- 1Geospatial Ecology of Marine Megafauna Lab, Marine Mammal Institute, Oregon State University, Newport, OR, United States
- 2Departamento de Ciencias Marinas y Coseras, Universidad Autónoma de Baja California Sur, La Paz, Mexico
- 3Aarhus Institute of Advanced Studies, Aarhus University, Aarhus, Denmark
- 4Zoophysiology, Department of Biology, Aarhus University, Aarhus C, Denmark
- 5Marine Mammal Research Program, Hawaii Institute of Marine Biology, University of Hawai'i at Manoa, Manoa, HI, United States
- 6Florida Institute of Environment, College of Arts, Science & Education, Florida International University, North Miami, FL, United States
- 7Laguna San Ignacio Ecosystem Science Program (LSIESP), Darnestown, MD, United States
- 8University of Washington, Cooperative Institute for Climate, Ocean, and Ecosystem Studies, Seattle, WA, United States
- 9National Oceanic and Atmospheric Administration (NOAA), Alaska Fisheries Science Center, Marine Mammal Laboratory, Seattle, WA, United States
- 10Department of Statistical Science, Duke University, Durham, NC, United States
Climate change is a global phenomenon, yet impacts on resource availability to predators may be spatially and temporally diverse and asynchronous. As capital breeders, whales are dependent on dense, predictable prey resources during foraging seasons. An Unusual Mortality Event (UME) of Eastern North Pacific (ENP) gray whales (Eschrichtius robustus) was declared in 2019 due to a dramatic rise in stranded animals, many emaciated. Climate change impacts may have affected prey availability on the primary foraging grounds of ENP gray whales (~20,000 individuals) in the Arctic and sub-Arctic region and in coastal habitats between northern California, USA and British Columbia, Canada where a small sub-group of ENP whales called the Pacific Coast Feeding Group (PCFG; ~230 individuals) forages. To investigate variability of gray whale body condition relative to changing ocean conditions, we compare two datasets of gray whale aerial photogrammetry images collected via Unoccupied Aircraft Systems (UAS) on the ENP wintering grounds in San Ignacio Lagoon, Mexico (SIL; n=111) and on the PCFG feeding grounds in Oregon, USA (n=72) over the same three-year period (2017–2019). We document concurrent body condition improvement of PCFG whales in Oregon while body condition of whales in SIL declined. This result indicates that the UME may have affected ENP whales due to reduced energetic gain on some Arctic/sub-Arctic foraging grounds, while PCFG whales are recovering from poor prey conditions during the NE Pacific marine heatwave event of 2014–2016. Surprisingly, we found that PCFG whales in Oregon had significantly worse body condition than whales in SIL, even when accounting for year and phenology. We derive support for this unexpected finding via photogrammetry analysis of opportunistic aerial images of gray whales on Arctic foraging grounds (n=18) compared to PCFG whales in Oregon (n=30): the body condition of PCFG whales was significantly lower (t=2.96, p=0.005), which may cause PCFG whales to have reduced reproductive capacity or resilience to environmental perturbations compared to ENP whales. Overall, our study elucidates divergent gray whale body condition across sub-groups and time, and we demonstrate the value of UAS to effectively monitor and identify the physiological response of whales to climate change.
Introduction
A major avenue of climate change impacts on biodiversity is disruptions to availability and predictability of prey resources (Hamilton et al., 2017; Bastille-Rousseau et al., 2018), making the monitoring and metrics of body condition (relative energy reserves) a valuable tool to assess population response and resiliency to climate variability (Jirinec et al., 2021). As capital breeders, whales are particularly vulnerable to changes in prey resource patterns as their phenology requires efficient foraging periods to rapidly gain energetic stores to support reproduction and basal metabolic demands (Jönsson, 1997; Stephens et al., 2014). Declines in availability of crucial prey can lead to reduced body condition within a year (Braithwaite et al., 2015), and potentially reduced reproductive output and eventual death following some threshold of multiple years of low prey (Williams et al., 2013).
Yet, the impacts of climate warming on ecosystem variability are not uniform across space and time, particularly in the marine environment where multiple dynamic forces can delay, compound, disperse, or amplify (Lenoir et al., 2020). If a hypothetical whale population relies on a single foraging ground, individuals in the population should generally display similar body condition responses (excluding the potential influence of demographic unit and individual foraging specializations). However, if the population disperses to multiple foraging grounds and display flexible prey selection, then individuals will likely show variable body condition responses to climate driven ecosystem changes. Therefore, monitoring body condition of whales can inform how different segments of a population respond to climate variation over space and time, revealing non-uniform impacts of climate change.
Over the past decade, the advent and proliferation of Unoccupied Aircraft Systems (UAS) has enabled the safe, rapid, and non-invasive collection of high-resolution photogrammetry datasets to quantify and describe the body condition for multiple species of free-living whales (Johnston, 2019). These data have illustrated seasonal changes across individuals in a population (Christiansen et al., 2016; Durban et al., 2016; Lemos et al., 2020), maternal costs (Christiansen et al., 2018), interannual variability (Lemos et al., 2020; Christiansen et al., 2021), and differences between populations (Christiansen et al., 2020). However, cross population (and cross research lab) comparisons using UAS-based photogrammetry data are rare due to non-standardization of methods and UAS equipment across labs that leads to a lack of interoperability. Yet, methods recently developed by Bierlich et al. (2021b) account for these differences by quantifying uncertainty in photogrammetric measurements, which allows unification and cross-comparison of datasets. Furthermore, we apply the Body Area Index (BAI; Burnett et al., 2018), which has high precision with low uncertainty (Bierlich et al., 2021a), to compare whale body condition.
Application of UAS technology in cetacean studies is flourishing, making cross dataset comparisons a highly feasible and valuable method to identify differences between populations, sub-groups, and life-history stages, and to describe potential drivers of whale body condition variability. During overlapping years (2017 to 2019) separate research programs used UAS to monitor the body condition of gray whales (Eschrichtius robustus) feeding in coastal waters of Oregon, USA during summer and fall months, and on their wintering grounds in San Ignacio Lagoon (SIL) on the west coast of the Baja California Peninsula, Mexico. During this period, an Unusual Mortality Event (UME) of gray whales was declared in January 2019 due to elevated numbers of stranded gray whales along the Pacific coast from northern Mexico through the Arctic region of Alaska, USA. Dead whales were frequently in emaciated body condition, indicating that reduced energetic gain could be a causal factor of death, either due to reduced foraging success, increased energy expenditure, or disease (Christiansen et al., 2021). We utilize these two photogrammetry datasets in a comparative analysis to (1) assess temporal patterns of gray whale body condition between feeding and wintering grounds within the context of variable ocean conditions that controlled prey availability leading up to the UME period, and (2) investigate potential sub-population differences in body condition.
The population size of the gray whales in the North Pacific has undergone significant variation over the past two centuries. Pre-whaling estimates of population size exceed 60,000 individuals (Alter et al., 2007), with a dramatic decline to less than 4,000 individuals by 1900 (Butterworth et al., 2002; Swartz et al., 2006). Through international protection, the Eastern North Pacific (ENP) population has recovered, to a peak of 26,916 (CV = 0.058) in 1987 (Laake et al., 2012). The abundance of the ENP population has continued to fluctuate, including undergoing two UMEs that reduced the population size significantly: After the 1999–2000 UME, the population reduced from 21,135 (CV = 0.068) in 1997 to 16,033 in 2001 (CV = 0.069) (Laake et al., 2012), and during the current 2019-Present UME the population has dropped from 26,960 (95% CI = 24,420–29,830) in 2016 to 20,580 (95% CI = 18,700–22,870) in 2020 (Stewart and Weller, 2021). This variation in abundance implies that gray whales are susceptible to variation in ocean conditions, resource availability, and other impacts, yet are capable of population rebound when conditions are favorable.
The majority of ENP whales migrate annually to foraging grounds in Alaska, targeting benthic amphipods as prey (Moore et al., 2003; Brower et al., 2017). However, a sub-group of 232 (SE = 25.2) ENP gray whales called the Pacific Coast Feeding Group (PCFG) truncates their migration about halfway up the Pacific west coast and forages in coastal habitats between northern California, USA and British Columbia, Canada, including Oregon (Calambokidis et al., 2019). The PCFG has been studied through photo-identification methods since 1980, indicating high residency, site-fidelity, and calf recruitment (Darling et al., 1998; Calambokidis and Pérez, 2017b; Calambokidis et al., 2019). The drivers for the existence of this PCFG sub-group remain unclear, yet caloric analysis of prey items indicates that prey in the PCFG range is of equal or higher value than the main amphipod prey (Ampelisca macrocephala) in the Arctic and sub-Arctic, leading to the hypothesis that greater prey density and/or lower energetic costs of foraging in the Arctic and sub-Arctic may explain the greater number of whales foraging in that region (Hildebrand et al., 2021). Furthermore, although gray whales from both the ENP and PCFG migrate to wintering grounds in the Baja California Peninsula (Rice and Wolman, 1971), the population connectivity between the two groups remains unclear, with mitochondrial DNA comparison showing low genetic exchange between sub-groups and microsatellite markers showing no evidence of reproductive isolation (Frasier et al., 2011; Lang et al., 2014). Gray whales on the wintering grounds in SIL are predominantly from the relatively abundant ENP, yet individuals from the PCFG and the Western North Pacific (WNP) gray whale population also occur in SIL during the winter months (Weller et al., 2012; Mate et al., 2015; Calambokidis and Pérez, 2017a; Urbán et al., 2019). The WNP is an endangered population (2019 abundance estimate = 231 non-calves; Cooke et al., 2019) that forage in far east Russia during summer months with some portion of the population migrating to the Baja California Peninsula lagoons (~20%–55%; Cooke et al., 2019).
Previous analysis of the UAS photogrammetry dataset of PCFG gray whales in Oregon waters demonstrated increasing body condition through the foraging season, variation in body condition by demographic unit, and interannual variation in body condition that was hypothesized to be linked with carryover effects of local oceanographic conditions one year prior (Lemos et al., 2020). A marine heat wave known as “The Blob” affected the oceanography, productivity, and biodiversity in the Pacific northwest from 2014 through 2016 (Peterson et al., 2017; Fewings and Brown, 2019), and may have also impacted PCFG gray whale foraging efficiency (Lemos et al., 2020). Furthermore, the body condition of PCFG gray whales determined by lateral photographs captured between 1996 and 2013 showed an increase across the foraging season, and interannual variation had a significant negative correlation with the Pacific Decadal Oscillation (running average of the two prior years; Akmajian et al., 2021).
