- 1School of Biological Sciences, Monash University, Clayton, VIC, Australia
- 2Halpin Wildlife Research, Vancouver, BC, Canada
- 3School of Biological Sciences, University of Adelaide, Adelaide, SA, Australia
- 4Institute of Marine Sciences, University of California, Santa Cruz, Santa Cruz, CA, United States
- 5HiDef Aerial Surveying Ltd., Edinburgh, Scotland, United Kingdom
- 6Independent Researcher, Nanaimo, BC, Canada
- 7Department of Planning, Industry and Environment, Parramatta, NSW, Australia
Gadfly petrels (genus Pterodroma) are one of the most threatened groups of birds. They are exceptionally well adapted to forage over enormous areas to maximize chances of encountering prey. Their wide-ranging travel, extensive use of oceanic habitats beyond national jurisdictions (the high seas), and limited information on their at-sea distributions and foraging ecology pose several management challenges. Here, we examined the foraging distributions and habitat preferences of three gadfly petrels that breed on Phillip Island (Norfolk Island Group), in the southwest Pacific Ocean, and tested the ability of species distribution models (SDMs) to predict important marine habitats. GPS loggers were deployed in 2018 and 2019 on chick-provisioning black-winged petrels (P. nigripennis) and white-necked petrels (P. cervicalis) and in 2020 on Kermadec petrels (P. neglecta), and hidden Markov models (HMMs) were used to estimate behavioral states across 387 foraging trips. SDMs were built using six algorithms and the predictive performance of models constructed using conventional random cross-validation (CV) was compared to those constructed with spatially independent CV. All three species demonstrated dual-foraging strategies with short trips closer to the colony and longer, presumably self-provisioning, trips with maximum distances from the colony of several thousand kilometers for black-winged and white-necked petrels. Foraging areas of each species were distinctly partitioned across the Tasman Sea during long trips, but there was high overlap during short trips. Black-winged and white-necked petrels exhibited area-restricted search foraging behavior throughout their foraging ranges which spanned almost the entire Tasman Sea and into the western Pacific, whereas the foraging range of Kermadec petrels was restricted closer to the colony. Approximately half of each species’ foraging range extended into the high seas. Response curves and variable importance between the two SDM CV approaches were similar, suggesting that model fitting was robust to the CV approach. However, evaluation using spatially independent CV indicated that generalizability of ensemble SDMs to new data ranged from poor to fair for all three species. This suggests that the maximal-area foraging strategy of gadfly petrels (whereby they search opportunistically for resources across expansive oceanic habitats) results in weak or wide associations with environmental features making predicting important habitats extremely challenging.
1 Introduction
Seabirds are top predators that play important functional roles in marine and terrestrial ecosystems (Stapp et al., 1999; Sánchez-Piñero and Polis, 2000; Smith et al., 2011; Graham et al., 2018) and are widely recognized as valuable bioindicators of changes in the state of ocean ecosystems (Diamond and Devlin, 2003; Boyd et al., 2006; Parsons et al., 2008). Despite their ecological importance, seabirds are among the most threatened groups of birds with many populations having undergone rapid declines over recent decades (Croxall et al., 2012; Paleczny et al., 2015). Seabird conservation is often confounded by a lack of basic information about life histories and distributions at sea. This is especially true of seabirds in the tropics and subtropics (Bernard et al., 2021), regions which support diverse seabird communities, and which have received little research effort (Mott and Clarke, 2018). There is a particular lack of information about at-sea distributions for many South Pacific seabird taxa, despite this region representing the largest expanse of ocean on Earth (Croxall et al., 2012; Rodríguez et al., 2019). Such fundamental ecological knowledge is key to achieving effective conservation and enables researchers and policy makers to more accurately identify current and future threats (Burger and Shaffer, 2008; Lescroël et al., 2016; Bernard et al., 2021).
While the lack of information on many seabirds persists, anthropogenic pressures continue to degrade marine habitats and transform the integrity and stability of marine ecosystems, including the extent, availability, and predictability of prey resources for marine predators (Halpern et al., 2008; Hoegh-Guldberg and Bruno, 2010; McCauley et al., 2015). Obtaining information about the foraging distributions of wide-ranging seabirds has historically been logistically and financially challenging and biased towards shipboard surveys in coastal or nearshore habitats. Furthermore, studies using tracking technologies have been restricted by device sizes that could be deployed only on larger-bodied species (Burger and Shaffer, 2008). However, recent advancement in electronic tracking technology has led to miniaturized devices and transformed what can be learned about the ecology and distributions of even the smallest of seabirds (Nathan et al., 2008; Block et al., 2011; Halpin et al., 2018; Fischer et al., 2021). Furthermore, the advancement of the biologging field presents new opportunities to investigate the importance of seabird habitat on the high seas (i.e., marine areas extending beyond the 200 nautical mile limit of countries’ Exclusive Economic Zones) (Beal et al., 2021; Davies et al., 2021). Although the high seas are classically considered important for migratory seabirds (Harrison et al., 2018), for many wide-ranging species, particularly those in the order Procellariiformes, the high seas also represent critical foraging habitat during breeding stages (Ramírez et al., 2013; Clay et al., 2017; Ramos et al., 2017). Although a lack of global coordination for managing the high seas makes conservation challenging, growing awareness of the need for a global governance framework presents opportunities to implement appropriately planned conservation measures in the near future (Davies et al., 2021).
Procellariform seabirds in the genus Pterodroma (“gadfly petrels”) are among the most threatened of all seabirds (Croxall et al., 2012). Yet, there is often a lack of basic information about their biology, foraging behavior and at sea distributions (Rodríguez et al., 2019). Gadfly petrels are colonial-breeding species that generally nest on isolated oceanic islands (Warham, 1996; Brooke, 2004). These long-lived, monogamous, and strongly philopatric seabirds exhibit low fecundity, raising one altricial offspring per breeding season over an extended nesting period (Warham, 1996; Brooke, 2004). Gadfly petrels are highly pelagic, undertaking long foraging journeys over vast oceanic areas during both reproductive and migratory life stages (Rayner et al., 2012; Ramírez et al., 2013; Priddel et al., 2014; Rayner et al., 2016; Ramos et al., 2016; Clay et al., 2017; Ramos et al., 2017; Ventura et al., 2020). Among seabirds, gadfly petrels have especially high aspect ratio relative to wing loading, which makes them especially well-adapted for optimal use of wind conditions, enabling fast and efficient flight with low energetic costs (Spear and Ainley, 1997; Ventura et al., 2020). Although there is some evidence that gadfly petrels forage around oceanographic features such as seamounts (Rayner et al., 2012) and frontal regions (Rayner et al., 2008), the few existing studies on gadfly petrels that use high resolution GPS tracking (e.g., Ventura et al., 2020; Raine et al., 2021) tend to demonstrate that these species often do not have distinct preferences for, or rely completely on, one or two static or dynamic oceanographic features when foraging and make some of the longest foraging trips in the animal kingdom (Clay et al., 2019; Taylor et al., 2020; Ventura et al., 2020). Through optimal use of ocean basin-scale prevailing wind patterns, gadfly petrels appear to adopt a maximal-area foraging strategy to cover extremely large areas thereby increasing their chances of encountering food resources (Adams and Flora, 2010; Ventura et al., 2020). For example, Ventura et al. (2020) demonstrated that Desertas petrels (Pterodroma deserta) do not concentrate foraging in highly productive regions with predictable resources, and Clay et al. (2017) established that Murphy’s petrels (P. ultima) do not have clear preferences for oceanographic or topographic features.
Here, we present the first Global Positioning System (GPS) tracking datasets for three species of gadfly petrel: black-winged petrel (P. nigripennis; IUCN status: Least Concern), white-necked petrel (P. cervicalis; IUCN status: Vulnerable) and Kermadec petrel (P. neglecta; IUCN status: Least Concern). We sought to first identify the hitherto unknown foraging behavior and at-sea distributions of these species using GPS loggers over multiple years at a single colony in the South Pacific Ocean. Second, we constructed ensemble species distribution models (SDMs) with three main goals: 1) to determine how these three sympatric species partition the environment when foraging; 2) to test if foraging habitat of the gadfly petrels in the present study could be generalized to new data using a spatially independent model evaluation approach; and 3) to consider how important foraging areas can be identified in the event that SDMs poorly predict foraging habitat in chick-provisioning gadfly petrels.
2 Materials and Methods
2.1 Field Methods
2.1.1 Study Site and Species
Fieldwork was conducted on Phillip Island (29°07’S, 167°57’E, Figure 1). Phillip Island is a small (207 ha) and uninhabited subtropical island in the Norfolk Island Group, an Australian external territory in the South Pacific Ocean. Phillip Island is a globally important colony for seabirds and supports considerable diversity with 13 species breeding there annually. The island is particularly important because it supports breeding populations of four gadfly petrel species. The most abundant of the four species is the black-winged petrel (15,000 – 19,000 breeding pairs; Priddel et al., 2010; N. Carlile, unpublished data). The colony also supports the only Australian population of white-necked petrels [20 – 30 breeding pairs (Halpin et al., 2021)]; one of only two Australian populations of Kermadec petrel [56 breeding pairs (Carlile et al., 2021a; Carlile et al., 2021b)]; and a remnant population of the formerly abundant providence petrel [P. solandri; 10 – 100 breeding pairs (Carlile et al., 2021a; Carlile et al., 2021b)]. Elsewhere, established black-winged petrel breeding colonies occur in New Zealand on the Kermadec Islands, Manawatäwhi/Three Kings (Great King, South West), Motuopao, Motupia, Simmonds, Motukokako (Piercy), East (Whangaokeno), Portland and Chatham Islands and on an islet off Cape Brett; in Australia on Lord Howe Island; in New Caledonia, Tonga and in French Polynesia on the Austral Islands (Gill et al., 2010; Priddel et al., 2010; Miskelly et al., 2019). White-necked petrel colonies occur on the Macauley Island (Kermadec Islands) (Miskelly et al., 2019); and Kermadec petrel colonies occur on the Kermadec Islands, Lord Howe Island; in French Polynesia on the Austral Islands and Tuamotu Islands; in Chile on Easter Island, Juan Fernandez Island and San Ambrosio Island.; and in Mauritius on Round Island (Priddel et al., 2010; Miskelly et al., 2019).
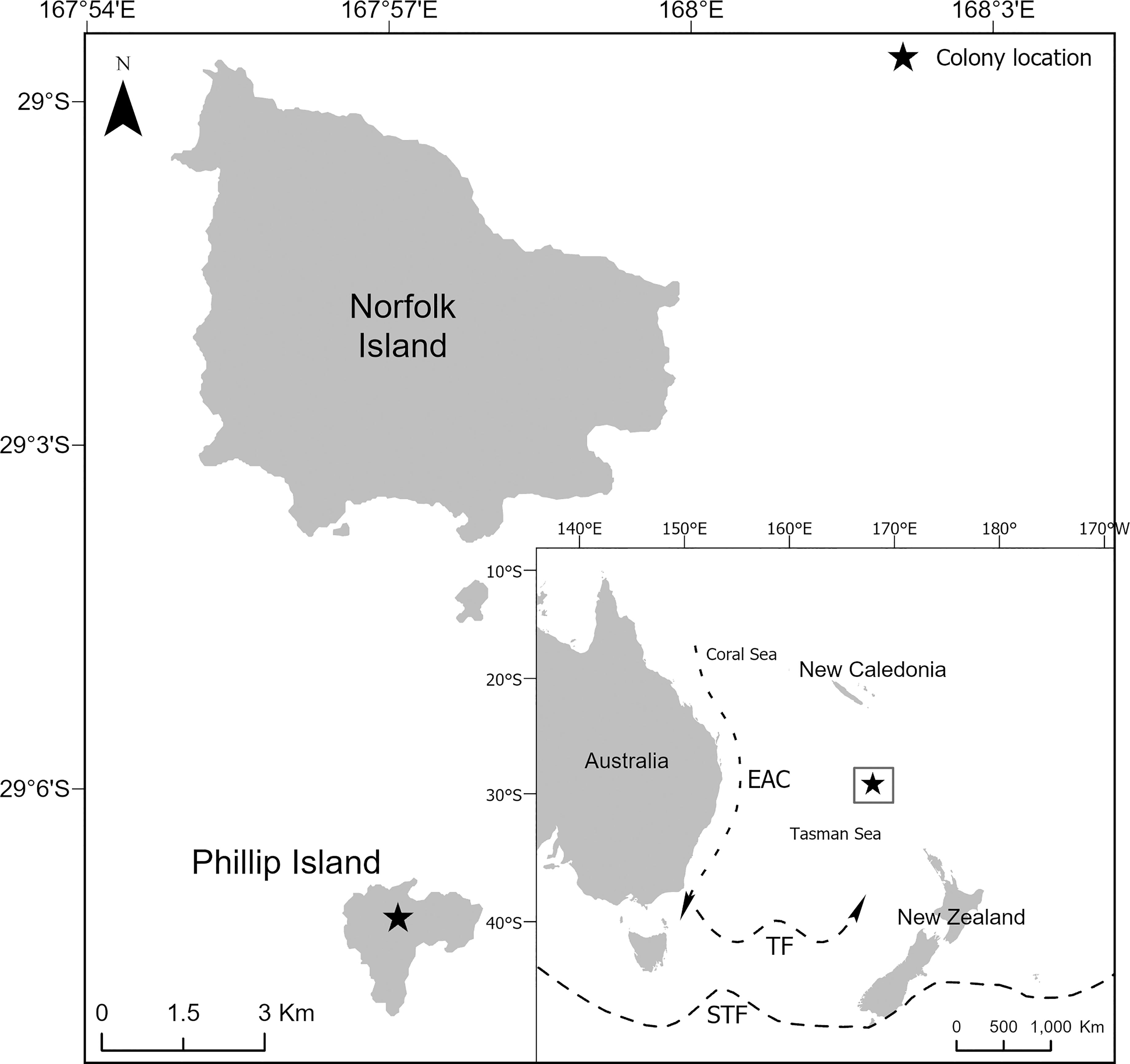
Figure 1 The location of the Norfolk Island Group in relation to Australia, New Zealand and New Caledonia. Included are the approximate locations and direction of flow of the East Australian Current (EAC), the Tasman Front (TF) and the Subtropical Front (STF).
