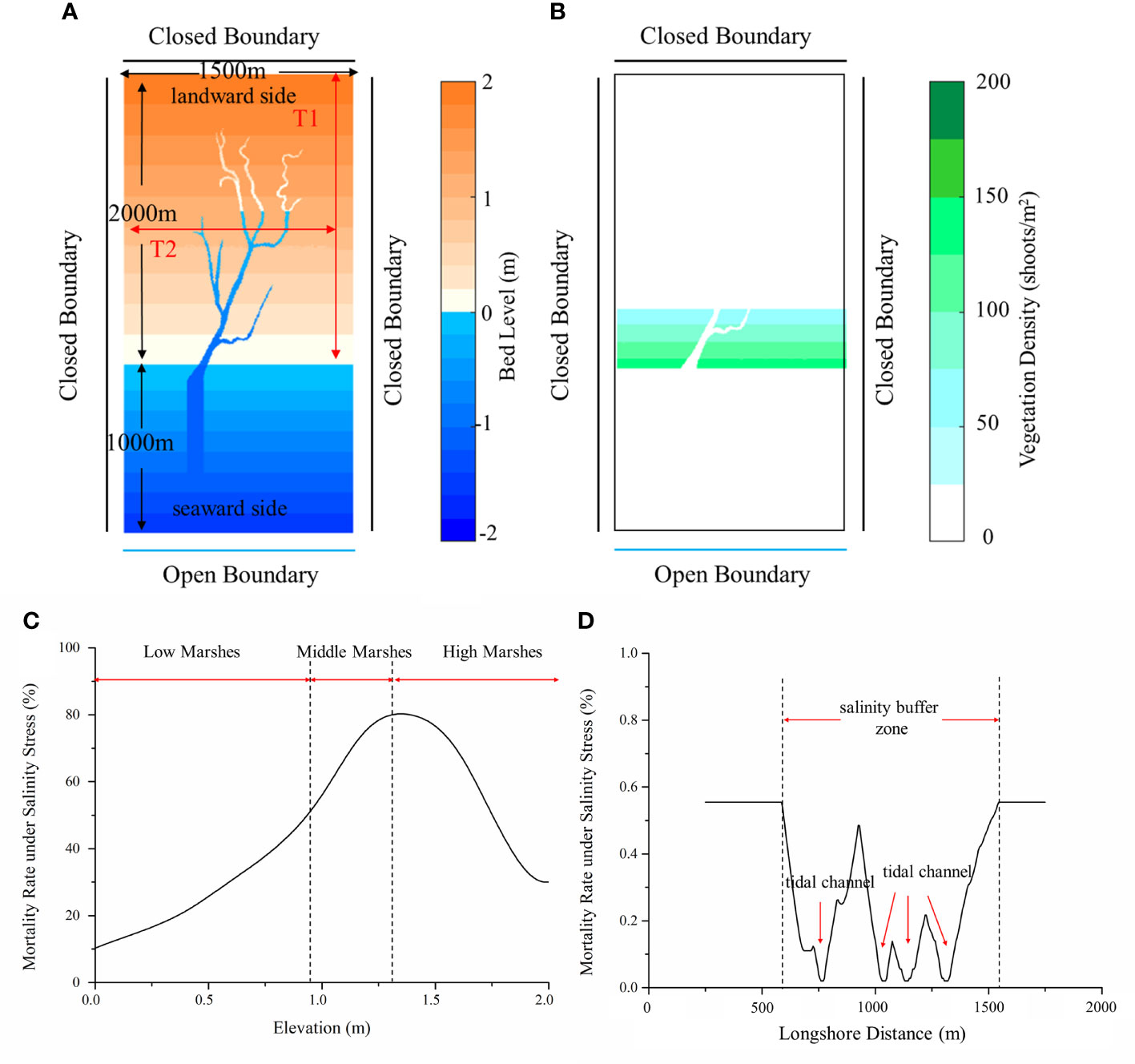
Figure 3 Initial model setting of scenario B: (A) Bed level, domain and boundary conditions; (B) Initial vegetation distribution; (C) Mortality rate under salinity stress along transect 1 (T1) [adapted from Wang et al. (2018)]; (D) Mortality rate under salinity stress along transect 2 (T2).
We set the tidal range, water depth, sediment concentration, and sediment grain size with values representative of the YRD following Wang et al. (2021), which developed a biogeomorphic model to simulate marsh platform evolution in the YRD. Specifically, we assumed suspended sediment concentration to be 0.002 kg/m3 at the seaward boundary. Two types of sediments with a median grain size of 7.5 μm and 28 μm were adopted. Similar to Best et al. (2018), a Thatcher-Harleman time lag of 120 min was defined to prevent sudden variations in the suspended sediment concentration and the associated numerical instabilities. Here, we only considered tides and neglected waves because the transport of propagules and seeds was mainly due to tidal currents (Huiskes et al., 1995; Chang et al., 2008), and fluctuating water level due to simplified 1 m semidiurnal tides at the seaward boundary was set accordingly. The critical shear stress for erosion was 0.1 N/m2. A uniform Chézy roughness value of 65 m1/2/s was set. The hydrodynamic time-step was set to 15 s for Delft3D to ensure simulation accuracy and stability. The morphological acceleration factors of 12 and 120 were set for the seed dispersal period and the rest of the year, respectively, as more accurate hydrodynamic results were required for the simulation of hydrochorous seed dispersal in the former case.
Finotello et al. (2019) set a uniform initial water depth of 4 m and ran Delft3D to allow geomorphological evolution to attain quasi-equilibrium conditions. This setting would induce significant hydro- and morpho-dynamic perturbations during the initial simulation stage and thus require sufficient spin-up time (Finotello et al., 2019). Here, following Finotello et al. (2019), we adopted a uniform initial tidal channel depth to be 1 m based on field observation in the YRD (Wu et al., 2020). Then, we set a 24-hour hydrodynamic spin-up time and run another 1-year simulation for each scenario to evolve tidal channels and allow the geomorphological evolution to reach the equilibrium condition. The obtained tidal channel networks were used as a geomorphological template for subsequent coupled ecogeomorphological simulations.
Spartina alterniflora Dynamics Model
The S. alterniflora dynamics model was adapted from Zheng et al. (2022). A hydrochorous seed dispersal module was incorporated to account for seed dispersal in tidal channel networks. A brief introduction of the upgraded S. alterniflora dynamics model was presented in the following. The detailed information of the existing model can be found in Zheng et al. (2022).
Model Framework
As an aggressive invasive species, S. alterniflora has the ability in both sexual reproduction through seed dispersal and asexual reproduction through clonal spread (Xiao et al., 2011). In accordance with the life history and traits of S. alterniflora, our model comprised four components, i.e., seed germination, clonal spread, hydrochorous seed dispersal and mortality. Following Zheng et al. (2022), we introduced Windows of Opportunity (WoO) and plant life cycle to capture vegetation dynamics in different plant growth stages. The plant life cycle contained four components, i.e., seed germination, clonal spread, seed production and hydrochorous seed dispersal. These four ecological processes were controlled by four phenological points (t1, t2, t3, t4) (see Figure 4) and their durations were further defined using Boxcar function, i.e.
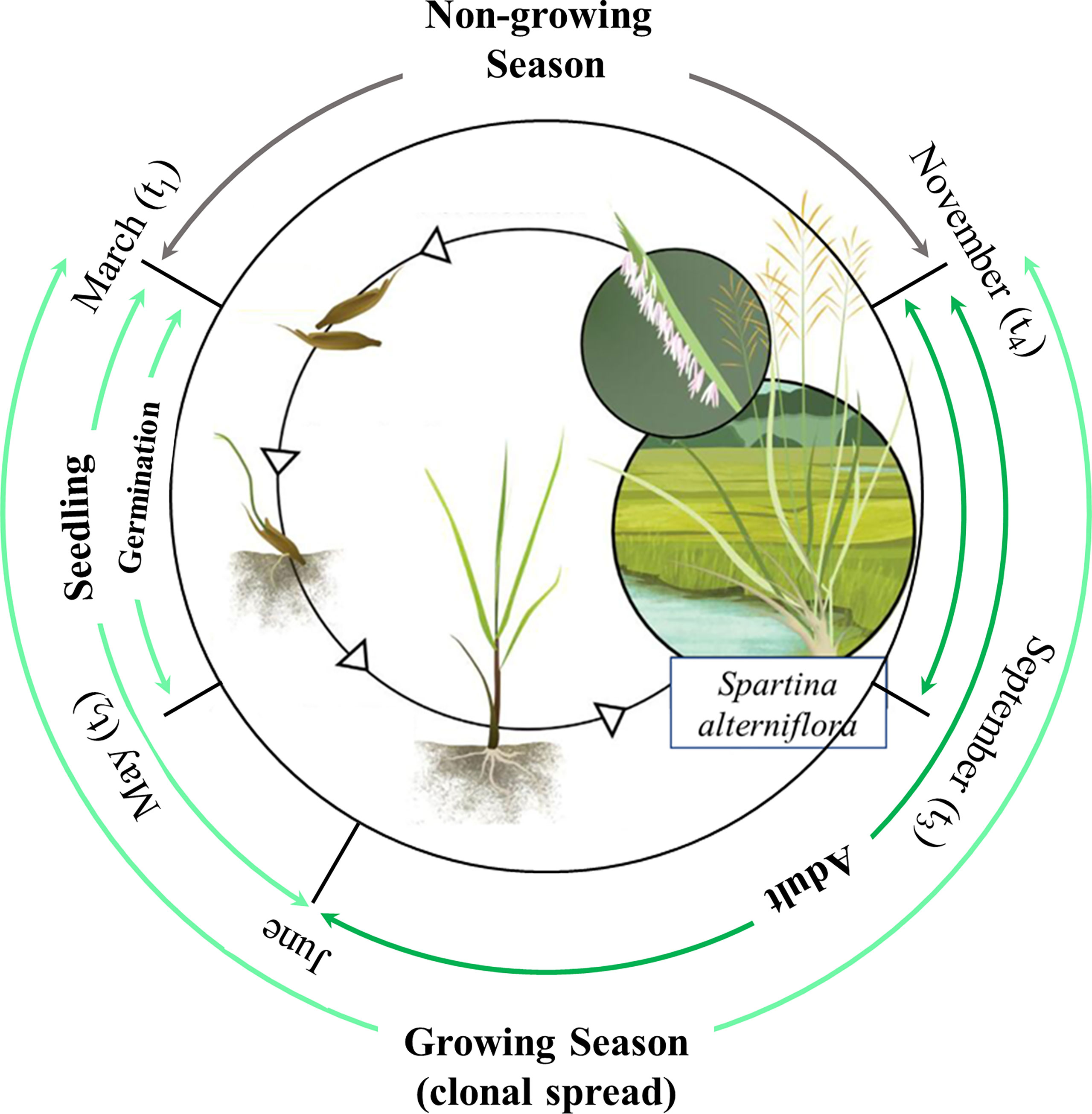
Figure 4 Plant life cycle and Windows of Opportunity of Spartina alterniflora [adapted from Vanderklift et al. (2020)].
