- Australian Antarctic Program Partnership, Institute for Marine & Antarctic Studies, University of Tasmania, Hobart, TAS, Australia
A newly developed protocol to semi-automate egg counting in Southern Ocean shelled (thecosome) pteropods using image analysis software and machine learning algorithms was developed and tested for accuracy. Preserved thecosome pteropod (Limacina helicina antarctica) egg masses collected from two austral summer research voyages in East Antarctica were digitally photographed to develop a streamlined approach to enumerate eggs within egg masses using Fiji/ImageJ and the associated machine learning plugin known as Trainable Weka Segmentation. Results from this semi-automated approach were then used to compare with manual egg counts from eggs dissected from egg masses under stereomicroscope. A statistically significant correlation was observed between manual and semi-automated approaches (R2 = 0.92, p < 0.05). There was no significant difference between manual and automated protocols when egg counts were divided by the egg mass areas (mm2) (t(29.6) = 1.98, p = 0.06). However, the average time to conduct semi-automated counts (M = 7.4, SD = 1.2) was significantly less than that for the manual enumeration technique (M = 35.9, SD = 5.7; t(30) = 2.042, p < 0.05). This new approach is promising and, unlike manual enumeration, could allow specimens to remain intact for use in live culturing experiments. Despite some limitations that are discussed, this user-friendly and simplistic protocol can provide the basis for further future development, including the addition of macro scripts to improve reproducibility and through the association with other imaging platforms to enhance interoperability. Furthermore, egg counting using this technique may lead to a relatively unexplored monitoring tool to better understand the responses of a species highly sensitive to multiple stressors connected to climate change.
Introduction
It is now widely recognized that a multitude of concurrent biological, chemical and physical stressors caused by human activities are posing significant threats to global marine ecosystems and their components (IPCC, 2022). In polar regions, research has shown that changes to the development and reproduction of many marine organisms, including zooplankton, are particularly vulnerable to warming and ocean acidification (Johnston et al., 2022). Some zooplanktonic groups, such as gastropod molluscs known as thecosome (shelled) pteropods, are regarded as early responders to climate change (Bednaršek et al., 2016), as they produce fragile, aragonite shells that are highly susceptible to dissolution linked to high CO2 partial pressures (pCO2) due to increasing ocean acidification (Riebesell et al., 2000; Orr et al., 2005; Kroeker et al., 2013). Whilst recent studies have shown a relatively higher capacity to withstand such effects than previously assumed (Peck et al., 2018), it is the early developmental stages of thecosome pteropods that are at greatest risk to changing ocean chemistry (Gardner et al., 2018). These risks will undoubtedly present wider consequences throughout marine ecosystems as, like many zooplankton taxa, thecosome pteropods provide a key energetic link between basal and higher trophic levels as well as an important contributor to the global export of carbon and carbonate to the deep sea through the fluxing of fast-sinking fecal pellets and shells post-mortem (Manno et al., 2010; Manno et al., 2018).
Shell dissolution in thecosome pteropods has often been studied using the common species Limacina helicina from the Northern Hemisphere (Comeau et al., 2010; Lischka et al., 2011; Comeau et al., 2012b; Bednaršek et al., 2014), and its Southern Ocean congener species, Limacina helicina antarctica (Manno et al., 2007; Seibel et al., 2012; Johnson and Hofmann, 2017; Gardner et al., 2018). Whilst one study by Bednaršek et al. (2012) revealed in situ shell dissolution of juvenile L. h. antarctica from the Scotia Sea, situated in the Atlantic sector of the Southern Ocean, much of the effects of climate change on early life development of thecosome pteropods have been observed through laboratory-based manipulation experiments. Examined under predicted levels of ocean acidification and warming, incubated thecosome pteropods have shown a range of adverse responses, including degradation, reduction in and/or lack of shell development (Lischka et al., 2011; Comeau et al., 2012a; Gardner et al., 2018), increased larval mortality (Lischka et al., 2011; Thabet et al., 2015; Gardner et al., 2018), and a decrease in the proportion of eggs developing to advanced embryogenetic stages (Manno et al., 2016). These responses are bound to have wider ecological and long-term ramifications related to population stability and recruitment.
Thecosome pteropods are holoplanktonic with unique life history strategies. Most species begin life as males until they reach a particular size (e.g., shell diameter of ~4 mm for L. helicina) then subsequently develop female organs and mature into females whilst their male organs are resorbed (Lalli and Wells, 1978; Lalli and Gilmer, 1989), which characterizes them as protandrous hermaphrodites. Females spawn tens of thousands of transparent eggs during their lifespan which are embedded into ribbons within gel matrix egg masses. Embryogenetic development occurs within these clutches, and hatching generally occurs at the trochophore larval stage (Lalli and Gilmer, 1989; Thabet et al., 2015; Wakabayashi, 2017). Optimal clutch size theory posits that mature females will spawn variable numbers of eggs to maximize the offspring fitness as it relates to resource availability, intraspecific competition, and mortality (Godfray et al., 1991). Different forms of parental care exist in marine gastropods, but for many species, females control the number of eggs contained within egg masses in an effort to manage their fecundity under changing conditions (Spight and Emlen, 1976; Perron, 1981).
Challenges related to estimating fecundity in thecosome pteropods can be attributed to the high number of microscopic eggs embedded within each egg mass. Manually counting them can be time consuming and using abundance of mature-aged adults is a relatively inaccurate alternative given the range of egg masses released by each pteropod adult. Manually counting thecosome eggs has previously involved dissecting the egg ribbons from the egg mass, which may introduce stress, particularly if eggs are being placed in live culture for subsequent observational studies (Manno et al., 2016). One study by Lalli and Wells (1978) used a conversion factor of 35 eggs mm-2 for L. helicina egg masses collected from Eastern Canada which is derived from estimating the number of eggs per area of egg mass measured, however this average value was based on complete measurements taken from only five egg masses. These challenges may be minimized with the use of image analyses platforms.
Autonomous image analysis techniques have previously been tested in plankton research involving the counting and measuring of round objects in aqueous solution, including the use of images and on-board, large-volume samples (Gorsky et al., 1989; Colas et al., 2018). Several studies have employed software platforms to automatically enumerate microscopic eggs of invertebrates from images with high degrees of success (Collin, 2010; Rosati et al., 2015; da Silva Júnior et al., 2018). The purpose of this study is to develop and validate a workflow that uses a combination of image segmentation and a supervised machine learning algorithmic approach to perform semi-automatic detection of thecosome pteropod eggs embedded within egg masses. This study aims to efficiently and accurately enumerate thecosome pteropod eggs embedded in their egg masses using the workflow developed in this study, and statistically compare this method to manual egg enumeration, which involves dissection under stereomicroscope. Reliably predicting the number of eggs within thecosome pteropod egg masses through non-destructive data imaging techniques can be beneficial to monitoring the health of marine ecosystems particularly prone to rapid chemical change.
Materials and Workflow Construction
Study Area and Sampling
Plankton sampling was conducted along the East Atlantic region of the Southern Ocean during two separate research voyages (Figure 1). The first was aboard the RV Aurora Australis as part of the Kerguelen Axis (K-Axis) program (January-February 2016) within the southern extent of the Kerguelen Plateau. Sampling for K-Axis spanned a region from 62.7°E to 93.5°E, and 57.6°S to 65.2°S. The second was aboard the TRV Umitaka-maru as part of the 20th Kaiyodai Antarctic Research Expedition (KARE20) program (January 2017) which covered a repeat transect southward along the 110°E longitudinal line.