All gadfly petrels produce a maximum of one offspring per year per pair. Adult black-winged petrels weigh on average 171 g (n = 48; this study) and nest predominantly in burrows, but occasionally deep crevices. Breeding occurs on Phillip Island from October to May with chicks hatching approximately in late January and fledging occurring in approximately May (Priddel et al., 2010). Little is known about white-necked petrel breeding biology, however, on Phillip Island adults weigh on average 464 g (n = 27; this study) and generally nest beneath stands of mature white oak (Lagunaria patersonia) among boulders, in rocky crevices, artificial cavities, and occasionally on the ground where dense vegetation cover is present. Breeding occurs on Phillip Island from approximately November to May or June (Priddel et al., 2010). White-necked petrel chicks generally hatch in January and February and fledge approximately in May and June. Adult Kermadec petrels weigh on average 444 g (n = 52; this study) and nest on the ground in sheltered areas between roots of mature white oak trees, or in dense thickets of introduced African olive (Olea europea). More protracted breeding occurs in Kermadec petrels with egg laying occurring from September to May with a peak in November. The population tracked in the present study is the summer-breeding group which breeds approximately from September with chick fledging occurring from March. Black-winged and white-necked petrels are strongly faithful to their nest sites, returning to the same nest site each year, but Kermadec petrels often use different nests within the same general area each year.
2.1.2 Seabird Capture and Sampling
Capture and tracking of gadfly petrels occurred exclusively during the chick-provisioning period for black-winged and white-necked petrels from February to April in 2018 and 2019 and in January 2020 for Kermadec petrels. All tracked birds were provisioning chicks at a similar stage of chick development. Adult birds were captured by hand in their nests during the early to middle chick-provisioning periods from February to April in 2018 and 2019 (black-winged and white-necked petrels) and in January 2020 (summer-breeding Kermadec petrels). Black-winged petrels, were captured by hand at their nests both during the day and at night because they exhibit cathemeral nest site attendance. Due to the unpredictable timing of black-winged petrel nest attendance, we fitted one-way trapdoors to their nest entrances and checked for returning adults approximately every three to four hours while traps were installed. White-necked petrels, which display strictly nocturnal colony attendance, were captured by hand at their nest sites at night. Kermadec petrels, which display crepuscular colony attendance, were captured by hand at nests from early dusk to night.
All three study species are sexually monomorphic. To sex birds we collected 1 – 2 μL of blood from the brachial vein using a sterile 26-gauge needle and whole blood was then placed onto FTA classic cards (Whatman International Ltd., Maidstone, UK). Sex of individuals was determined using PCR reactions following Griffiths et al. (1998) by DNA Solutions (Wantirna, Victoria, Australia). Prior to attachment of GPS loggers, birds were weighed using Pesola scales (± 0.3%, Pesola Präzisionswaagen AG, Switzerland) and fitted with a metal leg band supplied by the Australian Bird and Bat Banding Scheme.
We fitted petrels with custom Pathtrack Nanofix© archival GPS loggers (Pathtrack Ltd., Otley, United Kingdom) programmed to record position data every ten minutes and attached them to the two central rectrices using Tesa© tape (4651, Tesa Tape Inc., Charlotte, NC, USA). We fitted GPS loggers to 27 black-winged petrels in 2018 and 25 in 2019. In 2018, one GPS logger failed to record data, one was lost from the bird, and one was not retrieved before our departure from the colony. In 2019, one GPS logger was not retrieved before our departure from the colony. In 2018 we fitted 13 GPS loggers to white-necked petrels and 16 in 2019. One GPS logger in each year was not retrieved before our departure from the colony. In 2020 we deployed 9 GPS loggers on Kermadec petrels. All GPS loggers were retrieved. GPS loggers weighed an average of 3.23 ± 0.07 (g ± SD; range: 3.1–3.38 g; n = 34). Average percentages of body mass for GPS loggers were 1.88% for black-winged petrels (range: 1.22–2.32%; n = 48), 0.70% for white-necked petrels (range: 0.59–0.88%; n = 27), and 0.72% for Kermadec petrels (range: 0.64–0.88%; n = 9), lower than the 3% threshold that is thought to negatively affect procellariform seabirds (Phillips et al., 2003). The average duration (days ± sd) between GPS logger attachment and removal on individuals was 14.6 ± 9.2, 27.9 ± 11 and 12.1 ± 4.9, respectively, for black-winged, white-necked and Kermadec petrels.
2.2 Data Processing and Analysis
2.2.1 GPS Tracking Data
All data were processed in the R programming language, version 4.1.2 (R Core Team, 2021), and spatial measurements were calculated on the World Geodetic System (WGS 1984) ellipsoid. Maps were produced in ArcGIS Pro (version 2.4.0; ESRI Inc., CA, United States) and data were projected in the Lambert Azimuthal Equal Area projection, centered on the breeding colony. GPS data were filtered to remove erroneous locations where successive relocations would require flight velocities exceeding 27.8 m.s-1 (100 km.h–1) (Lascelles et al., 2016). GPS tracks were linearly interpolated using the package adehabitatLT (version 0.3.25; Calenge, 2006) by resampling all locations to an equal 10 min interval. We gap-filled GPS tracks except when periods of more than 1 hour occurred between location fixes.
2.2.2 At-Sea Distribution and Behavior
To determine foraging characteristics, we split tracking data into individual foraging trips originating from and returning to the colony using the ‘tripSplit’ function in the package, track2KBA (version 1.0.1; Beal et al., 2021). For each complete foraging trip, we used the ‘tripSummary’ function in track2KBA to calculate the duration (days) from departure to return to the colony and the maximum distance from the colony (foraging range, km). We tested for differences in foraging trip duration and maximum distance from the colony between species in complete foraging trips using linear mixed effects models in the lme4 package (Bates et al., 2015) and a post-hoc Tukey’s HSD test for multiple comparisons. To account for repeated trips made by the same individual we included individual identity as a random effect. We considered that sex-related differences in foraging behaviors would be highly unlikely because birds were tracked exclusively during chick-provisioning. Gadfly petrels share parental duties equally, with sex-related differences in foraging absent in chick-provisioning adults (Pinet et al., 2012; Clay et al., 2017). Nonetheless, we tested for intraspecific sex-related differences in maximum foraging range and trip duration using linear mixed effects models using the package lme4 with individual identities as random effects to account for repeated trips made by the same individual.
To identify important at-sea areas for each species, we first calculated the spatial scale of area-restricted search (ARS) using the function ‘findScale’ in the package track2KBA (Lascelles et al., 2016; Beal et al., 2021) for black-winged petrels (18 km), white-necked petrels (17 km) and Kermadec petrels (18 km). We then computed 50% kernel utilization distributions using the R package adehabitatHR (Calenge, 2006) and used the scale of each species’ ARS as the kernel smoothing parameter (h). Following previous studies, we define the 50% utilization distribution as the ‘core’ foraging area where birds spent 50% of their time (Ford and Krumme, 1979; Soanes et al., 2013; Lascelles et al., 2016). We estimated the representativeness of each species’ core foraging area as a function of sample size to ensure that data were sufficiently representative of the foraging distributions of the colony-level populations. To do this we used the bootstrapping approach described in Lascelles et al. (2016) using the function ‘repAssess’ in the track2KBA package (Beal et al., 2021). We classified foraging trips according to whether they were short or long. To do this, we first qualitatively determined the distance classifications for each species by examining histograms of the frequency distribution of maximum trip distances (Figure 2). We then classified short trips as those with a maximum distance from the colony of < 1000 km for black-winged petrels; < 500 km for white-necked petrels and < 200 km for Kermadec petrels. We then produced a map of the short and long trips to demonstrate bimodal foraging strategies exhibited by each species.
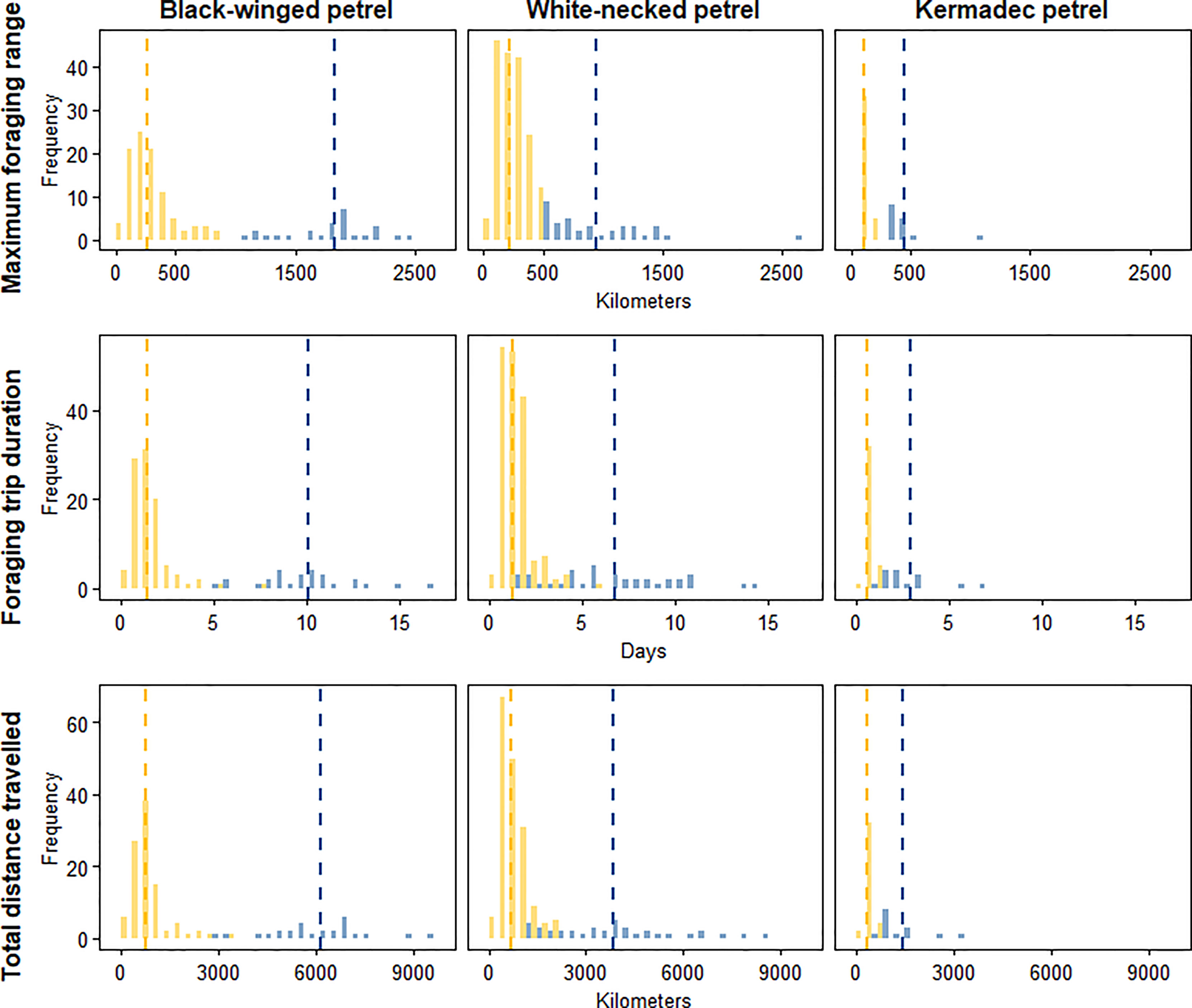
Figure 2 Frequency distributions of maximum foraging range (km; upper row), duration of foraging trips (days; center row) and total distance traveled (km; lower row) for short trips (yellow) and long trips (blue) for black-winged petrels (left column), white-necked petrels (center column) and Kermadec petrels (right column). The dashed lines represent the mean of the frequency for short trips (yellow) and long trips (blue).
