- 1Department of Biology, Lund University, Lund, Sweden
- 2Department of Arctic and Marine Biology, Arctic University of Norway, Tromsø, Norway
- 3Faculty of Biosciences, Fisheries and Economics, Arctic University of Norway, Tromsø, Norway
- 4Norwegian Polar Institute, Tromsø, Norway
- 5Department of Marine Ecosystem Acoustics, Institute of Marine, Research, Bergen, Norway
- 6Department of Marine Mammals, Institute of Marine Research, Tromsø, Norway
Singing behaviour by male humpback whales (Megaptera novaeangliae) has traditionally been associated with low-latitude breeding grounds. However, in recent years, this vocal behaviour has been increasingly reported outside these areas. All singers in a given population sing the same version of a song and this song is continually evolving over time with modifications on different levels within the song structure. Tracing changes in whale song will help to undercover the drivers underlying this vocal display and contribute to the understanding of animal culture and its evolution. To determine the progressive changes in songs found on a subarctic feeding ground and migratory stopover, a detailed analysis of humpback whale song recordings from Northern Norway was conducted. Passive acoustic data from the Lofoten-Vesterålen Ocean Observatory (LoVe), collected using a bottom-moored underwater hydrophone, were used from January - April 2018 and January 2019. Two measures of the song structure were examined: (1) sequence similarities using the Levenshtein distance and (2) song complexity using a principal component analysis (PCA). In total, 21 distinct themes were identified which presented highly directional, structural changes over time. Two themes from 2018 reoccurred in 2019, whereas all other themes in 2019 appeared to be evolved versions of 2018 themes. All songs grouped into three general clusters, reflecting the rapid evolution over the study period. With all sampled animals singing the same version of the song, this might indicate that the singers are either from the same breeding population or that song learning occurred before the study period. Song complexity appeared to follow the trend of song progression; songs became more complex as they evolved over the months in 2018 and decreased in complexity between the years, returning to a more simplified song in 2019. The results confirm that humpback whale song exhibits a rapid progression on a shared subarctic feeding ground, with strong potential for song exchange and opportunities for cultural transmission between populations in the North Atlantic.
Introduction
A broad range of animals communicate by means of vocal sound production and in various species males are known to perform specific vocalizations during mating rituals, such as singing in many avian species (Kroodsma, 2004). Generally, animal song is characterized by a sequence of patterned vocal sounds that are repeated in succession (Broughton, 1963; Payne and McVay, 1971). Singing behavior and the production of complex songs is rare among mammals and has been only documented for a few mammalian species (Stafford et al., 2018). This vocal display can be found in cetaceans but appears to be restricted to baleen whales and ranges in complexity from a few repetitive sound types produced by fin whales (Balaenoptera physalus; Delarue et al., 2009) and blue whales (Balaenoptera musculus; Stafford et al., 2001) to more complex songs of bowhead (Balaena mysticetus; Stafford et al., 2018) and humpback whales (Megaptera novaeangliae; Payne and McVay, 1971). Humpback whales exhibit a rich and complex acoustic display that is typically divided into two categories: ‘singing’ (Payne and McVay, 1971) and ‘social sounds’ or ‘non-song’ (Payne, 1978; Silber, 1986). In contrast to the social sounds, songs of humpback whales are rhythmic with a highly repetitive pattern (Payne and McVay, 1971) and to date, only males have been observed to sing (Payne and McVay, 1971; Cerchio et al., 2001; Cholewiak, 2008; Smith et al., 2008). Singing behavior by male humpback whales is considered to play a role in sexual selection, although its specific function as a signal is still debated (Herman, 2016). Several behavioral studies support an inter-and intrasexual purpose such as to attract a mate and/or facilitate male to male interactions (e.g. Darling and Bérubé, 2001; Darling et al., 2006; Cholewiak, 2008; Smith et al., 2008), indicating that song may be a multi-message display (Murray et al., 2018). Additionally, variation in sound types within the song has been suggested to have a communicative function in terms of conveying information about individual identity (Hafner et al., 1979) or it may reveal a singer’s reproductive fitness to other whales (e.g. Chu, 1988; Payne, 2000; Parsons et al., 2008; Herman, 2016).
The humpback whale song has an intricate structure due to its nested hierarchy with multiple levels of acoustically distinct ‘units’ arranged into ‘phrases’ which form a ‘theme’ (Payne and McVay, 1971; Cholewiak et al., 2013). It is a well-studied vocal behavior that is passed among individuals by horizontal cultural transmission (Guinee et al., 1983; Payne and Guinee, 1983; Payne and Payne, 1985; Noad et al., 2000; Cerchio et al., 2001; Garland et al., 2011; Garland and McGregor, 2020). Populations within the same ocean basin display a high degree of song sharing (Payne and Guinee, 1983; Helweg et al., 1990; Helweg et al., 1998; Cerchio et al., 2001). For example, the song of Pacific humpback whales has been documented to be transmitted horizontally in an eastward manner from eastern Australia to French Polynesia (Garland et al., 2011; Garland et al., 2013b; Schulze et al., 2022). Although all males within a population typically conform to the same version of the song at any given time with some inter-individual variation, the song undergoes progressive change within and between years (Payne and McVay, 1971; Frumhoff, 1983; Payne et al., 1983; Payne and Payne, 1985), a process referred to as ‘cultural evolution’ (Winn and Winn, 1978; Payne et al., 1983; Payne and Payne, 1985; Cato, 1991; Noad et al., 2000). By modifying spectral and temporal features of song elements, indicative of production learning (Janik and Slater, 2000), individual whales create a gradually evolving song structure that all males within a population maintain by incorporating changes as they occur (Payne and McVay, 1971; Guinee et al., 1983; Payne et al., 1983). When an existing song is rapidly and completely replaced by a novel version (i.e. song types that occur consecutively or overlap in time share 0% similarity), is a process described as ‘song revolution’ (Noad et al., 2000; Garland et al., 2011; Garland et al., 2013b; Allen et al., 2018). Recent work by Allen et al. (2018) and Garland et al. (2021) has revealed a pattern in which humpback songs increased in complexity as they evolved through progressive changes but became more simplified following events of song revolution.
Song transfer between male humpback whales is hypothesized to occur when individuals are in acoustic contact; one of the possible mechanisms might be when different breeding populations geographically overlap on a shared feeding ground (Payne and Guinee, 1983; Garland et al., 2013a; Allen et al., 2022; Schulze et al., 2022). Humpback whales typically undertake extensive annual migrations (ca. 10,000 km) between high-latitude feeding grounds and low-latitude breeding areas (Dawbin, 1966), representing a cultural tradition of this species (Baker et al., 1990). Traditional feeding grounds in the north-east Atlantic stretch from subarctic waters off Iceland, Jan Mayen, Greenland, and Northern Norway to the Barents Sea (Stevick et al., 2006). Telemetry and photo-ID studies have demonstrated that Norwegian fjords represent an important stopover in the southbound migration for humpback whales (Ramm, 2020; Kettemer et al., 2022). This migration route and feeding ground off Norway’s coast has shown to be shared with an interchange of different humpback breeding populations from Cape Verde (Africa) and the West Indies (America; Bérubé et al., 2004; Stevick et al., 2016; Stevick et al., 2018; Whaletrack UiT, 2018; Wenzel et al., 2020). Given the identified humpback whale song occurrence in Northern Norway as described in Tyarks et al. (2021), this might indicate a potential for acoustic interaction between the two breeding populations. Therefore, the aim of this study was to investigate the song structure and complexity and to find evidence of humpback whale song evolution on this subarctic feeding ground and migratory passage route. This work will give an important contribution to our understanding of the features of the humpback whale song in Norwegian waters and will help elucidate the importance of feeding grounds for song exchange at high latitudes within the North Atlantic. Furthermore, quantifying song changes and complexity in humpback whales can provide essential insights into song dynamics and aid our understanding of the vocal and social learning capacity in this species.
Methods
Song recordings and transcription
Humpback whale songs were recorded by the Lofoten-Vesterålen (LoVe) Ocean Observatory, a cabled network located in an ecological hotspot forming a westward transect over a shelf-slope area (Figure 1). Node 1 of this network (N68°54’, E14°23’, 257 m depth, 20 km offshore) includes an instrument platform which holds a hydrophone (Ocean Sonics SB35 ETH, maximum frequency range 10 Hz-200 kHz; for this study, the sampling rate was set to 32 kHz for 2018 files and 64 kHz for 2019 files) that continuously records biologically generated sounds and underwater noise within the area (Godø et al., 2014). The present study is based on the analysis of passive acoustic data collected from Node 1 between January 2018 and January 2019. Audio files were retrieved using the LoVe Ocean archive (Equinor and IMR, 2020), where continuous recordings are stored as adjacent 10-minute files with occasional gaps due to technical maintenance. All recordings containing humpback whale song previously identified by Tyarks et al. (2021) were manually examined using spectrographic views in Raven Pro 1.6 (Yang and Center for Conservation Bioacoustics, 2019; FFT size 8092, Hann-window and a 70% overlap). Recordings were rated high or low quality, based on the signal-to-noise ratio (SNR) with only high-quality songs (SNR approximately 10 dB above background noise) included in the analysis. Whenever an overall high-quality song file showed parts of lower quality within a song, Audacity’s Noise Reduction (version 2.4.2; 12dB, sensitivity 6, frequency smoothing bands 3) was used. This procedure is a sample-based filtering process, by first creating a noise profile (i.e. a clip containing only noise close to the sound) and then selecting the audio that is supposed to be filtered. The filter reduces noise signatures that are masking the signal’s acoustic characteristics and is a useful tool to prepare vocal signals for analyses (Baker and Logue, 2007).
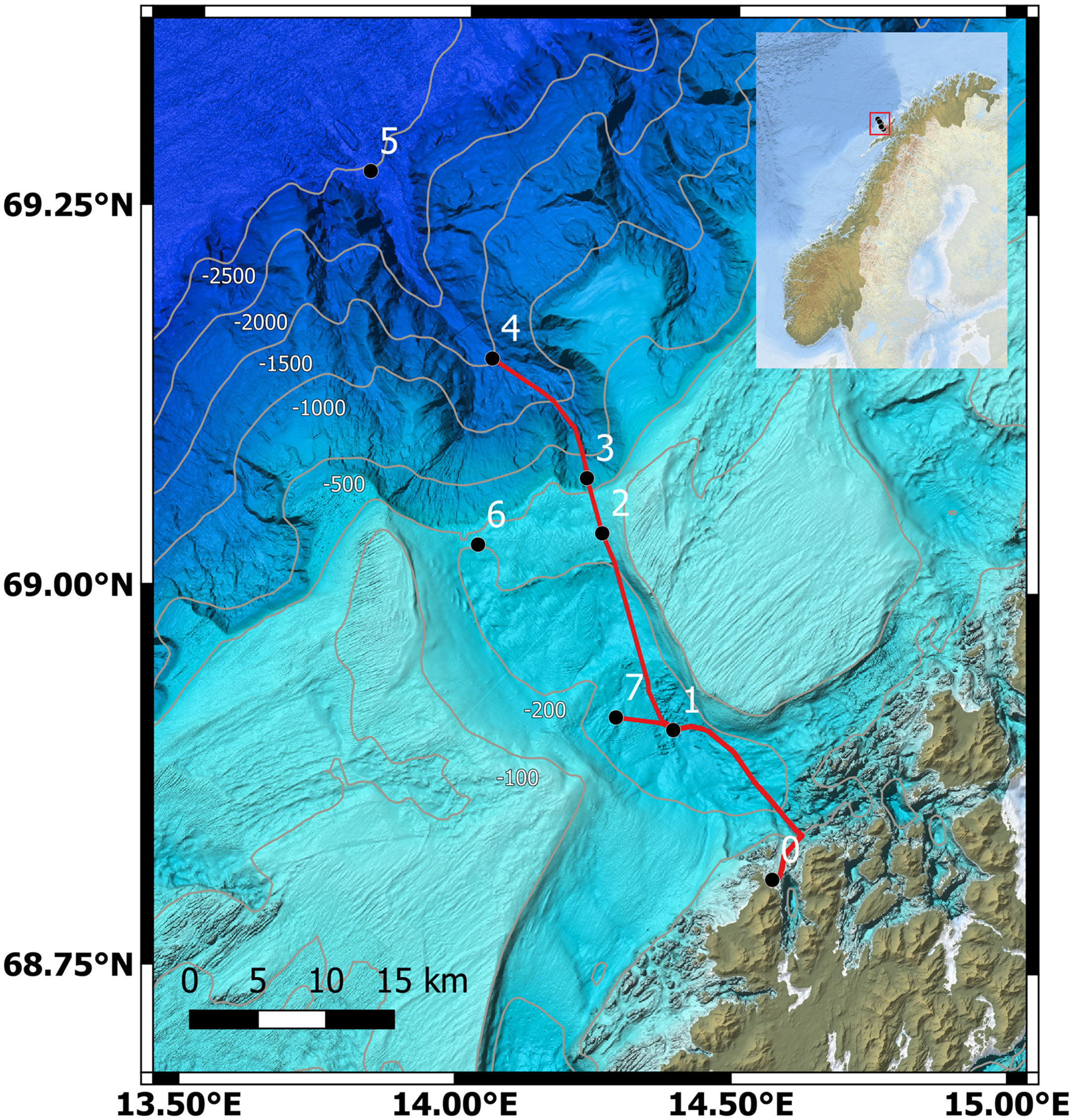
Figure 1 Map of the sampling-nodes of the LoVe Ocean Observatory, Norway. Data used in this study were collected by Node 1 (Equinor and IMR, 2020). Produced by Geir Pedersen and reprinted with permission from Tyarks et al., 2021 (Copyright 2021, Frontiers in Marine Science).
