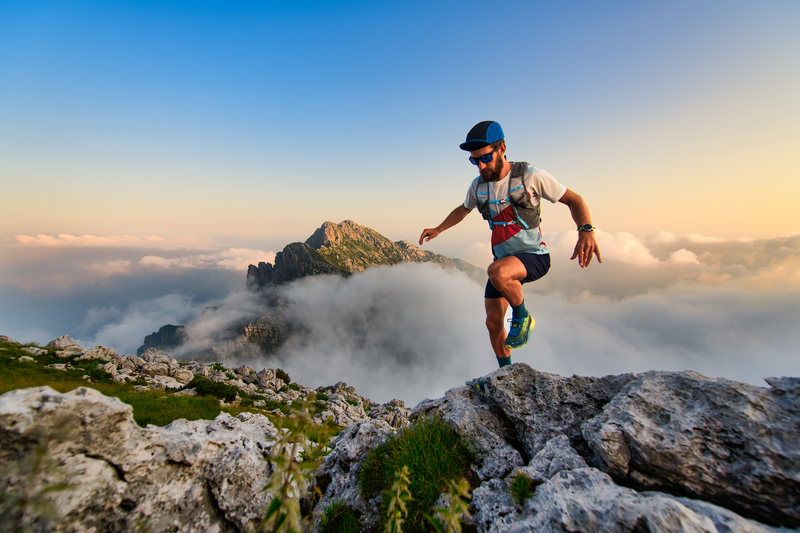
95% of researchers rate our articles as excellent or good
Learn more about the work of our research integrity team to safeguard the quality of each article we publish.
Find out more
ORIGINAL RESEARCH article
Front. Mar. Sci. , 14 July 2022
Sec. Marine Megafauna
Volume 9 - 2022 | https://doi.org/10.3389/fmars.2022.875731
This article is part of the Research Topic Tracking Marine Megafauna for Conservation and Marine Spatial Planning View all 35 articles
Efficient use of the energy budget is of fundamental importance for long-distance migrants, which must cope with seasonal energy demands and environmental conditions. Time-activity budgets can provide information on how animals balance energy use and acquisition over their annual cycle, and on the costs and benefits of different migratory strategies. Baleen whales, such as the fin whale, perform long migrations between feeding and breeding grounds. Although there are now a handful of studies describing the diving and foraging behavior of fin whales, most were carried out at their high-latitude foraging grounds, and very little is known about their behavior in wintering habitats or during migration. We analyzed time-depth recorder data to describe the diving behavior and activity patterns of fin whales in a migratory habitat. Using a hierarchical cluster analysis based on a set of dive variables, we identified six dive types. Four of these dive types (shallow exploratory, shallow active, deep exploratory and deep active) were likely associated to foraging. The other two comprised long non-active dives and dives of variable shape, which may represent resting, traveling or even vocalizing behavior. Shallow exploratory dives were the most frequent dive type (23%) and shallow active were the least frequent (5%). The two deepest dive types, deep active and exploratory, were predominantly carried out during the day, and night dives were significantly shallower than daylight dives, suggesting that fin whales tracked the vertical migration of prey. Whales spent 60% of their dive time engaged in dives associated with feeding and/or prey searching, suggesting they prioritized energy intake over energy conservation. Finally, we found that whales spent more time at or close (<15 m depth) to the surface at night (73%) than during the day (55%), indicating a higher vulnerability to ship strikes during this period. Our study provides novel information on the behavioral patterns and time-activity budgets of fin whales in a migratory habitat. This information is essential for bioenergetic analyses and to predict how fin whales respond to human activities and ongoing environmental changes.
Animals live on limited time and energy, and engagement in some activities often excludes engagement in others. How individuals allocate their time to different activities (e.g., foraging, breeding, resting, traveling) can be critical to their survival and reproductive success, and trade-offs between activities depend on a range of intrinsic (e.g., sex, reproductive status, body condition) and extrinsic drivers (e.g., food availability, density of predators and competitors) (Illius et al., 2002; Rauter and Moore, 2004; Dias et al., 2011).
Many animal populations respond to seasonal changes in resource distribution by migrating between spatially and temporally distinct ranges. Migration allows animals to track variation in food availability, favorable breeding conditions, or avoid harsh environmental conditions, thereby maximizing survival and reproductive success (Alerstam and Bäckman, 2018). However, migrants, especially long-distance migrants, also face unique energetic challenges. During migration, animals not only experience strong fluctuations in resources and environmental conditions, but also prioritize different activities with distinct energetic gains and costs. As resource availability and demands shift throughout migration, animals must adapt their behavior to manage their energy budget (Stern, 2009). Hence, the realized migratory behavior of an individual emerges from the trade-offs between external factors and individual traits. Information on time-activity budgets at different stages of animal migration and under different environmental scenarios can therefore provide valuable insights into these trade-offs, as well as on the consequences of different migratory strategies.
The annual cycle of migratory fin whales (Balaenoptera physalus) consists of various spatially and temporally distinct phases. Most whales spend the summer at high-latitude productive areas feeding on dense aggregations of euphausiids and small pelagic schooling fish (Christensen et al., 1992; Sigurjónsson and Vikingsson, 1997). During this period, fin whales acquire substantial quantities of energy reserves and store the surplus energy in the form of fat depots (Lockyer, 1987; Aguilar and Borrell, 1990). Food availability at these foraging grounds is highly seasonal, and fin whale migration to lower latitudes in late autumn appears to coincide with decreasing water temperatures, salinity, and ultimately prey biomass (Tsujii et al., 2016). Although locomotion costs are lower in large swimming whales, migration to breeding grounds nonetheless requires an energy investment; such investment happens during a stage of the life cycle where feeding is believed to be scarce. Moreover, reproduction in fin whales, like in other large mammals, is energetically demanding, especially during lactation (Oftedal, 2000; Christiansen et al., 2014; Christiansen et al., 2016; Pirotta et al., 2018). As with other capital breeders (Jönsson, 1997; Stephens et al., 2009), the energy necessary for breeding and calving is believed to come mostly from the energy stores gained at the foraging grounds. Thus, fin whales must carefully manage their energy reserves throughout migration, so they can reach breeding grounds, reproduce successfully, and return to productive foraging grounds before their energy reserves are depleted (Miller et al., 2011; Williams et al., 2013; Christiansen et al., 2021).
Nevertheless, feeding outside high-latitude feeding grounds has been reported in fin whales (Silva et al., 2013; Geijer et al., 2016; Silva et al., 2019). Fin whales are commonly encountered off the Azores in spring and early summer (Silva et al., 2014), while migrating to the northern feeding grounds (Silva et al., 2013). The timing of their arrival in the Azores matches the spring phytoplankton bloom in the area (Visser et al., 2011; Prieto et al., 2017) and their movements towards high latitudes are strongly associated with the spatiotemporal distribution of their prey (Pérez-Jorge et al., 2020), suggesting that whales adjust their movements to exploit prey availability along their migratory pathways. Feeding during migration, even if at reduced levels, may be important for the whales’ energy balance. However, foraging is also a costly behavior for these whales. As air-breathing predators, fin whales are constrained by oxygen consumption during a dive, breath holding capacity, and surface recuperation time (Hazen et al., 2015). As a result, it is expected that animals weight the energetic benefit of foraging against the time, metabolic and physiological costs of diving (Boyd et al, 1997). In addition, the filter-feeding strategy (called lunging) used by fin whales to explore efficiently dense patches of small prey items is energetically demanding, limiting dive duration and the number of lunges that can be performed during a single dive, and extending the time needed to recover at the surface (Acevedo-Gutiérrez et al., 2002; Goldbogen et al., 2006; Potvin et al., 2010; Goldbogen et al., 2012). Investigating how much time migrating whales allocate to foraging and how they adjust foraging behavior in comparison to other stages of their annual cycles can provide critical insights into their energy budgets and to the potential benefits of foraging along migration.
Quantifying time-activity budgets in large baleen whales is particularly challenging because these animals are highly mobile and spend prolonged periods underwater, where directly observing their behavior is impossible. Advancements in animal-borne data loggers have enabled the collection of high-resolution data on the vertical and/or horizontal movements of animals (Evans et al., 2013). Several analytical methods using these kinematic data have been developed to infer foraging behavior (Goldbogen et al., 2006; Goldbogen et al., 2013; Sweeney et al., 2019) and describe different activities in several baleen whale species (Oleson et al., 2007; van der Hoop et al., 2017; Izadi et al., 2018; Guazzo et al., 2021). Yet, despite technological developments, most data loggers available for large whales can only stay attached for short periods of time (typically hours to a few days) and cannot be used to document changes in behavior over the annual cycle. The only way to overcome this limitation is to investigate whales’ behavior at different life stages to gain a perspective of their full annual cycle.
