- 1College of Marine Sciences, Shanghai Ocean University, Shanghai, China
- 2Third Institute of Oceanography, Ministry of Natural Resources, Xiamen, China
- 3School of Ecology and Nature Conservation, Beijing Forestry University, Beijing, China
- 4College of the Environment and Ecology, Xiamen University, Xiamen, China
- 5Observation and Research Station of Island and Coastal Ecosystems in the Western Taiwan Strait, Ministry of Natural Resources, Xiamen, China
Fisheries management policies (FMPs) have been implemented in coastal countries to ensure a sustainable supply of seafood and the recovery of species diversity. Because of the depletion of fishery stocks, China has introduced a series of FMPs since 1995, including a seasonal fishing moratorium (SFM), a zero-growth strategy, and a minimum mesh size for fishing nets. Here, we built two mass balance models for 1997–2000 (M1997) and 2018–2019 (M2018) using Ecopath with Ecosim 6.6 to illustrate the interannual changes over the past two decades in the East China Sea (ECS). We then simulated two dynamic scenarios from 1997 to 2018, SFM (M2018SFM) and no SFM (M2018no-SFM), to test the role of the SFM under fishing pressure in the ECS. Ecopath showed that the ECS ecosystem is becoming more mature, although it is still unstable, featuring lower total primary production/total respiration, longer cycles, faster organic material circulation speed, and a higher omnivorous degree. This suggests a slow recovery for the ECS ecosystem in the past two decades. The biomass of fish in the ECS—especially the planktivores, dominated by small-sized Benthosema pterotum—significantly increased in M2018 versus M1997, but there were fewer medium- and large-sized fish. The keystone species switched from the planktivores/piscivores dominated by Decapterus maruadsi in M1997 to planktivores in M2018. Ecosim illustrated that the SFM has positive effects on fishery resources recovery, especially for commercial fishes (i.e., large yellow croakers and hairtails), as reflected by the significantly higher predicted biomass of fish in M2018SFM compared to M2018no-SFM and M1997, although the bioaccumulation was consumed by the intense fishing pressure after the SFM. However, the M2018SFM prediction for nektons was still lower than the actual value, especially for planktivores, which display a sharp increase in biomass. This should be partly attributable to the policy of the minimum mesh size (<5 cm was banned), which benefits B. pterotum due to its 3.5 cm maximum body size. Therefore, a series of FMPs, rather than only the SFM, functioned together in the ECS ecosystem. However, the mixed trophic impact indicated a negative impact if the fisheries were further developed. Fishery management in the ECS needs to be strengthened by extending the SFM and reducing fishing pressure after the SFM.
1 Introduction
Aquatic products are a primary protein source for humans (Lira et al., 2021). As such, global marine capture production had reached 92.51 million tons by 2017, with an average increase of 10-fold relative to 1950, resulting in the catch per unit effort declining by 50% to 80% (Zhou et al., 2015; Link and Watson, 2019). Therefore, to ensure a sustainable supply of seafood and the recovery of species diversity, fisheries management policies (FMPs) such as total allowable catches, individual transferable quotas, seasonal and area closures, and stock assessments have been widely implemented by coastal countries (Bromley, 2005; Fulton et al., 2014). Similarly, China has introduced a series of FMPs since 1995 that include zero and minus growth targets, a seasonal fishing moratorium (SFM), a minimum mesh size for fishing nets, and a minimum catch size for fishing targets (Cao et al., 2017). There has also been a ban on destructive fishing methods, the construction of artificial fish reefs, and the release of some commercial fish (i.e., Larimichthys crocea) to restore the disturbed marine ecosystem (Han, 2018; Xin et al., 2020).
Fisheries management policies (FMPs) have received considerable critical attention for their protective effect on resource recoveries. Many studies have reported the positive effects of FMPs. For example, Yue et al. (2015) found that after the SFM, the catch increased in the East China Sea (ECS) and the South China Sea. Wang et al. (2020) found that the reduction of fishermen, fishing vessels, and catches all had a positive effect on the recovery of fishery stocks in the Pearl River Delta. Lee and Midani (2014) also showed that the catch per unit effort of sandfish nearly doubled from 2005 to 2011 in the East Sea of Korea after the implementation of a fishing stock-rebuild plan. However, some studies have found that the effectiveness of these fishery strategies had a spatiotemporal limit. Yan et al. (2019a) concluded that in the ECS, the accumulation of biomass during the SFM was rapidly removed by the subsequent intense fishing pressure. In addition, the Fujian fishery statistical yearbook shows that the landing of large yellow croaker (L. crocea) has been maintained at a low level since 2000, although millions of L. crocea larvae have been released (Wu et al., 2021). Di Franco et al. (2009) also reported no differences in the fish assemblages between partially protected areas and a location outside the marine protected area in northeast Sardinia (Italy).
The ECS is one of the most important fishing areas, providing about 40% of the total catch in China (Zhang et al., 2018). In the 1990s, the number of fishing vessels in this region exceeded 100,000 and accounted for nearly half of the total fishing vessels in the China Sea (Shi, 1995). Under such intense fishing pressure, the ECS ecosystem had already been overloaded. Many traditional commercial fish stocks such as the large yellow croaker were exhausted, and the length of the fishing season has declined in some fishing grounds (Liu et al., 2012; Mei, 2019; Xu et al., 2021). Similarly, much uncertainty remains about whether the FMPs in the ECS work in the long term. For the ECS ecosystem, FMPs such as the minimum mesh size (Tokai et al., 2019), zero and minus growth targets (Ye and Rosenberg, 1991), SFM (Cheng et al., 2004), and release enhancement (Lü et al., 2008) have been recognized to be the major drivers rebuilding the fishery resources (Shih et al., 2009; Shen and Heino, 2014; Zhou et al., 2019). However, the previous evaluations of FMPs in the ECS have been focused at the short-term (Yan et al., 2019b), single-policy (Liu and Cheng, 2015; Yue et al., 2015), or species level (Xu and Liu, 2007) rather than at the long-term, multiple-policy, or ecosystem level. Therefore, whether or not FMPs, especially the SFM and reduced fishing pressure, drove the variances in the structure and function of the ECS ecosystem over the last 20 years needs to be further verified.
Ecopath with Ecosim (EwE), a widely used tool to support ecosystem-based fisheries management, prioritizes the ecosystem rather than a single species population (Pikitch et al., 2004; Halpern et al., 2008; Surma et al., 2019; Reum et al., 2021), and it can explore the long-term performances of multiple FMPs under different scenarios (Li, 2009; Russo et al., 2017; Papapanagiotou et al., 2020; Wang et al., 2020; Paradell et al., 2021). Therefore, to verify the hypothesis on FMPs’ effects, this work attempted to estimate the interannual variation of the ECS ecosystem from 1997 to 2018 with EwE and explored the long-term effects of the SFM under the actual fishing pressures present during the two decades. We constructed two mass balance models for 1997–2000 (referred to as M1997) and 2018–2019 (referred to as M2018) in the ECS, to depict the variation in the ecosystem’s structure and function over the past two decades. We further conducted scenario simulations based on the M1997 model to evaluate the contribution of the SFM under fishing pressure to the variations of the ECS ecosystem during the two decades. These results reveal the combined effects of the SFM and fishing pressures on the rehabilitation of the structure and function of the ECS ecosystem and offer advice on the management of fishery resources in the ECS for governmental policymakers.
2 Materials and Methods
2.1 Study Area
The ECS is located in the western Pacific Ocean and is connected with the Sea of Japan through the Tsushima Strait and with the South China Sea through the Taiwan Strait (Figure 1; Li and Zhang, 2012). It is influenced by the Kuroshio Current and dilute water from the Yangtze River. The ECS is one of the most productive regions globally, leading to many important fishing grounds such as the Zhoushan and Minzhong (Liu, 2013). The ECS has experienced three periods associated with dramatically increased fishing equipment and changes in fishing methods: slow growth (1951 to the 1990s), rapid growth (1991 to the 2000s), and high yield (after the 2000s; Chen et al., 1997; Mei, 2019). High-intensity fishing pressure has brought immense economic benefits, but it has also driven a great change in the catch composition from the ECS (Chen et al., 2004).
The SFM, which can offer a suitable time for adult spawning and larvae growth, is considered the utmost protection to rebuild fish stocks (Su et al., 2019). Initially, it was implemented in the northern ECS (27°N to 35°N) from 1 June to 31 August in 1995. During this period, only the trawl and sailing nets were banned. In 2017, all fishing gear with the exception of hooks and lines was banned in the ECS from noon on 1 May to noon on 16 September (Yan et al., 2019a). A minimum mesh size for nets was implemented in 2004 to protect recruitment. In 2017, the Ministry of Agriculture and Rural Affairs announced a minimum allowable size for 15 marine economic fish species. A series of FMPs were also introduced to reduce the fishing pressure, including zero and minus growth targets, a licensing system, a vessel buyback program, dual control, and a fishermen relocation program. The Ministry of Agriculture designed the zero and minus growth system in 1999 and the fishing quota management system based on the maximum sustainable yield in 2000. In 2017, species quota fishing was implemented in the Zhejiang and Shandong provinces (Mei, 2019). The licensing system, vessel buyback program, dual control, and fishermen relocation program were also implemented after 2002 (Cao et al., 2017).
2.2 Ecopath Model Construction and Parameterization
Ecopath offers a static snapshot that reflects the structure and function of the ecosystem at a specific time. The Ecopath model is based on a set of linear equations for each function group in the system. It is formed by the food consumption equation and energy theory (Polovina, 1984; Ulanowicz, 1986). Ecopath needs the following input parameters: biomass (B), the production/biomass ratio (P/B), the consumption/biomass ratio (Q/B), diet composition (DC), and ecotrophic efficiency (EE). Usually, only three of the four groups of parameters need to be entered, and the model can then automatically obtain the fourth parameter. The EE is difficult to obtain, and thus, the other three parameters are usually entered (Christensen et al., 2005).
The basic equation is
where (P/B)i and Bi are the production/biomass ratio and biomass of group i, respectively; (Q/B)j is the consumption/biomass ratio of group j, DCji is the proportion of the diet that predator group i obtains from prey group j, EEi is the ecotrophic efficiency, Bi (1 - EEi) is other mortality, Yi is the catch of group i, Ei is the net migration of group i, and BAi is the biomass accumulation rate for i.
To simplify the trophic structure of the ecosystem, species with a similar ecological niche were aggregated in one function group (Christensen et al., 2005). The function group can also be composed of a single species or the different age structures of a species (Zheng et al., 2020; Lin et al., 2021). There were 24 function groups in the ECS ecosystem, including phytoplankton, zooplankton, polychaetes, mollusks, benthic crustaceans, echinoderms, other invertebrates, crabs, shrimps, cephalopods, planktivores, benthivores, piscivores, planktivores/piscivores, planktivores/benthivores, benthivores/piscivores, omnivores, sharks, marine mammals, and detritus (Table S1). In addition, large yellow croakers, small yellow croakers, hairtails, and Bombay duck were treated as separate function groups due to their high economic values and resources.
The biomass data were collected from field surveys and published literature. Data for the first model was from a 1997–2000 marine living resources supplementary survey and resource evaluation. The second model data were from a joint survey in the ECS in the autumn of 2018 and the spring of 2019. The P/B and Q/B values were obtained using empirical equations and from published literature (Palomares and Pauly, 1989; Pauly et al., 1990; Christensen et al., 2005; Cheng et al., 2009; OuYang and Guo, 2010; Li and Zhang, 2012). The diet data were estimated based on stomach content analyses in the published literature (Tables S2, S3). The food matrix of the function groups was calculated based on the biomass weight of each species in the function group. We used similar diet matrices in the M1997 and M2018 models but made slight changes in several groups, e.g., hairtails, piscivores, and planktivores. Information on catch was obtained from the China Fishery Statistical Yearbook (CFSY), but there was no discard data in the two models due to a lack of data.