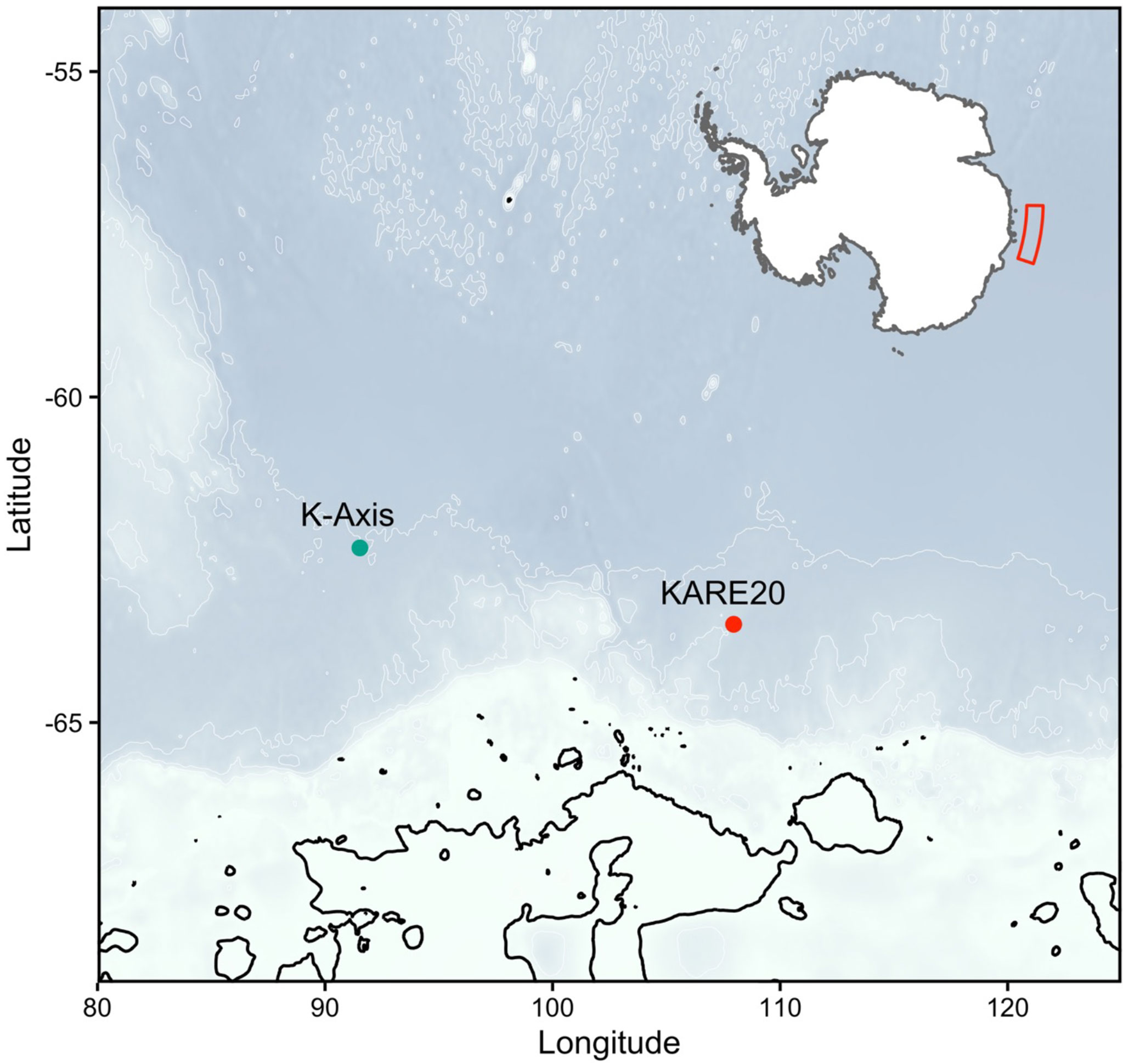
Figure 1 Map of two sampling stations representing two separate research voyages aboard the TRV Umitaka-maru (KARE20) and the RV Aurora Australis (KAXIS), surveyed during the 2017 and 2016 austral summer, respectively.
Mesozooplankton samples from K-Axis were obtained using a Rectangular Midwater Trawl (RMT 1 + 8) net with a mouth area of 8 m2 and a mesh size of 4.5 mm that tapered to a mesh size of 1.5 mm in the last 1.8 m of net [see Hosie et al. (2000) for more details]. Undamaged specimens collected with the RMT1 net, with a mesh size of 315 μm and a mouth area of 1 m2, were measured for this study. Samples from KARE20 were obtained using an Ocean Research Institute (ORI) net with a mouth diameter of 160 cm and a mesh size of 500 μm [see Sakurai et al. (2018); Sakurai et al. (2020) for more details]. Both zooplankton collection methods sampled from a maximum depth of 200 m. All samples were preserved in 5% buffered formaldehyde and seawater solution and transported back to the Institute for Marine and Antarctic Studies in Hobart, Tasmania.
Pteropod egg masses (Figure 2) selected for this study were obtained from two sampling sites determined to have the highest number of intact egg masses, one from each voyage. The sampling site selected from K-Axis was located at -62.318°S and 91.531°E, and the site selected from KARE20 was located 453 nm away at -63.491°S and 107.958°E (Figure 1).
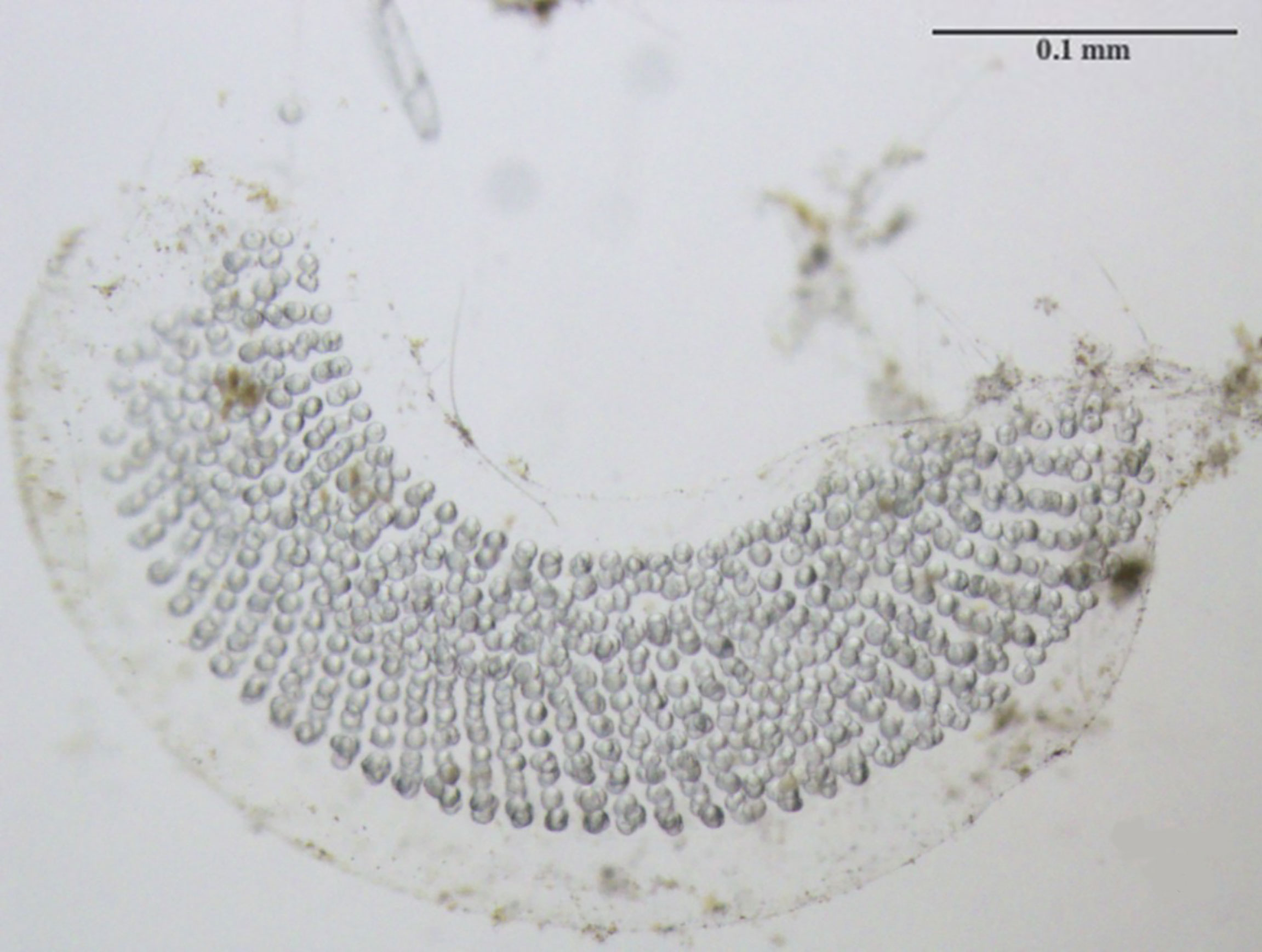
Figure 2 Newly hatched egg mass consisting of hundreds of oval-shaped eggs arranged in a ribbon and embedded within an outer gelatinous matrix.