The UAS photogrammetry dataset of gray whales on their wintering grounds in SIL has also been analyzed previously, indicating decreasing body condition from 2017 to 2020, which aligns with the onset and duration of the UME (Christiansen et al., 2021). While gray whales from the ENP, PCFG, and WNP all occur on SIL wintering grounds, ENP whales likely dominate the UAS photogrammetry dataset collected in SIL based on their considerably higher population abundance. Therefore, we assume that the SIL dataset reflects the body condition of ENP gray whales that feed in Arctic and sub-Arctic foraging grounds, including Kodiak Island in the Gulf of Alaska, along the Aleutian Chain, in the Chirikov Basin, and the northern Bering and Chukchi seas (Braham et al., 1984; Moore et al., 2003; Coyle et al., 2007; Moore et al., 2007; Brower et al., 2017). Climate change in the Arctic and sub-Arctic regions has impacted the biological oceanography and productivity across the region (Overland et al., 2019; Huntington et al., 2020; Lewis et al., 2020), and while the density of amphipod prey of gray whales has declined in the Chirikov Basin and Northern Bering Sea since the late 1980s (Highsmith and Coyle, 1992; Moore et al., 2003), changes in prey availability on other foraging grounds have not been documented (Grebmeier et al., 2015).
Since climate variability and subsequent impacts on gray whale prey availability may manifest at different rates and spatio-temporal scales across Arctic, sub-Arctic, and Pacific northwest foraging grounds, we compare the body condition of gray whales on their PCFG feeding grounds in Oregon and ENP wintering grounds in SIL using UAS-based photogrammetry to elucidate sub-population physiological response to variable environmental forces on different foraging grounds. We test the following hypotheses: (H1) The body condition of gray whales on Oregon feeding and SIL wintering grounds is the same when compared at similar phenological time points: End of summer feeding seasons (departure Oregon vs. arrival SIL); End of wintering season (arrival Oregon vs. departure SIL). (H2) If similar environmental conditions regulate prey availability in Arctic, sub-Arctic, and Oregon feeding grounds - allowing gray whales equivalent access to energetic gain - we expect the same trajectory of body condition change across years in Oregon and SIL. Gray whale oxygen consumption rates (Sumich, 1983) and derived metabolic rates (Villegas-Amtmann et al., 2017) are much lower during migration than on foraging grounds; thus we assume that gray whales incur low energetic cost during migration. We also analyze an opportunistic dataset of aerial images of gray whales foraging in the Northeastern Chukchi Sea (NCS) of the Arctic region collected from a survey plane to compare body condition across foraging grounds (NCS vs. Oregon). Overall, our study compiles aerial image data collected by three different research programs using five different platforms (four UAS, one plane). Through robust assessment of uncertainty, we utilize this collaborative dataset to cross compare gray whale body condition at multiple phenological time steps on foraging and wintering grounds to inform population response to environmental change.
Materials and Methods
To reduce body condition variation due to demographic unit, our analysis is limited to adult whales only, not including lactating and pregnant females determined by the presence of a calf at the sighting or in the next year respectively, although undetected pregnancies (e.g., lost calf) were possible. Additionally, the photogrammetry datasets from Oregon and SIL are limited to the beginning and end of season periods to enhance detection of body condition change between periods. For each UAS image of a gray whale, we incorporate photogrammetric uncertainty to account for error in altitude recorded using an on-board barometer or laser altimeter (Bierlich et al., 2021b). An opportunistic dataset of 18 aerial images of gray whales foraging in the Arctic collected from a survey plane is also analyzed in this study to support conclusions and direct future research efforts. We calculate the Body Area Index (BAI) for each imaged whale as the comparative metric of overall body condition. BAI is a unitless and scale-invariant metric that accounts for whale length, allowing direct comparison of body condition across all individuals (Burnett et al., 2018), and demonstrated to have high precision with low uncertainty (Bierlich et al., 2021a).
Primary Analysis
Data Collection
Oregon:
We recorded videos of gray whales off the coast of Newport (44°38′13″ N, 124°03′08″ W) and Port Orford (42°44′59″ N, 124°29′53″ W), Oregon, USA during the 2017–2019 foraging seasons (June–October) using three UAS quadcopters: DJI Phantom 3 Pro (P3Pro), DJI Phantom 4 (P4), and DJI Phantom 4 Pro (P4Pro) (Figure 1). The cameras on both the P3Pro and P4 had a 6.16 x 4.6 mm sensor, 3840 x 2160 pixel-resolution, and a 3.61 mm focal length lens. The camera on the P4Pro had a 13.2 x 8.8 mm sensor, 3840 x 2160-pixel resolution, and 8.8 mm focal length lens. The P3Pro and P4 were both flown in 2017 and the P4Pro was flown in 2017, 2018, and 2019. Each aircraft had an onboard barometer for recording altitude and the launch height of the drone (measured as the surface of the water to the camera lens) was later added to the recorded barometer to account for bias introduced from the barometer zeroed at the launch point (Bierlich et al., 2021b). We conducted boat-based UAS flights as described in Burnett et al. (2018). Videos were collected at altitudes between 20 and 36 m of whales as they surfaced to breathe.
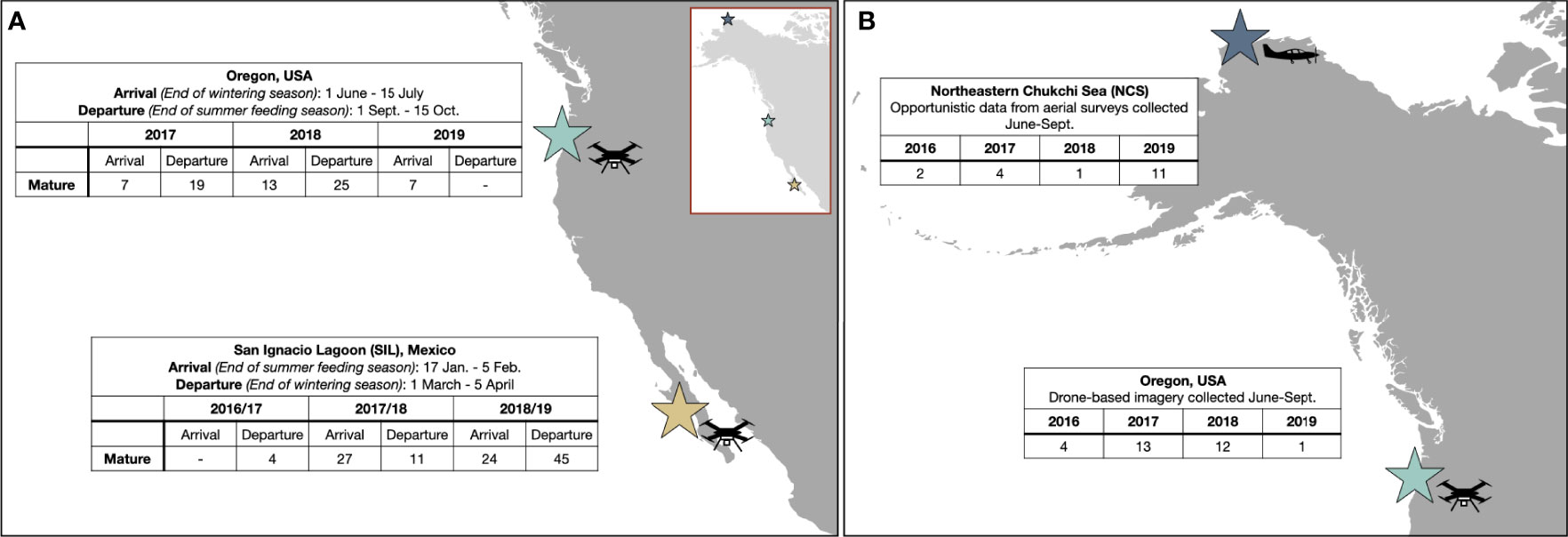
Figure 1 Maps illustrating the sample sites, sample sizes, and time periods of the datasets. (A) Dataset used for the primary analysis based on drone-based data collected off of Oregon, USA and San Ignacio Lagoon, Baja California Sur, Mexico (SIL). The tables provide sample size of images analyzed per period and sub-group. (B) Opportunistic dataset of images collected during aerial surveys in the Arctic (Northeastern Chukchi Sea; NCS) and a subset of drone-based images collected during the same years and months off the Oregon coast, USA. The inset in (A) illustrates the locations of all three sites.
Although PCFG whales have high site fidelity to this foraging range, individuals also show variability of where they feed within and between years (Calambokidis et al., 2019; Lagerquist et al., 2019). We assume the body condition of whales imaged off Oregon reflect the prey availability across the whole PCFG range, not just conditions in Oregon. To confirm the representativeness of our sampled whales to reflect conditions in this range, rather than vagrant whales migrating through, we calculate the sighting histories of the imaged whales to describe their foraging site fidelity to our study system.
SIL:
We recorded videos of gray whales inside San Ignacio Lagoon, Baja California Sur, Mexico during the 2017–2019 breeding seasons (January–April) using a DJI Inspire 1 Pro (I1Pro) quadcopter (Figure 1). The I1Pro was fitted with a Zenmuse X5 camera with a Micro Four Thirds (17.3 x 13 mm) sensor, 3840 x 2160-pixel resolution, a 25 mm focal length lens, and a Lightware SF11/C laser altimeter for recording altitude. We conducted shore- and boat-based UAS flights following Christiansen et al. (2021). Videos were collected of whales at altitudes between 22 and 49 m as they surfaced to breathe.
Data Processing
Image Filtering:
To avoid individual replicates within phenology group and site, individual whales were identified by assessing unique skin pigmentation and markings visible from the UAS and photo-identification images of the left-hand side, right-hand side, and fluke. Snapshots of each individual were taken from the UAS videos using VLC Media player software (version 3.0.16; VideoLAN, Paris, France). Each snapshot was ranked and filtered for quality in measurability based on body posture and image clarity and contrast following Christiansen et al. (2018).
Morphometric calculations and uncertainty:
We measured the total body length (TL) from rostrum to fluke notch and perpendicular widths at 5% increments of the TL in pixels using MorphoMetriX open-source photogrammetry software (Torres and Bierlich, 2020) for whales from Oregon (Figure 2A) and using a custom R script (Christiansen et al., 2016) for whales from SIL. To confirm measurements from the two software were comparable, we calculated the coefficient of variation for a subsample of 15 whales measured by a single analyst in both software. Results showed a mean CV% = 1.31% (sd = 1.09, min = 0.15, max = 3.67), indicating that the two software produce comparable measurements.
