Corrigendum: Revising the Impact and Prospects of Activity and Ventilation Rate Bio-Loggers for Tracking Welfare and Fish-Environment Interactions in Salmonids and Mediterranean Farmed Fish
- 1Nutrigenomics and Fish Growth Endocrinology Group, Institute of Aquaculture Torre de la Sal (IATS-CSIC), Castellón, Spain
- 2Technological Centre for Innovation in Communications (iDeTIC), University of Las Palmas de Gran Canaria (ULPGC), Las Palmas, Spain
- 3Institute for Applied Microelectronics (IUMA), University of Las Palmas de Gran Canaria, Las Palmas, Spain
- 4INRAE, Laboratoire de Physiologie et de Génomique des Poissons (LPGP), Rennes, France
- 5Nofima AS, Tromsø, Norway
- 6MARBEC, Univ. Montpellier, CNRS, Ifremer, IRD, Palavas-les-Flots, France
- 7Faculty of Biosciences, Fisheries and Economics, The Norwegian College of Fishery Science, UiT The Arctic University of Norway, Tromsø, Norway
- 8INRAE, PEIMA, Sizun, France
- 9Aquaculture Research Group, Institute of Sustainable Aquaculture and Marine Ecosystems (IU-ECOAQUA), University of Las Palmas de Gran Canaria, Las Palmas, Spain
Behavioral parameters are reliable and useful operational welfare indicators that yield information on fish health and welfare status in aquaculture. However, aquatic environment is still constraining for some solutions based on underwater cameras or echo sounder transmitters. Thus, the use of bio-loggers internally or externally attached to sentinel fish emerges as a solution for fish welfare monitoring in tanks- and sea cages-rearing systems. This review is focused on the recently developed AEFishBIT, a small and light data storage tag designed to be externally attached to fish operculum for individual and simultaneous monitoring of swimming activity and ventilation rates under steady and unsteady swimming conditions for short-term periods. AEFishBIT is a tri-axial accelerometer with a frequency sampling of 50–100 Hz that is able to provide proxy measurements of physical and metabolic activities validated by video recording, exercise tests in swim tunnel respirometers, and differential operculum and body tail movements across fish species with differences in swimming capabilities. Tagging procedures based on tag piercing and surgery procedures are adapted to species anatomical head and operculum features, which allowed trained operators to quickly complete the tagging procedure with a fast post-tagging recovery of just 2.5–7 h in both salmonid (rainbow trout, Atlantic salmon) and non-salmonid (gilthead sea bream, European sea bass) farmed fish. Dual recorded data are processed by on-board algorithms, providing valuable information on adaptive behavior through the productive cycle with the changing environment and genetics. Such biosensing approach also provides valuable information on social behavior in terms of adaptive capacities or changes in daily or seasonal activity, linking respiratory rates with changes in metabolic rates and energy partitioning between growth and physical activity. At short-term, upcoming improvements in device design and accompanying software are envisaged, including energy-harvesting techniques aimed to prolong the battery life and the addition of a gyroscope for the estimation of the spatial distribution of fish movements. Altogether, the measured features of AEFishBIT will assist researchers, fish farmers and breeders to establish stricter welfare criteria, suitable feeding strategies, and to produce more robust and efficient fish in a changing environment, helping to improve fish management and aquaculture profitability.
Introduction
To meet the increasing global demand of fish protein, aquaculture production is becoming more intensified, which requires the selection of non-aggressive individuals that perform well at high densities (Huntingford, 2004; Huntingford et al., 2006). The potential benefits are obvious because fish with high stress resilience are less susceptible to diseases, show better growth performance, and provide a better-quality product (Ashley, 2007). However, animal health and welfare issues are of increasing public concern, and livestock industries and aquaculture in particular require to project a welfare-friendly image of their products (Hemsworth and Coleman, 2011; Toni et al., 2019; Barreto et al., 2021). The challenge is that fish aquaculture involves a huge number of species and the understanding of their welfare biology is limited, which explains why relatively few operational welfare indicators (OWI) are used to assess welfare status on commercial aquaculture facilities (Segner et al., 2019). Major advances on the establishment of OWIs have been achieved in Atlantic salmon (Noble et al., 2018; Rey et al., 2019), trout (Noble et al., 2020), and tilapia (Pedrazzani et al., 2020). Less standardized are the welfare assessment protocols in other farmed fish, such as gilthead sea bream and European sea bass (Papaharisis et al., 2019; Sadoul et al., 2021), though specific key performance indicator-based benchmarking systems have been recently validated within the framework of MedAID1 and PerformFISH2 H2020 EU projects. All this will contribute to ensure that fish welfare is properly assessed in different aquaculture production systems to warrant that currently cultured fish strains are not far from their optimum welfare (Saraiva et al., 2018; Saraiva and Arechavala-Lopez, 2019). It is thereby important to encompass the development of aquaculture with novel and stricter criteria of welfare for the simultaneous improvement of the productivity and the welfare of group-housed animals. This includes the use of both animal-based indicators (something you monitor from the fish) and indirect resource-based indicators (measurements of rearing environment), but further research is needed for a full and rapid transition from highly informative laboratory-based welfare indicators (LABWI) to OWI (Noble et al., 2018). A good example of a fast transition is the portable MinION nanopore sequencer, which allows fish farmers and researchers to obtain rapid microbial profiles and RNA viruses diagnoses of reared fish (Gallagher et al., 2018; Gonçalves et al., 2020). Hence, with the advent of new technologies it will be relatively easy to integrate insights from the environment, microbial loads, and fish behavior and appearance, which can be then scored according to the suggested welfare status from 0 (worst) to 1 (best) (Noble et al., 2018, 2019).
Behavioral indicators are becoming especially useful for alerting farmers that something is potentially wrong and warrants further investigation before significant welfare issues and mortality occur (Martins et al., 2012; Sharma, 2019). Thus, exploratory behavior or feed anticipatory activities are considered indicators of good welfare. By contrast, abnormal swimming and poor response to feed or novel objects are indicators of disease and stressful conditions, depending on how a particular animal perceives handling and restraint on the previous experiences and inherited traits. Thus, selective breeding of more social animals resulted in the improved behavior of the offspring (de Goede et al., 2013; Balasch and Tort, 2019). The challenge is that very often behavioral indicators are difficult to quantify in the aquatic environment (absolute changes in swimming speed, aggression levels, and gill beat frequency) and proper assessment of fish welfare often requires additional physiological measurements (Sadoul et al., 2021). Moreover, these analysis becomes tedious and laborious, though underwater cameras and echo sounder technology are relatively inexpensive and provide the opportunity for real time observation of fish behavior and intelligent feeding (Hung et al., 2016; Li et al., 2020; An et al., 2021; Georgopoulou et al., 2021). Therefore, to turn quantitative behavioral analysis into practical OWI, technological advances on machine vision, biotelemetry and miniaturized bio-loggers need to be applied and adapted to the demand of fish welfare monitoring. Thus, as reviewed by Macaulay et al. (2021), electronic transmitter tags (e.g., acoustic accelerometers) were first developed for studying wild fish behavior, but they are now rapidly transitioned into the world of farmed fish for an individual-based behavioral assessment, acting tagged individuals as “sentinel fish” to provide useful welfare-related information for monitoring and managing feeding, growth and disease outbreaks (Kolarevic et al., 2016; Føre et al., 2017, 2018; Warren-Myers et al., 2021). In any case, the proposed monitoring solutions should be different depending on the asked question, the species biology, and the culture system (tank-based systems, ponds, lagoons, sea cages, etc.).
A primary assumption of “smart farming” is that the behavior of tagged individuals is representative of that of untagged individuals, but tagging can be a stressful experience causing altered behavior and increased mortality (Jepsen et al., 2015; Newton et al., 2016; Vollset et al., 2020; Macaulay et al., 2021. Such negative effects should be mitigated as transmitter tags evolve and become more miniaturized. Meanwhile, the smaller size of Data Storage Tags (DST) (working in standalone mode) makes it easier to monitor small fish, while minimizing the negative impact of tagging protocols for a reliable underscore of welfare in fish exposed to different aquaculture stressors (Rosell-Moll et al., 2021). However, the retrieval of DST devices for data download remains a major constraint (especially in the case of sea-cage aquaculture). Besides, tagging procedures should be refined and improved using species-specific approaches for minimizing not only animal discomfort and data skew, but also the success of tag recovery for the download of recorded and/or pre-processed data by on-board algorithms. Otherwise, energy harvesting, energy storage, micro-power management of DST tags and their system integration need to be addressed in depth to define the best trade-off between battery power life and the accuracy of data recording, processing and transmitting. Each sensing system has thereby different advantages and disadvantages, and the aim of this review is to revisit the use of DST devices as an alternative and some times more accurate solution for progressing toward the use of IoT in aquaculture by both academic and industry researchers. Attention was focused on accelerometer technology with special emphasis on the DST prototype (AEFishBIT), designed and validated within the framework of AQUAEXCEL2020 H2020 EU project for the individual and simultaneous monitoring of physical activity and gill ventilation rates (two in one). AEFishBIT battery is rechargeable and it can be re-used several times with reprogrammable data sampling schedules. This device has been tested in European sea bass and more extensively in gilthead sea bream under a wide range of experimental conditions (Martos-Sitcha et al., 2019b; Ferrer et al., 2020; Rosell-Moll et al., 2021). It has thereby a Technology Readiness Level (TLR) higher than 7, and current studies in rainbow trout and Atlantic salmon have proven its potential in other farmed fish using species-specific procedures for the device operculum tagging (see below). We discuss here how further research and technological improvements in fish sensing can contribute to associate different activity and behavior patterns with better performance or differences in stress and disease resilience, emphasizing the importance of the better understanding of the species and genotype-by-environment interactions for the improvement of fish health and welfare in the framework of AQUAEXCEL3.0 EU project.
Data Recording and On-Board Algorithm Data Processing
The assessment of fish welfare through behavior and movement analyses requires a precise and accurate representation of movements. This is initiated by neuronal electrical impulses, causing muscle contractions that produce a mechanical impulse or jerk. In this way, the wave-like swimming activity is an overlap of jerks caused by the repetitive electrical stimulation of the muscles by the neurons. This sequence of jerks causes a bell-shaped speed representation with a period similar to the period of the electrical stimulation. A proper sampling frequency of these bell-shaped changes in speed is essential for an accurate description of fish movements. Most devices in the market rely on a low sampling frequency and average the accelerometer data over a sampling window of usually 20–40 s. This average corresponds to a low pass filtering of the absolute acceleration values, and they are adequate to represent changes in the tendency of swimming speed, as variation from steady to unsteady swimming will result in differences in the averaged data. Low sampling frequencies are also convenient for a real-time transmission of the averaged accelerations. Thus, the use of low frequency sampling devices is adequate for a continuous fish monitoring in sea cages of unsteady movements and to transmit low bit rate data in real time via acoustic communication channels, though they are less informative about other subtler events that can be of importance for the assessment of the overall welfare status (Murchie et al., 2011; Wilson et al., 2013; Arechavala-Lopez et al., 2021). Indeed, the capture of sustained movement with an accelerometer requires not only an adequate tag implantation location (Alfonso et al., 2021), but also high sampling rates that have been usually established to be higher than 30 Hz (Broell et al., 2013). Higher sampling frequencies are free of any aliasing distortion (Chen and Bassett, 2005) and allow to work out the fish velocity by integration of the acceleration and the real distance covered by the fish by integration of the velocity. Similarly, the time derivation of the acceleration will be the jerk and the jerk derivation the snap of the fish. These principles were taken into account for the design and use of the low power AEFishBIT device, a tri-axial accelerometer intended to be attached to the operculum of small fish for data recording at a sampling frequency of 100 and 50 Hz (Martos-Sitcha et al., 2019b; Ferrer et al., 2020) for raw data and on-board data processing, respectively.
Briefly, the recorded z-axis acceleration from AEFishBIT device generates positive and negative signals, related to the operculum opening and closing that serves as a direct measure of respiratory frequency. This signal was band pass filtered between 0.5 and 8 Hz to reduce the noise, to attenuate the influence of other movements and to highlight the periodic properties of the signal. Afterward, the number of crosses through zero was divided by two to obtain the signal period, which was then averaged over several frames for 2 min to reduce the bias and estimate respiratory frequency. Similarly, description of physical activity was based on the simple optimization principle that human and livestock try to perform maximally smooth movements by minimizing the first temporal derivative of acceleration, while constraining all higher derivatives to zero (Flash and Hogan, 1985; Hamäläinen et al., 2011). Thus, x- and y-axis accelerations were derived to obtain the jerk, being considered their averaged energy over 2 min a representative indicator of physical activity. Software development also offers the possibility to process the recorded data by on-board algorithms (no memory consuming process), increasing the autonomy of the system up to 6 h of continuous data recording with different short-time programmable schedules, routinely reduced to 2–4 days with 2 min measures each 15–30 min windows. This dual recording system (activity and gill breathing) has been validated in a swim tunnel respirometer, which yielded strong correlations of AEFishBIT outputs with data of forced swimming speed and O2 consumption in juveniles of European sea bass and gilthead sea bream (Martos-Sitcha et al., 2019b). As part of the validation procedure, fish species differences (gilthead sea bream vs. European sea bass) in the amplitude and frequency of operculum and body tail movements were also evidenced using recorded raw data from exercised fish, which highlighted the anatomical and metabolic adaptive features of European sea bass as a fast swimming predator (Ferrer et al., 2020). Additionally, in Atlantic salmon, good correlations between AEFishBIT and video recording data have been provided by Kolarevic et al. (2021), providing additional evidence for a reliable use of AEFishBIT as a smart tool for sensing farmed fish of high economic value for aquaculture. The tracking of these validation/calibration procedures is summarized in Figure 1. Of note, the device is not initially intended for extended recording periods, though future improvements in AEFishBIT miniaturization, energy harvest and battery auto-recharge will result in a smaller and lighter device that will facilitate fish sensing from earlier life stages and extended periods of time.