Manual Counting, Image Capturing, Pre-Processing, Calibration and Threshold Setting
A workflow for image pre-processing, segmenting images and enumerating eggs within thecosome pteropod egg masses is shown in Figure 3. Separated pteropod egg masses (n = 20) were rinsed in filtered seawater and transferred to glass petri dishes in preparation for imaging. Sharpened metal needles were used to gently remove any debris that may affect the segmentation process. Photographs of egg masses were taken with a Canon EOS Mark II 5D camera mounted on a Leica M165 C stereoscopic microscope and using EOS Utility software (Canon USA), while taking note of magnification. For converting measurements from pixels to μm, photographs were also taken of a micrometer slide at the same magnification used for the egg mass images. A selection of images (n = 16) was chosen to include all variations of typically encountered characteristics (e.g., eggs, matrix, phytoplankton cells), and the egg ribbons from the egg masses featured in these images were then carefully dissected under the microscope using a sharpened needle. The eggs from each ribbon were then enumerated to ground truth counts estimated from the automated technique image analysis.
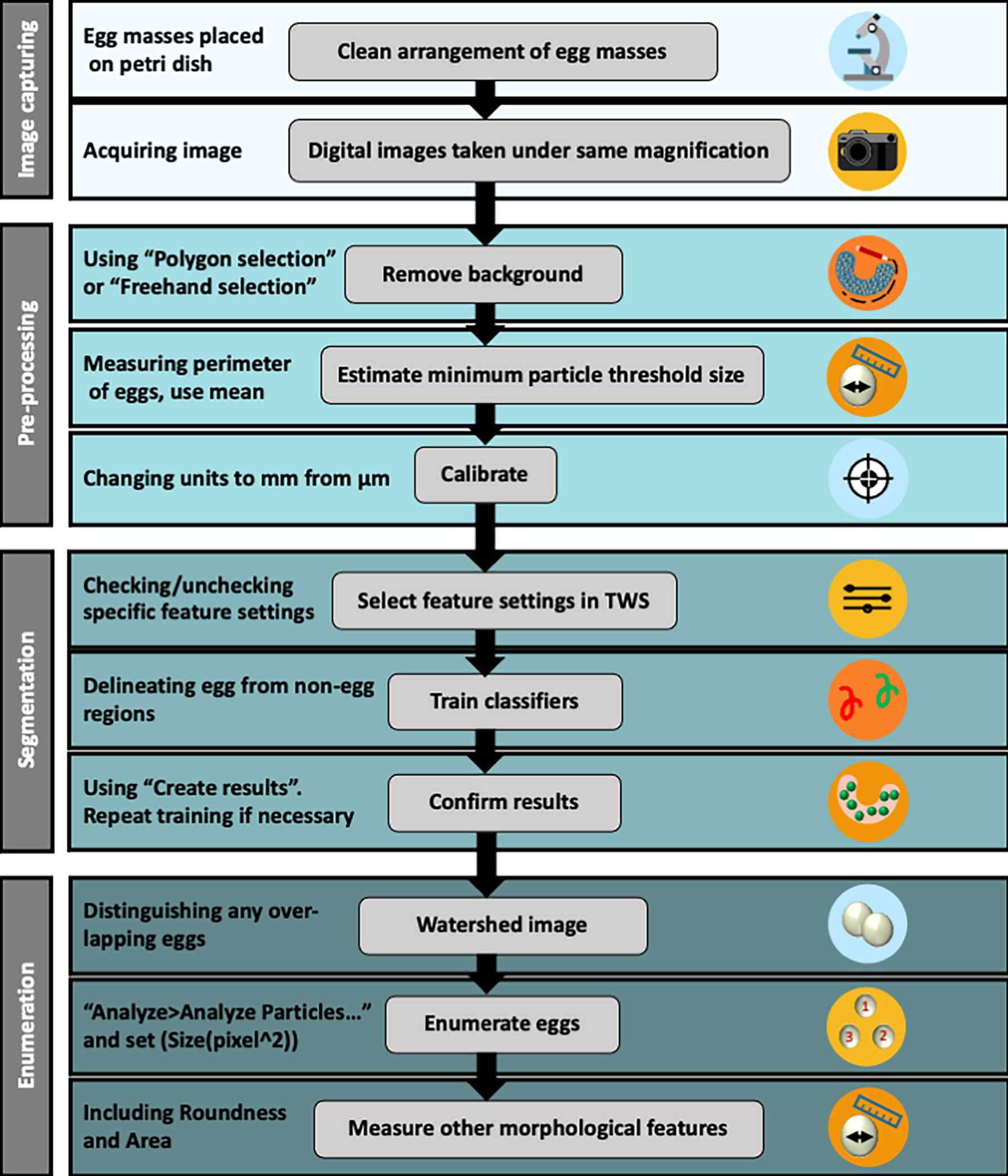
Figure 3 Workflow procedures detailing steps beginning at image capturing, followed by data image pre-processing, segmentation and egg enumeration. See Supplementary Figure 1 for a more detailed version of the workflow procedure.
Each digital image was opened in the Fiji/ImageJ software (RRID : SCR_002285) v. 2.3.1 (Schindelin et al., 2012) and a Wacom Intuos drawing tablet and pen (CTL-6100WL) was used for accurate digital drawing on images. To exclude material around the egg masses, the “Polygon selection” or “Freehand selection” tools from the toolbar were used to draw an overlap around the perimeter of the egg masses (Figure 4A). The “Clear outside” function, located under the Edit drop-down menu, was selected and each image was then saved. To calibrate measurements for each egg mass image, the micrometer slide images were opened first, and the “Straight line” tool from the toolbar was superimposed over the micrometer slide ruler using the “Analyze>Set Scale…” function. A value in pixels was then linked to a known distance value of 1 mm from the line drawn over the slide ruler in the image (spatial calibration value = 1224.06 pixels/mm at 3.2x magnification). A threshold cell size needed to be set due to both the large concentration of non-egg material (e.g., phytoplankton cells) and egg overlapping within the egg masses. To estimate this, the areas (μm2) of a random subset of 20 eggs from four egg mass images were individually measured using the “Polygon selections” tool to draw around the perimeter of each egg and determined to be an average of 10 μm2 from the values in the results table using the “Analyze>Measure” function. Egg mass lengths and areas were also determined using Fiji/ImageJ, using the “Straight line” and “Freehand selection” tools, respectively.