To distinguish the behavioral states of individuals during their foraging trips, we used hidden Markov models (HMMs). For each species, we fitted a three-state HMM to the interpolated GPS tracks using the momentuHMM package (version 1.5.4; McClintock and Michelot, 2018). Following Clay et al. (2020), to determine choice of initial values for step length and turning angle distributions, we selected these values randomly 100 times from within a range of biologically plausible values, then determined the most appropriate values as those that were closest to the most frequent estimation. We then estimated three behavioral states in the GPS tracks using two input variables: step lengths and turning angles. We considered directed flight (i.e., travel) to be associated with high flight speeds and shallow turning angles, area-restricted search (i.e., foraging) to be associated with moderate flight speeds and moderate to wide turning angles; and rest associated with low speeds and shallow to moderate turning angles. We used a gamma distribution for step lengths and a von Mises distribution for turning angles. We used the Viterbi algorithm in the package momentuHMM to estimate the most likely sequence of behavioral states from fitted models (Rabiner, 1989). Performance of the HMM behavioral state assignment was assessed by examining histograms of step lengths and turning angles for each species (Supplementary Figures 1–3), and each track was then visually assessed to ensure that state-space assignments were plausible. For each trip we calculated the percentage of time (% ± SD) that birds spent in each behavioral state and averaged the proportion across all trips to understand activity budgets. To test for differences between species in the proportion of time spent in ARS, we performed a one-way ANOVA test and a post-hoc Tukey’s HSD test for multiple comparisons. For each trip we also calculated the average duration (hours ± SD) that birds spent in each behavioral state before switching to another state during a foraging trip. To determine whether nocturnal foraging might be an important feature of the species we tracked, we tested for differences in the proportion of time that each species spent foraging in daylight versus darkness using Student’s t-tests.
Lastly, to evaluate the extent of foraging across the species’ ranges we mapped the occurrence of locations inferred to be foraging (i.e., area-restricted search) by the HMMs on a 50 x 50 km grid cell size for each year of tracking and, for black-winged and white-necked petrels, which were tracked in more than one year, we overlaid gridded rasters to produce maps showing repeated grid cell use between years.
2.2.3 Species Distribution Modelling
We used SDMs to both characterize important foraging habitats for gadfly petrels and to assess the generalizability of models between spatial regions. We modeled the foraging locations identified by the HMMs against a suite of environmental predictors (Table 1). Environmental predictors were selected based on ecological knowledge of the drivers of subtropical pelagic seabirds’ foraging activity (Ballance et al., 2006; Hyrenbach et al., 2006; Ramos et al., 2015; Clay et al., 2017; Miller et al., 2018; Waugh et al., 2018). We used three static environmental predictors and seven dynamic environmental predictors in our models (Table 1). Static predictors were: 1) the bathymetric slope, represented in degrees, and 2) distance (km) to seamounts and knolls as indicative of shelf-breaks and bathymetric features that could represent productive areas of upwelling; and 3) transit distance (km) from the colony as a proxy for the importance of accessibility to foraging habitat given that all birds were centrally-placed (i.e., had an intrinsic requirement to regularly return to the colony to provision nestlings). The seven dynamic oceanographic variables were: 1) sea surface temperature (°C), and 2) sea surface temperature fronts measured by calculating the standard deviation of the sea surface temperature (°C) within a 10 km buffer of the original 1 km resolution of the sea surface temperature grid as an indicator of frontal regions; 3) sea level anomaly (m) as an indicator of mesoscale eddies and ridges; 4) wind speed (m.s-1) as an indicator of optimal transit conditions for gadfly petrels (i.e., Ventura et al., 2020); 5) chlorophyll a concentration (mg.m-3) as a proxy for ocean productivity; 6) the finite-size Lyapunov exponent (FSLE; days-1) to test the preference of birds for foraging in sub-mesoscale transport fronts; and 7) the depth of the thermocline layer (m). FSLE is based on Lagrangian reanalysis of satellite altimetry-derived surface currents (d’Ovidio et al., 2004; Cotté et al., 2015). Further information on the original spatial resolution and sources of environmental variables is provided in Table 1. Predictor variable rasters were scaled to a 1 × 1 km grid cell resolution using bilinear interpolation and were projected in the Lambert Azimuthal Equal Area projection centered on the breeding colony. Predictor variables were standardized using the ‘scale’ function in the raster package (Hijmans and van Etten, 2021) to improve the spread of the data. We applied a variance inflation factor test to ensure that predictor variables were not collinear and found no collinearity problems among the ten predictor variables.
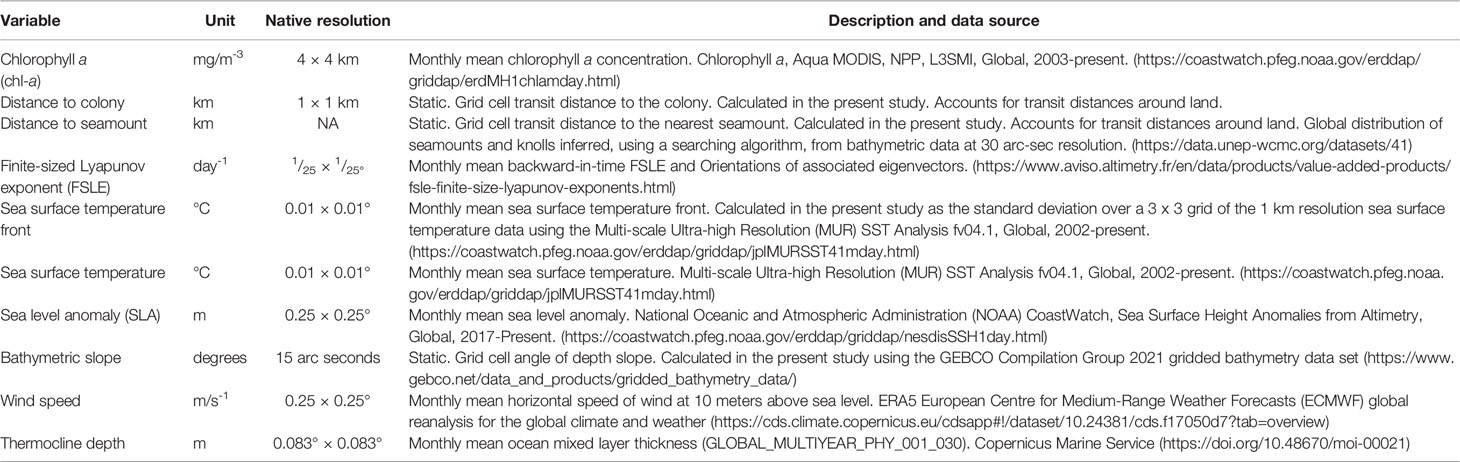
Table 1 Static and dynamic oceanographic variables, resolutions, measurement units and sources used in models to predict the foraging habitat of gadfly petrels.
We used a multi-model ensemble approach to test whether the environmental features could predict gadfly petrel foraging habitat. Using the biomod2 package (version 3.5.1; Thuiller et al., 2009; Thuiller et al., 2021) we fitted six algorithm types: Artificial Neural Networks (ANN), Classification Tree Analysis (CTA), Generalized Additive Models (GAM), Generalized Boosted Models (GBM), Multiple Adaptive Regression Splines (MARS) and Random Forests (RF). We used the default model parameters in biomod2 for all models except GBM where the bag fraction was set to 0.75 and maximum number of trees was set to 3000 (e.g., Elith et al., 2008). GAMs were specified to use Restricted Maximum Likelihood (REML); and the number of learning trees in Random Forests was set to 1000. We modeled the GPS locations that were inferred by HMMs to be foraging behavior as the response variable. For each foraging location we randomly sampled a single matched pseudo-absence because a ratio of 1:1 presence:pseudo-absence is most suited to the learning algorithms that we used to model the characteristics of foraging habitat (Barbet-Massin et al., 2012). Pseudo-absences were generated within foraging extents, which we separately defined for each species, by computing a minimum convex polygon around all available tracking locations.
To evaluate the ability of the models to predict the probability of foraging on new data, we compared two approaches, 1) conventional random K-fold cross-validation (CV) with 80% of each species’ data for model fitting and the remaining 20% for testing (Elith et al., 2008) and 2) spatially independent K-fold CV (Roberts et al., 2017) using the blockCV package (Valavi et al., 2019). The latter approach to assessing model predictive performance is more robust because it ensures spatial independence of testing and training data thereby accounting for spatial structure in cross-validation data. Conventional random selection of training and testing folds that are not spatially independent commonly leads to underestimated error in spatial predictions and overestimated model performance and predictive power (Telford and Birks, 2009; Roberts et al., 2017; Hao et al., 2019; Valavi et al., 2019). Five folds were specified for both approaches. Spatially-independent CV used a spatial blocking size of 800 km for black-winged and white-necked petrels but had to be reduced to 250 km for Kermadec petrels due to their much smaller range size. Spatial blocks were randomly assigned using 200 iterations to find evenly dispersed folds and were generated based on the extent of each species’ foraging range. Block size was chosen as the approximate range over which observations become spatially independent and was determined by constructing empirical variograms using the function ‘spatialAutoRange’ in the package blockCV. Predictive performance of both approaches was then evaluated using the True Skill Statistic (TSS) and Area Under the Receiver Operating Characteristic curve (AUCROC). AUCROC is a widely used measure of a model’s predictive performance and its ability to differentiate presence and absence locations (Lobo et al., 2008). AUCROC values and associated performance evaluations range from 0 to 1, with excellent AUCROC: > 0.90; good AUCROC: > 0.80 ≤ 0.90; fair AUCROC: > 0.70 ≤ 0.80; poor AUCROC: > 0.60 ≤ 0.70; very poor AUCROC: > 0.50 ≤ 0.60; and AUCROC: ≤ 0.50 indicating predictive performance that is no better than random (Hosmer et al., 2013). We averaged the respective TSS and AUCROC values across model folds for each algorithm type and model. Due to the robust spatial independence of the blocked cross-validation approach we took the model evaluation metrics of this method as superior to those of conventional K-fold random CV models. We therefore interpreted the AUCROC values of the spatially blocked models as the more statistically credible approximation of model generalizability to new data for gadfly petrels.
Total consensus ensemble models were constructed based on all models for each CV method (so that fair comparisons between the performance of formal and ensemble models could be made). The conventional CV ensemble was built with an evaluation metric threshold applied such that models with AUCROC < 0.8 were excluded, but no evaluation metric threshold was applied to the spatial blocking ensemble due to the inherently low AUCROC values. Species-specific ensemble model response curves were constructed from the spatially blocked models and calculated as the median response across 10 replicate model runs within the biomod2 package using the algorithm-independent evaluation strip method following Elith et al. (2005), which facilitates direct comparison of predicted responses from different statistical approaches on the same data.
We used multivariate environmental similarity surfaces (MESS; Elith et al., 2010) to check that differences in behavior of Kermadec petrels were not likely to have been caused by divergent environmental conditions in the year that they were tracked (January 2020) versus the years that black-winged and white-necked petrels were simultaneously tracked (February to April in 2018 and 2019). We computed MESS grids for each dynamic predictor for each month in which black-winged and white-necked petrels were tracked and used the overall MESS grid to assess the percentage of cells with negative values (cells with negative values represent those with conditions outside of the range present in the reference time period).
3 Results
Over three breeding seasons, we obtained 387 complete foraging trips from 80 individuals of three species of sympatrically breeding gadfly petrels (Table 2). Core foraging areas (i.e., 50% utilization distributions) used by the sampled individuals were estimated to be highly representative of the core foraging areas of the respective colony-level populations for black-winged petrels (87.5%) and white-necked petrels (93.5%), but representativeness was lower for Kermadec petrels (68.5%) due to the lower sample size.
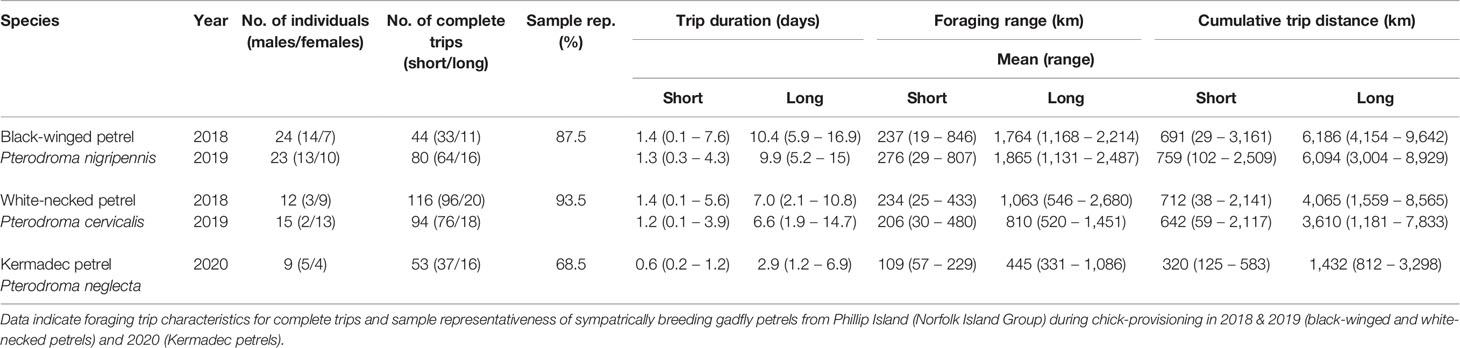
Table 2 Summary of the GPS tracking dataset for birds that returned data (i.e., tracking device was recovered and remained functional for at least one trip).