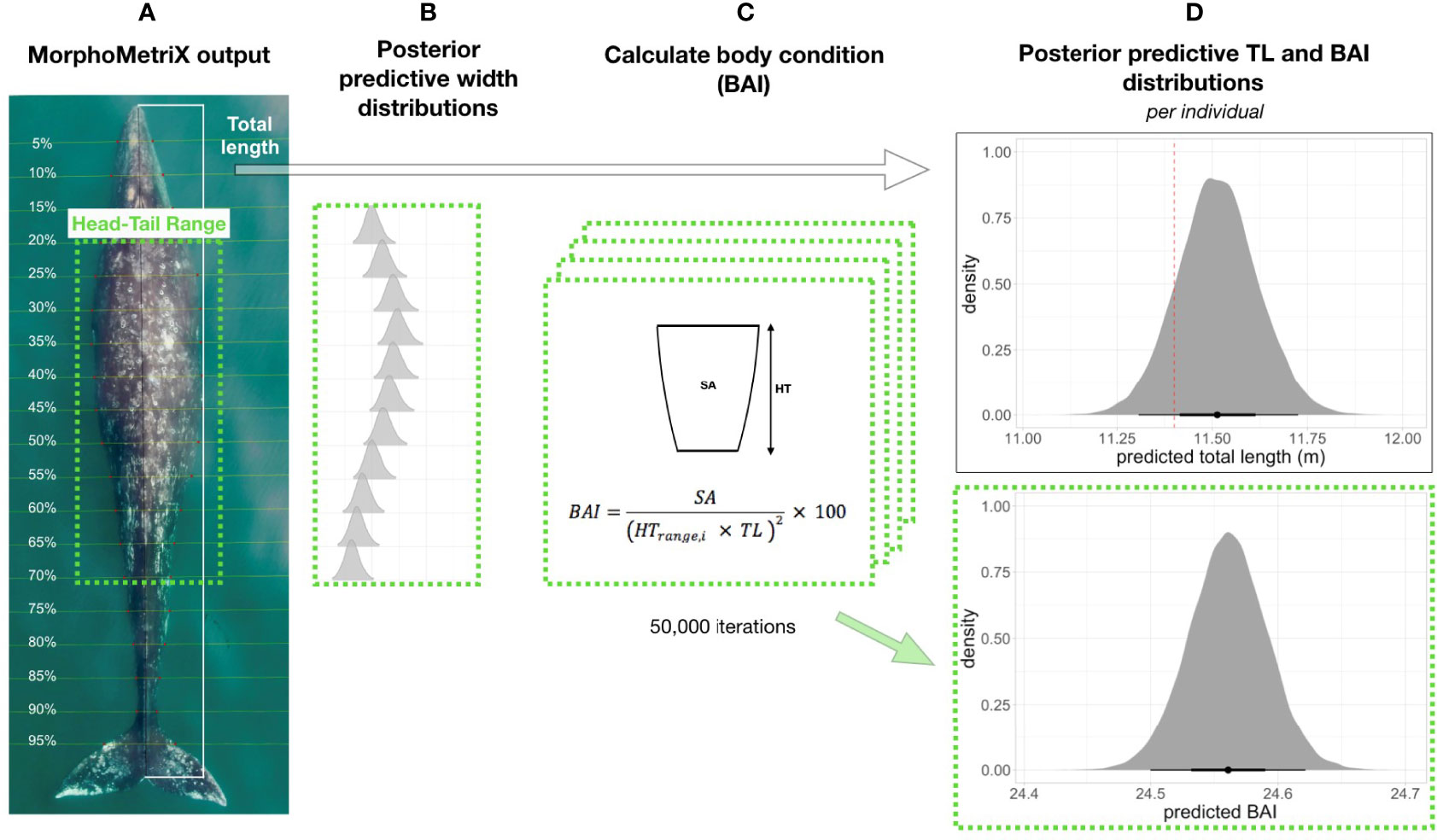
Figure 2 Overview of Bayesian framework for calculating the body condition of gray whales. (A) An example of a MorphoMetriX output (Torres and Bierlich, 2020) from a UAS image of a gray whale. Total length (TL) measured from rostrum to fluke notch with perpendicular widths segmented in 5% increments of TL. Head-Tail Range (20–70%) represents the region of the body used to calculate body condition, which excludes the fins, head, and tail. (B) Posterior predictive distributions for each 5% width included in the Head-Tail Range that is used to calculate body condition. (C) Body condition is calculated as Body Area Index (BAI) for each iteration in the Markov Chain Monte Carlo output of each posterior predictive width distribution using CollatriX (Bird and Bierlich, 2020). (D) Posterior predictive distributions for TL and BAI for a single individual. On the x-axis, the longer thin black bars represent the 95% highest posterior density (HPD) interval, the thicker shorter black bars represent the 65% HPD interval, and the black dot represents the mean value. The red dashed line represents the maturity cutoff length (11.4 m).
Bierlich et al. (2021b) demonstrate that photogrammetric uncertainty varies depending on the camera, focal length lens, altimeter, and altitude of the UAS, highlighting the necessity of evaluating uncertainty in assessments of whale body condition via UAS imagery. Bierlich et al. (2021b) developed a Bayesian statistical model to quantify and incorporate uncertainty by using measurements of known-sized objects at various altitudes as training data to predict the length measurements of unknown sized whales. These Bayesian statistical model outputs of measurement uncertainty for whale TL and width measurements can then be incorporated into estimations of whale body condition, including BAI (Bierlich et al., 2021a; Figure 2). Therefore, we account for measurement uncertainty associated with each UAS used in this study by applying the Bayesian statistical model described in Bierlich et al. (2021b). For the P3Pro, P4, and P4Pro, images of a known-sized board (1.0 m) floating at the surface collected at altitudes between 20–36 m were used as training data. For the IP1Pro, we used measurements of a known sized mat (1.45 m) on land flown between 4–120 m altitude (from Christiansen et al., 2018). We measured widths between a Head-Tail Range of 20–70% of each whale’s TL following Bierlich et al. (2021a) to then calculate body condition using BAI (Burnett et al., 2018; Figure 2). Rather than a single point estimate, the model quantifies uncertainty in TL, width measurements, and BAI for each individual whale through the model’s posterior distribution. We summarize uncertainty using 95% highest posterior density (HPD) intervals (Figure 2). Model development was conducted in R (Version 4.0.2; R Development Core Team, 2020) as described in Bierlich et al. (2021a; 2021b). To compare measurement uncertainty amongst each UAS, we calculated the coefficient of variation (CV%) for each individual’s posterior predictive distribution for TL and BAI. The CV% compares the relative width of an individual’s posterior predictive distribution, with a lower CV% translating to a more precise predicted measure (Bierlich et al., 2021a).
Maturity and Reproductive Status:
Maturity was assigned using either minimum age estimated from the individual’s date of first sighting or a TL cutoff when sighting history data was insufficient or unavailable. Age estimates derived from sighting history data were available for a subset of the Oregon dataset and unavailable for the SIL dataset. Individuals were assumed to be mature if they had a minimum age of at least eight years (Rice and Wolman, 1971). Whales were assumed to be immature if they were originally sighted as a calf, meaning that they were of known age, and were younger than eight years old. Individuals not seen as calves with minimum ages under eight years were considered to be of an unknown age; maturity was subsequently assigned to these individuals using a TL cutoff. It was assumed that (1) calves were small whales (<8 m) closely associated with large whales (>11 m) who were assumed to be the mother and mature, lactating females, (2) lactating females become post-weaning females once they are no longer associated with their calves in that year, and (3) lactating females were pregnant the previous year. When maturity was assigned using TL, individuals were considered mature if at least 50% of their posterior predictive distribution for TL was above a cutoff of 11.4 m (Rice and Wolman, 1971; Bierlich et al., 2021b; Figure 2).
Statistical Analysis
The temporal phases of gray whale phenology were grouped as follows (Figure 1A): “Departure Oregon” whales were imaged between 1 September and 15 October, and “Arrival SIL” whales were imaged between 17 January and 5 February, forming the End of summer feeding season group. “Departure SIL” whales were imaged between 1 March and 5 April, and “Arrival Oregon” whales were imaged between 1 June and 15 July, forming the End of wintering season group. Longer sampling windows were used in Oregon (6 weeks) than in SIL (3–4 weeks) to increase sample size.
This analysis included only mature adults, excluding pregnant, lactating, and post weaning females, that were imaged on foraging or wintering grounds. A Monte Carlo analysis of ANOVA tests was used to account for uncertainty associated with BAI measurements. We apply a unified model to test both hypotheses since BAI is potentially impacted by site, phenological time point, and year. We assume each BAI measurement without uncertainty has a mean specified with a full interactions model via
with μ quantifying a baseline BAI value, αi quantifying the mean-shift attributable to site (i = 1 for Oregon, and 2 for SIL); βj quantifying the mean-shift attributable to phenological timepoint (j = 1 for End of Winter, and 2 for End of Summer); γk quantifying the mean-shift attributable to year (k = 1 for 2017, 2 for 2018, and 3 for 2019); αβij, αγik, and βγjk quantifying the mean-shifts attributable to the pairwise interactions between site and phenology, site and year, and phenology and year, respectively; αβγijkquantifying the three-way interaction between all terms; and ϵijkl quantifying individual variation. The term ϵijkl is modeled as a mean zero normal random variable, with a common variance parameter across all groups (to be estimated). The model treats the observation year as a categorical predictor because the study is not long enough to identify consistent, long-term relationships across time; this modeling decision also allows us to model the data without assuming BAI follows a linear trend across years.
Monte Carlo methods allow us to account for the uncertainty in BAI observations by averaging the results of 10,000 replications of our ANOVA analyses. Each replicate uses a dataset in which the BAI measurement for each whale is sampled from the photogrammetric model’s posterior distributions, which are approximately normally distributed. For each ANOVA replicate, we sample each whale’s BAI measurement from a normal distribution parameterized with the photogrammetric model’s posterior mean and variance for that whale’s BAI value.
To test H1 for each replicate dataset, a generalized linear hypothesis test is employed. The test’s null hypothesis is that mean BAI between OR and SIL is the same within phenological time steps between years—i.e., that E(BAI11kl) = E(BAI21kl) for k=1,2,3, and E(BAI12kl) = E(BAI22kl) for k=1,2. The null hypothesis does not assess E(BAI12kl) = E(BAI22kl) for k=3 because SIL data are not available for the End of Summer phenology time point in 2019. To test H2 for each replicate dataset, a nested ANOVA F-test is employed. The test’s null hypothesis is that the mean BAI between OR and SIL experience the same changes across time—i.e., that all interaction effects between site and observation year are zero, αγik = 0 and αβγijk = 0 for i,j=1,2, and k=1,2,3. The average p-values from the replicated tests serve as the overall, Monte Carlo p-values used to evaluate H1 and H2. Analyses were conducted in R (R Development Core Team, 2020).
