- 1Southwest Fisheries Science Center, National Marine Fisheries Service, La Jolla Shores Dr., La Jolla, CA, United States
- 2Northeast Fisheries Science Center, National Marine Fisheries Service, Woods Hole, MA, United States
Entanglements and vessel strikes impact large whales worldwide. Post-event health status is often unknown because whales are seen once or over short spans that conceal long-term health declines. Well-studied populations with high site fidelity verified by photo-ID offer opportunity to confirm deaths, health declines and recoveries. We used known outcome entanglements and vessel strikes of right whales (Eubalaena glacialis) and humpback whales (Megaptera novaeangliae) to model probabilities of deaths, health declines and recoveries with Random Forest (RF) classification trees. Variables included presence or absence of phrases from case narratives (‘deep laceration’, ‘cyamid’, ‘healing’, ‘superficial’) and a categorical variable for vessel size. Health status post-entanglement was correctly classified in 95.7% of right whale and 93.6% of humpback whale cases (expected by chance=50%). Health status post-vessel strike was correctly classified in 91.4% of right whale and 88.6% of humpback whale cases. Important variables included cyamid presence, emaciation, discolored skin, constricting entanglements, gear-free resightings, superficial or healing lacerations, and vessel size. Cross-validated RF models were applied to unknown outcome cases to estimate the probability of deaths, health declines and recoveries. Total serious injuries (probability of death or health decline > 0.50) assigned by RF were nearly equal to current injury assessment methods applied by biologists for known outcomes. However, RF consistently predicted higher serious injury totals for unknown outcomes, suggesting that current assessment methods may underestimate risk for cases lacking details or long-term observations. Advantages of the RF method include: 1) risk models are based on known outcomes; 2) unknown outcomes are assigned post-event health status probabilities; and 3) identification of important predictor variables improves data collection standards.
Introduction
Mortality and serious injury (MSI) of large whales caused by entanglements and vessel strikes occurs worldwide and is a chronic problem in several populations (Clapham et al., 1999; Neilson et al., 2009; Conn and Silber, 2013; Robbins et al., 2015; Rockwood et al., 2017; van der Hoop et al., 2017). True impacts of chronic entanglements, in particular, are difficult to quantify, with some noting that large whale populations essentially have an ‘entanglement life history stage’ (van der Hoop et al., 2017). Scientists are challenged with assessing entanglement and vessel strike health impacts, but an inability to follow all cases through time means many outcomes are unknown, including undocumented deaths, or ‘cryptic mortality’ (Gilman et al., 2013, Pace et al., 2021). Several studies have quantified cryptic mortality levels by estimating the percentage of available carcasses that are detected to be between 0% and 46%, depending on species, habitat, and mortality source (Kraus et al., 2005; Williams et al., 2011; Gilman et al., 2013; Prado et al., 2013; Wells et al., 2015; Carretta et al., 2016; Rockwood et al., 2017; Harting et al., 2021; Pace et al., 2021). The ability to follow individual whales with entanglement or vessel strike injuries through time via photo identification of unique entangling gear, scars, lacerations, or natural markings provides the opportunity to model the outcomes of such interactions. However, these can produce biased information as more lethal injuries may have a lower chance of being observed later, especially if they occur far from shore (Pace et al., 2021, Williams et al., 2011).
In the United States, classification of non-serious vs serious whale injuries from entanglements and vessel strikes is determined by National Oceanic and Atmospheric Administration (NOAA) biologists manually applying decision tree criteria to case narratives (NOAA, 2012a; NOAA, 2012b). NOAA defines a serious injury as any injury that is “more likely than not to result in mortality, or any injury that presents a greater than 50 percent chance of death to a marine mammal.” (NOAA, 2012b). Injury assessments are divided into multiple discrete categories, based on whether entangling gear is constricting vs loose, physical evidence of health declines (emaciation, heavy cyamid loads), presence of trailing gear, severity of vessel strike or entanglement lacerations, and vessel sizes and speeds involved in a strike relative to whale size (Angliss and DeMaster, 1998; Pettis et al., 2004; Andersen et al., 2008; NOAA, 2012a; NOAA, 2012b; Conn and Silber, 2013; Rolland et al., 2016). In practice, many entanglement and vessel strike cases represent whales seen only once with generic narratives such as ‘whale seen dragging 4 buoys’ or ‘sailboat reported striking whale, vessel size and speed unknown’. The policy for assessing such ‘data poor’ or unknown outcome cases is to prorate the probability of injury or death based upon the fraction of known outcome entanglement and vessel strikes resulting in a death or health decline between 2004 and 2008 (NOAA, 2012a; NOAA, 2012b). One such example (a proration factor = 0.75) is applied to entanglements where the amount and configuration of entangling material is unknown and the final health status of the whale is unknown. The serious injury policy states: “of the 114 documented entanglement events with known outcomes from 2004-2008, 85 (75%) either resulted in the whales’ deteriorating health or death, or would have resulted in the whales’ death if not for intervention (40 were disentangled from constricting wraps).” (NOAA, 2012a; NOAA, 2012b). Because some injury categories are based on small sample sizes and serious injuries are defined as any injury more likely than not to result in mortality, current injury categories also incorporate a binomial test to estimate the likelihood of observed mortality rates of a given category exceeding 50% (NOAA, 2012a; NOAA, 2012b). Current protocols utilize several discrete injury assignment values ranging from zero (a non-serious injury) to one (a serious injury), including prorated serious injury values of 0.14, 0.20, 0.36, 0.52, 0.56, and 0.75, which are counted against anthropogenic removal levels (Potential Biological Removal or PBR) under the Marine Mammal Protection Act (Wade, 1998).
Some whale populations have excellent mark-recapture sighting histories, facilitating long-term tracking of entanglement and vessel strike injuries that may take many months to manifest themselves as health declines or deaths (Moore and Van der Hoop, 2012; Robbins et al., 2015). In particular, researchers have monitored entangled North Atlantic right whales (Eubalaena glacialis) over long enough spans to estimate a 20% reduction in adult and juvenile survival compared to baseline survival rates of unaffected whales (Robbins et al., 2015). For entanglements where the amount and configuration of attached fishing gear is unknown, NOAA applies a similar probability (p=0.75) of a death or health decline, based on 85 of 114 known outcome entanglement cases resulting in deaths or health declines between 2004-2008 (NOAA, 2012a; NOAA, 2012b). NOAA is reviewing current large whale serious injury assessment procedures, using known outcome entanglement and vessel strike cases to model the probability of deaths, health declines, and recoveries based on case narratives. We describe an approach to improve and automate the large whale serious injury assessment process using variables developed from current serious injury procedures and Random Forest models.
Methods
Our study includes 698 entanglement and vessel strike cases over a 19-year period (2000-2018) for North Atlantic right whales and North Atlantic and North Pacific humpback whales (Megaptera novaeangliae), including 370 known outcomes and 328 unknown outcomes (Table 1). Cases include published data from annual serious injury reports (Carretta et al., 2020; Henry et al., 2020), journal articles (Knowlton et al., 2012; Sharp et al., 2019) and NOAA marine mammal stock assessments (Hayes et al., 2019; Carretta et al., 2020). Entanglement cases include 125 right whale and 398 humpback whale records. Vessel strike cases include 69 right whale and 106 humpback whale records. Known outcome cases include two possible response variables: ‘Dead.Decline’ or ‘Recovered’, corresponding to deaths or health declines, and recoveries. Health declines includes cases with evidence of heavy cyamid loads, skin sloughing or discoloration, necrosis, deep lacerations, rake marks, emaciation, appendage loss or functionality, and deformities (e.g. scoliosis) due to long-term entanglements (Pettis et al., 2004; Moore and Van der Hoop, 2012; van der Hoop et al., 2017). Health declines or deaths may occur over any observation period, while recoveries are defined as whales that are seen ≥1 year post-event in good health and body condition. To model entanglement outcomes, we excluded cases where human intervention to remove fishing gear potentially changed the long-term impact of an entanglement. Our study treats deaths and health declines as equal known outcomes, as both are counted as a ‘mortality or ‘serious injury’ (MSI), which are compared to the anthropogenic removal threshold (Potential Biological Removal; PBR) as defined under the Marine Mammal Protection Act (Wade, 1998). In cases where whales are never seen again post-injury, these are generally considered to be unknown outcomes, unless the individual whale is known, belongs to an intensively-photographed population (i.e. North Atlantic right whale) and it hasn’t been seen in several years, after which it may be inferred to have died, depending on the individual case. However, attributing cause of death to such ‘missing whales’ over such a time period involves great uncertainty.