Model Equations
Following Takahashi et al. (2019) and Zheng et al. (2022), the vegetation density (number of shoots/m2) was modelled by incorporating clonal spread, plant self-growth, seed germination and mortality. Here, we used diffusion term to simulate the lateral clonal spread of S. alterniflora as it could successfully reproduce its circular colony pattern (Takahashi et al., 2019; Wang et al., 2021). Afterwards, logistic growth function was used to simulate plant self-growth under Allee effect in a new colony (Taylor et al., 2004; Taylor and Hastings, 2004; Wang et al., 2021). The governing equation is as follows,
is the Laplacian operator, r is the maximum growth rate (day-1), Cmaxis the maximum density supported by the system (i.e. carrying capacity, number of shoots/m2), Rger is seed germination rate depending on hydrodynamics and soil salinity (shoots/seeds), Ss is the number of seeds in seedbank after hydrochorous seed dispersal (number of seeds/(m2.day)), Rsmor_v is the mortality rate of seedling under hydrodynamic stress (%), Rmor_s is the mortality rate of vegetation under salinity stress (%), Ramor_v is the mortality rate of adult plant under hydrodynamic stress (day-1), is the logistic growth term to simulate the population self-growth,RgerSs(1-RsmorH_v)(1-Rmor_s) is the survived seedling under the stress of hydrodynamics and salinity, and PRamor_v is the dead adult plants under hydrodynamic stress.
As reported by Griffith and Forseth (2002), there are two phases in their dispersal once seeds leave their parental plants. The primary dispersal is the movement from their parental plants to the nearby ground by gravity, which is characterized generally as short-distance dispersal. Most of the seeds fall fairly close to the parental plants, and the seed density declines exponentially with the distance away from the plants (Griffith and Forseth, 2002). Therefore, we used another diffusion term (Ds) to simulate the primary seed dispersal. The second phase is hydrochorous seed dispersal, which is relatively more important for vegetation colonization than primary seed dispersal, especially for invasive species (Shi et al., 2019). As reported in previous studies, the fallen seeds could be dispersed by tidal currents for long-distance after falling on the ground or entering a tidal channel (Ge et al., 2015; Ning et al., 2021b). Therefore, similar to Ge et al. (2015), we assumed that the fallen seeds were directly carried away by tidal currents when the grids were occupied by water. For vegetated grids distributed along the margins of the tidal channels, we followed Crawford et al. (2015) and assumed that the seeds could directly fall into tidal channels even if the grids were dry.
The advection equation has been successfully used in simulating long-distance seed and propagule dispersal in fluvial (Cunnings et al., 2016), tidal (Ruiz-Montoya et al., 2015) and lacustrine (Vilas et al., 2017) environments. Therefore, we used the advection term (∇RspreadSm) to simulate hydrochorous seed dispersal. We assumed that mobile seeds with concentration Sm (number of seeds/m2) moved in the same direction as tidal currents. Based on this assumption, seeds would not move again if they arrived on a grid that was flooded and subsequently dried. Besides, we followed Crawford et al. (2015) and assumed that only if the deposition location of a mobile seed in a channel was less than 5 m (one grid size) from the channel edge could the seed be captured and redistributed in the grids near the channel. Otherwise, the seed would drown. The resulted governing equation for hydrochorous seed dispersal is as follows,
where Rspread is the velocity of seed dispersal due to tides-induced advection (m.day-1), τd is the ratio of mobile seeds floating time to one year (%), k is the rate of conversion of mobile seeds to seeds in seedbank (day-1), Rpro is the number of seeds produced per day (number of seeds/(shoot. day)), Dsis the diffusion coefficient from primary seed dispersal (m. day-1).
The final distribution of seeds after dispersal is strongly influenced by the interplay between tidal currents and trapping agents such as vegetation and microtopographic features (Wang et al., 2018). Previous study has reported that spatial distribution of seeds in seedbanks across salt marshes is strongly correlated with the patterns of adult plant abundance, due to greater seed production where adults are more abundant and limited subsequent dispersal away from the parental plants (Rand, 2000). Therefore, we adopted a normalized index to calculate seed retention rate, which was related to vegetation density in each grid (see Eq. 5). Specifically, the seed retention rate on grids with maximum vegetation density was calculated by the number of seeds in seedbank divided by total seed production during the fruiting stage, which was around 20% based on field observation (Xiao et al., 2009). For bare flats, i.e., the part of the marsh platform without vegetation, even though seed rarely ends up on bare flats (Xiao et al., 2009), we set a minimal probability of 0.01% for seed retention to account for accidental events. Moreover, the number of seeds in seedbank varied as a result of herbivory, fungal attack and rot (Xiao et al., 2009; Zheng et al., 2018; Ning et al., 2019). Hence, we set a seed exponential decay term to incorporate the change in the number of seeds in seedbank over time until the next growing season based on field observation results (Xiao et al., 2009). The resulted governing equation for seedbank dynamics is as follows,
When seeds start to germinate in the next growing season, their survival are subject to soil salinity and hydrodynamic condition (Ning et al., 2020; Ning et al., 2021b). Similar to Brückner et al. (2019), linear dose-effect relations in which the mortality rate increases with increasing pressure were adopted to calculate the mortality caused by hydrodynamic stress during the seedling and adult stages as follows,
where v is depth-averaged grid velocity (m/s); vsmax/vsmin is the velocity upper/lower limit for linear seedling mortality range (m/s); vamax/vamin is the velocity upper/lower limit for linear adult mortality range (m/s).
Based on field observation, Ning et al. (2021b) found that even though S. alterniflora landward invasion was strongly inhibited by hypersaline zones in middle and high marshes, tidal channels significantly reduced soil salinity along channel margins and provided a buffer zone for S. alterniflora survival (see Figure 3C). In this study, we did not simulate soil salinity directly, because soil salinity in a marsh-channel system is controlled by complex interactions between tides, groundwater, evaporation and precipitation (Xin et al., 2017; Wang et al., 2020). As suggested by Belliard et al. (2015) and Wang et al. (2021), elevation could be considered as a proxy for multiple abiotic factors, such as soil salinity and inundation, in salt marshes. It could be further used to determine the survival of vegetation along the elevation gradient. Therefore, based on the field survey results and relevant previous studies, we made a simplifying assumption that soil salinity followed one-hump variation along an elevation gradient from low marshes to high marshes (Wang et al., 2018; Ning et al., 2021b), and further assumed that vegetation mortality under salinity stress was correlated with local salinity (see Figure 3C). Besides, the mortality in the salinity buffer zone along the tidal channel was assumed to increase linearly with the lateral distance from the channel (see Figure 3D) (Ning et al., 2021b), and the normalized mortality rate of vegetation under salinity stress was adapted from Qi et al. (2017). Detailed information of all modeling variables and parameters are documented in Table 2.
Simulation of Coupled Ecogeomorphological Model
Before all formal scenario simulations, we ran a preliminary simulation to obtain initial vegetation distribution (Figure 3B). Specifically, we started with the assumption that all grids located in low marshes (0-0.9m) were covered by vegetation, and simulated vegetation evolution using the one-year results of the template hydro- and morpho-dynamics simulation (see Figure 3A). We further used the resultant vegetation distribution to run a three-year simulation of both Delft3D and the vegetation dynamics model. The hydrochorous seed dispersal was simulated in the first and second year, and terminated in the third year as the resulting changes in seedbank were irrelevant for the simulation period. For simulation stability and accuracy, the coupling time interval for the two models was two hours during the fruiting and seed dispersal periods in the first and second years. To save computing costs, it was adjusted to one day in the remaining simulation period. The outputs of the Delft3D simulation (bed level, water depth, land/sea boundary and velocity) during this interval were averaged and fed as input for the vegetation dynamics model.
To focus on the effects of tidal channel network on S. alterniflora landward invasion, we adopted the one-way coupling approach in this study to simulate S. alterniflora invasion in established tidal channel network in the equilibrium state, i.e., minor change in its geomorphology regardless of the vegetation. The flowchart of the modeling approach is shown in Figure 5. This simplification is reasonable because vegetation mainly exerts a stabilizing effect on tidal channel geomorphology if the channels are already formed on mudflats (Taramelli et al., 2018). The vegetation feedback on tidal channel evolution is more significant during the initial evolution of low-order channels at the local scale (Schwarz et al., 2014; Liu et al., 2021), while the evolution of high-order channels at the watershed scale usually lasts for decades with negligible vegetation intervention (Schwarz et al., 2016; Wang et al., 2021). In our simulation scenarios, we only selected the channel networks with minor changes in geomorphology after S. alterniflora invasion and further ran Delft3D to allow the geomorphology of the schematized tidal channel network to reach equilibrium condition, to ensure that the above assumptions were held.
Verification and Analyses of Numerical Results
To verify the reality of our simulated results, the invasion patterns and evolution dynamics between simulated results and two real cases in the YRD were compared. The distributions of S. alterniflora in the YRD at the landscape scale were mainly extracted from Gaofen remote sensing images, which were downloaded from China Centre for Resources Satellite Data and Application (http://www.cresda.com/CN/). Due to its long revisit period and high cloud cover, the Gaofen images were unable to cover the entire invasion period of the real cases. Therefore, Landsat images from the United States Geological Survey (https://earthexplorer.usgs.gov/) were also selected as a complementary data source. Following Sun et al. (2020), we used the maximum likelihood classification method in ENVI 5.3 to interpret S. alterniflora distribution. The information of remote sensing images was shown in Table 1.
As for the numerical results, we firstly analysed the relationships between S. alterniflora area and functional features of the tidal channel network, which were quantified by drainage efficiency metrics including TCD, OPL and GE (Marani et al., 2003; Chirol et al., 2018). Following Ge et al. (2015), a grid was considered to be occupied by vegetation when vegetation density exceeded 2/3 of maximum capacity. The unchanneled path length (UPL) is defined as the distance a drop of water has to travel on the marsh platform before it reaches the closest channel (Marani et al., 2003; Sun et al., 2020). The slope of the first 50 values of the exceedance probability distribution of UPL is defined as OPL (Chirol et al., 2018). Compared to TCD, it further distinguishes tidal channel networks with different spatial patterns, and a smaller OPL value indicates a well-distributed channel system (Marani et al., 2003). The geometric efficiency is defined as the Hortonian length (the inverse of channel density) divided by OPL, i.e.
This indicator reveals how well a channel network serves the marsh platform through its branching features and meandering characteristics. For a given channel length and basin area, a larger geometric efficiency means a better-distributed channel system and a larger portion of the marsh platform is closed to the channel (Kearney and Fagherazzi, 2016).
To test our secondary hypothesis on whether S. alterniflora landward invasion is sensitive to channel geometric features at the watershed scale, we further examined the relationships between vegetation area and geometric mean bifurcation ratio (B) (Tucker et al., 2001). The geometric mean bifurcation ratio is calculated by the number of the 1st-order channels and the maximum order of tidal channel network (Ichoku and Chorowicz, 1994), i.e.
where N1 is the number of the 1st-order channels, and Ω is the maximum order of tidal channel network. The order of tidal channel was determined using Horton-Strahler method (Strahler, 1957). All terminal channels were assigned as order 1. When two channels of the same order met, their confluence channel was one order higher. Then, we analysed how the geometric mean bifurcation ratio affected vegetation area under similar drainage efficiency using partial correlation analysis (Carroll and Green, 1997).
Furthermore, we analysed how the tidal channel network affected S. alterniflora landward invasion at the local scale through spatially varying TCDs. Previous studies reported that channel sinuosity and order were both the basic metrics defined at the scale of a single channel (Sanderson et al., 2001; Kim et al., 2013b). Following Sun et al. (2020), spatially-varying TCDs were defined within a specified search circle moving around the domain and further incorporating a weighting factor related to the order and/or sinuosity of the tidal channel. Following Taramelli et al. (2017), channel sinuosity was calculated as the ratio of the axial channel length l to the straight line distance L between the starting and ending points of each channel. After calculating the spatially-varying TCDs in each grid, we sorted all TCD values and counted the corresponding frequency number. The associated equations are as follows,
where worder is the weighting factor of channel order; ri is the sinuosity ratio of ith order tidal channel; Li is the length of ith order tidal channel that falls within the search circle; A is the area of the search circle. TCDo is spatially-varying TCD related to order of the tidal channel; TCDr is spatially-varying TCD related to sinuosity of the tidal channel; TCDor is spatially-varying TCD related to the order and sinuosity of the tidal channel. Detailed information on the spatially-varying TCDs as well as their calculation can be found in Sun et al. (2020).