To allow for comparability across a temporal scale, a consistent number of songs per month with available data were transcribed. A total of 15 high-quality song sessions (see song element definitions below) with three song cycles each were selected for song analysis, resulting in 45 song cycles between January-April 2018 and January 2019. A summary of all acoustic files used in the analyses can be found in Table 1. The song files were then transcribed for sequences of song elements (Figure 2), following the hierarchical structure first described by Payne and McVay (1971). Every visually and aurally distinguishable sound (i.e. units) was transcribed for all songs included in the analysis. Units were labelled based on their acoustic characteristics including the slope and duration (e.g. short ascending moan, abbreviated ‘sam’) as in previous studies (Dunlop et al., 2007; Garland et al., 2017). A sequence of multiple units formed a ‘phrase’ and similar phrases repeated in succession were then assigned to a ‘theme’. A combination of distinct themes joined in a predictable sequence then makes up a ‘song’, which is then again repeated multiple times as ‘song cycles’ to form a ‘song session’ (Payne and McVay, 1971; Cholewiak et al., 2013).
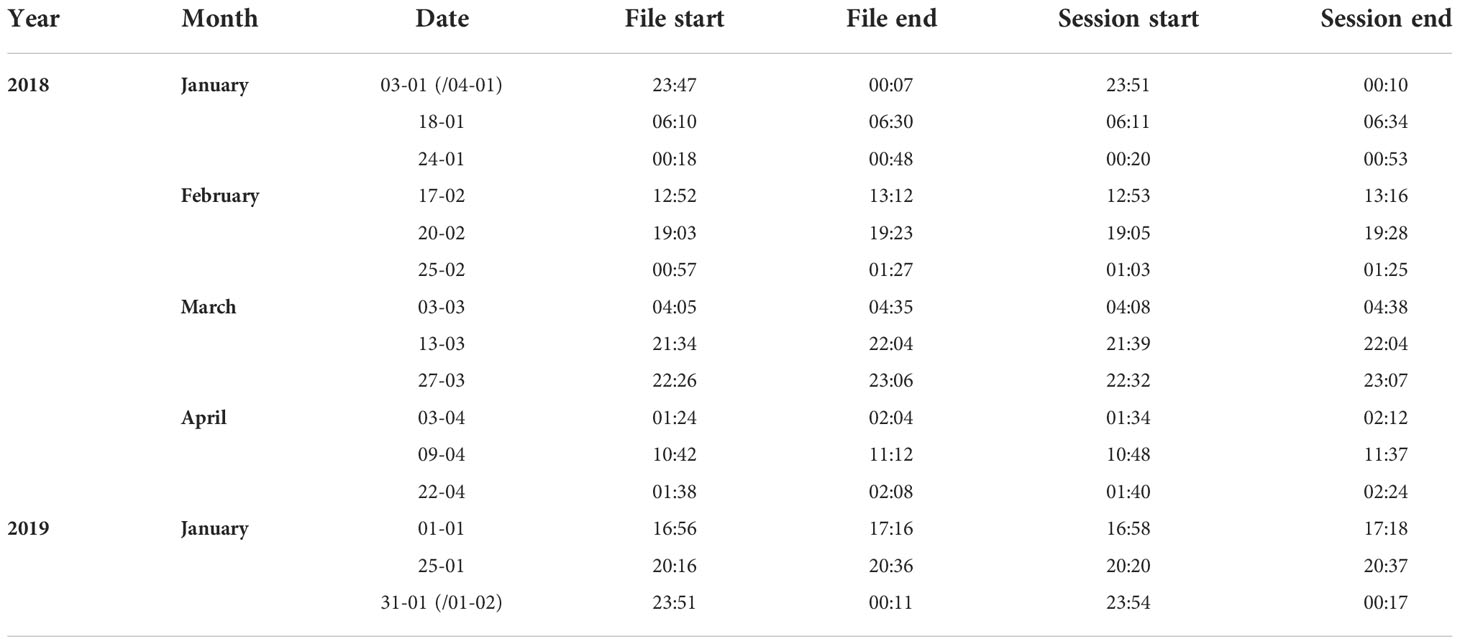
Table 1 Summary of all acoustic files used in song sequence analyses. File start and end refers to the recordings retrieved from the LoVe Ocean archive (Equinor and IMR, 2020).
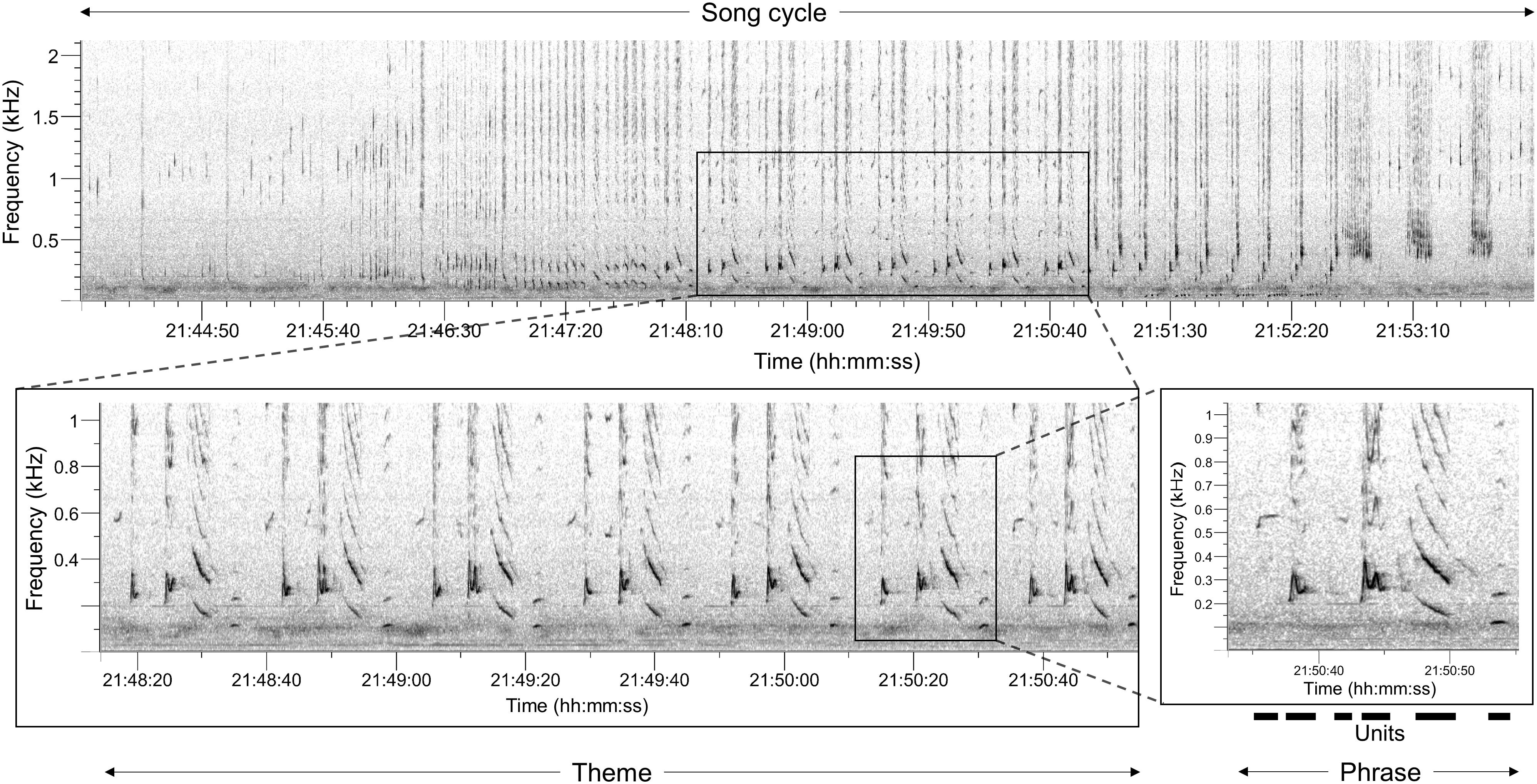
Figure 2 Spectrograms illustrating the humpback whale song and its hierarchically structured elements (units, phrase, theme, song cycle), recorded by LoVe Ocean Observatory in March 2018. The spectrograms were produced using fast Fourier transform (FFT) size 8092 Hann-window with a frequency resolution of 5.69 Hz and a 70% overlap. The illustrated full song cycle consists of several themes. The theme example of the song cycle is composed of several phrase repeats. One phrase is composed of a repeating pattern of individual units.
The delineation of phrases, and hence themes, can be subjective, as emphasized by several studies (e.g. Cholewiak et al., 2013; Mercado, 2021). In this study, the guidelines for delineating and measuring phrases suggested by Cholewiak et al. (2013) were adopted. Successive phrases within a theme are “inexact replicas” (Payne and McVay, 1971) and may change in the number of units, as well as spectral and/or temporal characteristics of units. Therefore, a small amount of variation was permitted in (sub-) units used within phrases. Phrases were considered as part of the same theme when acoustically similar units (e.g. ‘short ascending moan’ and ‘moan’) occurred in the same position in the phrase (Helweg et al., 1998; Garland et al., 2012). On some occasions, so-called ‘transitional phrases’ were sung between themes, which are combinations of units or subphrases of the two adjacent themes (Payne and Payne, 1985). This could either be combined subphrases or units combining characteristics of some units in the two adjacent themes (Frumhoff, 1983; Cholewiak et al., 2013). In the present study, transitional phrases were delineated as such and excluded from the analysis. Themes and associated phrases found during song transcription were labelled with numbers and new versions included letters (e.g. 2A) as used in previous humpback whale song studies (Garland et al., 2013b; Garland et al., 2015; Garland et al., 2017; Owen et al., 2019). A theme that shows progressive changes over multiple phrase repetitions is called a shifting theme (Payne and Payne, 1985; Cholewiak et al., 2013) and was labelled using letters (e.g. phrase type 3-a shifted to 3-ab which shifted to 3-b).