2.2.1 Ecological Indicators
Ecological indicators, which can be used as measures to assess ecosystem status, are included and presented along with other Chinese models and models at similar latitudes. The Finn’s cycling index indicates the speed of organic material circulation in the ecosystem, and the mean path length represents the total number of trophic links divided by the number of pathways (Finn, 1976; Christensen et al., 2005). The connectivity index reveals the interaction between species in terms of predation, and the system omnivory index (SOI) is defined as the average omnivorous degree of the consumers (Nee, 1990; Pauly and Christensen, 1993). These indicators are linked with the maturity of the ecosystem (Ulanowicz, 2012). Total system throughput (TST) sums all flows in this model according to Ulanowicz (2012). Mixed trophic impact (MTI) can reveal a direct or indirect influence of one function group on another function group, which can explain the relationship between groups in the ecosystem (Ulanowicz and Puccia, 1990). The keystone index can identify the key species of the ecosystem by selecting indexes greater than zero. The keystones play a primary role in maintaining stability and complexity and have a disproportionate impact on biomass (Libralato et al., 2006).
2.2.2 Model Balance and Sensitivity Analysis
After all data were input, the EE should be below 1, and most of the gross efficiency values should be between 0.1 and 0.3, with the exception of some fast-growing organisms (Christensen et al., 2005). Pedigree and a sensitivity analysis were used to verify the reliability of the model. The pedigree can mark the source and calculate the credibility of the input data (Majkowski, 1982; Funtowicz and Ravetz, 1990). It is also the reference used to adjust the parameters of the model. The parameter with the lowest confidence would thus be adjusted when the model is unbalanced (Funtowicz and Ravetz, 1990). The sensitivity analysis can evaluate the uncertainty of the output data of the model when the input data fluctuate. The sensitive analysis routine was set to ±20% uncertainty for all input parameters (Han et al., 2017).
2.3 Dynamic Simulation
The Ecosim model conducts a temporal dynamic analysis with key original parameters from the Ecopath model, which is used as a reference to estimate changes in the biomass of function groups driven by time series data (Christensen et al., 2005). In the modeling framework, a series of differential equations that consider predator–prey interactions and foraging behaviors inherent to Ecopath can be expressed as follows (Christensen et al., 2005):
where dBi/dt is the change in the biomass of group i over time, (P/Q)i is the net growth efficiency, Mi is the non-predation mortality rate, Fi is the fishing mortality rate, ei is the emigration rate, Ii is the immigration rate, Bi is the biomass of group i, Σj Qji is the total consumption rate by group i, and ΣjQij is the predation by all predators on the same group i.
The Ecosim incorporated historical data, including biomass, catches, and fishing mortalities for different function groups to facilitate accurate model predictions. The partial biomass time series calculated for hairtails, small yellow croakers, and piscivores were derived from the SAU database (http://www.seaaroundus.org/). For most nektonic groups, the time series on absolute biomass, catch, and fishing mortalities were obtained from the CFSY and China’s offshore marine comprehensive survey and evaluation project. The time series Chl-a was from the dataset of Aqua MODIS and SeaWiFS and was used to calibrate primary production anomalies. Once the time series data (Table S4) were included in the model, the fit model with the lowest Akaike information criterion value was selected (Burnham and Anderson, 2004). The vulnerabilities (v), which represent the impacts of predator biomass for a given prey, are an important parameter in the process of model fitting to time series data (Christensen et al., 2005). To reduce human error and obtain actual v in the calibrating process, the automated “stepwise fitting” procedure was used (Scott et al., 2016).
As mentioned, a series of FMPs have been implemented in the ECS since the 1990s (Cao et al., 2017). However, in this study, we only evaluated the impacts of the SFM under actual fishing pressure (Figure S1) on the recovery of fishery resources. Then, two Ecosim models that did or did not integrate the SFM under the actual fishing pressures were built, which are expressed by M2018SFM and M2018no-SFM. Because the annual landing in Zhoushan (Zhejiang province) can account for 20~40% of the total landing in the ECS (http://zstj.zhoushan.gov.cn/)—there was significant correlation for landing between the ECS and Zhoushan (r = 0.628, p < 0.05)—the relative fishing effort of every month in the ECS was assumed to be consistent with that in the Zhoushan in M2018SFM. In addition, we also employed the fleet data in Global Fishing Watch (https://globalfishingwatch.org/data-download/datasets/public-fishing-effort) to fit the actual fishing effort in the ECS (Table S5), whereas the fishing effort of every month in a year was assumed to be the same in the M2018no-SFM model. Although a minimum mesh size could be beneficial to the juveniles of nektonic groups by selecting suitable lengths for the species (Heikinheimo et al., 2006; Nguyen et al., 2021), the model cannot load this policy due to the lack of body length distribution data for the kinds of function groups. Climate change was considered to affect the physiology, distribution, and biomass of the marine species and alter the community composition of the marine ecosystems (Cheung et al., 2013; Kroeker et al., 2013; Zeng et al., 2019). However, it was negligible from 2000 to 2018 in this study, as shown by Zeng et al. (2019) in the Pearl River estuary, where the non-producer biomass decreased by only 5% from 2000 to 2060. Therefore, the variants for climate change (seawater surface temperature, pH, and dissolved oxygen) were not included in the two Ecosim models.
3 Results
3.1 Quality of the Ecopath Model and the Sensitivity Analysis
The basic data and output results for M1997 and M2018 are shown in Table 1. Except for the plankton and high-trophic groups, the EE of most function groups was close to 1, suggesting that there was a high utilization rate of most groups in the ECS. The gross efficiency was almost in the 0.1–0.3 range. Finally, the pedigrees of the two Ecopath models were both 0.497, which is a reasonable interval; 0.16–0.68 is the range of pedigrees for most EwE models (Morissette, 2007), indicating that the quality of our Ecopath models was acceptable.
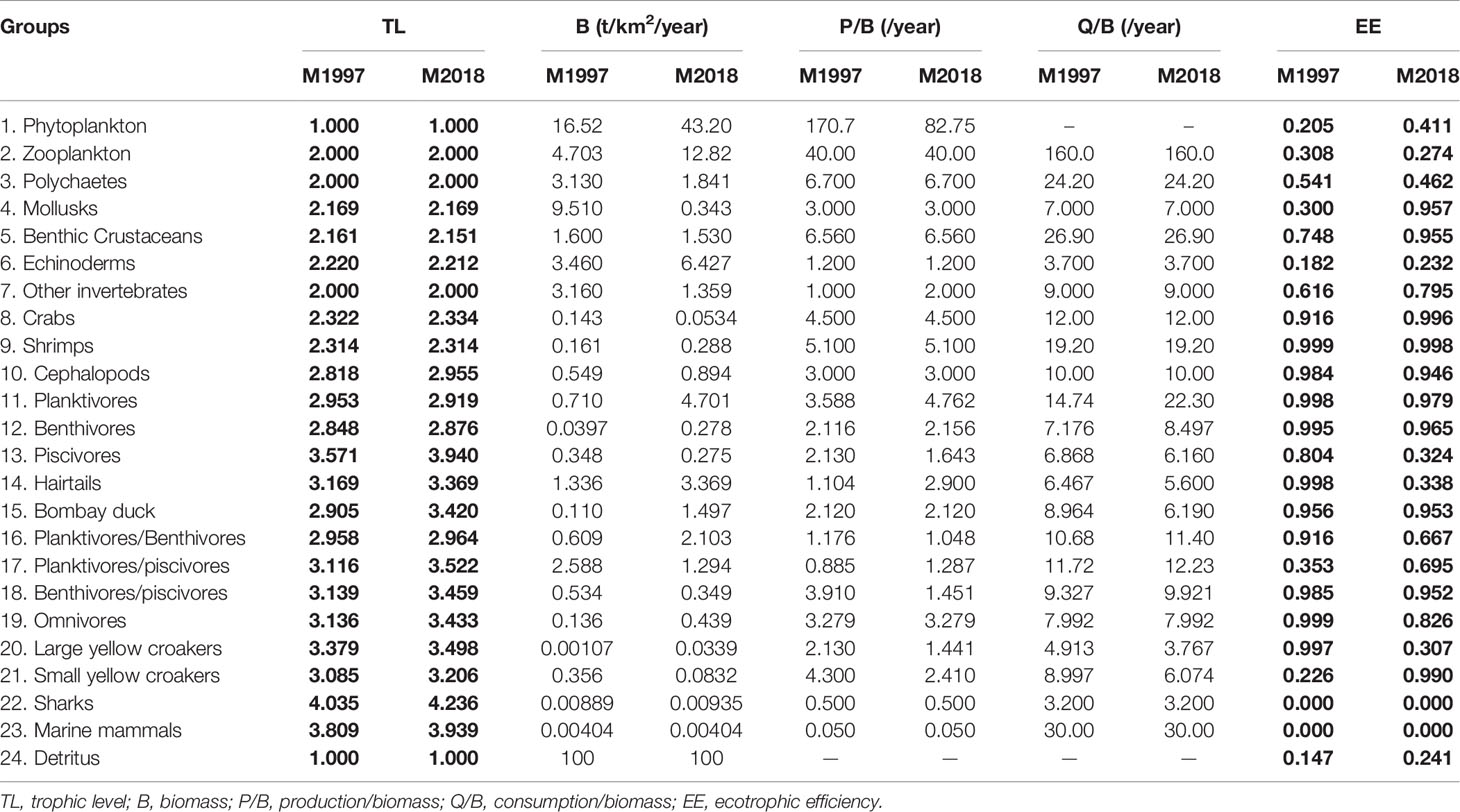
Table 1 Input and output (bold) parameters for the East China Sea ecosystem mass balance models M1997 (1997–2000) and M2018 (2018–2019).
A sensitivity analysis was used to evaluate the influence of the variation in input data on the output results. A similar pattern was observed in the two models (Figure 2). The biomass of the function groups appeared to be the most influential parameter, with a range of ±30%. The diet appeared to be the least influential parameter on the two balanced models, which is consistent with observations by Han et al. (2017). This further indicated that adjusting the food matrix had the lowest impact on the overall model output.