Tagging Methods: How and Where
As pointed out in previous reviews (Lucas and Baras, 2000; Cooke et al., 2013; Jepsen et al., 2015), there are three main methods for attaching electronic tags to fish: surgical implantation into the body cavity, external attachment, and gastric insertion via the mouth. Each method offers different advantages and disadvantages that need to be considered in each particular case. Surgical implantation of the tag does not attract predators and it is ideal for long-term studies, but it requires longer recovery times and generally more practice and skills than other tagging methods. Alternatively, gastric insertion can be a less invasive and quick tagging method, though it is mainly suitable at life stages with growth and feed cessation, and thereby of limited interest in fish farming where a continuous feeding at different rates mostly occurs through all the productive cycle. Conversely, the most commonly reported problems with external tags are tissue damage, tag loss and decreased swimming capacity, but external tagging has the advantage to be used rapidly after tagging or in fish with a body shape not suitable for surgical implantation due to gonadal development or lateral or dorso-ventral compression (flat fish). Otherwise, external tagging is especially useful if sensors are used to record not only external variables (i.e., O2, temperature, light intensity), but also some physiological features such as gill breathing. In particular, the dual recording of AEFishBIT (physical activity and respiratory frequency) requires it to be externally attached to fish operculum, minimizing as much as possible tissue damage, and physiological and behavioral disturbances to ensure that tagged animals are representative of the untagged population in challenging farming conditions and that the tagging procedure does not impact the reliability of the recorded data (Macaulay et al., 2021). In that sense, the AEFishBIT device benefits of a small size and weight (15 × 6 × 6 mm, 1.1 g in air), though the tagging method needs to be adapted to each species to make it a reliable tool for smart sensing farmed fish.
In gilthead sea bream and European sea bass, the AEFishBIT attachment was initially attained using laboratory tags for identification of experimentation animals (RapID tags), which were rapidly pierced to operculum and on which a polyamide pocket was fixed in juvenile fish of 50–100 g onward (Martos-Sitcha et al., 2019b). This attachment procedure did not alter significantly plasma levels of cortisol, glucose and lactate after 1 week of tagging. However, after 10 days, the appearance of macroscopic indications of damage and necrosis at the piercing location were evident in a high percentage of individuals reared in a tank-based system. This undesirable effect was solved by using corrosion-resistant self-piercing fish tags with a flexible heat shrink polyethylene tube to fit the device, which avoided growth impairment or pathological signs of hemorrhage or tissue damage after 1–3 weeks of tagging (Rosell-Moll et al., 2021). Such approach also works well in flat fish (turbot, sole), but it is not feasible in rainbow trout or Atlantic salmon, probably due to differences in head shape and operculum ossification (Huby and Parmentier, 2019). Indeed, following this tagging protocol, significant tissue damage and necrosis were observed few days post-tagging in both trout and salmon at the level of the piercing location and also on the gill tissue located below. Thus, alternative surgery approaches were developed for trout and salmon (400–500 g onward), using a flexible heat shrink polyethylene tube ring sutured on the operculum and with AEFishBIT held in this ring. This tagging method led to 100% retention rate (7 days post-tagging) with no significant tissue lesions or gill lamellae necrosis in salmon of 400–600 g body weight (Kolarevic et al., 2021). This procedure still provoked some tissue damages in trout, though it can be prevented by means of a suture step at operculum 2 weeks before the implantation of the heat shrink polyethylene ring with the AEFishBIT device. The success of the different tagging protocols was evidenced by a fast achievement of a steady state for the AEFishBIT measurements of physical activity, which was found within 2.5–3 h post-tagging in gilthead sea bream and trout, whereas 7 h were required for salmon with a lasting surgery tagging procedure in a single step (Figure 2). In any case, the tagging recovery after AEFishBIT implantation was fast in all species, which makes feasible to start data recording few hours after the completion of tagging procedure.
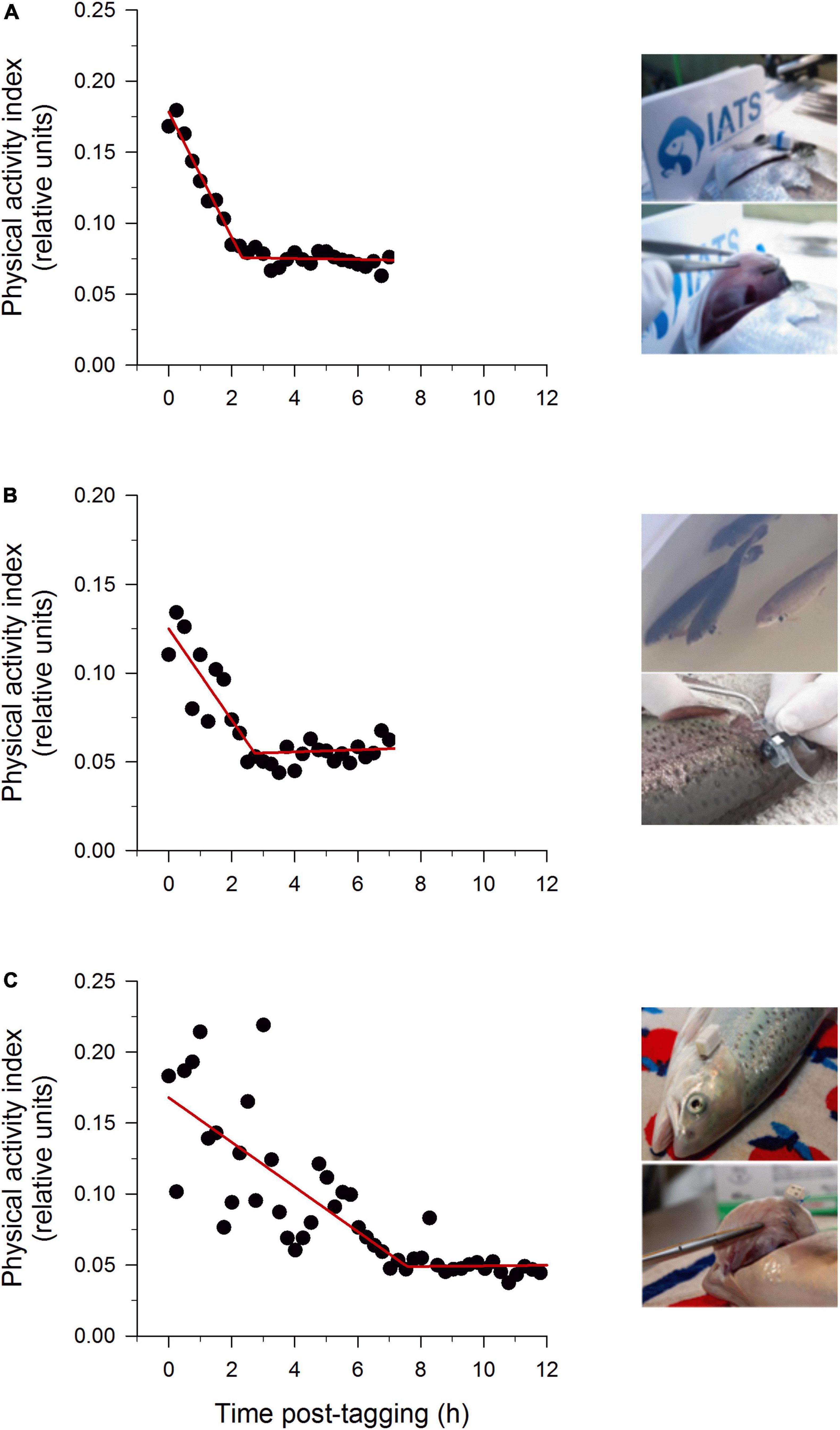
Figure 2. AEFishBIT records of physical activity of a representative individual, (A) gilthead sea bream; (B) rainbow trout; (C) Atlantic salmon) after tagging and return to the tank. Measures (black circles) were taken every 15 min. Lines represent piecewise linear fit of data with one break point. Inserts show the operculum attachment in 200–300 g gilthead sea bream (monel tags with a flexible heat shrink polyethylene tube), 550–650 rainbow trout (heat shrink polyethylene tube ring 2 weeks after suture) and 700–800 g Atlantic salmon (suture and heat shrink polyethylene tube ring in a single step). Partially adapted from data of Kolarevic et al. (2021) and Rosell-Moll et al. (2021).
Evaluating How is Fish Sensing and Responding
Problems and Pitfalls in Spatial Cognition and Physical Swimming Activity
Natural stressors tend to be brief and/or avoidable, while stressors in captivity are often prolonged or repetitive, activating in a repeated manner physiological stress responses detrimental to the animal (Fraser and Duncan, 1998; Veissier and Boissy, 2007). This becomes especially evident in the case of random aquaculture stressors (Bermejo-Nogales et al., 2014), showing transcriptomic meta-analysis that mitochondria are among the first responders to environmental and nutritional stress stimuli in fish (Calduch-Giner et al., 2014; Healy et al., 2017; Sokolova, 2018). Particularly in gilthead sea bream, several mitochondrial markers with a role in oxidative phosphorylation, antioxidant defense and respiration uncoupling have been used as reference values of well-being to monitor and mitigate the negative impacts of captive rearing (Pérez-Sánchez et al., 2011; Bermejo-Nogales et al., 2015; Magnoni et al., 2017; Martos-Sitcha et al., 2017, 2019a). However, this interest has now expanded into recognizing the importance of positive effects of environmental enrichment (EE) as a promising tool to guarantee or improve the welfare of laboratory and farmed fish (Brydges and Braithwaite, 2009; Evans et al., 2015; Stevens et al., 2021). Such approach provides the opportunity to experience new situations typical of the species in the wild, and it is generally accepted that well-designed EE (e.g., stones, roots, logs, plants, algae, sand, sessile animals, ice, artificial objects, etc.) improves behavioral flexibility and cognitive ability of fish by changes in physical activity. Indeed, modifications in swimming behavior reflect how a fish is sensing and responding to its environment, which is reflected by changes in spatial distribution and ventilation activity to ensure the supply of O2 at the exact rate required by the organism (Martins et al., 2012; Zupa et al., 2015; Arechavala-Lopez et al., 2021). Thus, video recording analysis highlighted that physical EE promoted the increase of antioxidant enzyme activity, exploratory behavior and learning abilities of gilthead sea bream juveniles reared in tank-based systems (Arechavala-Lopez et al., 2020). Likewise, the provision of EE in sea cages promoted the overall improvement of gilthead sea bream welfare, reducing aggressiveness and interactions with the net, which might serve to reduce the incidence of fish escapes (Arechavala-Lopez et al., 2019; Muñoz et al., 2020). However, data records from independent studies are often poorly consistent and it remains difficult to establish a general pattern of space occupancy and physical swimming activity as valuable insights in the behavior, but also in the physiological well-being and resilience of farmed fish around the world and Mediterranean fish in particular.
The above findings reinforce the need of further research addressing the type of body movements that are recorded from the externally or internally implanted fish sensors. Certainly, Palstra et al. (2021) pointed out that gilthead sea bream accelerations from acoustic transmitter tags (ventrally implanted) are a good proxy of unsteady swimming activity (e.g., escape reactions and aggressive or foraging behavior) in experimental sea cages, but not of sustained swimming exercise. This assumption is based on the correlations between accelerations and the weight of heart and mesenteric fat weight, which were the opposite to that found and expected in exercised training fish (Blasco et al., 2015; Rodnick and Planas, 2016; Palstra et al., 2020). Indeed, most commercially available acoustic accelerometers work at low data recording frequency, and they mainly record deviations from sustained activity in swim-tunnel exercised fish at different flow speeds (Arechavala-Lopez et al., 2021). In contrast, as indicated before, the inferred physical activity from on-board AEFishBIT outputs in exercised juveniles of gilthead sea bream and European sea bass is able to characterize the whole jerk produced by the muscular twitching that impulse the fish and reconstruct the bell-shaped acceleration of the muscular jerk. High sampling frequency also allows detecting consistent differences in sustained swimming at various speeds (1–6 body length/s) (Martos-Sitcha et al., 2019b). Moreover, in depth analysis of raw data from exercised fish highlighted that the large amplitude of operculum aperture and body tail movements of European sea bass is part of the adaptive features of this fish species as a fast swimming predator (Ferrer et al., 2020), linked to the natural evolution and behavioral ecology in a predator-prey system (Berger, 2010; de Matos Dias et al., 2018). Besides, selection for growth within the PROGENSA® gilthead sea bream breeding program modified behavioral and physical activity traits, priming growth in genetically fast-growing fish and swimming performance in genetically slow-growing fish (Perera et al., 2021a). This was supported by the improved resistance to fatigue in swim tunnels, but also by the more active swimming behavior of free-swimming fish assessed by AEFishBIT measurements of grouped-housed fish in rearing tanks. Likewise, AEFishBIT outputs revealed a more continuous physical swimming activity of younger fish when comparisons were made between gilthead sea bream individuals of class of age + 1 and class of age + 3 (Rosell-Moll et al., 2021). Older fish also showed a significant reduction of operculum beats, supporting a reduction of metabolic rates with the age-mediated decrease in growth rates. Also in this study, jerk accelerations of fish allocated in 90 L, 500 L and 3,000 L tanks increased with the decrease of available space as a result of erratic and chaotic trajectories, which again indicates that AEFishBIT outputs of swimming activity are a good proxy for both unsteady and sustained swimming activity. Indeed, monitoring of physical activity is becoming a useful tool for meeting the behavioral needs (e.g., optimal space availability regardless of density), or time of adaptation of farmed fish for reducing stress, aggressiveness and anxiety after stressful aquaculture operations (Stevens et al., 2021).