With song sessions usually sung in a continuous bout (Winn and Winn, 1978) with arbitrary choice of a starting theme (Cholewiak et al., 2013), determining the start and end of song cycles can be difficult. Following the definition by Frumhoff (1983), a song is a theme sequence that consists of not less than three themes which are repeated in the same order at least twice. In the present study, a song was defined as a complete cycle of themes with no repetition of a theme (Payne and Payne, 1985). The order of themes throughout all song sessions included in the analyses were invariant, meaning that e.g. theme 1 was always followed by theme 2. And although some themes were dropped in some song cycles, the starting theme in the example above consistently appears to be theme 1. Thus, a song cycle in the present study was not delineated based on a complete rendition of all themes but by the overall theme order.
Validation of unit classification consistency
To validate the consistency of the qualitative unit classification, a random forest analysis was carried out (Warren et al., 2020). Random forest is a machine learning algorithm that consists of an ensemble of decision trees from a randomly selected subset of data and an effective tool in prediction (Breiman, 2001). This quantitative test assesses the manual classification of humpback whale units as outlined by Allen et al. (2017) and Garland et al. (2017). To provide a representative subsample of units within song sessions, all units of every first full phrase of each theme were measured for various acoustic parameters following Dunlop et al. (2007) and Garland et al. (2017). For shifting themes, the first phrase of each phrase type was measured. Additionally, units that solely occurred in transitional phrases or only once within the entire dataset were also measured at least once. Using Raven Pro (version 1.6; Yang and Center for Conservation Bioacoustics, 2019; FFT size of 8092, Hann-window frequency resolution of 5.69 Hz for 2018 files and 11.4 Hz for 2019 files, and a 70% overlap), the following parameters were calculated for the fundamental frequency per unit: duration, high and low frequency, bandwidth, frequency range, start and end frequency, peak frequency, and number of inflections (see Supplementary Table 1 for parameter descriptions). All statistical analyses were carried out in R (version 4.0.4; R Core Team, 2021). The random forest analysis was conducted using the ‘randomForest’ function (Liaw and Wiener, 2002). The assigned unit name was used as the dependent variable (mtry=8, ntrees=2000) and the random forest model then classified each unit based on the measured parameters. This resulted in a confusion matrix, representing the number of times where the predicted label matches the assigned label, i.e. when a unit was classified correctly or was mislabeled. The model also outputs an out-of-bag (OOB) error rate, measuring the prediction error, and indicating the overall level of agreement between the classifications.
Song and theme similarity using the Levenshtein distance
The stereotyped structure of humpback whale song with its nested hierarchy allows for a quantitative measure using common sequence analysis metrics. The Levenshtein distance (LD) is a robust and powerful edit distance technique that provides information of similarities between vocal sequences (Levenshtein, 1966). This method has previously been applied to investigate humpback whale song evolution though time and/or among populations (e.g. Garland et al., 2012). Following the definition by Kohonen (1985), the simple LD calculates the minimum number of changes (substitutions, insertions, or deletions) needed in order to convert one string into another. To ensure direct comparability of sequences, the LD score needed to be standardized to account for the length of the strings, resulting in the Levenshtein Distance Similarity Index (LSI) at a set level (Helweg et al., 1998; Eriksen et al., 2005; Tougaard and Eriksen, 2006; Garland et al., 2012; Garland et al., 2013b; Garland et al., 2015). To evaluate song sequence progression throughout the study period, a representative string, also called a set median (SM), was calculated (Kohonen, 1985; Helweg et al., 1998; Tougaard and Eriksen, 2006; Garland et al., 2013b);. Using SMs ensured that the typical string of song elements sung for each sequence was included.
Comparisons of sequences were carried out on two levels of song hierarchy, with similarities analyzed between (1) songs, where the string was a sequence of themes, and (2) themes, where the string was comprised of units (phrase). Song-level analysis enables the identification of different song types within the same area and between periods, whereas fine-scale similarities within and between each song can only be detected using the theme-level, represented by different phrase types. Phrase-based analyses have been described to be most appropriate within humpback whale song, with the duration of phrases being one of the most stable features within the hierarchical structure of the song (Frumhoff, 1983; Payne et al., 1983; Cerchio et al., 2001; Cholewiak et al., 2013). Therefore, themes were represented by a SM which was made up by one phrase and not the entire sequence of all phrase repetitions within a theme (Payne and McVay, 1971). The LSI was also used to assess the qualitative assignments of phrases to a theme and calculated between every phrase (i.e. sequence of units) across the entire dataset, regardless of recording period. Comparisons of songs were carried out by condensing the data using set medians per song session to evaluate and display song progression over time. In this study, the weighted LSI analysis (ß=1) was implemented to provide a robust representation of song similarity (Allen et al., 2017; Garland et al., 2017; Allen et al., 2018). To create weighting costs (i.e. penalties) for the theme-level analysis, the similarity between unit types were based on the distance among them. For this, the same acoustic measurements as for the random forest analysis were applied based on Garland et al. (2017). For the upper song-level analysis, the LSI dissimilarity matrix between themes, regardless of different phrase types of transitional themes, was used as penalty scores to trace evolving themes (Garland et al., 2017).
All distance analyses were calculated using the R package ‘leven’ (a custom written code, available at http://github.com/ellengarland/leven, by Garland and Lilley (2020)). To visualize connections among sequences, the results of all LSI similarity matrices were hierarchically clustered using the functions ‘hclust’, ‘pvclust’ and ‘pvrect’ (Suzuki and Shimodaira, 2006). The appropriate clustering method for the data was determined by comparing the cophenetic correlation coefficient (CCC) between dendrograms with average and single linkage (value >0.8 is considered a good representation; Sokal and Rohlf, 1962). An unweighted LSI analysis was also run which produced similar results, however, the weighted analysis yielded a slightly higher CCC value on the theme-level (CCC=0.89 compared to CCC=0.86 for the unweighted LSI analysis) and the same CCC value (=0.93) on the song-level, confirming the inclusion of weighting. To assess the stability of the resulting clusters from the similarity matrix, each matrix was also bootstrapped using multi-scale resampling which generated approximately unbiased (AU) values and normal bootstrap probabilities (BP; Garland et al., 2017; Rekdahl et al., 2018). Tree structures were considered stable and strongly supported by the data with AU >0.95 and BP >0.70 (Suzuki and Shimodaira, 2006; Garland et al., 2012), whereas lower values indicate variability in their division. Applying the methods described above allows to evaluate the song progression on both upper (song) and lower (theme) levels within the nested humpback whale song structure.
Song complexity
The complexity of all transcribed songs was assessed using scores generated on the song-level where a sequence of all units per song was used. This type of analysis has previously been performed by Allen et al. (2018) and Garland et al. (2021), based on Boogert et al. (2008) and Templeton et al. (2014). The content of song complexity comprised the entire sequence of units, irrespective of phrases and themes, where three variables were used per song session: average number of units, average number of unit types, and the average duration of the three song cycles (in seconds). To represent the data of the multiple dimensions within the song, a principal component analysis (PCA) was computed using the function ‘princomp’ including the three variables for song-level. A PCA allows to compress multidimensional data onto lower dimensions and thereby making interpretation easier, while preserving as much information as possible (Jolliffe, 2002). The first principal component scores (PC1) were used as the measure of song complexity, representing relative rather than absolute values that indicate whether a song is simple or complex in relation to another. Factor loadings were investigated to determine correlations between each variable and the respective principal component. To allow the PC1 scores to represent a measure of complexity, all variables must follow the same direction (i.e. all positive or all negative). For the analysis of song complexity, all transcribed songs were used to capture the variability within as well as between singers.
Results
Theme structure and similarity
Songs recorded on the subarctic feeding ground in Norway displayed the typical hierarchical structure of different themes composed of phrase repetitions reported for humpback whales. The transcription of the 45 high-quality song recordings resulted in a total of 73 distinct unit types. The random forest analysis using all identified units measured across the dataset (n=1501) resulted in an OOB error rate of 13.26%. Consequently, 86.74% of all unit types were classified in the same way as by the human classifier. This high level of agreement confirms that the labelling of units was robust and repeatable across the dataset.
During song transcription, a total of 1244 phrases were identified, with the shortest and longest phrases comprised of 2 and 46 units, respectively. Transitional phrases were found on 135 occasions, making up 11% of all phrases. All transcribed sequences, excluding transitional phrases (n=1109), were assigned to 27 different phrase types, which represent 21 distinct themes for the defined study period (Figure 3). Five themes (3, 3A, 5, 5A and 7) were identified as shifting themes with phrases changing with each repetition (Figure 3). Phrases of themes 5 and 5A were assigned to the same phrase type despite the increase in repetition of the last unit within one phrase. On the other hand, themes 3, 3A and 7 presented units evolving from one form to another with each successive phrase, resulting in three phrase types (a, ab, b). Within-set comparisons of all phrases assigned to the same phrase type regardless of recording period showed high similarities (≥46%), verifying assignments of phrases to phrase types. A summary of all identified phrase types and themes, their set medians, and within-set similarities can be found in Supplementary Table 2. Songs between January and April of 2018 resulted in 17 distinct themes, whereas seven themes were identified in songs of January 2019 (Table 2). Four themes (5B, 6A, 6B and 7) were only observed in one song session each and, therefore, termed rare or uncommon themes.
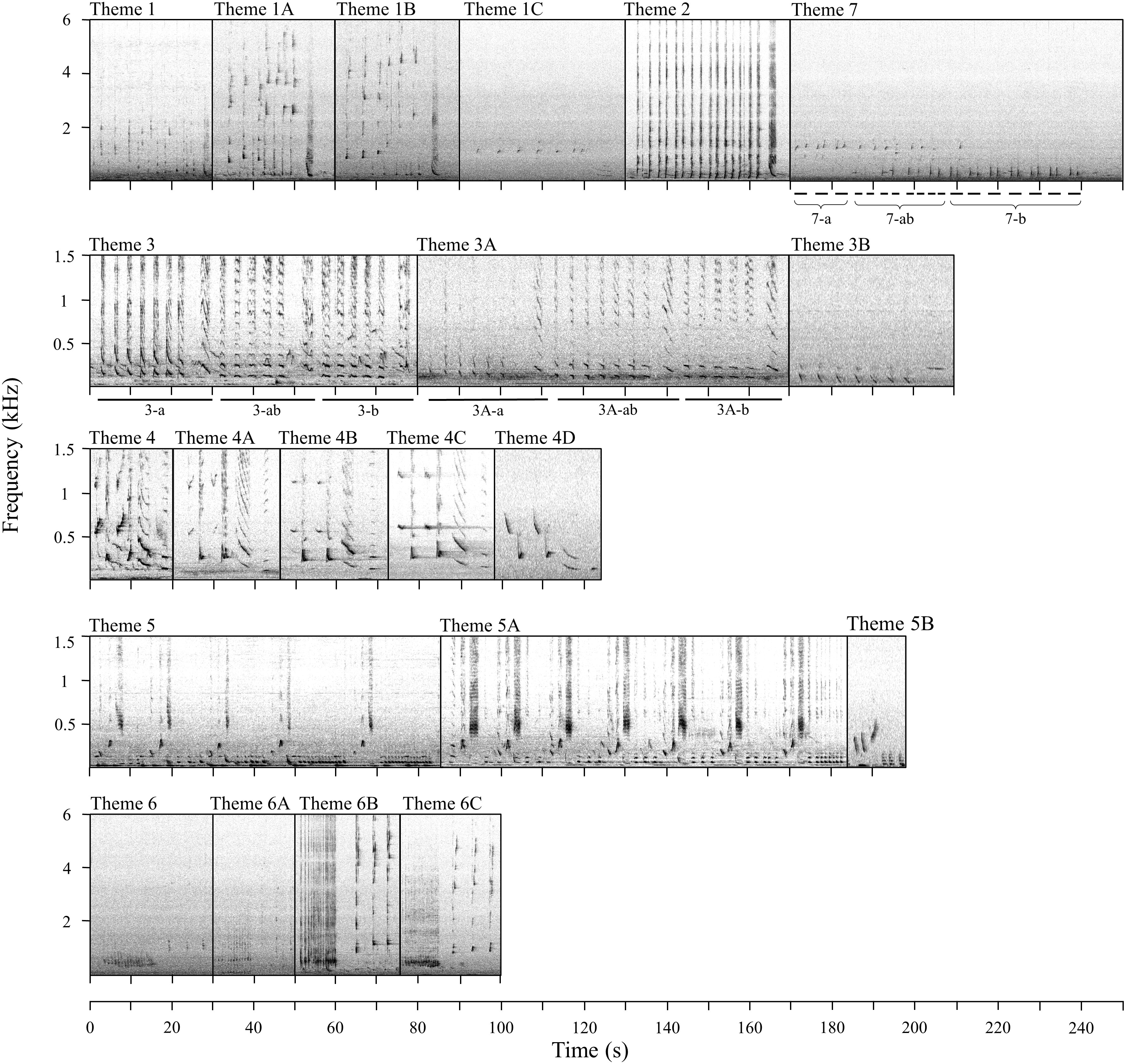
Figure 3 Spectrographic representation of all observed themes and phrase types from the recording period January 2018 - January 2019. The spectrograms were produced using fast Fourier transform (FFT) size 8092 Hann-window with frequency resolutions of 5.69 Hz (for 2018 files) and 11.4 Hz (for 2019 files), and a 70% overlap. Corresponding audio files are provided in Supplementary Audios 1-11. Note that themes are not ordered according to how they occurred in songs but rather follow the evolutionary changes.