Opportunistic Analysis
Data Collection
The Aerial Surveys of Arctic Marine Mammals (ASAMM) project obtained aerial images of gray whales encountered during line-transect surveys in the Northeastern Chukchi Sea (NCS; 67°–72°N, 157°–169°W) in June–October, from 2016 to 2019 (Clarke et al., 2020; Figure 1B). Images were obtained using a Canon 1DX or 7D DSLR camera with a 100–400 mm zoom lens. While circling whales in the plane at ~40° bank, an observer used an open window located on the port side of the aircraft to obtain images at a nearly nadir angle (~90° below; see Supplementary Material for details). A stratified-random sample of gray whale UAS images in Oregon waters (n = 30) during the same years and months as the collected NCS images were selected for body condition comparison.
Post Processing
Image Filtering
NCS images were rated based on the body regions visible, the angle of the whale’s body relative to the aircraft, and the overall image quality (Full description of methods in Supplemental Material). Only high-quality images taken near nadir where the full body of the whale was visible and flat at the surface were selected. Images from Oregon were filtered following the protocol described in the Primary analysis above in the Data processing subsection Image filtering.
Morphometric Calculations
Images from both the NCS and Oregon were measured by a single analyst following the protocol described in the Morphometric calculations and uncertainty subsection in Primary analysis. There was no altitude information for images collected in the Arctic, therefore we were unable to incorporate uncertainty and BAI was calculated from raw pixel count measurements using the CollatriX body condition function (Bird and Bierlich, 2020). BAI measurements are usable and comparable because BAI is a standardized length invariant metric that can be equally calculated and interpreted using raw pixel counts as metric units (Burnett et al., 2018; Lemos et al., 2020; Bierlich et al., 2021a).
Statistical Analysis
Images collected between June and September of 2016–2019 in the NCS and Oregon waters were used for this analysis (Figure 1B). A Welch’s t-test was used to compare BAI between sites. Analyses were conducted in R (R Development Core Team, 2020).
Results
Across the three years of this study, images of 183 mature gray whales captured in Oregon and SIL were included in the analysis (Figure 1). Of the 71 whales imaged in Oregon water during the Arrival and Departure periods, 64 whales (90%) were observed on other days and years (between 2016 and 2021) in our study region, indicating residency by these whales to the PCFG foraging grounds.
Measurement uncertainty, measured as the coefficient of variation (CV%), varied among each UAS aircraft for TL and BAI. The I1Pro had the least amount of uncertainty associated with TL measurements (CV%: mean = 2.42, sd = 0.27, min = 1.56%, max = 3.23%) followed by the P4Pro (CV%: mean = 10.10, sd = 1.83% min = 6.96, max = 14.22%) and the P3Pro and P4 (CV%: mean = 11.93%, sd = 1.08%, min = 10.09%, max = 13.55%). The same trend followed for uncertainty associated with BAI, with I1Pro having the least uncertainty (CV%: mean = 0.12%, sd = 0.01%, min = 0.08%, max = 0.18%), followed by the P4Pro (CV%: mean = 0.91%, sd = 0.46%, min = 0.39%, max = 1.88%), and the P4 and P3Pro (CV%: mean = 2.97%, sd = 0.40%, min = 2.66%, max = 3.86%).
Assessment of predicted gray whale BAI values by site and phenological time point illustrates the expected trend that whales are in better body condition at the end of the summer foraging season compared to the end of the wintering season (Figure 3). The generalized linear hypothesis test compared the BAI of gray whales in Oregon and SIL within phenological time points across observation years and found significant evidence to reject an assumption that H1 is true, which expected similar body condition of gray whales imaged in Oregon and SIL when compared at the same phenological time points (p = 1e- 6). Simultaneous Monte Carlo confidence intervals associated with H1 reveal that mean BAI for SIL whales tends to be higher than mean BAI for Oregon whales (Figure 4). Furthermore, the BAI of PCFG gray whales in Oregon (n = 30) was significantly lower (Welch’s t-test, t(39) = 2.96, p = 0.005; Figure 5) than gray whales imaged while feeding in the NCS region of the Arctic (n = 18), providing more evidence of an intrinsic difference in body condition between PCFG gray whales foraging in Oregon and ENP whales foraging in the Arctic and sub-Arctic. This result also supports our assumption that the majority of gray whales imaged in SIL are from the ENP, and does not reflect the body condition of PCFG whales.
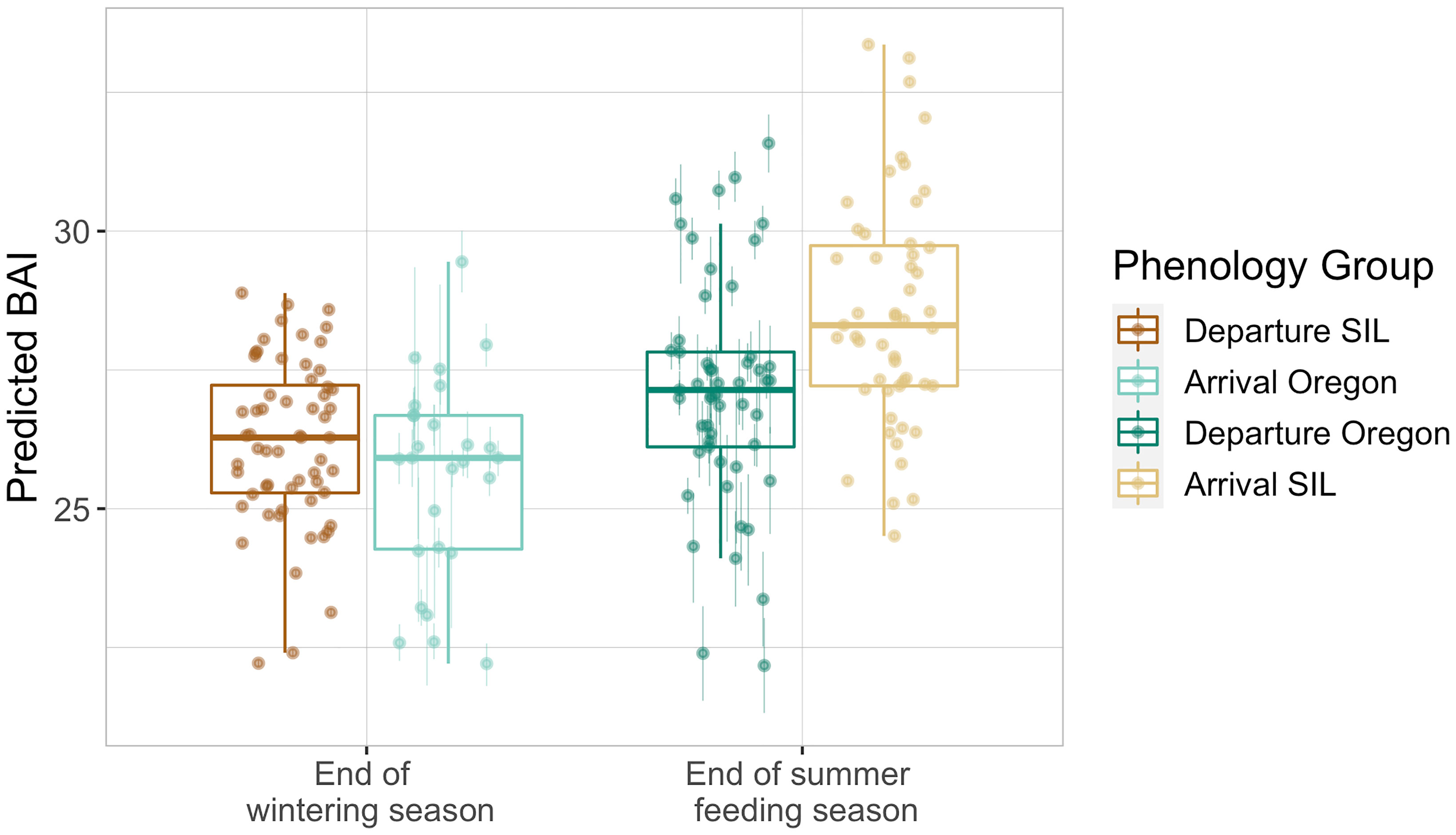
Figure 3 Boxplots illustrating the distribution of predicted Body Area Index (BAI) values of gray whales imaged by UAS in San Ignacio Lagoon (SIL), Mexico and Oregon, USA as produced by the Bayesian statistical model accounting for measurement uncertainty. Data are grouped by phenology group: End of summer feeding season (departure Oregon vs. arrival SIL) and End of wintering season (arrival Oregon vs. departure SIL). The group median (horizontal line), interquartile range (IQR, box), maximum and minimum 1.5*IQR (vertical lines), and outliers (dots) are depicted in the boxplots. The overlaid points represent the mean of the posterior predictive distribution for BAI of an individual and the bars represent the upper and lower bounds of the 95% HPD interval.