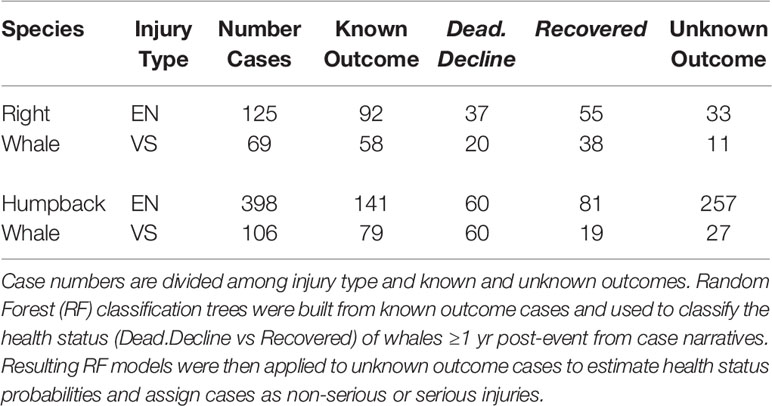
Table 1 Summary of right whale and humpback whale entanglement (EN) and vessel strike (VS) injury cases used in this study.
Right and humpback whale entanglement and vessel strike data were stratified to create separate species and injury type models. Case narratives from known outcomes were data mined for the presence or absence of words or phrases anticipated to be good predictors of the health status responses ‘Dead.Decline’ vs ‘Recovered’. Similar language analyses have been used to identify and successfully predict cultural, gender, and racial biases in news stories and political speeches (Gabrielatos and Baker, 2008; Mastro et al., 2011; Dahllöf, 2012; Caliskan et al., 2017). For example, a heavy cyamid load on a whale is excellent evidence of a health decline and increased probability of death (Pettis et al., 2004, Pettis et al., 2017). Thus, presence or absence of the word ‘cyamid’ or phrase ‘whale lice’ (or other derivatives) in an injury narrative was recorded for each injury case. Additional evidence of health declines include observations of emaciation, sloughing skin, rake marks, limited mobility, and deformities resulting from injuries, thus multiple phrases characterizing a health decline are pooled into a single presence/absence variable called ‘decline’. Similarly, the word ‘constricting’ is a better indicator of a severe entanglement than the word ‘loose’, so phrases that include ‘constrict, ‘embedded’, ‘impression’ ‘cutting into’ or ‘pinned’ were pooled into a single presence/absence variable called ‘constricting’. In some cases, the same word may be used to represent more than one variable, such as ‘amputate’, which is indicative of a severe injury that is related to a constricting entanglement. Thus, ‘amputate’ (or derivatives thereof such as ‘cutting into’) were included in the variables extensive.severe and constricting. For vessel strikes, phrases such as ‘deep laceration’ indicate a more severe injury than ‘superficial laceration’. Specific regions of a whale involved in injuries, such as the head, pectoral fins, and caudal peduncle are also included in our suite of variables, because they are related to a whale’s ability to feed (head), change swimming direction (pectoral fins) or involve body areas where a constricting entanglement or vessel strike could involve major arteries that are near the skin surface (NOAA, 2012a; NOAA, 2012b). We also include a variable indicating whether a whale is identified as a calf or juvenile, as entanglements and/or vessel strikes may impact smaller individuals of a species more severely, and to recognize that a dependent calf of an injured mother also has reduced chances of survival (NOAA, 2012a; NOAA, 2012b). Such phrases inform current serious injury protocols and were therefore coded as presence/absence variables. Some vessel strike narratives include information on the size and speed of vessels involved, which can be predictive of injury severity (Kelley et al., 2020). Phrases and character strings such as ‘ferry traveling @ 11 kts’, ‘navy ship’, ‘sailboat’, ‘fishing boat’, ‘ferry’, ‘>10 kts’, ‘< 10 kts’, ‘vessel much smaller/larger than whale’, ‘>65 ft’, ‘<65 ft’ were used to distinguish between small/large vessels and slow/fast travel speeds to create categorical variables for vessel size (small, large, unknown) and speed (slow, fast, unknown). Phrases associated with only one of two known outcome classes (‘Dead.Decline’ or ‘Recovered’) and absent from unknown outcome cases were omitted from models, as these variables would result in model overfitting that isn’t informative. Omitted examples include ‘carcass’, ‘necropsy’, ‘hemorrhaging’, and ‘fracture’. Variables used in entanglement and vessel strike models are identified in Table 2. Identification and selection of potential variables was aided by reviewing current injury protocols for use of such phrases and plotting and examining their association with known health status responses (Figure 1).
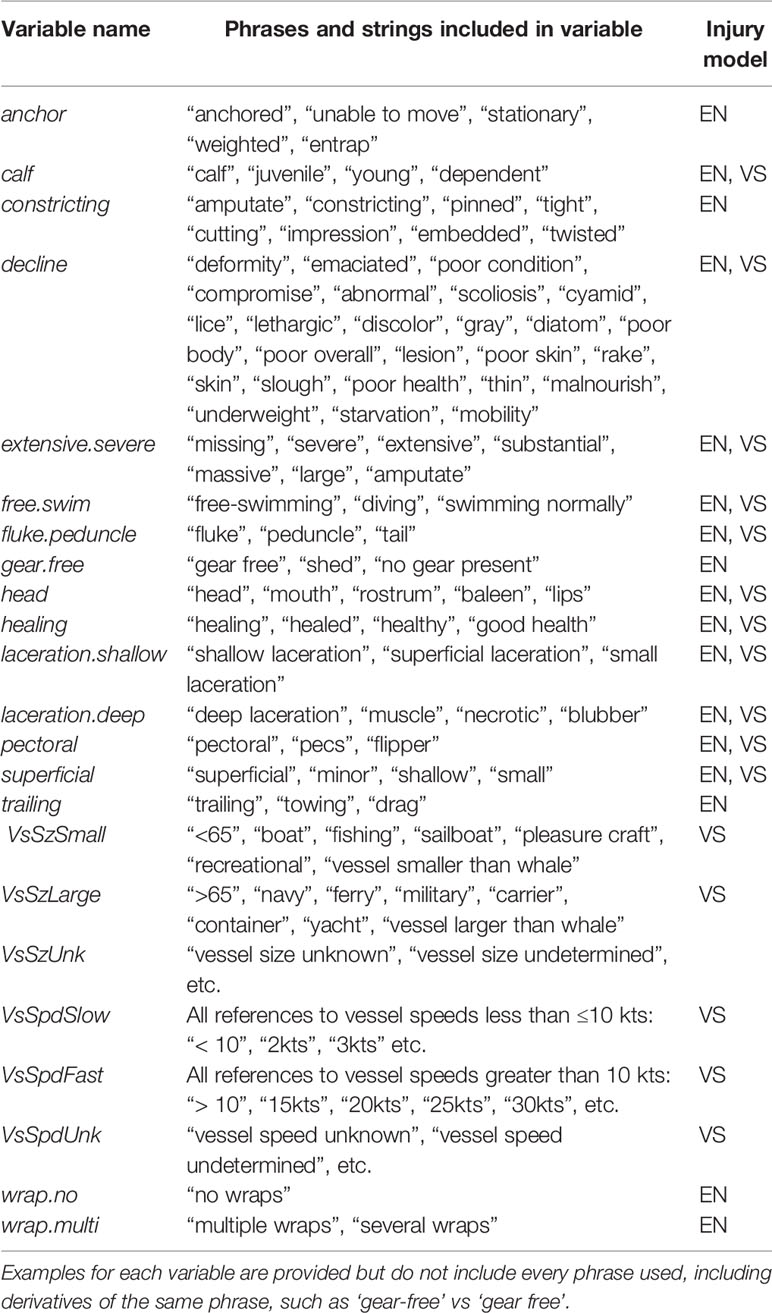
Table 2 List of narrative words, phrases or characters used as variables in Random Forest entanglement (EN) and vessel strike (VS) models.