Most themes were evolving rapidly as time progressed; theme 1, 3, 4, 5, and 6 presented gradual changes over several months within and between years (Figure 3). New variants of these themes evolved by unit modifications (e.g. changes in slope, frequency and/or duration) or insertions of additional units within a phrase. Over time, the fine-scale structural features of the new variants became consistently different to their origin, and thus, were classified as new themes (e.g. theme 4 to 4a etc.; see Figure 3). The LSI showed that the original themes and their evolved versions overlap in similarities (LSI matrix can be found in Supplementary Table 3) and form stable clusters and/or close together on a higher branch (Figure 4). The CCC for the average-linkage dendrogram of SM-phrases to themes comparison was 0.89, indicating a good representation of the structure within the data despite some branches not reaching AU or BP significance. Two themes (1C and 2), usually adjacent within the order of themes if both were present in a song, appeared to have merged by the end of January 2019 (session 19_3), forming a new theme, numbered 7 (Figure 3). However, the phrase types of these themes do not form a stable cluster (Figure 4). This was possibly due to the shifting structure of theme 7, with subtle modifications between phrase repetitions (7a, 7ab, 7b; Figure 3). The previous themes 1C and 2 consisted of one long phrase each, whereas theme 7 presented phrases similar to theme 1C (7-a) shifting to theme 2 (7-b), connected by a mix of phrase type 7-ab. This connection between the themes was also confirmed by the LSI which revealed 22% similarity between phrase types 1C and 7-a, and 16% between 2 and 7-b (Supplementary Table 3). Other themes were also classified as shifting themes; phrases of theme 3 and its evolved version 3A showed high similarities across the slightly modified phrase types (e.g. 3-a to 3-ab 43%; Supplementary Table 3). The different phrase types grouped into several clusters, however, a clear separation of -a and -b versions is apparent on a higher branch (Figure 4). Although identified as a shifting theme, phrase repetitions of theme 5 and 5A were not split into different phrase types. For these themes, the shift was characterized by an increase in repetition of the last unit with each successive phrase (Figure 4). Despite these differences between phrases of the same theme, within-set similarities were high (theme 5 = 57%, theme 5A=74%; Supplementary Table 2). Theme 5 evolved to theme 5A over the course of January to April 2018 and according to the LSI, these themes are 63% similar to another (Supplementary Table 3) and form a stable cluster (Figure 4). In the following year, theme 5A has evolved further to theme 5B and shifts have been replaced by a relatively stable phrase type. Consequently, theme 5B does not cluster together with its previous versions (i.e. themes 5 and 5A), however, the LSI resulted in 27% similarity between themes 5A and 5B (Supplementary Table 3), showing their connection.
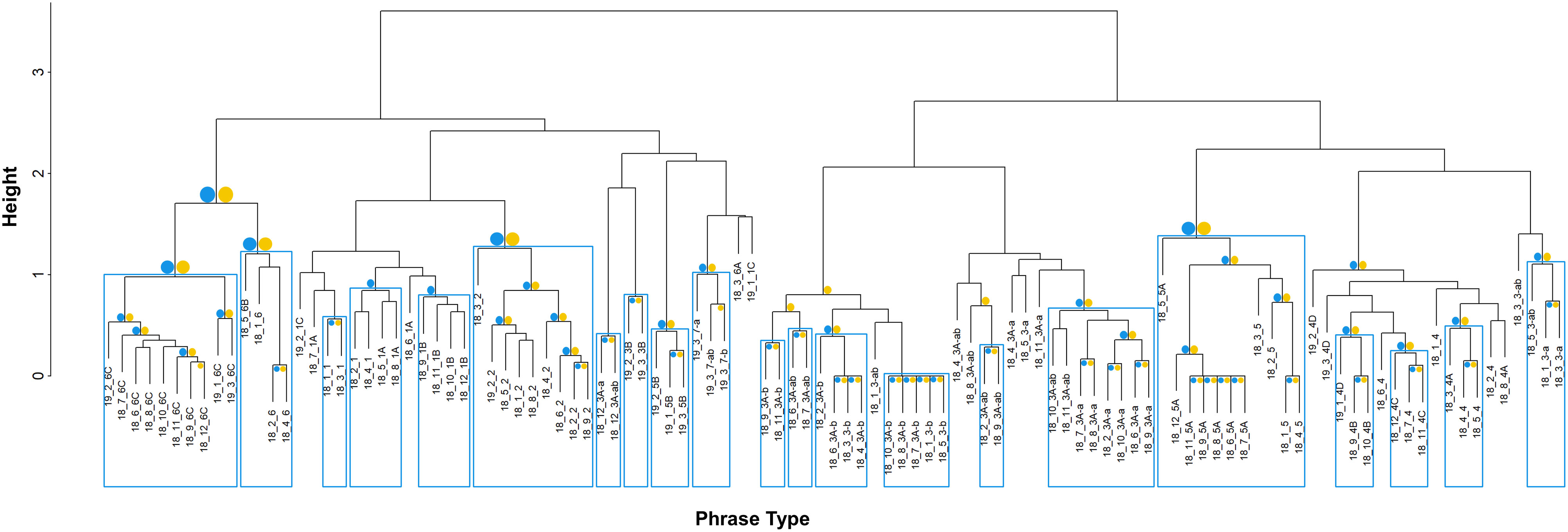
Figure 4 Dendrogram of bootstrapped (1000) LSI of average-linkage hierarchical clustered set medians per theme (most representative phrase) per song session. The Cophenetic Correlation Coefficient (CCC)=0.89, indicating a good representation of the structure within the data. Multiscale bootstrap resampling is represented by dots: blue (left) indicates AU > 95% and yellow (right) indicates normal BP > 70%. Lower values (no dots) indicate variability in their division whereas branches with high AU values represent stable divisions. Stable and highly supported clusters were marked using rectangles at the highest level.
Song similarity and complexity
Comparisons on the song-level (sequence of themes) within the months of 2018 and between the years 2018 and 2019 presented a gradual change in the content and composition of themes as well as song length (see Supplementary Figure 1 for spectrographic examples). Overall, the data showed some variation in the number of themes composing a song, ranging between 4-6 themes and with a mean of 5.31 (SD=0.72) themes per song cycle. The average number of themes in song cycles of 2018 was 5.44 (SD=0.64), whereas songs in January 2019 appeared shorter, presenting a decrease in number of themes, with a mean of 4.78 (SD=0.79),. All transcribed songs of all sessions in both years seem to conform to the respective order and patterns of themes. Designated starting themes in 2018 was predominantly theme 1 and its evolved versions 1A and 1B (35/36 song cycles=97%), and songs exclusively terminated with theme 6 and its evolved versions 6A, 6B and 6C (Table 3). Songs in January 2019 mostly started with theme 1C (6/9 = 67%), whereas the three song cycles of the last measured song session started with the new theme 7, the evolved version of themes 1C and 2. Terminal themes in 2019 were exclusively assigned to theme 6C in all songs, as observed in songs of 2018. The sequence of themes appeared to follow a predictable order in all months of both years with some themes being dropped occasionally (Table 3). Based on the changes on the theme level, the LSI on the song level revealed a high degree of similarities across songs of 2018 and 2019 (Supplementary Table 4). The hierarchically clustered and bootstrapped theme sequences produced two major branches and four stable clusters, belonging to three major groups (Figure 5, CCC=0.93). Within the cluster of 2018 songs, the branches split into two groups, distinguishing between songs of January to mid-February (sessions 18_1 - 18_5) and end of February to end of April 2018 (18_6 - 18_12), whereas songs of January 2019 formed one stable cluster. The LSI using the set median song of each session revealed that songs were highly similar (>52%) within each month but became progressively less similar throughout the study period (Supplementary Table 4). Within the four months of January to April in 2018, songs showed a decrease in similarity; comparing the very first and last song sessions measured in 2018 (18_1 in January and 18_12 in April), the similarity between the songs has dropped down to 38%. Other sessions of the same months showed even less similarity between songs, with only 26% between 18_3 and 18_12. By the following year of 2019, song similarities have decreased even further; songs of January 2019 (19_1 and 19_3) shared only 17% similarity with the song sung in January 2018 (18_3).
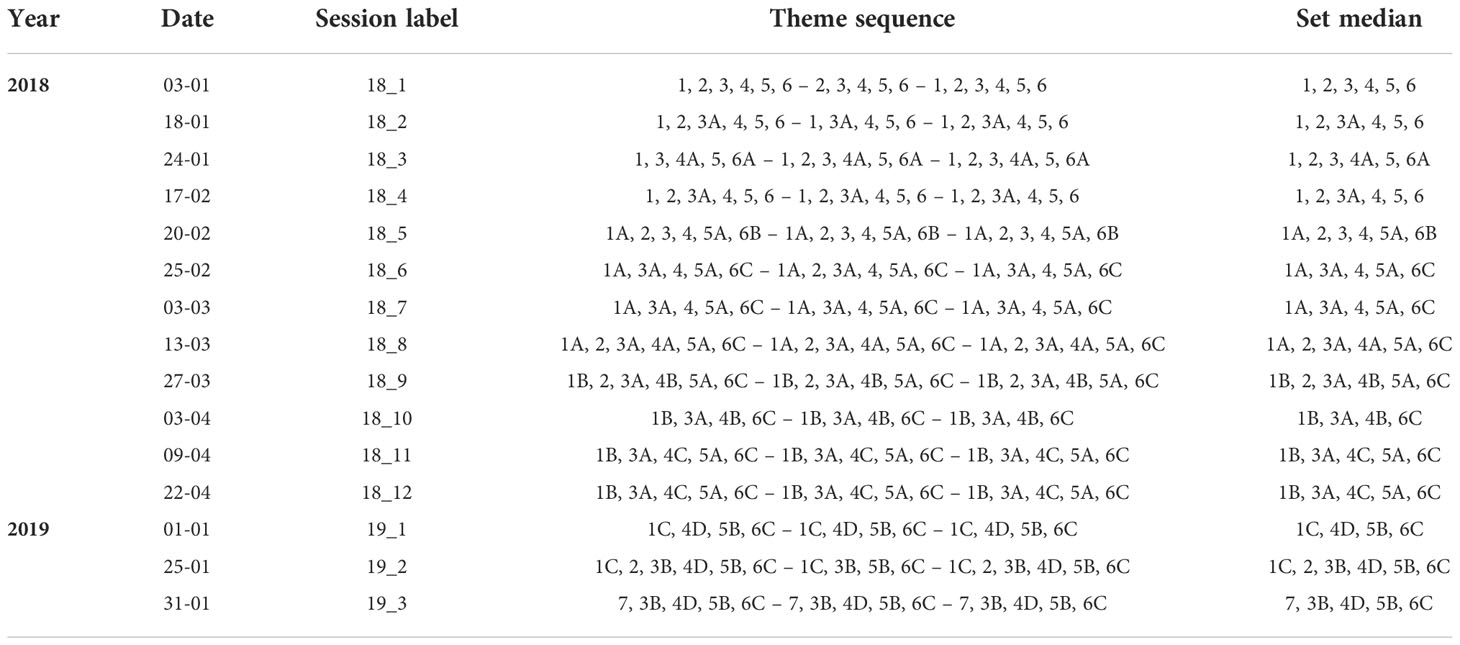
Table 3 Transcribed theme sequences of all sessions throughout the study period January 2018 - January 2019. Hyphen (–) represent the split between song cycles.