Adaptive Capacities and Robustness
In a time of rapid environmental changes, with increases in ocean temperatures and in the frequency and severity of hypoxic events, serious concerns are arising about the profitability of some aquaculture production systems (Brander, 2007; Gamperl et al., 2020; Burke et al., 2021). Therefore, the better understanding of the mechanisms operating in fish to mitigate heat stress in combination with mild hypoxia is becoming a challenge. Recently, important advances have been made in this field, and a number of genes have been identified in Atlantic salmon as molecular biomarkers for the quantification of hypoxia, but also for the detection of epigenetic markers that can predict thermotolerance (Beemelmanns et al., 2021). There is also now evidence in zebrafish that paternal hypoxia exposure primes offspring for increased hypoxia resistance (Ragsdale et al., 2020). As a general pattern, these hypoxic adaptive features involve the induction of hypo-metabolic states, the increased O2-mitochondria affinity, and the aerobic/anaerobic switches in substrate preference as metabolic fuels according to the metabolic capabilities of each tissue (Hoppeler and Vogt, 2001; Murray, 2009; Gamboa and Andrade, 2012; Martos-Sitcha et al., 2017, 2019a). However, in gilthead sea bream juveniles, this process is more complex than initially envisaged. Thus, the resetting of the skeletal muscle transcriptome by mild hypoxia condition first primed an increased contribution of lipid metabolism to the whole energy supply to preserve the aerobic energy production, and thereafter (with the restoration of normoxia) a reduced protein turnover and improved anaerobic fitness (Naya-Català et al., 2021b). How fish physiologists, farmers and breeders can fully exploit this metabolic plasticity remains open, though it becomes clear that it can serve to preserve an efficient nutrient utilization but also the swimming performance under predictable hypoxic stress episodes.
It is also known that the different growing within gilthead sea bream families from the PROGENSA® breeding program are associated with differences in resistance to fatigue and probably with different aerobic/anaerobic fitness to cope with a low O2 availability (Perera et al., 2021b). These traits are co-selected with a growth pattern that promoted growth spurts in summer rather than a more continuous growth across season (Perera et al., 2019). However, the genetic variability of current farmed gilthead sea bream populations remains high enough and individual tracking of physical activity patterns with AEFishBIT served to differentiate active and inactive behaviors after exposure to acute hypoxia (Rosell-Moll et al., 2021). Active individuals typically show dynamic avoidance in response to challenges and are more aggressive and socially dominant. On the contrary, inactive (or passive) individuals generally respond to challenges with caution and immobility, being socially subordinate, and generally more flexible in their behavioral responses to environmental change (Koolhaas et al., 1999; Korte et al., 2005; Huntingford et al., 2010). AEFishBIT determined that all hypoxic fish increased their ventilation rates almost equally in our challenge, but jerk accelerations highlighted the enhanced physical activity of a subset of fish that will support their escape reactions (active fish) during hypoxia episodes.
Robustness, defined as the ability to combine high production potential with resilience to stressors, becomes a key trait for selection in aquaculture (Knap, 2005). Physiological and behavioral resistance and resilience to environmental challenges are thereby considered a robustness feature. Robustness typology has been studied in two divergent isogenic lines of trout, namely A22 and R23, with a different ability to cope with plant-based diets (Geurden et al., 2013). These fish are also a good model for studying differences in hypothalamus-pituitary-interrenal (HPI) reactivity, as an alternative to the previously studied high and low cortisol responsive fish strains of trout (Pottinger and Carrick, 1999; Øverli et al., 2005). Indeed, animals of line R23 were described as less aggressive and more robust fish showing a low cortisol response to crowding stress in comparison to reactive individuals (line A22) that showed a strong corticosteroid response (Sadoul et al., 2015). Nevertheless, comparisons between different environmental stressors pointed out that animals can modulate their responses depending on the type of stressor. Thus, line R23 showed a higher sensitivity than A22 to an acute CO2 (hypercapnia) challenge (Sadoul et al., 2017). These divergences in adaptive capabilities are further corroborated by AEFishBIT measurements in hypoxic exposed fish. In fact, the two trout isogenic lines highlighted an increased physical activity during hypoxia, and this response was more persistent in reactive A22 fish (Figures 3A,B). Regarding respiratory frequency, it was noticeable that R23 fish displayed a lower basal activity than A22, and this feature could facilitate an enhanced respiration responsiveness in fish facing a hypoxic challenge, whereas respiratory frequency of challenged A22 fish remained closer to normoxia values prior the challenge (Figures 3C,D). These data illustrate the different behavioral and physiological strategies developed by the two isogenic trout lines when exposed to acute hypoxia stress and confirm the notion that differences in basal behavior determined with AEFishBIT help to establish different fish adaptive capabilities. Indeed, comparison of fish species differences in hypoxia tolerance and responsiveness highlighted that, contrarily to gilthead sea bream, the primary response to hypoxia in trout is the increase of locomotor activity rather than ventilation rates. This would reflect the prioritization of the escape reactions in a fish species with a well-known limited tolerance to a hypoxic environment (Dunn and Hochachka, 1986; Hou et al., 2020). Altogether, AEFishBIT can serve to determine differences in basal behavior and how robust are the current domesticated/selected farmed fish when they are facing perturbations associated to common culture practice.
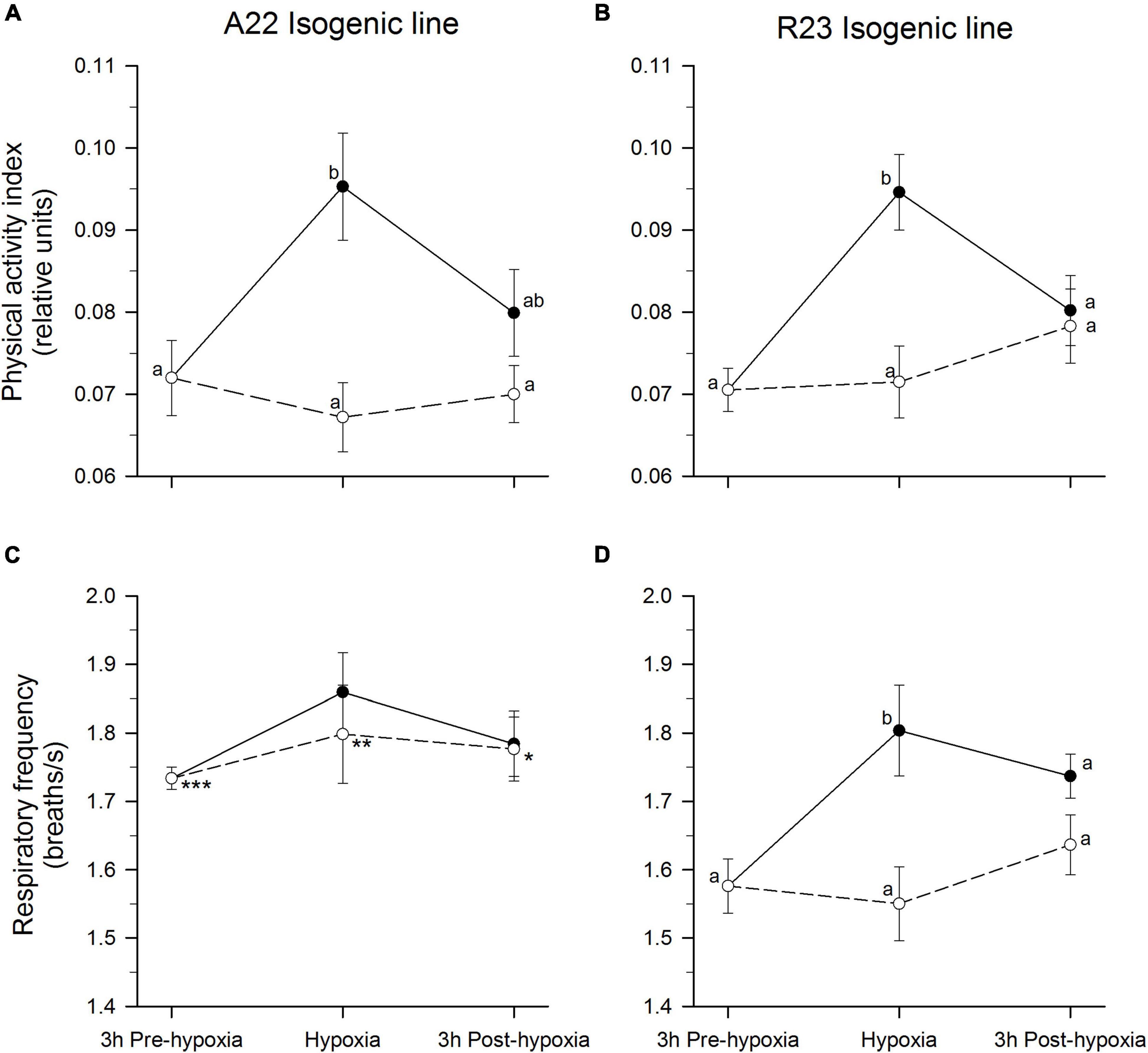
Figure 3. AEFishBIT records of physical activity (A,B) and respiratory frequency (C,D) of rainbow trout isogenic lines A22 (A,C) and R23 (B,D) exposed to a 3 h hypoxia (from 8.0–9.0 ppm to 2.8–3.0 O2 ppm) challenge. For each individual (598 ± 10 g body weight), measures taken every 15 min are averaged at each time point (3 h pre-challenge, 3 h hypoxia, 3 h post-challenge). Black circles are the values of hypoxia-challenged fish, whereas white circles are those of unchallenged fish. Values are expressed as mean ± SEM (n = 10 for hypoxia and post-challenge groups, n = 20 for pre-challenge fish). Different letters represent significant (P < 0.05, ANOVA, SNK test) differences among challenge time points. Asterisks represent significant (*P < 0.05; **P < 0.01; ***P < 0.001, t-test) between trout lines at the same time point and challenge.
Daily Patterns of Diurnal/Nocturnal Behavior
Feeding in wild life takes place periodically during a certain temporal niche (e.g., day-time or night-time), depending on whether the species is diurnal or nocturnal. This timing system is comprised of a network of endogenous circadian clocks that generate, via their local or distributed outputs, an internal rhythmicity close to 24 h (Challet, 2013). Hence, when food access is limited to a few hours every day at the same time (temporal restricted feeding), most organisms display a food-anticipatory activity, accompanied of food-appetitive behaviors and hormonal responses prior to the expected food presentation (Bloch et al., 2013; Skvortsova et al., 2021). Moreover, disruption of the circadian cycle is strongly associated with metabolic imbalances in rodent models of circadian arrhythmia (Eckel-Mahan and Sassone-Corsi, 2013). Indeed, the pervasiveness of circadian rhythms in life suggests that circadian clocks are adaptive in nature, functioning as a timing reference that allow organisms to anticipate the diel fluctuations in their environments, being also the basis for the transduction of seasonality from the changing photoperiod (Van der Zee et al., 2008). Certainly, it is becoming apparent from metabolites to transcription factors that circadian clock interacts with metabolism in numerous ways that are essential in a multitude of processes related to cellular metabolism, stress, and senescence (Tevy et al., 2013; Majeed et al., 2020), which can operate in the absence of the transcriptional/translational circadian clockwork as reported for instance for ubiquitous antioxidant enzymes (e.g., peroxiredoxins) and NAD+-dependent histone deacetylases (sirtuins). Overall, it opens discussions about the etiological mechanisms regulating food anticipatory responses and metabolic disorders arising from the loss of circadian rhythms. In this regard, early studies in Atlantic salmon highlighted that acute stress can be measured in terms of changes to feeding motivation, becoming this criterion a more sensitive indicator of stress than measurements of changes in cortisol excretion (Folkedal et al., 2012). Certainly, locomotor and feeding rhythms are of endogenous origin, as they can persist under constant conditions without external time cues (Madrid et al., 2001; Sánchez-Vázquez and Madrid, 2001). However, it is also known that vertebrate animals in polar regions show highly diverse activity patterns ranging from entrained circadian rhythms to arrhythmicity patterns (Cockrem, 1990; Ashley et al., 2012), as reported for AEFishBIT measurements of locomotor activity and respiratory rates in Atlantic salmon under continuous light regimes and hourly access to food excess (Kolarevic et al., 2021).
The above findings contrast with the observations made in most other fish studies, showing daily rhythms in locomotor and feeding behavior that can be diurnal or nocturnal depending of the species biology (Madrid et al., 2001; Reebs, 2002). In addition, some fish display a clear seasonal change of diurnal/nocturnal behavior, having European sea bass a nocturnal feeding behavior in winter that is changing to be diurnal in summer and autumn (Azzaydi et al., 2007). Otherwise, using self-feeding system specially designed for small fish, del Pozo et al. (2011) pointed out an independent phasing between locomotor and feeding activities in zebrafish, which were mostly nocturnal or diurnal, respectively. This dualism has also been reported in gilthead sea bream, where the scheduled-feeding drove the self-feeding pattern (López-Olmeda et al., 2009), as it became diurnal or nocturnal under day- or night-feeding, respectively. In contrast, in the same study, the locomotor activity (measured by means of infrared photocells) was little affected by feeding time, and remained mostly diurnal with a photoperiod set at 12 h light: 12 h dark. However, AEFishBIT outputs evidenced the high ability of gilthead sea bream to concentrate self-feeding activity around the programmed meals in fish taught to different temporal restricted feeding schedules (one single meal at 9 or 12 h; hourly feeding times from 9 to 12 h) (Figure 4). In addition, feeding would serve to synchronize the phase of locomotor and metabolic rhythms (respiratory rates), which are not necessarily in the same phase in adults of European sea bass feeding at midday (Ferrer et al., 2020). How the synchronization of feeding, locomotor and metabolic rhythms can contribute to improve aquaculture profit is under debate, but the methodological advances in fish monitoring in a tank-based system can also be extrapolated to the field. For instance, gilthead sea bream in winter becomes mostly arrhythmic with feeding schedules and physiological rhythms of physical activity and respiratory rates out of phase. However, current studies highlight that the use of feeding schedules with alternating days (maintaining constant the total food intake, but with an increased feed supply the feeding day), served to restore the typical feeding behavior of summer with an enhanced activity around the feeding time (Figure 5A). By contrast, this daily synchronization is more difficult in European sea bass than in gilthead sea bream, as feeding level by itself does not act as a main synchronizer in actively feeding fish. Indeed, 2-year old European sea bass, taught to feed at a fixed time at 12:00 h under natural photoperiod and temperature conditions displayed (84% of population) a nocturnal behavior with a peak of activity at 5:01 h (22:31 ZT) during late spring, but only 19% of population remained nocturnal at midsummer with feeding levels of the same order of magnitude (1.0–1.1% body weight) (Figure 5B). It is likely that the predator nature of this species drives it to shift its activity behavior according to the longer phase (nocturnal/diurnal) of photoperiod. In any case, how these behavioral features can be used as a suitable indicator of fish welfare and/or domestication success of farmed fish under intensive aquaculture production requires further research.