The objective of this study is to characterize the dive behavior and activity patterns of fin whales in a migratory habitat. We used data collected from time-depth recorders (TDRs) to (1) describe the dominant dive types of fin whales and infer their potential function, (2) quantify time-activity budgets and (3) determine how these budgets vary over diel periods. This information is critical to support future investigations into the energetic constraints of baleen whale migration and for a better understanding of the relative benefits of different migratory strategies. Moreover, studying whales’ behavior during their migration is essential to understand their vulnerability to impacts from human activities (Christiansen et al., 2013; Marra et al., 2015). This is of particular importance for these long-distance migrants who cross vast ocean regions during which they are exposed to various threats.
Migrating fin whales were tagged off the Azores (38° N 28° W) between April and September, from 2007 to 2017 (Figure 1; Table S1). Tags consisted of a TDR (Wildlife Computers Mk9), a VHF radio transmitter (Telonics MOD125 cast3 or ATS F1835B) and syntactic foam for positive buoyancy. The TDR recorded depth data at the highest sampling rate of 1 s with a resolution of 0.5 m and precision of ±1%. Tags were attached anterior to the dorsal fin of the whales by a suction cup (in 2007) or using umbrella darts (all other years) (Table S1; Domeier et al., 2005) attached sub-dermally, and connected to the tag by the use of a galvanic timed-release system. Darts were sterilized in an UV light chamber and by immersion in 70% ethanol and stored in a sealed sterilized plastic bag until deployment. Tags were deployed from a rigid-hulled inflatable boat using either a telescopic pole (for suction cup attachments) or a compressed air gun (ARTS/RN, Restech Norway). A VHF beacon in the tag aids in tracking and recovering the device. Tagged whales were followed for as long as weather and light conditions allowed to record their surfacing positions (Figure 1) and behavior. After released from the whales, the TDRs were located and recovered using VHF transmissions. Fieldwork and tagging were approved and conducted under research permits from the competent authorities (Regional Directorate of the Environment and Regional Directorate for Sea Affairs, Regional Government of the Azores) and tagging procedures followed the guidelines of the American Society of Mammalogists (Sikes and Gannon, 2011, & The Animal Care and Use Committee of the American Society of Mammalogists, 2011). A total of 21 fin whales were tagged with duration of deployments ranging from approximately 2 to 41 hours (Table S1).
Figure 1 Map of the study area showing the tracks of 21 fin whales (color coded by individual whale) tagged with TDRs. Tracks were plotted using the GPS coordinates retrieved at surfacing locations of tagged whales during focal follows and represent on average 25% of the total duration of tag attachments.
TDR data were processed using the “diveMove” package in R (Luque, 2007; R Core Team, 2021). Before analysis, data were truncated to remove periods before tag deployment and after the tag detached from the whale. A zero offset correction was first applied to correct shifts in the surface baseline of depth recordings. A dive was then defined as any submergence deeper than 15 m to exclude surface respiration activity (Croll et al., 2001). Each dive was automatically divided into three phases: descent, bottom and ascent, based on changes in vertical rate of the smoothed dive profile. A brief stop at shallow depths occurred during the descent and ascent phases in some dives. Presence of these “shoulders” led to the incorrect inclusion of a large part of these transit segments in the bottom phase of the dives (e.g., Irvine et al., 2017) (Figure S1). These segments were manually corrected, with the bottom phase starting at the first inflexion point following a “shoulder” in the descent phase, or ending at the last inflexion point before the “shoulder” in the ascent phase.
A total of ten variables were calculated for each dive: maximum depth, dive duration, post-dive duration, descent and ascent rate, duration of bottom phase, mean and standard deviation (SD) of bottom depth, proportion of time spent at the bottom in relation to the dive duration (hereafter bottom time proportion) and depth range of the bottom phase (hereafter bottom depth range) (Table 1). Univariate outliers were found for post-dive duration and the corresponding dives were removed, representing 0.4% of all dives. All variables were checked for normality and transformed when necessary. Unless stated otherwise, data are reported as median values.
Table 1 Description and summary statistics (median and range) of the dive variables analyzed in this study.
TDR data has been used extensively to quantify activity patterns in air-breathing diving vertebrates. This requires identifying specific dive types associated with different activities (e.g., feeding, traveling, resting, etc.) based on dive profiles to produce a continuous activity profile, from which time allocated to different activities can be calculated (Bodkin et al., 2007). Here, we used the dive variables listed above to classify all dives into different groups. Following Irvine et al. (2017) approach, we used a Principal Component Analysis (PCA) and a hierarchical clustering analysis to identify dive groups, without a priori knowledge of the total number of clusters. Because some dive variables were strongly correlated, the PCA was performed on the scaled and centered data to minimize multicollinearity and reduce the dimensionality of the data. Variable loadings were calculated to determine which variables had a higher positive or negative correlation to each principal component. Then, the components explaining more variance and complying with the latent root criterion (which states that a factor must account for at least the amount of variance associated with one hypothetical variable) (McGarigal et al., 2000) were used as inputs in the clustering analysis using the Euclidean distance and Ward’s method (Legendre and Legendre, 1998). The optimal number of clusters was defined by trimming the resulting dendrogram at different levels and evaluating the stability and biological meaningfulness of the resulting clusters. The cluster stability was assessed through the Jaccard similarity coefficient (hereafter Jaccard index), which ranges from 0 to 1, with values <0.6 indicating an unstable cluster, values between 0.6-0.75 indicating a pattern in the data, and those >0.75, a stable cluster (Hennig, 2014). Mean Jaccard index values were obtained through a bootstrap with 1000 iterations using the “clusterboot” function in the “fpc” R package (Hennig, 2014). A visual inspection of the dives composing each cluster was carried out to assess the presence of dominant dive shapes (e.g., squared-, U- or V-shaped) previously described in the marine mammal literature (Heide-Jørgensen et al., 2013; Irvine et al., 2017; Citta et al., 2021).
Based on the cluster analysis results, time-activity budgets were constructed by calculating the number of dives of each type as a proportion of the total dives performed by each individual whale, as well as the proportion of time allocated to each dive type as a proportion of total diving time (sum of all dive durations) and total tagging time. Percentage of time spent at the surface between dives was calculated by dividing the total post-dive durations by the duration of tag attachment. We also calculated the percentage of time each whale remained in the first 15 m of the water column by dividing it by total tagging time. Lastly, linear mixed models (LMMs) were used to investigate diel patterns in dive type and in surface usage, using data from whales with deployments longer than 15 h. LMMs were fitted with a Gamma family distribution and a logit link function, using the restricted maximum likelihood as an optimization method and individual whale ID as a random effect to account for individual effects (“mgcv” R package; Wood, 2011). We chose this family because either the response variable clearly followed a gamma distribution and/or it provided the best fit for the model, which we assessed through residual analysis (Wu, 2005). Separate models were fit for each of the following response variables: maximum dive depth, absolute number of dives of each type, percentage of post-dive duration (hereafter surface periods) and percentage of time spent within the first 15 m of the water column. Dive type and/or diel period (Day/Night) were used as explanatory variables, with an interaction term when the models included both variables (Table S4). Dives were assigned to daylight or night-time regimes based on local sunrise and sunset times provided by NOAA Solar Calculator (http://www.esrl.noaa.gov/gmd/grad/solcalc/calcdetails.html). When deemed necessary, a correlation structure was applied to account for temporal autocorrelation. All numerical and statistical analysis were performed in R (R Core Team, 2021).
We analyzed a total of 2594 dives performed by 21 fin whales. The deepest dive reached 339 m and the longest was 17.3 min, but only 20% of the dives were deeper than 100 m and 30% longer than 5 min. Number of dives analyzed per individual whale ranged from 11 to 344, and number of dives/hour varied between 2.5 and 16.7 (Table S1). Of the 21 deployments, 10 were longer than 15 h, comprising 1930 dives.