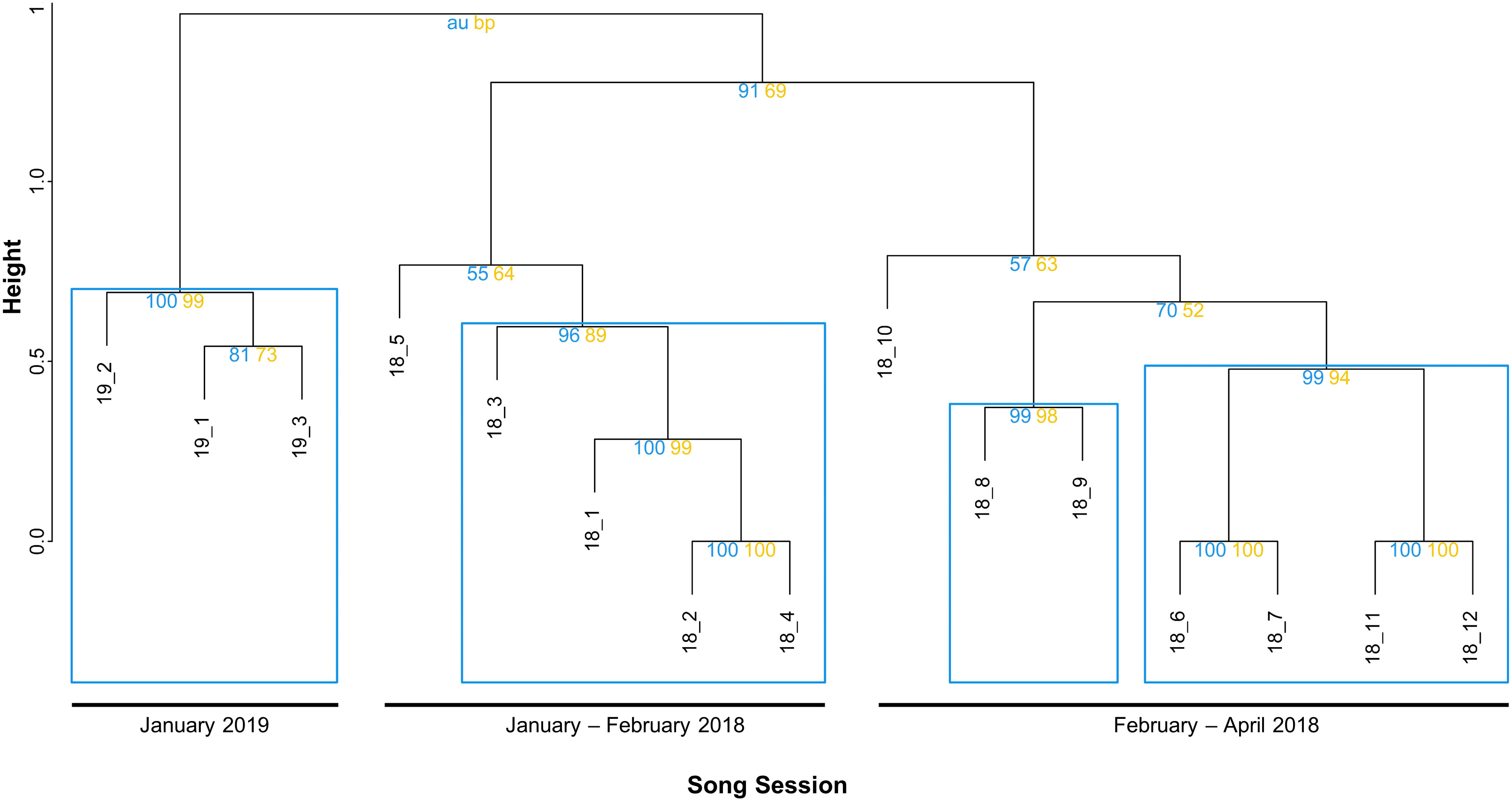
Figure 5 Dendrogram of bootstrapped (1000) LSI of average-linkage hierarchical clustered set medians per song cycle (sequence of themes). The Cophenetic Correlation Coefficient (CCC)=0.93, indicating a good representation of the structure within the data. Numbers represent multiscale bootstrap resampling (AU, blue left) and normal bootstrap probability (BP, yellow right) which is considered significant if AU p>95% and BP p>70%. Lower values indicate variability in their division whereas branches with high AU values represent stable divisions. Stable and highly supported clusters were marked using dashed rectangles at the highest level.
The results of the complexity analysis also displayed temporal changes in song content and composition; songs presented an overall increase in complexity as they evolved over the months in 2018 and decreased drastically between April 2018 and January 2019 (Figure 6). Songs between January and April 2018 increased in length as time progressed, containing a higher number of units, unique unit types, and were longer in duration. The shortest song cycle contained 102 units found in January 2018 whereas the longest was composed of 534 units in April 2018. By the following year, songs were considerably shorter, resulting in a decrease of complexity in January 2019 compared to April 2018. Yet, the complexity of the sessions in January 2019 appeared to be within in the same range as the complexity in January of the previous year. The PC1 score of the component 1 in the PCA resulted in a cumulative proportion of 0.77, indicating that a large amount of the variance is explained by PC1 that was used to represent the song complexity. The three variables included in the PCA showed to contribute unevenly with the number of units having the highest loading score (=0.64), followed the song duration (=0.60) and unique unit types (=0.48) per song cycle.
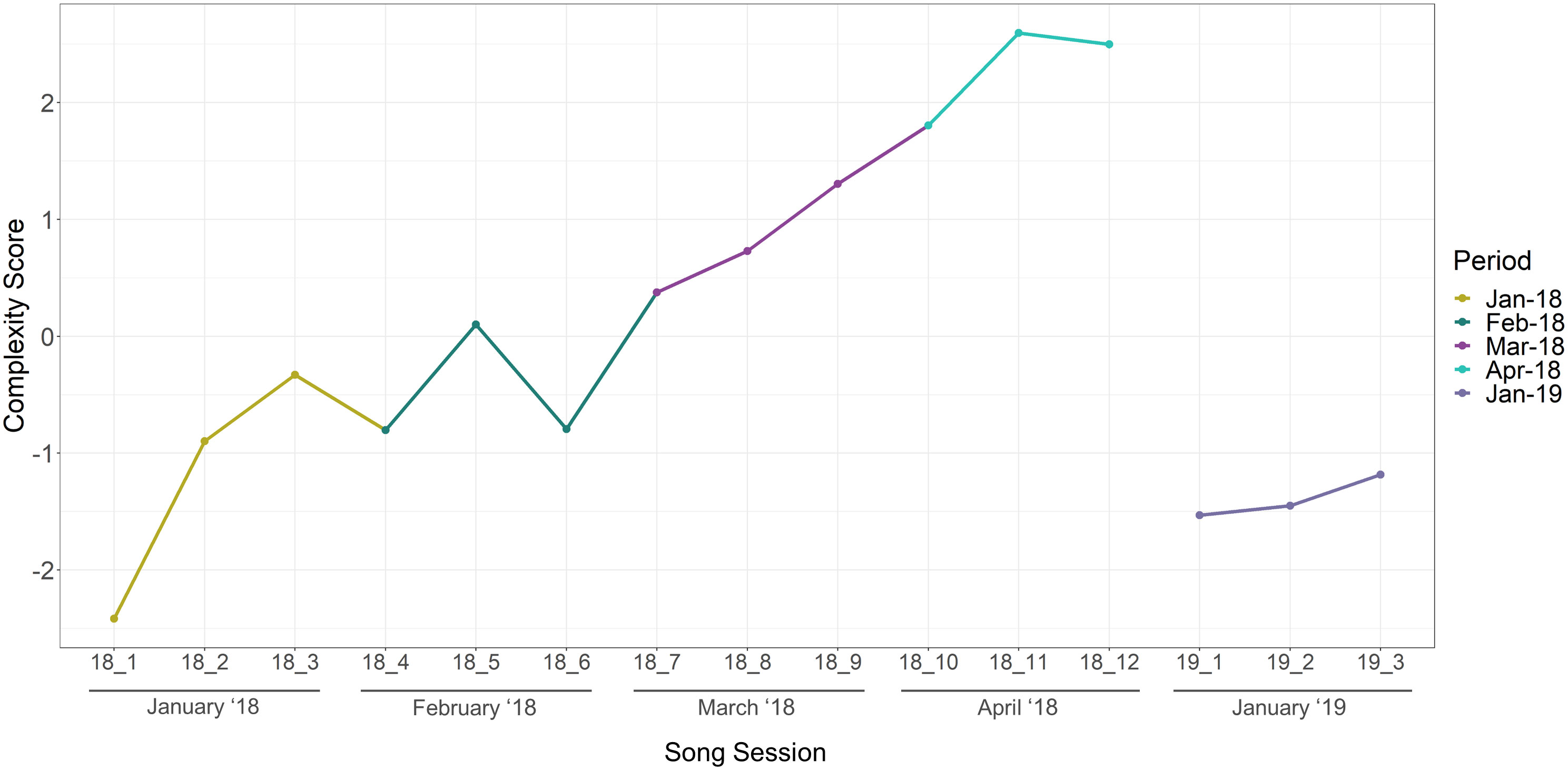
Figure 6 Song complexity over the study period January 2018 - January 2019. Each song session represents the average of three song cycles measured. The split in lines indicates lack of data (July-November).
Discussion
Humpback whale song from a subarctic feeding ground in Norwegian waters underwent a noticeable change through progressive evolution over one year. Songs were grouped into three general clusters, reflecting the rapidly evolving display over the study period, covering two migratory seasons. The observed songs comprised multiple themes which presented a rapid change in song content within the year of 2018 and between the years 2018 and 2019. The complexity of songs seemed to follow this pattern; songs became more complex throughout 2018 but presented a decrease in complexity between April 2018 and January 2019.
Temporal changes in song structure
The qualitative assessment of the humpback song structure resulted in several distinct themes with the majority occurring regularly and evolving over time. Only four themes were uncommon and theme order did not appear to be random, confirming the description made by Frumhoff (1983). The quantitative analyses using the weighted LSI revealed a gradual progression in song structure over one year. Despite shifting themes contributing to a greater variation, the LSI identified highly directional changes with all themes presenting modifications as time progressed. Two themes from 2018 reoccurred in the song the following year (2019), while all other themes were evolved versions of the previous year. This is largely in line with findings of previous studies investigating structural features of songs between seasons and/or years on breeding grounds and along their migratory route (e.g. Winn and Winn, 1978; Guinee et al., 1983; Payne et al., 1983; Payne and Payne, 1985; Eriksen et al., 2005). In Iceland, humpback whale song structure has also been documented to undergo structural changes over time with modifications occurring in the song unit and phrase repertoire (Magnúsdóttir et al., 2015). However, in contrast to the findings of the present study, the overlap in song content (phrase types, i.e. themes) was greater between two winters in Iceland, with half the phrases found to be either the same or sharing similar spectral features. This discrepancy could be due to the variability in the degree of change between periods, as studies have shown that in some years song progresses more rapidly than in others (Payne and Payne, 1985; Noad et al., 2000; Garland et al., 2011). Relatively little is known what prompts humpback whales to modify song features. It is generally accepted that the gradual evolution of the song is a result of different factors such as the introduction of novel material, production errors and/or errors in copying/learning (Noad et al., 2000; Garland et al., 2011; Cholewiak et al., 2013; Mcloughlin et al., 2018; Darling et al., 2019; Garland and McGregor, 2020; Garland et al., 2021).