3.1 Foraging Characteristics
We found no sex-related differences in maximum foraging ranges and durations of trips made by black-winged petrels (foraging range: F122 = 0.738, p = 0.541; trip duration: F122 = 0.613, p = 0.462), white-necked petrels (foraging range: F208 = 0.533, p = 0.595; trip duration: F208 = 0.198, p = 0.843) and Kermadec petrels (foraging range: F6.1 = -0.773, p = 0.469; trip duration: F5.8 = -0.59, p = 0.577). Therefore, we pooled the data from both sexes within each species. When accounting for random effects of individuals making repeated trips, maximum foraging ranges differed between black-winged and white-necked petrels and black-winged and Kermadec petrels (Tukey’s post-hoc test: p < 0.001 for both relationships; Table 2), but not between white-necked and Kermadec petrels (Tukey’s post-hoc test: p = 0.098). Similarly, the duration of foraging trips was different between black-winged and white-necked petrels (Tukey’s post-hoc test: p = 0.0105) and between black-winged and Kermadec petrels (Tukey’s post-hoc test: p < 0.001), but not between white-necked and Kermadec petrels (Tukey’s post-hoc test: p = 0.0679). Tracking data also indicated that all three species exhibited bimodal foraging strategies, alternating between short and long foraging trips (Figures 2, 3).
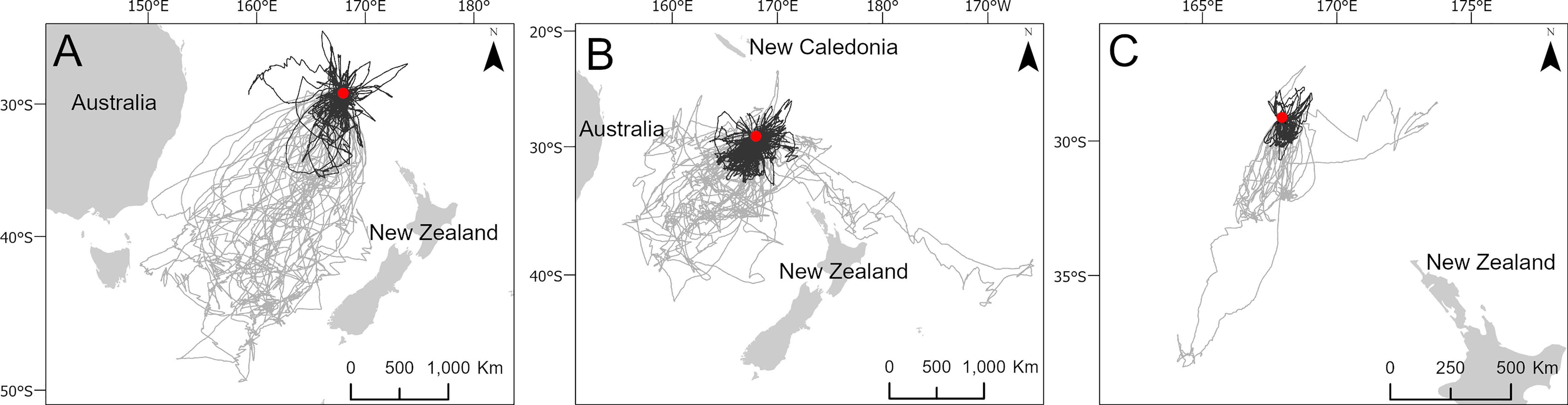
Figure 3 Maps demonstrating the short (black) and long (grey) foraging trips of black-winged petrel (A), white-necked petrel (B) and Kermadec petrel (C) bimodal foraging strategies. The colony location is indicated by a red circle. The number of trips in each category are detailed in Table 2.
Behavioral state space modeling revealed that although all three species demonstrated area-restricted search foraging behavior throughout the entire range of their breeding distributions, they tended to concentrate foraging activity nearer (< 500 km) the colony (Figures 4–6). On average all species spent more than half of their time traveling, and a relatively small proportion of their time resting during foraging trips (Table 3). We found significant between-species differences in the proportion of time spent in ARS behavior (F2 = 10.98, p = < 0.001). Black-winged petrels spent proportionally less time in ARS than white-necked (p < 0.001, 95% C.I. = [0.02 – 0.09]) and Kermadec petrels (p < 0.001, 95% C.I. = [0.04 – 0.14]), but there was no difference between white-necked and Kermadec petrels (p = 0.2, 95% C.I. = [-0.08 – 0.01]). We found no significant difference in the proportion of time spent foraging during daylight and darkness in black-winged petrels (daylight: 30.4 ± 14.2, darkness: 28.6 ± 17.1; t-test: t(258) = -0.986, p = 0.325) and Kermadec (daylight: 37.9 ± 17.7, darkness: 37.8 ± 20.8; t-test: t(102) = -0.013, p = 0.989), but found that white-necked petrels spent proportionally more time foraging during daylight (daylight: 40.4 ± 15.4, darkness: 28.5 ± 21.2; t-test: t(400) = -6.72, p < 0.001).
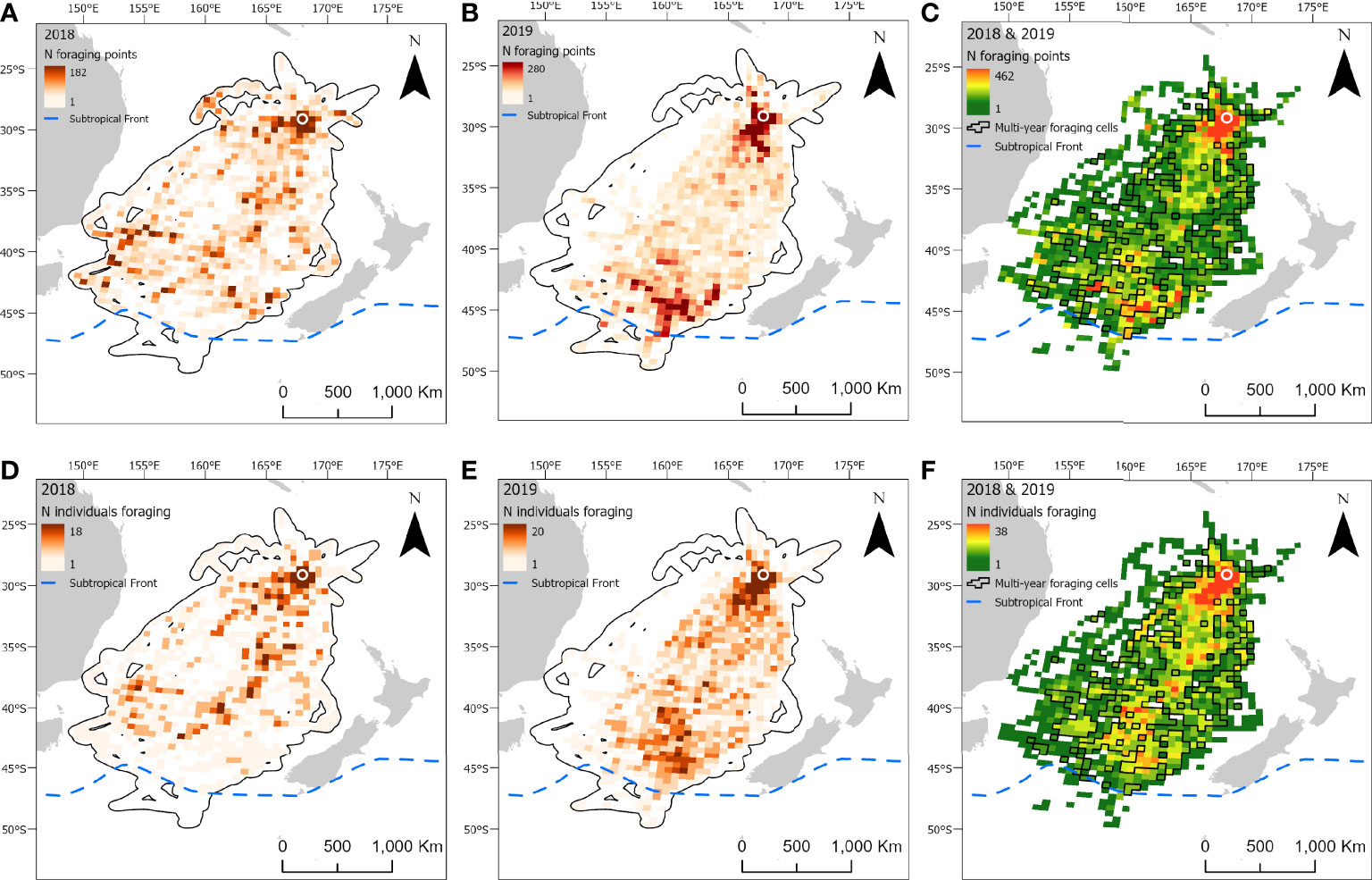
Figure 4 At-sea distribution and locations of foraging behavior in chick-provisioning black-winged petrels (Pterodroma nigripennis). Grid cells (50 x 50 km) depict the number of foraging locations in 2018 (A; n = 24 individuals) and 2019 (B; n = 24 individuals) and combined number of foraging locations in 2018 and 2019 (C). Number of individuals foraging in each grid cell in 2018 (D) and 2019 (E); and the combined number of individuals foraging in each grid cell in both 2018 and 2019 (F). Polygonised cells in C and F represent grid cells in which birds foraged in both years. The location of the breeding colony is represented by a white circle. Black polygons around the foraging areas (A, B, D, E) represent the home range (99% utilization distribution). The approximate location of the Subtropical Front is represented by a dashed blue line.
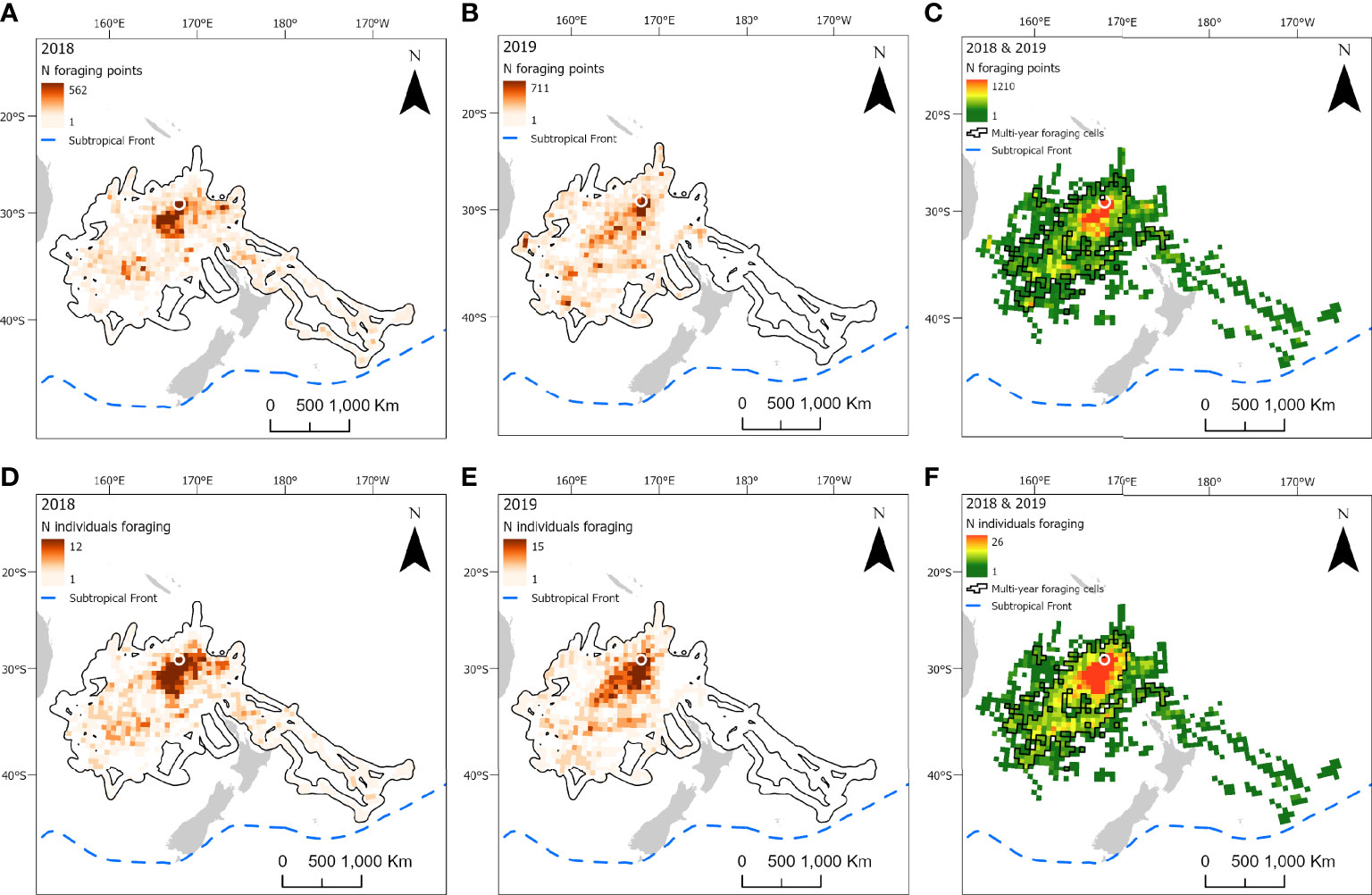
Figure 5 At-sea distribution and locations of foraging behavior in chick-provisioning white-necked petrels (Pterodroma cervicalis). Grid cells (50 x 50 km) represent the number of foraging locations in 2018 (A; n = 12 individuals) and 2019 (B; n = 15 individuals) and combined number of foraging locations in 2018 and 2019 (C). Number of individuals foraging in each grid cell in 2018 (D) and 2019 (E); and the combined number of individuals foraging in each grid cell in both 2018 and 2019 (F). Polygonised cells in C and F represent grid cells in which birds foraged in both years. The location of the breeding colony is represented by a white circle. Black polygons around the foraging areas (A, B, D, E) represent the home range (99% utilization distribution). The approximate location of the Subtropical Front is represented by a dashed blue line.