Results
Simulation Results of Tidal Channel Geomorphology and Hydrodynamics
There was no significant change in geomorphology for each scenario after the three-year simulation period (see Supplementary Figure 1), which indicated that all tidal channel networks remained in equilibrium condition during the simulation. Figure 6 shows the representative hydrodynamics simulation results of H(1) during flood tide on the 241th Julian day (fruiting stage in mid-summer). Clearly, tidal channel geometric features, such as channel sinuosity and channel order, affected tidal currents. During high tides, the concentrated tidal currents in the channel network showed much larger velocity relative to sheet flow on the marsh platform (Figure 6A). Moreover, tidal currents in the main channel were faster than those in lower-order channels (Figures 6B, C). Velocity reduction was also observed at locations where the flow was affected by abrupt channel morphological changes, e.g., where the channel meandered and bifurcated (Figure 6B).
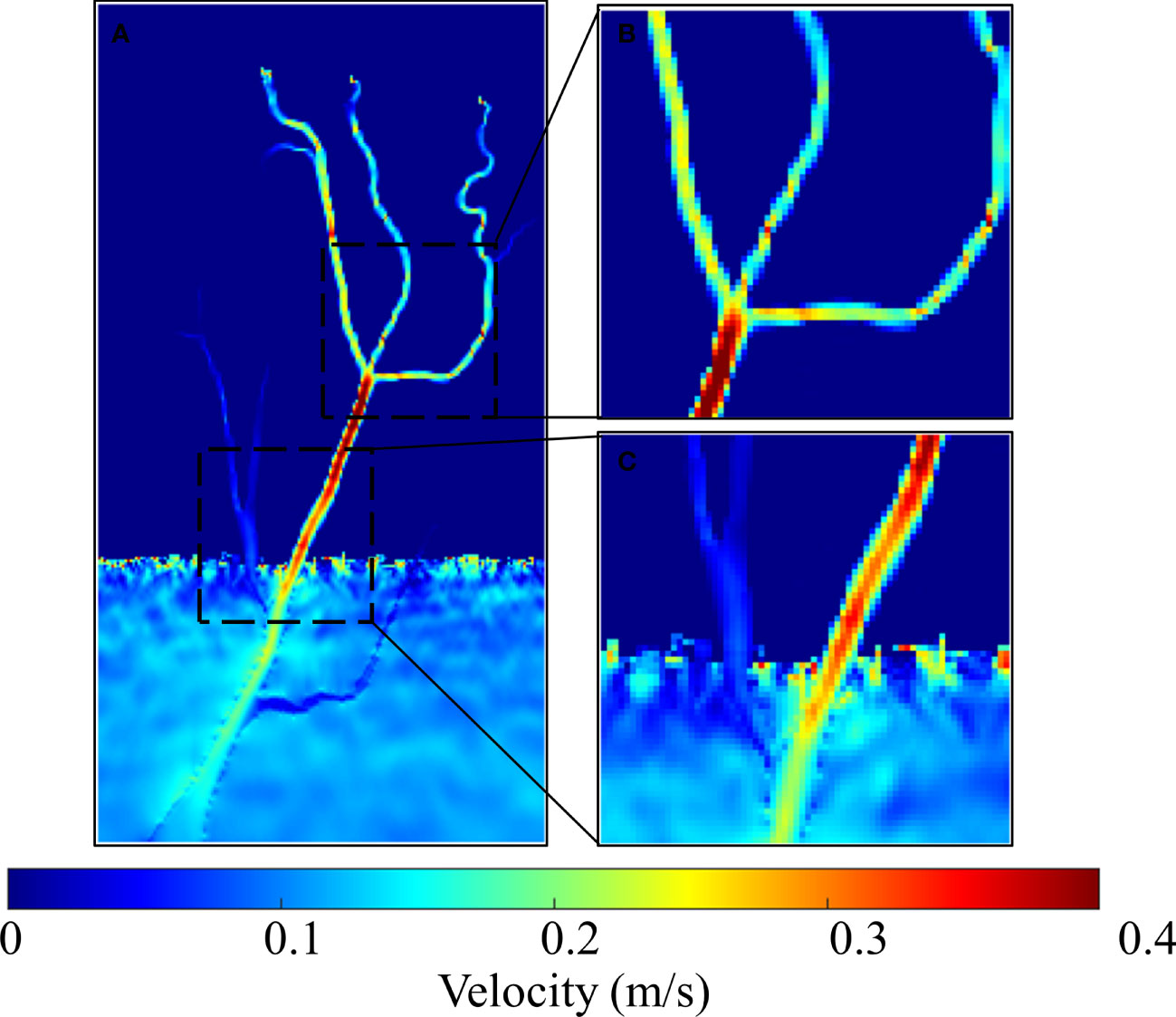
Figure 6 The hydrodynamics simulation results of H(1) during flood tide on the 241th Julian day: (A) the whole tidal channel network; (B, C) subregions of tidal channel network.
Evolution of S. alterniflora Landward Invasion
Figure 7 shows the representative seedbank density simulation results of H(1) on the 60th Julian day in the second and third years. A significant difference in seedbank distribution was found between low and middle marshes. Even though large quantities of seeds were carried by tides and transported into middle marshes during flood tides in the fruiting stage, they were not easily retained on bare flats in middle marshes due to the lack of trapping agents (see Eq. 5). By contrast, seeds were widely found along the tidal channels in middle and high marshes, and their density showed a significant decrease with the lateral distance away from channels. This is because the area near channels has more opportunities to capture the floating seeds. Moreover, some isolated seedbank patches emerged in high marshes (Figures 7B–D). These locations were the upper boundaries of hydrochorous seed dispersal in tidal channels. Compared to low and middle marshes, the flooding duration in high marshes was much shorter, resulting in stranded seeds after the episodic short-lived floods retreated.
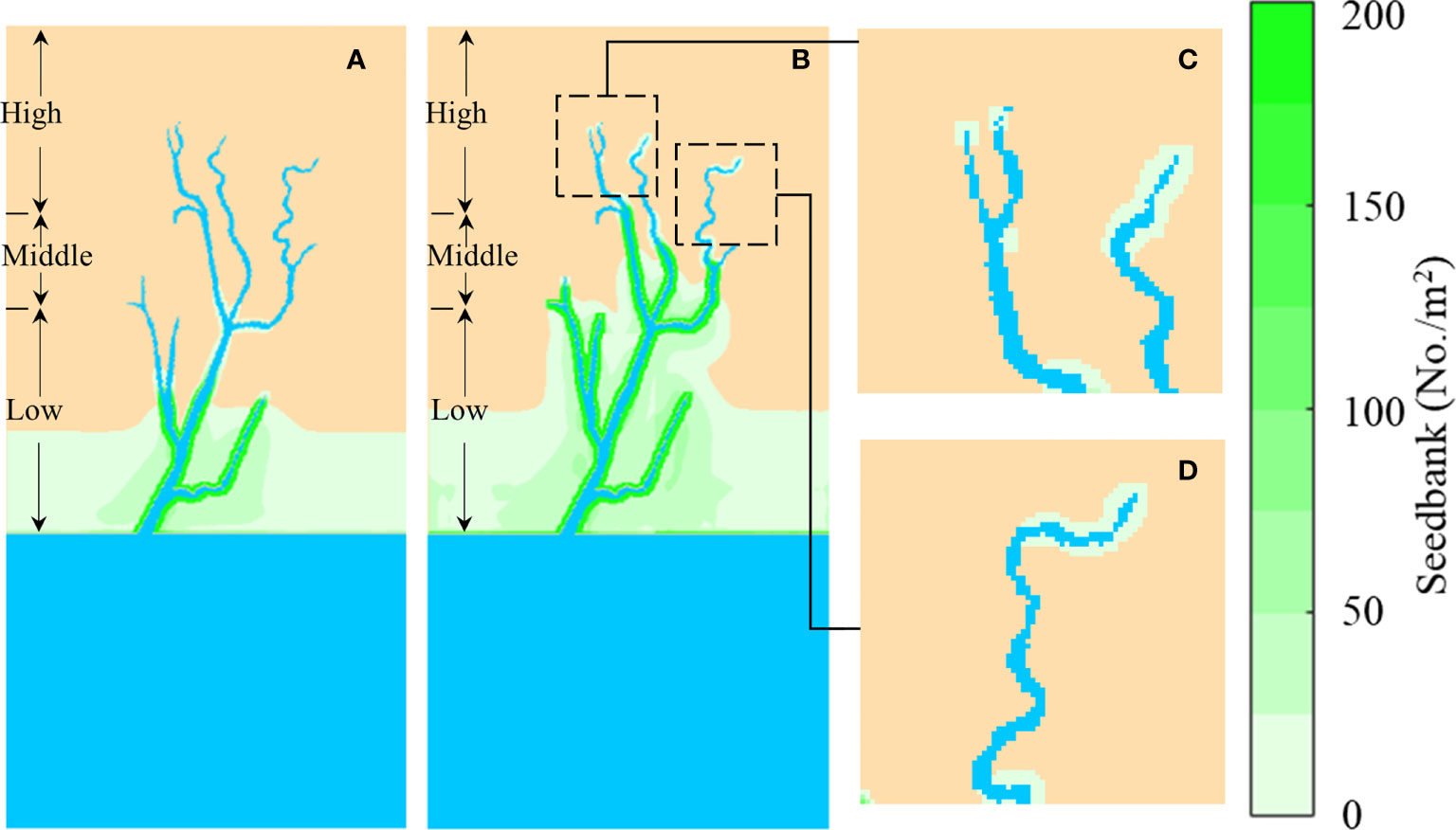
Figure 7 Seedbank density simulation results of H(1) on the 60th Julian day in the second and third year. (A) Second year; (B) Third year; (C) and (D) are seedbank distributions of the third year in high marshes. The blue is tidal channel network and sea; the yellow is bare flats. The marsh platform was divided into low, middle and high marshes based on elevation.
Figure 8 shows the representative evolution of vegetation distribution of H(1) during the three-year simulation. Vegetation landward invasion was strongly restricted by tidal channel geomorphology and salinity stress in the first year (see Figure 8A). The vegetation was unable to cross the tidal channel and invade into the other side in the initial invasion stage because of no hydrochorous seed dispersal in the first year. As expected, the invasion speed along the tidal channels was much faster than that on bare flats (see Figure 8B). This indicated that hydrochorous seed dispersal played a critical role in S. alterniflora landward invasion. Hydrochorous seed dispersal broke the channel barriers, allowing S. alterniflora to cross the tidal channel bifurcation and colonize the other side of the channel. S. alterniflora laterally expanded and gradually colonized the salinity buffer zone after the seedling stage, which contrasted with no vegetation on bare flats in middle and high marshes (Figure 8C). In high marshes in the following third year, no vegetation patch was observed after the seedling stage due to low vegetation density (< 2/3 of maximum capacity). However, the gradually increasing density in the following growing season resulted in isolated S. alterniflora patches, which was consistent with the sporadic seedbank distribution in the third year (Figure 7B).