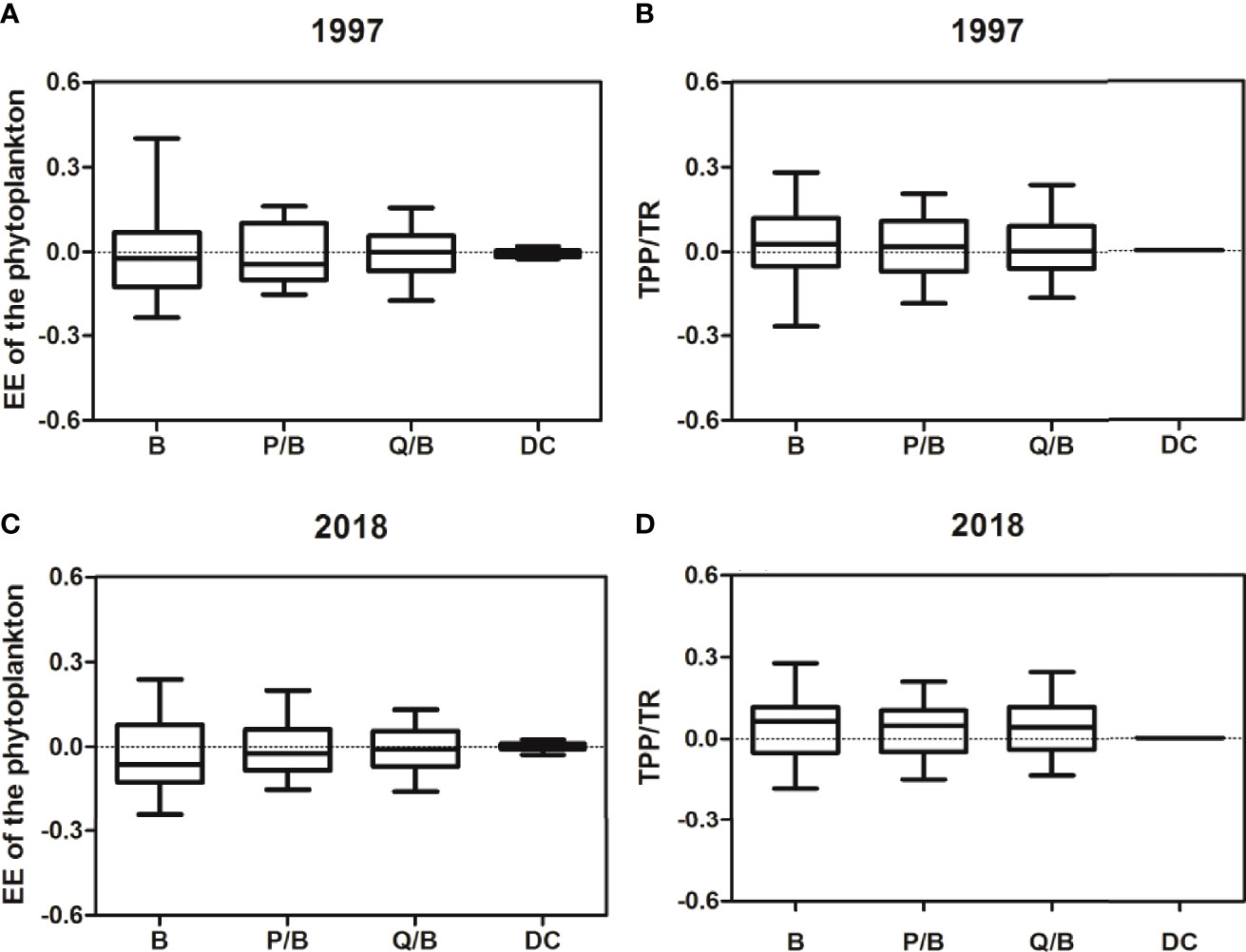
Figure 2 The sensitivity analysis results for the 20% uncertainty of the Ecopath input parameters. TPP, total primary production; TR, total respiration; EE, ecotrophic efficiency; B, biomass; P/B, production/biomass; Q/B, consumption/biomass; DC, diet composition. The sensitivity analysis results for the 20% uncertainty of the Ecopath input parameters. TPP, total primary production; TR, total respiration; EE, ecotrophic efficiency; B, biomass; P/B, production/biomass; Q/B, consumption/biomass; DC, diet composition. (A) represents the impact of the input parameters (B, P/B, Q/B, DC) with 20% uncertainty on the EE of the phytoplankton (M1997); (B) represents the impact of the input parameters (B, P/B, Q/B, DC) with 20% uncertainty on the TPP/TR (M1997); (C) represents the impact of the input parameters (B, P/B, Q/B, DC) with 20% uncertainty on the EE of the phytoplankton (M2018); (D) represents the impact of the input parameters (B, P/B, Q/B, DC) with 20% uncertainty on the TPP/TR (M2018).
3.2 The 20-Year Change in the East China Sea Ecosystem
The flow diagram for the ECS showed similar trophic levels (TLs) during the two periods, from 1.00–4.05 and 1.00–4.24 in M1997 and M2018, respectively (Table 1 and Figure S2). The mean TLs of caught fishes showed a slight increase, from 3.11 in M1997 to 3.33 in M2018. Transfer efficiency was 10.56% in the M2018 model, which was less than the M1997 model (12.01%) but close to the theoretical ranges (10%; Christensen and Pauly, 1992). The TST developed further, from 6503.85 t/km2/year to 8925.33 t/km2/year in the two decades, with detritus flow accounting for 33.33% in M2018 and 40.36% in M1997 (Table 2). The consumption and respiration in the TST significantly increased by 14.33% and 5.77% in M2018, respectively, compared to M1997 (Table 2).
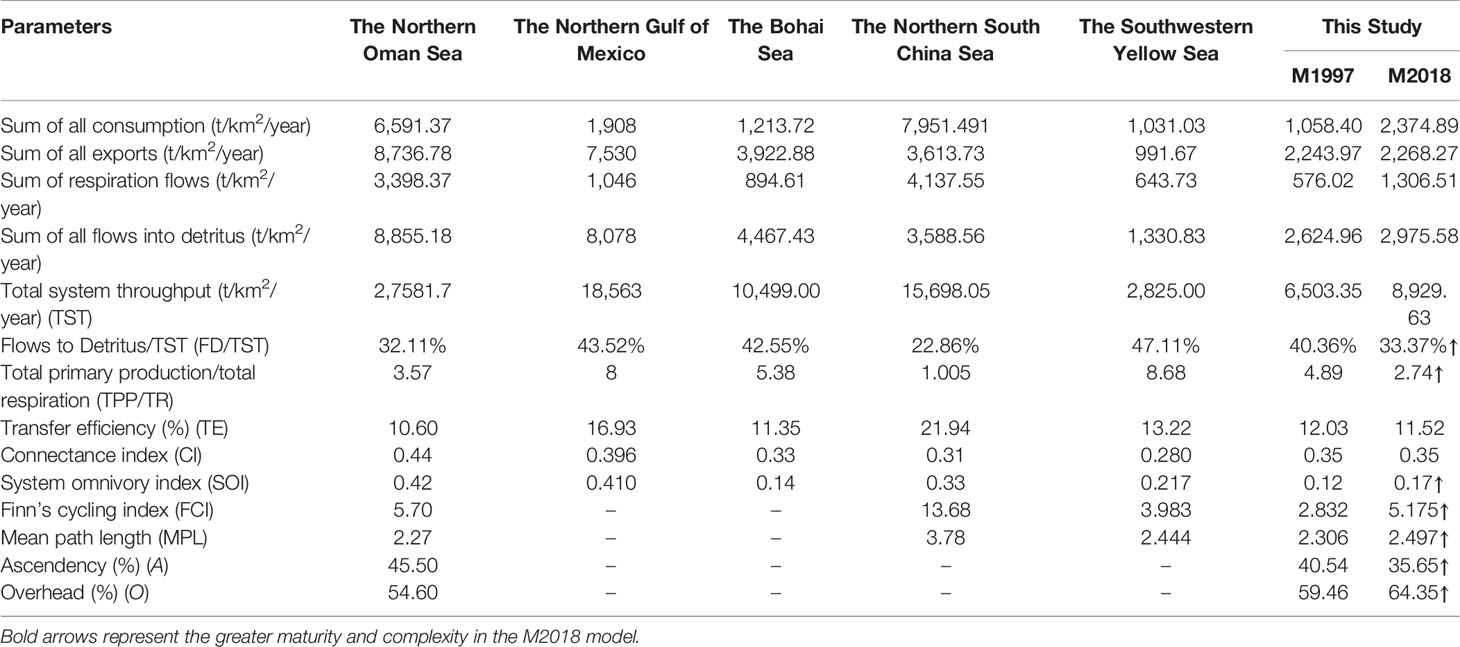
Table 2 Comparison of ecosystem indicators for the East China Sea Ecopath mass balance models M1997 (1997–2000) and M2018 (2018–2019) with other available Ecopath models in adjacent waters (Bohai, Northern South China, and Southwestern Yellow Seas) and in other seas at similar latitudes (Northern Oman Sea and Northern Gulf of Mexico).
Total biomass increased considerably, from a value of 49.72 t/km2/year in M1997 to 83.19 t/km2/year in M2018 (Figure 3). The biomass of the plankton and fish in M2018 was nearly doubled relative to M1997, especially the planktivores, whose biomass in M2018 increased nearly seven times that in M1997. However, obvious and significant downtrends were noted in the benthic organisms, especially mollusks.
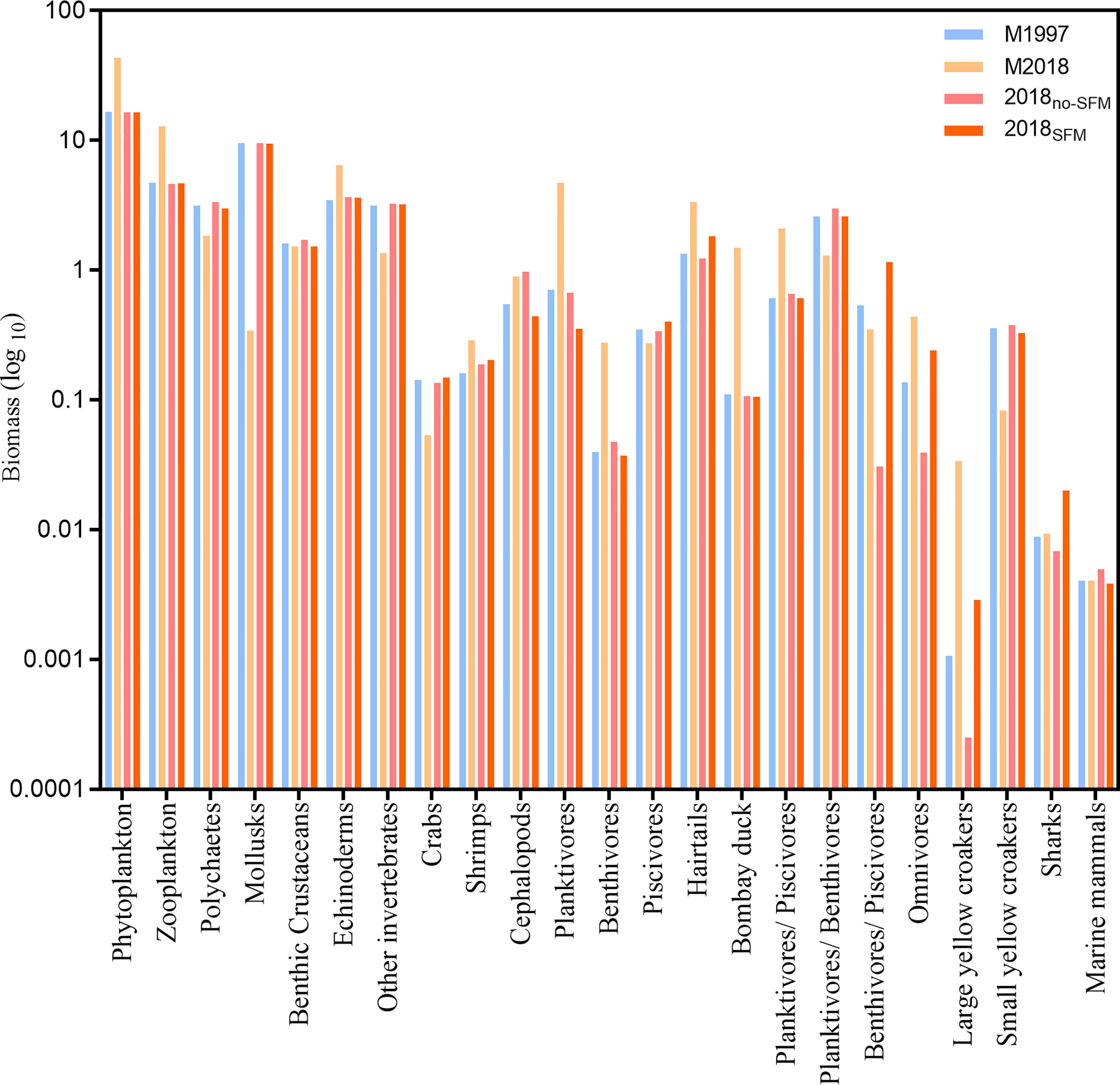
Figure 3 Biomass of the function groups in the East China Sea ecosystem in the M1997 (1997–2000) and M2018 (2018–2019) mass balance models and the M2018no-SFM (no seasonal fishing moratorium) and M2018SFM (with a seasonal fishing moratorium) dynamic simulations (1997–2018).
There was a major shift in the keystone indexes of the function groups in the two models (Figure 4). Several groups increased, including the benthic crustaceans, Bombay duck, large yellow croakers, sharks, and planktivores. The keystone species in the ECS changed from planktivores/piscivores to planktivores. Zooplankton and planktivores/piscivores (e.g., Decapterus maruadsi and Trachurus japonicus) were the keystone species revealed by the keystone indexes close to zero in M1997 (−0.0355 and −0.0711, respectively), and they had the largest influence on the ecosystem structure. However, piscivores, hairtails, and small yellow croakers decreased in importance during the study period.