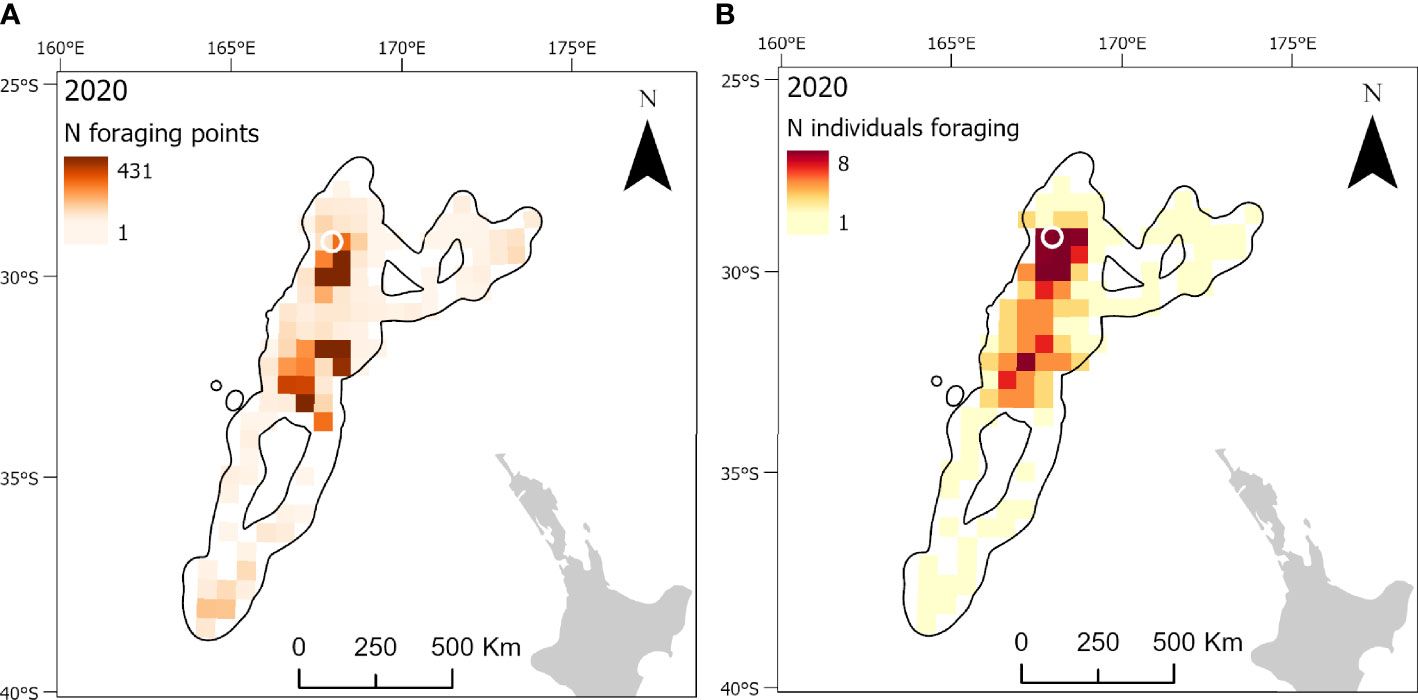
Figure 6 At-sea distribution and locations of foraging behavior in chick-provisioning Kermadec petrels (Pterodroma neglecta). Grid cells (50 x 50 km) represent the number of foraging locations in each cell in 2020 (A; n = 9 individuals) and the number of individuals foraging in each grid cell in 2020 (B). The location of the breeding colony is represented by a white circle. Black polygons around the foraging areas represent the home range (99% utilization distribution).
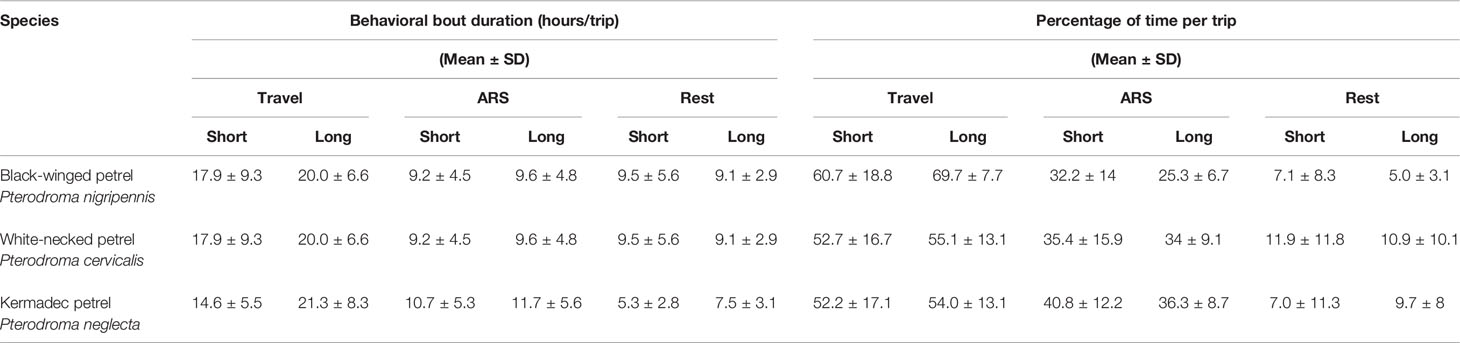
Table 3 Average duration and percentage that gadfly petrels spent in travel, area-restricted search (ARS) and rest during foraging trips. Behavioral states were inferred from hidden Markov models (HMMs).
3.2 At-Sea Foraging Distribution
Foraging ranges extended over vast oceanic areas including, for black-winged (Figure 4) and white-necked petrels (Figure 5), almost throughout the entirety of the Tasman Sea and, to a lesser extent, at the southern limits of the Coral Sea at its 30° south limit. Approximately 55%, 35% and 42%, respectively, of black-winged, white-necked and Kermadec petrel area-restricted search foraging locations occurred in international waters (outside of EEZs). All three species foraged broadly within the warm waters of the wind-driven, southward flowing East Australian Current (EAC) and to the southern extent of the eastward-flowing Tasman Front (TF), which separates the Coral Sea to the north and the Tasman Sea to the south and flows from the east coast of Australia into the western Pacific Ocean. Black-winged petrels made use of areas bordering the Subtropical Frontal Zone in the south of the Tasman Sea and foraged over an area of almost 4 million km2, white-necked petrels over approximately 5 million km2 including to the east of New Zealand bordering the Subtropical Frontal Zone, but Kermadec petrels (Figure 6) remained relatively close to the colony with a foraging range that spanned almost 0.5 million km2. Grid cell analysis of foraging behavior revealed that gadfly petrels engaged in area-restricted search behavior throughout their entire foraging ranges. However, most grid cells occupied by foraging black-winged petrels on long trips were concentrated in the south of the Tasman Sea (Figure 4). Foraging ranges of both black-winged and white-necked petrels were larger in 2018 than in 2019 (Figures 4, 5). There was a high degree of overlap in foraging ranges of all three species, but black-winged petrels appeared to concentrate their long foraging trips considerably further south than the other species (Figures 4–6).
3.3 Species Distribution Modeling
Evaluation metrics for habitat models constructed with spatially independent CV were substantially poorer (AUCROC of 0.55 – 0.77; Table 4) than of models fit using conventional random CV (AUCROC of 0.93 – 0.95; Table 4). Despite these apparent differences in model performance, response curves and relative importance of predictor variable contributions of conventional CV and spatially independent CV ensemble models were similar (Supplementary Figures 4, 5) indicating consistent environmental relationships, regardless of the CV method. Both methods are likely to have poor generalizability to new spatial and temporal environment data because the spatially independent CV method is specifically designed to evaluate how well model predictions transfer to new environmental contexts. Ensemble model predictive performance for black-winged petrels was approximately equivalent to random classification (AUCROC = 0.55; TSS = 0.19), and performance was fair for white-necked (AUCROC = 0.71; TSS = 0.39) and Kermadec petrels (AUCROC: = 0.77; TSS = 0.48) (Araujo et al., 2005; Hosmer et al., 2013). Distance from the colony (Figure 7) was the most important predictor, with all three species demonstrating a high probability of foraging closer to the colony. All species showed a preference for foraging in warmer sea-surface temperatures, and for black-winged and white-necked petrels SST was the second most important environment variable for predicting the location of foraging. Black-winged and white-necked petrels preferred to forage in areas with higher wind speeds (albeit wind speed had only low (3.1%) variable importance for white-necked petrels), but Kermadec petrels preferred lower wind speeds (Figure 7). Models presented some evidence that black-winged petrels expressed preference for foraging in areas with shallower thermocline depths (i.e., 9.9% variable importance; Figure 7), whereas white-necked petrel foraging was associated with deeper thermocline depths (i.e., 6% variable importance; Figure 7). Thermocline depth had weaker contribution to the Kermadec petrel models (i.e., 3.1% variable importance) with no obvious preference for foraging in waters with shallow or deep thermocline layers.
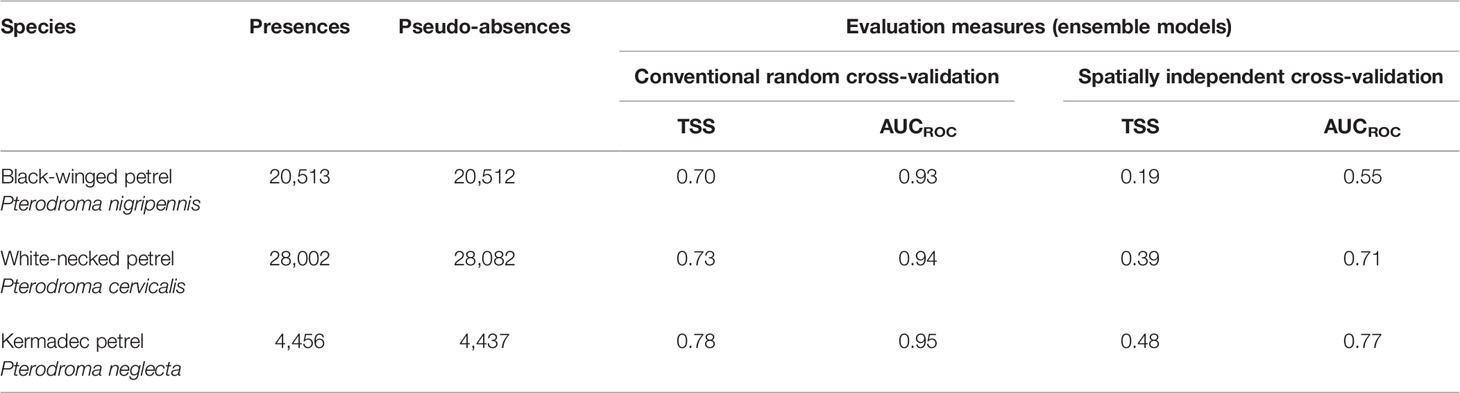
Table 4 Number of presences and pseudo-absences used to build ensemble species distribution models (SDM) and the average values of fivefold conventional random cross-validation and spatially independent cross-validation for the two calculated model evaluation measures including true skill statistic (TSS) and area under the receiver operating characteristic curve (AUCROC).