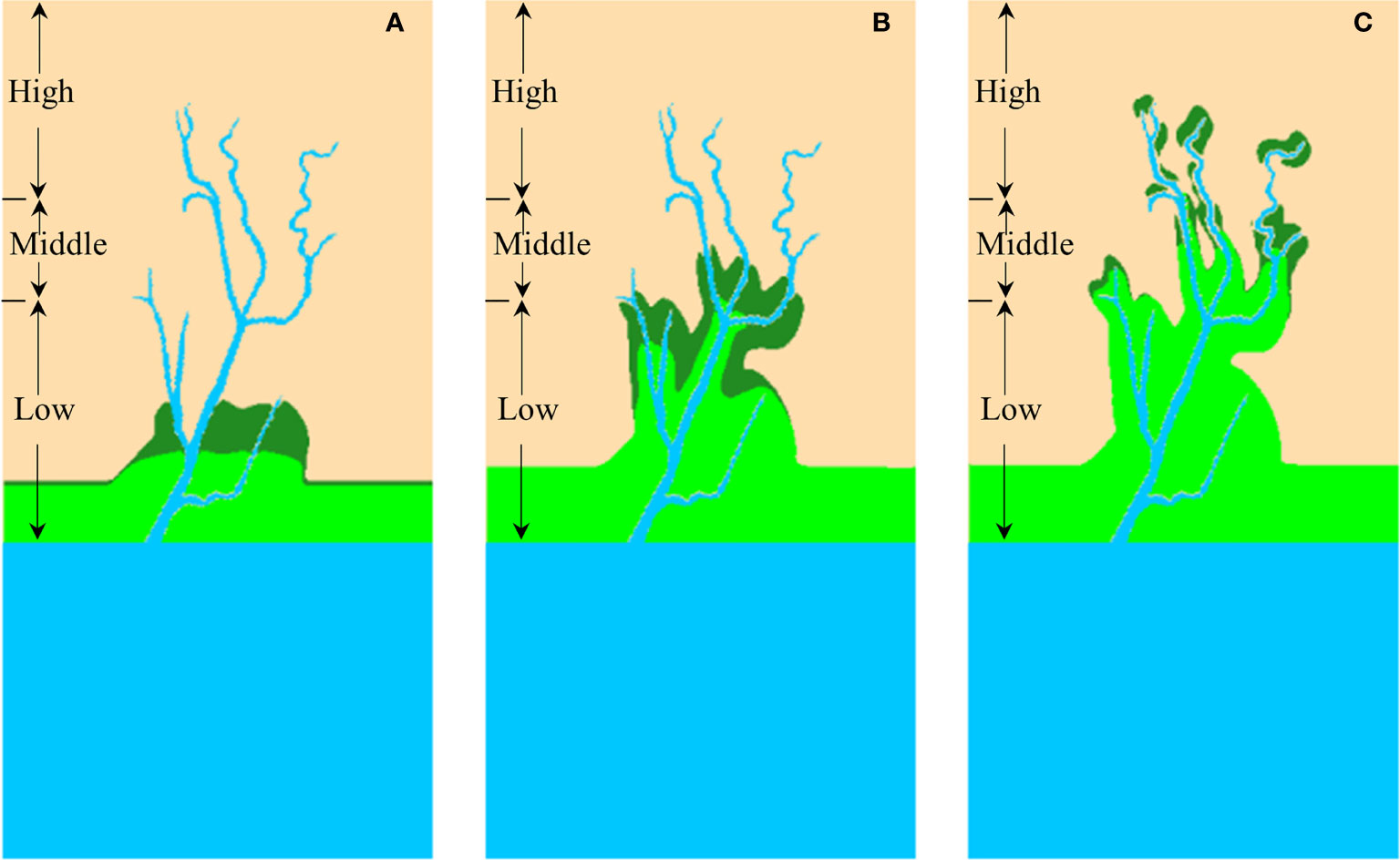
Figure 8 The evolution of S. alterniflora landward invasion over time: (A) First year; (B) Second year; (C) Third year. The blue is tidal channel network and sea; the yellow is bare flats; the green is S. alterniflora distribution at the end of seedling season; the dark green is the added area of S. alterniflora between seedling stage and end of growing season. The marsh platform was divided into low, middle and high marshes based on elevation.
Relationships Between Tidal Channel Functional and Geometric Features and Vegetation Area
We firstly examined the relationships between the vegetation area and functional features of the channel network at the watershed scale, which were indicated by various drainage efficiency metrics. As expected, the vegetated area at the end of the third growing season showed a significant positive correlation with TCD (p<0.01) and GE (p<0.05), while it showed a significant negative correlation with OPL (p<0.05, Table 3). All results indicated that tidal channel network with higher drainage efficiency enhanced S. alterniflora landward invasion. Besides, our results revealed that geometric features of the tidal channel network were correlated with functional features at the watershed scale (Table 3). The geometric mean bifurcation ratio showed significant positive correlations with vegetation area (p<0.01), TCD (p<0.05) and GE (p<0.05), and significant negative correlation with OPL (p<0.05). At the same time, partial correlation analysis results showed that under the similar drainage efficiency, a higher geometric mean bifurcation ratio facilitated S. alterniflora landward invasion (Table 4).
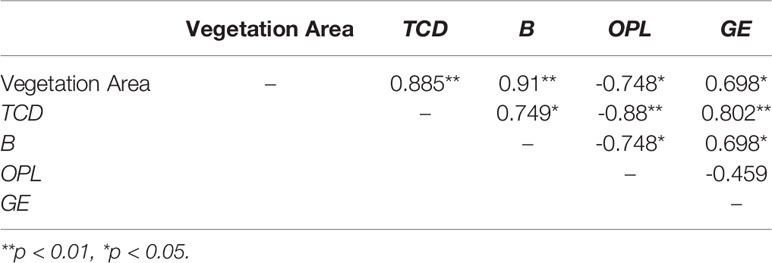
Table 3 The Pearson correlations between vegetation area and tidal channel functional and geometric metrics.
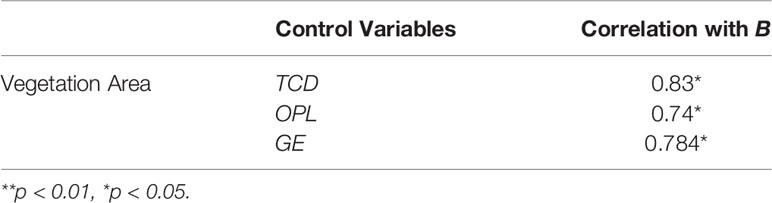
Table 4 Partial correlation analysis relating geometric mean bifurcation ratio and vegetation area to different drainage efficiency metrics.
To assess how the tidal channel network affects S. alterniflora landward invasion at the local scale, we defined three spatially-varying TCDs by incorporating a weighting factor related to the channel order and/or channel sinuosity, and further analyzed their correlations with vegetation area (Table 5). The results showed that there was a consistently significant positive correlation between the proportion of S. alterniflora (see its definition in Sun et al. (2020)) and TCDo(p<0.01), and a positive correlation with TCDr and TCDor also manifested in most cases, which indicated that local channel sinuosity and channel order both affected S. alterniflora landward invasion and the latter appeared to have a greater influence.
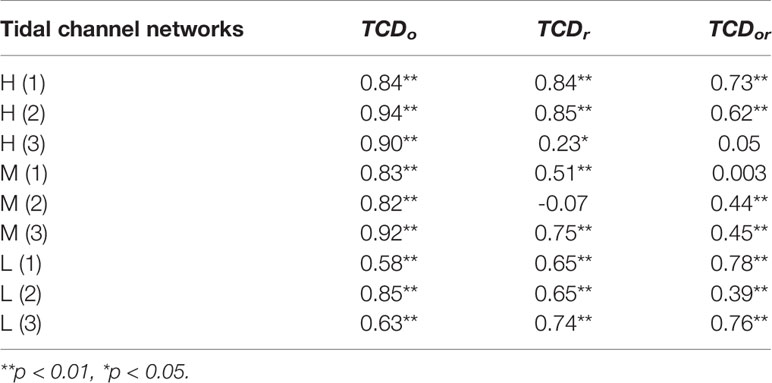
Table 5 The Pearson correlations between proportion of S. alterniflora [see its definition in Sun et al. (2020)] and normalized spatially-varying TCD indices.
Discussion
Effects of Tidal Channel Network on S. alterniflora Hydrochorous Seed Dispersal
The functions of tidal channel networks in the marsh-channel system are shown to control hydrodynamics, sediment and biota transport in and between salt marshes (Kearney and Fagherazzi, 2016; Taramelli et al., 2018). Previous studies have established the use of drainage efficiency and the associated metrics as an effective measure of functional features of tidal channel networks (Marani et al., 2003; Chirol et al., 2018). Patterning relationships between tidal channel networks and S. alterniflora landward invasion have been further reported in the literature (Zheng et al., 2016; Fan et al., 2020; Sun et al., 2020). Our findings suggested that S. alterniflora invasion area had positive correlations with TCD and GE and negative correlations with OPL (Table 3), which are in line with the relevant previous findings.
Generally speaking, the abundance and distribution of vegetation in salt marsh ecosystems are strongly influenced by the connectivity of seed dispersal pathways and tidal floods (Elsey-Quirk and Leck, 2015). A number of studies also indicated that the spatial patterns of propagule arrival were important for the success of plant invasion in salt marshes, and used propagule pressure to reflect the number of invasive individuals released into new areas and the probability of successful invasion (Johnston et al., 2009; Simberloff, 2009; Balestri et al., 2018). In this study, watersheds with higher drainage efficiency (larger TCD/GE, smaller OPL) provided more pathways for seeds to travel into the marsh interior and redistributed the seedbank along channels (Neff and Baldwin, 2005; Stark et al., 2017), which completely changed the initial seed distribution that was roughly correlated with adult plant abundance across the marshes (Rand, 2000; Ning et al., 2020). When the tides redistributed the seeds during tidal cycles, the areas closer to tidal channels had higher chances to trap more seeds than bare flats further away from channels (Figure 7) and thus developed a higher propagule pressure. This finding is in line with the field observation in the YRD that propagule pressure decreased with lateral distance from channel margins (Ning et al., 2021b). Tidal channel network with higher drainage efficiency exhibits higher capacity in seed redistribution and has more abundance of seeds along channel margins, resulting in relatively high propagule pressure and high probability of S. alterniflora landward invasion.
Our results showed that seeds distributed in a patchy pattern and tended to concentrate in the bifurcation area in high marshes (Figure 7). Given similar drainage efficiency, the additional nuance of geometric features, i.e. geometric mean bifurcation ratio, also led to differences in vegetation area (Table 4). Both results indicated the significant influences of bifurcation on vegetation distribution. Hydrodynamic simulation results suggest that during the flood tides, tidal current velocity tended to be lower in low-order channels compared to high-order channels (Figure 6). Previous studies also revealed that complex tidal currents usually occurred in the bifurcation area, and the flow split into the bifurcating channels, leading to a relatively small velocity (Fischer et al., 2000; Stark et al., 2017). Reduced flow velocity due to bifurcation may increase the probability of seed deposition, thereby increasing the propagule pressure of plant invasion. Similar findings that seed deposition and colonization events are more likely to appear in the bifurcation area have been reported by Fischer et al. (2000).