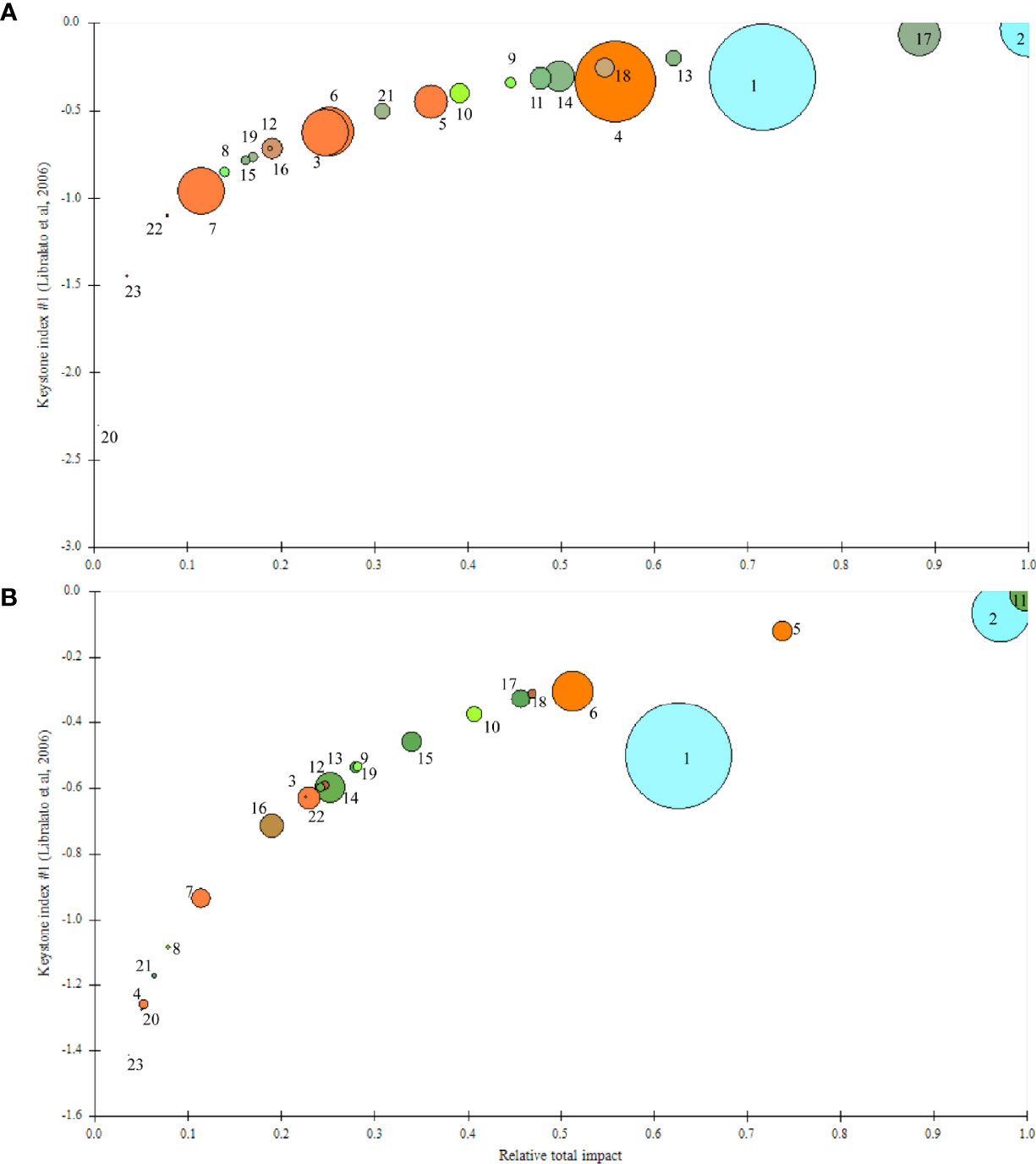
Figure 4 Keystoneness index and overall effects of each function group from the East China Sea ecosystem in the (A) M1997 (1997–2000) and (B) M2018 (2018–2019) mass balance models. 1, Phytoplankton; 2, Zooplankton; 3, Polychaetes; 4, Mollusks; 5, Benthic crustaceans; 6, Echinoderms; 7, Other invertebrates; 8, Crabs; 9, Shrimps; 10, Cephalopods; 11, Planktivores; 12, Benthivores; 13, Piscivores; 14, Hairtail; 15, Bombay duck; 16, Planktivores/Benthivores; 17, Planktivores/Piscivores; 18, Benthivores/Piscivores; 19, Omnivores; 20, Large yellow croakers; 21, Small yellow croakers; 22, Sharks; 23, Marine mammals; 24, Detritus.
The MTI showed the increasingly negative impact of fisheries in the ECS between M1997 and M2008 (Figure 5). In M1997, 36% of the function groups such as large yellow croakers, piscivores, and benthivores/piscivores were negatively affected by the fisheries. The positive impacts on the planktivores and benthivores (around 24%) were ascribed to the indirect effect of removing predators. In M2018, the negative impact of the fisheries further extended to small yellow croakers and omnivores. In addition, the MTI of the fisheries on important fish species such as hairtails and large yellow croakers slightly increased in M2018 relative to M1997, from −0.295 to −0.034 for hairtails and from −0.729 to −0.516 for large yellow croakers. Small yellow croakers had opposite values, 0.0635 in 1997 and −0.395 in 2018. However, the impact of the fisheries on Bombay duck appeared neutral over the two periods due to the limited and poor fishery data.
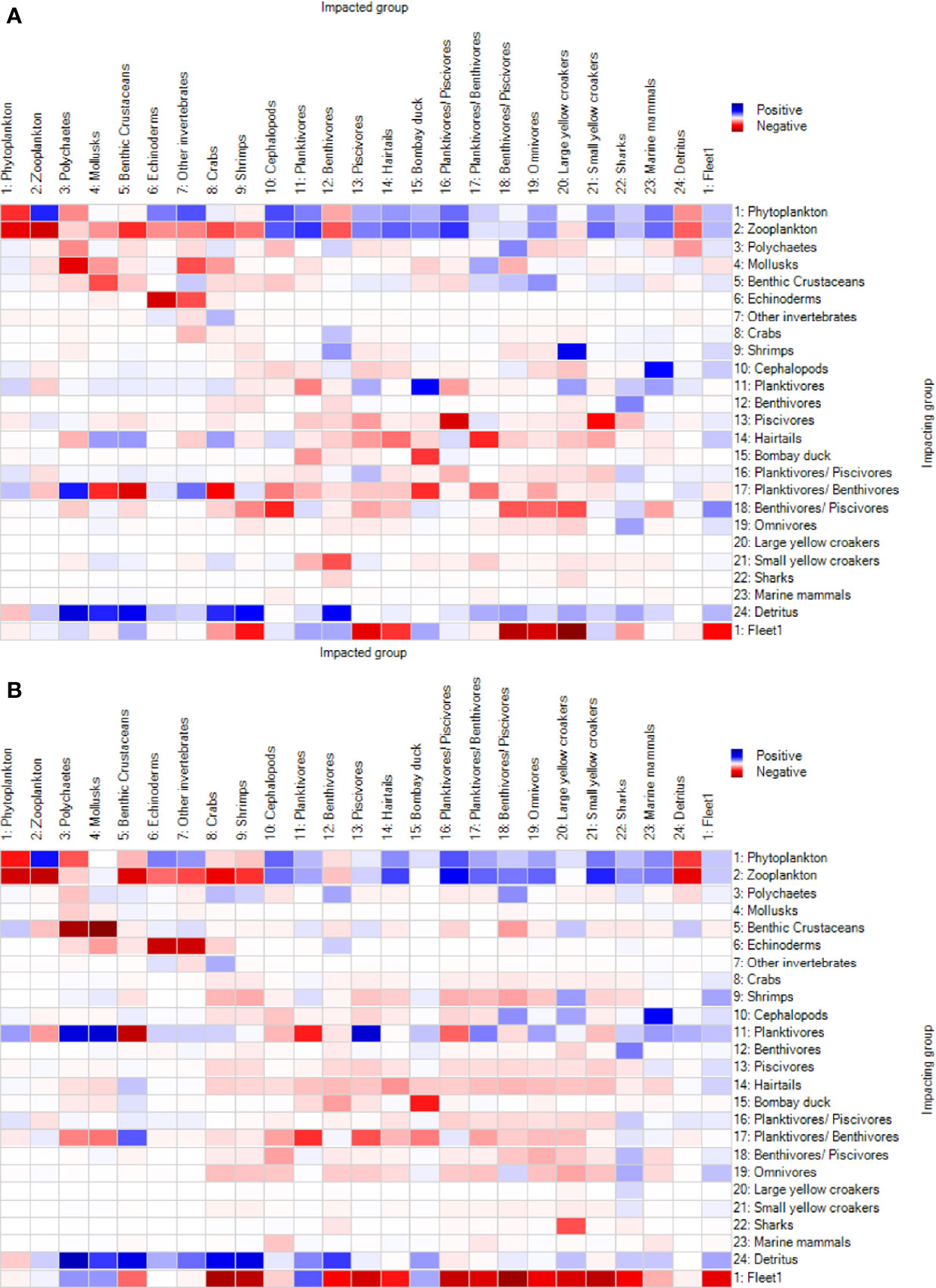
Figure 5 Mixed trophic impacts of the function groups in the East China Sea ecosystem in the (A) M1997 (1997–2000) and (B) M2018 (2018–2019) mass balance models.
Ecosystem indicators were used to evaluate the status and variation of the structure and function of the ecosystem (Table 2). The ECS ecosystem was more mature and stable in M2018, as reflected by the higher total primary production/total respiration (TPP/TR) and SOI as well as by the lower Finn’s cycling index and mean path length (Table 2). The TPP/TR declined from 4.89 to 2.74 between M1997 and M2018. The Flow to Detritus/TST was 33.33% in M2018 and 40.36% in M1997, indicating that more energy flowed into production rather than detritus. Ascendency decreased from 40.54% to 35.65% from 1997 to 2018, and overhead increased from 59.46% to 64.26%, suggesting that the ECS ecosystem was more robust to resist external disturbance. In addition, the Finn’s cycling index values approximately doubled from 1997 to 2018, and there was a slight increase in mean path length (from 2.31 in M1997 to 2.50 in M2018). This further implied an increase in the proportion of material recycling. The same performance was also observed in the SOI. Compared with M1997, the SOI increased from 0.13 to 0.18, whereas the connectivity index remained constant between M1997 and M2018. In summary, a series of ecosystem indicators showed that the maturity and stability of the ECS ecosystem in 2018 had further developed. However, the ECS is still a developing ecosystem with a mass of unused energy.
3.3 Effect of the Seasonal Fishing Moratorium
The optimal model for the stepwise fitting process based on time series data was selected by the lowest Akaike information criterion for important economic species, in which the v values were caught (Table S6 and Figure S3). There were significant differences in the biomasses of the kinds of function groups for the four models. There was a higher biomass of low-TL function groups in M2018no-SFM than in M2018SFM and M2018, i.e., polychaetes, mollusks, and shrimps; conversely, the higher biomass in the high-TL function groups was in M2018SFM, i.e., piscivores and benthivores/piscivores. By linearly fitting the actual biomass in M2018 with the one in M1997 and the predicted biomasses in M2018no-SFM and M2018SFM (Figure 6), we found that the slope for M2018SFM was the highest, followed by M2018no-SFM and M1997. The M2018SFM and M2018no-SFM models could explain 69.87% and 45.39% of the biomass change, respectively. Note that the linear fit excluded planktivores, planktivores/piscivores, and planktivores/benthivores due to the poor prediction quality for plankton organisms in the two Ecosim models.