Although songs were grouped into three general clusters on the upper level, they do not represent multiple song types but rather reflect the progressive song evolution throughout the study period. This was specifically apparent on the lower level where themes and their phrase types shared high similarities and clustered together across years. With all sampled whales conforming to the same version of a song, it might indicate that they are either from the same breeding population or that song learning occurred before the study period. Magnúsdóttir and Lim (2019) showed that the structure of humpback whale song recorded during one winter season in Icelandic waters resulted in one song type, corroborating the results of the present study. Areas around Iceland have been documented to represent a feeding ground and migratory passage route for North Atlantic humpbacks that are known to pass Norway (Whaletrack UiT, 2018; Kettemer et al., 2022). Using acoustic differences in song types and their content can identify the presence of a population and provide insight into its structure and migratory movements over a large spatial scale (Garland et al., 2015).
While the information of connections between themes (i.e. “new” and “old” versions) is available to the human classifier, it may not be as evident in the quantitative analysis using LSI (Garland et al., 2013b). Although sequence similarities on the theme level showed linkages between most themes, the connection between some themes was not or only partly detected (e.g. themes 1C and 7) by the hierarchically clustered and bootstrapped LSI. Thus, this quantitative approach using the LSI resulted in a shortcoming of identified linkages. Additionally, the delineation process of humpback whale song remains highly subjective (Cholewiak et al., 2013; Mercado, 2021). Although the protocol on how to delineate and measure phrases defined by Cholewiak et al. (2013) was followed in the present study, the acceptable level of variability within the classification procedure has a large subjective component (Mercado, 2021), making comparisons between studies difficult. Yet, a combined qualitative and quantitative assessment as applied in the present study has been suggested to produce more robust results (Garland et al., 2013b). Human classifiers were found to perform as well, or even better, as various quantitative computer methods (Janik, 1999; Riesch and Deecke, 2011). Thus, a combination of manual and computer techniques ensures that important components within humpback whale song are not overlooked (Garland et al., 2013b).
Complexity of songs
The analysis of humpback whale song complexity showed that songs became more complex as time progressed in 2018 but returned to a lower complexity the following year (January 2019), suggesting that the song properties changed between the years. This pattern is in line with previous findings by Allen et al. (2018) and Garland et al. (2021). Both studies presented a consistent long-term pattern with songs becoming more complex as they evolved but more simplified after a song has been completely replaced by a new version (i.e. song revolution). However, rather than revolutionary events where new material was introduced from another population being the cause for the decrease in song complexity, it appears that the whales have reset their songs over the migratory cycle. The positive association between complexity and individuality found by Allen et al. (2018) indicated that singers become more individually unique with increased song complexity. This theory of individual whales singing more complex songs in order to stand out has previously been proposed by Noad (2002). The reduced complexity of novel or evolved humpback whale songs, such as found in the present study, might be due to a potential limit of the song learning capacity over a certain period (Allen et al., 2018). The whales’ ability to rapidly learn and incorporate novel and/or more complex song may signal more developed cognitive abilities, indicative of the ‘cognitive capacity hypothesis’ (Allen et al., 2018; Garland et al., 2021). In birds, song complexity has shown to signal the learning ability of singing males (Boogert et al., 2008). Highly complex songs might indicate a higher cognitive capacity of singers, and thus may provide information about the quality as a mate (Catchpole, 1996; Nowicki et al., 2000; DeVoogd, 2004). The function of song as a metric of male fitness has also been proposed for humpback whale song. Song copying by males, while constantly incorporating changes as they occur, is believed to reveal a singer’s reproductive fitness to conspecifics (Payne, 2000; Herman, 2016). Sexual selection has generally been agreed to be a driving force in humpback whale song (Payne, 2000; Parsons et al., 2008). If the ability to rapidly adopt changes within the song demonstrates a higher cognitive ability in humpback whales, and with females selecting for this trait, then the content of the song itself may be less meaningful. Thus, it might rather be the learning ability of the singer that increases its reproductive chances (Garland et al., 2021; Allen et al., 2022). It remains unclear, however, whether processes of mate selection can explain why humpback whales constantly modify their songs over time, and whether females prefer males with larger song repertoires. Although previous studies describing humpback whale song structure reported a high level of variation in song duration (Fristrup et al., 2003), this variable is only informative if theme order is relatively invariant (Cholewiak et al., 2013). The number of units per song as well as the closely linked variable of song duration appeared to be the main causes for the higher song complexity in the present study. Allen et al. (2022) reported similar observations, suggesting that these changes are presumably copy errors or flexibility within song elements (e.g. unit repetition) used by the singers.
Given the relatively low sample size (three song cycles representing each of the 15 individuals) and considerable variability in song complexity within and between individuals, the results of this study must be interpreted with caution. As noted by Rekdahl (2012), the included songs in the analyses might have aberrant versions or a combination of different song types as previously documented by Noad et al. (2000). Thus, there is a potential for additional variability that is not captured by the sample and might have affected the outcomes by the complexity analysis trends. It is unclear what sample size is needed to reflect specific characteristics using a small subset, and consequently, to obtain representative results for humpback whale songs. Additionally, future research is encouraged to investigate the link between individually identified singers and their reproductive success in the light of more complex and/or novel songs, as already proposed by Garland et al. (2021).
Potential song exchange
Humpback whales in Norway have been reported to originate from two different breeding populations: Cape Verde, Africa, and the West Indies, America, (Broms et al., 2015; Whaletrack UiT, 2018; Wenzel et al., 2020). These findings indicate strong potential for acoustic interaction between humpbacks from two different breeding stocks and opportunities for cultural transmission to occur on this shared feeding ground in Northern Norway. This is supported by the results from the present study that all singers appeared to constantly incorporate the progressive changes throughout the study period. Song sharing within each month was high, indicating that animals from the two different populations might exchange song content already before reaching their breeding grounds. In addition, the song seemed to have progressed so rapidly that all themes were replaced by new versions after 12 months. The variation exhibited at this temporal level suggests that the song might be completely replaced by a new version the following migration seasons. It must be noted that due to the small sample size in 2019, additional variability may not have been captured, suggestive of different overlaps in similarity between songs of each year.
Song learning is most likely facilitated through contact of different breeding populations on feeding grounds or overlapping migration routes, representing a potential mechanism of song transmission (Garland et al., 2011; Garland et al., 2013a; Garland et al., 2013b; Allen et al., 2022; Schulze et al., 2022). The rather extensive modifications in some themes between the years (e.g. theme 5A to theme 5B) presented here, might have occurred when whales moved away from the shared feeding aggregation, with a different or modified version being sung at each breeding ground. These two versions might have then, in turn, been transmitted again when singers of the two breeding stocks came in acoustic contact. Such overlap in the acoustic space may happen on the shared feeding ground in the Barents Sea and/or Norwegian waters, resulting in song mixing and/or matching. Compositions of humpback whale song in Bermuda and Hawaii were documented to have a complete turnover within 4-5 years (Payne et al., 1983; Payne and Payne, 1985), whereas a study off eastern Australia reported a turnover rate of a two-year period (Noad et al., 2000). The song revolution found off eastern Australia by Noad et al. (2000) was due to an interchange of two populations where humpbacks from eastern Australia adopted the song type from the western Australian population. However, this song revolution event to incorporate a new song into a population’s repertoire might have taken longer than initially believed, as proposed by Rekdahl (2012). Recent work by Zandberg et al. (2021) using cultural evolutionary models showed that humpback whales within the Northern Hemisphere exhibit a high degree of song sharing among populations and lower turnover rates within populations. Consequently, the authors concluded that song revolutions are highly unlikely within humpback whale populations of the Northern Hemisphere, with song evolution being the dominating process (Zandberg et al., 2021). This argument is strengthened by the results presented here, demonstrating that humpback whale song from the north-east Atlantic is rapidly evolving. Future empirical work, including more samples over several seasons and years, would help in identifying the turnover rate of humpback whale song on this feeding ground and migratory route off Northern Norway. Song exchange on shared feeding grounds might be a key driving force behind cultural transmission for humpback whale populations within the North Atlantic, as put forward by Magnúsdóttir and Lim (2019). To elucidate the degree of song exchange between different breeding populations coming together on this shared, high-latitude feeding ground, detailed comparisons of song patterns across locations and multiple years are necessary. The use of passive acoustic monitoring (PAM) to collect data and comparisons of song types across the North Atlantic could identify the degree of mixing of these two populations.
Although the underlying drivers of humpback whale song remain elusive, a number of explanations for high-latitude singing behavior have been proposed (Clark and Clapham, 2004; Stimpert et al., 2012; Garland et al., 2013a; Herman et al., 2013; Magnúsdóttir et al., 2015), the main involving the reproduction cycle (Clark and Clapham, 2004; Vu et al., 2012; Magnúsdóttir et al., 2015) and song practicing and/or learning by males (Clark and Clapham, 2004; Herman et al., 2013). Assuming that singing behavior in humpback whales is primarily related to breeding activities (Herman, 2016), Norwegian waters might not only serve as a feeding ground but also as an alternative breeding area for individuals that skip migration. More systematic visual year-round observations would help in uncovering if humpback whales engage in breeding activities other than singing. Nonetheless, this high-latitude region represents more than a migratory feeding stopover for humpback whales within the north-east Atlantic, which also display mating behavior (i.e. singing).
Conclusion
This study provides a detailed account of humpback whale song progression in subarctic waters of Northern Norway using PAM. Norwegian waters represent a biologically important feeding and migratory area for different breeding populations of humpback whales in the North Atlantic. Comparisons of the song structure showed noticeable modifications through progressive evolution over the study period of one year. The changes in song complexity appeared to mirror the song evolution with increasingly complex songs as time progressed within the season, returning to more simplified songs by the following year. This underlines the rapidly evolving display between two years and supports the hypothesis of a potential limit to the learning capacity of humpback whales, regardless of a cultural revolution event being present. This study suggest that there is strong potential for song exchange and opportunities for cultural transmission on this shared feeding ground. The results presented here bridge a knowledge gap on North Atlantic humpback whale song evolution and provide insights into potential drivers of the transmission of a cultural trait and social learning in humpback whales. Future work including larger sample sizes from multiple years and locations (e.g. North Atlantic breeding ground) are required before cultural transmission in this high-latitude area can be determined conclusively. Establishing a common technique for the delineation of humpback whale song elements is essential for cross-comparisons between different areas. Analyses of acoustic similarities can identify different populations and determine their connectivity. Therefore, song sequence comparisons present an efficient method to examine humpback whale population structure, its migratory movements, and possible song transmission between different populations. Additionally, more visual observations would help unravel the importance of this high-latitude region for humpback whales in terms of plasticity in mating area selection within the North Atlantic.
Data availability statement
Publicly available datasets were analyzed in this study. The raw data can be found here: https://love.statoil.com. The processed data, detailing transcribed humpback whale song elements can be acquired upon request to the corresponding authors of this article. The raw data supporting the conclusions of this article will be made available by the authors, without undue reservation.
Author contributions
ST and AA designed the study. ST processed the data, performed the analysis, and took the lead in writing the manuscript. AA and UL supervised the project. AA, HA, GP, and UL provided critical feedback and helped shape the research, analysis, and manuscript. All authors contributed to the article and approved the submitted version.
Acknowledgments
We would like to express our appreciation to Dr. Luke Rendell for providing critical feedback to this work. We are grateful to Dr. Ellen Garland for the valuable insights in and support with the classification of song elements. For statistical advice, a big thank you to Drs. Michael Greenacre, Raul Primicerio, and Jessica Abbott.