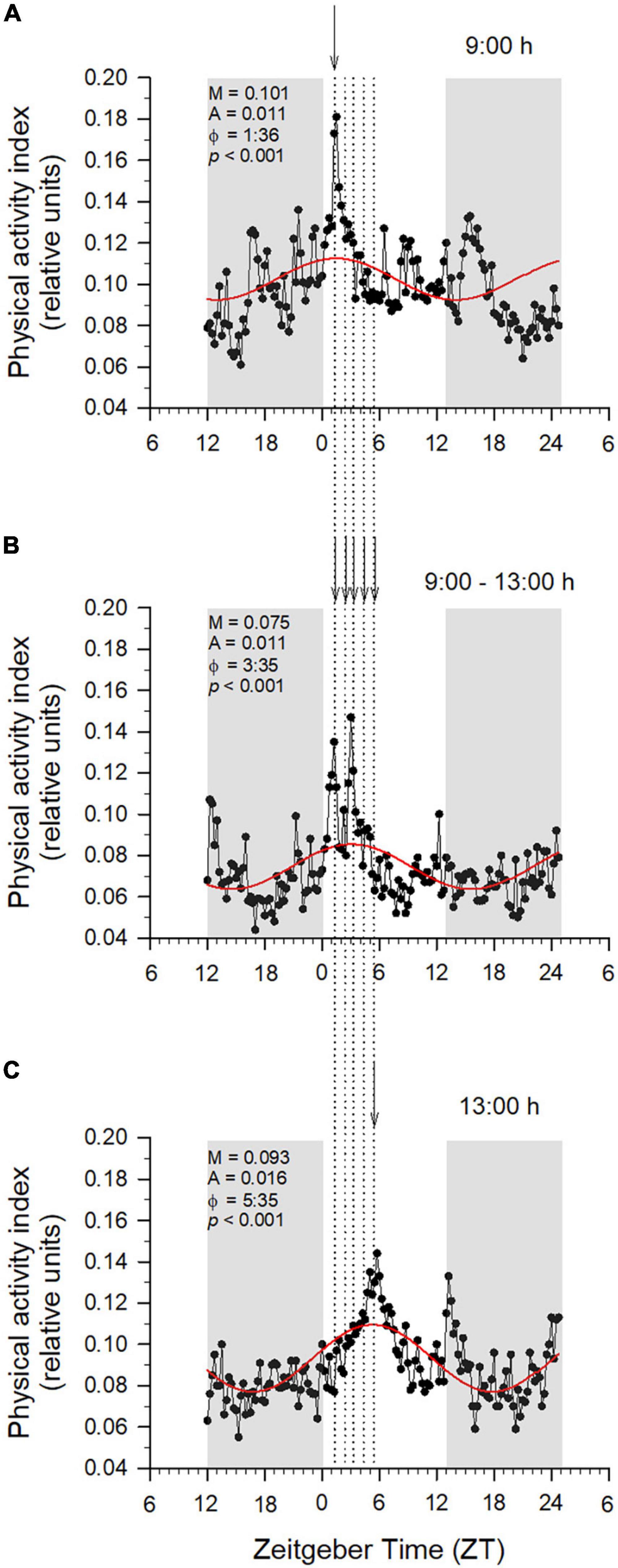
Figure 4. Gilthead sea bream (mean body weight 305 g) activity synchronization by feeding with different schedules: 9:00 h (A), once per hour between 9:00 and 13:00 h (B), and at 13:00 h (C). In each panel, the best-fit curve derived from cosinor analysis of physical activity AEFishBIT data (measures taken every 15 min along 2 days) of representative individuals is shown as a continuous solid line. Values of mesor (M), amplitude (A), acrophase (ϕ), and p-value (P) of best-fit curves are shown. Gray shaded areas represent dark phases. Arrows in vertical dotted lines indicates the mealtimes.
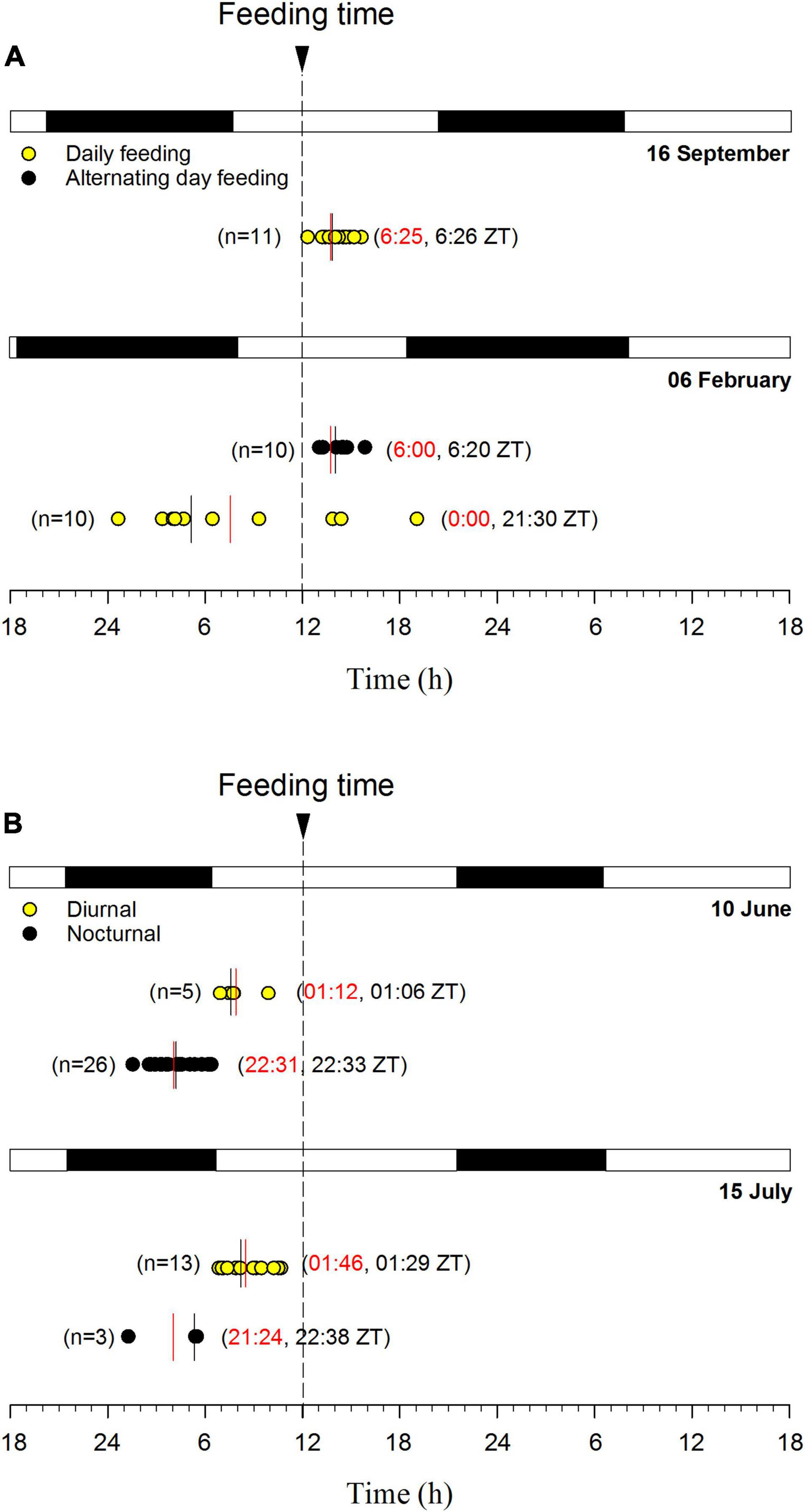
Figure 5. Seasonal activity synchronization by feeding in gilthead sea bream (A) and European sea bass (B). Circles represent the activity acrophase of the best-fit curve derived from cosinor analysis of physical activity AEFishBIT data (measures taken every 15 min along 2 days) of each individual. At each month, light and dark phases (natural photoperiod) are represented, as well as the feeding time. For gilthead sea bream, fish fed daily (mean body weight 225 g in September, 390 g in February) are represented by red circles, and fish fed during alternate days (mean body weight 385 g) are represented by black circles. For European sea bass fed daily, individuals with diurnal (yellow circles, mean body weight 295 g in June and 345 g in July) and nocturnal (black circles, mean body weight 250 g in June and 255 g in July) behavior are represented. For each group, green bar marks the mean acrophase, and black bar the median. The number of individuals is stated for each group.
Energy Partitioning for Growth and Locomotor Activity
How genetics and environment affect individual size variation and growth compensatory processes in fish with different heritable growth also evolves as a valuable criterion to evaluate and improve growth, production and welfare under intensive fish farming. Certainly, gilthead sea bream families characterized as fast, intermediate and slow growing within the PROGENSA® breeding program had differences in growth trajectories, size heterogeneity, swimming capabilities and gut microbiota composition across the production cycle (Perera et al., 2019, 2021a,2021b; Piazzon et al., 2020). This is also susceptible of an effective environmental programing that can produce different growth and survival phenotypes depending for instance on the time window of hypoxia episodes. Hence, early mild hypoxia exposure (60–81 dph, 3.6–3.8 ppm) did not compromise growth compensation processes driving a reduced size heterogeneity during early life juvenile stages (Perera et al., 2021b). However, growth compensation becomes constrained with recurrent or late hypoxia episodes in life, which impaired the capacity to reduce size variation during the dominant phase of muscle growth hyperplasia. Such considerations are especially relevant to discriminate the main source of variation in growth rates along time or between different fish families, helping to avoid the use of confounding criteria for improving fish management and aquaculture profitability (Power et al., 2011). Indeed, selective breeding would lead the selection of animals that are not necessarily the fastest growers, but that have a positive effect on the group as a whole (Devlin et al., 2004; de Goede et al., 2013). In this regard, it must be noted that measures of critical swimming speed (defined as the speed that can theoretically be maintained without exhaustion) in swim tunnel tests negatively correlated with growth in gilthead sea bream (Perera et al., 2021a). Likewise, AEFishBIT outputs of group-housed fish highlighted changes in swimming behavior, priming fast growth rather than swimming performance, as reported for highly domesticated strains of salmon and trout that becomes athletically less robust than wild fish (Anttila and Mänttäri, 2009; Bellinger et al., 2014; Zhang et al., 2016). This reveals a complex trade-off that would prime growth in genetically fast-growing fish, or swimming performance (and maybe escape reactions) in genetically slow-growing fish, resulting in a lower capacity of larger fish to cope with the increased water temperature and reduced O2 availability around the entire world due to global warming. How current farmed fish have evolved for adapting to global climate change is thereby a key issue, and welfare indicators informing, directly or indirectly, of this phenotypic plasticity are of high applicability in aquaculture for fish farmers and breeders.
The general thinking is that there is a positive relationship between metabolism and food availability (Reid et al., 2011). However, this relationship can also vary among studies and species (Auer et al., 2015; Liu and Fu, 2017), showing different types of rearrangements between growth and metabolism. For instance, in a common garden system with all fish under identical environmental conditions in the same experimental tank, respiratory rates are negatively or positively correlated with body mass in gilthead sea bream juveniles having slow- and fast-growing phenotypes, respectively (Perera et al., 2021a). This feature highlighted a higher contribution of energy to growth in fast growing fish, which was also evidenced when AEFishBIT comparisons were made between salmon and gilthead sea bream (Kolarevic et al., 2021). Likewise, measurements of respiratory rates in gilthead sea bream varied with growth changes across season and development, while changes in locomotor activity appeared less variable (Figure 6A), a feature that reinforces the concept of AEFishBIT respiratory frequency as an indirect measure of basal metabolism and hence growth potentiality among fish species and studies. In other words, the ratio between respiratory frequency and physical activity values can be considered as a metabolic index whose variations can be indicative of differences in the energy partitioning. Indeed, the variations of this metabolic index have been proven useful to assess the adaptation of salmon to different light regimes (Figure 6B; Kolarevic et al., 2021). How this knowledge can be used to define new algorithms based on the combination of physical activity and respiratory frequency as integrative welfare indicators is underway to better dissociate the growth changes related to development, natural environmental cues, and aquaculture stressors, with an impact among other factors on the optimization of water quality and available space for the interaction of individuals with the environment and their congeners.