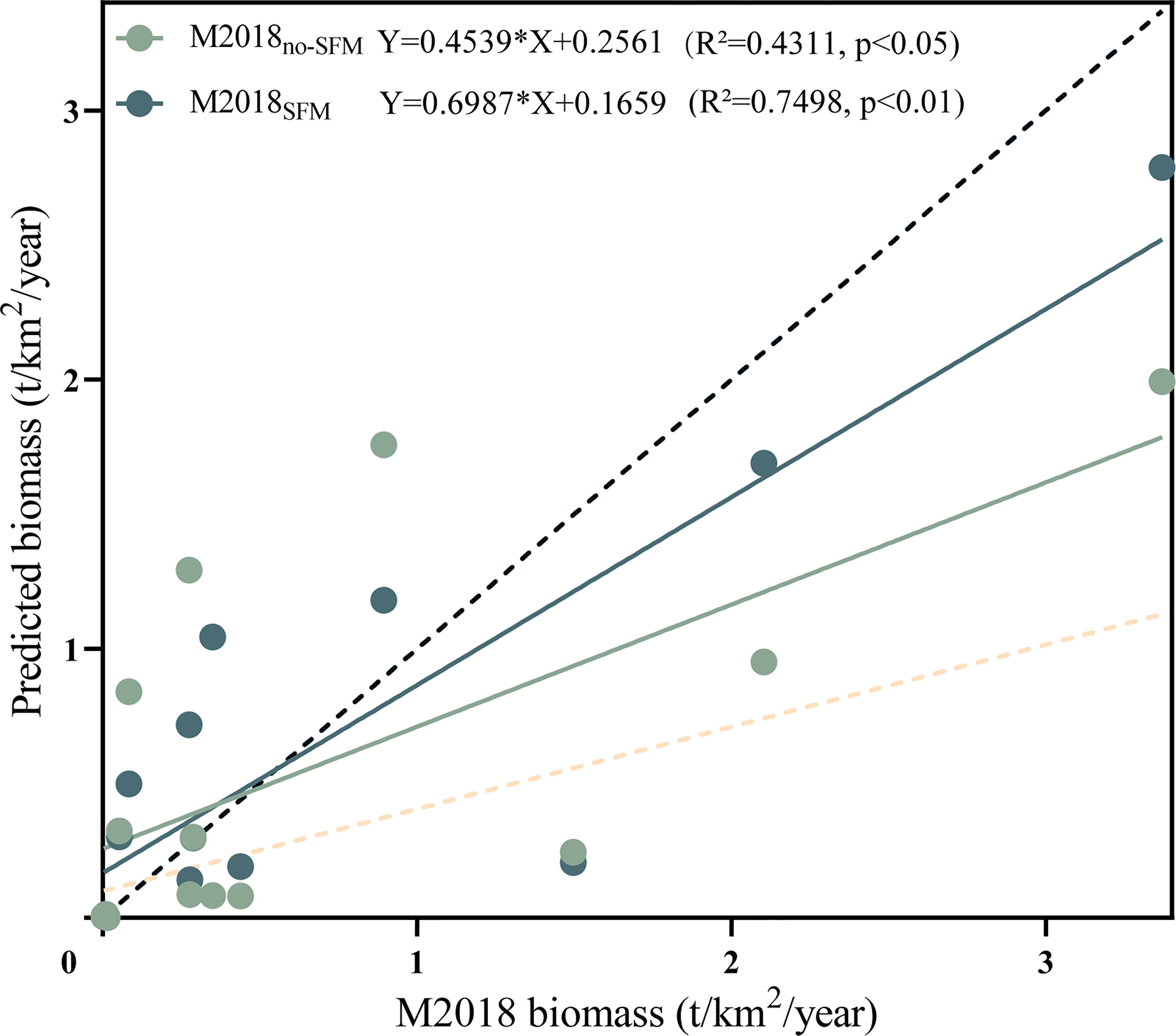
Figure 6 The linear fit of the actual biomass in M2018 (2018–2019) with the ones predicted by the M2018no-SFM and M2018SFM dynamic simulations (1997–2018), which represent the predicted model without and with a seasonal fishing moratorium (SFM), respectively. Dotted lines represent the actual biomasses in M1997 (yellow) and M2018 (black).
Increased biomasses were observed for most function groups during the SFM, but they sharply decreased after the SFM due to the intense fishing intensity (Figure 7). The predicted biomass for most function groups was higher compared to M1997, suggesting reduced fishing pressure has a positive influence on resource recovery. However, discrepancies in the biomasses between function groups were found in the two simulated scenarios. Similar patterns emerged in hairtails, benthivores/piscivores, omnivores, and large yellow croakers (Figures 7G, H, J, L, respectively). In M2018no-SFM, the biomass of these groups declined substantially from that in M1997 but increased dramatically in M2018SFM. This suggests that the SFM facilitates the recovery of these function groups. There were no differences in crabs; shrimps; benthivores, piscivores, and Bombay duck; planktivores/benthivores; and small yellow croakers in the two Ecosim models (Figures 7A, B, D–F, I, K, respectively). The landing of benthivores and Bombay duck was not recorded in the CFSY, so the change in fishing effort provided a limited effect on these groups. The excessive exploitation of piscivores after the SFM led to a similar trend in the two Ecosim models. As to crabs and shrimps, the combined effect of fishing and trophic interaction rapidly removed the bioaccumulation from the SFM and brought similar results in the two scenarios. Although the SFM facilitated the recovery of large yellow croakers and benthivores/piscivores, the small yellow croakers and planktivores/benthivores did not increase in the M2018SFM model. The biomass of the cephalopods was lower in M2018SFM than in M2018no-SFM (Figure 7C), which was mainly due to the stronger feeding pressure from higher TL organisms.
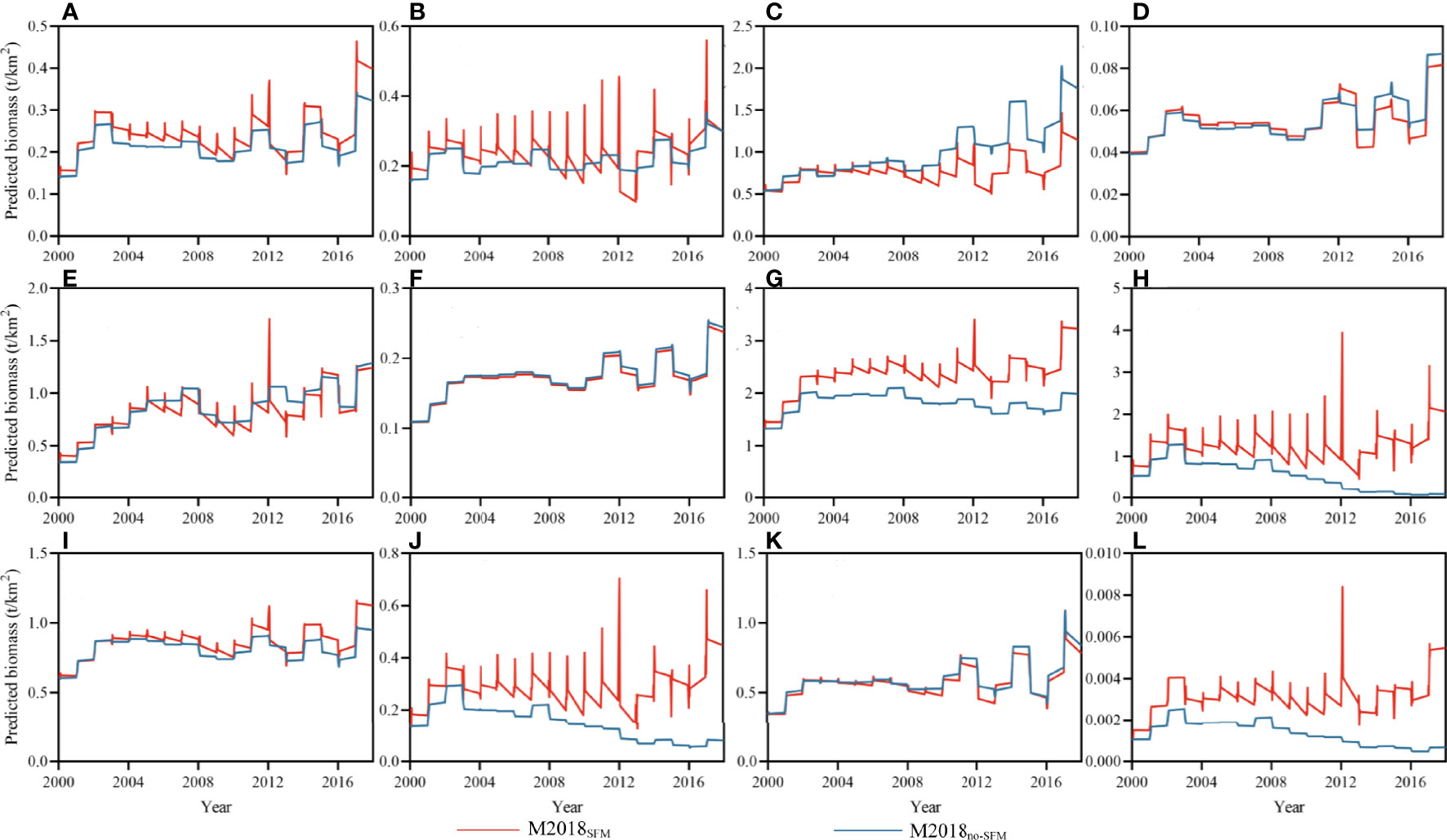
Figure 7 Biomass of important function groups in the M2018no-SFM and M2018SFM dynamic simulations (1997–2018), which are the predicted model without and with a seasonal fishing moratorium, respectively; the peak value in M2018SFM represents the seasonal fishing moratorium. (A) Crabs, (B) Shrimps, (C) Cephalopods, (D) Benthivores, (E) Piscivores, (F) Bombay duck, (G) Hairtails, (H) Benthivores/Piscivores, (I) Planktivores/Benthivores, (J) Omnivores, (K) Small yellow croakers, (L) Large yellow croakers.
4 Discussion
4.1 Analysis of the Variations in the East China Sea Ecosystem Structure
The widely used EwE software provides for the easy implementation of different network indices that can describe the developing status of an ecosystem structure. Our results indicated a 20-year increase in the ecosystem’s maturity and stability after a series of FMPs featuring a higher TST, a lower TPP/TR, longer cycles, faster organic material circulation speed, a higher degree of omnivory in the consumers, and less energy flowing into detritus. Although TST is not necessarily linked with ecosystem status, i.e., degradation or recovery, increased TST means an increase in the “size” of the entire system after a 20-year recovery. The expectation is that there are changes in TST consistent with changes in productivity (Coll et al., 2008b). However, the TPP/TR value indicative of the maturity of the ecosystem in M2018 still reached 2.76. Despite being far less than those of two adjacent seas—the southwest Yellow Sea (Wang et al., 2019) and the Bohai Sea (Lin et al., 2018)—as well as two seas at a comparable latitude, the northern Oman Sea (Tajzadehnamin et al., 2020) and northern Gulf of Mexico (Sagarese et al., 2017), this value was far higher than the 1 that represents a mature status (Christensen et al., 2005) (Table 2). This value was also lower than that for the northern South China Sea (Ma et al., 2020). These data suggest that the ECS ecosystem is still immature and unstable, with much energy that still cannot be utilized directly.
The Ecopath results showed a substantial increase in fish biomass, especially for the planktivores in M2018. These findings are consistent with other studies, where an increase in low-TL fish resulted from implementing FMPs after intensive exploitation (Pitcher, 2001; Vijverberg et al., 2012; Gebremedhin et al., 2021). In particular, the biomass of the planktivores increased from 11.06% of the total fish biomass in M1997 to 34.5% in M2018. This has led to an obvious variation in the species composition and a keystone species change from planktivores/piscivores to planktivores (Figure 4). This variation in keystone species is consistent with findings in the Bohai Sea and the northern South China Sea, where the dominant species were also low-TL organisms such as cephalopods and mollusks (Chen, 2017; Li, 2020). This might be ascribed to the policy on the minimum mesh size. Since 2004, the minimum mesh size of fishing nets in the China Sea has been 5 cm, but B. pterotum, the dominant planktivore species, has only a 3.5-cm maximum body length (Fishbase); thus, this species can benefit from this policy. These low-TL fishes have a short growth period and high reproductive rate that allows them to adapt to intense fishing pressure (Reum et al., 2021). Jiang et al. (2009) also showed that after a fishing moratorium, the community tends to be dominated by fast-growing small groups, which facilitates the expansion of fish in the community. Small fishes also play a considerable role in bridging the low and high TLs (Lira et al., 2021).