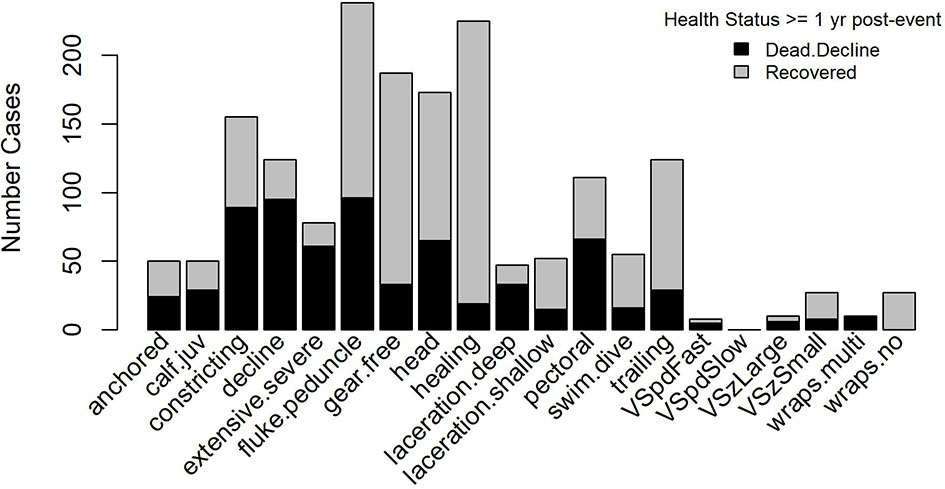
Figure 1 Occurrence of variables from entanglement and vessel strike narratives, stratified by known health status ≥ 1 year post-event. In most injury cases, vessel size and speed were unknown and effectively represented a variable with a constant value. Thus the number of ‘VsSzUnk’ and ‘VsSpdUnk’ values are not shown to prevent y-axis distortion that would mask patterns evident for known vessel sizes and speeds.
Random Forest Classification Models
Random Forest (hereafter ‘RF’) models (Breiman et al., 1984; Breiman, 2001a; Breiman, 2001b) were created to classify the health outcomes of large whale entanglements and vessel strikes using the R programming language (R Core Team, 2020) and the packages randomForest, version 4.6-14 (Liaw and Wiener, 2002) and rfPermute version 2.5 (Archer, 2021). RF models are a recursive partitioning algorithm that use random subsets of variables to split data into successive daughter nodes (see Supplementary Material). The variable that maximizes the purity of classes in daughter nodes is chosen for each split. Our response variable is a binary class of health status: ‘Dead.Decline’ or ‘Recovered’. A perfect data split would fully-separate ‘Dead.Decline’ and ‘Recovered’ cases, or maximize the Gini coefficient of data in subsequent nodes (Gini, 1921). Splits continue until terminal nodes contain a single response class. Each tree is constructed from a random subsample of the cases and many trees are grown, which prevents overfitting of data that can occur with single trees and produces robust predictive models when variables are informative (Breiman, 2001a; Breiman, 2001b). Cases omitted from the construction of each tree in the forest are called ‘out-of-bag’ (OOB) and are used for cross-validation. Each tree then predicts the status of cases that were OOB (either ‘Dead.Decline’ or ‘Recovered’), based on the status of the training cases in the terminal nodes they are assigned to. The fraction of trees in a forest voting for a class for each case when it was OOB is used to estimate the error rate of the model. These errors are summarized as a confusion matrix for all response classes.
The relative importance of predictors in classifications of the RF model is assessed by permuting each predictor, and the resulting decreases in classification accuracy are measured. Important variables will result in the largest decreases in classification accuracy, while unimportant variables result in negligible decreases. For each predictor, an importance ‘score’ is defined as the mean decrease in classification accuracy (number of additional cases misclassified) across all trees when it was permuted.
Random Forest injury models included 1000 individual trees, each constructed from equal numbers of ‘Dead.Decline’ and ‘Recovered’ cases (n=1/2 of smaller class size). This balanced data approach is analogous to a uniform prior distribution and is necessary to mitigate bootstrap oversampling of majority response classes, resulting in poor classification accuracy for minority classes when response class frequencies are highly-unbalanced (Chawla et al., 2004; Anaissi et al., 2013). We use this approach because we are equally concerned with correctly classifying ‘Dead.Decline’ and ‘Recovered’ response classes and our data are imbalanced with respect to these classes. To evaluate RF model performance, we compare OOB classification accuracy to that expected by chance, which is based on the respective sample sizes of each response class. Because each tree is constructed with an equal number of cases from each response class, the classification accuracy expected by chance = 50%.
RF Model Application to Novel Data
Entanglements and vessel strikes with unknown outcomes were predicted using the RF models to yield probability assignments of ‘Dead.Decline’ or ‘Recovered’, based on the fraction of trees predicting each response. For example, a RF model of 1000 trees may yield 920 predictions of ‘Dead.Decline’ vs 80 predictions of ‘Recovered’ for a given injury case. The probability of ‘Dead.Decline’ for that case is p = 0.92 and the ‘majority prediction’ is ‘Dead.Decline’. In this paper, ‘classification’ refers to RF assignments of health status for known outcome cases, vs ‘predictions’ that represent probability-based assignments of health status for unknown outcome cases.
For right and humpback whales combined, we compared RF classifications and probabilistic predictions of known and unknown outcomes with injury determinations made by biologists applying current injury protocols and MSI assignments to the same cases, to identify any notable differences in estimated numbers of deaths or serious injuries between methods. Our emphasis for comparison with MSI totals is RF majority predictions of Dead.Decline (total cases with Dead.Decline probabilities > 0.50, not the sum of predicted Dead.Decline probabilities), because the definition of a serious injury under the MMPA is one that results in a greater than 50% chance of mortality.
Results
Classification accuracy of health status (Dead.Decline vs Recovered) for known outcome right and humpback whale entanglements and vessel strikes ≥ 1 yr post-event greatly exceeded the 50% accuracy rate expected by chance (Tables 3A–D). Entanglement health status ≥ 1 yr post-event was correctly classified in 95.7% [95% CI = 89.2% - 98.8%] of right whale and 93.6% [88.2% - 97.0%] of humpback whale cases (Tables 3A, C). Vessel strike health status ≥ 1 yr post-event was correctly classified in 91.4% [81.0% - 97.1%] of right whale and 88.6% [79.5% - 94.7%] of humpback whale cases (Tables 3B, D).
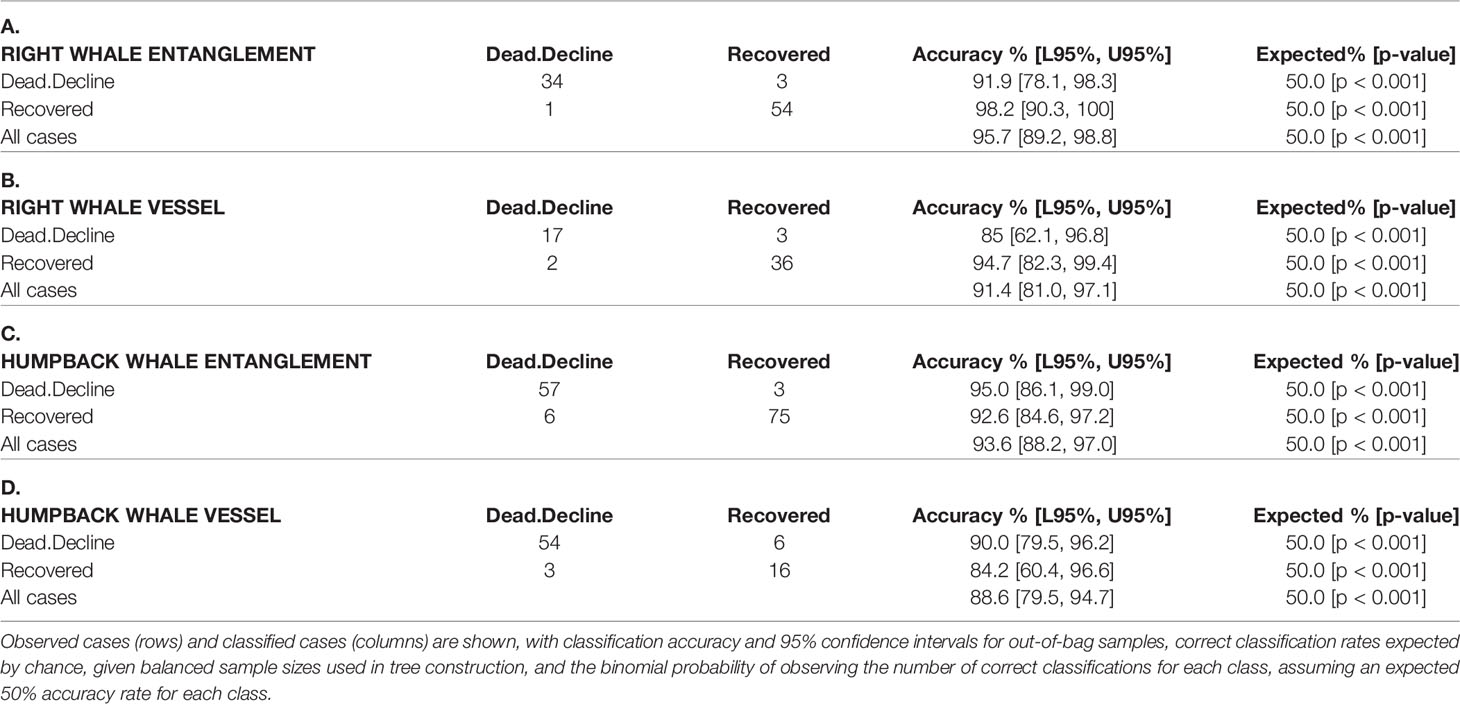
Table 3 A–D Confusion matrices for entanglement and vessel strike models from known outcome health status cases of right and humpback whales.