Most of the variability in whales’ diving behavior was explained by the first three principal components (84% of total variance) (Table S2). PC1 explained 40.6% of the variance and had strong positive loadings for bottom depth range, standard deviation of bottom depth, dive duration, bottom duration and bottom time proportion (Table S3). PC2 explained 28.9% of the variance and had strong negative loadings for mean bottom depth, ascent rate, maximum depth and descent rate. PC3 explained the least variance (14.6%) and had strong positive loadings for post-dive and dive durations, and a strong negative loading for descent rate.
The hierarchical cluster analysis indicated that all dives grouped into six clusters (Figure 2). According to the Jaccard similarity values, one of these clusters was stable, two clusters showed a pattern in the data and three clusters had a low degree of stability (Table 2). Cluster stability did not improve by changing the number of clusters, suggesting that the optimal number of clusters was six. Clusters were labelled: “shallow exploratory”, “long non-active”, “shallow active”, “deep exploratory”, “deep active” and “variable” (Table 2; Figure 2). Dive clusters were primarily split by: (i) depth; i.e., shallow (shallow exploratory, long non-active, shallow active) versus deep/intermediate dives (deep exploratory, deep active, variable); and (ii) the characteristics of the bottom phase; i.e., active (long bottom phase and high variability in depth), exploratory (short or inexistent bottom phase) and non-active (long bottom phase with little or no depth variability).
Figure 2 3D scatterplot of the first three components of the PCA (A) and dendrogram of the hierarchical clustering analysis of 10 dive variables (B), where the y-axis represents the dissimilarity between clusters (height) and the red line the level at which the dendrogram was “cut”.
Table 2 Median and range of values of each variable for the six dive types identified by the cluster analysis.
Shallow exploratory dives represented the most stable (Jaccard index: 0.763) and common dive type (Table 2). These dives were shallow (median maximum depth of 32.7 m) and short (1.6 min), with a short or no bottom phase, and little depth variability (Figure 3). Long non-active dives (Jaccard index: 0.626) were shallower, longer (4.3 min) and had longer post-dive durations than the remaining shallow dives. In fact, post-dive duration in these dives was within the range of that of deep dives. Vertical descent and ascent rates of long non-active dives were the slowest of all dives and bottom phase was generally long (2.2 min), accounting for about 55% of dive duration. However, compared to other dives with long bottom phases, variability in depth was considerably lower. Shallow active dives (Jaccard index: 0.562) were the only shallow dive with an active bottom phase. Maximum depth and post-dive duration were similar to shallow exploratory dives but dives were 1.6 times longer and had a long bottom phase (48% of dive time) with high variability in depth. The bottom phase of deep exploratory dives (Jaccard index: 0.651) was short or non-existent and varied little in depth, similarly to shallow exploratory dives. The two dive types differed mostly in maximum depth (difference of ~80 m), as well as in dive and post-dive duration, respectively 2.8 and 2.3 times longer in deep exploratory dives. This was the only dive type for which descent rate was slower than ascent rate. Deep active dives (Jaccard index: 0.545) had the greatest bottom depth range and variability, and ascent and descent rates. Maximum depth and duration were similar to those of deep exploratory dives. Lastly, variable dives had the lowest degree of stability (Jaccard index: 0.471). These dives were at intermediate depths between shallow and deep dives, had the longest dive and bottom phase durations, and the highest bottom time proportion (59.1%). Similar to deep dives, post-dive duration was long (1.7 min).
Figure 3 A section of the dive profile of whale #19 with dives colored according to the dive type identified by the cluster analysis.
The majority of tagged whales performed all dive types (Figure 4A; Table S5). Overall, whales performed more deep/intermediate dives (median: 60%) than shallow excursions (40%), and more exploratory (39%) than active dives (22%), even though variability between individuals was high. Shallow exploratory (23%) and variable (20%) dives were the most frequent dive types. However, the time whales spent performing variable dives (27%) was much longer in comparison to the shallow exploratory dive type (11%) (Figure 5). Deep exploratory and deep active dives represented only 14% and 16%, respectively, of all dives. Long non-active (6%) and shallow active (5%) were the least frequent dives and represented only 8% and 4%, respectively, of whale’s dive time budget.
Figure 4 Percentage number of each dive type (shown with different colors) by individual for 21 fin whales tagged in this study (A). Percentage number of each dive type during the day (B) and night (C) for 10 fin whales with tag attachments longer than 15 h.
Figure 5 Percentage of time spent in each dive type (shown with different colors) by individual for 21 fin whales tagged in this study (A). Percentage of time spent in each dive type during the day (B) and night (C) for 10 fin whales with tag attachments longer than 15 h.
The mixed-effects models on the absolute number of dives and maximum dive depth showed significant differences in diel patterns for most dive types (Table S4; Figure S4). Deep active and deep exploratory dives were more frequent during the day than at night (Figures 4B, C, 5), with diving depth progressively decreasing close to sunset and increasing again towards sunrise (Figures 6, S3). Long non-active and variable dives were also more common during the day than at night. Only shallow exploratory dives were significantly more frequent at night than during the day. No significant diel differences were found in the proportion of shallow active dives. However, not all whales showed the same pattern, with two individuals tagged on consecutive days (12 and 13) spending most of their diving time in variable dives and nigh-time at the surface (Figures 5, 7). The mixed-effects models also revealed significant diel differences in surface usage (Table S4). Whales spent significantly more time in surface periods at night (median: 60%) than during the day (41%). Nonetheless, there was high individual variability in surface use at night, with the proportion of time spent in surface periods varying from ~30% to over 90% between whales (Figure 7). In addition, proportion of time spent in the upper 15 m of the water column was also significantly higher at night (73%) than during the day (55%).
Figure 6 Dive profile of whale #16 illustrating the diel pattern in diving behavior and dive types, as identified by the cluster analysis. The shaded area indicates the night period.
Figure 7 Percentage of tag attachment time spent in surface periods during the day (A) and night (B) for 10 fin whales with tag attachments longer than 15 h.
We used dive metrics calculated from TDR data to characterize different dive types, infer their potential function, and determine time-activity budgets of fin whales in a migratory habitat. To the best of our knowledge, this represents the first detailed description of the diving behavior and activity patterns of this species in a migratory habitat.
Based on the results of the PCA, the hierarchical cluster analysis identified six dive clusters. We should stress, however, that the unsupervised classification method utilized is unable to precisely categorize each dive. While automated methods allow a fast and objective classification of dives, the classification is not free from errors, particularly for underrepresented dive classes (Thums et al., 2008). Therefore, dive types represent generalizations of the more complex dive behavior of fin whales. Additionally, characterization and discrimination of different dive types would advance significantly by using tags with tri-axial accelerometers, enabling the measurement of behavioral metrics (e.g., whale orientation, speed, acceleration, stroking activity) that can help distinguish active locomotory from inactive behaviors (Brown et al., 2013) or identify feeding lunges (Goldbogen et al., 2006; Ware et al., 2011).
Active dives had a prolonged bottom phase, sometimes reaching 10 min, and variable bottom depth, as a result of single or multiple vertical excursions (Figure S2C). These vertical excursions generally occurred within a restricted depth layer at the bottom of the dive, but occasionally happened during the ascent or descent. A prolonged bottom phase is characteristic of the foraging dives of several marine predators and arises from the individuals’ attempt to maximize time at a foraging patch (Le Boeuf et al., 1988; Fedak et al., 2001; Thompson and Fedak, 2001; Schreer et al., 2001; Witteveen et al., 2008). Foraging dives also have fast transit phases (Goldbogen et al., 2011), as observed here in deep and shallow active dives. In addition, in fin whales and other balaenopterid whales, vertical excursions close to the bottom are believed to represent lunging behavior, in which the whales accelerate upward towards a prey patch (Goldbogen et al., 2006; Simon et al., 2012). Finally, the clear diel pattern in active dives, where shallow dives dominated at night and deep dives during the day, is consistent with tracking the diel vertical migration of prey (see below). Taken together, these results provide strong evidence that deep and shallow active dives are associated with feeding activity. On the other hand, exploratory dives were short, with little or no bottom time, strongly resembling the stereotypical V-shaped dive described for baleen whales (Schreer et al., 2001; Heide-Jørgensen et al., 2013) (Figure S2A). Shallow and deep exploratory dives reached similar depths and showed the same diel pattern as their active counterparts, suggesting they could serve to assess prey availability in the water column. They may also include aborted or failed foraging dives. Shallow exploratory dives could also encompass periods of horizontal travel. The relatively slow descent and ascent rates of these dives indicate that whales were either swimming slowly at a steep angle or more horizontally at normal speeds (Martin et al., 1998). Shallow V-dives may enable whales to avoid surface drag (see below), thus increasing swimming efficiency.