Furthermore, channel geometric features including channel order and sinuosity were found to influence vegetation area at the local scale (Table 5). As reported by Sanderson et al. (2000), vegetation abundance exhibited a significant linear increase with channel size, which was approximately correlated with channel order. This is consistent with the finding of positive correlations between S. alterniflora area and channel order among all drainage efficiency scenarios. However, varying correlations between vegetation area and channel sinuosity were found in our results (Table 5), which indicated that channel order dominated channel sinuosity in influencing vegetation area at the local scale. The varying correlations could be due to the locally varying channel sinuosity throughout the channel segment. At the local scale, Taramelli et al. (2018) revealed the negative relationship between channel sinuosity and patch size. Small vegetation patches were consistently found along channels with higher sinuosity (>1.5), while larger patches were associated with almost straight channels. This local effect was captured in our study as well (see Figure 8C). Seeds carried by tides tended to deposit in meandering areas, leading to small isolated vegetation patches, whereas contiguous patches were mostly found along with straight channel segments.
Moreover, our simulation results captured the correlations between geometric features, i.e. geometric mean bifurcation ratio, and functional features at the watershed scale (Table 3). This finding is also supported by a recent finding that channel bifurcation ratio had a negative correlation with OPL in the YRD (Mou et al., 2021). Besides, another conceptual analysis performed by Marani et al. (2003) showed that for a given total channel length per unit area, a channel network with bifurcation resulted in a large geometric efficiency than that of single-channel, which revealed an optimal spatial arrangement of bifurcations could efficiently reduce the OPL while keeping the TCD invariant.
Effects of Tidal Channel Network on S. alterniflora Colonization
In addition to the seed dispersal process, adaptability and tolerance to a range of abiotic stressors are important determinants of vegetation colonization and expansion in new environments (Balestri et al., 2018). Our results showed that S. alterniflora grew along tidal channels, in contrast to no vegetation on bare flats away from channels (Figure 8). This can be attributed to spatial salinity heterogeneity resulting from the tidal channel network. In low marshes, salinity is relatively low and there is little spatial salinity heterogeneity, leading to large contiguous vegetation patches (Pennings et al., 2005; Ning et al., 2021b). Our simulated vegetation distributions are in line with such a pattern, i.e., S. alterniflora usually colonizes in low marshes first and forms large patches (Li et al., 2009; Qi et al., 2017).
Previous studies reported that salinity stress usually increased along the elevation gradient and restrained the growth of S. alterniflora (Pennings et al., 2005; Qi et al., 2017). However, this salinity stress on vegetation was not observed near tidal channels in middle and high marshes (see Figure 8). Frequent inundation near tidal channels lowers soil salinity along channel banks, creating a salinity buffer zone and promoting vegetation colonization (Kim et al., 2013a; Xin et al., 2013; Kearney and Fagherazzi, 2016). Therefore, the lateral distance away from tidal channels is an important factor that affects salt-marsh plant distribution and growth (Sanderson et al., 2000; Sun et al., 2020; Wu et al., 2020; Ning et al., 2021b). Furthermore, this facilitating effect gradually decreased with increasing elevation because of reduced inundation, which was manifested by the narrowing width of vegetation patches in high marshes (see Figure 8). Such decreasing facilitating effect of tidal channels on vegetation growth along elevation gradient was reported in the YRD as well (Ning et al., 2021b).
The hydrodynamic disturbance is another important abiotic factor affecting the establishment and survival of salt marsh vegetation. Our results showed that flow velocity in high-order channels was faster than that in low-order channels (Figures 6B, C). This indicated that vegetation growing around high-order channels suffered greater hydrodynamic disturbance. This simulated hydrodynamic disturbance pattern is in line with field observation in the YRD (Ning et al., 2020). However, the flow velocity in both high- and low-order channels was below the critical disturbance threshold during the majority of the simulation periods. Therefore, the loss of vegetation was rapidly compensated by new seedlings and clonal reproduction in disturbance-free periods. The sufficiently long disturbance-free periods during the growing season are essential for successful vegetation colonization in the salinity buffer zone.
Verification With Real Cases
In this section, we firstly verified our results with previous studies on the specific patterns of S. alterniflora landward invasion in relation to tidal channel networks (Hou et al., 2014; Zheng et al., 2016; Fan et al., 2020; Sun et al., 2020). Previous studies indicated that at the watershed scale, tidal channel networks with higher drainage efficiency (larger TCD, smaller OPL and UPL) attained larger S. alterniflora area (Fan et al., 2020; Sun et al., 2020), which are consistent with our findings (Table 3). Besides, S. alterniflora exhibited a more concentrated spreading pattern along channel margins at the local scale (Hou et al., 2014; Fan et al., 2020; Sun et al., 2020), and high-order channels were found to play a dominant role in S. alterniflora landward invasion. Most vegetation grew in buffer zones along the tidal channels, and the number of patches decreased with lateral distance as expansion stabilized (Zheng et al., 2016; Fan et al., 2020; Sun et al., 2020). Similar vegetation patterns were observed in our simulation results (Table 5 and Figure 8). S. alterniflora established and grew near channels, in contrast to no vegetation further away from channels in middle and high marshes due to salinity stress.
The specific S. alterniflora landward invasion processes were further verified with two exemplary cases in the north and south banks of the YRD, respectively (Figure 9). S. alterniflora gradually invaded through tidal channels from low marshes to high marshes. Clonally spreading played the dominant role in S. alterniflora distribution in the first year, resulting in a large contiguous patch (Figure 8A). This pattern can be found in the field where large patches formed in low marshes, whereas few isolated patches emerged in middle and high marshes (Figures 9B, D). Presumably, some seeds of the mature plants ended up in the tidal channel and were dispersed along the channel, which set the stage for the subsequent invasion in the following year (see Figures 9C, E). The isolated vegetation patches in the middle and high marshes resulted from hydrochorous seed dispersal gradually connected with each other and merged into a larger patch through clonal expansion in the salinity buffer zone, which contrasted with the limited vegetation expansion on the bare flats (Figures 9C, E). These landscape evolution dynamics were captured by our model simulations as well (Figures 8B, C).
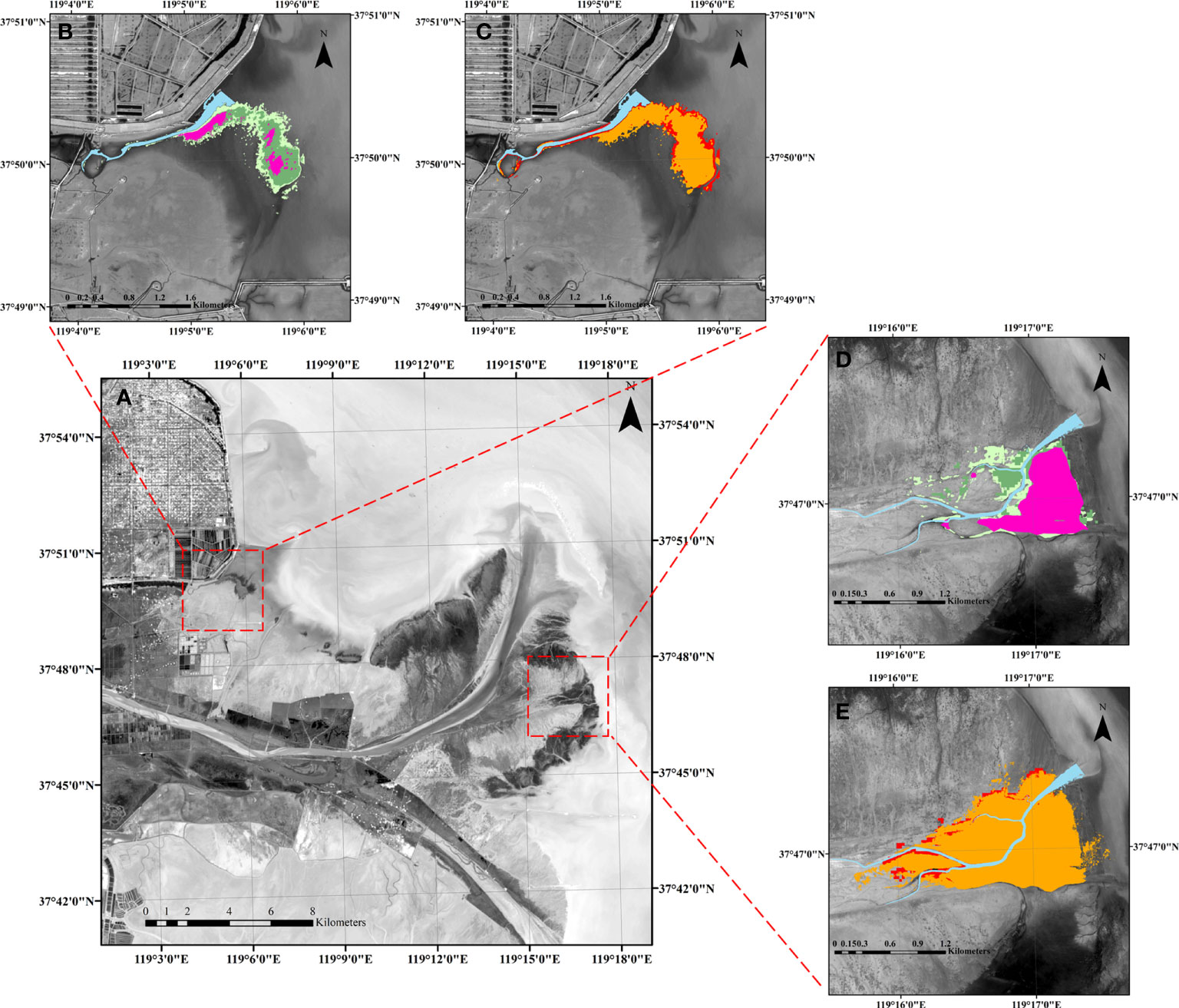
Figure 9 Exemplary cases of S. alterniflora landward invasion in the YRD. (A) Regional map of the YRD. (B, C) Are spatio-temporal dynamics of S. alterniflora landward invasion in the northern bank of the YRD. (D, E) Are the spatio-temporal dynamics of S. alterniflora landward invasion in the southern bank of the YRD. The purple, light green, green, orange and red are the vegetation distribution at the end of the first growing season, second seedling stage, second growing season, third seedling stage, and third growing season, respectively.
Metric Framework of S. alterniflora Landward Invasion Along Tidal Channel Networks
In this study, we hypothesized that tidal channel networks with higher drainage efficiency obtained a larger vegetation area. Our results showed that S. alterniflora invasion was strongly associated with the functional features of tidal channel networks on the watershed scale, which proved the hypothesis. Specifically, watersheds with higher drainage efficiency (larger TCD and GE, smaller OPL) attained larger S. alterniflora area (Table 3).
Among all metrics of watershed-scale functional features, TCD only contains the basic length information of tidal channel networks and requires a minimum amount of computation at the expense of excluding geometric features including channel sinuosity and bifurcation. OPL is dependent on the spatial configuration of the channel network. Therefore, it reveals how the tidal channel network with varying spatial patterns serves the marsh platform as a whole (Marani et al., 2003). However, the calculation of OPL involves the distance of all grids to the nearest channel throughout the entire watershed and thus is much more computationally expensive than TCD. Based on TCD and OPL, GE metric further provides a quantitative evaluation on how well the channel network is spatially arranged for a given channel length (Kearney and Fagherazzi, 2016). Despite their varying complexity, our results showed that TCD, OPL and GE were strongly correlated with each other, and all three metrics showed similar results regarding the effects of network functional features on vegetation area (Table 3). Further considering their respective computing costs, we recommend using TCD as the metric of watershed-scale functional features (drainage efficiency).