Variable importance varied by species and injury source (Figures 2, 3). The most important entanglement variables, based on the largest decreases in classification accuracy when individual variables were permuted, included ‘healing’, ‘gear free’, ‘pectoral’ and ‘decline’. Entanglement variables resulting in the largest decreases in classification accuracy when permuted were ‘healing’ and ‘gear free’ for right and humpback whales, respectively. The most-important vessel strike variables included ‘VessSz’, ‘healing’, ‘head’, ‘calf.juv’ and ‘laceration.shallow’ for both right and humpback whales. The vessel strike variable resulting in the largest decreases in classification accuracy when permuted was ‘healing’ for both species (Figures 2, 3).
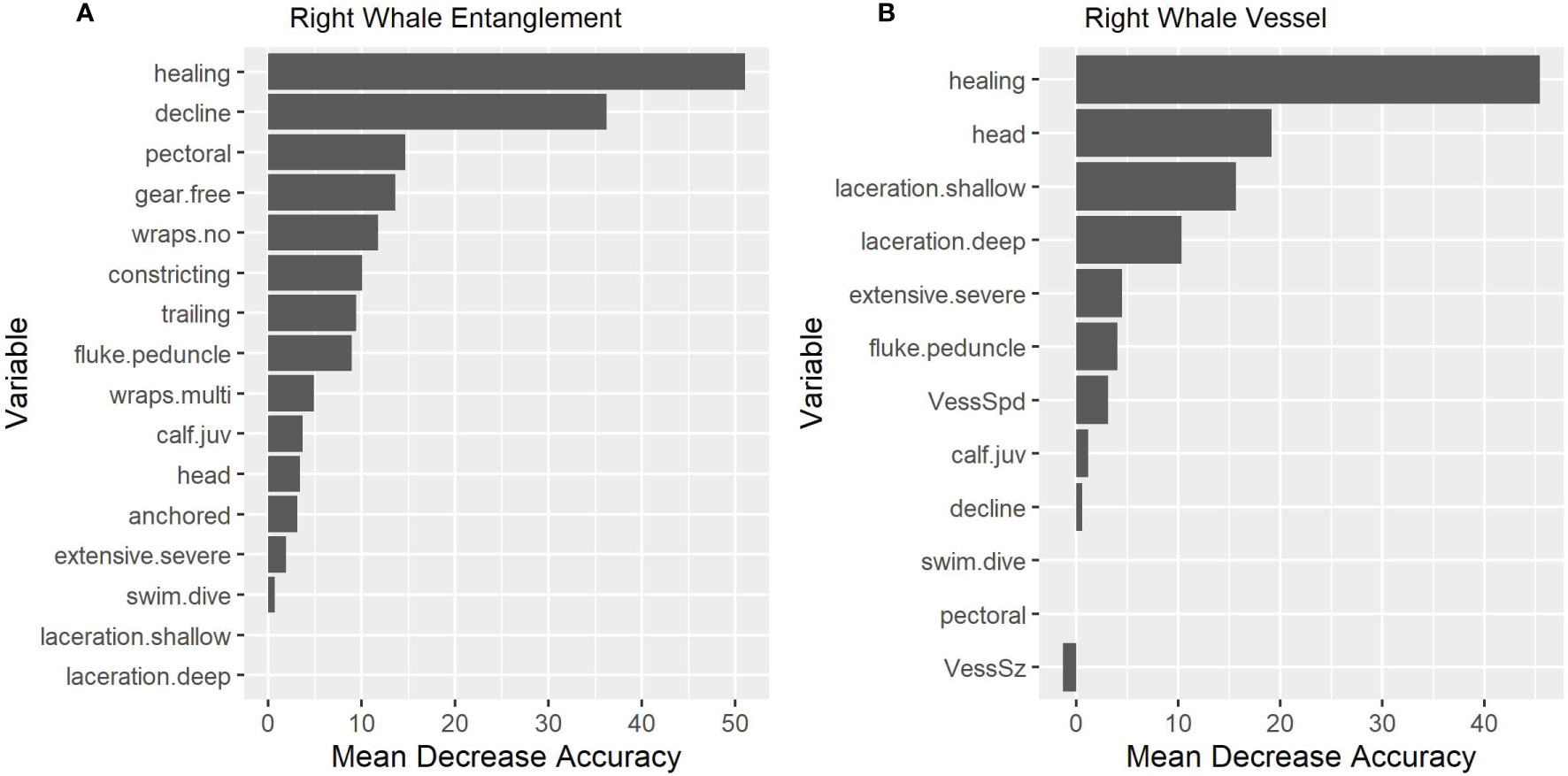
Figure 2 Variable importance for entanglement (A) and vessel strike (B) models for right whales. The x-axis shows the mean decrease in classification accuracy (number of additional cases misclassified across all trees) when individual variables are permuted.
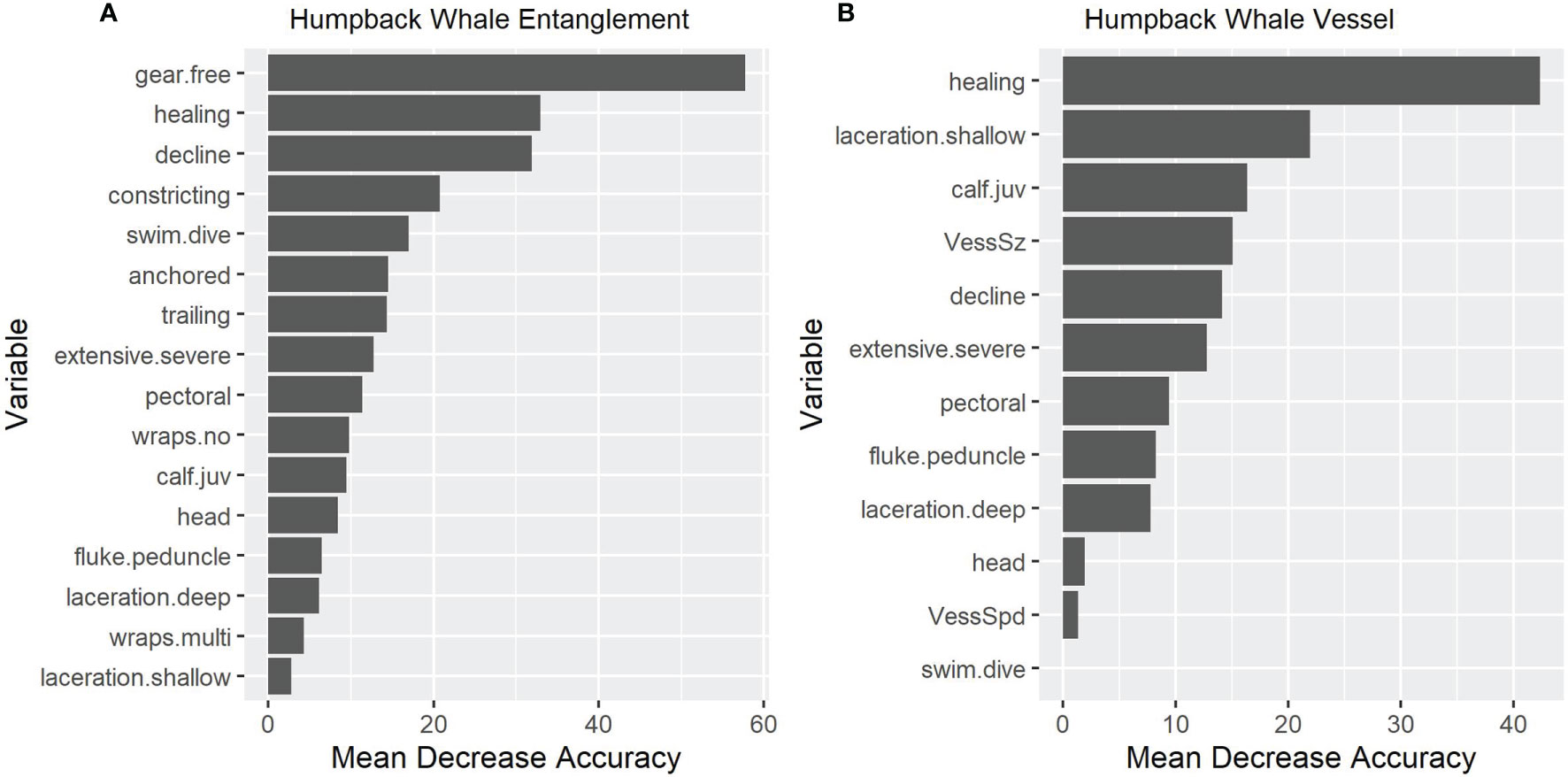
Figure 3 Variable importance for entanglement (A) and vessel strike (B) models for humpback whales. The x-axis shows the mean decrease in classification accuracy (number of additional cases misclassified across all trees) when individual variables are permuted.