The remaining two clusters comprised a high diversity of dive shapes, although some shapes were dominant. Long non-active dives included large numbers of squared dives (Figure S2B), which may represent resting or traveling. The long bottom phase and small depth variability of these dives suggest that whales were either motionless or moving horizontally. Given that the impact of wave drag is lowest at depths greater than three times the body diameter of an animal (Hertel, 1966), resting and traveling under this threshold, while still remaining close to the surface, could be energetically efficient. The estimated depth threshold below which blue whales should swim to avoid wave drag (12 m) (Owen et al., 2016) is close to the depth of long non-active dives. The characteristics of long non-active dives are also consistent with singing behavior. Singing fin whales generally swim slowly and display minimal body movement (Clark et al., 2019; Guazzo et al., 2021), remaining at 15-50 m depth (Watkins et al., 1987; Aroyan et al., 2000; Stimpert et al., 2015), where they are neutrally buoyant (Goldbogen et al., 2006). Singing is also believed to be energetically demanding (Clark et al., 2019), which could explain the long surface times of these dives. Although our data were mostly collected in spring and early summer, when fin whale songs are rarely detected in the study area (Romagosa et al., 2020), this dive type could be associated with the production of other acoustic signals, like the 40 Hz call regularly emitted by foraging whales (Romagosa et al., 2021). The variable dive type encompassed dives of many different shapes, which likely serve multiple functions. The long dive, bottom and post-dive durations, high bottom time proportion and great variability in depth, also typical of active dives, suggest that variable dives include periods of feeding activity. Other dive shapes were similar to the root-square dive documented in pinnipeds (Ramasco, 2008; McIntyre et al., 2011), where whales travelled to the maximum dive depth (usually <100 m), and immediately started ascending, barely spending time at the bottom, like in V-shaped dives. The unique feature of these dives was that, before or after this “peak” in depth, whales remained a long time (up to 10 min) in the first 35 m of the water column, with little variation in depth (Figure S2D). These dives could represent exploratory dives, followed by transiting to a different prey patch, resting, or even singing activity.
Fin whales exhibited a strong diel pattern in diving behavior, shifting between predominantly deep dives during the day and shallow dives at night. This diel pattern has been reported elsewhere in fin and other baleen whale species and is likely related to the diel vertical migration of their prey (Panigada et al., 1999; Calambokidis et al., 2007; Friedlaender et al., 2009; Friedlaender et al., 2013). Most baleen whale prey undertake vertical migrations, residing in deep waters during the day, where they form dense aggregations, and ascending to the surface at night, where they disperse to forage (Mauchline and Fisher, 1967; Raymont, 1983). Baleen whales adapt their feeding strategies to the diel changes in density and vertical distribution of prey. Antarctic humpback whales (Megaptera novaeangliae) and St. Lawrence blue whales (Balaenoptera musculus) foraged preferentially on near-surface, less dense prey aggregations at night, presumably to minimize the energy costs and surface recovery time associated with longer, deeper dives, thereby maximizing foraging efficiency (Doniol-Valcroze et al., 2011; Ware et al., 2011; Friedlaender et al., 2013; Friedlaender et al., 2016). Conversely, fin and blue whales in the eastern North Pacific rarely foraged at night (Friedlaender et al., 2015). In fact, whales decreased the frequency of feeding dives and the number of feeding lunges when foraging on shallow, low-density prey, but increased foraging effort when targeting deep denser patches (Hazen et al., 2015; Friedlaender et al., 2020). By increasing the number of lunges on denser patches, whales can substantially increase prey intake and energy efficiency, despite of the higher costs of diving deep to reach these patches (Hazen et al., 2015; Friedlaender et al., 2020).
Our results indicate that deep active dives were longer and had more vertical excursions, which likely represent lunges, than shallow active dives. This is consistent with earlier studies (Hazen et al., 2015; Friedlaender et al., 2020) and suggests that fin whales increased their lunge-feeding rates in deep dives for foraging to become efficient. However, fin whales in this study mainly foraged in waters <100 m and the median depth of deep dives was considerably shallower than in other areas (Friedlaender et al., 2020). It is possible that the density of deeper prey was insufficient to compensate the higher costs of diving deeper to target these patches. Under these circumstances, foraging throughout the diel cycle on shallow prey may represent a more efficient foraging strategy, explaining why the frequency of deep foraging dives was similar to that of shallow foraging dives. Information on prey density and depth, as well as on consumption rates of fin whales in the area, is necessary to confirm this hypothesis and to estimate the potential energy gain of this strategy.
Nonetheless, not all whales exhibited the same pattern in foraging activity. Two individuals did not perform shallow active dives during the night and devoted less time than average to deep diving (active and exploratory) during the day. Instead, these whales spent the majority of their tagging time (18 and 38 h) engaged in variable dives. Given the diversity of shapes of variable dives, we believe they serve more than one function, complicating the interpretation of these results. Predominance of variable dives could indicate an alternative foraging strategy to exploit different prey (Goldbogen et al., 2015), that prey density was too low to allow efficient foraging, leading to increased transiting between prey patches, or that whales prioritized resting over foraging.
The diel pattern in fin whale dive behavior implies that, at night, whales remain most of the time near the surface, and even when diving, they spend substantial time in the upper water column (<15 m). Increased surface usage exposes whales to a higher risk of ship strikes at night (McKenna et al., 2015), when whales cannot be detected by visual observers’ onboard vessels. These results are in line with findings from other areas and times of year (Calambokidis et al., 2019; Keen et al., 2019), emphasizing the need to account for diel changes in whale’s dive behavior when estimating the risk of ship strikes and devising mitigation measures.
Tagged whales spent 56% of their time diving and 44% at or near the surface, with some variability among individuals (Figure 7). Time spent in surface activities includes surface recovery times between dives, as well as resting or traveling periods. While classifying the time spent at surface broadly as surface periods may have led to the underestimation of certain behavioral states, it is important to stress that the distinction of sub-surface activities is unfeasible with only two-dimensional dive data.
Fin whales spent about 8% of their diving time in long non-active dives, which we classified as potential horizontal traveling and resting. This value represents 4% of total tagging time, which is strikingly low when compared to the 23-35% of time spent traveling (Visser et al., 2011; Silva et al., 2013) and ~18% resting (Visser et al., 2011) previously reported for the same area. As stated above, one explanation is that traveling and resting occurs mostly in near-surface waters (<15 m), and therefore, below our dive threshold. It is also probable that traveling and resting are included in variable dives, which accounted for a median of 27% of the time-activity budget and 14% of total tagging time of fin whales.
The dominant dive types of fin whales were associated with foraging activity. Assuming that active and exploratory dives represent feeding and searching for prey, whales spent about 60% of their diving time, or 32% of total tagging time, in feeding-related activities. Our results are slightly lower than the values reported from fin whales instrumented with satellite tags in the same area, which indicated that whales spent 55% of their daily time in area-restricted search, believed to mostly represent foraging (Silva et al., 2013). However, we stress that ARS calculations also include time spent at surface, while our results reflect solely diving time.
Animal migrations are commonly understood as a response to the spatiotemporal variation in resources across broad scales (Dingle and Drake, 2007), but animals also respond to finer-scale variation in resources along their migrations. A wide range of terrestrial and marine migrants have been shown to track phenological variation in prey across space, thereby prolonging access to resources available and enhancing their energy gain (Armstrong et al., 2016). Recent work shows that migrating blue whales track resources by matching their movements with the long-term average phenology of the phytoplankton bloom (Abrahms et al., 2019). This work provides empirical support to modelling studies that demonstrate the emergence of blue whale migrations to track prey resources, allowing whales to support their energy demands (Pirotta et al., 2018).