The MTI showed the increasingly negative impacts of fishery on the function groups in M2018 versus M1997. The negative effect of fishing activity on large/medium-sized species such as benthivores/piscivores (Pennahia argentatus and Nemipterus virgatus) and piscivores (Scomberomorus niphonius) was obvious in M1997. This negative impact had further developed in M2018. The negative impact of harvesting on piscivores increased due to increased fishing efforts (MTI values declined from −5.30 to −5.81). Moreover, the negative impacts expanded to other groups that had a positive or neutral impact in M1997, including planktivores and small yellow croakers. At this point, the direct harvest effect from fishing exceeds the predator removal effect.
The MTI and keystone index of important commercial fish showed different performances during these two decades. The MTI results showed that the fishery still had a negative impact on hairtails and large yellow croakers over the two periods, but there was a slight increase in the MTI of the fishery. This is also reflected in the keystone index, which had a more important role in the ecosystem. The increase in biomass is responsible for this pattern. Compared with M1997, the biomass of hairtails was tripled and that of large yellow croakers was doubled in M2018. Conversely, the fishing effort for these two commercial fish decreased. Many fish larvae, especially large yellow croakers, have been released into the ECS ecosystem to rebuild the fish stocks (Zhang et al., 2010). However, a significant decrease in the MTI index of small yellow croakers was observed. Fishery landings (according to the SAU database) show that the harvest of small yellow croakers has exceeded the fishing maximum sustainable yield since 2007. Therefore, its keystone index decreased versus that in M1997. In addition, Bombay duck had significant increases in its MTI and keystone index versus other groups, indicating that its ecological roles broadened. Consistent with Liu et al. (2021) and Zhang et al. (2021), Bombay duck has been the dominant species in the ECS, but no catch data were recorded in the CFSY.
4.2 Impact of Fisheries Management Policies on Fishery Stocks in the East China Sea
The SFM simulations illustrated that the SFM in the ECS could increase the short-term fish biomass during the period and play a positive role in the long-term bioaccumulation of fish biomass. Compared with M2018no-SFM, the biomass for most function groups increased in M2018SFM. Consistent with the observations of Yan et al. (2019a), a significant increase in fish biomass was found in 2017, when there was a longer moratorium and fewer fishery landings (Figure 7). However, these efforts were weakened by the intense fishing pressure after the SFM, with the species with high exploitation rates influenced more than others (Chagaris et al., 2020). This result has also been reported in the Western Mediterranean Sea by Samy-Kamal et al. (2015) and in the Visayan Sea (Philippines) by Napata et al. (2020). The fishing effort after the SFM accounted for half of the annual fishing effort, so the effect revealed by the SFM implementation was not significant in the annual biomass survey (Chen et al., 1997; Lu and Zhao, 2015; Yan et al., 2019a).
The SFM led to about a 70% change in biomass, suggesting that there were other factors that promoted the biomass increase in the ECS in M2018. Increased primary production facilitated the biomass of fish communities, which is revealed by a strong linkage between the higher primary production and secondary production of higher-TL organisms or fishery resources (Chassot et al., 2007; Friedland et al., 2012). Compared with M1997, the biomass of the phytoplankton increased approximately three-fold in M2018. Phytoplankton is a basic compartment in an ecosystem to provide food sources for low-TL species and fish larvae (Sun and Liang, 2016). We suspect that the increase in primary production might be due to the high concentrations of dissolved inorganic nitrogen and dissolved inorganic phosphorus and the high rate of N/P from the nearshore (Ehrnsten et al., 2019; Yang et al., 2020). However, nutrient load was not included in the time series data to verify this pattern, and the predicted plankton biomass in the two simulations was lower than the actual in M2018. In addition, increased biomasses for planktivores and large yellow croakers were also observed by Zhai et al. (2020). However, the change in the fishing net mesh size and the release enhancement were not considered in the Ecosim models, perhaps resulting in the low planktivore and large yellow croaker biomasses in the two dynamic simulations. The seven-fold increase in planktivores (Figure 3) also confirmed the role of the policy on minimum mesh size. Release enhancement is also considered a means of restoring recruitment (Moore et al., 2007). For example, from 2001 to 2006, 2~6 million juvenile large yellow croakers were released annually in Zhejiang Province, and tagged adults were recaptured in a fishery survey (Lü et al., 2008). Unfortunately, there was no integrity database to offer reliable and detailed release data, including the release numbers and efforts (Yang et al., 2013). Therefore, it was very difficult to evaluate the effect of the release enhancement in the Ecosim model.
The FMPs in the ECS increased the fish biomass, but the effect was to some extent limited to the recovery of commercial fishes (Figure 7). In the 1990s, the total number of landings in the ECS have become quite intense and destructive, e.g., bottom trawling is still widely used (Han et al., 2017), which can destroy benthic communities (Olsgard et al., 2008; Van Denderen et al., 2015; Hiddink et al., 2019). The biomass of the zoobenthos, especially mollusks and polychaetes, sharply declined in M2018 (Table S1). This, in turn, influences the transfer of material and energy from primary producers or detritus to higher TLs. Therefore, the government should strengthen the implementation of the FMPs by limiting the depth of trawling and the number of catches as well as by prolonging the duration of the rest period. This would reduce the pressure from overfishing (Dimarchopoulou et al., 2019; Russo et al., 2019) and protect vulnerable benthic habitats (Clark et al., 2019). These measures were simulated in the Gulf of Gabes (Tunisia) by Halouani et al. (2016), who found that limiting the trawling depth and lengthening the rest period duration can both increase the TL of the catch. In addition, lessons can be drawn from successful programs implemented around the world and applied to the FMP system in China. Enhancing the selectivity of species, selecting an optimal body length for species, and evaluating the total allowable catch via historical data would also be beneficial to the recovery of fisheries (Coll et al., 2008a; Colloca et al., 2013).
5 Conclusion
The ECS ecosystem is becoming more mature, although it is still unstable. The high biomass of plankton stimulated an increase in other groups, especially planktivores. FMPs such as the SFM and minimum-mesh size also play positive roles in fish recovery. Despite this, fishing management still requires further development due to the decrease in high-TL groups and the change in keystone species. The commercial fish are still in an unrecovered state. A SFM could promote the fishery recovery, but extending the SFM and reducing fishing pressure after it would play a greater role in rehabilitating the depleted fisheries resources in the ECS.
It must be said that the dynamic simulation in Ecosim in this study only included the SFM and fishing pressure. We cannot evaluate the impacts of the minimum-mesh size and release enhancement due to the difficulty in obtaining precise estimates for fishing effort. The aquatic product and fleet can only represent the tendency of the fishing effort. Therefore, in the future long-term monitoring of keystone species and commercial fish, data on bycatch as well as climate and oceanographic variables are critical to evaluating and predicting future changes in the ECS ecosystem. Reliable and detailed fishery data are also missing, especially the discard data for non-commercial species, which contributes at least 8% to the entire fish yield (Cao et al., 2017). Therefore, it is critical to developing an integrity database for better stock assessment and fishery management.
Data Availability Statement
The original contributions presented in the study are included in the article/Supplementary Material. Further inquiries can be directed to the corresponding authors.
Ethics Statement
The animal study was reviewed and approved by the Third Institute of Oceanography, Ministry of Natural Resources, Xiamen 361005, China.
Author Contributions
XZ and LL contributed to the conception and design of the study. LX wrote the first draft of the manuscript. XZ, YW, LX, and PS analyzed the data and reviewed the manuscript. All authors contributed to the article and approved the submitted version.
Funding
This work was funded by the National Key Research and Development Program of China (Grant Numbers: 2018YFC1406301 and 2018YFC1406302) and the Scientific Research Foundation of Third Institute of Oceanography, MNR (Grant Number: 2019017).
Conflict of Interest
The authors declare that the research was conducted in the absence of any commercial or financial relationships that could be construed as a potential conflict of interest.
Publisher’s Note
All claims expressed in this article are solely those of the authors and do not necessarily represent those of their affiliated organizations, or those of the publisher, the editors and the reviewers. Any product that may be evaluated in this article, or claim that may be made by its manufacturer, is not guaranteed or endorsed by the publisher.
Supplementary Material
The Supplementary Material for this article can be found online at: https://www.frontiersin.org/articles/10.3389/fmars.2022.865645/full#supplementary-material
References
Bromley D. W. (2005). Purging the Frontier From Our Mind: Crafting a New Fisheries Policy. Rev. Fishr. Bio. Fisher. 15 (3), 217–229. doi: 10.1007/s11160-005-4866-z
Burnham K. P., Anderson D. R. (2004). Multimodel Inference: Understanding AIC and BIC in Model Selection. Sociol. Method. Res. 33 (2), 261–304. doi: 10.1177/0049124104268644
Cao L., Chen Y., Dong S., Hanson A., Huang B., Leadbitter D., et al. (2017). Opportunity for Marine Fisheries Reform in China. Proc. Natl. Acad. Sci. 114 (3), 435–442. doi: 10.1073/pnas.1616583114
Chagaris D. D., Patterson W. F. III, Allen M. S. (2020). Relative Effects of Multiple Stressors on Reef Food Webs in the Northern Gulf of Mexico Revealed Via Ecosystem Modeling. Front. Mar. Sci. 7, 513. doi: 10.3389/fmars.2020.00513
Chassot E., Mélin F., Le Pape O., Gascuel D. (2007). Bottom-Up Control Regulates Fisheries Production at the Scale of Eco-Regions in European Seas. Mar. Ecol. Prog. Ser. 343, 45–55. doi: 10.3354/meps06919
Chen Y. (2017). Analysis of the Present Situation and Prospects of the Fishery Resources Enhancement in Shandong Province (Shandong, China: Yantai University, Master thesis).
Chen D., Duan X., Liu S., Shi W. (2004). Status and Management of Fishery Resources of the Yangtze River. In: Proc. Second. Int. Symposium. Manage. Large. River. Fish. Vol. IR. Phnom. Penh. Kingdom. Cambodia. Citeseer. ed, Petr R. L. W. A. T.. 1, 173–182.
Cheng J., Cheung W. W. L., Pitcher T. J. (2009). Mass-Balance Ecosystem Model of the East China Sea. Prog. Nat. Sci. 19 (10), 1271–1280. doi: 10.1016/j.pnsc.2009.03.003
Cheng J., Lin L., Ling J., Li J., Ding F. (2004). Effects of Summer Close Season and Rational Utilization on Redlip Croaker (Larimichthys Polyactis Bleeker) Resource in the East China Sea Region. J. Fish. Sci. China. 11 (6), 554–560. doi: 10.3321/j.issn:1005-8737.2004.06.012
Chen W., Li C., Hu F. (1997). A Review of the Fisheries Resource Status in the East China Sea. J. Fish. Sci. China. 03), 40–44. doi: CNKI:SUN:ZSCK.0.1997-03-007
Cheung W. W. L., Watson R., Pauly D. (2013). Signature of Ocean Warming in Global Fisheries Catch. Nature. 497(7449), 365–368. doi: 10.1038/nature12156
Christensen V., Pauly D. (1992). Ecopath II—a Software for Balancing Steady-State Ecosystem Models and Calculating Network Characteristics. Ecol. Model. 61 (3-4), 169–185. doi: 10.1016/0304-3800(92)90016-8
Christensen V., Walters C. J., Pauly D. (2005). Ecopath With Ecosim: A User’s Guide. Fish. Centre. Univ. Br. Columbia. Vancouver. 154, 31.