Generally, RF predictions of health status agreed with MSI values assigned by biologists for known outcome entanglements and vessel strikes. In contrast, for unknown outcomes where biologists assigned MSI values of 0 (a non-serious injury), corresponding RF Dead.Decline probabilities were notably higher (Figures 4, 5). The sum of MSI values assigned to known outcome entanglement and vessel strikes by biologists using current injury protocols was similar to sums of RF majority predictions of deaths or health declines, with ∑MSI/RF majority prediction ratios close to unity (Table 4). In contrast, the sum of MSI assignments for unknown outcomes were lower than RF majority prediction totals for both injury sources. Differences in ∑MSI/RF majority prediction ratios were apparent between known and unknown outcomes (entanglements: p=0.22, odds ratio=1.24 and vessel strikes: p=0.008, odds ratio=2.72, Fisher Exact test) (Table 4 and Figures 4, 5). For right and humpback whales combined, the sum of RF majority predictions of deaths or health declines for known outcome entanglements (98) was equal to the sum of MSI assignments (98) (Table 4). In contrast, for unknown outcome entanglements, the sum of RF majority predictions of deaths or health declines (223) were 25% higher than MSI assignments (178.8) (Table 4). For known outcome vessel strikes, the sum of RF majority predictions of deaths or health declines (76) closely agreed with the sum of MSI assignments (80) (Table 4). In contrast, the sum of RF majority Dead.Decline predictions for unknown outcome vessel strikes (29) was much higher than the sum of MSI assignments (11.2), but was based on only 23 injury cases, excluding 7 cases where prorated vessel strike MSI assignments totaling 3.2 whales were made (Figure 5 and Table 4).
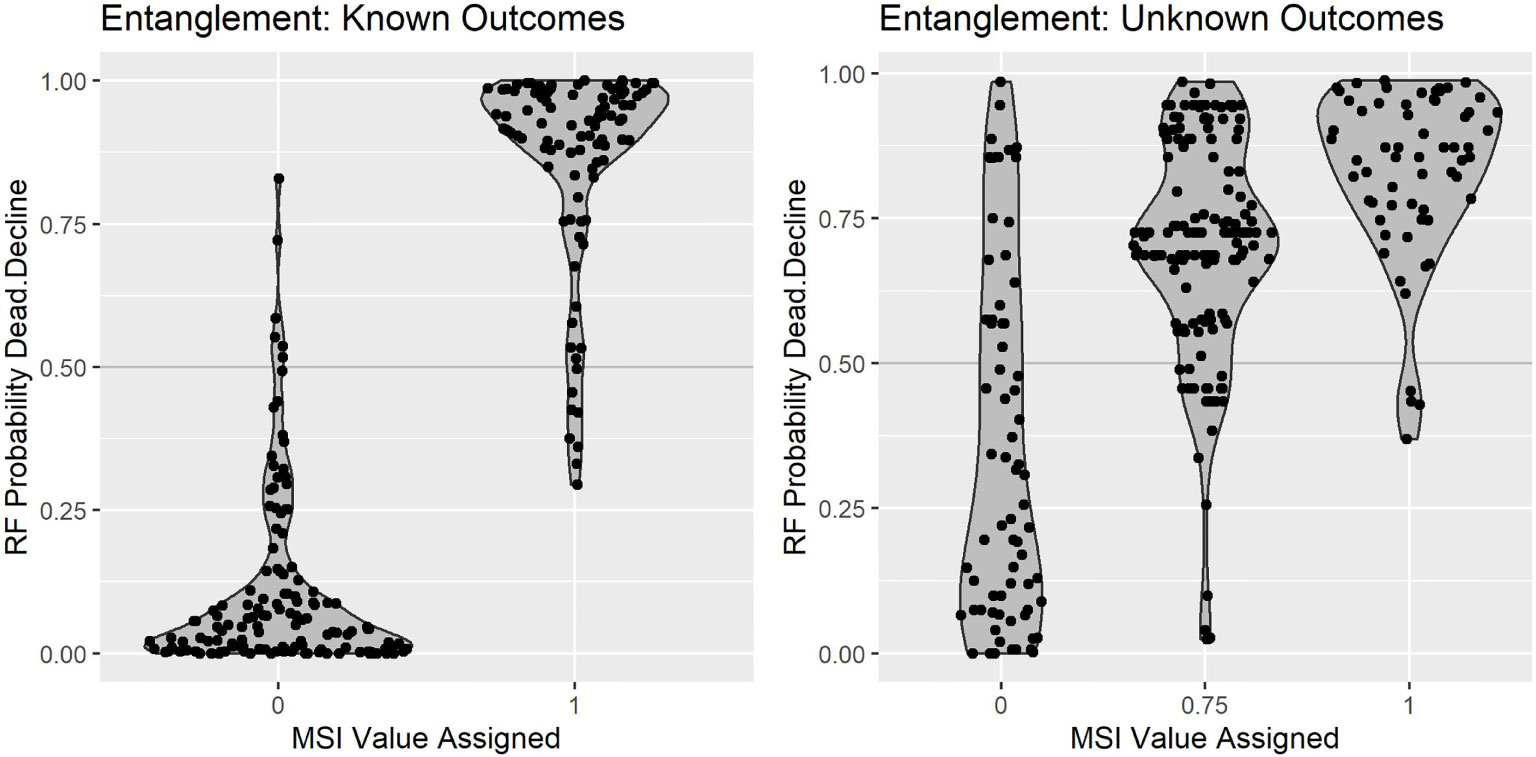
Figure 4 RF predicted probabilities of ‘Dead.Decline’ vs MSI values assigned by scientists using current serious injury guidelines for entanglement cases of right and humpback whales, combined. Non-serious injuries are represented by MSI values = 0 and serious injuries are represented by all other values.
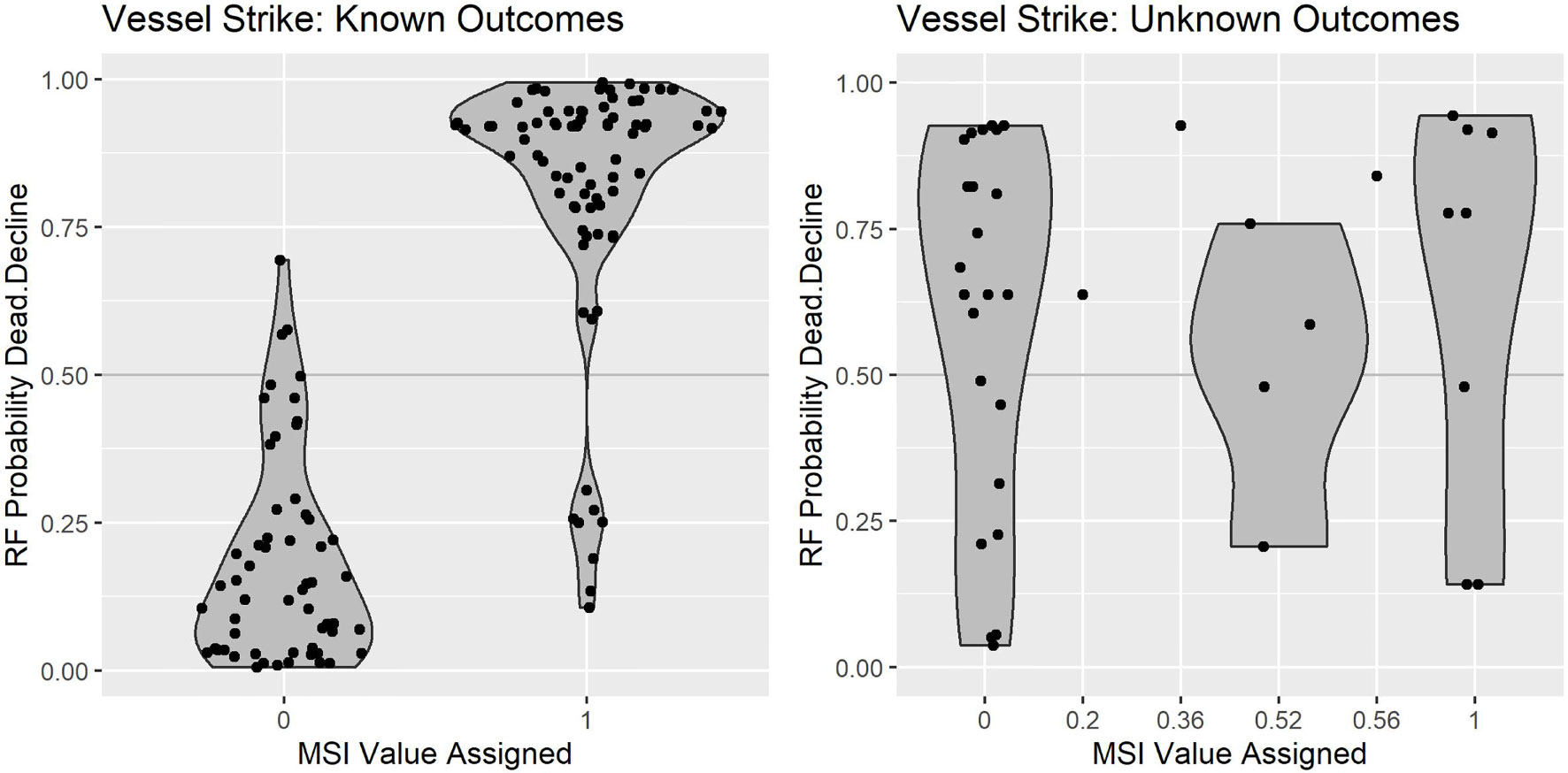
Figure 5 RF predicted probabilities of ‘Dead.Decline’ vs MSI values assigned by scientists using current serious injury guidelines for vessel strike cases of right and humpback whales, combined. Non-serious injuries are represented by MSI values = 0 and serious injuries are represented by all other values.