Previous studies have shown that the timing of fin whale arrival in the Azores migratory habitat matches the seasonal peak in productivity (Visser et al., 2011; Prieto et al., 2017) and individuals can remain in the area for days to a few weeks (Silva et al., 2013). Movements of fin whales in the Azores and towards higher latitudes are closely linked to prey biomass (Pérez-Jorge et al., 2020), suggesting that whales track shifting hotspots of prey availability across space, similarly to blue whales (Abrahms et al., 2019). Here we examined the fine-scale behavior of migratory fin whales at a foraging hotspot. We found that whales allocate a significant proportion of their diving time to active and exploratory diving, which likely represent foraging, suggesting that whales prioritize energy intake over energy conservation. Although we were unable to quantify the energy accrued by fin whales, together these findings suggest that energy intake during migration might be higher than previously assumed. By moving to track the seasonal phenology of prey, fin whales could maximize use of the energy available to them, despite the ephemerality of individual forage patches (Abrahms et al., 2021). While the benefits of this strategy seem intuitive, they remain to be quantified. Detailed measurements of the underwater behavior of fin whales, as well as information on prey availability, will be necessary to estimate the energy gain and cost of foraging during migration. Moreover, data covering a larger spatiotemporal scale will be necessary to assess how recurrent this behavioral pattern is along the migratory route and its contribution to fin whale’s annual energy budget. This knowledge will be particularly important to understand potential impacts from climate change. As climate change is modifying seasonal rhythms of resources, this may interfere with the ability of fin whales to efficiently track resources during migration, as reported across different taxa (Cohen et al., 2018). Assessing the consequences of phenological changes in prey distribution for fin whale energetics will be critical to determine effects at the population level.
The raw data supporting the conclusions of this article will be made available by the authors, without undue reservation.
The animal study was reviewed and approved by Regional Directorate of the Environment and Regional Directorate for Sea Affairs, Regional Government of the Azores.
CTF analyzed the data, wrote the manuscript, contributed to the methodology. SPJ contributed to the methodology, data analysis, and writing. RP conceived and designed the study, contributed to funding acquisition and project administration, undertook fieldwork and collected data. CO and MT undertook fieldwork and collected data. AS contributed to the methodology and data analysis. MAS conceived and designed the study, contributed to funding acquisition and project administration, undertook fieldwork and collected data, contributed to the methodology, and wrote sections of the manuscript. All authors reviewed the submitted version of the manuscript. All authors contributed to the article and approved the submitted version.
Research was supported by the Portuguese Science & Technology Foundation (FCT), the Azorean Science & Technology Fund (FRCT), and the EU through research projects TRACE-PTDC/MAR/74071/2006, MAPCET-M2.1.2/F/012/2011, FCT-IF/00943 /2013/CP1199/CT0001, SUMMER-H2020 GA 817806 (FEDER, COMPETE, QREN, POPH, ESF, Portuguese Ministry for Science and Education, AZORES2020), and individual contracts/grants to CTF, SPJ and MAS (SUMMER-H2020, GA 817806), RP (SFRH/BPD/108007/2015), CO (3/DRAM/2019 through RAGES), and MT (M3.1.a/F/072/2015). MS and RP are co-financed by AZORES2020, through the EU Fund 01-0145-FEDER-000140 “MarAZ Researchers: Consolidate a body of researchers in Marine Sciences in the Azores”. Okeanos is funded by FCT – Foundation for Science and Technology under the project UIDB/05634/2020 and UIDP/05634/2020, and through the Regional Government of the Azores through the initiative to support the Research Centers of the University of the Azores and through the project M1.1.A/REEQ.CIENTÍFICO UI&D/2021/010.
The authors declare that the research was conducted in the absence of any commercial or financial relationships that could be construed as a potential conflict of interest.
All claims expressed in this article are solely those of the authors and do not necessarily represent those of their affiliated organizations, or those of the publisher, the editors and the reviewers. Any product that may be evaluated in this article, or claim that may be made by its manufacturer, is not guaranteed or endorsed by the publisher.
We thank Irma Cascão, Rebecca Boys, Yves Cuenot, Pablo Chevallard Navarro, and numerous interns and volunteers who over the years helped with field trips. We are also grateful to our skippers, Renato Bettencourt, Paulo Martins and Vitor Rosa.
The Supplementary Material for this article can be found online at: https://www.frontiersin.org/articles/10.3389/fmars.2022.875731/full#supplementary-material
Abrahms B., Aikens E. O., Armstrong J. B., Deacy W. W., Kauffman M. J., Merkle J. A. (2021). Emerging Perspectives on Resource Tracking and Animal Movement Ecology. Trends Ecol. Evol. 36 (4), 308–320. doi: 10.1016/j.tree.2020.10.018
Abrahms B., Hazen E. L., Aikens E. O., Savoca M. S., Goldbogen J. A., Bograd S. J., et al. (2019). Memory and Resource Tracking Drive Blue Whale Migrations. Proc. Natl. Acad. Sci. U.S.A. 116 (12), 5582–5587. doi: 10.1073/pnas.1819031116
Acevedo-Gutiérrez A., Croll D. A., Tershy B. R. (2002). Feeding Costs Limit Dive Time in Large Whales, Vol. 7.
Acevedo-Gutiérrez A., Croll D. A., Tershy B. R. (2002). Feeding Costs Limit Dive Time in Large Whales. J Exp Biol. 205, 1747–1753
Aguilar A., Borrell A. (1990). Patterns of Lipid Content and Stratification in the Blubber of Fin Whales (Balaenoptera Physalus). J. Mammal. 71 (4), 544–554. doi: 10.2307/1381793
Alerstam T., Bäckman J. (2018). Ecology of Animal Migration. Curr. Biol. 28 (17), R968–R972. doi: 10.1016/j.cub.2018.04.043
Armstrong J. B., Takimoto G., Schindler D. E., Hayes M. M., Kauffman M. J. (2016). Resource Waves: Phenological Diversity Enhances Foraging Opportunities for Mobile Consumers. Ecology 97, 1099–1112. doi: 10.1890/15-0554.1
Aroyan J. L., McDonald M. A., Webb S. C., Hildebrand J. A., Clark D., Laitman J. T., et al. (2000). “Acoustic Models of Sound Production and Propagation,” in Hearing by Whales and Dolphins, vol. Vol. 12 . Eds. Au W. W. L., Fay R. R., Popper &A.N. (New York: Springer), 409–469. doi: 10.1007/978-1-4612-1150-1_10
Bodkin J. L., Monson D. H., Esslinger G. G. (2007). Activity Budgets Derived From Time–Depth Recorders in a Diving Mammal. J. Wildlife. Manage. 71 (6), 2034–2044. doi: 10.2193/2006-258
Boyd I. L., McCafferty D. J., Walker T. R. (1997). Variation in Foraging Effort by Lactating Antarctic Fur Seals: Response to Simulated Increased Foraging Costs. Behav. Ecol. Sociobiol. 40, 135–144. https://doi.org/10.1007/s002650050326
Brown D. D., Kays R., Wikelski M., Wilson R., Klimley A. (2013). Observing the Unwatchable Through Acceleration Logging of Animal Behavior. Anim. Biotelem. 1 (1), 20. doi: 10.1186/2050-3385-1-20
Calambokidis J., Fahlbusch J. A., Szesciorka A. R., Southall B. L., Cade D. E., Friedlaender A. S., et al. (2019). Differential Vulnerability to Ship Strikes Between Day and Night for Blue, Fin, and Humpback Whales Based on Dive and Movement Data From Medium Duration Archival Tags. Front. Mar. Sci. 6. doi: 10.3389/fmars.2019.00543
Calambokidis J., Schorr G. S., Steiger G. H., Francis J., Bakhtiari M., Marshall G., et al. (2007). Insights Into the Underwater Diving, Feeding, and Calling Behavior of Blue Whales From a Suction-Cup-Attached Video-Imaging Tag (Crittercam). Mar. Technol. Soc. J. 41 (4), 19–29. doi: 10.4031/002533207787441980
Christensen I., Haug T., Øien N. (1992). A Review of Feeding and Reproduction in Large Baleen Whales (Mysticeti) and Sperm Whales Physeter Macrocephalus in Norwegian and Adjacent Waters. Fauna. norv. Ser. A. 13, 39–48.