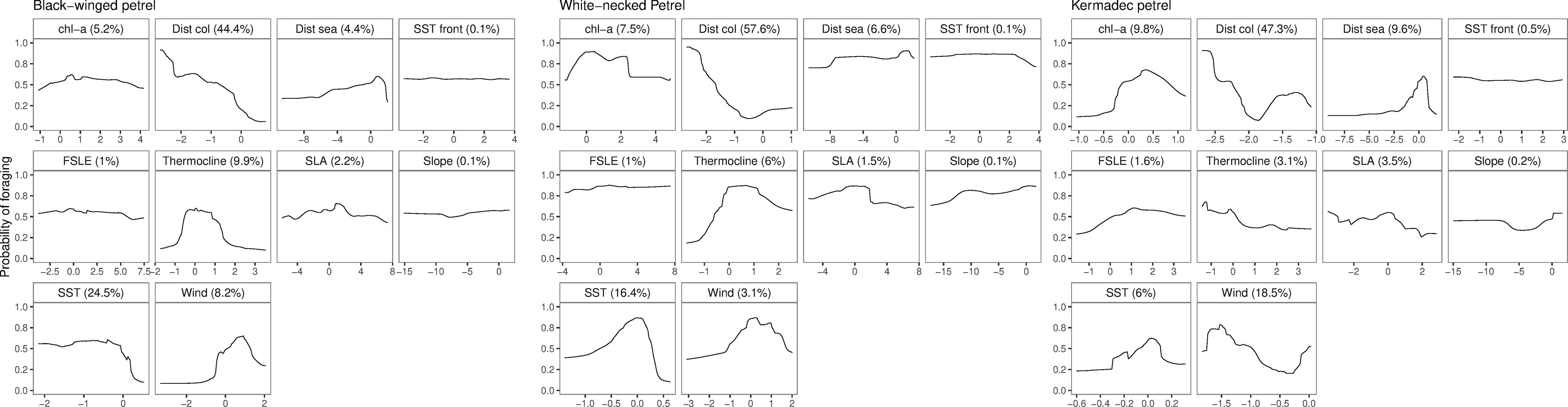
Figure 7 Response curves of scaled predictors and associated predictor importance in the spatially independent cross-validation ensemble models of black-winged (left), white-necked (center) and Kermadec (right) petrel foraging preferences. Predictor variables include chlorophyll a concentration (chl-a), distance from the colony (Dist col), distance from seamounts (Dist sea), sea-surface temperature fronts (SST front), Finite-sized Lyapunov Exponent (FSLE), thermocline depth (Thermocline), sea level anomaly (SLA), bathymetric slope (Slope), sea-surface temperature (SST) and wind speed (Wind).
We found no evidence of any of the species showing preferences for foraging close to seamounts. Conversely, the probability of foraging was uniformly higher among all species at greater distances from seamounts; and birds did not appear to target bathymetric slopes. Kermadec petrels demonstrated some preference for foraging in areas with higher chlorophyll a concentration compared to black-winged and white-necked petrels. Additionally, for all three petrels, species distribution models could not determine any clear preferences for foraging near sub-mesocale sea-surface temperature fronts, nor transport fronts (i.e., areas with lower FSLE values that represent the outer parts of transport fronts) or areas with higher sea level anomalies. However, black-winged petrels appeared to make some general use to the north of the Subtropical Frontal Zone (Bostock et al., 2015; Figure 4) during long foraging trips. In 2019, only two white-necked petrels traveled to the east of New Zealand, possibly making use of productive waters to the north of the Subtropical Frontal Zone (Figure 5).
MESS grids indicated that only 0.04 ± 0.08% (mean ± SD) of the foraging area during the time period when Kermadec petrels were tracked had environmental conditions outside the range of those during the time period when black-winged and white-necked petrels were tracked (Supplementary Figure 6).
4 Discussion
4.1 Foraging Characteristics
Our tracking data and analyses demonstrate that summer-breeding gadfly petrels from the Norfolk Island Group use waters of the East Australian Current and the Subtropical Frontal Zone. Behavioral state-space modeling demonstrated that while they do use some oceanographic features, they also travel very widely, foraging throughout most of the Tasman Sea, apparently opportunistically exploiting resources as they travel (e.g., Ventura et al., 2020). These results support the hypothesis that, while gadfly petrels do make some broad use of oceanographic features, they also use a maximal-area foraging strategy, travelling over large areas to maximize chances of encountering unpredictable resources in relatively unproductive marine ecosystems (e.g., Clay et al., 2017; Ventura et al., 2020).
Among seabirds, gadfly petrels undertake some of the most extensive foraging movements during breeding when they must travel repeatedly to and from their nests to incubate eggs or provision themselves and their altricial offspring (Clay et al., 2019; Taylor et al., 2020). The three species we studied all demonstrated bimodal foraging strategies (e.g., Weimerskirch et al., 1994) whereby birds made both short and long foraging journeys. Concentrated foraging around seabird colonies depletes local resources, which forces individuals to journey further in search of food: a phenomenon known as ‘Ashmole’s halo’ (Ashmole, 1963; Oppel et al., 2015; Weber et al., 2021). Seabirds exhibiting bimodal foraging strategies do so in response to near-colony resource depletion, whereby short foraging trips are used to provision their dependent young, whereas parents need to travel to more distant foraging grounds to meet their own energetic requirements (Weimerskirch et al., 1994; Oppel et al., 2015; Weber et al., 2021). Gadfly petrels appear exceptionally well suited to making long-distance self-provisioning journeys due to their unique morphological adaptations for fast, and energy efficient flight relative to other seabirds (Spear and Ainley, 1997; Ventura et al., 2020).
4.2 At-Sea Foraging Distributions
Tracking data collected over multiple years highlighted that some areas experience more intensive foraging than others. This is true for both the number of individuals foraging in a given region as well as the intensity of foraging activity of individuals within that region. All three species had high foraging activity immediately surrounding the colony, with other hotspots of foraging activity relatively diffusely spread over the remaining large foraging extent, which is typical of other central place foraging seabirds that display dual foraging strategies (Magalhães et al., 2008; Raine et al., 2021). Hotspots of black-winged petrel foraging activity occurred between approximately 40°S – 47°S. The large spatial area over which gadfly petrels foraged encompasses areas of the Exclusive Economic Zones (EEZs) of Australia and New Zealand with a large proportion of foraging ranges encompassing the high seas, a “global commons” for which no country holds sovereign jurisdiction. In this study, approximately 55%, 35% and 42%, respectively, of black-winged, white-necked and Kermadec petrel foraging locations occurred outside of EEZs, which highlights the importance of internationally coordinated efforts to conserve gadfly petrel foraging habitat (e.g., Davies et al., 2021).
We demonstrated a large degree of spatial overlap in foraging areas among all three species, suggesting that competition for resources may be mediated over finer spatial scales through differences in prey acquisition methods, presumably as a result of morphological differences (e.g., body size), instead of large-scale spatial or habitat segregation (Spear et al., 2007). Diet diversity in pelagic tropical seabirds is generally low (Diamond, 1983) with most species restricted to foraging on squid and flying fish, which results in high diet overlap (Ashmole and Ashmole, 1967; Harrison et al., 1983; Ballance et al., 1997). Indeed, observations of stomach regurgitations and of parents feeding young during fieldwork of the present study suggest that the three species provisioned chicks with unidentified squid (Decapodiformes) and flying fish (Exocoetidae) and, in black-winged petrels, unidentified zooplankton (L. Halpin, personal observations, 2018-2021). Kermadec petrels are reported to be partially kleptoparasitic (Spear and Ainley, 1993). That Kermadec petrels foraged closer to the colony and spent on average proportionally more time in area-restricted search behavior may be indicative of a kleptoparasitic foraging strategy, whereby birds do not need to travel far to find prey, but instead may harass other species close to the colony that have acquired food. This species also demonstrated preferences for foraging in areas with higher chlorophyll a concentration, suggesting that within their smaller foraging range, which encompasses more oligotrophic areas, they may need to seek patches with higher productivity to obtain sufficient prey resources. All three species intensively used the areas surrounding the colony in short foraging trips, but during long trips black-winged petrels tended to forage in cooler waters much further south than white-necked petrels. This may be indicative of black-winged petrels having increased flight proficiency relative to white-necked petrels thus enabling them to exploit resources further afield.
4.3 Foraging Habitat
Model predictive performance was generally poor to fair as is often the case for habitat generalists that lack strong affinity to a particular habitat type or feature (Elith et al., 2006; Andrew and Fox, 2020). We believe that the poor predictive performance was due in part to the behavioral characteristics (i.e., more generalist habitat requirements) of gadfly petrels rather than factors such as the choice of environmental variables used to predict foraging habitat. Furthermore, the response curves and relative importance of the predictor variables to model predictions were very similar between conventional cross-validation and spatially independent cross-validation approaches, demonstrating that birds have weak or wide preferences for available habitat, regardless of the cross-validation method. While the predictive performance of the Kermadec petrel species distribution model was within range of what is generally considered acceptable, we suggest that the performance of this model should be taken with caution due to the lower representativeness of the sample size (68.5%) to foraging movements of the colony-level population. MESS grids indicated a general similarity in environmental conditions experienced by Kermadec petrels compared to the other two species. We therefore do not believe that differences between the foraging characteristics of Kermadec petrels compared to the other two species were driven by differences in environmental conditions due to the different time periods in which the species were tracked.
Our results suggest that black-winged petrels target waters with shallower thermocline depths, which may indicate a more varied diet that includes zooplankton since planktivorous species tend to prefer waters with shallower thermocline depths (e.g., Spear et al., 2001; Ballance et al., 2006). Conversely, the preference for foraging in waters with deeper thermoclines expressed by white-necked petrels may indicate that the species is more piscivorous than black-winged and Kermadec petrels. That black-winged and Kermadec petrels spent almost equal proportions of their time foraging during darkness compared to daylight suggests that nocturnal foraging is likely to be an important feature of their behavior. These results are consistent with other studies indicating that tropical and subtropical seabirds often rely on deep water prey that perform diel vertical migrations to the sea surface at night (e.g., Hays, 2003). Such prey includes mesopelagic squid, fish and zooplankton (Ashmole, 1971; Imber et al., 1995; Dias et al., 2012). Similar to a closely related species, the Bonin petrel (Pterodroma hypoleuca; Harrison et al., 1983), black-winged petrels may be well-adapted for night time foraging and may rely substantially on diel migrant prey, possibly including bioluminescent squid (Ommastrephidae; Harrison et al., 1983) and zooplankton. Kermadec petrels are known to feed on diel migrant squids, particularly Onychoteuthidae (Imber et al., 1995).
Previous studies have suggested that, unlike many other pelagic seabirds, gadfly petrels have less clear habitat preferences, especially when engaged in long foraging trips during the breeding season (Clay et al., 2017; Clay et al., 2019; Ventura et al., 2020). Our study provides support for this hypothesis with evidence to suggest that the foraging strategy is likely based on opportunistic prey encounters rather than targeting of specific habitat features. For example, foraging occurred throughout the species ranges, and travel accounted for a disproportionately large percentage of time allocation within foraging trips. Additional support for this hypothesis is that, across all three species, the most important model predictor of probability of foraging behavior was the distance from the colony. From a species distribution modeling perspective, this suggests that, like other centrally placed foragers, breeding gadfly petrels’ foraging ranges are mainly constrained by their need to return to nests to provision chicks.
Possible evolutionary drivers of this lack of habitat specificity are that (sub)tropical waters and open ocean regions are less predictable than temperate and polar regions, and inshore waters. Indeed, it has been hypothesized that as ocean productivity decreases – which results in reduced prey abundance – seabird flight proficiency becomes more important because those with low flight costs will be able to reach more distant resources (Ballance et al., 1997). Furthermore, gadfly petrels’ high mobility and large foraging ranges allows birds to be less reliant on specific oceanographic features associated with moderate to high productivity, such as fronts or eddies, which can be ephemeral and are highly dynamic in marine systems (Weimerskirch, 2007), and instead allows birds to opportunistically target prey items or patches they encounter while in flight.
Gadfly petrels tracked in the present study showed no affinity for foraging near seamounts, similar to Raine et al. (2021). Several studies (e.g., Haney et al., 1995; Thompson, 2007; Morato et al., 2008) demonstrate that some seabirds – including gadfly petrels – occur in higher abundance near seamounts, particularly in the North Pacific and Atlantic. However, these studies have generally occurred during gadfly petrels’ non-breeding stages (i.e., in the austral winter) when birds are not constrained to routinely return to the colony. Given the low energetic costs of long-distance travel, it could be that for chick-provisioning gadfly petrels, targeting foraging at seamount locations yields lower foraging success than ranging widely across the Tasman Sea. Furthermore, little is known about seabird prey distributions in the oceanic Pacific (Ballance et al., 1997). It is thus also possible that the squid and flying fish prey targeted by gadfly petrels during chick-provisioning occur in no higher abundances at accessible seamounts within the species’ foraging ranges than in pelagic waters.