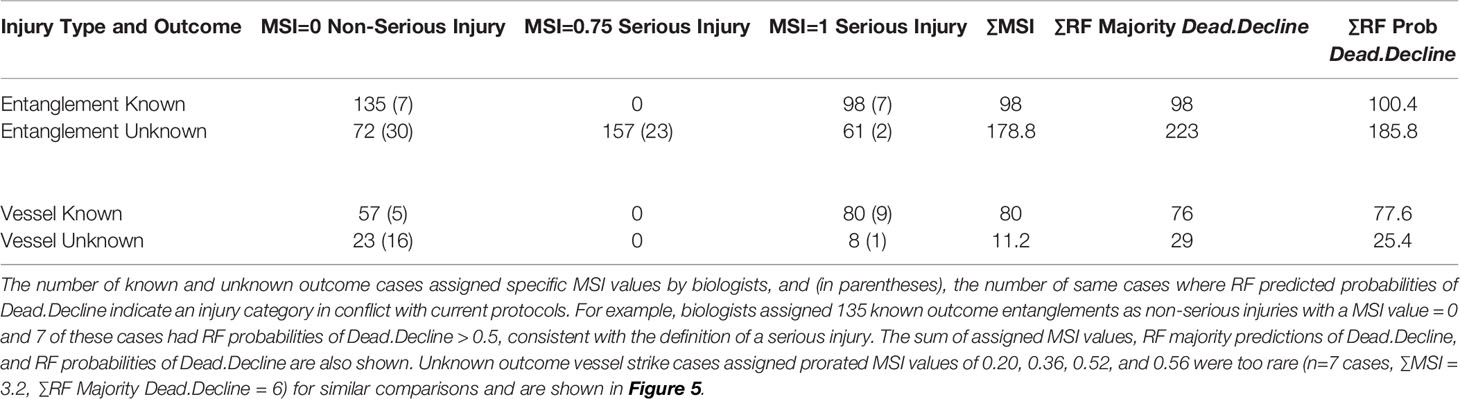
Table 4 Comparison of MSI assignments using current injury protocols and Random Forest (RF) predictions of health status for combined right whale and humpback whale entanglements and vessel strikes.
Discussion
Current large whale serious injury assessment uses a decision-tree set of rules applied to cases by biologists and assignment of non-serious vs serious injuries is based on observed ratios of deaths to total cases and expert opinion from 2004 - 2008 data (Andersen et al., 2008; NOAA, 2012b). In this study, we have automated this process by training a Random Forest classification model and using additional data. The results of our model suggest improved performance with some notable enhancements over the previous method. The clearest insights come from comparing known and unknown outcome MSI assignments using current injury protocols and Random Forest classification trees. Most notable was that MSI assignments made by biologists using current protocols were in excellent agreement with RF predictions of health status for known outcome cases. This isn’t unexpected, given the high assignment accuracy rates for known outcomes. However, for unknown outcomes, RF probability assignments of deaths and health declines were consistently higher than assigned MSI values, suggesting that current injury protocols may underestimate true impacts of entanglements and vessel strikes for cases lacking longer observation periods needed to document serious injuries or gear burdens not visible during brief encounters.
Predicted entanglement serious injury probabilities from RF models were similar to MSI assignments from biologists for known outcomes (Figure 4). In 135 known outcome entanglements where a MSI = 0 was assigned, the median RF probability of Dead.Decline was 0.138 [95% CI = 0 – 0.604] and only 7/135 (5%) of these cases had RF Dead.Decline probabilities > 0.5 that would imply a serious injury. In contrast, where biologists assigned a MSI value of 0 to unknown outcome entanglements, RF probabilities of Dead.Decline were > 0.5 for 30/72 (42%) of the cases, implying that entanglement risk for unknown outcomes using current protocols is underestimated. Known outcome entanglements assigned a MSI = 1 (n=98) had a median RF Dead.Decline probability = 0.92 [95% = 0.36 – 1.00] and only 7/98 (7%) of cases were assigned a Dead.Decline probability < 0.5 (Figure 4 and Table 4). For unknown outcome entanglements where a MSI = 1 was assigned, the median RF probability of Dead.Decline was 0.873 [95% CI = 0.548 - 0.977] and 2/61 (3%) of cases were assigned a Dead.Decline probability <0.5. For unknown outcome entanglements lacking detail on the amount and configuration of fishing gear or injury severity with assigned MSI values = 0.75, the median predicted RF probability of ‘Dead.Decline’ was 0.664 [95% CI = 0.345 – 0.941] for both species combined, with 23/157 (15%) cases assigned a ‘Dead.Decline’ probability <0.5, implying a non-serious injury (Figure 4). Evaluating the robustness of the ‘0.75 MSI’ entanglement proration factor used in current injury protocols is not straightforward, as it is derived from a sample of 114 known outcome entanglement cases from 2004-2008, where 85 resulted in deaths or health declines (NOAA, 2012a; NOAA, 2012b), while the current study uses twice as many cases (233 over the period 2000 - 2018) to generate predictive models. Current injury policy reflects entanglement and vessel strike conditions encountered by whales during the 2004-2008 period. If there are changes in the severity of injuries incurred over time, for example, if rope strength involved in entanglements increases over time, then the severity of entanglements may be greater in more recent years. This is reflected in known-outcome cases and therefore, is included in RF models, but may mean that current injury policies could underestimate entanglement risk for unknown outcomes. Agreement between MSI assignments and RF predictions for known outcome entanglements reflects that the variables used in each method allow for robust injury assessment, but performance of the current injury protocol for unknown outcomes assigned MSI values = 0 appears to underestimate risk of death or health decline compared with RF predictions.
For vessel strikes, differences in MSI values assigned by biologists and RF predictions for unknown outcome outcomes were apparent, particularly in cases where biologists assigned a MSI value of zero (Figure 5). The median RF probability of Dead.Decline for unknown outcome vessel strikes determined to be non-serious injuries with a MSI value = 0 was 0.57 [95% CI = 0.03 – 0.98] and 16/23 (69%) of these cases were assigned a ‘Dead.Decline’ probability > 0.5, implying that they were serious injuries (Figure 5 and Table 4). This suggests that the assigned risk of death or health decline for unknown outcome vessel strikes is underestimated with current protocols. Higher RF predictions of Dead.Decline for unknown outcome vessel strikes assigned a MSI value = 0 suggests that such ‘non-serious injury’ cases may lack longitudinal evidence of a health decline that may take many months to appear (Moore and Van der Hoop, 2012), or that evidence of a serious injury is not observed due to the brief encounter with the whale, as is typical with vessel strikes. For unknown outcome vessel strikes where biologists assigned a MSI value = 1, the corresponding RF probabilities of Dead.Decline were 0.869 [95% CI = 0.469 – 0.943] and 7/8 (88%) of these cases were assigned Dead.Decline probabilities > 0.5 (Figure 5, Table 4). Five cases involved observations of deep propeller lacerations, two cases involved fast vessels much larger than the whale (> 10 kts and >65 ft), and the last case was a dependent calf of a mother killed by a vessel strike, which under current protocols, are automatically assigned as serious injuries with a MSI value = 1 (NOAA, 2012a; NOAA, 2012b).