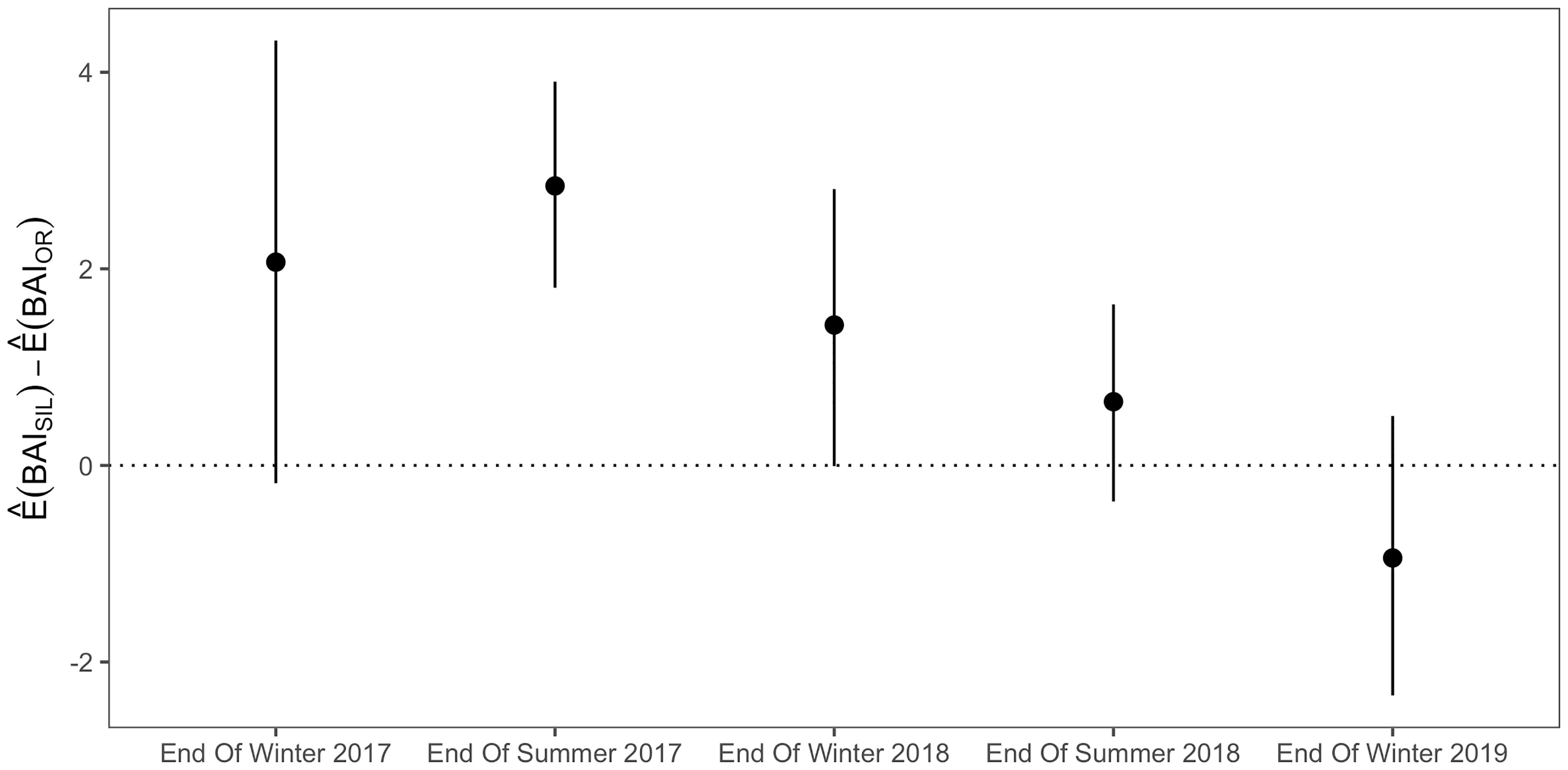
Figure 4 Simultaneous Monte Carlo confidence intervals produced by the generalized linear hypothesis test of H1 illustrating estimated differences between mean Body Area Index (BAI) by site (SIL – OR) within phenology groups. Points represent the mean estimated difference and vertical lines represent simultaneous Monte Carlo confidence intervals.
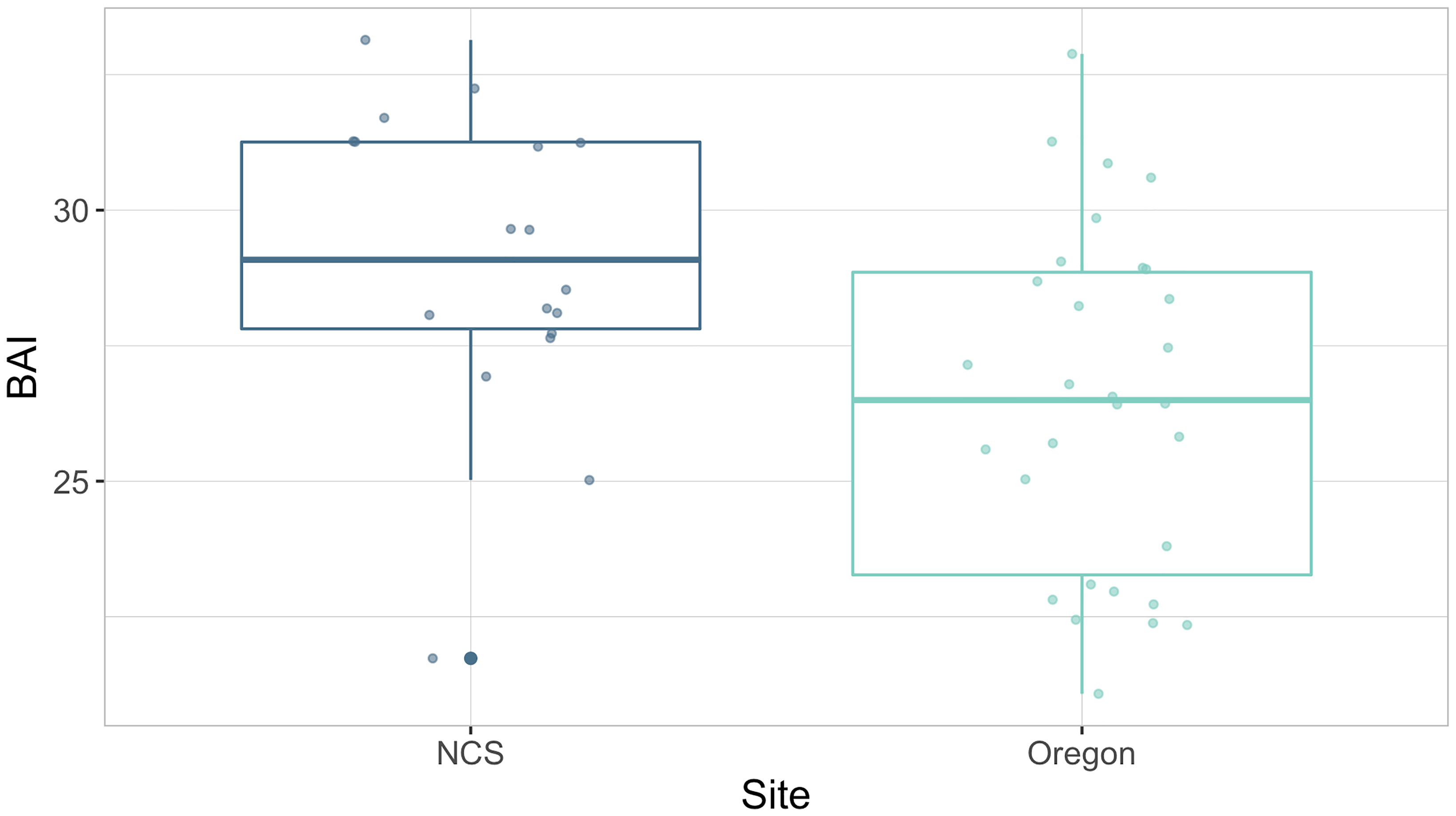
Figure 5 Boxplots illustrating the distribution of Body Area Index (BAI) values of gray whales imaged by survey plane in the Northeastern Chukchi Sea (NCS) of the Arctic and by UAS in Oregon. Data are grouped by site. The group median (horizontal line), interquartile range (IQR, box), maximum and minimum 1.5*IQR (vertical lines), and outliers (dots) are depicted in the boxplots. The overlaid points represent the BAI values from each image.
The nested ANOVA test for H2 assessed differences in the BAI trajectories between sites across years, finding significant evidence to reject an assumption that H2 is true (p = 1e-3). Monte Carlo confidence intervals associated with H2 suggest the BAI of gray whales at departure from SIL decreased over time, while the BAI of whales departing from Oregon increased over time (Figure 6). Rejecting H2 indicates that environmental conditions that influence prey availability on Oregon and Arctic or sub-Arctic foraging grounds are different, causing different trajectories of body condition change across the study years.
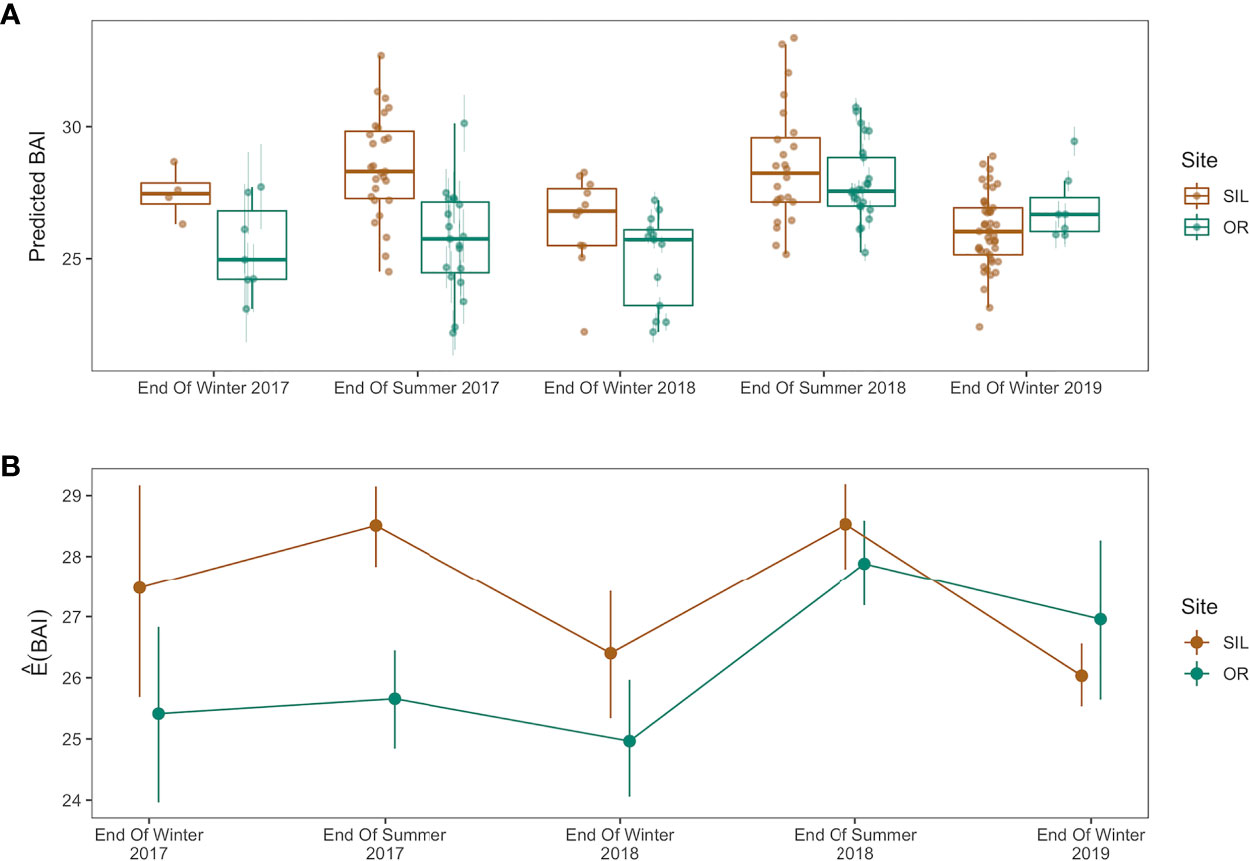
Figure 6 Change in gray whale body condition in San Ignacio Lagoon (SIL), Mexico and Oregon, USA by phenology group and year: End of wintering season (arrival Oregon vs. departure SIL) and End of summer feeding season (departure Oregon vs. arrival SIL). (A) Boxplots illustrating the distribution of gray whale estimated Body Area Index (BAI) values across years and phenology group as produced by the Bayesian statistical model accounting for measurement uncertainty. The group median (horizontal line), 75% distribution (box), 95% range (vertical lines), and outliers (dots) are depicted. The overlaid points represent the mean of the posterior predictive distribution for BAI for an individual and the bars represent the upper and lower bounds of the 95% HPD interval. (B) Estimated mean BAI values for SIL and Oregon across phenology groups and years derived from the nested ANOVA test of H2. Points represent the mean BAI estimate and vertical lines represent simultaneous Monte Carlo confidence intervals.