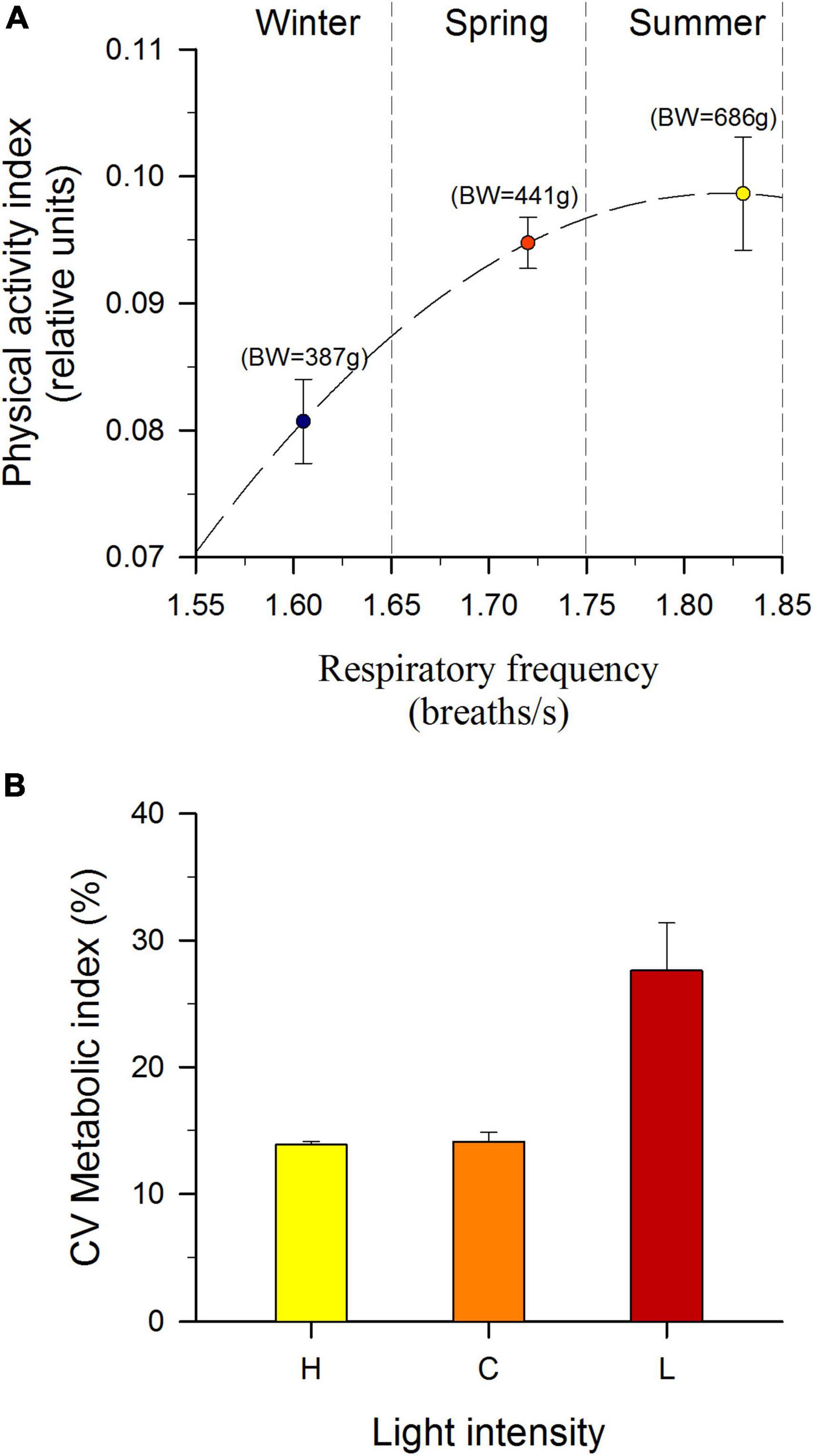
Figure 6. (A) Seasonal correlation plot between physical activity index and respiratory frequency in cultured gilthead sea bream (measures taken every 15 min along 2 days). Each value represents the mean mesor of 10–12 individuals (B) Coefficient of variation (CV) of the metabolic index (respiratory frequency/physical activity) of Atlantic salmon exposed to different light intensities: H (High, yellow bar, 18.90 μmol.m–2.s–1), C (Control, orange bar, 6.83 μmol.m–2.s–1) and L (Low, red bar, 0.28 μmol.m–2.s–1). Each value is the mean ± SEM of the daily coefficient of variation of 6–7 individuals for 3 days. Different letters indicate significant differences (P < 0.01, ANOVA). Partially adapted from data of Kolarevic et al. (2021).
Concluding Remarks and Future Prospects
AEFishBIT provides reproducible and accurate measurements of behavior and welfare status of farmed fish in tank-based systems. This is different of what we expect of electronic transmitter tags that are especially suitable for the real-time monitoring of unsteady swimming activity, associated for instance to feeding behavior or escape reactions in sea cages. Otherwise, the use of DST allows monitoring of small fish, which is of special relevance for farmed species with a harvest body weight of 500–800 g. This is the reason why the miniaturized AEFishBIT device was initially tested and validated on Mediterranean farmed fish rather than in salmonids with a harvest weight at commercialization too much higher than gilthead sea bream or European sea bass. In any case, a critical step in fish biosensing is the tagging procedure, being the adopted solution dependent on anatomical features, developmental stage, rearing system, duration of study, and more importantly on the research question. Altogether, these factors limit the current threshold size for fish AEFishBIT monitoring, which is ranging between 50 and 100 g in gilthead sea bream and European sea bass, and 400–500 g for salmonids. Future improvements in device design and miniaturization are envisaged at short-term to expand species and recording period through the production cycle. A major constraint factor for this achievement is the size and weight of the battery, whose life could be extended using different energy harvesting approaches that are under evaluation within the EnABLES H2020 EU project. The ultimate goal is to take advantage of fish movement to adopt self-powered technologies that would remove the large battery in the device design. This zero power approach would further minimize the impact of device attachment and allow monitoring of smaller fish. Additionally, it is planned to include a gyroscope, so the inertial unit (gyroscope and accelerometer) would be able to provide a “three-in-one”: physical activity, respiratory frequency and angular acceleration (and hence fish trajectory). Moreover, the information drawn from the gyroscope could be of utility to refine the currently used on-board algorithms for the calculus of respiratory frequency and physical activity. Additionally, the current user-friendly accompanying software for device programming and data recovery will be updated to include these new features, improving the programmed schedule features for a more dynamic fish monitoring with different sleep and recordings periods, synchronous and asynchronous, by means of a real-time clock (Figure 7).
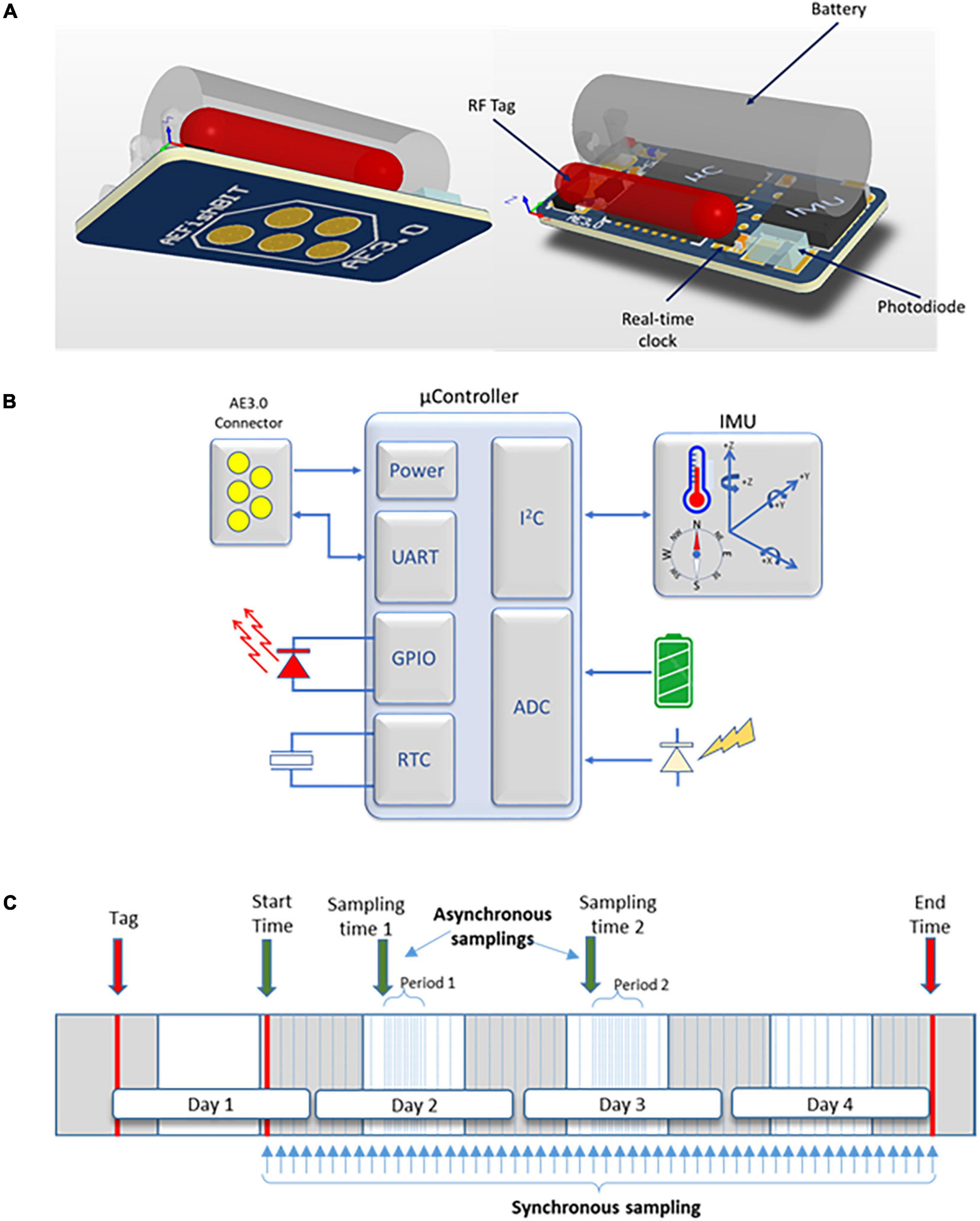
Figure 7. (A) 3D view of unencapsulated AEFishBIT prototype. Connection pins are shown in the left image. Prototype includes a real-time clock and a photodiode to ensure device functioning under insulation conditions in aquatic environment. IMU: inertial measurement unit (accelerometer, gyroscope, magnetometer, thermometer). μC: microcontroller. (B) Blocks diagram of AEFishBIT functioning. UART: universal asynchronous receiver-transmitter. GPIO: general purpose input/output. RTC: real-time clock. I2C: inter-integrated circuit. ADC: analog to digital converter for battery monitoring. (C) Diagram representation of AEFishBIT synchronous and asynchronous schedules.
As sum up in Figure 8, the main features of the current AEFishBIT device are different of those of other small gadgets available in the market, serving for different but also complementary purposes. Indeed, routine remote monitoring in large cage systems is mostly useful for real-time detection of unsteady fish reactions in response to predictable or unpredictable events, whereas AEFishBIT recording would assist farmers, breeders and physiologists for accurate phenotyping of behavior and whole metabolic traits under highly controlled conditions. Thus, this system is able to give useful information about how a fish is sensing and responding to changes in age, genetics and environment as a good proxy of both unsteady and sustained states of swimming and metabolic rates in a context of climate change, with cultured fish exposed to high temperatures and limited oxygen availability. The challenge is how this information at laboratory scale can be extrapolated and used to the field level for the improvement of aquaculture sustainability and profitability. In that sense, behavior changes related to the acceptance of new feed formulations and changing environmental conditions can be mimicked and tested at laboratory scale for merging genetics, nutrition, health and environmental science into a single transdisciplinary and fundamental knowledge system, supporting the future and viable growth of aquaculture. All this will serve to ensure the use of behavior monitoring as a reliable and routine approach to evaluate new fish feed formulations, largely based on sustainability and circular economy principles (Aragão et al., 2020; Naya-Català et al., 2021a).
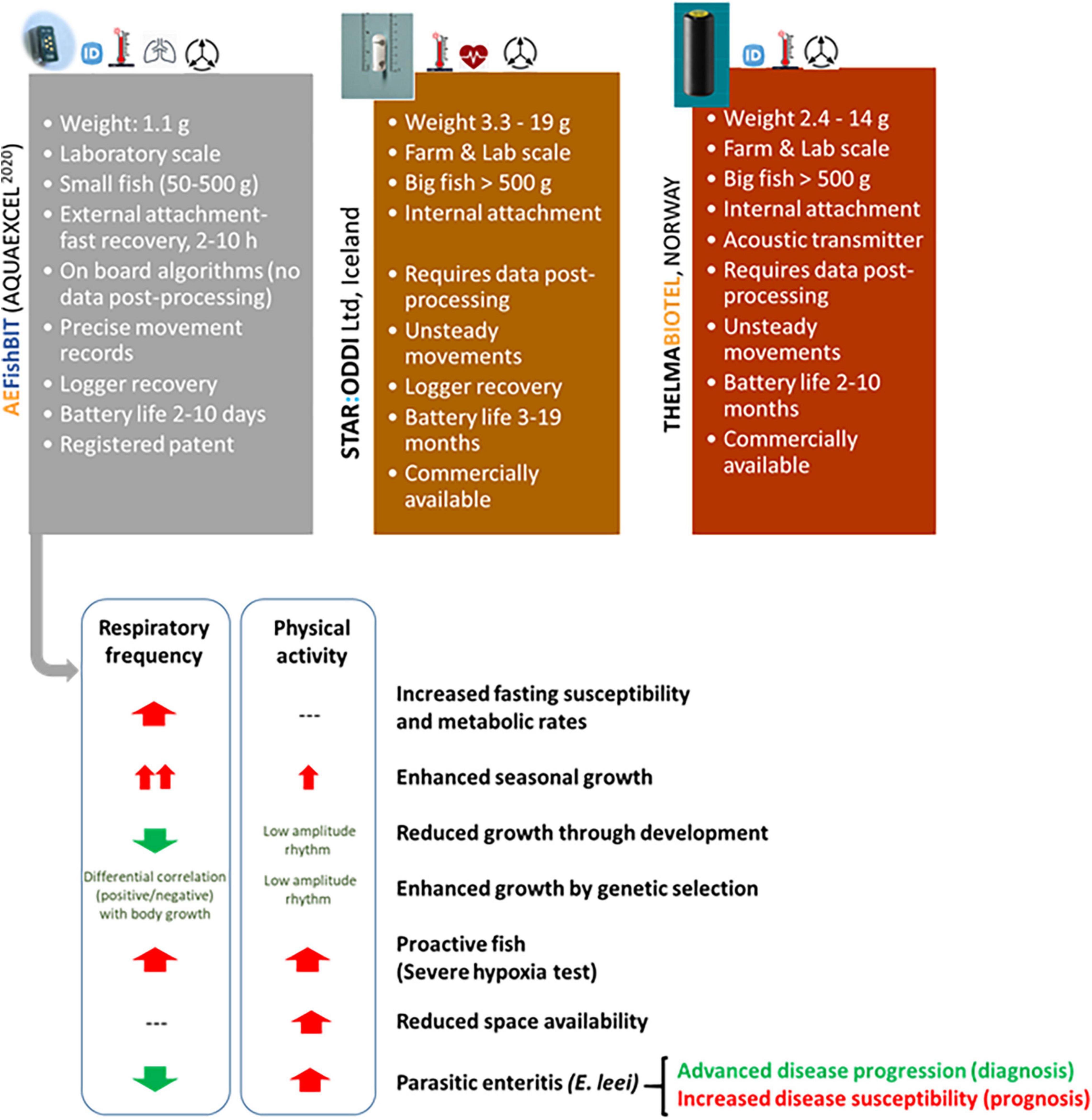
Figure 8. Main features of AEFishBIT and other comparable available devices for fish activity monitoring, with a graphical summary of AEFishBIT outcomes in gilthead sea bream. Partially adapted from data of Perera et al. (2021a) and Rosell-Moll et al. (2021).