Funding
Acoustic sampling was provided by Equinor ASA funding LoVe phase 1, and the Research Council of Norway under contract no. 245843 and partners funding LoVe phase 2. This project was made possible with the support of the Erasmus+ programme of the European Union and open access funding enabled by UiT Arctic University of Norway. Funding for this work was partially provided by a postdoc scholarship from VISTA – a research program in collaboration between the Norwegian Academy of Sciences and Letters and Equinor. The authors declare that this project was partly funded by Equinor ASA. The funder was not involved in the study analysis, interpretation of data, the writing of this article or the decision to submit it for publication.
Conflict of interest
The authors declare that the research was conducted in the absence of any commercial or financial relationships that could be construed as a potential conflict of interest.
Publisher’s note
All claims expressed in this article are solely those of the authors and do not necessarily represent those of their affiliated organizations, or those of the publisher, the editors and the reviewers. Any product that may be evaluated in this article, or claim that may be made by its manufacturer, is not guaranteed or endorsed by the publisher.
Supplementary material
The Supplementary Material for this article can be found online at: https://www.frontiersin.org/articles/10.3389/fmars.2022.862794/full#supplementary-material
References
Allen J., Garland E. C., Dunlop R. A., Noad M. J. (2018). Cultural revolutions reduce complexity in the songs of humpback whales. Proc. R. Soc. B: Biol. Sci. 285, 20182088. doi: 10.1098/rspb.2018.2088
Allen J., Murray A., Noad M. J., Dunlop R. A., Garland E. C. (2017). Using self-organizing maps to classify humpback whale song units and quantify their similarity. J. Acoustical Soc. America 142, 1943–1952. doi: 10.1121/1.4982040
Allen J. A., Garland E. C., Garrigue C., Dunlop R. A., Noad M. J.. (2022). Song complexity is maintained during inter-population cultural transmission of humpback whale songs. Sci. Rep. 12, 8999. doi: 10.1038/s41598-022-12784-3
Baker M. C., Logue D. M. (2007). A comparison of three noise reduction procedures applied to bird vocal signals. J. Field Ornithol 78, 240–253. doi: 10.1111/j.1557-9263.2007.00109.x
Baker C. S., Palumbi S. R., Lambertsen R. H., Weinrich M. T., Calambokidis J., O’Brien S. J. (1990). Influence of seasonal migration on geographic distribution of mitochondrial DNA haplotypes in humpback whales. Lett. to Nat. 344, 238–240. doi: 10.1038/344238a0
Bérubé M., Rew M. B., Cole T., Swartz S., Zolman E., Oien N., et al. (2004). Genetic identification of an individual humpback whale between the eastern Caribbean and the Norwegian sea. Mar. Mammal Sci. 20, 657–663. doi: 10.1111/j.1748-7692.2004.tb01185.x
Boogert N. J., Giraldeau L. A., Lefebvre L. (2008). Song complexity correlates with learning ability in zebra finch males. Anim. Behav. 76, 1735–1741. doi: 10.1016/j.anbehav.2008.08.009
Broms F., Wenzel F., Suárez P. L., Stevick P. T., Biuw M., Berrow S. (2015). “Recent research on the migratory destinations of humpback whales (Megaptera novaeangliae) from a mid-winter feeding stop-over area in northern Norway,” in Recent research on the migratory destinations of humpback whales. Ed. Wenzel F. W. (St. Juliens, Malta: European Cetacean Society Special Publication).
Broughton W. B. (1963). “Method in bioacoustic terminology,” in Acoustic behaviour of animals. Ed. Busnel R. G. (Amsterdam: Elsevier), 824–910.
Catchpole C. K. (1996). Song and female choice: good genes and big brains? Trends Ecol. Evol. 11, 358–360. doi: 10.7591/9781501736957-034
Cato D. H. (1991). Songs of humpback whales: the Australian perspective. Memoirs Queensland Museum 30.2, 277–290.
Cerchio S., Jacobsen J. K., Norris T. F. (2001). Temporal and geographical variation in songs of humpback whales, Megaptera novaeangliae: Synchronous change in Hawaiian and Mexican breeding assemblages. Anim. Behav. 62, 313–329. doi: 10.1006/anbe.2001.1747
Cholewiak D. M. (2008). Evaluating the role of song in the humpback whale (Megaptera novaeangliae) breeding system with respect to intra-sexual interactions. PhD thesis (Ithaca, NY: Cornell University), 159 p. Available at: http://ecommons.library.cornell.edu/handle/1813/11206.
Cholewiak D. M., Sousa-Lima R. S., Cerchio S. (2013). Humpback whale song hierarchical structure: Historical context and discussion of current classification issues. Mar. Mammal Sci. 29, 312–332. doi: 10.1111/mms.12005
Chu K. C. (1988). Dive times and ventilation patterns of singing humpback whales (Megaptera novaeangliae). Can. J. Zool 66, 1322–1327. doi: 10.1139/z88-194
Clark C. W., Clapham P. J. (2004). Acoustic monitoring on a humpback whale (Megaptera novaeangliae) feeding ground shows continual singing into late spring. Proc. R. Soc. B: Biol. Sci. 271, 1051–1057. doi: 10.1098/rspb.2004.2699
Darling J. D., Acebes J. M. V., Frey O., Jorge Urbán R., Yamaguchi M. (2019). Convergence and divergence of songs suggests ongoing, but annually variable, mixing of humpback whale populations throughout the north pacific. Sci. Rep. 9, 1–14. doi: 10.1038/s41598-019-42233-7
Darling J. D., Bérubé M. (2001). Interactions of singing humpback whales with other males. Mar. Mammal Sci. 17, 570–584. doi: 10.1111/j.1748-7692.2001.tb01005.x
Darling J. D., Jones M. E., Nicklin C. P. (2006). Humpback whale songs: Do they organize males during the breeding season? Behaviour 143, 1051–1101. doi: 10.1163/156853906778607381
Dawbin W. (1966). “The seasonal migratory cycle of humpback whales,” in Whales, dolphins and porpoises. Ed. Norris K. S. (Los Angeles: University of California Press), 145–170.
Delarue J., Todd S. K., Van Parijs S. M., Luica D. I. (2009). Geographic variation in Northwest Atlantic fin whale (Balaenoptera physalus) song: implications for stock structure assessment. J. Acoustical Soc. America 125, 1774–1782. doi: 10.1121/1.3068454
DeVoogd T. J. (2004). Neural constraints on the complexity of avian song. Brain Behav. Evol. 63, 221–232. doi: 10.1159/000076783
Dunlop R. A., Noad M. J., Cato D. H., Stokes D. (2007). The social vocalization repertoire of east Australian migrating humpback whales (Megaptera novaeangliae). J. Acoustical Soc. America 122, 2893–2905. doi: 10.1121/1.2783115
Equinor and IMR (2020) Ocean observatory archive. Available at: http://love.statoil.com/ (Accessed October 15, 2020).
Eriksen N., Miller L. A., Tougaard J. (2005). Cultural change in the songs of humpback whales (Megaptera novaeangliae) from Tonga. Behaviour 142, 305–328. doi: 10.1163/1568539053778283
Fristrup K. M., Hatch L. T., Clark C. W. (2003). Variation in humpback whale (Megaptera novaeangliae) song length in relation to low-frequency sound broadcasts. J. Acoustical Soc. America 113, 3411–3424. doi: 10.1121/1.1573637
Frumhoff P. (1983). “Aberrant Songs of Humpback Whales (Magaptera navaengliae): Clues to the Structure of Humpback Songs,” in Payne R. Ed. Communication and behavior of whales. AAAS Selected Symposium 76, 81–127.
Garland E. C., Garrigue C., Noad M. J. (2021). When does cultural evolution become cumulative culture? a case study of humpback whale song. Phil Trans. R. Soc B 377, 20200313. doi: 10.1098/rstb.2020.0313
Garland E. C., Gedamke J., Rekdahl M. L., Noad M. J., Garrigue C., Gales N. (2013a). Humpback whale song on the southern ocean feeding grounds: Implications for cultural transmission. PloS One 8 (11), e79422. doi: 10.1371/journal.pone.0079422
Garland E. C., Goldizen A. W., Lilley M. S., Rekdahl M. L., Garrigue C., Constantine R., et al. (2015). Population structure of humpback whales in the western and central south pacific ocean as determined by vocal exchange among populations. Conserv. Biol. 29, 1198–1207. doi: 10.1111/cobi.12492
Garland E. C., Goldizen A. W., Rekdahl M. L., Constantine R., Garrigue C., Hauser N. D., et al. (2011). Dynamic horizontal cultural transmission of humpback whale song at the ocean basin scale. Curr. Biol. 21, 687–691. doi: 10.1016/j.cub.2011.03.019
Garland E. C., Lilley (2020) Levenshtein calculations (package ‘leven’, version 0.2.1). Available at: https://github.com/ellengarland/leven (Accessed March 10, 2021).
Garland E. C., Lilley M. S., Goldizen A. W., Rekdahl M. L., Garrigue C., Noad M. J. (2012). Improved versions of the levenshtein distance method for comparing sequence information in animals’ vocalisations: Tests using humpback whale song. Behaviour 149, 1413–1441. doi: 10.1163/1568539X-00003032
Garland E. C., McGregor P. K. (2020). Cultural transmission, evolution, and revolution in vocal displays: Insights from bird and whale song. Front. Psychol. 11. doi: 10.3389/fpsyg.2020.544929
Garland E. C., Noad M. J., Goldizen A. W., Lilley M. S., Rekdahl M. L., Garrigue C., et al. (2013b). Quantifying humpback whale song sequences to understand the dynamics of song exchange at the ocean basin scale. J. Acoustical Soc. America 133, 560–569. doi: 10.1121/1.4770232
Garland E. C., Rendell L., Lilley M. S., Poole M. M., Allen J., Noad M. J. (2017). The devil is in the detail: Quantifying vocal variation in a complex, multi-levelled, and rapidly evolving display. J. Acoustical Soc. America 142, 460–472. doi: 10.1121/1.4991320
Godø O. R., Johnsen S., Torkelsen T. (2014). The LoVe ocean observatory is in operation. Mar. Technol. Soc. J. 48, 24–30. doi: 10.4031/MTSJ.48.2.2
Guinee L. N., Chu K., Dorsey E. M. (1983). “Changes over time in the songs of known individual humpback whales (Megaptera novaeangliae),” in Communication and behavior of whales. Ed. Payne R. S. (Boulder, Colorado: Westview Press, Inc), 59–80.
Hafner G. W., Hamilton C. L., Steiner W. W., Thompson T. J., Winn H. E. (1979). Signature information in the song of the humpback whale. J. Acoustical Soc. America 66, 1–6. doi: 10.1121/1.383072
Helweg D., Cato D. H., Jenkins P. F., Garrigue C., McCauley R. D. (1998). Geographic variation in south pacific humpback whale songs. Behaviour 135, 1–27. doi: 10.1163/156853998793066438
Helweg D., Herman L. M., Yamamoto S., Forestell P. H. (1990). Comparison of songs of humpback whales (Megaptera novaeangliae) recorded in Japan, Hawaii, and Mexico during the winter of 1989. Sci. Rep. Cetacean Res. 1, 1–20.
Herman L. M. (2016). The multiple functions of male song within the humpback whale (Megaptera novaeangliae) mating system: review, evaluation, and synthesis. Biol. Rev. 92 (3), 1795–1818. doi: 10.1111/brv.12309
Herman L. M., Pack A. A., Spitz S. S., Herman E. Y. K., Rose K., Hakala S., et al. (2013). Humpback whale song: Who sings? Behav. Ecol. Sociobiol 67, 1653–1663. doi: 10.1007/s00265-013-1576-8
Janik V. M. (1999). Pitfalls in the categorization of behaviour: A comparison of dolphin whistle classification methods. Anim. Behav. 57, 133–143. doi: 10.1006/anbe.1998.0923
Janik V. M., Slater P. J. B. (2000). The different roles of social learning in vocal communication. Anim. Behav. 60, 1–11. doi: 10.1006/anbe.2000.1410
Jolliffe I. T. (2002). Principal component analysis. 2nd ed. (New York: Springer-Verlag New York, Inc).