Clark M. R., Bowden D. A., Rowden A. A., Stewart R. (2019). Little Evidence of Benthic Community Resilience to Bottom Trawling on Seamounts After 15 Years. Front. Mar. Sci. 6, 63. doi: 10.3389/fmars.2019.00063
Coll M., Bahamon N., Sardà F., Palomera I., Tudela S., Suuronen P. (2008a). Improved Trawl Selectivity: Effects on the Ecosystem in the South Catalan Sea (Nw Mediterranean). Mar. Ecol. Prog. Ser. 355, 131–147. doi: 10.3354/meps07183
Coll M., Lotze H. K., Romanuk T. N. (2008b). Structural Degradation in Mediterranean Sea Food Webs: Testing Ecological Hypotheses Using Stochastic and Mass-Balance Modeling. Ecosystems 11 (6), 939–960. doi: 10.1007/s10021-008-9171-y
Colloca F., Cardinale M., Maynou F., Giannoulaki M., Scarcella G., Jenko K., et al. (2013). Rebuilding Mediterranean Fisheries: A New Paradigm for Ecological Sustainability. Fish. Res. 14 (1), 89–109. doi: 10.1111/j.1467-2979.2011.00453.x
Di Franco A., Bussotti S., Navone A., Panzalis P., Guidetti P. (2009). Evaluating Effects of Total and Partial Restrictions to Fishing on Mediterranean Rocky-Reef Fish Assemblages. Mar. Ecol. Prog. Ser. 387, 275–285. doi: 10.3354/meps08051
Dimarchopoulou D., Keramidas I., Tsagarakis K., Tsikliras A. C. (2019). Ecosystem Models and Effort Simulations of an Untrawled Gulf in the Central Aegean Sea. Front. Mar. Sci. 6, 648. doi: 10.3389/fmars.2019.00648
Ehrnsten E., Bauer B., Gustafsson B. G. (2019). Combined Effects of Environmental Drivers on Marine Trophic Groups–A Systematic Model Comparison. Front. Mar. Sci. 492. doi: 10.3389/fmars.2019.00492
Finn J. T. (1976). Measures of Ecosystem Structure and Function Derived From Analysis of Flows. J. Theor. Biol. 56 (2), 363–380. doi: 10.1016/S0022-5193(76)80080-X
Friedland K. D., Stock C., Drinkwater K. F., Link J. S., Leaf R. T., Shank B. V., et al. (2012). Pathways Between Primary Production and Fisheries Yields of Large Marine Ecosystems. PLos One 7 (1), e28945. doi: 10.1371/journal.pone.0028945
Fulton E. A., Smith A. D., Smith D. C., Johnson P. (2014). An Integrated Approach is Needed for Ecosystem Based Fisheries Management: Insights From Ecosystem-Level Management Strategy Evaluation. PloS One 9 (1), e84242. doi: 10.1371/journal.pone.0084242
Funtowicz S. O., Ravetz J. R. (1990). Uncertainty and Quality in Science for Policy (Netherlands: Kluwer Academic Publishers).
Gebremedhin S., Bruneel S., Getahun A., Anteneh W., Goethals P. (2021). Scientific Methods to Understand Fish Population Dynamics and Support Sustainable Fisheries Management. Water 13 (4), 574. doi: 10.3390/w13040574
Halpern B. S., Walbridge S., Selkoe K. A., Kappel C. V., Micheli F., D'Agrosa C., et al (2008). A Global Map of Human Impact on Marine Ecosystems. Science. 319 (5865), 948–952. doi: 10.1126/science.1149345
Halouani G., Abdou K., Hattab T., Romdhane M. S., Lasram F. B. R., Le Loc’h F. (2016). A Spatio-Temporal Ecosystem Model to Simulate Fishing Management Plans: A Case of Study in the Gulf of Gabes (Tunisia). Mar. Policy. 69, 62–72. doi: 10.1016/j.marpol.2016.04.002
Han Y. (2018). Marine Fishery Resources Management and Policy Adjustment in China Since 1949. Chin. Rural Economy. 09, 14–28.
Han D., Chen Y., Zhang C., Ren Y., Xue Y., Wan R. (2017). Evaluating Impacts of Intensive Shellfish Aquaculture on a Semi-Closed Marine Ecosystem. Ecol. Model. 359, 193–200. doi: 10.1016/j.ecolmodel.2017.05.024
Heikinheimo O., Setälä J., Saarni K., Raitaniemi J. (2006). Impacts of Mesh-Size Regulation of Gillnets on the Pikeperch Fisheries in the Archipelago Sea, Finland. Fish. Res. 77 (2), 192–199. doi: 10.1016/j.fishres.2005.11.005
Hiddink J. G., Jennings S., Sciberras M., Bolam S. G., Cambiè G., McConnaughey R. A., et al. (2019). Assessing Bottom Trawling Impacts Based on the Longevity of Benthic Invertebrates. J. Appl. Ecol. 56 (5), 1075–1084. doi: 10.1111/1365-2664.13278
Jiang Y., Cheng J., Li S. (2009). Temporal Changes in the Fish Community Resulting From a Summer Fishing Moratorium in the Northern East China Sea. Mar. Ecol. Prog. Ser. 387, 265–273. doi: 10.3354/meps08078
Kroeker K. J., Kordas R. L., Crim R., Hendriks I. E., Ramajo L., Singh G. S., et al (2013). Impacts of Ocean Acidification on Marine Organisms: Quantifying Sensitivities and Interaction With Warming. Global Change Biol. 19 (6), 1884–96. doi: 10.1111/gcb.12179
Lee S., Midani A. R. (2014). National Comprehensive Approaches for Rebuilding Fisheries in South Korea. Mar. Policy. 45, 156–162. doi: 10.1016/j.marpol.2013.12.010
Li Y. (2009). Ecological Modeling of the East China Sea Shelf Ecosystem (Shanghai, China: East China Normal University).
Li C. (2020). Brief Introduction of Fishery Resources Increase in Liaoning Province. Jiangxi. Agric. 08, 99–100. doi: 10.1016/j.marpol.2013.12.010
Libralato S., Christensen V., Pauly D. (2006). A Method for Identifying Keystone Species in Food Web Models. Ecol. Model. 195 (3-4), 153–171. doi: 10.1016/j.ecolmodel.2005.11.029
Lin J., Liu X., Lai T., He B., Du J., Zheng X. (2021). Trophic Importance of the Seagrass Halophila Ovalis in the Food Web of a Hepu Seagrass Bed and Adjacent Waters, Beihai, China. Ecol. Indic. 125, 107607. doi: 10.1016/j.ecolind.2021.107607
Lin Q., Shan X., Wang J., Li Z. (2018). Changes in Chinese Shrimp (Fenneropenaeus Chinensis) Carrying Capacity of the Bohai Sea. Prog. Fish.Sci. 39 (04), 19–29. doi: 10.19663/j.issn2095-9869.20170908001
Link J. S., Watson R. A. (2019). Global Ecosystem Overfishing: Clear Delineation Within Real Limits to Production. Sci. Adv. 5 (6), eaav0474. doi: 10.1126/sciadv.aav0474
Lira A. S., Lucena-Fredou F., Le Loc'h F. (2021). How the Fishing Effort Control and Environmental Changes Affect the Sustainability of a Tropical Shrimp Small Scale Fishery. Fish. Res. 235, 105824. doi: 10.1016/j.fishres.2020.105824
Liu J. (2013). Status of Marine Biodiversity of the China Seas. PLos One 8 (1), e50719. doi: 10.1371/journal.pone.0050719
Liu Y., Cheng J. (2015). A Preliminary Analysis of Variation Characteristics of Structure and Average Trophic Level of the Main Fishery Species Caught by Paired Bottom Trawl in the East China Sea and the Yellow Sea During the Fall Season. J. Fish. China. 39 (05), 691–702. doi: 10.11964/jfc.20141009521
Liu K., Duan J., Xu D., Zhang M., Fang D., Shi W. (2012). Present Situation of Coilia Nasus Population Features and Yield in Yangzte River Estuary Waters in Fishing Season. Chin. J. Ecol. 31 (12), 3138–3143. doi: 10.13292/j.1000-4890.2012.0407
Liu K., Yu C., Zheng J., Xu Y., Jiang X., Yu N., et al. (2021). Analysis of Function Groups Characteristics and Niche of Major Fish Species in the Coastal Waters of Zhoushan Islands in Spring and Autumn. J. Zhejiang. Univ. (Science Edition). 48 (05), 592–605. doi: 10.3785/j.issn.1008-9497.2021.05.011
Li Y., Zhang Y. (2012). Fisheries Impact on the East China Sea Shelf Ecosystem for 1969-2000. Helgoland. Mar. Res. 66 (3), 371–383. doi: 10.1007/s10152-011-0278-8
Lü H., Xu J., Vander Haegen G. (2008). Supplementing Marine Capture Fisheries in the East China Sea: Sea Ranching of Prawn Penaeus Orientalis, Restocking of Large Yellow Croaker Pseudosciaena Crocea, and Cage Culture. Rev. Fish. Sci. 16 (1-3), 366–376. doi: 10.1080/10641260701678207
Lu C., Zhao J. (2015). The Review and Prospect on Fish Moratorium Policy in the East China Sea. Fish. Info. Stra. 30 (03), 168–174. doi: 10.1080/10641260701678207
Ma M., Chen Z., Xu S., Zhang J., Yu W. (2020). Trophic Structure and Energy Flow of Continental Slope of the Northern South China Sea Ecosystem. J. Fish. Sci. China. 44 (10), 1685–1694. doi: 10.1080/10641260701678207
Majkowski J. (1982). Usefulness and Applicability of Sensitivity Analysis in a Multispecies Approach to Fisheries Management. Theory Manage. Trop. fisheries. ICLARM. Conf. Proc. 9, 149–165.
Mei J. (2019). Status, Problems and Suggestions of Marine Fishing in the Yellow Sea and the East China Sea Areas. J. Anhui. Agric. Sci. 47 (08), 241–243. doi: 10.3969/j.issn.0517-6611.2019.08.063
Moore M., Early G., Touhey K., Barco S., Gulland F., Wells R. (2007). Rehabilitation and Release of Marine Mammals in the United States: Risks and Benefits. Mar. Mammal Sci. 23, 4, 731–750. doi: 10.1111/j.1748-7692.2007.00146.x
Morissette L. (2007). Complexity, Cost and Quality of Ecosystem Models and Their Impact on Resilience: A Comparative Analysis, With Emphasis on Marine Mammals and the Gulf of St. Lawrence (Vancouver, Canada: University of British Columbia).
Napata R. P., Espectato L. N., Serofia G. D. (2020). Closed Season Policy in Visayan Sea, Philippines: A Second Look. Ocean. Coast. Manage. 187, 105–115. doi: 10.1016/j.ocecoaman.2020.105115
Nee S. (1990). Community Construction. Trends Ecol. Evol. 5 (10), 337–340. doi: 10.1016/0169-5347(90)90182-D
Nguyen K. Q., Do M. D., Phan H. T., Nguyen L. T., Van To P., Vu N. K., et al. (2021). Catch Composition and Codend Selectivity of Inshore Trawl Fishery With the Legal Minimum Mesh Size. Reg. Stud. Mar. Sci. 47, 101977. doi: 10.1016/j.rsma.2021.101977
Olsgard F., Schaanning M. T., Widdicombe S., Kendall M. A., Austen M. C. (2008). Effects of Bottom Trawling on Ecosystem Functioning. J. Exp. Mar. Biol. Ecol. 366 (1-2), 123–133. doi: 10.1016/j.jembe.2008.07.036
OuYang L., Guo X. (2010). Studies on the Q/B Values and Food Consumption of Major Fishes in the East China Sea and the Yellow Sea. Prog. Fish. Sci. 31 (02), 23–9. doi: 10.3969/j.issn.1000-7075.2010.02.004
Palomares M. L., Pauly D. (1989). A Multiple Regression Model for Prediction the Food Consumption of Marine Fish Populations. Mar. Freshwat. Res. 40 (3), 259–273. doi: 10.1071/MF9890259
Papapanagiotou G., Tsagarakis K., Koutsidi M., Tzanatos E. (2020). Using Traits to Build and Explain an Ecosystem Model: Ecopath With Ecosim Modelling of the North Aegean Sea (Eastern Mediterranean). Estuar. Coast. Shelf. S. 236, 106614. doi: 10.1016/j.ecss.2020.106614
Paradell O. G., Methion S., Rogan E., López B. D. (2021). Modelling Ecosystem Dynamics to Assess the Effect of Coastal Fisheries on Cetacean Species. J. Environ. Manage. 285, 112175. doi: 10.1016/j.jenvman.2021.112175
Pauly D., Christensen V. (1993). “Stratified Models of Large Marine Ecosystems: A General Approach and an Application to the South China Sea,” in Large Marine Ecosystems: Stress, Mitigation and Sustainability (Washington, DC: AAAS Press), 148–174.