Christiansen F., Dujon A. M., Sprogis K. R., Arnould J. P. Y., Bejder L. (2016). Noninvasive Unmanned Aerial Vehicle Provides Estimates of the Energetic Cost of Reproduction in Humpback Whales. Ecosphere 7 (10). e01468. doi: 10.1002/ecs2.1468
Christiansen F., Rasmussen M. H., Lusseau D. (2013). Inferring Activity Budgets in Wild Animals to Estimate the Consequences of Disturbances. Behav. Ecol. 24 (6), 1415–1425. doi: 10.1093/beheco/art086
Christiansen F., Rodríguez-González F., Martínez-Aguilar S., Urbán J., Swartz S., Warick H., et al. (2021). Poor Body Condition Associated With an Unusual Mortality Event in Gray Whales. Mar. Ecol. Prog. Ser. 658, 237–252. doi: 10.3354/meps13585
Christiansen F., Víkingsson G. A., Rasmussen M. H., Lusseau D. (2014). Female Body Condition Affects Foetal Growth in a Capital Breeding Mysticete. Funct. Ecol. 28 (3), 579–588. doi: 10.1111/1365-2435.12200
Citta J. J., Olnes J., Okkonen S. R., Quakenbush L., George J. C., Maslowski W., et al. (2021). Influence of Oceanography on Bowhead Whale (Balaena Mysticetus) Foraging in the Chukchi Sea as Inferred From Animal-Borne Instrumentation. Continent. Shelf. Res. 224, 104434. doi: 10.1016/j.csr.2021.104434
Clark C. W., Gagnon G. J., Frankel A. S. (2019). Fin Whale Singing Decreases With Increased Swimming Speed. R. Soc. Open Sci. 6 (6), 180525. doi: 10.1098/rsos.180525
Cohen J. M., Lajeunesse M. J., Rohr J. R. (2018). A Global Synthesis of Animal Phenological Responses to Climate Change. Nat. Clim. Change 8, 224–228. doi: 10.1038/s41558-018-0067-3
Croll D. A., Acevedo-Gutierrez A., Tershy B. R., Urban-Ramırez J. (2001). The Diving Behavior of Blue and fin Whales: Is Dive Duration Shorter Than Expected Based on Oxygen Stores? 13. Comp. Biochem. Physiol. A. 129 (4), 797–809. doi: 10.1016/S1095-6433(01)00348-8
Croll D. A., Acevedo-Gutierrez A., Tershy B. R., Urban-Ramirez J. (2001). The Diving Behavior of Blue and fin Whales: Is Dive Duration Shorter Than Expected Based on Oxygen Stores? Comp Biochem Physiol A. 129(4), 797–809. https://doi.org/10.1016/S1095-6433(01)00348-8
Dias P. A. D., Rangel-Negrín A., Canales-Espinosa D. (2011). Effects of Lactation on the Time-Budgets and Foraging Patterns of Female Black Howlers (Alouatta Pigra). Am. J. Phys. Anthropol. 145 (1), 137–146. doi: 10.1002/ajpa.21481
Domeier M. L., Kiefer D., Nasby-Lucas N., Wagschal A., O’Brien F. (2005). Tracking Pacific Bluefin Tuna (Thunnus Thynnus Orientalis) in the Northeastern Pacific With an Automated Algorithm That Estimates Latitude by Matching Sea-Surface-Temperature Data From Satellites With Temperature Data From Tags on Fish. Fish. Bull. 103 (2), 292–306.
Doniol-Valcroze T., Lesage V., Giard J., Michaud R. (2011). Optimal Foraging Theory Predicts Diving and Feeding Strategies of the Largest Marine Predator. Behav. Ecol. 22 (4), 880–888. doi: 10.1093/beheco/arr038
Evans K., Lea M. A., Patterson T. A. (2013). Recent Advances in Bio-Logging Science: Technologies and Methods for Understanding Animal Behaviour and Physiology and Their Environments. Deep. Sea. Res. Part II.: Topical. Stud. Oceanogr. 88–89, 1–6. doi: 10.1016/j.dsr2.2012.10.005
Fedak M. A., Lovell P., Grant S. M. (2001). Two Approaches to Compressing and Interpreting Time-Depth Information as Collected by Time-Depth Recorders and Satellite-Linked Data Recorders. Mar. Mammal. Sci. 17 (1), 94–110. doi: 10.1111/j.1748-7692.2001.tb00982.x
Friedlaender A. S., Bowers M. T., Cade D., Hazen E. L., Stimpert A. K., Allen A. N., et al. (2020). The Advantages of Diving Deep: Fin Whales Quadruple Their Energy Intake When Targeting Deep Krill Patches. Funct. Ecol. 34 (2), 497–506. doi: 10.1111/1365-2435.13471
Friedlaender A. S., Goldbogen J. A., Hazen E. L., Calambokidis J., Southall B. L. (2015). Feeding performance by sympatric blue and fin whales exploiting a common prey resource. Marine Mammal Science, 311, 345-354. https://doi.org/10.1111/mms.12134
Friedlaender A., Hazen E., Nowacek D., Halpin P., Ware C., Weinrich M., et al. (2009). Diel Changes in Humpback Whale Megaptera Novaeangliae Feeding Behavior in Response to Sand Lance Ammodytes Spp. Behavior and Distribution. Mar. Ecol. Prog. Ser. 395, 91–100. doi: 10.3354/meps08003
Friedlaender A. S., Johnston D. W., Tyson R. B., Kaltenberg A., Goldbogen J. A., Stimpert A. K., et al. (2016). Multiple-Stage Decisions in a Marine Central-Place Forager. R. Soc. Open Sci. 3 (5), 160043. doi: 10.1098/rsos.160043
Friedlaender A., Tyson R., Stimpert A., Read A., Nowacek D. (2013). Extreme Diel Variation in the Feeding Behavior of Humpback Whales Along the Western Antarctic Peninsula During Autumn. Mar. Ecol. Prog. Ser. 494, 281–289. doi: 10.3354/meps10541
Geijer C. K. A., Notarbartolo di Sciara G., Panigada S. (2016). Mysticete Migration Revisited: Are Mediterranean Fin Whales an Anomaly? Mammal. Rev. 46 (4), 284–296. doi: 10.1111/mam.12069
Goldbogen J. A., Calambokidis J., Croll D. A., McKenna M. F., Oleson E., Potvin J., et al. (2012). Scaling of Lunge-Feeding Performance in Rorqual Whales: Mass-Specific Energy Expenditure Increases With Body Size and Progressively Limits Diving Capacity: Scaling of Lunge-Feeding Energetic in Rorqual Whales. Funct. Ecol. 26 (1), 216–226. doi: 10.1111/j.1365-2435.2011.01905.x
Goldbogen J. A., Calambokidis J., Oleson E., Potvin J., Pyenson N. D., Schorr G., et al. (2011). Mechanics, Hydrodynamics and Energetics of Blue Whale Lunge Feeding: Efficiency Dependence on Krill Density. J. Exp. Biol. 214 (1), 131–146. doi: 10.1242/jeb.048157
Goldbogen J. A., Calambokidis J., Shadwick R. E., Oleson E. M., McDonald M. A., Hildebrand J. A. (2006). Kinematics of Foraging Dives and Lunge-Feeding in Fin Whales. J. Exp. Biol. 209 (7), 1231–1244. doi: 10.1242/jeb.02135
Goldbogen J. A., Friedlaender A. S., Calambokidis J., McKenna M. F., Simon M., Nowacek D. P. (2013). Integrative Approaches to the Study of Baleen Whale Diving Behavior, Feeding Performance, and Foraging Ecology. BioScience 63 (2), 90–100. doi: 10.1525/bio.2013.63.2.5
Goldbogen J. A., Hazen E. L., Friedlaender A. S., Calambokidis J., DeRuiter S. L., Stimpert A. K., et al. (2015). Prey Density and Distribution Drive the Three-Dimensional Foraging Strategies of the Largest Filter Feeder. Funct. Ecol. 29 (7), 951–961. doi: 10.1111/1365-2435.12395
Guazzo R. A., Durbach I. N., Helble T. A., Alongi G. C., Martin C. R., Martin S. W., et al. (2021). Singing Fin Whale Swimming Behavior in the Central North Pacific. Front. Mar. Sci. 8. doi: 10.3389/fmars.2021.696002
Hazen E. L., Friedlaender A. S., Goldbogen J. A. (2015). Blue Whales (Balaenoptera Musculus) Optimize Foraging Efficiency by Balancing Oxygen Use and Energy Gain as a Function of Prey Density. Sci. Adv. 1 (9), e1500469. doi: 10.1126/sciadv.1500469
Hazen E., Friedlaender A., Thompson M., Ware C., Weinrich M., Halpin P., et al. (2009). Fine-Scale Prey Aggregations and Foraging Ecology of Humpback Whales Megaptera Novaeangliae. Mar. Ecol. Prog. Ser. 395, 75–89. doi: 10.3354/meps08108
Heide-Jørgensen M., Laidre K. L., Nielsen N. H., Hansen R. G., Røstad A. (2013). Winter and Spring Diving Behavior of Bowhead Whales Relative to Prey. Anim. Biotelem. 1 (1), 15. doi: 10.1186/2050-3385-1-15
Hennig C. (2014). Fpc: flexible procedures for clustering, Version, 2. 2-9. Available: https://cran.r-project.org/web/packages/fpc/fpc.pdf.