In entanglement RF models, the importance of the variable ‘gear.free’ was evident not only from its large contribution to OOB error rate reduction in known outcome humpback whale cases, but also in how it influences predictions for unknown outcomes. For humpback whale entanglements with unknown outcomes, 41/257 were positive for the variable ‘gear.free’. Almost all gear free cases (37/41) had a majority prediction of ‘Recovered’, with a mean recovery probability = 0.791. In contrast, the mean recovery probability for 216 cases lacking the variable ‘gear.free’ was 0.315, with 194 cases predicted as ‘Dead.Decline’ and 22 as ‘Recovered’. For right whale entanglements, unknown outcomes were limited to 33 cases and the most important variable was ‘healing’. The mean recovery probability was 0.634 for 7 cases including reference to healing, compared to a mean recovery probability of 0.253 for 26 cases lacking reference to healing.
Automated data-mining of case narratives to identify and generate predictor variables poses challenges, including inadvertently identifying variable presence when reference to it actually indicates absence. Words such as ‘blood’ are expected to contain important clues about health status. However, we found the word ‘blood’ was as likely to be used to indicate an absence of blood (“no blood observed in water”) as to note its presence (“small amount of blood observed in water”). This requires editing of narratives to make presence/absence declarations explicit or involves expansion of search phrases to account for wider language use variance. Ultimately, we found the number of cases using the word ‘blood’ was relatively small, its utility in predictive models was negligible, and its presence was largely associated with necropsy cases, thus we omitted it from models. Other challenges include recognition of shorthand vernacular phrases, such as the word ‘prop’ to reference propeller marks on whales. This necessitates data-mining for the use of ‘prop’, while ensuring that words like ‘proper’ are not confounded for this variable. Application of the RF method to case narratives will require some combination of language standards to be implemented for narratives, in addition to careful editing of existing narratives to address confounding issues. Despite these challenges, RF model accuracy was much higher than expected by chance for both entanglement and vessel strike cases.
Conclusion
Our RF models include entanglements and vessel strikes of two species, but many large whale species are injured or killed from these sources. Our focus on right and humpback whales reflects that they are commonly involved in entanglement and vessel strike cases with long-term sighting histories, facilitated by ease of individual identification. In contrast, more pelagic species such as blue and fin whales are difficult to individually track over time, due to their offshore distribution and challenges in photographically identifying individuals. Although entanglement and vessel strike impacts may differ by species, there are insufficient data to generate species-specific models for all species. Models based on right and humpback whale observations likely have good utility for predicting the health status for other species, as ‘severe’ and ‘superficial’ injury narratives are likely to be equally-informative across species. Current NOAA serious injury policy utilizes a unified decision-tree framework applied by biologists that includes multiple species. Our models represent a ‘proof-of-concept’ that injury narratives can be data-mined for variables used to predict health status with high accuracy. Such models may be tailored to multispecies assessments by pooling all species into one entanglement or vessel strike model, though species represented by only a few known outcome cases will contribute little to overall inference.
Data Availability Statement
Publicly available datasets were analyzed in this study. This data can be found here: https://github.com/JimCarretta/WhaleInjuryData-and-Code and https://github.com/JimCarretta/WhaleInjuryCovariates.
Ethics Statement
Ethical review and approval was not required for the animal study because Whale injury cases are opportunistically observed. They are not experimental subjects.
Author Contributions
JC conceptualized the study, conducted the analyses, and created the tables and figures. AH maintained data from Atlantic injury records that formed the foundation of the data format used in the study and assigned and/or reviewed serious injury determinations from both Pacific and Atlantic cases. JC and AH wrote the manuscript. All authors contributed to manuscript revision, and read and approved the submitted version.
Funding
NOAA Fisheries Northeast Fisheries Science Center supported AH. NOAA Fisheries Southwest Fisheries Science Center supported JC. Funding from the Southwest Fisheries Science Center were used for publication fees.
Conflict of Interest
The authors declare that the research was conducted in the absence of any commercial or financial relationships that could be construed as a potential conflict of interest.
Publisher’s Note
All claims expressed in this article are solely those of the authors and do not necessarily represent those of their affiliated organizations, or those of the publisher, the editors and the reviewers. Any product that may be evaluated in this article, or claim that may be made by its manufacturer, is not guaranteed or endorsed by the publisher.
Acknowledgments
Canadian Wildlife Health Cooperative, Cascadia Research Collective, Department of Fisheries and Oceans Canada, Grand Manan Whale and Seabird Research Station, Humboldt State University, Marine Animal Response Society, New England Aquarium, NMFS West Coast Region, Northeast Fisheries Observer Program, Oregon State University, Provincetown Center for Coastal Studies, Sea to Shore Alliance, The Marine Mammal Center, U.S. Coast Guard, U.S. Navy, Whale Release and Strandings Group, Whale Center of New England and members of the public provided sighting information used in this work. The manuscript was improved from reviews and discussions with Eric Archer, Alex Curtis, Karin Forney, and two independent reviewers. We thank the Large Whale Serious Injury Working Group and NMFS Scientific Review Groups for thoughtful discussions on development of this approach.
Supplementary Material
The Supplementary Material for this article can be found online at: https://www.frontiersin.org/articles/10.3389/fmars.2022.863070/full#supplementary-material
References
Anaissi A., Kennedy P. J., Goyal M., Catchpoole D.R.. 2013. A Balanced Iterative Random Forest for Gene Selection From Microarray Data. BMC Bioinf. 14(1):1–10, 261. doi: 10.1186/1471-2105-14-261
Andersen M. S., Forney K. A., Cole T. V., Eagle T., Angliss R., Long K., et al. (2008). “Differentiating Serious and Non-Serious Injury of Marine Mammals: Report of the Serious Injury Technical Workshop,” in NOAA Tech. Memo. NMFS-OPR, vol. 39. , 94.
Angliss R. P., DeMaster D. P. (1998). “Differentiating Serious and Non-Serious Injury of Marine Mammals Taken Incidental to Commercial FO Operations,” in NOAA Tech. Memo. NMFS-OPR-13, vol. 48.
Archer E. (2021). GitHub - EricArcher/rfPermute: Estimate Permutation P-Values for Random Forest Importance Metrics Version 2.5.
Breiman L. (2001b). Statistical Modeling: The Two Cultures (With Comments and a Rejoinder by the Author). Stat. Sci. 16 (3), 199–231. doi: 10.1214/ss/1009213726
Breiman L., Friedman J., Stone C. J., Olshen R. A. (1984). Classification and Regression Trees (CRC press).
Caliskan A., Bryson J. J., Narayanan A. (2017). Semantics Derived Automatically from Language Corpora Contain Human-Like Biase. Sci. 356, 183–186. doi: 10.1126/science.aal4230
Carretta J. V. (2021) R-Package ‘Whaleinjurycovariates’. Available at: https://github.com/JimCarretta/WhaleInjuryCovariates/blob/main/R/WhaleInjuryCovariates.R.
Carretta J. V., Danil K., Chivers S. J., Weller D. W., Janiger D. S., Berman-Kowalewski M., et al. (2016). Recovery Rates of Bottlenose Dolphin (Tursiops Truncatus) Carcasses Estimated From Stranding and Survival Rate Data. Mar. Mamm. Sci. 32 (1), 349–362. doi: 10.1111/mms.12264
Carretta J. V., Delean B., Helker V., Muto M. M., Greenman J., Wilkinson K., et al. (2020). “Sources of Human-Related Injury and Mortality for U.S. Pacific West Coast Marine Mammal Stock Assessment-2018,” in NOAA Technical Memorandum, vol. 631. , 147. Available at: https://repository.library.noaa.gov/view/noaa/25230.