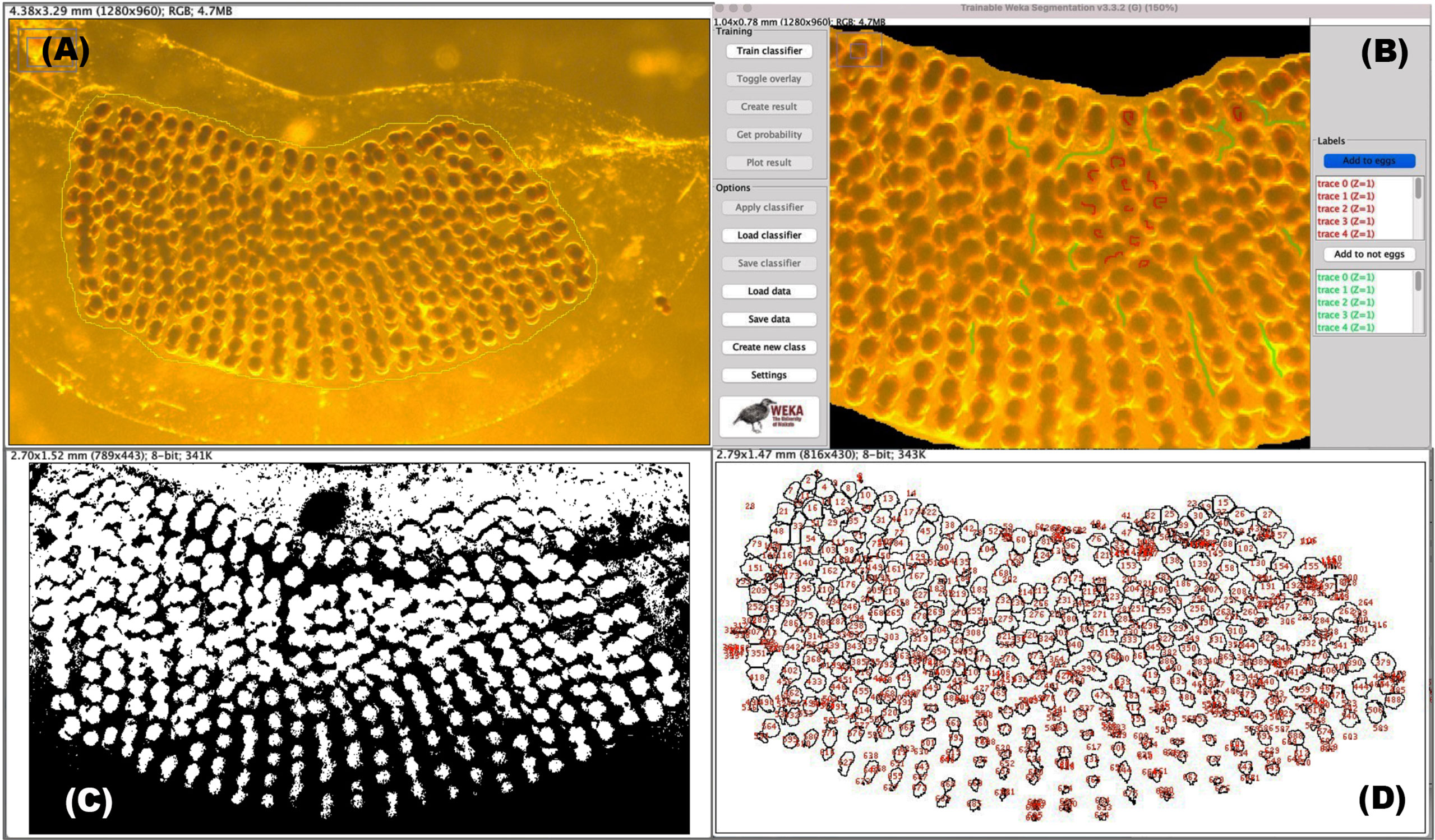
Figure 4 Workflow steps leading to egg enumeration using supervised machine learning. (A) Selection of the area surrounding all eggs by using the “freehand” tool. (B) Supervised training in the TWS graphical user interface corresponding to eggs (default red) and non-egg (default green) regions. (C) Segmentation is first achieved by using a binary image (which is generated by the TWS plugin) prior to employing the watershed function. (D) Selecting “Analyse > Analyse Particles…” gives a results table (not shown) and this image window showing estimations of egg each numbered in red.
Segmentation and Egg Enumeration
For Fiji/ImageJ to perform semi-automated enumeration, the eggs need to be differentiated from the backgrounds within each data image by a process known as segmentation. Built into Fiji/ImageJ is the Trainable Weka Segmentation (TWS; RRID: SCR_001214) plugin (http://imagej.net/Trainable_Weka_Segmentation) which is a tool that leans on machine learning and user-directed guidance to partition digital images into multiple segments, or classifiers, and subsequently perform automatic quantitative segmentation (Arganda-Carreras et al., 2017). Once both the image and TWS (version 3.3.2 was used in this study) are opened, classes were defined and renamed as “eggs” and “not eggs” (Figure 4B; Supplementary Figure 1). Training feature settings will generally depend on the quality of the image. For images taken for this study, Gaussian blur, Hessian, Membrane projections, Sobel filter, Difference of gaussians, Variance, and Structure were selected; Membrane thickness, Membrane patch size and Minimum sigma were kept at default settings, and Maximum sigma was changed to 32.0. The “Freehand” tool from the toolbar was used to mark the regions of each image under each class. For accuracy, a minimum of 10 marks per class was defined, before classifier training began. Training was repeated depending on the quality of the image. The image results from this segmentation workflow were saved and used for the final steps for counting eggs, detailed in the next section.
The binary result images generated from the TWS plugin were opened in Fiji/ImageJ (Figure 4C). Many eggs appeared fused due to the overlapping in regions and the watershed operator was applied to correct for this. The minimum size threshold was set to the previously determined 10 μm2 under Analyze>Analyze Particles … and egg counts were determined from the display results window (Figure 4D). A stopwatch was used to time both manual and semi-automated counting techniques.
Statistical Analyses
All statistical analyses were performed using RStudio (RRID : SCR_000432) version 2021.09.0 (Team, R.C. 2014). A linear regression was performed from a correlation calculated between manual and semi-automated counts; the latter performed through Fiji/ImageJ. Two-sample t-tests were used to statistically compare manual with automated estimations of egg per mass area (mm2) and duration of technique (minutes).
Workflow Assessment Results
Comparing Egg Enumeration Methods
Lengths and areas of pteropod egg masses measured digitally in this study varied between 2.9 mm and 12.2 mm and 4.1 mm2 and 21.4 mm2, respectively (Table 1). The averages calculated for the number of eggs per egg mass lengths were 48.4 eggs mm-2 (± 9.0 SD) for the manual enumeration technique and 41.7 eggs mm-2 (± 10.1 SD) for the semi-automated counting technique with Fiji/ImageJ.