4.4 Implications for Conservation
The opportunistic foraging strategies of gadfly petrels and the enormous areas over which they forage mean that it may be challenging or impractical to use traditional approaches to identifying and protecting key foraging habitats, such as static marine protected areas (Oppel et al., 2018). Conservation of gadfly petrel foraging habitat may therefore require focus on maintaining functional and healthy marine ecosystems and reducing generalized threats (e.g., commercial fisheries that cause high seabird bycatch, marine pollution) to birds in these areas, which will ensure long term food supplies for these oceanic wanderers. Lack of generalizability of habitat suitability models poses a challenge for conservation planners. If it is not possible to predict where suitable habitat is under current environmental conditions, then spatially dynamic conservation measures, which have been proposed and enacted in other marine contexts, may be impossible to implement (Hobday et al., 2011; Maxwell et al., 2015). However, our analysis of at sea distribution presents some opportunities. Our tracking dataset identifies some areas of high foraging activity by multiple individuals between years. This approach to examining areas with repeated use between years is likely more useful for determining important foraging areas for gadfly petrels and other seabirds that may lack predictable foraging preferences for any static and dynamic oceanographic features. Moreover, the dual foraging strategy that we identified also creates potential for different conservation strategies to provide protection for the different trip types. The short foraging trips cover a much smaller area, meaning that methods such as marine protected areas could be effective at this spatial scale (Oppel et al., 2018). Some existing protections that prohibit commercial fishing occur in the Norfolk Island National Park Zone to the north of the Island (Figure 8), which encompasses a small portion of the areas used by gadfly petrels during short trips. Conversely, the vast extent covered during longer foraging trips by self-provisioning gadfly petrels means that policy-based methods such as fisheries regulation (e.g., quotas and more comprehensive enforcement) or management of marine plastic pollution will likely represent the best tools for conserving birds undertaking these long-distance trips (Oppel et al., 2018). While the risk of interactions with commercial fisheries is considered low for the small to medium sized gadfly petrels in our study (Waugh et al., 2012), their populations will benefit from increased efforts to prevent the degradation of marine ecosystems through pollution, over-fishing, and climate change both within and outside of EEZs. Although the conservation of the high seas is much more challenging than within EEZs, several large high seas areas of the Tasman Sea – which are frequented by gadfly petrels in the present study – have been identified as ecological or biologically significant marine areas (EBSAs) by Parties to the Convention on Biological Diversity (Bax et al., 2016). These areas have been the topic of discussions initiated by a United Nations call to establish marine protections beyond national jurisdictions. Provided that their nesting colony on Phillip Island remains free of introduced predators, conservation efforts for these gadfly petrels should focus on collaborative multinational cross-border efforts to conserve and restore marine ecosystem function in the Tasman Sea and the wider South Pacific.
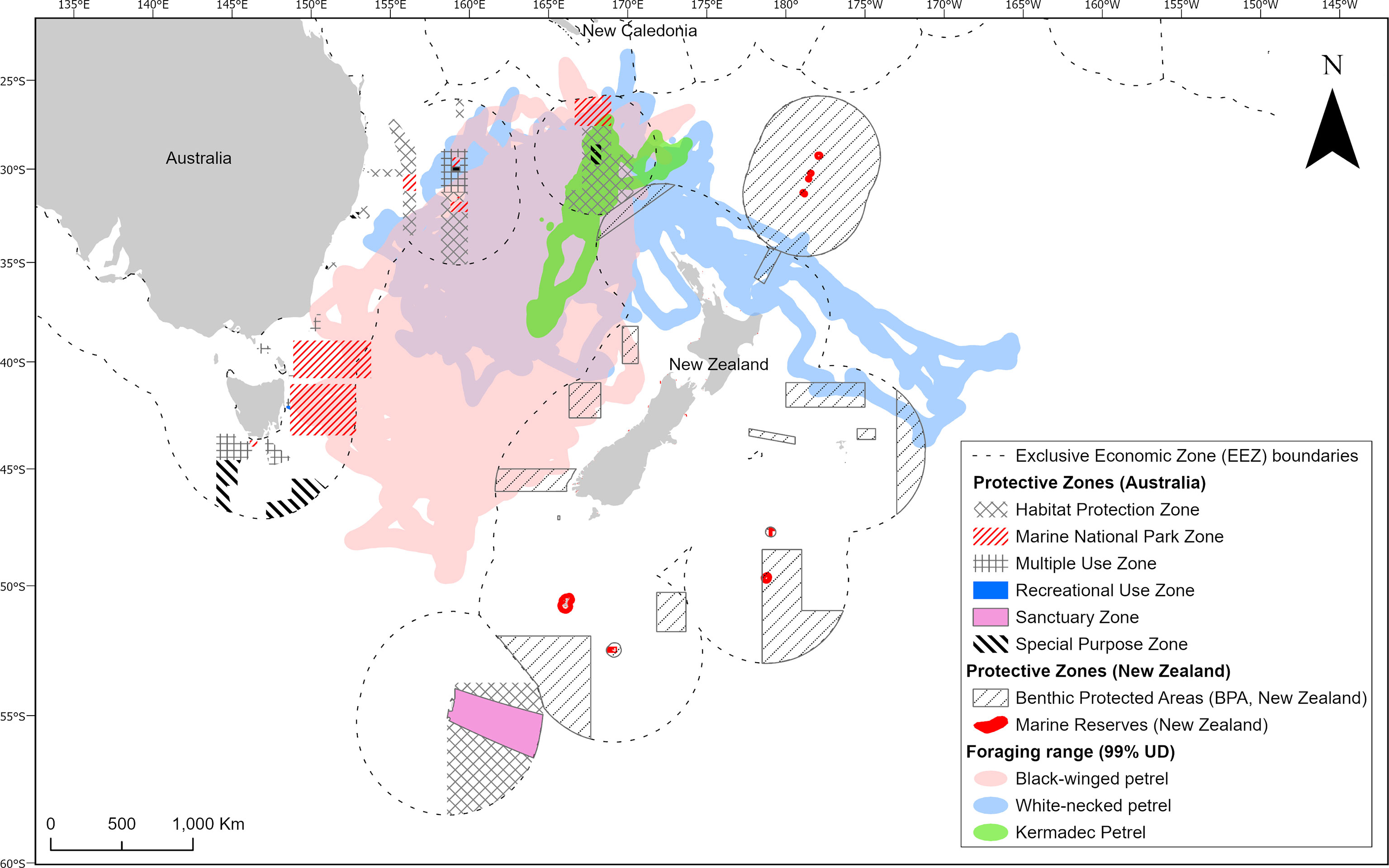
Figure 8 Foraging ranges of black-winged petrels (Pterodroma nigripennis), white-necked petrels (P. cervicalis) and Kermadec petrels (P. neglecta) in relation to existing protected areas and areas outside of national jurisdictions.
Data Availability Statement
The datasets presented in this study can be found in the Seabird Tracking Database (https://seabirdtracking.org) online repository (dataset IDs: 1499, 1453, 1422, 1452, 1423).
Ethics Statement
This animal study was reviewed and approved by Monash University School of Biological Sciences Animal Ethics Committee and New South Wales Department of Planning, Industry and Environment (DPIE) Animal Ethics Committee.
Author Contributions
LH conceived the project idea, acquired funding and scientific permits, conducted field expeditions, collected field data, analyzed the data, and led the writing of the manuscript. RM assisted with formulation of ideas, data analysis and contributed to project development. TCl and GH assisted with data analysis. TCh assisted with field expeditions and data collection. NC and RC contributed to fieldwork, project development and ideas, acquisition of scientific permits and funding. All authors contributed critically to manuscript drafts and gave final approval for publication.
Funding
This research was supported by Norfolk Island National Park, Parks Australia, and the New South Wales Department of Planning Industry and Environment. LH was supported with an Endeavour Postgraduate Leadership Award by the Australian government. This research was funded by the New South Wales Department of Industry and Planning, Norfolk Island National Park, and grants awarded to LH by the Holsworth Wildlife Research Endowment Equity Trustees Charitable Foundation and the Ecological Society of Australia, the Birdlife Australia Stuart Leslie Bird Research Award, and the Australasian Seabird Group Student Award.
Conflict of Interest
GH was employed by HiDef Aerial Surveying Ltd.
The remaining authors declare that the research was conducted in the absence of any commercial or financial relationships that could be construed as a potential conflict of interest.
The handling editor JP is currently organizing a Frontiers Research Topic with the author TCl.
Publisher’s Note
All claims expressed in this article are solely those of the authors and do not necessarily represent those of their affiliated organizations, or those of the publisher, the editors and the reviewers. Any product that may be evaluated in this article, or claim that may be made by its manufacturer, is not guaranteed or endorsed by the publisher.
Acknowledgments
We are grateful to volunteers, David Dow, Dr Christopher Lee and Daniel Terrington for assistance with fieldwork; the staff of Norfolk Island National Park, including Mel Wilson, Joel Christian, Ross Quintal, Kenny Christian, and Nigel Greenup for their support. We thank Charter Marine for providing transport to Phillip Island in challenging conditions and to the community of Norfolk Islanders for their enthusiasm and support.
Supplementary Material
The Supplementary Material for this article can be found online at: https://www.frontiersin.org/articles/10.3389/fmars.2022.853104/full#supplementary-material
References
Adams J., Flora S. (2010). Correlating Seabird Movements With Ocean Winds: Linking Satellite Telemetry With Ocean Scatterometry. Mar. Biol. 157, 915–929. doi: 10.1007/s00227-009-1367-y
Andrew M. E., Fox E. (2020). Modelling Species Distributions in Dynamic Landscapes: The Importance of the Temporal Dimension. J. Biogeogr. 47, 1510–1529. doi: 10.1111/jbi.13832
Araujo M., Pearson R., Thuiller W., Erhard M. (2005). Validation of Species-Climate Impact Models Under Climate Change. Global Change Biol. 11, 1504–1513. doi: 10.1111/j.1365-2486.2005.001000.x
Ashmole N. P. (1963). The Regulation of Numbers of Tropical Oceanic Birds. Ibis 103, 458–473. doi: 10.1111/j.1474-919X.1963.tb06766.x
Ashmole N. P. (1971). “Seabird Ecology and the Marine Environment,” in Avian Biology, vol. 1 . Eds. Farner D. S., King J. R. (New York: Academic Press).
Ashmole N. P., Ashmole M. J. (1967). Comparative Feeding Ecology of Sea Birds of a Tropical Oceanic Island. Peabody Mus. Nat. Hist. Bull. 24, 1–131.
Ballance L. T., Pitman R. L., Fiedler P. C. (2006). Oceanographic Influences on Seabirds and Cetaceans of the Eastern Tropical Pacific: A Review. Prog. Oceanogr. 69, 360–390. doi: 10.1016/j.pocean.2006.03.013
Ballance L. T., Pitman R. L., Reilly S. B. (1997). Seabird Community Structure Along a Productivity Gradient: Importance of Competition and Energetic Constraint. Ecology 78, 1502–1518. doi: 10.1890/0012-9658(1997)078[1502:SCSAAP]2.0.CO;2
Barbet-Massin M., Jiguet F., Albert C. H., Thuiller W. (2012). Selecting Pseudo-Absences for Species Distribution Models: How, Where and How Many? Methods Ecol. Evol. 3, 327–338. doi: 10.1111/j.2041-210X.2011.00172.x
Bates D., Mächler M., Bolker B. M., Walker S. (2015). Fitting Linear Mixed-Effects Models Using Lme4. J. Stat. Softw. 67, 1–48. doi: 10.18637/jss.v067.i01
Bax N. J., Cleary J., Donnelly B., Dunn D. C., Dunstan P. K., Fuller M., et al (2016). Results of Efforts by the Convention on Biological Diversity to Describe Ecologically or Biologically Significant Marine Areas. Conserv. Biol. 30, 571–581. doi: 10.1111/cobi.12649
Beal M., Oppel S., Handley J., Pearmain E. J., Morera-Pujol V., Carneiro A. P. B., et al (2021). Track2kba: An R Package for Identifying Important Sites for Biodiversity From Tracking Data. Methods Ecol. Evol. 12, 2372–2378. doi: 10.1111/2041-210X.13713
Bernard A., Rodrigues A. S. L., Cazalis V., Grémillet D. (2021). Toward a Global Strategy for Seabird Tracking. Conserv. Lett. 14, e12804. doi: 10.1111/conl.12804
Block B. A., Jonsen I. D., Jorgensen S. J., Winship A. J., Shaffer S. A., Bograd S. J., et al (2011). Tracking Apex Marine Predator Movements in a Dynamic Ocean. Nature 475, 86–90. doi: 10.1038/nature10082
Bostock H. C., Hayward B. W., Neil H. L., Sabaa A. T., Scott G. H. (2015). Changes in the Position of the Subtropical Front South of New Zealand Since the Last Glacial Period. Paleoceanography 30, 824–844. doi: 10.1002/2014PA002652
Boyd I. L., Wanless S., Camphuysen C. J. (Eds.) (2006). Top Predators in Marine Ecosystems: Their Role in Monitoring and Management (Cambridge: Cambridge University Press).
Burger A. E., Shaffer S. A. (2008). Perspectives in Ornithology Application of Tracking and Data-Logging Technology in Research and Conservation of Seabirds. Auk: Ornithol. Adv. 125, 253–264. doi: 10.1525/auk.2008.1408
Calenge C. (2006). The Package “Adehabitat” for the R Software: A Tool for the Analysis of Space and Habitat Use by Animals. Ecol. Modell. 197, 516–519. doi: 10.1016/J.ECOLMODEL.2006.03.017
Carlile N., Clarke R. H., Wilson M., Brown S. M., Baker G. B., Garnett S. T. (2021b). “Providence Petrel Pterodroma Solandri,” in The Action Plan for Australian Birds 2020 (Melbourne: CSIRO Publishing), 167–169.