Chawla N. V., Japkowicz N., Kotcz A. (2004). Special Issue on Learning From Imbalanced Data Sets. ACM SIGKDD Explor. Newslett. 6 (1), 1–6. doi: 10.1145/1007730.1007733
Clapham P. J., Young S., Brownell R. J. (1999). Baleen Whales: Conservation Issues and the Status of the Most Endangered Populations. Mammal Rev. 29, 35–60. doi: 10.1046/j.1365-2907.1999.00035.x
Conn P. B., Silber G. K. (2013). Vessel Speed Restrictions Reduce Risk of Collision-Related Mortality for North Atlantic Right Whales. Ecosphere 4 (4), 1–16. doi: 10.1890/ES13-00004.1
Dahllöf M. (2012). Automatic Prediction of Gender, Political Affiliation, and Age in Swedish Politicians From the Wording of Their Speeches—A Comparative Study of Classifiability. Literary Linguistic Computing 27 (2), 139–153. doi: 10.1093/llc/fqs010
Gabrielatos C., Baker P. (2008). Fleeing, Sneaking, Flooding: A Corpus Analysis of Discursive Constructions of Refugees and Asylum Seekers in the UK Pres-2005. J. English Linguistics 36 (1), 5–38. doi: 10.1177/0075424207311247
Gilman E., Suuronen P., Hall M., Kennelly S. (2013). Causes and Methods to Estimate Cryptic Sources of Fishing Mortality. J. Fish Biol. 83 (4), 766–803. doi: 10.1111/jfb.12148
Gini C. (1921). Measurement of Inequality of Incomes. Economic J. 31 (121), 124–126. doi: 10.2307/2223319
Harting A. L., Barbieri M. M., Baker J. D., Mercer T. A., Johanos T. C., Robinson S. J., et al. (2021). Population-Level Impacts of Natural and Anthropogenic Causes-of-Death for Hawaiian Monk Seals in the Main Hawaiian Islands. Mar. Mamm. Sci. 37, 235–250. doi: 10.1111/mms.12742
Hayes S. A., Josephson E., Maze-Foley K., Rosel P. E. (Eds.) (2019). “US Atlantic and Gulf of Mexico Marine Mammal Stock Assessments – 2018,” in NOAA Technical Memorandum NMFS-NE-258, 306.
Henry A. G., Garron M., Morin D., Reid A., Ledwell W., Cole T. V. N. (2020) Serious Injury and Mortality Determinations for Baleen Whale Stocks along the Gulf of Mexico, United States East Coast, and Atlantic Canadian Provinces, 2013-2017. 20-06, 53. doi: 10.25923/fbc7-ky15
Kelley D. E., Vlasic J. P., Brillant S. W. (2020). Assessing the Lethality of Ship Strikes on Whales Using Simple Biophysical Models. Mar. Mam. Sci. 2020, 1–17. doi: 10.1111/mms.12745
Knowlton A. R., Hamilton P. K., Marx M. K., Pettis H. M., Kraus S. D. (2012). Monitoring North Atlantic Right Whale Eubalaena Glacialis Entanglement Rates: A 30 Year Retrospective. Mar. Ecol. Prog. Ser. 466, 293–302. doi: 10.3354/meps09923
Kraus S. D., Brown M. W., Caswell H., Clark C. W., Fujiwara M., Hamilton P. K., et al. (2005). North Atlantic Right Whales In Crisis. Science 309, 561–562. doi: 10.1126/science.1111200
Mastro D. E., Blecha E., Seate A. (2011). Characterizations of Criminal Athletes: A Systematic Examination of Sports News Depictions of Race and Crime. J. Broadcasting Electronic Media 55 (4), 526–542. doi: 10.1080/08838151.2011.620664
Moore M. J., Van der Hoop J. M. (2012). The Painful Side of Trap and Fixed Net Fisheries: Chronic Entanglement of Large Whales. J. Marine Biol. 2012, 1–4. doi: 10.1155/2012/230653
Neilson J. L., Straley J. M., Gabriele C. M., Hills S. (2009). Non-Lethal Entanglement of Humpback Whales (Megaptera Novaeangliae) in Fishing Gear in Northern Southeast Alaska. J. Biogeography 36, 452–464. doi: 10.1111/j.1365-2699.2007.01820.x
NOAA (2012a). National Policy for Distinguishing Serious From Non-Serious Injuries of Marine Mammals. Federal Register 77, 3233.
NOAA (2012b) Process for Distinguishing Serious From Non-Serious Injury of Marine Mammals. Available at: https://media.fisheries.noaa.gov/dam-migration/02-238-01.pdf.
Pace R. M. III, Williams R., Kraus S. D., Knowlton A. R., Pettis H. M. (2021). Cryptic Mortality of North Atlantic Right Whales. Conserv. Sci. Pract. 3, e346. doi: 10.1111/csp2.346
Pettis H. M., Rolland R. M., Hamilton P. K., Brault S., Knowlton A. R., Kraus S. D. (2004). Visual Health Assessment of North Atlantic Right Whales (Eubalaena Glacialis) Using Photographs. Can. J. Zool. 82, 8–19. doi: 10.1139/z03-207
Pettis H. M., Rolland R. M., Hamilton P. K., Knowlton A. R., Burgess E. A., Kraus S. D. (2017). Body Condition Changes Arising From Natural Factors and Fishing Gear Entanglements in North Atlantic Right Whales Eubalaena Glacialis. Endang. Species Res. 32, 237–249. doi: 10.3354/esr00800
Prado J. H. F., Secchi E. R., Kinas P. G. (2013). Mark-Recapture of the Endangered Franciscana Dolphin (Pontoporia Blainvillei) Killed in Gillnet Fisheries to Estimate Past Bycatch From Time Series of Stranded Carcasses in Southern Brazil. Ecol. Indic. 32, 35–41. doi: 10.1016/j.ecolind.2013.03.005
R Core Team (2020). “R: A Language and Environment for Statistical Computing,” in R Foundation for Statistical Computing(Vienna, Austria). Available at: https://www.R-project.org/.
Robbins J., Knowlton A. R., Landry S. (2015). Apparent Survival of North Atlantic Right Whales After Entanglement in Fishing Gear. Biol. Conserv. 191, 421–427. doi: 10.1016/j.biocon.2015.07.023
Rockwood R. C., Calambokidis J., Jahncke J. (2017). High Mortality of Blue, Humpback and Fin Whales From Modeling of Vessel Collisions on the U.S. West Coast Suggests Population Impacts and Insufficient Protection. PloS One 12 (8), e0183052. doi: 10.1371/journal.pone.0183052
Rolland R. M., Schick R. S., Pettis H. M., Knowlton A. R., Hamilton P. K., Clark J. S., et al. (2016). Health of North Atlantic Right Whales Eubalaena Glacialis Over Three Decades: From Individual Health to Demographic and Population Health Trends. Mar. Ecol. Prog. Ser. 542, 265–282. doi: 10.3354/meps11547
Sharp S. M., McLellan W. A., Rotstein D. S., Costidis A. M., Barco S. G., Durham K., et al. (2019). Gross and Histopathologic Diagnoses From North Atlantic Right Whale Eubalaena Glacialis Mortalities Between 2003 and 2018. Dis. Aquat. Organisms 135 (1), 1–31. doi: 10.3354/dao03376
van der Hoop J., Corkeron P., Moore M. (2017). Entanglement is a Costly Life-History Stage in Large Whales. Ecol. Evol. 7 (1), 92–106. doi: 10.1002/ece3.2615
Wade P. R. (1998). Calculating Limits to the Allowable Human-Caused Mortality of Cetaceans and Pinnipeds. Mar. Mamm. Sci. 14, 1–37. doi: 10.1111/j.1748-7692.1998.tb00688.x
Wells R. S., Allen J. B., Lovewell G., Gorzelany J., Delynn R. E., Fauquier D. A., et al. (2015). Carcass-Recovery Rates for Resident Bottlenose Dolphins in Sarasota Bay, Florida. Marine Mammal Sci. 31 (1), 355–368. doi: 10.1111/mms.12142
Keywords: whale, injuries, vessel strike, entanglement, random forest, classification tree
Citation: Carretta JV and Henry AG (2022) Risk Assessment of Whale Entanglement and Vessel Strike Injuries From Case Narratives and Classification Trees. Front. Mar. Sci. 9:863070. doi: 10.3389/fmars.2022.863070
Received: 26 January 2022; Accepted: 17 May 2022;
Published: 24 June 2022.
Edited by:
Phil J. Bouchet, University of St Andrews, United KingdomReviewed by:
Elanor Bell, Australian Antarctic Division, AustraliaAmy Richardson Knowlton, New England Aquarium, United States
Copyright © 2022 Carretta and Henry. This is an open-access article distributed under the terms of the Creative Commons Attribution License (CC BY). The use, distribution or reproduction in other forums is permitted, provided the original author(s) and the copyright owner(s) are credited and that the original publication in this journal is cited, in accordance with accepted academic practice. No use, distribution or reproduction is permitted which does not comply with these terms.
*Correspondence: James V. Carretta, amltLmNhcnJldHRhQG5vYWEuZ292