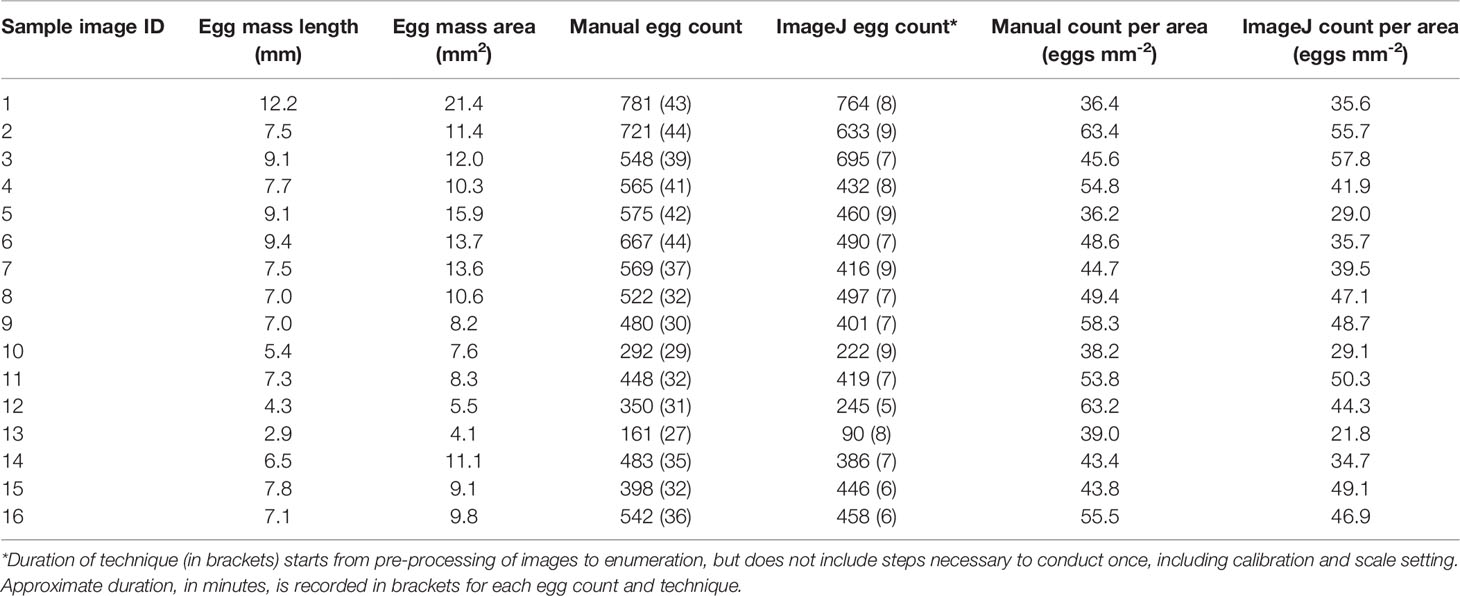
Table 1 Length (mm), area (mm2) and count data of randomly selected pteropod egg masses from manual and semi-automatic techniques.
The comparison of egg counting techniques showed a statistically significant correlation between manual and semi-automated egg counts (R2 = 0.92, p < 0.05; Figure 5). In all but two of the totals, the manual counting technique produced higher egg counts as compared to those conducted digitally. When egg counts were divided by the egg mass areas (mm2), these values were compared between the manual and semi-automated methods and there was no significant difference [t(29.6) = 1.98, p = 0.06; Figure 5 inset plot]. The semi-automated counting technique averaged 7.4 minutes (± 1.2 SD) in duration, which took statistically significantly less time than manual egg counting, which averaged 35.9 minutes (± 5.7 SD) to complete (t(30) = 2.042, p < 0.05).
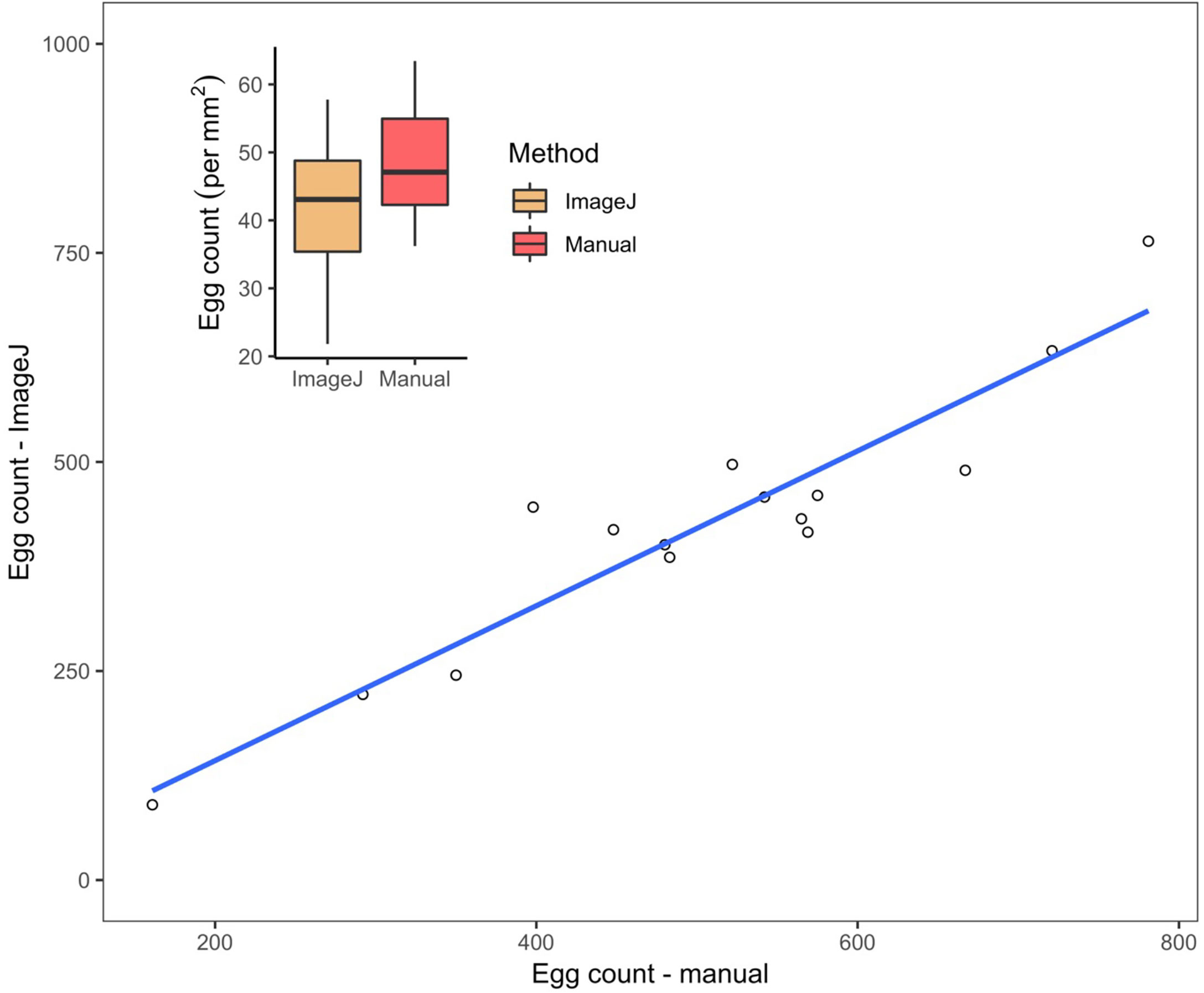
Figure 5 Estimated counts of eggs within L. h. antarctica egg masses (n=16). Linear regression from the correlation calculated between manual and semi-automated counts obtained through Fiji/ImageJ is y = 0.9787x – 55.9883, where y is the predicted number of eggs estimated through automation, and x is manual count variable; R2 = 0.9217, p <0.05. Dashed line is 1:1 reference. Inset plot: Results of L. h. antarctica egg counts mm-2 conducted by automation and manually. Median values of egg counts per egg mass area are depicted by horizontal lines within the 50% interquartiles (boxes). Upper and lower vertical lines, or “whiskers” refer to maximum and minimum dependent values, respectively. No significant difference was observed between methods, p > 0.05.