Illius A. W., Tolkamp B. J., Yearsley J. (2002). The Evolution of the Control of Food Intake. Proc. Nutr. Soc. 61 (4), 465–472. doi: 10.1079/PNS2002179
Irvine L., Palacios D. M., Urbán J., Mate B. (2017). Sperm Whale Dive Behavior Characteristics Derived From Intermediate-Duration Archival Tag Data. Ecol. Evol. 7 (19), 7822–7837. doi: 10.1002/ece3.3322
Izadi S., Johnson M., de Soto N. A., Constantine R. (2018). Night-Life of Bryde’s Whales: Ecological Implications of Resting in a Baleen Whale. Behav. Ecol. Sociobiol. 72 (5), 78. doi: 10.1007/s00265-018-2492-8
Jönsson K. I. (1997). Capital and Income Breeding as Alternative Tactics of Resource Use in Reproduction. Oikos 78 (1), 57. doi: 10.2307/3545800
Keen E. M., Scales K. L., Rone B. K., Hazen E. L., Falcone E. A., Schorr G. S. (2019). Night and Day: Diel Differences in Ship Strike Risk for Fin Whales (Balaenoptera Physalus) in the California Current System. Front. Mar. Sci. 6. doi: 10.3389/fmars.2019.00730
Le Boeuf B. J., Costa D. P., Huntley A. C., Feldkamp S. D. (1988). Continuous, Deep Diving in Female Northern Elephant Seals, Mirounga Angustirostris. Can. J. Zoology. 66 (2), 446–458. doi: 10.1139/z88-064
Lockyer C. H. (1987). “Evaluation of the Role of Fat Reserves in Relation to the Ecology of North Atlantic Fin and Sei Whales,” in Approaches to Marine Mammal Energetics. Eds. Huntley A. C., Costa D. P., Worthy G. A. J., Castellini M. A. (Lawrence, KS: Special Publication Number 1, The Society for Marine Mammalogy), 183–203.
Luque S. P. (2007). Diving Behaviour Analysis in R: An Introduction to the Divemove Package. Rnews. 7, 8–14.
Marra P. P., Cohen E. B., Loss S. R., Rutter J. E., Tonra C. M. (2015). A Call for Full Annual Cycle Research in Animal Ecology. Biol. Lett. 11 (8), 20150552. doi: 10.1098/rsbl.2015.0552
Martin A. R., Smith T. G., Cox O. P. (1998). Dive Form and Function in Belugas Delphinapterus Leucas of the Eastern Canadian High Arctic. Polar. Biol. 20 (3), 218–228. doi: 10.1007/s003000050299
Mauchline J., Fisher L. R. (1967). The Distribution of the Euphausiid Crustacean Meganyctiphanes Norvegica. Ser. Atlas. Mar. Environ. 13, 2.
McGarigal K., Cushman S. A., Stafford S. (2000). Multivariate Statistics for Wildlife and Ecology Research (New York, New York, USA: Springer Verlag).
McIntyre T., Ansorge I., Bornemann H., Plötz J., Tosh C., Bester M. (2011). Elephant Seal Dive Behaviour is Influenced by Ocean Temperature: Implications for Climate Change Impacts on an Ocean Predator. Mar. Ecol. Prog. Ser. 441, 257–272. doi: 10.3354/meps09383
McKenna M. F., Calambokidis J., Oleson E. M., Laist D. W., Golbogen J. A. (2015). Simultaneous Tracking of Blue Whales and Large Ships Demonstrates Limited Behavioral Responses for Avoiding Collision. Endangered. Species. Res. 27, 219–232. doi: 10.3354/esr00666
Miller C., Reeb D., Best P., Knowlton A., Brown M., Moore M. (2011). Blubber Thickness in Right Whales Eubalaena Glacialis and Eubalaena Australis Related With Reproduction, Life History Status and Prey Abundance. Mar. Ecol. Prog. Ser. 438, 267–283. doi: 10.3354/meps09174
Oftedal O. T. (2000). Use of Maternal Reserves as a Lactation Strategy in Large Mammals. Proc. Nutr. Soc. 59 (1), 99–106. doi: 10.1017/S0029665100000124
Oleson E., Calambokidis J., Burgess W., McDonald M., LeDuc C., Hildebrand J. (2007). Behavioral Context of Call Production by Eastern North Pacific Blue Whales. Mar. Ecol. Prog. Ser. 330, 269–284. doi: 10.3354/meps330269
Owen K., Jenner C. S., Jenner M.-N. M., Andrews R. D. (2016). A Week in the Life of a Pygmy Blue Whale: Migratory Dive Depth Overlaps With Large Vessel Drafts. Anim. Biotelem. 4 (1), 17. doi: 10.1186/s40317-016-0109-4
Panigada S., Donovan G. P., Druon J.-N., Lauriano G., Pierantonio N., Pirotta E., et al. (2017). Satellite Tagging of Mediterranean Fin Whales: Working Towards the Identification of Critical Habitats and the Focussing of Mitigation Measures. Sci. Rep. 7 (1), 3365. doi: 10.1038/s41598-017-03560-9
Panigada S., Zanardelli M., Canese S., Jahoda M. (1999). How Deep can Baleen Whales Dive? Mar. Ecol. Prog. Ser. 187, 309–311. doi: 10.3354/meps187309
Pérez-Jorge S., Tobeña M., Prieto R., Vandeperre F., Calmettes B., Lehodey P., et al. (2020). Environmental Drivers of Large-Scale Movements of Baleen Whales in the Mid-North Atlantic Ocean. Diversity Distributions. 26 (6), 683–698. doi: 10.1111/ddi.13038
Pirotta E., Mangel M., Costa D. P., Mate B., Goldbogen J. A., Palacios D. M., et al. (2018). A Dynamic State Model of Migratory Behavior and Physiology to Assess the Consequences of Environmental Variation and Anthropogenic Disturbance on Marine Vertebrates. Am. Nat. 191 (2), E40–E56. doi: 10.1086/695135
Potvin J., Goldbogen J. A., Shadwick R. E. (2010). Scaling of Lunge Feeding in Rorqual Whales: An Integrated Model of Engulfment Duration. J. Theor. Biol. 267 (3), 437–453. doi: 10.1016/j.jtbi.2010.08.026
Prieto R., Tobeña M., Silva M. A. (2017). Habitat Preferences of Baleen Whales in a Mid-Latitude Habitat. Deep. Sea. Res. Part II.: Topical. Stud. Oceanogr. 141, 155–167. doi: 10.1016/j.dsr2.2016.07.015
Ramasco V. (2008). Habitat Use and Feeding Behaviour of Harbour Seals (Phoca Vitulina) in Vesterålen, Vol. 62.
Ramasco V. (2008). Habitat use and feeding behaviour of harbour seals (Phoca vitulina) in 678 Vesterålen [master’s thesis]. Tromsø, Norway: The Arctic University of Norway
Rauter C. M., Moore A. J. (2004). Time Constraints and Trade-Offs Among Parental Care Behaviours: Effects of Brood Size, Sex and Loss of Mate. Anim. Behav. 68 (4), 695–702. doi: 10.1016/j.anbehav.2003.09.018
Raymont J. (1983). Plankton and Productivity in the Oceans. 2 (Oxford: Zooplankton. Pergamon Press).
R Core Team (2021). R: A Language and Environment for Statistical Computing (Vienna, Austria: R Foundation for Statistical Computing). Available at: https://www.R-project.org/.