Discussion
Through a comparative analysis of gray whale body condition at multiple temporal periods during different phenology stages we document contrasting trends and ranges of body condition. Gray whale body condition on the wintering grounds in SIL deteriorated across the study years (2017–2019), while the body condition of PCFG gray whales on the foraging grounds off Oregon concurrently increased. These juxtaposing trajectories of body condition between the ENP and PCFG suggests use of distinct foraging grounds with different prey availability, likely driven by variable oceanographic patterns in the Arctic/sub-Arctic region and PCFG range. Additionally, we document significantly lower overall body condition of PCFG whales feeding in Oregon coastal waters compared to whales imaged in SIL or the NCS region of the Arctic. This lower body condition of PCFG whales may cause reduced resilience to perturbations in prey quality or availability relative to ENP whales, and could have implications for the reproductive capacity of the PCFG sub-group.
The decreasing body condition of gray whales imaged in SIL reflects declining nutritive gain by the ENP population, which may be associated with environmental change in the Arctic and sub-Arctic regions that impacted the predictability and availability of gray whale prey. In contrast, the increasing body condition of PCFG gray whales imaged in Oregon may reflect recovery of these whales from a period of low prey resource availability in the PCFG foraging range as the ecosystem rebounded from the marine heatwave event of 2014–2016 known as “The Blob” (Peterson et al., 2017; Fewings and Brown, 2019). While global climate change drives environmental change in both regions, our results demonstrate that dynamic oceanographic processes cause temporal variability of resource availability to gray whales in geographically distinct regions. Although the deteriorating body condition of whales in SIL indicates malnourishment of ENP whales on foraging grounds in the Arctic and sub-Arctic, it is interesting to note that the body condition of PCFG whales in Oregon is regularly and significantly lower than the body condition of whales in SIL (and the NCS). In fact, only when whales were at their lowest BAI as they departed SIL in 2019 was the mean body condition of whales arriving in Oregon higher (Figure 6).
Gray whales make fine-scale foraging decisions, within diverse foraging habitats, using different strategies to target varied prey across their whole North Pacific range (Nerini, 1984; Mallonée, 1991; Darling et al., 1998; Dunham and Duffus, 2001; Stelle et al., 2008; Torres et al., 2018). Gray whales may make similar, flexible foraging decisions at multiple scales to optimize energy intake. This non-stereotyped approach to energy acquisition has potentially led to the adaptability of gray whales to target novel prey resources (e.g. herring roe, crab larvae, ghost shrimp; Darling et al., 1998; Haifley, 2021; Hildebrand et al., 2021) and responsiveness to environmental change through behavioral adaptations over short evolutionary time scales. This behavioral plasticity can buffer animals from resource shortages and shifts (e.g., Riddell et al., 2018) if the energetic tradeoffs between prey quality and capture costs of novel prey enable adequate energetic gain to support population viability. Yet, if environmental change happens too quickly or too broadly, gray whales may not be able to adapt fast enough or find adequate prey alternatives, leading to increased competition for limited prey and eventual malnutrition.
Our finding that PCFG gray whales in Oregon have lower body condition than gray whales foraging in the NCS of the Arctic (small sample size) and whales on the wintering grounds in SIL (larger sample size) is surprising and raises many prudent questions. Do whales with lower body condition recruit into the PCFG because this strategy is adapted to a smaller morphology, perhaps due to the use of specialized foraging strategies (Torres et al., 2018)? Or is the PCFG range a culturally inherited foraging destination (e.g., Baker et al., 2013) where lower body condition is a genetically inherited trait and whales are adapted to survive with reduced energy stores? Energetic costs of gray whale migration appear to be low (Sumich, 1983), indicating that the lower body condition of PCFG whales may not be entirely related to the truncated migration distance. However, given the lower body condition of PCFG whales, even the additional energetic cost of migration to continue to the Arctic or sub-Arctic may be unsupportable. Alternatively, PCFG whales may be unable to accumulate as much fat reserves relying on prey in the PCFG range as compared to Arctic/sub-Arctic foraging whales. This possibility may explain why gray whales departing summer foraging grounds in Oregon were regularly in worse body condition than whales arriving in SIL after the migration south (Figures 3, 6A). Yet, PCFG whales do recruit calves to this sub-group regularly (Calambokidis and Pérez, 2017b), indicating that females can obtain adequate energy stores to support the demands of reproduction and lactation. However, the calving interval of PCFG female whales is unknown and could be less than ENP females (2 years; Jones, 1990), as it may take PCFG females longer to recover from previous pregnancies and amass enough energetic storage to support another reproductive cycle. Additionally, the age at first reproduction of PCFG whales may be higher if whales are slower to mature and gain adequate mass. Future research efforts should focus on determining the calving rate and age at first reproduction of PCFG whales relative to ENP whales and applying photogrammetry methods to quantify the threshold of body condition necessary to support pregnancy in gray whales. These factors are critical pieces of information needed to inform realistic population models.
The timing of the 2019–2021 gray whale UME aligns with the decline in body condition of whales imaged in SIL between 2017 and 2019 (this study and Christiansen et al., 2021), indicating that the ENP population was primarily impacted in this die-off, not PCFG whales that showed an increase in body condition during this same time period. This coincident timing between the UME and deteriorating body condition of SIL whales suggests that a threshold of malnutrition was met by many ENP whales, as suggested by Christiansen et al. (2021), potentially due to sustained low prey availability on Arctic or sub-Arctic foraging grounds over several years. While the PCFG appears to be less affected by this 2019-2021 UME, this distinct sub-group of gray whales shows significant interannual variation in body condition (Lemos et al., 2020; Akmajian et al., 2021) that is likely related to prey limitations in response to local oceanographic disruptions (e.g., marine heat waves in the NE Pacific Ocean) and broad-scale climate change. Given their apparent lower body condition, PCFG gray whales may have a lower energetic buffer to prolonged periods of low resource availability than ENP whales. If the PCFG did succumb to a die-off event, this small sub-group (~230 individuals) risks losing the cultural knowledge of this foraging range. Prey availability in the PCFG range may only be sufficient to support this small population segment of whales, but this resource and strategy may be less stochastic than foraging in the Arctic or sub-Arctic where the ENP has undergone two UMEs in that last two decades, with dramatic population fluctuations including potentially reaching carrying capacity limits (Moore et al., 2001; Coyle et al., 2007). Therefore, continued and expanded monitoring of gray whale body condition across their entire range, including wintering grounds in Baja California, the PCFG range, the Arctic and sub-Arctic foraging grounds, and on the foraging grounds of the WNP whales in eastern Russian, would provide critical information on the health and response of each population to ecosystem change, particularly in a collaborative and comparative fashion as demonstrated in this study.
UAS collection of whale photogrammetry data is cost-effective, non-invasive, and data rich. Cross-population studies of baleen whale body condition can provide valuable insight, and as demonstrated in this study is feasible even if data are collected by multiple UAS platforms, with different image and data quality. We united and robustly compared these diverse datasets through the comprehensive assessment of uncertainty and calculation of the scale-invariant BAI metric of whale body condition. In addition to behavioral and distributional shifts in response to changes in prey availability (e.g., Torres et al., 2013; Ramp et al., 2015; Brower et al., 2017), baleen whales will respond physiologically through variation in body condition, making UAS assessment of body condition a powerful tool to document how whale populations respond to climate change.
Data Availability Statement
The body area index (BAI) dataset used in this analysis can be accessed via FigShare: https://doi.org/10.6084/m9.figshare.19469966.v1. Further inquiries can bedirected to the corresponding author.
Ethics Statement
The animal study was reviewed and approved by Oregon State University, Animal Program Office and Murdoch University. Research conducted in Oregon was done under the NOAA/NMFS permits #16011 and #21678 issued to John Calambokidis. UAS operations were conducted by a Federal Aviation Authority (FAA) certified private pilot with a Part107 license. All field Research in San Ignacio Lagoon, Baja California Sur was carried out under scientific research permits from the Secretaría del Medio Ambiente y Recursos Naturales, Subsecretaría de Gestión Para La Protectión Ambiental, Dirección General De Vida Silvestre, de México (SGPA/DGVS/ 013210/18, SGPA/DGVS/000531/18, SGPA/DGVS/ 01822/ 17) and an animal ethics permit from Murdoch University (R3012/ 18), Australia.
Author Contributions
LT, CB, KB, and FC contributed to conception and design of study. LT, CB, FR-G, FC, LB, LL, JR, SS, and AW contributed to data collection. LT, CB, KB, FC, AW, and LL contributed to data processing. LT, CB, KB, and JH contributed to data analysis and visualization. LT, FC, LB, JR, SS and AW contributed to funding acquisition. LT, CB and KB led manuscript writing with all authors contributing to revision and in agreement to the published version of the submitted version.
Funding
Data collected in Oregon was supported by the NOAA National Marine Fisheries Service Office of Science and Technology Ocean Acoustics Program [2016 and 2017; 50-27], the Oregon Sea Grant Program Development funds [2018; RECO-40-PD], and the Oregon State University Marine Mammal Institute [2019]. Data collected in San Ignacio Lagoon, Baja California Sur, was funded by Murdoch University, the Universidad Autónoma de Baja California Sur and The Laguna San Ignacio Ecosystem Science Program, a Project of The Ocean Foundation. Data collected in the Arctic was funded by the Bureau of Ocean Energy Management (BOEM), Alaska Region, under Interagency Agreements M16PG00013 and M17PG00031 with the Alaska Fisheries Science Center (AFSC). The Aerial Surveys of Arctic Marine Mammals project was co-managed by BOEM and the Marine Mammal Laboratory, AFSC, National Oceanic and Atmospheric Administration. Data collected in the Arctic was funded by the Bureau of Ocean Energy Management (BOEM), Alaska Region, under Interagency Agreements M16PG00013 and M17PG00031 with the Alaska Fisheries Science Center (AFSC). The Aerial Surveys of Arctic Marine Mammals project was co-managed by BOEM and the Marine Mammal Laboratory, AFSC, National Oceanic and Atmospheric Administration.