Future prospects on behavior and emotional indicators can also serve to measure and interpret the positive reactions of fish to the environmental enrichment. Otherwise, regarding disease outcomes, it is of particular interest that the reduction of respiratory frequency was a consistent marker of the progression of parasitic enteritis in gilthead sea bream experimentally infected with the myxozoan parasite Enteromyxum leei (Rosell-Moll et al., 2021). This intestinal parasite is responsible of severe epizooties and production losses in Mediterranean fish farms (Sitjà-Bobadilla and Palenzuela, 2012), and there is now experimental evidence showing that differences in the basal behavior and physical activity are indicative of their predisposition to infection (unpublished results). This feature would add a predictive value to AEFishBIT records, which becomes especially interesting for driving selective breeding toward tailored productive traits. Finally, major efforts for the integration of complex data informing of the host response to nutritional inputs and genetics and microbiota interactions have been accomplished recently in farmed fish and gilthead sea bream in particular (Piazzon et al., 2017, 2019, 2020; Naya-Català et al., 2021a; Solé-Jiménez et al., 2021), but how this also affects to behavioral traits remains mostly unknown. Altogether, this opens new research opportunities for incorporating measurements of organism behavior as a routine procedure of academia researchers as well as fish farmers, and fish feed producers and breeders.
Author Contributions
JP-S: conceptualization. JC-G, MÁF, PP, and JP-S: writing-original manuscript. All authors writing-review and editing, read and approved the final manuscript.
Funding
This work was supported by the EU H2020 Research Innovation Program under grant agreement no. 871108 (AQUAEXCEL3.0). This output reflects only the author’s view and the European Union cannot be held responsible for any use that may be made of the information contained therein. Additional funding was obtained by the Spanish MCIN project Bream-AquaINTECH (RTI2018–094128-B-I00, AEI/FEDER, UE) to JP-S and JC-G. This study forms part of the ThinkInAzul programme and was supported by MCIN with funding from European Union NextGenerationEU (PRTR-C17.I1) and by Generalitat Valenciana (THINKINAZUL/2021/024) to JP-S.
Author Disclaimer
This output reflects only the authors’ view and the European Union cannot be held responsible for any use that may be made of the information contained therein.
Conflict of Interest
The authors declare that the research was conducted in the absence of any commercial or financial relationships that could be construed as a potential conflict of interest.
Publisher’s Note
All claims expressed in this article are solely those of the authors and do not necessarily represent those of their affiliated organizations, or those of the publisher, the editors and the reviewers. Any product that may be evaluated in this article, or claim that may be made by its manufacturer, is not guaranteed or endorsed by the publisher.
Footnotes
References
Alfonso, S., Zupa, W., Spedicato, M. T., Lembo, G., and Carbonara, P. l (2021). Mapping the energetic costs of free-swimming gilthead sea bream (Sparus aurata), a key species in European marine aquaculture. Biology 10:1357. doi: 10.3390/biology10121357
An, D., Huang, J., and Wei, Y. (2021). A survey of fish behaviour quantification indexes and methods in aquaculture. Rev. Aquacult. 13, 2169–2189. doi: 10.1111/raq.12564
Anttila, K., and Mänttäri, S. (2009). Ultrastructural differences and histochemical characteristics in swimming muscles between wild and reared Atlantic salmon. Acta Physiol. 196, 249–257. doi: 10.1111/j.1748-1716.2008.01911.x
Aragão, C., Cabano, M., Colen, R., Fuentes, J., and Dias, J. (2020). Alternative formulations for gilthead seabream diets: towards a more sustainable production. Aquac. Nutr. 26, 444–455. doi: 10.1111/anu.13007
Arechavala-Lopez, P., Caballero-Froilán, J. C., Jiménez-García, M., Capó, X., Tejada, S., Saraiva, J. L., et al. (2020). Enriched environments enhance cognition, exploratory behaviour and brain physiological functions of Sparus aurata. Sci. Rep. 10:11252. doi: 10.1038/s41598-020-68306-6
Arechavala-Lopez, P., Diaz-Gil, C., Saraiva, J. L., Moranta, D., Castanheira, M. F., Nuñez-Velázquez, S., et al. (2019). Effects of structural environmental enrichment on welfare of juvenile seabream (Sparus aurata). Aquac. Rep. 15:100224. doi: 10.1016/j.aqrep.2019.100224
Arechavala-Lopez, P., Lankheet, M. J., Díaz-Gil, C., Abbink, W., and Palstra, A. P. (2021). Swimming activity of gilthead seabream (Sparus aurata) in swim-tunnels: acoustic accelerometry, oxygen consumption and body motion. Front. Anim. Sci. 2:25. doi: 10.3389/fanim.2021.679848
Ashley, N. T., Schwabl, I., Goymann, W., and Buck, C. L. (2012). Keeping time under the midnight sun: behavioral and plasma melatonin profiles of free-living lapland longspurs (Calcarius lapponicus) during the Arctic Summer. J. Exp. Zool. A Ecol. Genet. Physiol. 319, 10–22. doi: 10.1002/jez.1768
Ashley, P. J. (2007). Fish welfare: current issues in aquaculture. Appl. Anim. Behav. Sci. 104, 199–235. doi: 10.1016/j.applanim.2006.09.001
Auer, S. K., Salin, K., Rudolf, A. M., Anderson, G. J., and Metcalfe, N. B. (2015). The optimal combination of standard metabolic rate and aerobic scope for somatic growth depends on food availability. Funct. Ecol. 29, 479–486. doi: 10.1111/1365-2435.12396
Azzaydi, M., Rubio, V. C., Martínez López, F. J., Sánchez-Vázquez, F. J., Zamora, S., and Madrid, J. A. (2007). Effect of restricted feeding schedule on seasonal shifting of daily demand-feeding pattern and food anticipatory activity in European sea bass (Dicentrarchus labrax L.). Chronobiol. Int. 24, 859–874. doi: 10.1080/07420520701658399
Balasch, J. C., and Tort, L. (2019). Netting the stress responses in fish. Front. Endocrinol. 10:62. doi: 10.3389/fendo.2019.00062
Barreto, M. O., Rey Planellas, S., Yang, Y., Phillips, C., and Descovich, K. (2021). Emerging indicators of fish welfare in aquaculture. Rev. Aquac. 107, 2411–2502. doi: 10.1111/raq.12601
Beemelmanns, A., Zanuzzo, F. S., Xue, X., Sandrelli, R. M., Rise, M. L., and Gamperl, A. K. (2021). The transcriptomic responses of Atlantic salmon (Salmo salar) to high temperature stress alone, and in combination with moderate hypoxia. BMC Genomics 22:261. doi: 10.1186/s12864-021-07464-x
Bellinger, K. L., Thorgaard, G. H., and Carter, P. A. (2014). Domestication is associated with reduced burst swimming performance and increased body size in clonal rainbow trout lines. Aquaculture 420, 154–159. doi: 10.1016/j.aquaculture.2013.10.028
Berger, J. (2010). “Fear-mediated food webs,” in Trophic Cascades: Predators, Prey and the Changing Dynamics of Nature, eds J. Terborgh and J. A. Estes (Washington, DC: Island Press), 241–254.
Bermejo-Nogales, A., Calduch-Giner, J. A., and Pérez-Sánchez, J. (2015). Unraveling the molecular signatures of oxidative phosphorylation to cope with the nutritionally changing metabolic capabilities of liver and muscle tissues in farmed fish. PLoS One 10:e0122889. doi: 10.1371/journal.pone.0122889
Bermejo-Nogales, A., Nederlof, M., Benedito-Palos, L., Ballester-Lozano, G. F., Folkedal, O., Olsen, R. E., et al. (2014). Metabolic and transcriptional responses of gilthead sea bream (Sparus aurata L.) to environmental stress: new insights in fish mitochondrial phenotyping. Gen. Comp. Endocrinol. 205, 305–315. doi: 10.1016/j.ygcen.2014.04.016
Blasco, J., Moya, A., Millán-Cubillo, A., Vélez, E. J., Capilla, E., Pérez-Sánchez, J., et al. (2015). Growth-promoting effects of sustained swimming in fingerlings of gilthead sea bream (Sparus aurata L.). J. Comp. Physiol. B 185, 859–868. doi: 10.1007/s00360-015-0933-5
Bloch, G., Barnes, B. M., Gerkema, M. P., and Helm, B. (2013). Animal activity around the clock with no overt circadian rhythms: patterns, mechanisms and adaptive value. Proc. R. Soc. B 280:20130019. doi: 10.1098/rspb.2013.0019
Brander, K. M. (2007). Global fish production and climate change. Proc. Natl. Acad. Sci. 104, 19709–19714. doi: 10.1073/pnas.0702059104
Broell, F., Noda, T., Wright, S., Domenici, P., Steffensen, J. F., Auclair, J.-P., et al. (2013). Accelerometer tags: detecting and identifying activities in fish and the effect of sampling frequency. J. Exp. Biol. 216, 1255–1264. doi: 10.1242/jeb.077396
Brydges, N. M., and Braithwaite, V. A. (2009). Does environmental enrichment affect the behaviour of fish commonly used in laboratory work? Appl. Anim. Behav. Sci. 118, 137–143. doi: 10.1016/j.applanim.2009.02.017
Burke, M., Grant, J., Filgueira, R., and Stone, T. (2021). Oceanographic processes control dissolved oxygen variability at a commercial Atlantic salmon farm: application of a real-time sensor network. Aquaculture 533:736143. doi: 10.1016/j.aquaculture.2020.736143
Calduch-Giner, J. A., Echasseriau, Y., Crespo, D., Baron, D., Planas, J. V., Prunet, P., et al. (2014). Transcriptional assessment by microarray analysis and large-scale meta-analysis of the metabolic capacity of cardiac and skeletal muscle tissues to cope with reduced nutrient availability in gilthead sea bream (Sparus aurata L.). Mar. Biotechnol. 16, 423–435. doi: 10.1007/s10126-014-9562-3
Challet, E. (2013). Circadian clocks, food intake, and metabolism. Prog. Mol. Biol. Transl. Sci. 119, 105–135. doi: 10.1016/B978-0-12-396971-2.00005-1
Chen, K. Y., and Bassett, D. R. Jr. (2005). The technology of accelerometry-based activity monitors: current and future. Med. Sci. Sports Exerc. 37, S490–S500. doi: 10.1249/01.mss.0000185571.49104.82
Cockrem, J. F. (1990). “Circadian rhythms,” in Antarctic Penguins. Penguin biology, eds L. S. Davis and J. T. Darby (San Diego, CA: Academic Press), 319–344.
Cooke, S. J., Nguyen, V. M., Murchie, K. J., Thiem, J. D., Donaldson, M. R., Hinch, S. G., et al. (2013). To tag or not to tag: animal welfare, conservation, and stakeholder considerations in fish tracking studies that use electronic tags. J. Int. Wildlife Law Policy 16, 352–374. doi: 10.1080/13880292.2013.805075
de Goede, D., Gremmen, B., Rodenburg, T. B., Bolhuis, J. E., Bijma, P., Scholten, M., et al. (2013). Reducing damaging behaviour in robust livestock farming. NJAS Wagen. J. Life. Sci. 66, 49–53. doi: 10.1016/j.njas.2013.05.006
de Matos Dias, D., de Campos, C. B., and Rodrigues, F. H. G. (2018). Behavioural ecology in a predator-prey system. Mamm. Biol. 92, 30–36. doi: 10.1016/j.mambio.2018.04.005
del Pozo, A., Sánchez-Férez, J. A., and Sánchez-Vázquez, F. J. (2011). Circadian rhythms of self-feeding and locomotor activity in zebrafish (Danio rerio). Chronobiol. Int. 28, 39–47. doi: 10.3109/07420528.2010.530728
Devlin, R. H., D’Andrade, M., Uh, M., and Biagi, C. A. (2004). Population effects of growth hormone transgenic coho salmon depend on food availability and genotype by environment interactions. Proc. Natl. Acad. Sci. U. S. A. 101, 9303–9308. doi: 10.1073/pnas.0400023101
Dunn, J. F., and Hochachka, P. W. (1986). Metabolic responses of trout (Salmo gairdneri) to acute environmental hypoxia. J. Exp. Biol. 123, 229–242.
Eckel-Mahan, K., and Sassone-Corsi, P. (2013). Metabolism and the circadian clock converge. Physiol. Rev. 93, 107–135. doi: 10.1152/physrev.00016.2012
Evans, M. L., Hori, T. S., Rise, M. L., and Fleming, I. A. (2015). Transcriptomic responses of Atlantic Salmon (Salmo salar) to environmental enrichment during juvenile rearing. PLoS One 10:e0118378. doi: 10.1371/journal.pone.0118378
Ferrer, M. A., Calduch-Giner, J. A., Díaz, M., Sosa, J., Rosell-Moll, E., Santana Abril, J., et al. (2020). From operculum and body tail movements to different coupling of physical activity and respiratory frequency in farmed gilthead sea bream and European sea bass. Insights on aquaculture biosensing. Comput. Electron. Agric. 175:105531. doi: 10.1016/j.compag.2020.105531
Flash, T., and Hogan, N. (1985). The coordination of arm movements: an experimentally confirmed mathematical model. J. Neurosci. 5, 1688–1703. doi: 10.1523/JNEUROSCI.05-07-01688.1985
Folkedal, O., Stien, L. H., Torgersen, T., Oppedal, F., Olsen, R. E., Fosseidengen, J. E., et al. (2012). Food anticipatory behaviour as an indicator of stress response and recovery in Atlantic salmon post-smolt after exposure to acute temperature fluctuation. Physiol. Behav. 105, 350–356. doi: 10.1016/j.physbeh.2011.08.008
Føre, M., Frank, K., Dempster, T., Alfredsen, J. A., and Høy, E. (2017). Biomonitoring using tagged sentinel fish and acoustic telemetry in commercial salmon aquaculture: a feasibility study. Aquac. Eng. 78, 163–172. doi: 10.1016/j.aquaeng.2017.07.004
Føre, M., Frank, K., Norton, T., Svendsen, E., Alfredsen, J. A., Dempster, T., et al. (2018). Precision fish farming: a new framework to improve production in aquaculture. Biosyst. Eng. 173, 176–193. doi: 10.1016/j.biosystemseng.2017.10.014
Fraser, D., and Duncan, I. J. (1998). ‘Pleasures’,‘pains’ and animal welfare: toward a natural history of affect. Anim. Welf. 1998, 383–396.