Furthermore, we proved the secondary hypothesis that given a similar drainage efficiency, the additional nuance of geometric features also makes a difference, i.e. tidal channel network with increasing geometric mean bifurcation ratio enhances S. alterniflora invasion (Table 4). This result indicates that it is essential to take the geometric mean bifurcation ratio into consideration when assessing the effects of the tidal channel network on vegetation expansion. At the same time, the geometric mean bifurcation ratio also fills the gap of TCD in missing information related to channel spatial configuration.
Our results also indicated that compared to channel sinuosity, channel order was more critical for vegetation area at the local scale (Table 5). As one of the local-scale functional feature metrics, spatially-varying TCDo incorporates channel order, the basic geometric feature for a single channel, as well as channel length information within a specified local area. Hence, it provides more precise drainage efficiency information at the local scale and can be used to assess the potential hot spots where the vegetation is likely to colonize. However, the calculation of spatially-varying TCDo requires the most complex procedures and thus the highest computational cost among all metrics tested in this study. Therefore, we recommend applying this metric when local or precise drainage efficiency information is demanded.
In summary, we recommend using watershed-scale TCD, geometric mean bifurcation ratio and spatially-varying TCDo as a comprehensive metric group of a functional and geometric feature of tidal channel network across different spatial scales. Based on the recommended metrics, we proposed a general framework for assessing how tidal channel network affects vegetation expansion and distribution from local to watershed scale in the marsh-channel system and further used S. alterniflora landward invasion as an example (Figure 10). On the watershed scale, a tidal channel network with larger TCD indicates a higher drainage efficiency and obtains a larger S. alterniflora area (Figures 10A vs B). Given a similar drainage efficiency, the additional geometric nuances, i.e. geometric mean bifurcation ratio, also make a difference (Figure 10B vs C). Tidal channel network with increasing geometric mean bifurcation ratio indicates a better spatial configuration of channel network system and thus enhances S. alterniflora invasion. Based on these two metrics, we could assess which tidal channel networks are under higher propagule pressure and are more prone to S. alterniflora landward invasion. At the local scale, channel order affects the relevant ecogeomorphological processes, and thus influences vegetation establishment and growth near channel margins (Figure 10C) (Sanderson et al., 2001). A larger spatially-varying TCDo value is associated with a larger vegetation area, as it represents a larger local drainage efficiency. The spatially-varying TCDo can be used to identify the potential hot spots for S. alterniflora colonization at the local scale and assist in designing invasion control measures. Our proposed metric framework could also be used to assess the effects of tidal channel networks on a salt marsh plant expansion in general.
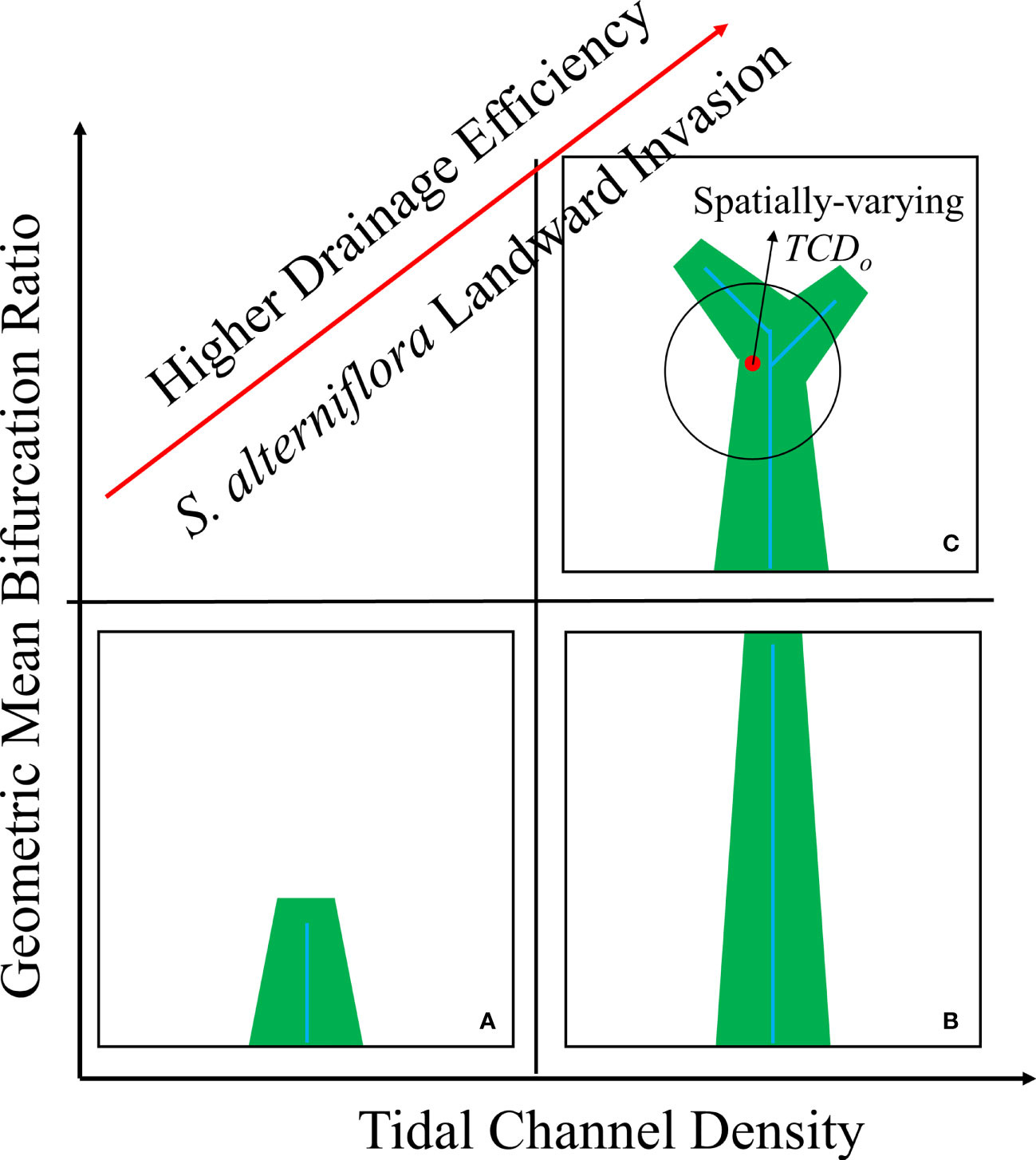
Figure 10 Metric framework on how tidal channel networks mediate S. alterniflora landward invasion in the marsh-channel system. (A) Low tidal channel density; (B) High tidal channel density with low geometric mean bifurcation ratio; (C) High tidal channel density with high geometric mean bifurcation ratio. Blue colour shows the tidal channel network, while the green colour is the vegetation distribution.
Conclusion
In this study, tidal channel networks with varying drainage efficiency were extracted from remote sensing images of the Yellow River Delta, China as an exemplary active S. alterniflora invasion sites and further used in schematized numerical experiments to test the hypothesis that tidal network works with higher drainage efficiency obtain a larger invasion area. Tidal channel networks with similar drainage efficiency and yet varying geometric mean bifurcation ratios were further used to test a secondary hypothesis that given the same drainage efficiency, geometric features further affect S. alterniflora invasion. Furthermore, the statistical relationships between a comprehensive set of metrics characteristic of functional and geometric features of tidal channel network and vegetation area were further analysed.
Our results show that: 1) At the watershed scale, the effects of tidal channel network on S. alterniflora landward invasion are revealed by various drainage efficiency metrics. S. alterniflora area has a positive correlation with TCD and GE and a negative correlation with OPL. 2) Geometric features, such as geometric mean bifurcation ratio, are related to the functional features of the channel network and further affect vegetation area. Under similar drainage efficiency, a larger geometric mean bifurcation ratio further enhances S. alterniflora invasion. 3) At the local scale, geometric features including channel order and sinuosity, could affect local drainage efficiency and further mediate S. alterniflora invasion. In viewing their efficacy and computational cost, we recommend using global metrics including the watershed-scale TCD, geometric mean bifurcation ratio and local metric including the spatially-varying TCDoas a comprehensive metric group of the functional and geometric feature of tidal channel network in marsh channel system across different spatial scales. Our proposed framework can be applied to assess the effects of tidal channel network on a salt marsh plant expansion in general, and S. alterniflora invasion in particular.
When tidal channel geomorphology is in equilibrium condition as assumed in this study, vegetation feedback on tidal channel evolution is to exert a stabilizing effect and can be neglected (Schwarz et al., 2014; Taramelli et al., 2018; Liu et al., 2021; Wang et al., 2021). Using a more sophisticated ecogeomorphological model that incorporates the interactions among vegetation, hydro- and morpho-dynamics in a two-way fully coupled fashion to explore the coevolution of tidal channel networks with vegetation expansion in the marsh-channel system is recommended for future studies.
Data Availability Statement
The original contributions presented in the study are included in the article/Supplementary Material. Further inquiries can be directed to the corresponding author.
Author Contributions
Conceptualization, SZ, DS, and TS. Methodology, SZ and WG. Validation, DS and TS. Investigation, SZ, ZN, and ZL. Data Curation, ZS. Writing - original draft preparation, SZ. Writing - review & editing, DS, TS, BC, WN, ZN, and ZL. Supervision, DS and TS. Funding acquisition, DS. All authors contributed to the article and approved the submitted version.
Funding
This work was supported by the National Key R&D Program of China (grant 2019YFE0121500), the Joint Funds of the National Natural Science Foundation of China (grant U1806217), Key Project of the National Natural Science Foundation of China (grant 51639001), and the Interdisciplinary Research Funds of Beijing Normal University. WG acknowledges support from the Young Scientists Fund of National Natural Science Foundation of China (grant 52101297) and the Fellowship of China Post-doctoral Science Foundation (grant 2020M680438).
Conflict of Interest
The authors declare that the research was conducted in the absence of any commercial or financial relationships that could be construed as a potential conflict of interest.
Publisher’s Note
All claims expressed in this article are solely those of the authors and do not necessarily represent those of their affiliated organizations, or those of the publisher, the editors and the reviewers. Any product that may be evaluated in this article, or claim that may be made by its manufacturer, is not guaranteed or endorsed by the publisher.
Acknowledgments
We gratefully acknowledge Miss Sun Limin for her valuable assistance in remote sensing interpretation. We are very thankful for the dedicated and patient guidance from Matthew P. Adams and Kate O’Brien on vegetation dynamic model and hydrochorous seed dispersal module.
Supplementary Material
The Supplementary Material for this article can be found online at: https://www.frontiersin.org/articles/10.3389/fmars.2022.888597/full#supplementary-material
References
An S. Q., Gu B. H., Zhou C. F., Wang Z. S., Deng Z. F., Zhi Y. B., et al. (2007). Spartina Invasion in China: Implications for Invasive Species Management and Future Research. Weed. Res. 47, 183–191. doi: 10.1111/j.1365-3180.2007.00559.x
Balestri E., Vallerini F., Menicagli V., Barnaba S., Lardicci C. (2018). Biotic Resistance and Vegetative Propagule Pressure Co-Regulate the Invasion Success of a Marine Clonal Macrophyte. Sci. Rep. 8, 16621. doi: 10.1038/s41598-018-35015-0
Barbier E. B., Hacker S. D., Kennedy C., Koch E. W., Stier A. C., Silliman B. R. (2011). The Value of Estuarine and Coastal Ecosystem Services. Ecol. Monogr. 81, 169–193. doi: 10.1890/10-1510.1
Belliard J. P., Toffolon M., Carniello L., D’alpaos A. (2015). An Ecogeomorphic Model of Tidal Channel Initiation and Elaboration in Progressive Marsh Accretional Contexts. J. Geophys. Res.-Earth. Surf. 120, 1040–1064. doi: 10.1002/2015jf003445
Best Ü.S.N., Van Der Wegen M., Dijkstra J., Willemsen P. W. J. M., Borsje B. W., Roelvink D. J. A. (2018). Do Salt Marshes Survive Sea Level Rise? Modelling Wave Action, Morphodynamics and Vegetation Dynamics. Environ. Modell. Software 109, 152–166. doi: 10.1016/j.envsoft.2018.08.004
Brückner M. Z. M., Schwarz C., Dijk W. M., Oorschot M., Douma H., Kleinhans M. G (2019). Salt Marsh Establishment and Eco-Engineering Effects in Dynamic Estuaries Determined by Species Growth and Mortality. J. Geophys. Res.-Earth. Surf. 124, 2962–2986. doi: 10.1029/2019jf005092
Carroll J. D., Green P. E. (1997). Mathematical Tools for Applied Multivariate Analysis (Washington, DC: Academic Press), 259–294. doi: 10.1016/B978-012160954-2/50007-X
Chang E. R., Veeneklaas R. M., Bakker J. P. (2007). Seed Dynamics Linked to Variability in Movement of Tidal Water. J. Veg. Sci. 18, 253–262.