Conflict of Interest
The authors declare that the research was conducted in the absence of any commercial or financial relationships that could be construed as a potential conflict of interest.
The reviewer SM declared a shared affiliation with the author AW to the handling editor at the time of review.
Publisher’s Note
All claims expressed in this article are solely those of the authors and do not necessarily represent those of their affiliated organizations, or those of the publisher, the editors and the reviewers. Any product that may be evaluated in this article, or claim that may be made by its manufacturer, is not guaranteed or endorsed by the publisher.
Acknowledgments
We thank Todd Chandler for dedicated UAS operations in Oregon. We thank Cascadia Research Collective for assistance with the photo-identification analysis of whales observed in Oregon. We thank Sergio Martínez-Aguilar, Fabien Vivier and Hunter Warick for contributing to the data collection in Baja California Sur. We also thank Amanda Mayfield for photogrammetry analysis of images collected in the Arctic, and two anonymous reviewers for insightful feedback.
Supplementary Material
The Supplementary Material for this article can be found online at: https://www.frontiersin.org/articles/10.3389/fmars.2022.867258/full#supplementary-material
References
Akmajian A. M., Scordino J. J., Gearin P. J., Gosho M. (2021). Body Condition of Gray Whales (Eschrichtius Robustus) Feeding on the Pacific Coast Reflects Local and Basin-Wide Environmental Drivers. J. Cetacean. Res. Manage. 22, 87–110. doi: 10.47536/jcrm.v22i1.223
Alter S. E., Rynes E., Palumbi S. R. (2007). DNA Evidence for Historic Population Size and Past Ecosystem Impacts of Gray Whales. Proc. Natl. Acad. Sci. 104, 15162–15167. doi: 10.1073/pnas.0706056104
Baker C., Steel D., Calambokidis J., Falcone E., Gonzlez-Peral U., Barlow J., et al. (2013). Strong Maternal Fidelity and Natal Philopatry Shape Genetic Structure in North Pacific Humpback Whales. Mar. Ecol. Prog. Ser. 494, 291–306. doi: 10.3354/meps10508
Bastille-Rousseau G., Schaefer J. A., Peers M. J. L., Ellington E. H., Mumma M. A., Rayl N. D., et al. (2018). Climate Change can Alter Predator–Prey Dynamics and Population Viability of Prey. Oecologia 186, 141–150. doi: 10.1007/s00442-017-4017-y
Bierlich K. C., Hewitt J., Bird C. N., Schick R. S., Friedlaender A., Torres L. G., et al. (2021a). Comparing Uncertainty Associated With 1-, 2-, and 3D Aerial Photogrammetry-Based Body Condition Measurements of Baleen Whales. Front. Mar. Sci. 8. doi: 10.3389/fmars.2021.749943
Bierlich K. C., Schick R. S., Hewitt J., Dale J., Goldbogen J. A., Friedlaender A. S., et al. (2021b). Bayesian Approach for Predicting Photogrammetric Uncertainty in Morphometric Measurements Derived From Drones. Mar. Ecol. Prog. Ser. 673, 193–210. doi: 10.3354/meps13814
Bird C. N., Bierlich K. (2020). CollatriX: A GUI to Collate MorphoMetriX Outputs. J. Open Source Soft. 5, 2328. doi: 10.21105/joss.02328
Braham H. W., Jones M., Swartz S., Leatherwood S. (1984). Distribution and Migration of Gray Whales in Alaska.Jones M. L., Swartz S. L., Leatherwood S. eds. The Gray Whale: Eschrichtius robustus Academic Press. pp. 249–266. doi: 10.1016/B978-0-08-092372-7.50017-0
Braithwaite J. E., Meeuwig J. J., Letessier T. B., Jenner K. C. S., Brierley A. S. (2015). From Sea Ice to Blubber: Linking Whale Condition to Krill Abundance Using Historical Whaling Records. Polar. Biol. 38, 1195–1202. doi: 10.1007/s00300-015-1685-0
Brower A. A., Ferguson M. C., Schonberg S. V., Jewett S. C., Clarke J. T. (2017). Gray Whale Distribution Relative to Benthic Invertebrate Biomass and Abundance: Northeastern Chukchi Sea 2009–2012. Deep. Sea. Res. Part II: Top. Stud. Oceanograph. 144, 156–174. doi: 10.1016/j.dsr2.2016.12.007
Burnett J. D., Lemos L., Barlow D., Wing M. G., Chandler T., Torres L. G. (2018). Estimating Morphometric Attributes of Baleen Whales With Photogrammetry From Small UASs: A Case Study With Blue and Gray Whales. Mar. Mammal. Sci. 35, 108–139. doi: 10.1111/mms.12527
Butterworth D., Korrûbel J., Punt A. (2002). What is Needed to Make a Simple Density-Dependent Response Population Model Consistent With Data for the Eastern North Pacific Gray Whales? J. Cetacean. Res. Manage. 4, 63–76.
Calambokidis J., Pérez A. (2017a). “Association of PCFG Gray Whales on Migration”, in Pap SC/A17/GW/02 Present to Sci Comm Int Whal Comm(Cambridge, UK: The International Whaling Commission).
Calambokidis J., Pérez A. (2017b). “Internal Recruitment to the PCFG From Births to PCFG Mothers,” in Workshop on the Status of North Pacific Gray Whales (La Jolla, CA: International Whaling Commission).
Calambokidis J., Pérez A., Laake J. (2019). Updated Analysis of Abundance and Population Structure of Seasonal Gray Whales in the Pacific Northwest 1996–2017 (Seattle, Washington: Final Report to NOAA), 1–72.
Christiansen F., Dawson S. M., Durban J. W., Fearnbach H., Miller C. A., Bejder L., et al. (2020). Population Comparison of Right Whale Body Condition Reveals Poor State of the North Atlantic Right Whale. Mar. Ecol. Prog. Ser. 640, 1–16. doi: 10.3354/meps13299
Christiansen F., Dujon A. M., Sprogis K. R., Arnould J. P. Y., Bejder L. (2016). Noninvasive Unmanned Aerial Vehicle Provides Estimates of the Energetic Cost of Reproduction in Humpback Whales. Ecosphere 7, e01468–e01n/a. doi: 10.1002/ecs2.1468
Christiansen F., Rodríguez-González F., Martínez-Aguilar S., Urbán J., Swartz S., Warick H., et al. (2021). Poor Body Condition Associated With an Unusual Mortality Event in Gray Whales. Mar. Ecol. Prog. Ser. 658, 237–252. doi: 10.3354/meps13585
Christiansen F., Vivier F., Charlton C., Ward R., Amerson A., Burnell S., et al. (2018). Maternal Body Size and Condition Determine Calf Growth Rates in Southern Right Whales. Mar. Ecol. Prog. Ser. 592, 267–281. doi: 10.3354/meps12522
Clarke J. T., Brower A. A., Ferguson M. C., Willoughby A. L., Rotrock A. D. (2020). “Distribution and Relative Abundance of Marine Mammals in the Eastern Chukchi Sea, Eastern and Western Beaufort Sea, and Amundsen Gul,” in Annual Report, OCS Study BOEM 2020-027 (7600 Sand Point Way NE, F/AKC3, Seattle, WA 98115-6349: Marine Mammal Laboratory, Alaska Fisheries Science Center, NMFS, NOAA).
Cooke J. G., Sychenko O., Burdin A., Weller D., Bradford A., Lang A., et al. (2019). “Population Assessment Update for Sakhalin Gray Whales,” in Western Gray Whale Advisory Panel, 20th Panel. (Cambridge, UK: The International Whaling Commission). pp. 6–8.
Coyle K. O., Bluhm B., Konar B., Blanchard A., Highsmith R. C. (2007). Amphipod Prey of Gray Whales in the Northern Bering Sea: Comparison of Biomass and Distribution Between the 1980s and 2002–2003. Deep. Sea. Res. Part II: Top. Stud. Oceanograph. 54, 2906–2918. doi: 10.1016/j.dsr2.2007.08.026
Darling J. D., Keogh K. E., Steeves T. E. (1998). Gray Whale (Eschrichtius Robustus) Habitat Utilization and Prey Species Off Vancouver Island, BC. Mar. Mammal. Sci. 14, 692–720. doi: 10.1111/j.1748-7692.1998.tb00757.x
Dunham J. S., Duffus D. A. (2001). Foraging Patterns of Gray Whales in Central Clayoquot Sound, British Columbia, Canada. Mar. Ecol. Prog. Ser. 223, 299–310. doi: 10.3354/meps223299
Durban J. W., Moore M. J., Chiang G., Hickmott L. S., Bocconcelli A., Howes G., et al. (2016). Photogrammetry of Blue Whales With an Unmanned Hexacopter. Mar. Mammal. Sci. 32, 1510–1515. doi: 10.1111/mms.12328
Fewings M. R., Brown K. S. (2019). Regional Structure in the Marine Heat Wave of Summer 2015 Off the Western United States. Front. Mar. Sci. 6, 564. doi: 10.3389/fmars.2019.00564
Frasier T. R., Koroscil S. M., White B. N., Darling J. D. (2011). Assessment of Population Substructure in Relation to Summer Feeding Ground Use in the Eastern North Pacific Gray Whale. Endanger. Species. Res. 14, 39–48. doi: 10.3354/esr00340
Grebmeier J. M., Bluhm B. A., Cooper L. W., Danielson S. L., Arrigo K. R., Blanchard A. L., et al. (2015). Ecosystem Characteristics and Processes Facilitating Persistent Macrobenthic Biomass Hotspots and Associated Benthivory in the Pacific Arctic. Prog. Oceanograph. 136, 92–114. doi: 10.1016/j.pocean.2015.05.006
Haifley A. L. (2021). Habitat Distribution of North Puget Sound Gray Whales Between 1990-2019 (Olympia, WA, USA: Masters of Environmental Studies, The Evergreen State College).