Gallagher, M. D., Matejusova, I., Nguyen, L., Ruane, N. M., Falk, K., and Macqueen, D. J. (2018). Nanopore sequencing for rapid diagnostics of salmonid RNA viruses. Sci. Rep. 8:16307. doi: 10.1038/s41598-018-34464-x
Gamboa, J. L., and Andrade, F. H. (2012). Muscle endurance and mitochondrial function after chronic normobaric hypoxia: contrast of respiratory and limb muscles. Pflügers Arch. Eur. J. Physiol. 463, 327–338. doi: 10.1007/s00424-011-1057-8
Gamperl, A. K., Ajiboye, O. O., Zanuzzo, F. S., Sandrelli, R. M., Ellen de Fátima, C. P., and Beemelmanns, A. (2020). The impacts of increasing temperature and moderate hypoxia on the production characteristics, cardiac morphology and haematology of Atlantic Salmon (Salmo salar). Aquaculture 519, 1–14. doi: 10.1016/j.aquaculture.2019.734874
Georgopoulou, D. G., Stavrakidis-Zachou, O., Mitrizakis, N., and Papandroulakis, N. (2021). Tracking and analysis of the movement behavior of European seabass (Dicentrarchus labrax) in aquaculture systems. Front. Anim. Sci. 2:754520. doi: 10.3389/fanim.2021.754520
Geurden, I., Borchert, P., Balasubramanian, M. N., Schrama, J. W., Dupont-Nivet, M., Quillet, E., et al. (2013). The positive impact of the early-feeding of a plant-based diet on its future acceptance and utilisation in rainbow trout. PLoS One 8:e83162. doi: 10.1371/journal.pone.0083162
Gonçalves, A. T., Collipal-Matamal, R., Valenzuela-Muñoz, V., Nuñez-Acuña, G., Valenzuela-Miranda, D., and Gallardo-Escárate, C. (2020). Nanopore sequencing of microbial communities reveals the potential role of sea lice as a reservoir for fish pathogens. Sci. Rep. 10:2895. doi: 10.1038/s41598-020-59747-0
Hamäläinen, W., Järvinen, M., Martiskainen, P., and Mononen, J. (2011). “Jerk-based feature extraction for robust activity recognition from acceleration data,” in Proeedings of the 2011 11th International Conference on Intelligent Systems Design and Applications (Cordoba: IEEE), 831–836. doi: 10.1109/ISDA.2011.6121760
Healy, T. M., Chung, D. J., Crowther, K. G., and Schulte, P. M. (2017). Metabolic and regulatory responses involved in cold acclimation in Atlantic killifish, Fundulus heteroclitus. J. Comp. Physiol. B. 187, 463–475. doi: 10.1007/s00360-016-1042-9
Hemsworth, P. H., and Coleman, G. J. (2011). “Human-animal interactions and animal productivity and welfare,” in Human-livestock interactions. The Stockperson and the Productivity of Intensively Farmed Animals, eds P. H. Hemsworth and G. J. Coleman (Oxford: CAB International), 47–83. doi: 10.1079/9781845936730.0047
Hoppeler, H., and Vogt, M. (2001). Muscle tissue adaptations to hypoxia. J. Exp. Biol. 204, 3133–3139. doi: 10.1242/jeb.204.18.3133
Hou, Z. S., Wen, H. S., Li, J. F., He, F., Li, Y., and Qi, X. (2020). Environmental hypoxia causes growth retardation, osteoclast differentiation and calcium dyshomeostasis in juvenile rainbow trout (Oncorhynchus mykiss). Sci. Total Environ. 705:135272. doi: 10.1016/j.scitotenv.2019.135272
Huby, A., and Parmentier, E. (2019). “Actinopterygians: head, jaws and muscles,” in Heads, Jaws, and Muscles. Fascinating Life Sciences, eds J. Ziermann, R. Jr. Diaz, R. Diogo (Cham: Springer), doi: 10.1007/978-3-319-93560-7_5C
Hung, C. C., Tsao, S. C., Huang, K. H., Jang, J. P., Chang, H. K., and Dobbs, F. C. (2016). A highly sensitive underwater video system for use in turbid aquaculture ponds. Sci. Rep. 6:31810. doi: 10.1038/srep31810
Huntingford, F. A. (2004). Implications of domestication and rearing conditions for the behaviour of cultivated fishes. J. Fish Biol. 65, 122–142. doi: 10.1111/j.0022-1112.2004.00562.x
Huntingford, F. A., Adams, C., Braithwaite, V. A., Kadri, S., Pottinger, T. G., Sandøe, P., et al. (2006). Current issues in fish welfare. J. Fish Biol. 68, 332–372. doi: 10.1111/j.0022-1112.2006.001046.x
Huntingford, F. A., Andrew, G., Mackenzie, S., Morera, D., Coyle, S. M., Pilarczyk, M., et al. (2010). Coping strategies in a strongly schooling fish, the common carp Cyprinus carpio. J. Fish Biol. 76, 1576–1591. doi: 10.1111/j.1095-8649.2010.02582.x
Jepsen, N., Thorstad, E. B., Havn, T., and Lucas, M. C. (2015). The use of external electronic tags on fish: an evaluation of tag retention and tagging effects. Anim. Biotelem. 3:49. doi: 10.1186/s40317-015-0086-z
Kolarevic, J., Aas-Hansen, Ø, Espmark, Å, Baeverfjord, G., Terjesen, B. F., and Damsgård, B. (2016). The use of acoustic acceleration transmitter tags for monitoring of Atlantic salmon swimming activity in recirculating aquaculture systems (RAS). Aquat. Eng. 72, 30–39. doi: 10.1016/j.aquaeng.2016.03.002
Kolarevic, J., Calduch-Giner, J., Espmark, ÅM., Evensen, T., Sosa, J., and Pérez-Sánchez, J. (2021). A novel miniaturized biosensor for monitoring Atlantic salmon swimming activity and respiratory frequency. Animals 11:2403. doi: 10.3390/ani11082403
Koolhaas, J. M., Korte, S. M., De Boer, S. F., Van Der Vegt, B. J., Van Reenen, C. G., Hopster, H., et al. (1999). Coping styles in animals: current status in behavior and stress-physiology. Neurosci. Biobehav. Rev. 23, 925–935. doi: 10.1016/S0149-7634(99)00026-3
Korte, S. M., Koolhaas, J. M., Wingfield, J. C., and McEwen, B. S. (2005). The Darwinian concept of stress: benefits of allostasis and costs of allostatic load and the trade-offs in health and disease. Neurosci. Biobehav. Rev. 29, 3–38. doi: 10.1016/j.neubiorev.2004.08.009
Li, D. L., Wang, Z. H., Wu, S. Y., Miao, Z., Du, L., and Duan, Y. Q. (2020). Automatic recognition methods of fish feeding behavior in aquaculture: a review. Aquaculture 528:735508. doi: 10.1016/j.aquaculture.2020.735508
Liu, S., and Fu, S. J. (2017). Effects of food availability on metabolism, behaviour, growth and their relationships in a triploid carp. J. Exp. Biol. 220, 4711–4719. doi: 10.1242/jeb.167783
López-Olmeda, J. F., Montoya, A., Oliveira, C., and Sánchez-Vázquez, F. J. (2009). Synchronization to light and restricted-feeding schedules of behavioral and humoral daily rhythms in gilthead sea bream (Sparus aurata). Chronobiol. Int. 26, 1389–1408. doi: 10.3109/07420520903421922
Lucas, M. C., and Baras, E. (2000). Methods for studying spatial behaviour of freshwater fishes in the natural environment. Fish Fish. 1, 283–316. doi: 10.1046/j.1467-2979.2000.00028.x
Macaulay, G., Warren-Myers, F., Barrett, L. T., Oppedal, F., Føre, M., and Dempster, T. (2021). Tag use to monitor fish behaviour in aquaculture: a review of benefits, problems and solutions. Rev. Aquac. 13, 1565–1582. doi: 10.1111/raq.12534
Madrid, J. A., Boujard, T., and Sánchez-Vázquez, F. J. (2001). “Feeding rhythms,” in Food intake in fish, eds D. Houlihan, T. Boujard, and M. Jobling (Oxford: Blackwell Science Ltd), 189–215. doi: 10.1002/9780470999516.ch8
Magnoni, L. J., Martos-Sitcha, J. A., Queiroz, A., Calduch-Giner, J. A., Magalhàes Gonçalves, J. F., Rocha, C. M. R., et al. (2017). Dietary supplementation of heat-treated Gracillaria and Ulva seaweeds enhanced acute hypoxia tolerance in gilthead seabream (Sparus aurata). Biol. Open 6, 897–908. doi: 10.1242/bio.024299
Majeed, N., Panigrahi, K. C., Sukla, L. B., John, R., and Panigrahy, M. (2020). Regulatory mechanisms across networks of the circadian clock and senescence pathways. J. Plant Biochem. Biotechnol. 29, 665–674. doi: 10.1007/s13562-020-00612-6
Martins, C. I. M., Galhardo, L., Noble, C., Damsgård, B., Spedicato, M. T., Zupa, W., et al. (2012). Behavioural indicators of welfare in farmed fish. Fish Physiol. Biochem. 38, 17–41. doi: 10.1007/s10695-011-9518-8
Martos-Sitcha, J. A., Bermejo-Nogales, A., Calduch-Giner, J. A., and Pérez-Sánchez, J. (2017). Gene expression profiling of whole blood cells supports a more efficient mitochondrial respiration in hypoxia-challenged gilthead sea bream (Sparus aurata). Front. Zool. 14:34. doi: 10.1186/s12983-017-0220-2
Martos-Sitcha, J. A., Simó-Mirabet, P., de las Heras, V., Calduch-Giner, J. A., and Pérez-Sánchez, J. (2019a). Tissue-specific orchestration of gilthead sea bream resilience to hypoxia and high stocking density. Front. Physiol. 10:840. doi: 10.3389/fphys.2019.00840
Martos-Sitcha, J. A., Sosa, J., Ramos-Valido, D., Bravo, F. J., Carmona-Duarte, C., Gomes, H. L., et al. (2019b). Ultra-low power sensor devices for monitoring physical activity and respiratory frequency in farmed fish. Front. Physiol. 10:667. doi: 10.3389/fphys.2019.00667
Muñoz, L., Aspillaga, E., Palmer, M., Saraiva, J. L., and Arechavala-Lopez, P. (2020). Acoustic telemetry: a tool to monitor fish swimming behavior in sea-cage aquaculture. Front. Mar. Sci. 7:645. doi: 10.3389/fmars.2020.00645
Murchie, K. J., Cooke, S. J., Danylchuk, A. J., and Suski, C. D. (2011). Estimates of field activity and metabolic rates of bonefish (Albula vulpes) in coastal marine habitats using acoustic tri-axial accelerometer transmitters and intermittent-flow respirometry. J. Exp. Mar. Biol. Ecol. 396, 147–155. doi: 10.1016/j.jembe.2010.10.019
Murray, A. J. (2009). Metabolic adaptation of skeletal muscle to high altitude hypoxia: how new technologies could resolve the controversies. Genome Med. 1:117. doi: 10.1186/gm117
Naya-Català, F., do Vale Pereira, G., Piazzon, M. C., Fernandes, A. M., Calduch-Giner, J. A., Sitjà-Bobadilla, A., et al. (2021a). Cross-talk between intestinal microbiota and host gene expression in gilthead sea bream (Sparus aurata) Juveniles: insights in fish feeds for increased circularity and resource utilization. Front. Physiol. 12:748265. doi: 10.3389/fphys.2021.748265
Naya-Català, F., Simó-Mirabet, P., Calduch-Giner, J., and Pérez-Sánchez, J. (2021b). Transcriptomic profiling of Gh/Igf system reveals a prompted tissue-specifc diferentiation and novel hypoxia responsive genes in gilthead sea bream. Sci. Rep. 11:16466. doi: 10.1038/s41598-021-95408-6
Newton, M., Barry, J., Dodd, J. A., Lucas, M. C., Boylan, P., and Adams, C. E. (2016). Does size matter? A test of size-specific mortality in Atlantic salmon Salmo salar smolts tagged with acoustic transmitters. J. Fish Biol. 89, 1641–1650. doi: 10.1111/jfb.13066
Noble, E. C., Gismervik, K., Iversen, M. H., Kolarevic, J., Nilsson, J., Stien, L. H., et al. (2018). Welfare Indicators for Farmed Atlantic Salmon—Tools for Assessing Fish Welfare. Tromso: Nofima, 351.
Noble, E. C., Gismervik, K., Iversen, M. H., Kolarevic, J., Nilsson, J., Stien, L. H., et al. (2020). Welfare Indicators for Farmed Rainbow Trout: Tools for Assessing Fish Welfare. Tromso: Nofima, 310.
Noble, E. C., Iversen, M. H., Lein, I., Kolarevic, J., Johansen, L. H., Burgerhout, E., et al. (2019). Rensevel OWI Fact Sheet Series: an Introduction to Operational and Laboratory based Welfare Indicators for Ballan wrasse (Labrus bergylta). Tromso: Nofima.