Limitations and Suggested Improvements
Before discussing the ecological implications associated with the egg counting outputs originating from the Fiji/ImageJ platform, it is critical to determine the reliability of these results. Many of the images depicted egg mass samples surrounded by non-egg particles, such as phytoplankton cells, that would likely also be counted by the software platform. The inability of the platform to distinguish eggs from other materials, identify egg and egg mass abnormalities or differentiate between eggs within close proximity are all limitations of this technique. Precision is enhanced through pre-analysis image preparation, involving setting size threshold limits, and drawing regions of interest (ROIs) encompassing high concentrations of intended materials. Despite these limitations, the statistically significant similarity obtained between manual and automated techniques validate the latter as a suitable solution for developing future studies that estimate fecundity.
Suggested improvements to these limitations should focus on the pre-processing of the egg mass samples prior to imaging. This could be through staining the sample with an agent that would enable the saturation value of eggs to be detected easily from non-egg materials, a step that is often used in medical imaging and histological studies. This was demonstrated by Malhan et al. (2018) who used various stains to distinguish, by color, various elements of connective tissue, including mineralized bone, cartilage, elastic fibers and muscles. Future studies are encouraged require the identification of constituents within and typically adjacent (e.g., phytoplankton, marine snow) to thecosome eggs and egg masses to select the appropriate stain used to separate these constituents by color or other identifier in preparation for pixel-based segmentation. Pre-process staining may eliminate the early workflow steps that focus on manually selecting ROIs and size threshold limits from images as well as decrease machine learning supervision while improving the overall speed and reproducibility of this methodology. Staining, though an extra step in the pre-processing stage, may effectively reduce the inclusion of background noise while also enabling fully automated batch processing of multiple images through scripts.
Other potential improvements to semi-automated egg enumeration involve advanced machine learning strategies, such as deep learning-based methods with a particular focus on the segmentation of nucleus-like shapes and overlapping objects of interest. Deep learning methods have become increasingly popular in recent years, with many applications used in medical research (Hesamian et al., 2019). Emerging deep learning image analysis tools have been developed to address these issues, including the Fiji plugin StarDist, for cell and nuclei detection from images that detects star-convex shaped objects (Weigert et al., 2020), and Cellpose, which is a segmentation algorithm designed to efficiently segment cells stained for a variety of markers (Stringer et al., 2021). Future studies would benefit from testing deep learning algorithms in zooplankton research.
Anticipated Results Using Semi-Automated Egg Enumeration
Measuring the efficiency of a newly constructed, semi-automated egg enumeration technique is difficult when the eggs are microscopic in size, numerous, and the gel matrices in which they are embedded have other particles present, which creates noise, and consequently, potential for error. Therefore, using data retrieved from manual egg enumeration to compare techniques can enable an appropriate assessment of the effectiveness of the semi-automated technique described here. Pteropod egg counts determined accurately and efficiently can then be used to model drivers of both spatial and temporal patterns of early life development and fecundity throughout a rapidly changing Southern Ocean. Egg count data may thus lead to fruitful gains in assembling monitoring programs used to forecast how spatial, ecological and environmental cues affect variability in egg production of a sentinel species in response to increased ocean acidification, deoxygenation and temperatures (Bednaršek et al., 2016; Manno et al., 2017).
Discussion
Shelled pteropods perform essential ecological roles in polar regions and serve as sentinels of climate change, though much work is yet to be done to better understand how these changes affect their early life development. This study constructed a framework to perform automatic, albeit supervised, enumeration of microscopic eggs from thecosome pteropod egg masses using image analysis and machine learning algorithms. Prior to this study, egg counting from thecosome pteropod egg masses had been performed manually under stereomicroscope either through counting eggs along ribbons dissected from each clutch or through estimating the number of eggs over a known area (mm2) then extrapolating this value over the entire length of the egg mass. While the former is more accurate, this method is far more invasive and destructive, whereas the latter method does not account for high variability in egg density present along the length of the egg masses. The purpose of this study was to determine if a digital protocol for egg counting could be as accurate and efficient as the more invasive manual counting method and results here reveal no statistically significant difference between methods. There was a strong correlation found between semi-automatic and manual counting methods.
There are very few published data focused on thecosome pteropod egg numbers and morphology, and how these attributes change over time and under future predicted climate scenarios, though spatio-temporal studies on manually enumerated eggs have been conducted in other marine gastropod species for decades (Berry, 1987; Mandal et al., 2010). However, very few studies have examined gastropod egg number variation along a gradient of environmental factors (Przeslawski, 2014). An accurate egg enumeration workflow has the potential to answer questions pertaining to early life responses of shelled pteropods to climate change, and the application of machine learning within these studies allows for the automation and simplified analyses of large-sized datasets. While only a few studies have counted thecosome eggs for various research purposes, at the time of this study, no other studies have closely analyzed different enumeration techniques for pteropod eggs nor developed an image processing technique incorporating supervised or unsupervised machine learning algorithms. This is the first study to develop and propose a framework to analyze thecosome pteropod eggs digitally using open-source image analysis software and machine learning algorithms.
Digital egg enumeration has advantages over manual counting. Namely, it does not impose damage and potential stress to the individual eggs, thus allowing the eggs and egg masses to be maintained in live cultures for further ontogenetic studies. Images can be captured while the live egg masses are placed in petri dishes or well slides under stereomicroscope, and subsequently available to use for ontogenetic experiments. This can facilitate more research into understanding uncertainties related to early life development of species sensitive to ocean acidification and ocean sea surface temperature change.
There is capacity for improving detection accuracy and speed of operation on the workflow presented here. Firstly, the discovery of a stain that would easily differentiate eggs from non-egg materials would be a fruitful next step. This would require a deeper understanding of the constituents that make up organic and inorganic materials within and adjacent to the egg masses and result in more accurate segmentation by the TWS plugin. Secondly, there are additional Fiji/ImageJ-based plugins and tools that have shown promising results in pre- and postprocessing cell enumeration, including cell staining followed by the in-built Fiji/ImageJ Color Deconvolution plugin for color segmentation (Ruifrok and Johnston, 2001). This research included a single observer, an assessment of variability in results between multiple observers would be recommended to test user-induced bias and standardize steps, beginning with settings (e.g., magnification) and equipment (e.g., microscope and camera make and model) related to the acquisition of digital images. And finally, through the development of new macros with customizable parameters (based on egg roundness, area, diameter, etc.) that would enable batch processing of multiple image files, rendering the process more automatic. The workflow described here can serve as a baseline for future development with new functionality.
In conclusion, the semi-automatic machine learning approach to analyzing pteropod egg mass images developed here is a promising user-friendly, non-destructive, and highly practical methodology for enumerating eggs within their gel matrices. This study outlines a simple, stepwise workflow necessary to accomplish accurate pixel-based segmentation of pteropod egg mass images using the image analysis software, Fiji/ImageJ, and the in-build TWS plugin. The effectiveness of this workflow was shown through a comparative analysis with manual counting requiring dissection of egg ribbons embedded within the egg mass gel matrix under a stereomicroscope that revealed high correlation.
Data Availability Statement
The raw data supporting the conclusions of this article will be made available by the authors, without undue reservation.
Author Contributions
The author confirms all responsibility for study conception and design, sample and data collection, analyses and interpretation of results, and manuscript preparation.