Chang E. R., Veeneklaas R. M., Buitenwerf R., Bakker J. P., Bouma T. J. (2008). To Move or Not to Move: Determinants of Seed Retention in a Tidal Marsh. Funct. Ecol. 22, 720–727. doi: 10.1111/j.1365-2435.2008.01434.x
Chirol C., Haigh I. D., Pontee N., Thompson C. E., Gallop S. L. (2018). Parametrizing Tidal Creek Morphology in Mature Saltmarshes Using Semi-Automated Extraction From Lidar. Remote Sens. Environ. 209, 291–311. doi: 10.1016/j.rse.2017.11.012
Crawford M., Davies S., Griffith A. (2015). Predicting Metapopulation Responses of a Tidal Wetland Annual to Environmental Stochasticity and Water Dispersal Through an Individual-Based Model. Ecol. Model. 316, 217–229. doi: 10.1016/j.ecolmodel.2015.08.019
Cunnings A., Johnson E., Martin Y. (2016). Fluvial Seed Dispersal of Riparian Trees: Transport and Depositional Processes. Earth Surf. Proc. Landf. 41, 615–625. doi: 10.1002/esp.3850
Elsey-Quirk T., Leck M. A. (2015). Patterns of Seed Bank and Vegetation Diversity Along a Tidal Freshwater River. Am. J. Bot. 102, 1996–2012. doi: 10.3732/ajb.1500314
Elsey-Quirk T., Leck M. A. (2021). High Reinvasion Potential of Phragmites Australis in a Delaware River (USA) Tidal Freshwater Marsh Following Chemical Treatment: The Role of the Seedbank. Wetlands 41. doi: 10.1007/s13157-021-01398-6
Fan Y., Zhou D., Ke Y., Wang Y., Wang Q., Zhang L. (2020). Quantifying the Correlated Spatial Distributions Between Tidal Creeks and Coastal Wetland Vegetation in the Yellow River Estuary. Wetlands 40, 2701–2711. doi: 10.1007/s13157-020-01292-7
Finotello A., Canestrelli A., Carniello L., Ghinassi M., D’alpaos A. (2019). Tidal Flow Asymmetry and Discharge of Lateral Tributaries Drive the Evolution of a Microtidal Meander in the Venice Lagoon (Italy). J. Geophys. Res.-Earth. Surf. 124, 3043–3066. doi: 10.1029/2019JF005193
Fischer J. M., Reed-Andersen T., Klug J. L., Chalmers A. G. (2000). Spatial Pattern of Localized Disturbance Along a Southeastern Salt Marsh Tidal Creek. Estuaries 23, 565–571. doi: 10.2307/1353146
Ge Z. M., Cao H. B., Zhang L. Q. (2013). A Process-Based Grid Model for the Simulation of Range Expansion of Spartina Alterniflora on the Coastal Saltmarshes in the Yangtze Estuary. Ecol. Eng. 58, 105–112. doi: 10.1016/j.ecoleng.2013.06.024
Ge Z. M., Zhang L. Q., Yuan L. (2015). Spatiotemporal Dynamics of Salt Marsh Vegetation Regulated by Plant Invasion and Abiotic Processes in the Yangtze Estuary: Observations With a Modeling Approach. Estuar. Coast. 38, 310–324. doi: 10.1007/s12237-014-9804-7
Griffith A. B., Forseth I. N. (2002). Primary and Secondary Seed Dispersal of a Rare, Tidal Wetland Annual, Aeschynomene Virginica. Wetlands 22, 696–704.
Hopfensperger K. N., Engelhardt K., Lookingbill T. R. (2009). Vegetation and Seed Bank Dynamics in a Tidal Freshwater Marsh. J. Veg. Sci. 20, 767–778. doi: 10.1111/j.1654-1103.2009.01083.x
Hou M., Liu H., Zhang H. (2014). Effection of Tidal Creek System on the Expansion of the Invasive Spartina in the Coastal Wetland of Yancheng. Acta Ecol. Sin. 34, 400–409.
Hughes Z. J. (2012). “Tidal Channels on Tidal Flats and Marshes,” in Principles of Tidal Sedimentology. Eds. Davis R. A. Jr., Dalrymple R. W. (Dordrecht.: Springer), 269–300. doi: 10.1007/978-94-007-0123-6_11
Huiskes A. H. L., Koutstaal B. P., Herman P. M. J., Beeftink W. G., Markusse M. M., Munck W. D. (1995). Seed Dispersal of Halophytes in Tidal Salt Marshes. J. Ecol. 83, 559–567. doi: 10.2307/2261624
Ichoku C., Chorowicz J. (1994). A Numerical Approach to the Analysis and Classification of Channel Network Patterns. Water Resour. Res. 30, 161–174. doi: 10.1029/93WR02279
Jenks G. F. (1967). The Data Model Concept in Statistical Mapping. Int. Yearbook Cartogr. 7, 186–190.
Johnston E. L., Piola R. F., Clark G. F. (2009). “The Role of Propagule Pressure in Invasion Success,” in Biological Invasions in Marine Ecosystems. Ecological Studies (Analysis and Synthesis), vol. 204. Eds. Rilov G., Crooks J. A. (Berlin, Heidelberg: Springer). doi: 10.1007/978-3-540-79236-9_7
Kaspar S. (2019). Testing Bifurcation Stability for Both River and Tidal Systems in a Physical Model. [Utrecht]:Utrecht. Univ. 6.
Kearney W. S., Fagherazzi S. (2016). Salt Marsh Vegetation Promotes Efficient Tidal Channel Networks. Nat. Commun. 7, 12287. doi: 10.1038/ncomms12287
Kim D., Cairns D. M., Bartholdy J. (2013a). Environmental Controls on Multiscale Spatial Patterns of Salt Marsh Vegetation. Phys. Geogr. 31, 58–78. doi: 10.2747/0272-3646.31.1.58
Kim D., Cairns D. M., Bartholdy J. (2013b). Tidal Creek Morphology and Sediment Type Influence Spatial Trends in Salt Marsh Vegetation. Prof. Geogr. 65, 544–560. doi: 10.1080/00330124.2013.820617
Lathrop R. G., Windham L., Montesano P. (2003). DoesPhragmites Expansion Alter the Structure and Function of Marsh Landscapes? Patterns and Processes Revisited. Estuaries 26, 423–435. doi: 10.1007/BF02823719
Leck M. A. (2003). Seed-Bank and Vegetation Development in a Created Tidal Freshwater Wetland on the Delaware River, Trenton, New Jersey, Usa. Wetlands 23, 310–343. doi: 10.1672/9-20
Lesser G. R., Roelvink J. A., Van Kester J., Stelling G. S. (2004). Development and Validation of a Three-Dimensional Morphological Model. Coast. Eng. 51, 883–915. doi: 10.1016/j.coastaleng.2004.07.014
Li X., Leonardi N., Plater A. J. (2019). A Stochastic Approach to Modeling Tidal Creek Evolution: Exploring Environmental Influences on CreekTopologies Through Ensemble Predictions. Geophys. Res. Lett. 46, 13836–13844. doi: 10.1029/2019gl085214
Li B., Liao C. H., Zhang X. D., Chen H. L., Wang Q., Chen Z. Y., et al. (2009). Spartina Alterniflora Invasions in the Yangtze River Estuary, China: An Overview of Current Status and Ecosystem Effects. Ecol. Eng. 35, 511–520. doi: 10.1016/j.ecoleng.2008.05.013
Liu Z., Fagherazzi S., She X., Ma X., Xie C., Cui B. (2020). Efficient Tidal Channel Networks Alleviate the Drought-Induced Die-Off of Salt Marshes: Implications for Coastal Restoration and Management. Sci. Tot. Environ. 749, 141493. doi: 10.1016/j.scitotenv.2020.141493
Liu Z., Gourgue O., Fagherazzi S. (2021). Biotic and Abiotic Factors Control the Geomorphic Characteristics of Channel Networks in Salt Marshes. Limnol. Oceanogr 67, 89–101. doi: 10.1002/lno.11977
Liu H. Y., Lin Z. S., Qi X. Z., Zhang M. Y., Yang H. (2014). The Relative Importance of Sexual and Asexual Reproduction in the Spread of Spartina Alterniflora Using a Spatially Explicit Individual-Based Model. Ecol. Res. 29, 905–915. doi: 10.1007/s11284-014-1181-y
Marani M., Belluco E., D’alpaos A., Defina A., Lanzoni S., Rinaldo A. (2003). On the Drainage Density of Tidal Networks. Water Resour. Res. 39. doi: 10.1029/2001WR001051
McFeeters S. K. (1996). The Use of the Normalized Difference Water Index (NDWI) in the Delineation of Open Water Features. Int. J. Remote Sens. 17, 1425–1432. doi: 10.1080/01431169608948714
Mou K., Gong Z., Qiu H. (2021). Spatiotemporal Differentiation and Development Process of Tidal Creek Network Morphological Characteristics in the Yellow River Delta. J. Geogr. Sci. 31, 1633–1654. doi: 10.1007/s11442-021-1915-z
Neff K. P., Baldwin A. H. (2005). Seed Dispersal Into Wetlands: Techniques and Results for a Restored Tidal Freshwater Marsh. Wetlands 25, 392–404. doi: 10.1672/14
Ning Z., Chen C., Xie T., Wang Q., Bai J., Shao D., et al. (2020). Windows of Opportunity for Smooth Cordgrass Landward Invasion to Tidal Channel Margins: The Importance of Hydrodynamic Disturbance to Seedling Establishment. J. Environ. Manag. 266, 110559. doi: 10.1016/j.jenvman.2020.110559
Ning Z., Chen C., Xie T., Zhu Z., Wang Q., Cui B., et al. (2021a). Can the Native Faunal Communities be Restored From Removal of Invasive Plants in Coastal Ecosystems? A Global Meta-Analysis. Glob. Chang. Biol. 27, 4644–4656. doi: 10.1111/gcb.15765
Ning Z., Chen C., Zhu Z., Xie T., Wang Q., Cui B., et al. (2021b). Tidal Channel-Mediated Gradients Facilitate Spartina Alterniflora Invasion in Coastal Ecosystems: Implications for Invasive Species Management. Mar. Ecol.-Prog. Ser. 659, 59–73. doi: 10.3354/meps13560
Ning Z. H., Xie T., Liu Z. Z., Bai J. H., Cui B. S. (2019). Native Herbivores Enhance the Resistance of an Anthropogenically Disturbed Salt Marsh to Spartina Alterniflora Invasion. Ecosphere 10, 1–13. doi: 10.1002/ecs2.2565
Pennings S. C., Grant M.-B., Bertness M. D. (2005). Plant Zonation in Low-Latitude Salt Marshes: Disentangling the Roles of Flooding, Salinity and Competition. J. Ecol. 93, 159–167. doi: 10.1111/j.1365-2745.2004.00959.x
Qi M., Sun T., Zhang H. Y., Zhu M. S., Yang W., Shao D. D., et al. (2017). Maintenance of Salt Barrens Inhibited Landward Invasion of Spartina Species in Salt Marshes. Ecosphere 8, 1–11. doi: 10.1002/ecs2.2565