Øverli, Ø, Winberg, S., and Pottinger, T. G. (2005). Behavioral and neuroendocrine correlates of selection for stress responsiveness in rainbow trout—a review. Integr. Comp. Biol. 45, 463–474. doi: 10.1093/icb/45.3.463
Palstra, A. P., Arechavala-Lopez, P., Xue, Y., and Roque, A. (2021). Accelerometry of seabream in a sea-cage: Is acceleration a good proxy for activity? Front. Mar. Sci. 8:144. doi: 10.3389/fmars.2021.639608
Palstra, A. P., Roque, A., Kruijt, L., Jéhannet, P., Pérez-Sánchez, J., and Dirks, R. P. (2020). Physiological effects of water flow induced swimming exercise in seabream Sparus aurata. Front. Physiol. 11:610049. doi: 10.3389/fphys.2020.610049
Papaharisis, L., Tsironi, T., Dimitroglou, A., Taoukis, P., and Pavlidis, M. (2019). Stress assessment, quality indicators and shelf life of three aquaculture important marine fish, in relation to harvest practices, water temperature and slaughter method. Aquac. Res. 50, 2608–2620. doi: 10.1111/are.14217
Pedrazzani, A. S., Quintiliano, M. H., Bolfe, F., Sans, E. C. D. O., and Molento, C. F. M. (2020). Tilapia on-farm welfare assessment protocol for semi-intensive production systems. Front. Vet. Sci. 7:991. doi: 10.3389/fvets.2020.606388
Perera, E., Rosell-Moll, E., Martos-Sitcha, J. A., Naya-Català, F., Simó-Mirabet, P., Calduch-Giner, J. A., et al. (2021a). Physiological trade-offs associated with fasting weight loss, resistance to exercise and behavioral traits in farmed gilthead sea bream (Sparus aurata) selected by growth. Aquac. Rep. 20:100645. doi: 10.1016/j.aqrep.2021.100645
Perera, E., Rosell-Moll, E., Naya-Català, F., Simó-Mirabet, P., Calduch-Giner, J., and Pérez-Sánchez, J. (2021b). Effects of genetics and early-life mild hypoxia on size variation in farmed gilthead sea bream (Sparus aurata). Fish Physiol. Biochem. 47, 121–133. doi: 10.1007/s10695-020-00899-1
Perera, E., Simó-Mirabet, P., SukShin, H., Rosell-Moll, E., Naya-Català, F., De las Heras, V., et al. (2019). Selection for growth is associated in gilthead sea bream (Sparus aurata) with diet flexibility, changes in growth patterns and higher intestine plasticity. Aquaculture 507, 349–360. doi: 10.1016/j.aquaculture.2019.04.052
Pérez-Sánchez, J., Bermejo-Nogales, A., Calduch-Giner, J. A., Kaushik, S., and Sitjà-Bobadilla, A. (2011). Molecular characterization and expression analysis of six peroxiredoxin paralogous genes in gilthead sea bream (Sparus aurata): insights from fish exposed to dietary, pathogen and confinement stressors. Fish Shellfish Immunol. 31, 294–302. doi: 10.1016/j.fsi.2011.05.015
Piazzon, M. C., Calduch-Giner, J. A., Fouz, B., Estensoro, I., Simó-Mirabet, P., Puyalto, M., et al. (2017). Under control: how a dietary additive can restore the gut microbiome and proteomic profile, and improve disease resilience in a marine teleostean fish fed vegetable diets. Microbiome 5:164. doi: 10.1186/s40168-017-0390-3
Piazzon, M. C., Naya-Català, F., Perera, E., Palenzuela, O., Sitjà-Bobadilla, A., and Pérez-Sànchez, J. (2020). Genetic selection for growth drives differences in intestinal microbiota composition and parasite disease resistance in gilthead sea bream. Microbiome 8:168. doi: 10.1186/s40168-020-00922-w
Piazzon, M. C., Naya-Català, F., Simó-Mirabet, P., Picard-Sánchez, A., Roig, F. J., Calduch-Giner, J. A., et al. (2019). Sex, age, and bacteria: how the intestinal microbiota is modulated in a protandrous hermaphrodite fish. Front. Microbiol. 10:2512. doi: 10.3389/fmicb.2019.02512
Pottinger, T. G., and Carrick, T. R. (1999). Modification of the plasma cortisol response to stress in rainbow trout by selective breeding. Gen. Comp. Endocrinol. 116, 122–132. doi: 10.1006/gcen.1999.7355
Power, D. M., Louro, B., Houston, R., Anjos, L., and Cardoso, J. C. R. (2011). “Genomic-proteomic research in Sparidae and its application to genetic improvement,” in Sparidae: Biology and Aquaculture of Gilthead Sea Bream and other Species, 1st Edn, eds M. A. Pavlidis and C. C. Mylonas (Oxford: Wiley-Blackwell), 359–381.
Ragsdale, A., Ortega-Recalde, O., Dutoit, L., Besson, A. A., Chia, J. H., King, T., et al. (2020). Paternal hypoxia exposure primes offspring for increased hypoxia resistance. bioRxiv [Preprint]. doi: 10.1101/2020.12.09.416727
Reebs, S. G. (2002). Plasticity of diel and circadian activity rhythms in fishes. Rev. Fish Biol. Fish. 12, 349–371. doi: 10.1023/A:1025371804611
Reid, D., Armstrong, J. D., and Metcalfe, N. B. (2011). Estimated standard metabolic rate interacts with territory quality and density to determine the growth rates of juvenile Atlantic salmon. Funct. Ecol. 25, 1360–1367. doi: 10.1111/j.1365-2435.2011.01894.x
Rey, S., Little, D. C., and Ellis, M. A. (2019). Farmed Fish Welfare Practices: Salmon Farming as a Case Study. GAA publications. Available online at: https://www.aquaculturealliance.org/wp-content/uploads/2020/05/FarmedFishWelfarePractices_26_May_2020.pdf [accessed on May 26, 2020]
Rodnick, K. J., and Planas, J. V. (2016). The stress and stress mitigation effects of exercise: cardiovascular, metabolic, and skeletal muscle adjustments. Fish Physiol. 35, 251–294.
Rosell-Moll, E., Piazzon, M. C., Sosa, J., Ferrer, M. A., Cabruja, E., Vega, A., et al. (2021). Use of accelerometer technology for individual tracking of activity patterns, metabolic rates and welfare in farmed gilthead sea bream (Sparus aurata) facing a wide range of stressors. Aquaculture 539:736609. doi: 10.1016/j.aquaculture.2021.736609
Sadoul, B., Alfonso, S., Cousin, X., Prunet, P., Bégout, M. L., and Leguen, I. (2021). Global assessment of the response to chronic stress in European sea bass. Aquaculture 544:737072. doi: 10.1016/j.aquaculture.2021.737072
Sadoul, B., Friggens, N. C., Valotaire, C., Labbé, L., Colson, V., Prunet, P., et al. (2017). Physiological and behavioral flexibility to an acute CO2 challenge, within and between genotypes in rainbow trout. Comp. Biochem. Physiol. Part A 209, 25–33. doi: 10.1016/j.cbpa.2017.04.002
Sadoul, B., Leguen, I., Colson, V., Friggens, N. C., and Prunet, P. (2015). A multivariate analysis using physiology and behavior to characterize robustness in two isogenic lines of rainbow trout exposed to a confinement stress. Physiol. Behav. 140, 139–147. doi: 10.1016/j.physbeh.2014.12.006
Sánchez-Vázquez, F. J., and Madrid, J. A. (2001). “Feeding anticipatory activity,” in Food Intake in Fish, eds D. Houlihan, T. Boujard, and M. Jobling (Oxford: Blackwell Science), 216–232.
Saraiva, J. L., and Arechavala-Lopez, P. (2019). Welfare of fish-no longer the elephant in the room. Fishes 4, 39. doi: 10.3390/fishes4030039
Saraiva, J. L., Castanheira, M. F., Arechavala-Lopez, P., Volstorf, J., and Studer, B. H. (2018). “Domestication and Welfare in Farmed Fish,” in Animal Domestication, ed. F. Teletchea (London: IntechOpen).
Segner, H., Reiser, S., Ruane, N., Rösch, R., Steinhagen, D., and Vehanen, T. (2019). Welfare of Fishes in Aquaculture. FAO Fisheries and Aquaculture Circular No. C1189. Budapest: FAO.
Sharma, M. (2019). Behavioural responses in effect to chemical stress in fish: a review. Int. J. Fish. Aquat. Stud. 7, 1–5.
Sitjà-Bobadilla, A., and Palenzuela, O. (2012). “Enteromyxum species,” in Fish Parasites: Pathology and Protection, eds P. T. K. Woo and K. Buchmann (Oxfordshire: CAB International), 163–176. doi: 10.1079/9781845938062.0163
Skvortsova, A., Veldhuijzen, D. S., Kloosterman, I. E., Pacheco-López, G., and Evers, A. W. (2021). Food anticipatory hormonal responses: a systematic review of animal and human studies. Neurosci. Biobehav. Rev. 126, 447–464. doi: 10.1016/j.neubiorev.2021.03.030
Sokolova, I. (2018). Mitochondrial adaptations to variable environments and their role in animals’ stress tolerance. Integr. Comp. Biol. 58, 519–531. doi: 10.1093/icb/icy017
Solé-Jiménez, P., Naya-Català, F., Piazzon, M. C., Estensoro, I., Calduch-Giner, J. À, Sitjà-Bobadilla, A., et al. (2021). Reshaping of gut microbiota in gilthead sea bream fed microbial and processed animal proteins as the main dietary protein source. Front. Mar. Sci. 8:842. doi: 10.3389/fmars.2021.705041
Stevens, A., Doneley, R., Cogny, A., and Phillips, C. J. (2021). The effects of environmental enrichment on the behaviour of cockatiels (Nymphicus hollandicus) in aviaries. Appl. Anim. Behav. Sci. 235:105154. doi: 10.1016/j.applanim.2020.105154
Tevy, M. F., Giebultowicz, J., Pincus, Z., Mazzoccoli, G., and Vinciguerra, M. (2013). Aging signaling pathways and circadian clock-dependent metabolic derangements. Trends Endocrinol. Metab. 24, 229–237. doi: 10.1016/j.tem.2012.12.002
Toni, M., Manciocco, A., Angiulli, E., Alleva, E., Cioni, C., and Malavasi, S. (2019). Review: assessing fish welfare in research and aquaculture, with a focus on European directives. Animal 13, 161–170. doi: 10.1017/S1751731118000940
Van der Zee, E. A., Havekes, R., Barf, R. P., Hut, R. A., Nijholt, I. M., Jacobs, E. H., et al. (2008). Circadian time-place learning in mice depends on Cry genes. Curr. Biol. 18, 844–848. doi: 10.1016/j.cub.2008.04.077
Veissier, I., and Boissy, A. (2007). Stress and welfare: two complementary concepts that are intrinsically related to the animal’s point of view. Physiol. Behav. 92, 429–433. doi: 10.1016/j.physbeh.2006.11.008
Vollset, K. W., Lennox, R. J., Thorstad, E. B., Auer, S., Bär, K., Larsen, M. H., et al. (2020). Systematic review and meta-analysis of PIT tagging effects on mortality and growth of juvenile salmonids. Rev. Fish Biol. Fish. 30, 553–568. doi: 10.1007/s11160-020-09611-1
Warren-Myers, F., Hvas, M., Vågseth, T., Dempster, T., and Oppedal, F. (2021). Sentinels in salmon aquaculture: heart rates across seasons and during crowding events. Front. Physiol. 12:755659. doi: 10.3389/fphys.2021.755659
Wilson, S. M., Hinch, S. G., Eliason, E. J., Farrell, A. P., and Cooke, S. J. (2013). Calibrating acoustic acceleration transmitters for estimating energy use by wild adult Pacific salmon. Comp. Biochem. Physiol. Part A Mol. Integr. Physiol. 164, 491–498. doi: 10.1016/j.cbpa.2012.12.002
Zhang, Y., Timmerhaus, G., Anttila, K., Mauduit, F., Jørgensen, S. M., Kristensen, T., et al. (2016). eDomestication compromises athleticism and respiratory plasticity in response to aerobic exercise training in Atlantic salmon (Salmo salar). Aquaculture 463, 79–88. doi: 10.1016/j.aquaculture.2016.05.015
Keywords: bio-loggers, welfare indicator, activity patterns, ventilation rate, fish behavior, fish robustness
Citation: Calduch-Giner J, Holhorea PG, Ferrer MÁ, Naya-Català F, Rosell-Moll E, Vega García C, Prunet P, Espmark ÅM, Leguen I, Kolarevic J, Vega A, Kerneis T, Goardon L, Afonso JM and Pérez-Sánchez J (2022) Revising the Impact and Prospects of Activity and Ventilation Rate Bio-Loggers for Tracking Welfare and Fish-Environment Interactions in Salmonids and Mediterranean Farmed Fish. Front. Mar. Sci. 9:854888. doi: 10.3389/fmars.2022.854888
Received: 14 January 2022; Accepted: 09 February 2022;
Published: 07 March 2022.
Edited by:
Enric Gisbert, Institute of Agrifood Research and Technology (IRTA), SpainReviewed by:
Lluis Tort, Universitat Autònoma de Barcelona, SpainMarce Herrera, IFAPA Centro Agua del Pino, Spain
Copyright © 2022 Calduch-Giner, Holhorea, Ferrer, Naya-Català, Rosell-Moll, Vega García, Prunet, Espmark, Leguen, Kolarevic, Vega, Kerneis, Goardon, Afonso and Pérez-Sánchez. This is an open-access article distributed under the terms of the Creative Commons Attribution License (CC BY). The use, distribution or reproduction in other forums is permitted, provided the original author(s) and the copyright owner(s) are credited and that the original publication in this journal is cited, in accordance with accepted academic practice. No use, distribution or reproduction is permitted which does not comply with these terms.
*Correspondence: Jaume Pérez-Sánchez, amFpbWUucGVyZXouc2FuY2hlekBjc2ljLmVz