Funding
This study was financially supported through a grant provided by the Holsworth Wildlife Research Endowment (grant #109804) of the Ecological Society of Australia, as well as funding from the Australian Government as part of the Antarctic Science Collaboration Initiative program (grant # ASCI000002). The Australian Antarctic Program Partnership is led by the University of Tasmania, and includes the Australian Antarctic Division, CSIRO Oceans and Atmosphere, Geoscience Australia, the Bureau of Meteorology, the Tasmanian State Government and Australia’s Integrated Marine Observing System.
Conflict of Interest
The author declares that the research was conducted in the absence of any commercial or financial relationships that could be construed as a potential conflict of interest.
Publisher’s Note
All claims expressed in this article are solely those of the authors and do not necessarily represent those of their affiliated organizations, or those of the publisher, the editors and the reviewers. Any product that may be evaluated in this article, or claim that may be made by its manufacturer, is not guaranteed or endorsed by the publisher.
Acknowledgments
This research was made possible through the assistance of those involved in plankton sampling, including the master, crew, scientists and technical support teams aboard both the RV Aurora Australis and TRV Umitaka-maru. I am grateful to the anonymous reviewers who provided constructive feedback that significantly improved the quality of this manuscript.
Supplementary Material
The Supplementary Material for this article can be found online at: https://www.frontiersin.org/articles/10.3389/fmars.2022.869252/full#supplementary-material
References
Arganda-Carreras I., Kaynig V., Rueden C., Eliceiri K. W., Schindelin J., Cardona A., et al. (2017). Trainable Weka Segmentation: A Machine Learning Tool for Microscopy Pixel Classification. Bioinformatics 33, 2424–2426. doi: 10.1093/bioinformatics/btx180
Bednaršek N., Feely R. A., Reum J. C. P., Peterson B., Menkel J., Alin S. R., et al. (2014). Limacina Helicina Shell Dissolution as an Indicator of Declining Habitat Suitability Owing to Ocean Acidification in the California Current Ecosystem. Proc. R. Soc B Biol. Sci. 281, 20140123. doi: 10.1098/rspb.2014.0123
Bednaršek N., Harvey C. J., Kaplan I. C., Feely R. A., Možina J. (2016). Pteropods on the Edge: Cumulative Effects of Ocean Acidification, Warming, and Deoxygenation. Prog. Oceanogr. 145, 1–24. doi: 10.1016/j.pocean.2016.04.002
Bednaršek N., Tarling G. A., Bakker D. C., Fielding S., Cohen A., Kuzirian A., et al. (2012). Description and Quantification of Pteropod Shell Dissolution: A Sensitive Bioindicator of Ocean Acidification. Glob. Change Biol. 18, 2378–2388. doi: 10.1111/j.1365-2486.2012.02668.x
Berry A. J. (1987). Reproductive Cycles, Egg Production and Recruitment in the Indo-Pacific Intertidal Gastropod Umbonium Vestiarium (L.). Estuar. Coast. Shelf Sci. 24, 711–723. doi: 10.1016/0272-7714(87)90109-0
Colas F., Tardivel M., Perchoc J., Lunven M., Forest B., Guyader G., et al. (2018). The ZooCAM, a New in-Flow Imaging System for Fast Onboard Counting, Sizing and Classification of Fish Eggs and Metazooplankton. Prog. Oceanogr. 166, 54–65. doi: 10.1016/j.pocean.2017.10.014
Collin R. (2010). Repeatability of Egg Size in Two Marine Gastropods: Brood Order and Female Size do Not Contribute to Intraspecific Variation. Mar. Ecol. Prog. Ser. 410, 89–96. doi: 10.3354/meps08638
Comeau S., Alliouane S., Gattuso J. P. (2012a). Effects of Ocean Acidification on Overwintering Juvenile Arctic Pteropods Limacina Helicina. Mar. Ecol. Prog. Ser. 456, 279–284. doi: 10.3354/meps09696
Comeau S., Gattuso J.-P., Nisumaa a.-M., Orr J. (2012b). Impact of Aragonite Saturation State Changes on Migratory Pteropods. Proc. R. Soc B Biol. Sci. 279, 732–738. doi: 10.1098/rspb.2011.0910
Comeau S., Jeffree R., Teyssie J. L., Gattuso J. P. (2010). Response of the Arctic Pteropod Limacina Helicina to Projected Future Environmental Conditions. PloS One 5, 1–7. doi: 10.1371/journal.pone.0011362
da Silva Júnior F. A., Monteiro da Silva C., de Almeida F. B., Rodrigues-Silva R. (2018). Digital Image Analysis to Estimate the Minimum Number of Eurytrema Coelomaticum Eggs in the Uterus of Adult Specimens. Helminthologia 55, 204–212. doi: 10.2478/helm-2018-0014
Gardner J., Manno C., Bakker D. C. E., Peck V. L., Tarling G. A. (2018). Southern Ocean Pteropods at Risk From Ocean Warming and Acidification. Mar. Biol. 165, 8. doi: 10.1007/s00227-017-3261-3
Godfray H. C. J., Partridge L., Harvey P. H. (1991). Clutch Size. Annu. Rev. Ecol. Syst. 22, 409–429. doi: 10.1146/annurev.es.22.110191.002205
Gorsky G., Guilbert P., Valenta E. (1989). The Autonomous Image Analyzer - Enumeration, Measurement and Identification of Marine Phytoplankton. Mar. Ecol. Prog. Ser. 58, 133–142. doi: 10.3354/meps058133
Hesamian M. H., Jia W., He X., Kennedy P. (2019). Deep Learning Techniques for Medical Image Segmentation: Achievements and Challenges. J. Digit. Imaging 32, 582–596. doi: 10.1007/s10278-019-00227-x
Hosie G. W., Schultz M. B., Kitchener J. A., Cochran T. G., Richards K. (2000). Macrozooplankton Community Structure Off East Antarctica (80-150°E ) During the Austral Summer of 1995/1996. Deep Sea Res. Part II 47, 2437–2463. doi: 10.1016/S0967-0645(00)00031-X
Hosie G. W., Schultz M. B., Kitchener J. A., Cochran T. G., Richards K. (2000). Macrozooplankton Community Structure Off East Antarctica (80-150°E ) During the Austral Summer of 1995/1996. Deep Sea Res. Part II 47, 2437–2463. doi: 10.1016/S0967-0645(00)00031-X
IPCC. (2022) Climate Change 2022: Impacts, Adaptation, and Vulnerability. Contribution of Working Group II to the Sixth Assessment Report of the Intergovernmental Panel on Climate Change. Eds. Pörtner H. -O., Roberts D. C., Tignor M., Poloczanska E. S., Mintenbeck K., et al. (Cambridge University Press). In Press.
Johnston N. M., Murphy E. J., Atkinson A. A., Constable A. J., Cotté S., Cox M., et al. (2022). Status, Change and Futures of Zooplankton in the Southern Ocean. Front. Mar. Sci 9, 624692. doi: 10.3389/fevo.2021.624692
Kroeker K. J., Kordas R. L., Crim R., Hendriks I. E., Ramajo L., Singh G. S., et al. (2013). Impacts of Ocean Acidification on Marine Organisms: Quantifying Sensitivities and Interaction With Warming. Glob. Change Biol. 19, 1884–1896. doi: 10.1111/gcb.12179
Lalli C. M., Gilmer R. W. (1989). Pelagic Snails: The Biology of Holoplanktonic Gastropod Mollusks (Stanford, CA: Stanford University Press).