Pauly D., Christensen V., Sambilay V. Jr. (1990). Some Features of Fish Food Consumption Estimates Used by Ecosystem Modelers (Metro manlia, Philippines: International Council for the Exploration of the Sea (ICES).
Pikitch E. K., Santora C., Babcock E. A., Bakun A., Bonfil R., Conover D. O., et al (2004). Ecosystem-Based Fishery Management. Science 305 (5682), 346–347. doi: 10.1890/1051-0761(2001)011[0601:FMTRER]2.0.CO;2
Pitcher T. J. (2001). Fisheries Managed to Rebuild Ecosystems? Reconstructing the Past to Salvage the Future. Ecol. Appl. 11 (2), 601–617. doi: 10.1890/1051-0761(2001)011[0601:FMTRER]2.0.CO;2
Reum J. C. P., Townsend H., Gaichas S., Sagarese S., Kaplan I. C., Grüss A. (2021). It’s Not the Destination, It’s the Journey: Multispecies Model Ensembles for Ecosystem Approaches to Fisheries Management. Front. Mar. Sci. 8, 75. doi: 10.3389/fmars.2021.631839
Russo T., Bitetto I., Carbonara P., Carlucci R., D'Andrea L., Facchini M. T., et al. (2017). A Holistic Approach to Fishery Management: Evidence and Insights From a Central Mediterranean Case Study (Western Ionian Sea). Front. Mar. Sci. 4, 193. doi: 10.3389/fmars.2017.00193
Russo T., D'Andrea L., Franceschini S., Accadia P., Cucco A., Garofalo G., et al. (2019). Simulating the Effects of Alternative Management Measures of Trawl Fisheries in the Central Mediterranean Sea: Application of a Multi-Species Bio-Economic Modeling Approach. Front. Mar. Sci. 542. doi: 10.3389/fmars.2019.00542
Sagarese S. R., Lauretta M. V., Walter J. F. (2017). Progress Towards a Next-Generation Fisheries Ecosystem Model for the Northern Gulf of Mexico. Ecol. Model. 345, 75–98. doi: 10.1016/j.ecolmodel.2016.11.001
Samy-Kamal M., Forcada A., Lizaso J. L. S. (2015). Effects of Seasonal Closures in a Multi-Specific Fishery. Fish. Res. 172, 303–317. doi: 10.1016/j.fishres.2015.07.027
Scott E., Serpetti N., Steenbeek J., Heymans J. J. (2016). A Stepwise Fitting Procedure for Automated Fitting of Ecopath With Ecosim Models. Software. X. 5, 25–30. doi: 10.1016/j.softx.2016.02.002
Shen G., Heino M. (2014). An Overview of Marine Fisheries Management in China. Mar. Pol. 44, 265–272. doi: 10.1016/j.marpol.2013.09.012
Shi X. (1995). Fishery Environment and Utilization Status of Important Fishing Resources in the East China Sea. Mar. Inf. 09, 16.
Shih N., Cai Y., Ni I. (2009). A Concept to Protect Fisheries Recruits by Seasonal Closure During Spawning Periods for Commercial Fishes Off Taiwan and the East China Sea. J. Appl. Ichthyology. 25 (6), 676–685. doi: 10.1111/j.1439-0426.2009.01328.x
Su Y., Chen G., Zhou Y., Ma S., Wu Q. (2019). Assessment of Impact of Summer Fishing Moratorium in South China Sea During 2015–2017. South China Fish. Sci. 15 (2), 20–28. doi: 10.12131/20180149
Sun C., Liang G. (2016). Common Fishery Policy and Fishery Subsidies in the EU. World Agri. 06, 78–85. doi: 10.13856/j.cn11-1097/s.2016.06.014
Surma S., Christensen V., Kumar R., Ainsworth C. H., Pitcher T. J. (2019). High-Resolution Trophic Models Reveal Structure and Function of a Northeast Pacific Ecosystem. Front. Mar. Sci. 625. doi: 10.3389/fmars.2019.00625
Tajzadehnamin M., Valinassab T., Ramezani-Fard E., Ehteshami F. (2020). Trophic Dynamics Analysis and Ecosystem Structure for Some Fish Species of Northern Oman Sea. Iran. J. Fish. Sci. 19 (6), 2804–2823.
Tokai T., Shiode D., Sakai T., Yoda M. (2019). Codend Selectivity in the East China Sea of a Trawl Net With the Legal Minimum Mesh Size. Fish. Sci. 85 (1), 19–32. doi: 10.1007/s12562-018-1270-x
Ulanowicz R. E. (2012). Growth and Development: Ecosystems Phenomenology (New York, the US: Springer Science & Business Media).
Ulanowicz R. E. (1986). Growth and Development: Ecosystems Phenomenology. Estuaries 11(1), 73–74. doi: 10.2307/1351721
Ulanowicz R. E., Puccia C. J. (1990). Mixed Trophic Impacts in Ecosystems. Coenoses 5, 7–16. doi: 10.2307/43461017
Van Denderen P. D., Bolam S. G., Hiddink J. G., Jennings S., Kenny A., Rijnsdorp A. D., et al. (2015). Similar Effects of Bottom Trawling and Natural Disturbance on Composition and Function of Benthic Communities Across Habitats. Mar. Ecol. Prog. Ser. 541, 31–43. doi: 10.3354/meps11550
Vijverberg J., Dejen E., Getahun A., Nagelkerke L. A. (2012). The Composition of Fish Communities of Nine Ethiopian Lakes Along a North-South Gradient: Threats and Possible Solutions. Anim. Biol. 62 (3), 315–535. doi: 10.1163/157075611X618246
Wang Y., Hu J., Pan H., Failler P. (2020). Ecosystem-Based Fisheries Management in the Pearl River Delta: Applying a Computable General Equilibrium Model. Mar. Policy. 112, 103784. doi: 10.1016/j.marpol.2019.103784
Wang W., Wang J., Zuo P., Li Y., Zou X. (2019). Analysis of Structure and Energy Flow in Southwestern Yellow Sea Ecosystem Based on Ecopath Model. J. Appl. Oceanog. 38 (04), 528–539. doi: 10.3969/J.ISSN.2095-4972.2019.04.008
Wu L., Zhang N., Sun S., Yuan J., Chen J., Li M., et al. (2021). Application of Microsatellite Markers for Evaluating the Effect of Restocking Enhancement in Larimichthys Crocea. J. Fish. Sci. China. 28, 09, 1100–1108. doi: 10.12264/JFSC2020-0542
Xin Y., Yu C., Jian K., Liu H., Zhang P., Liu K. (2020). The Effectiveness of China's Fishing Policy of Marine Fishing Industry in Zhejiang Province. Ocean. Dev. Manage. 37 (05), 25–31. doi: 10.3969/j.issn.1005-9857.2020.05.005
Xu P., Ke Q., Su Y., Liu J., Zheng W. (2021). Protection and Utilization and Prospect of Large Yellow Croaker (Larimichthys Crocea) Germplasm Resources. J. Fish. China 46 (4), 1–9. doi: 10.11964/jfc.20210312688
Xu K., Liu Z. (2007). The Current Stock of Large Yellow Croaker Pseudosciaena Crocea in the East China Sea With Respects of its Stock Decline. J. Dalian. Ocean. University. 05, 392–396. doi: 10.16535/j.cnki.dlhyxb.2007.05.015
Yang Y., Liu P., Zhou H., Xia L. (2020). Evaluation of the Biodiversity Variation and Ecosystem Health Assessment in Changjiang Estuary During the Past 15 Years. Acta Ecol. Sin. 40 (24), 8892–8904. doi: 10.5846/stxb201912272811
Yang J., Pan X., Chen X., Wang X., Zhao Y., Li J., et al. (2013). Overview of the Artificial Enhancement and Release of Endemic Freshwater Fish in China. Zoo. Res. 34 (04), 267–280. doi: CNKI:SUN:DWXY.0.2013-04-007
Yan L., Liu Z., Jin Y., Cheng J. (2019a). Effects of Prolonging Summer Fishing Moratorium in the East China Sea on the Increment of Fishery Resources. Mar. Fish. 41 (05), 513–519. doi: 10.13233/j.cnki.mar.fish.2019.05.001
Yan L., Liu Z., Jin Y., Cheng J. (2019b). Effects of Prolonging the Trawl Net Summer Fishing Moratorium in the East China Sea on the Conservation of Fishery Resources. J. Fish. China. 26 (01), 118–123. doi: 10.3724/SP.J.1118.2019.18243
Ye Y., Rosenberg A. A. (1991). A Study of the Dynamics and Management of the Hairtail Fishery, Trichiurus Haumela, in the East China Sea. Aquat. Living. Res. 4 (2), 65–75. doi: 10.1051/alr:1991007
Yue D., Wang L., Zhang X., Zheng H., Zhang H. (2015). Status and Reflections of the Summer Closed Fishing in the East China Sea. J. Agric. Sci. Technol. 17 (04), 122–128. doi: 10.13304/j.nykjdb.2015.113
Zeng Z., Cheung W. W. L., Li S., Hu J., Wang Y. (2019). Effects of Climate Change and Fishing on the Pearl River Estuary Ecosystem and Fisheries. Rev. Fish. Biol. Fisher. 29, (4). doi: 10.1007/s11160-019-09574-y
Zhai L., Liang C., Pauly D. (2020). Assessments of 16 Exploited Fish Stocks in Chinese Waters Using the CMSY and BSM Methods. Front. Mar. Sci. 7, 1002. doi: 10.3389/fmars.2020.483993
Zhang H., Song P., Li Y., Liu S., Wang X., Zheng J., et al. (2021). Diversity and Community Structure of Nekton in the Central and Southern East China Sea in Autumn. J. Appl. Oce. 40 (04), 575–586. doi: 10.3969/J.ISSN.2095-4972.2021.04.003
Zhang Q., Hong W., Yang S., Liu M.. (2010). Survey on Status of Fishery Resources Along the Coastal Waters of the Liuheng Town in Zhoushan City. Fish. Inf. Stra. 25 (12), 10–12. doi: 10.3969/j.issn.1004-8340.2010.12.003
Zhang Y., Xu K., Li D., Zhang H., Dai G., Li Z., et al. (2018). The Distribution and Biological Characteristics of Chelidonichthy Kumu in the North East China Sea. J. Zhejiang. Ocean. Univ. (Nat. Sci). 37 (05), 418–423. doi: 10.3969/j.issn.1008-830X.2018.05.007
Zheng X., Como S., Huang L., Magni P. (2020). Temporal Changes of a Food Web Structure Driven by Different Primary Producers in a Subtropical Eutrophic Lagoon. Mar. Environ. Res. 161, 105128. doi: 10.1016/j.marenvres.2020.105128
Zhou S., Smith A. D., Knudsen E. E. (2015). Ending Overfishing While Catching More Fish. Fish. Res. 16 (4), 716–722. doi: 10.1111/faf.12077
Keywords: Ecopath with Ecosim, East China Sea, seasonal fishing moratorium, commercial fish, fisheries management policies
Citation: Xu L, Song P, Wang Y, Xie B, Huang L, Li Y, Zheng X and Lin L (2022) Estimating the Impact of a Seasonal Fishing Moratorium on the East China Sea Ecosystem From 1997 to 2018. Front. Mar. Sci. 9:865645. doi: 10.3389/fmars.2022.865645
Received: 30 January 2022; Accepted: 20 April 2022;
Published: 09 June 2022.
Edited by:
Jun Xu, Institute of Hydrobiology (CAS), ChinaReviewed by:
Zhongxin Wu, Dalian Ocean University, ChinaChongliang Zhang, Ocean University of China, China
Copyright © 2022 Xu, Song, Wang, Xie, Huang, Li, Zheng and Lin. This is an open-access article distributed under the terms of the Creative Commons Attribution License (CC BY). The use, distribution or reproduction in other forums is permitted, provided the original author(s) and the copyright owner(s) are credited and that the original publication in this journal is cited, in accordance with accepted academic practice. No use, distribution or reproduction is permitted which does not comply with these terms.
*Correspondence: Xinqing Zheng, emhlbmd4aW5xaW5nQHRpby5vcmcuY24=; Longshan Lin, bHNsaW5AdGlvLm9yZy5jbg==
†These authors have contributed equally to this work and share first authorship