Romagosa M., Baumgartner M., Cascão I., Lammers M. O., Marques T. A., Santos R. S., et al. (2020). Baleen Whale Acoustic Presence and Behaviour at a Mid-Atlantic Migratory Habitat, the Azores Archipelago. Sci. Rep. 10 (1), 4766. doi: 10.1038/s41598-020-61849-8
Romagosa M., Pérez-Jorge S., Cascão I., Mouriño H., Lehodey P., Pereira A., et al. (2021). Food Talk: 40-Hz Fin Whale Calls are Associated With Prey Biomass. Proc. R. Soc. B.: Biol. Sci. 288 (1954), 20211156. doi: 10.1098/rspb.2021.1156
Schreer J. F., Kovacs K. M., Hines R. J. O. (2001). Comparative Diving Patterns of Pinnipeds and Seabirds. Ecol. Monogr. 71 (1), 26. doi: 10.1890/0012-9615(2001)071[0137:CDPOPA]2.0.CO;2
Sigurjónsson J., Víkingsson G. (1997). Seasonal Abundance of and Estimated Food Consumption by Cetaceans in Icelandic and Adjacent Waters. J. Northwest. Atlantic. Fish. Sci. 22, 271–287. doi: 10.2960/J.v22.a20
Sikes R. S., Gannon W. L. (2011). Guidelines of the American Society of Mammalogists for the Use of Wild Mammals in Research. J. Mammal. 92 (1), 235–253. doi: 10.1644/10-MAMM-F-355.1
Silva M. A., Borrell A., Prieto R., Gauffier P., Bérubé M., Palsbøl P. J., et al. (2019). Stable Isotopes Reveal Winter Feeding in Different Habitats in Blue, Fin and Sei Whales Migrating Through the Azores. R. Soc. Open Sci. 6 (8), 181800. doi: 10.1098/rsos.181800
Silva M. A., Prieto R., Cascão I., Seabra M. I., Machete M., Baumgartner M. F., et al. (2014). Spatial and Temporal Distribution of Cetaceans in the Mid-Atlantic Waters Around the Azores. Mar. Biol. Res. 10 (2), 123–137. doi: 10.1080/17451000.2013.793814
Silva M. A., Prieto R., Jonsen I., Baumgartner M. F., Santos R. S. (2013). North Atlantic Blue and Fin Whales Suspend Their Spring Migration to Forage in Middle Latitudes: Building Up Energy Reserves for the Journey? PloS One 8 (10), e76507. doi: 10.1371/journal.pone.0076507
Simon M., Johnson M., Madsen P. T. (2012). Keeping Momentum With a Mouthful of Water: Behavior and Kinematics of Humpback Whale Lunge Feeding. J. Exp. Biol. 215 (21), 3786–3798. doi: 10.1242/jeb.071092
Stephens P. A., Boyd I. L., McNamara J. M., Houston A. I. (2009). Capital Breeding and Income Breeding: Their Meaning, Measurement, and Worth. Ecology 90 (8), 2057–2067. doi: 10.1890/08-1369.1
Stern S. J. (2009). Migration and Movement Patterns. Em. Encyclopedia. Mar. Mammals., 726–730). doi: 10.1016/B978-0-12-373553-9.00168-1
Stimpert A. K., DeRuiter S. L., Falcone E. A., Joseph J., Douglas A. B., Moretti D. J., et al. (2015). Sound Production and Associated Behavior of Tagged Fin Whales (Balaenoptera Physalus) in the Southern California Bight. Anim. Biotelem. 3 (1), 23. doi: 10.1186/s40317-015-0058-3
Sweeney D. A., DeRuiter S. L., McNamara-Oh Y. J., Marques T. A., Arranz P., Calambokidis J. (2019). Automated Peak Detection Method for Behavioral Event Identification: Detecting Balaenoptera Musculus and Grampus Griseus Feeding Attempts. Anim. Biotelem. 7 (1), 7. doi: 10.1186/s40317-019-0169-3
Thompson D., Fedak M. A. (2001). How Long Should a Dive Last? A Simple Model of Foraging Decisions by Breath-Hold Divers in a Patchy Environment. Anim. Behav. 61 (2), 287–296. doi: 10.1006/anbe.2000.1539
Thums M., Bradshaw C. J. A., Hindell M. A. (2008). A Validated Approach for Supervised Dive Classification in Diving Vertebrates. J. Exp. Mar. Biol. Ecol. 363 (1–2), 75–83. doi: 10.1016/j.jembe.2008.06.024
Tsujii K., Otsuki M., Akamatsu T., Matsuo I., Kitamura M., Kikuchi T., et al. (2016). Migration Monitoring of Fin Whales in the Southern Chukchi Sea With Acoustic Methods During 2012–2015. 2016. Techno-Ocean. (Techno-Ocean)., 49–53. doi: 10.1109/Techno-Ocean.2016.7890746
van der Hoop J., Nowacek D., Moore M., Triantafyllou M. (2017). Swimming Kinematics and Efficiency of Entangled North Atlantic Right Whales. Endangered. Species. Res. 32, 1–17. doi: 10.3354/esr00781
Visser F., Hartman K., Pierce G., Valavanis V., Huisman J. (2011). Timing of Migratory Baleen Whales at the Azores in Relation to the North Atlantic Spring Bloom. Mar. Ecol. Prog. Ser. 440, 267–279. doi: 10.3354/meps09349
Ware C., Friedlaender A. S., Nowacek D. P. (2011). Shallow and Deep Lunge Feeding of Humpback Whales in Fjords of the West Antarctic Peninsula. Mar. Mammal. Sci. 27 (3), 587–605. doi: 10.1111/j.1748-7692.2010.00427.x
Watkins W. A., Tyack P., Moore K. E., Bird J. E. (1987). The 20-Hz Signals of Finback Whales (Balaenoptera Physalus). J. Acoustical. Soc. America 82 (6), 1901–1912. doi: 10.1121/1.395685
Williams R., Vikingsson G. A., Gislason A., Lockyer C., New L., Thomas L., et al. (2013). Evidence for Density-Dependent Changes in Body Condition and Pregnancy Rate of North Atlantic Fin Whales Over Four Decades of Varying Environmental Conditions. ICES. J. Mar. Sci. 70 (6), 1273–1280. doi: 10.1093/icesjms/fst059
Witteveen B. H., Foy R. J., Wynne K. M., Tremblay Y. (2008). Investigation of Foraging Habits and Prey Selection by Humpback Whales (Megaptera Novaeangliae) Using Acoustic Tags and Concurrent Fish Surveys. Mar. Mammal. Sci. 24 (3), 516–534. doi: 10.1111/j.1748-7692.2008.00193.x
Wood S. N. (2011). Fast Stable Restricted Maximum Likelihood and Marginal Likelihood Estimation of Semiparametric Generalized Linear Models: Estimation of Semiparametric Generalized Linear Models. J. R. Stat. Society.: Ser. B. (Statistical. Methodology). 73 (1), 3–36. doi: 10.1111/j.1467-9868.2010.00749.x
Wu Z. (2005). Generalized Linear Models in Family Studies. J Marriage Fam. 67, 1029–1047. doi: 10.1111/j.1741-3737.2005.00192.x
Keywords: balaenoptera physalus, migration, baleen whales, time-depth recorders, dive classification, diving behavior, time-activity budget, foraging
Citation: Fonseca CT, Pérez-Jorge S, Prieto R, Oliveira C, Tobeña M, Scheffer A and Silva MA (2022) Dive Behavior and Activity Patterns of Fin Whales in a Migratory Habitat. Front. Mar. Sci. 9:875731. doi: 10.3389/fmars.2022.875731
Received: 14 February 2022; Accepted: 06 June 2022;
Published: 14 July 2022.
Edited by:
Jorge M. Pereira, University of Coimbra, PortugalReviewed by:
Ladd M. Irvine, Oregon State University, United StatesCopyright © 2022 Fonseca, Pérez-Jorge, Prieto, Oliveira, Tobeña, Scheffer and Silva. This is an open-access article distributed under the terms of the Creative Commons Attribution License (CC BY). The use, distribution or reproduction in other forums is permitted, provided the original author(s) and the copyright owner(s) are credited and that the original publication in this journal is cited, in accordance with accepted academic practice. No use, distribution or reproduction is permitted which does not comply with these terms.
*Correspondence: Catarina T. Fonseca, Y2F0YXJpbmF0b3NjYW5vNEBnbWFpbC5jb20=
ORCID: †Catarina T. Fonseca, orcid.org/0000-0001-8702-2584
Sergi Pérez-Jorge, orcid.org/0000-0002-4843-0443
Rui Prieto, orcid.org/0000-0002-0354-2572
Marta Tobeña, orcid.org/0000-0001-9976-7962
Cláudia Oliveira, orcid.org/0000-0002-3590-2915
Mónica A. Silva, orcid.org/0000-0002-2683-309X
Disclaimer: All claims expressed in this article are solely those of the authors and do not necessarily represent those of their affiliated organizations, or those of the publisher, the editors and the reviewers. Any product that may be evaluated in this article or claim that may be made by its manufacturer is not guaranteed or endorsed by the publisher.
Research integrity at Frontiers
Learn more about the work of our research integrity team to safeguard the quality of each article we publish.