Rand T. A. (2000). Seed Dispersal, Habitat Suitability and the Distribution of Halophytes Across a Salt Marsh Tidal Gradient. J. Ecol. 88, 608–621. doi: 10.1046/j.1365-2745.2000.00484.x
Ruiz-Montoya L., Lowe R. J., Kendrick G. A. (2015). Contemporary Connectivity Is Sustained by Wind-and Current-Driven Seed Dispersal Among Seagrass Meadows. Mov. Ecol. 3. doi: 10.1186/s40462-015-0034-9
Sanderson E. W., Foin T. C., Ustin S. L. (2001). A Simple Empirical Model of Salt Marsh Plant Spatial Distributions With Respect to a Tidal Channel Network. Ecol. Model. 139, 293–307. doi: 10.1016/S0304-3800(01)00253-8
Sanderson E. W., Ustin S. L., Theodore C. F. (2000). The Influence of Tidal Channels on the Distribution of Salt Marsh Plant Species in Petaluma Marsh, CA, Usa. Plant Ecol. 146, 9–41. doi: 10.1023/A:1009882110988
Schwarz C., Ye Q. H., Van Der Wal D., Zhang L. Q., Bouma T., Ysebaert T., et al. (2014). Impacts of Salt Marsh Plants on Tidal Channel Initiation and Inheritance. J. Geophys. Res. Earth Surf. 119, 385–400. doi: 10.1002/2013JF002900
Schwarz C., Ysebaert T., Vandenbruwaene W., Temmerman S., Zhang L., Herman P. M. J. (2016). On the Potential of Plant Species Invasion Influencing Bio-Geomorphologic Landscape Formation in Salt Marshes. Earth Surf. Process. Landf. 41, 2047–2057. doi: 10.1002/esp.3971
Shi W., Shao D., Gualtieri C., Purnama A., Cui B. (2019). Modelling Long-Distance Floating Seed Dispersal in Salt Marsh Tidal Channels. Ecohydrology 13. doi: 10.1002/eco.2157
Silvestri S., Defina A., Marani M. (2005). Tidal Regime, Salinity and Salt Marsh Plant Zonation. Estuar. Coast. Shelf. Sci. 62, 119–130. doi: 10.1016/j.ecss.2004.08.010
Simberloff D. (2009). The Role of Propagule Pressure in Biological Invasions. Annu. Rev. Ecol. Evol. Syst. 40, 81–102. doi: 10.1146/annurev.ecolsys.110308.120304
Stark J., Meire P., Temmerman S. (2017). Changing Tidal Hydrodynamics During Different Stages of Eco-Geomorphological Development of a Tidal Marsh: A Numerical Modeling Study. Estuar. Coast. Shelf. Sci. 188, 56–68. doi: 10.1016/j.ecss.2017.02.014
Strahler A. N. (1957). Quantitative Analysis of Watershed Geomorphology. EOS. Trans. Am. Geophys. Union. 38, 913–920. doi: 10.1029/TR038i006p00913
Sun L., Shao D., Xie T., Gao W., Ma X., Ning Z., et al. (2020). How Does Spartina Alterniflora Invade in Salt Marsh in Relation to Tidal Channel Networks? Patterns and Processes. Remote Sens. 12, 2983. doi: 10.3390/rs12182983
Takahashi D., Sim S.-W., Park E.-J. (2019). Colonial Population Dynamics of Spartina Alterniflora. Ecol. Model. 395, 45–50. doi: 10.1016/j.ecolmodel.2019.01.013
Taramelli A., Valentini E., Cornacchia L., Bozzeda F. (2017). A Hybrid Power Law Approach for Spatial and Temporal Pattern Analysis of Salt Marsh Evolution. J. Coast. Res. 77, 62–72. doi: 10.2112/si77-007.1
Taramelli A., Valentini E., Cornacchia L., Monbaliu J., Sabbe K. (2018). Indications of Dynamic Effects on Scaling Relationships Between Channel Sinuosity and Vegetation Patch Size Across a Salt Marsh Platform. J. Geophys. Res.-Earth. Surf. 123, 2714–2731. doi: 10.1029/2017JF004540
Taylor C. M., Davis H. G., Civille J. C., Grevstad F. S., Hastings A. (2004). Consequences of an Allee Effect in the Invasion of a Pacific Estuary by Spartina Alterniflora. Ecology 85, 3254–3266. doi: 10.1890/03-0640
Taylor C. M., Hastings A. (2004). Finding Optimal Control Strategies for Invasive Species: A Density-Structured Model for Spartina Alterniflora. J. Appl. Ecol. 41, 1049–1057. doi: 10.1111/j.0021-8901.2004.00979.x
Tucker G. E., Catani F., Rinaldo A., Bras R. L. (2001). Statistical Analysis of Drainage Density From Digital Terrain Data. Geomorphology 36, 187–202. doi: 10.1016/S0169-555X(00)00056-8
Tyler A. C., Zieman J. C. (1999). Patterns of Development in the Creekbank Region of a Barrier Island Spartina Alterniflora Marsh. Mar. Ecol.-Prog. Ser. 180, 161–177.
Vanderklift M. A., Doropoulos C., Gorman D., Leal I., Minne A. J. P., Statton J., et al. (2020). Using Propagules to Restore Coastal Marine Ecosystems. Front. Mar. Sci. 7. doi: 10.3389/fmars.2020.00724
Vilas M. P., Adams M. P., Oldham C. E., Marti C. L., Hipsey M. R. (2017). Fragment Dispersal and Plant-Induced Dieback Explain Irregular Ring-Shaped Pattern Formation in a Clonal Submerged Macrophyte. Ecol. Model. 363, 111–121. doi: 10.1016/j.ecolmodel.2017.09.001
Wang D., Bai J., Gu C., Gao W., Zhang C., Gong Z., et al. (2021). Scale-Dependent Biogeomorphic Feedbacks Control the Tidal Marsh Evolution Under Spartina Alterniflora Invasion. Sci. Tot. Environ. 146495. doi: 10.1016/j.scitotenv.2021.146495
Wang Q., Cui B., Luo M. (2018). Effectiveness of Microtopographic Structure in Species Recovery in Degraded Salt Marshes. Mar. Pollut. Bull. 133, 173–181. doi: 10.1016/j.marpolbul.2018.05.037
Wang H., Krauss K. W., Noe G. B., Stagg C. L., Swarzenski C. M., Duberstein J. A., et al. (2020). Modeling Soil Porewater Salinity Response to Drought in Tidal Freshwater Forested Wetlands. J. Geophys. Res.-Biogeosci. 125. doi: 10.1029/2018jg004996
Wu Y., Liu J., Yan G., Zhai J., Cong L., Dai L., et al. (2020). The Size and Distribution of Tidal Creeks Affects Salt Marsh Restoration. J. Environ. Manag. 259, 110070. doi: 10.1016/j.jenvman.2020.110070
Xiao Y., Tang J. B., Qing H., Zhou C. F., Kong W. J., An S. Q. (2011). Trade-Offs Among Growth, Clonal, and Sexual Reproduction in an Invasive Plant Spartina Alterniflora Responding to Inundation and Clonal Integration. Hydrobiologia 658, 353–363.
Xiao D. R., Zhang L. Q., Zhu Z. C. (2009). A Study on Seed Characteristics and Seed Bank of Spartina Alterniflora at Saltmarshes in the Yangtze Estuary, China. Estuar. Coast. Shelf. Sci. 83, 105–110. doi: 10.1016/j.ecss.2009.03.024
Xie T., Cui B. S., Li S. Z., Bai J. H. (2019). Topography Regulates Edaphic Suitability for Seedling Establishment Associated With Tidal Elevation in Coastal Salt Marshes. Geoderma 337, 1258–1266. doi: 10.1016/j.geoderma.2018.07.053
Xin P., Kong J., Li L., Barry D. A. (2013). Modelling of Groundwater–Vegetation Interactions in a Tidal Marsh. Adv. Water Resour. 57, 52–68. doi: 10.1016/j.advwatres.2013.04.005
Xin P., Zhou T., Lu C., Shen C., Zhang C., D’alpaos A., et al. (2017). Combined Effects of Tides, Evaporation and Rainfall on the Soil Conditions in an Intertidal Creek-Marsh System. Adv. Water Resour. 103, 1–15. doi: 10.1016/j.advwatres.2017.02.014
Zheng S. Y., Gao W. L., Shao D. D., Nardin W., Gualtieri C., Sun T. (2022). The Effects of Intra-Annual Variability of River Discharge on the Spatio-Temporal Dynamics of Saltmarsh Vegetation at River Mouth Bar: Insights From an Ecogeomorphological Model. J. Environ. Inform.
Zheng S. Y., Shao D. D., Sun T. (2018). Productivity of Invasive Saltmarsh Plant Spartina Alterniflora Along the Coast of China: A Meta-Analysis. Ecol. Eng. 117, 104–110. doi: 10.1016/j.ecoleng.2018.03.015
Zheng Z., Zhou Y., Tian B., Ding X. (2016). The Spatial Relationship Between Salt Marsh Vegetation Patterns, Soil Elevation and Tidal Channels Using Remote Sensing at Chongming Dongtan Nature Reserve, China. Acta Oceanol. Sin. 35, 26–34. doi: 10.1007/s13131-016-0831-z
Zhu Z., Bouma T. J., Ysebaert T., Zhang L., Herman P. M. J. (2014). Seed Arrival and Persistence at the Tidal Mudflat: Identifying Key Processes for Pioneer Seedling Establishment in Salt Marshes. Mar. Ecol.-Prog. Ser. 513, 97–109. doi: 10.3354/meps10920
Keywords: tidal channel network, drainage efficiency, geometric features, Spartina alterniflora, vegetation dynamic model, remote sensing
Citation: Zheng S, Shao D, Gao W, Nardin W, Ning Z, Liu Z, Cui B and Sun T (2022) Drainage Efficiency and Geometric Nuances of Tidal Channel Network Mediate Spartina alterniflora Landward Invasion in Marsh-Channel System. Front. Mar. Sci. 9:888597. doi: 10.3389/fmars.2022.888597
Received: 03 March 2022; Accepted: 27 April 2022;
Published: 02 June 2022.
Edited by:
Yang Yang, Nanjing Normal University, ChinaReviewed by:
James Morris, University of South Carolina, United StatesJianzhong Ge, East China Normal University, China
Copyright © 2022 Zheng, Shao, Gao, Nardin, Ning, Liu, Cui and Sun. This is an open-access article distributed under the terms of the Creative Commons Attribution License (CC BY). The use, distribution or reproduction in other forums is permitted, provided the original author(s) and the copyright owner(s) are credited and that the original publication in this journal is cited, in accordance with accepted academic practice. No use, distribution or reproduction is permitted which does not comply with these terms.
*Correspondence: Dongdong Shao, ddshao@bnu.edu.cn