Kettemer L. E., Rikardsen A. H., Biuw M., Broms F., Mul E., Blanchet M.-A. (2022). Round-trip migration and energy budget of a breeding female humpback whale in the northeast Atlantic. PloS One 17 (5), e0268355. doi: 10.1371/journal.pone.0268355
Kohonen T. (1985). Median strings. Pattern Recognition Lett. 3, 309–313. doi: 10.1016/0167-8655(85)90061-3
Kroodsma D. (2004). “The diversity and plasticity of birdsong,” in Nature’s music: The science of birdsong (USA: Elsevier), 108–131. doi: 10.1016/B978-012473070-0/50007-4
Levenshtein V. I. (1966). Binary codes capable of correcting deletions, insertions and reversals. Soviet Phys. Doklady 10, 707–710.
Magnúsdóttir E. E., Lim R. (2019). Subarctic singers: Humpback whale (Megaptera novaeangliae) song structure and progression from an icelandic feeding ground during winter. PloS One 14 (1), e0210057. doi: 10.1371/journal.pone.0210057
Magnúsdóttir E. E., Miller P. J. O., Lim R., Rasmussen M. H., Lammers M. O., Svavarsson J. (2015). Humpback whale (Megaptera novaeangliae) song unit and phrase repertoire progression on a subarctic feeding ground. J. Acoustical Soc. America 138, 3362–3374. doi: 10.1121/1.4935517
Mcloughlin M., Lamoni L., Garland E. C., Ingram S., Kirke A., Noad M. J., et al. (2018). Using agent-based models to understand the role of individuals in the song evolution of humpback whales (Megaptera novaeangliae). Music Sci. 1, 1–17. doi: 10.1177/2059204318757021
Mercado E. (2021). Song morphing by humpback whales: Cultural or epiphenomenal? Front. Psychol. 11. doi: 10.3389/fpsyg.2020.574403
Murray A., Dunlop R. A., Noad M. J., Goldizen A. W. (2018). Stereotypic and complex phrase types provide structural evidence for a multi-message display in humpback whales (Megaptera novaeangliae). J. Acoustical Soc. America 143, 980–994. doi: 10.1121/1.5023680
Noad M. J. (2002). The use of song by humpback whales (Megaptera novaeangliae) during migration off the east coast of australia. PhD thesis (Australia: University of Sydney).
Noad M. J., Cato D. H., Bryden M. M., Jenner M.-N., Jenner K. C. S. (2000). Cultural revolution in whale songs. Nature 408, 537. doi: 10.1038/35046199
Nowicki S., Hasselquist D., Bensch S., Peters S. (2000). Nestling growth and song repertoire size in great reed warblers: evidence for song learning as an indicator mechanism in mate choice. Proc. R. Soc. B: Biol. Sci. 267, 2419–2424. doi: 10.1098/rspb.2000.1300
Owen C., Rendell L., Constantine R., Noad M. J., Allen J., Andrews O., et al. (2019). Migratory convergence facilitates cultural transmission of humpback whale song. R. Soc. Open Sci. 6, 190337. doi: 10.1098/rsos.190337
Parsons E. C. M., Gore M. A., Wright A. J. (2008). The nature of humpback whale (Megaptera novaeangliae) song. J. Mar. Anim. Their Ecol. 1(1), 22–31
Payne R. S. (1978). “Behavior and vocalizations of humpback whales (Megaptera sp.),” in Report on a Workshop on Problems Related to Humpback Whales (Megaptera novaeangliae) in Hawaii. Eds. Norris K. S., Reeves R. R. (Washington, D.C.: Report prepared for the U.S. Marine Mammal Commission), NTIS PB-280 794, 56–78.
Payne K. (2000). “The progressively changing songs of humpback whales: a window on the creative process in a wild animal,” in Origins of music. Eds. Wallin N. L., Merker B., Brown S. (Cambridge, MA: MIT Press), 135–150.
Payne R. S., Guinee L. N. (1983). “Humpback whale songs as an indicator of ‘stocks,” in Communication and behavior of whales (Boulder, CO: Westview Press), 333–358.
Payne R. S., McVay S. (1971). Songs of humpback whales. Science 173, 585–597. doi: 10.1126/science.173.3997.585
Payne K., Payne R. S. (1985). Large Scale changes over 19 years in songs of humpback whales in Bermuda. Z. für Tierpsychologie 68, 89–114. doi: 10.1111/j.1439-0310.1985.tb00118ö.x
Payne K., Tyack P., Payne R. S. (1983). Progressive changes in the songs of humpback whales (Megaptera novaeangliae): A detailed analysis of two seasons in Hawaii. Communication Behav. Whales, 9–57.
Ramm T. (2020). Hungry during migration? humpback whale movement from the barents Sea to a feeding stopover in northern Norway revealed by photo-ID analysis. M.Sc thesis (Tromsø: Arctic University of Norway), 41 p. Available at: https://munin.uit.no/handle/10037/19109.
R Core Team (2021). R: A language and environment for statistical computing (Vienna, Austria: R Foundation for Statistical Computing). Available at: https://www.R-project.org/.
Rekdahl M. (2012). Humpback whale vocal communication: Use and stability of social calls and revolutions in the songs of east Australian whales. PhD thesis (Australia: University of Queensland), 174 p.
Rekdahl M. L., Garland E. C., Carvajal A., King C. D., Collins T., Razafindrakoto Y., et al. (2018). Culturally transmitted song exchange between humpback whales (Megaptera novaeangliae) in the southeast Atlantic and southwest Indian ocean basins. R. Soc. 5, 1–15. doi: 10.1098/rsos.172305
Riesch R., Deecke V. B. (2011). Whistle communication in mammal-eating killer whales (Orcinus orca): further evidence for acoustic divergence between ecotypes. Behav. Ecol. Sociobiol 65, 1377–1387. doi: 10.1007/s00265-011-1148-8
Schulze J. N., Denkinger J., Oña J., Poole M. M., Garland E. C. (2022). Humpback whale song revolutions continue to spread from the central into the eastern south pacific. R. Soc Open Sci. 9, 220158. doi: 10.1098/rsos.220158
Silber G. K. (1986). The relationship of social vocalizations to surface behavior and aggression in the Hawaiian humpback whale (Megaptera novaeangliae). Can. J. Zool 64, 2075–2080. doi: 10.1139/z86-316
Smith J. N., Goldizen A. W., Dunlop R. A., Noad M. J. (2008). Songs of male humpback whales, Megaptera novaeangliae, are involved in intersexual interactions. Anim. Behav. 76, 467–477. doi: 10.1016/j.anbehav.2008.02.013
Sokal R. R., Rohlf F. J. (1962). The comparison of dendrograms by objective methods. Taxon 11, 33–40. doi: 10.2307/1217208
Stafford K. M., Lydersen C., Wiig Ø., Kovacs K. M. (2018). Extreme diversity in the songs of spitsbergen’s bowhead whales. Biol. Lett. 14, 20180056. doi: 10.1098/rsbl.2018.0056
Stafford K. M., Nieukirk S. L., Fox C. G. (2001). Geographical and seasonal variation of blue whale calls of the north pacific. J. Cetacean Res. Manage. 3, 65–76.
Stevick P. T., Allen J., Clapham P. J., Katona S. K., Larsen F., Lien J., et al. (2006). Population spatial structuring on the feeding grounds in north Atlantic humpback whales (Megaptera novaeangliae). J. Zool 270, 244–255. doi: 10.1111/j.1469-7998.2006.00128.x
Stevick P. T., Berrow S. D., Bérubé M., Bouveret L., Broms F., Jann B., et al. (2016). There and back again: Multiple and return exchange of humpback whales between breeding habitats separated by an ocean basin. J. Mar. Biol. Assoc. United Kingdom 1, 1–6. doi: 10.1017/S0025315416000321
Stevick P. T., Bouveret L., Gandilhon N., Rinaldi C., Rinaldi R., Broms F., et al. (2018). Migratory destinations and timing of humpback whales in the southeastern Caribbean differ from those off the Dominican republic. J. Cetacean Res. Manage. 18, 127–133.
Stimpert A. K., Peavey L. E., Friedlaender A. S., Nowacek D. P. (2012). Humpback whale song and foraging behavior on an Antarctic feeding ground. PloS One 7, 1–8. doi: 10.1371/journal.pone.0051214
Suzuki R., Shimodaira H. (2006). Pvclust: an r package for assessing the uncertainty in hierarchical clustering. Bioinformatics 22, 1540–1542. doi: 10.1093/bioinformatics/btl117
Templeton C. N., Laland K. N., Boogert N. J. (2014). Does song complexity correlate with problem-solving performance inflocks of zebra finches? Anim. Behav. 92, 63–71. doi: 10.1016/j.anbehav.2014.03.019
Tougaard J., Eriksen N. (2006). Analysing differences among animal songs quantitatively by means of the levenshtein distance. Behaviour 143, 239–252.
Tyarks S. C., Aniceto A. S., Ahonen H., Pedersen G., Lindstrøm U. (2021). Humpback whale (Megaptera novaeangliae) song on a subarctic feeding ground. Front. Mar. Sci. 8. doi: 10.3389/fmars.2021.669748
Vu E. T., Risch D., Clark C. W., Gaylord S., Hatch L., Thompson M. A., et al. (2012). Humpback whale song occurs extensively on feeding grounds in the western north Atlantic ocean. Aquat. Biol. 14, 175–183. doi: 10.3354/ab00390
Warren V. E., Constantine R., Noad M. J., Garrigue C., Garland E. C., Warren V. E. (2020). Migratory insights from singing humpback whales recorded around central new Zealand. R. Soc. Open Sci. 7, 201084. doi: 10.1098/rsos.201084
Wenzel F., Broms F., López-Suárez P., Lopes K., Veiga N., Yeoman K., et al. (2020). Humpback whales (Megaptera novaeangliae) in the cape verde islands: Migratory patterns, resightings, and abundance. Aquat. Mammals 46, 21–31. doi: 10.1578/AM.46.1.2020.21
Whaletrack UiT (2018) (Whaletrack). Available at: https://en.uit.no/prosjekter/prosjekt?p_document_id=505966 (Accessed May 29, 2021).
Winn H. E., Winn L. K. (1978). The song of the humpback whale Megaptera novaeangliae in the West indies. Mar. Biol. 47, 97–114. doi: 10.1007/BF00395631
Yang K. L., Center for Conservation Bioacoustics (2019). Raven pro: Interactive sound analysis software (Version 1.6.1) (Ithaca, NY: The Cornell Lab of Ornithology). Available at: http://ravensoundsoftware.com/.
Keywords: passive acoustic monitoring, singing, Megaptera novaeangliae, North Atlantic, vocal behavior, song progression
Citation: Tyarks SC, Aniceto AS, Ahonen H, Pedersen G and Lindstrøm U (2022) Changes in humpback whale song structure and complexity reveal a rapid evolution on a feeding ground in Northern Norway. Front. Mar. Sci. 9:862794. doi: 10.3389/fmars.2022.862794
Received: 26 January 2022; Accepted: 24 November 2022;
Published: 09 December 2022.
Edited by:
Rebecca Dunlop, The University of Queensland, AustraliaReviewed by:
Michael Noad, The University of Queensland, AustraliaJennifer Allen, Griffith University, Australia
Angela Paola Recalde-Salas, Curtin University, Australia
Copyright © 2022 Tyarks, Aniceto, Ahonen, Pedersen and Lindstrøm. This is an open-access article distributed under the terms of the Creative Commons Attribution License (CC BY). The use, distribution or reproduction in other forums is permitted, provided the original author(s) and the copyright owner(s) are credited and that the original publication in this journal is cited, in accordance with accepted academic practice. No use, distribution or reproduction is permitted which does not comply with these terms.
*Correspondence: Saskia C. Tyarks, c2Fza2lhdHlhcmtzQGdtYWlsLmNvbQ==