Lalli M., Wells F. E. (1978). Reproduction in the Genus Limacina (Opisthobranchia: Thecosomata). J. Zool. London 186, 95–108. doi: 10.1111/j.1469-7998.1978.tb03359.x
Lischka S., Budenbender J., Boxhammer T., Riebesell U. (2011). Impact of Ocean Acidification and Elevated Temperatures on Early Juveniles of the Polar Shelled Pteropod Limacina Helicina: Mortality, Shell Degradation, and Shell Growth. Biogeosciences 8, 919–932. doi: 10.5194/bg-8-919-2011
Malhan D., Muelke M., Rosch S., Schaefer A. B., Merboth F., Weisweiler D., et al. (2018). An Optimized Approach to Perform Bone Histomorphometry. Front. Endocrinol. (Lausanne) 9. doi: 10.3389/fendo.2018.00666
Mandal S., Tamaki A., Ohashi S., Takeuchi S., Agata Y., Takahara Y., et al. (2010). How Newly Recruited Cohorts are Formed in the Trochid Gastropod Population (Umbonium Moniliferum) on an Intertidal Sandflat in Western Kyushu, Japan. J. Exp. Mar. Bio. Ecol. 389, 18–37. doi: 10.1016/j.jembe.2010.04.001
Manno C., Bednaršek N., Tarling G. A., Peck V. L., Comeau S., Adhikari D., et al. (2017). Shelled Pteropods in Peril: Assessing Vulnerability in a High CO2 Ocean. Earth-Sci. Rev 169, 132–145. doi: 10.1016/j.earscirev.2017.04.005
Manno C., Giglio F., Stowasser G., Fielding S., Enderlein P., Tarling G. A. (2018). Threatened Species Drive the Strength of the Carbonate Pump in the Northern Scotia Sea. Nat. Commun. 9, 4592. doi: 10.1038/s41467-018-07088-y
Manno C., Peck V. L., Tarling G. A. (2016). Pteropod Eggs Released at High Pco2 Lack Resilience to Ocean Acidification. Nat. Publ. Gr. 6, 25752. doi: 10.1038/srep25752
Manno C., Sandrini S., Tositti L., Accornero A. (2007). First Stages of Degradation of Limacina Helicina Shells Observed Above the Aragonite Chemical Lysocline in Terra Nova Bay (Antarctica). J. Mar. Syst. 68, 91–102. doi: 10.1016/j.jmarsys.2006.11.002
Manno C., Tirelli V., Accornero A., Fonda Umani S. (2010). Importance of the Contribution of Limacina Helicina Faecal Pellets to the Carbon Pump in Terra Nova Bay (Antarctica). J. Plankton Res. 32, 145–152. doi: 10.1093/plankt/fbp108
Orr J. C., Fabry V. J., Aumont O., Bopp L., Doney S. C., Feely R. A., et al. (2005). Anthropogenic Ocean Acidification Over the Twenty-First Century and its Impact on Calcifying Organisms. Nature 437, 681–686. doi: 10.1038/nature04095
Peck V. L., Oakes R. L., Harper E. M., Manno C., Tarling G. A. (2018). Pteropods Counter Mechanical Damage and Dissolution Through Extensive Shell Repair. Nat. Commun. 9, 264. doi: 10.1038/s41467-017-02692-w
Perron F. E. (1981). The Partitioning of Reproductive Energy Between Ova and Protective Capsules in Marine Gastropods of the Genus Conus. Am. Nat. 118, 110–118. doi: 10.1086/283805
Przeslawski R. (2014). A Review of the Effects of Environmental Stress on Embryonic Development Within Intertidal Gastropod Egg Masses. Molluscan Res. 24, 43–63. doi: 10.1071/MR04001
Riebesell U., Zondervan I., Rost B., Tortell P. D., Zeebe R. E., Morel F. M. (2000). Reduced Calcification of Marine Plankton in Response to Increased Atmospheric Co2. Nature 407, 364–367. doi: 10.1038/35030078
Rosati J. Y., Pacheco V. A., Vankosky M. A., Vanlaerhoven S. L. (2015). Estimating the Number of Eggs in Blow Fly (Diptera: Calliphoridae) Egg Masses Using Photographic Analysis. J. Med. Entomol. 52, 658–662. doi: 10.1093/jme/tjv053
Ruifrok A., Johnston D. (2001). Quantification of Histochemical Staining by Color Deconvolution. Anal. Quantif. Cytol. Histol. Histol. 23, 291–299.
Sakurai H., Moteki M., Mizobata K., Shimada K., Matsuno K., Okubo Y., et al. (2018). Plankton Sampling by the Training Vessel Umitaka-Maru in the Indian Sector of the Indian Sector of the Southern Ocean in the Austral Summer of 2017. JARE Data Rep. 363, 1–13. doi: 10.15094/00014871
Sakurai H., Moteki M., Shimada K., Tachibana A., Yasuda M., Tanaka A., et al. (2020). Japanese Antarctic Research Expedition (JARE) Serial Data. Plankton Sampling by the Training Vessel Umitaka-Maru in the Indian Sector of the Southern Ocean in the Austral Summer of 2020. 366, 1–12. doi: 10.15094/00015897
Schindelin J., Arganda-Carreras I., Frise E., Kaynig V., Longair M., Pietzsch T., et al. (2012). Fiji: An Open-Source Platform for Biological-Image Analysis. Nat. Methods 9, 676–682. doi: 10.1038/nmeth.2019
Seibel B. A., Maas A. E., Dierssen H. M. (2012). Energetic Plasticity Underlies a Variable Response to Ocean Acidification in the Pteropod, Limacina Helicina Antarctica. PloS One 7, e30464. doi: 10.1371/journal.pone.0030464
Spight T. O. M. M., Emlen J. (1976). Clutch Sizes of Two Marine Snails With a Changing Food Supply. Ecology 57, 1162–1178. doi: 10.2307/1935042
Stringer C., Wang T., Michaelos M., Pachitariu M. (2021). Cellpose: A Generalist Algorithm for Cellular Segmentation. Nat. Methods 18, 100–106. doi: 10.1038/s41592-020-01018-x
Team, R. C. (2014) R: A Language and Environment for Statistical Computing. Available at: http://www.r-project.org/.
Thabet A. A., Maas A. E., Lawson G. L., Tarrant A. M. (2015). Life Cycle and Early Development of the Thecosomatous Pteropod Limacina Retroversa in the Gulf of Maine, Including the Effect of Elevated CO2 Levels. Mar. Biol. 162, 2235–2249. doi: 10.1007/s00227-015-2754-1
Wakabayashi K. (2017). Embryonic Development of the Sea Butterfly Desmopterus Papilio (Gastropoda: Thecosomata). Invertebr. Reprod. Dev. 61, 142–146. doi: 10.1080/07924259.2017.1311952
Keywords: egg counting, egg masses, image analysis, machine learning, pteropods, Southern Ocean, thecosomes, zooplankton
Citation: Weldrick CK (2022) A Promising Approach to Quantifying Pteropod Eggs Using Image Analysis and Machine Learning. Front. Mar. Sci. 9:869252. doi: 10.3389/fmars.2022.869252
Received: 04 February 2022; Accepted: 04 April 2022;
Published: 29 April 2022.
Edited by:
Sophie G. Pitois, Centre for Environment, Fisheries and Aquaculture Science (CEFAS), United KingdomReviewed by:
Clara Manno, British Antarctic Survey (BAS), United KingdomDavid Legland, Institut National de recherche pour l’agriculture, l’alimentation et l’environnement (INRAE), France
Copyright © 2022 Weldrick. This is an open-access article distributed under the terms of the Creative Commons Attribution License (CC BY). The use, distribution or reproduction in other forums is permitted, provided the original author(s) and the copyright owner(s) are credited and that the original publication in this journal is cited, in accordance with accepted academic practice. No use, distribution or reproduction is permitted which does not comply with these terms.
*Correspondence: Christine K. Weldrick, christine.weldrick@utas.edu.au