Hamilton C. D., Kovacs K. M., Ims R. A., Aars J., Lydersen C. (2017). An Arctic Predator–Prey System in Flux: Climate Change Impacts on Coastal Space Use by Polar Bears and Ringed Seals. J. Anim. Ecol. 86, 1054–1064. doi: 10.1111/1365-2656.12685
Highsmith R. C., Coyle K. O. (1992). Productivity of Arctic Amphipods Relative to Gray Whale Energy Requirements. Mar. Ecol. Prog. Ser. 83, 141–150. doi: 10.3354/meps083141
Hildebrand L., Bernard K. S., Torres L. G. (2021). Do Gray Whales Count Calories? Comparing Energetic Values of Gray Whale Prey Across Two Different Feeding Grounds in the Eastern North Pacific. Front. Mar. Sci. 8. doi: 10.3389/fmars.2021.683634
Huntington H. P., Danielson S. L., Wiese F. K., Baker M., Boveng P., Citta J. J., et al. (2020). Evidence Suggests Potential Transformation of the Pacific Arctic Ecosystem Is Underway. Nat. Climate Change 10, 342–348. doi: 10.1038/s41558-020-0695-2
Jirinec V., Burner R. C., Amaral B. R., Bierregaard R. O., Fernández-Arellano G., Hernández-Palma A., et al. (2021). Morphological Consequences of Climate Change for Resident Birds in Intact Amazonian Rainforest. Sci. Adv. 7, eabk1743. doi: 10.1126/sciadv.abk1743
Johnston D. W. (2019). Unoccupied Aircraft Systems in Marine Science and Conservation. Annu. Rev. Mar. Sci. 11, 439–463. doi: 10.1146/annurev-marine-010318-095323
Jones M. L. (1990). The Reproductive Cycle in Gray Whales Based on Photographic Resightings of Females on the Breeding Grounds From 1977–82. Rep. Int. Whaling. Commission. (Special. Issue. 12) 177, 182.
Jönsson K. I. (1997). Capital and Income Breeding as Alternative Tactics of Resource Use in Reproduction. Oikos 78, 57–66. doi: 10.2307/3545800
Laake J. L., Punt A. E., Hobbs R., Ferguson M., Rugh D., Breiwick J. (2012). Gray Whale Southbound Migration Surveys 1967–2006: An Integrated Re-Analysis. J. Cetacean. Res. Manage. 12, 287–306.
Lagerquist B. A., Palacios D. M., Winsor M. H., Irvine L. M., Follett T. M., Mate B. R. (2019). Feeding Home Ranges of Pacific Coast Feeding Group Gray Whales. J. Wildlife. Manage. 83, 925–937. doi: 10.1002/jwmg.21642
Lang A. R., Calambokidis J., Scordino J., Pease V. L., Klimek A., Burkanov V. N., et al. (2014). Assessment of Genetic Structure Among Eastern North Pacific Gray Whales on Their Feeding Grounds. Mar. Mammal. Sci. 30, 1473–1493. doi: 10.1111/mms.12129
Lemos L., Burnett J., Chandler T., Sumich J. L., Torres L. G. (2020). Intra- and Inter-Annual Variation in Gray Whale Body Condition on a Foraging Ground. Ecosphere 11, e03094. doi: 10.1002/ecs2.3094
Lenoir J., Bertrand R., Comte L., Bourgeaud L., Hattab T., Murienne J., et al. (2020). Species Better Track Climate Warming in the Oceans Than on Land. Nat. Ecol. Evol. 4, 1044–1059. doi: 10.1038/s41559-020-1198-2
Lewis K. M., Dijken G. L. V., Arrigo K. R. (2020). Changes in Phytoplankton Concentration Now Drive Increased Arctic Ocean Primary Production. Science 369, 198–202. doi: 10.1126/science.aay8380
Mallonée J. S. (1991). Behaviour of Gray Whales (Eschrichtius Robustus) Summering Off the Northern California Coast, From Patrick’s Point to Crescent City. Can. J. Zoology. 69, 681–690. doi: 10.1139/z91-100
Mate B. R., Ilyashenko V. Y., Bradford A. L., Vertyankin V. V., Tsidulko G. A., Rozhnov V. V., et al. (2015). Critically Endangered Western Gray Whales Migrate to the Eastern North Pacific. Biol. Lett. 11, 20150071. doi: 10.1098/rsbl.2015.0071
Moore S. E., Grebmeier J. M., Davies J. R. (2003). Gray Whale Distribution Relative to Forage Habitat in the Northern Bering Sea: Current Conditions and Retrospective Summary. Can. J. Zoology. 81, 734–742. doi: 10.1139/z03-043
Moore S. E., Perryman W. L., Gulland F., Wade P. R., Rojas-Bracho L., Rowles T. (2001). Are Gray Whales Hitting “K” Hard? Mar. Mammal. Sci. 17, 954–958. doi: 10.1111/j.1748-7692.2001.tb01310.x
Moore S. E., Wynne K. M., Kinney J. C., Grebmeier J. M. (2007). Gray Whale Occurrence and Forage Southeast of Kodiak, Island, Alaska. Mar. Mammal. Sci. 23, 419–428. doi: 10.1111/j.1748-7692.2007.00102.
Nerini M. (1984). A Review of Gray Whale Feeding Ecology. Jones M. L., Swartz S. L., Leatherwood S. eds. The Gray Whale: Eschrichtius robustus Academic Press. pp. 423–450. doi: 10.1016/B978-0-08-092372-7.50024-8
Overland J., Dunlea E., Box J. E., Corell R., Forsius M., Kattsov V., et al. (2019). The Urgency of Arctic Change. Polar. Sci. 21, 6–13. doi: 10.1016/j.polar.2018.11.008
Peterson W. T., Fisher J. L., Strub P. T., Du X., Risien C., Peterson J., et al. (2017). The Pelagic Ecosystem in the Northern California Current Off Oregon During the 2014–2016 Warm Anomalies Within the Context of the Past 20 Years. J. Geophy. Res.: Oceans. 122, 7267–7290. doi: 10.1002/2017JC012952
Ramp C., Delarue J., Palsbøll P. J., Sears R., Hammond P. S. (2015). Adapting to a Warmer Ocean—Seasonal Shift of Baleen Whale Movements Over Three Decades. PloS One 10, e0121374. doi: 10.1371/journal.pone.0121374
R Development Core Team (2020). R: A Language and Environment for Statistical Computing”. 3.2.2 Ed (Vienna, Austria: R Foundation for Statistical Computing).
Rice D. W., Wolman A. A. (1971). The Life History and Ecology of the Gray Whale (Eschrichtius Robustus) (Stillwater, Oklahoma: American Society of Mammalogists).
Riddell E. A., Odom J. P., Damm J. D., Sears M. W. (2018). Plasticity Reveals Hidden Resistance to Extinction Under Climate Change in the Global Hotspot of Salamander Diversity. Sci. Adv. 4, eaar5471. doi: 10.1126/sciadv.aar5471
Stelle L. L., Megill W. M., Kinzel M. R. (2008). Activity Budget and Diving Behavior of Gray Whales (Eschrichtius Robustus) in Feeding Grounds Off Coastal British Columbia. Mar. Mammal. Sci. 24, 462–478. doi: 10.1111/j.1748-7692.2008.00205.x
Stephens P. A., Houston A. I., Harding K. C., Boyd I. L., Mcnamara J. M. (2014). Capital and Income Breeding: The Role of Food Supply. Ecology 95, 882–896. doi: 10.1890/13-1434.1
Stewart J. D., Weller D. (2021). Abundance of Eastern North Pacific Gray Whales 2019/2020 (San Diego, CA: NOAA/NMFS).
Sumich J. L. (1983). Swimming Velocities, Breathing Patterns, and Estimated Costs of Locomotion in Migrating Gray Whales, Eschrichtius Robustus. Can. J. Zoology. 61, 647–652. doi: 10.1139/z83-086
Swartz S., Taylor B., Rugh D. J. (2006). Gray Whale Eschrichtius Robustus Population and Stock Identity. Mammal. Rev. 36, 66–84. doi: 10.1111/j.1365-2907.2006.00082.
Torres L. G., Nieukirk S. L., Lemos L., Chandler T. E. (2018). Drone Up! Quantifying Whale Behavior From a New Perspective Improves Observational Capacity. Front. Mar. Sci. 5. doi: 10.3389/fmars.2018.00319
Torres L. G., Smith T. D., Sutton P., Macdiarmid A., Bannister J., Miyashita T. (2013). From Exploitation to Conservation: Habitat Models Using Whaling Data Predict Distribution Patterns and Threat Exposure of an Endangered Whale. Diversity Distrib. 19, 1138–1152. doi: 10.1111/ddi.12069
Torres W. I., Bierlich K. (2020). MorphoMetriX: A Photogrammetric Measurement GUI for Morphometric Analysis of Megafauna. J. Open Source Softw. 5 (45), 1825.
Urbán R. J., Weller D., Martínez A. S., Tyurneva O., Bradford A., Burdin A. M., et al. (2019). New Information on the Gray Whale Migratory Movements Between the Western and Eastern North Pacific (Cambridge, UK: Scientific Committee of the International Whaling Commission).
Villegas-Amtmann S., Schwarz L., Gailey G., Sychenko O., Costa D. (2017). East or West: The Energetic Cost of Being a Gray Whale and the Consequence of Losing Energy to Disturbance. Endanger. Species. Res. 34, 167–183. doi: 10.3354/esr00843
Weller D. W., Klimek A., Bradford A. L., Calambokidis J., Lang A. R., Gisborne B., et al. (2012). Movements of Gray Whales Between the Western and Eastern North Pacific. Endanger. Species. Res. 18, 193–199. doi: 10.3354/esr00447
Williams R., Vikingsson G. A., Gislason A., Lockyer C., New L., Thomas L., et al. (2013). Evidence for Density-Dependent Changes in Body Condition and Pregnancy Rate of North Atlantic Fin Whales Over Four Decades of Varying Environmental Conditions. ICES. J. Mar. Sci. 70, 1273–1280. doi: 10.1093/icesjms/fst059
Keywords: body condition, drones/UAS, ecosystem variability, photogrammetry, population health, prey availability, uncertainty, whales
Citation: Torres LG, Bird CN, Rodríguez-González F, Christiansen F, Bejder L, Lemos L, Urban R J, Swartz S, Willoughby A, Hewitt J and Bierlich KC (2022) Range-Wide Comparison of Gray Whale Body Condition Reveals Contrasting Sub-Population Health Characteristics and Vulnerability to Environmental Change. Front. Mar. Sci. 9:867258. doi: 10.3389/fmars.2022.867258
Received: 31 January 2022; Accepted: 21 March 2022;
Published: 19 April 2022.
Edited by:
Olaf Meynecke, Griffith University, AustraliaReviewed by:
Sue Moore, University of Washington, United StatesDavid W Weller, NOAA Fisheries, Southwest Fisheries Science Center, United States
Copyright © 2022 Torres, Bird, Rodríguez-González, Christiansen, Bejder, Lemos, Urban R, Swartz, Willoughby, Hewitt and Bierlich. This is an open-access article distributed under the terms of the Creative Commons Attribution License (CC BY). The use, distribution or reproduction in other forums is permitted, provided the original author(s) and the copyright owner(s) are credited and that the original publication in this journal is cited, in accordance with accepted academic practice. No use, distribution or reproduction is permitted which does not comply with these terms.
*Correspondence: Leigh G. Torres, TGVpZ2guVG9ycmVzQG9yZWdvbnN0YXRlLmVkdQ==