Carlile N., O’Dwyer T., Wilson M., Clarke R. H., Brown S. M., Baker G. B., et al (2021a). “Western Kermadec Petrel Pterodroma Neglecta Neglecta,” in The Action Plan for Australian Birds 2020 (Melbourne: CSIRO Publishing), 169–172.
Clay T. A., Joo R., Weimerskirch H., Phillips R. A., den Ouden O., Basille M., et al (2020). Sex-Specific Effects of Wind on the Flight Decisions of a Sexually Dimorphic Soaring Bird. J. Anim. Ecol. 89, 1811–1823. doi: 10.1111/1365-2656.13267
Clay T. A., Oppel S., Lavers J. L., Phillips R. A., Brooke M. d. L. (2019). Divergent Foraging Strategies During Incubation of an Unusually Wide-Ranging Seabird, the Murphy’s Petrel. Mar. Biol. 166:1–14. doi: 10.1007/s00227-018-3451-7
Clay T. A., Phillips R. A., Manica A., Jackson H. A., Brooke M. d. L. (2017). Escaping the Oligotrophic Gyre? The Year-Round Movements, Foraging Behaviour and Habitat Preferences of Murphy’s Petrels. Mar. Ecol. Prog. Ser. 579, 139–155. doi: 10.3354/meps12244
Cotté C., d’Ovidio F., Dragon A. C., Guinet C., Lévy M. (2015). Flexible Preference of Southern Elephant Seals for Distinct Mesoscale Features Within the Antarctic Circumpolar Current. Prog. Oceanogr. 131, 46–58. doi: 10.1016/J.POCEAN.2014.11.011
Croxall J. P., Butchart S. H. M., Lascelles B., Stattersfield A. J., Sullivan B., Symes A., et al (2012). Seabird Conservation Status, Threats and Priority Actions: A Global Assessment. Bird Conserv. Int. 22, 1–34. doi: 10.1017/S0959270912000020
Davies T. E., Carneiro A. P. B., Campos B., Hazin C., Dunn D. C., Gjerde K. M., et al (2021). Tracking Data and the Conservation of the High Seas: Opportunities and Challenges. J. Appl. Ecol. 58, 2703–2710. doi: 10.1111/1365-2664.14032
Diamond A. W. (1983). Feeding Overlap in Some Tropical and Temperate Seabird Communities. Stud. Avian Biol. 8, 24–46.
Diamond A. W., Devlin C. M. (2003). Seabirds as Indicators of Changes in Marine Ecosystems: Ecological Monitoring on Machias Seal Island. Environ. Monit. Assess. 88, 153–175. doi: 10.1023/a:1025560805788
Dias M. P., Granadeiro J. P., Catry P. (2012). Working the Day or the Night Shift? Foraging Schedules of Cory’s Shearwaters Vary According to Marine Habitat. Mar. Ecol. Prog. Ser. 467, 245–252. doi: 10.3354/meps09966
d’Ovidio F., Fernández V., Hernández-García E., López C. (2004). Mixing Structures in the Mediterranean Sea From Finite-Size Lyapunov Exponents. Geophys. Res. Lett. 31, 17203. doi: 10.1029/2004GL020328
Elith J., Ferrier S., Huettmann F., Leathwick J. (2005). The Evaluation Strip: A New and Robust Method for Plotting Predicted Responses From Species Distribution Models. Ecol. Modell. 186, 280–289. doi: 10.1016/J.ECOLMODEL.2004.12.007
Elith J., Graham H., C P., Anderson R., Dudík M., Ferrier S., et al (2006). Novel Methods Improve Prediction of Species’ Distributions From Occurrence Data. Ecography 29, 129–151. doi: 10.1111/j.2006.0906-7590.04596.x
Elith J., Kearney M., Phillips S. (2010). The Art of Modelling Range-Shifting Species. Methods Ecol. Evol. 1, 330–342. doi: 10.1111/j.2041-210X.2010.00036.x
Elith J., Leathwick J. R., Hastie T. (2008). A Working Guide to Boosted Regression Trees. J. Anim. Ecol. 77, 802–813. doi: 10.1111/J.1365-2656.2008.01390.X
Fischer J. H., Debski I., Spitz D. B., Taylor G. A., Wittmer H. U. (2021). Year-Round Offshore Distribution, Behaviour, and Overlap With Commercial Fisheries of a Critically Endangered Small Petrel. Mar. Ecol. Prog. Ser. 660, 171–187. doi: 10.3354/meps13586
Ford R. G., Krumme D. W. (1979). The Analysis of Space Use Patterns. J. Theor. Biol. 76, 125–155. doi: 10.1016/0022-5193(79)90366-7
Gill B. J., Bell B. D., Chambers B. D., Medway D. G., Palma R. I., Scofield R. P., et al (2010). Checklist of the Birds of New Zealand, Norfolk and Macquarie Islands, and Ross Dependency, Antarctica. 4th edition (Wellington: Te Papa Press).
Graham N. A. J., Wilson S. K., Carr P., Hoey A. S., Jennings S., MacNeil M. A. (2018). Seabirds Enhance Coral Reef Productivity and Functioning in the Absence of Invasive Rats. Nature 559, 250–253. doi: 10.1038/s41586-018-0202-3
Griffiths R., Double M. C., Orr K., Dawson R. J. G. (1998). A DNA Test to Sex Most Birds. Mol. Ecol. 7, 1071–1075. doi: 10.1046/J.1365-294X.1998.00389.X
Halpern B. S., Walbridge S., Selkoe K. A., Kappel C. V., Micheli F., D’Agrosa C., et al (2008). A Global Map of Human Impact on Marine Ecosystems. Science 319, 948–952. doi: 10.1126/science.1149345
Halpin L. R., Carlile N., Baker G. B., Garnett S. T. (2021). “White-Necked Petrel Pterodroma Cervicalis,” in The Action Plan for Australian Birds 2020. (Melbourne: CSIRO Publishing), 177–179.
Halpin L. R., Pollet I. L., Lee C., Morgan K. H., Carter H. R. (2018). Year-Round Movements of Sympatric Fork-Tailed (Oceanodroma Furcata) and Leach’s (O. Leucorhoa) Storm-Petrels. J. Field Ornithol. 89, 207–220. doi: 10.1111/jofo.12255
Haney J. C., Haury L. R., Mullineaux L. S., Fey C. L. (1995). Sea-Bird Aggregation at a Deep North Pacific Seamount. Mar. Biol. 123, 1–9. doi: 10.1007/BF00350317
Hao T., Elith J., Guillera-Arroita G., Lahoz-Monfort J. J. (2019). A Review of Evidence About Use and Performance of Species Distribution Modelling Ensembles Like BIOMOD. Divers. Distrib. 25, 839–852. doi: 10.1111/ddi.12892
Harrison A.-L., Costa D. P., Winship A. J., Benson S. R., Bograd S. J., Antolos M., et al (2018). The Political Biogeography of Migratory Marine Predators. Nat. Ecol. Evol. 2, 1571–1578. doi: 10.1038/s41559-018-0646-8
Harrison C. S., Hida T. S., Seki M. P. (1983). Hawaiian Seabird Feeding Ecology. Wildl. Monogr. 85, 3–71.
Hays G. C. (2003). A Review of the Adaptive Significance and Ecosystem Consequences of Zooplankton Diel Vertical Migrations. Hydrobiologia 503, 163–170. doi: 10.1023/B:HYDR.0000008476.23617.b0
Hijmans R. J., van Etten J. (2021). Raster: Geographic Analysis and Modeling, R Package Version 3.5.9.
Hobday A. J., Hartog J. R., Spillman C. M., Alves O. (2011). Seasonal Forecasting of Tuna Habitat for Dynamic Spatial Management. Can. J. Fish. Aquat. Sci. 68, 898–911. doi: 10.1139/f2011-031
Hoegh-Guldberg O., Bruno J. F. (2010). The Impact of Climate Change on the World’s Marine Ecosystems. Science 328, 1523–1528. doi: 10.1126/science.1189930
Hosmer D. W., Lemeshow S., Sturdivant R. X. (2013). Applied Logistic Regression. 3rd Edition (New York: John Wiley & Sons).
Hyrenbach K. D., Veit R. R., Weimerskirch H., Hunt G. L. Jr. (2006). Seabird Associations With Mesoscale Eddies: The Subtropical Indian Ocean. Mar. Ecol. Prog. Ser. 324, 271–279. doi: 10.3354/meps324271
Imber M. J., Jolly J. N., Brooke M. d. L. (1995). Food of Three Sympatric Gadfly Petrels (Pterodroma Spp.) Breeding on the Pitcairn Islands. Biol. J. Linn. Soc 56, 233–240. doi: 10.1111/j.1095-8312.1995.tb01087.x
Lascelles B. G., Taylor P. R., Miller M. G. R., Dias M. P., Oppel S., Torres L., et al (2016). Applying Global Criteria to Tracking Data to Define Important Areas for Marine Conservation. Divers. Distrib. 22, 422–431. doi: 10.1111/ddi.12411
Lescroël A., Mathevet R., Péron C., Authier M., Provost P., Takahashi A., et al (2016). Seeing the Ocean Through the Eyes of Seabirds: A New Path for Marine Conservation? Mar. Policy 68, 212–220. doi: 10.1016/j.marpol.2016.02.015
Lobo J. M., Jiménez-Valverde A., Real R. (2008). AUC: A Misleading Measure of the Performance of Predictive Distribution Models. Glob. Ecol. Biogeogr. 17, 145–151. doi: 10.1111/J.1466-8238.2007.00358.X
Magalhães M. C., Santos R. S., Hamer K. C. (2008). Dual-Foraging of Cory’s Shearwaters in the Azores: Feeding Locations, Behaviour at Sea and Implications for Food Provisioning of Chicks. Mar. Ecol. Prog. Ser. 359, 283–293. doi: 10.3354/meps07340
Maxwell S. M., Hazen E. L., Lewison R. L., Dunn D. C., Bailey H., Bograd S. J., et al (2015). Dynamic Ocean Management: Defining and Conceptualizing Real-Time Management of the Ocean. Mar. Policy 58, 42–50. doi: 10.1016/j.marpol.2015.03.014
McCauley D. J., Pinsky M. L., Palumbi S. R., Estes J. A., Joyce F. H., Warner R. R. (2015). Marine Defaunation: Animal Loss in the Global Ocean. Science 347. doi: 10.1126/science.1255641
McClintock B. T., Michelot T. (2018). momentuHMM: R Package for Generalized Hidden Markov Models of Animal Movement. Methods Ecol. Evol. 9, 1518–1530. doi: 10.1111/2041-210X.12995
Miller M. G. R., Carlile N., Phillips J. S., McDuie F., Congdon B. C. (2018). Importance of Tropical Tuna for Seabird Foraging Over a Marine Productivity Gradient. Mar. Ecol. Prog. Ser. 586, 233–249. doi: 10.3354/meps12376
Miskelly C. M., Gilad D., Taylor G. A., Tennyson A. J. D., Waugh S. M. (2019). A Review of the Distribution and Size of Gadfly Petrel (Pterodroma Spp.) Colonies Throughout New Zealand. Tuhinga 30, 93–177.
Morato T., Varkey D. A., Damaso C., Machete M., Santos M., Prieto R., et al (2008). Evidence of a Seamount Effect on Aggregating Visitors. Mar. Ecol. Prog. Ser. 357, 23–32. doi: 10.3354/meps07269
Mott R., Clarke R. H. (2018). Systematic Review of Geographic Biases in the Collection of at-Sea Distribution Data for Seabirds. Emu - Austral Ornithol. 118, 235–246. doi: 10.1080/01584197.2017.1416957
Nathan R., Getz W. M., Revilla E., Holyoak M., Kadmon R., Saltz D., et al (2008). A Movement Ecology Paradigm for Unifying Organismal Movement Research. Proc. Natl. Acad. Sci. 105, 19052. doi: 10.1073/pnas.0800375105
Oppel S., Beard A., Fox D., Mackley E., Leat E., Henry L., et al (2015). Foraging Distribution of a Tropical Seabird Supports Ashmole’s Hypothesis of Population Regulation. Behav. Ecol. Sociobiol. 69, 915–926. doi: 10.1007/s00265-015-1903-3
Oppel S., Bolton M., Carneiro A. P. B., Dias M. P., Green J. A., Masello J. F., et al (2018). Spatial Scales of Marine Conservation Management for Breeding Seabirds. Mar. Policy 98, 37–46. doi: 10.1016/J.MARPOL.2018.08.024
Paleczny M., Hammill E., Karpouzi V., Pauly D. (2015). Population Trend of the World’s Monitored Seabirds 1950-2010. PloS One 10, e0129342. doi: 10.1371/journal.pone.0129342
Parsons M., Mitchell I., Butler A., Ratcliffe N., Frederiksen M., Foster S., et al (2008). Seabirds as Indicators of the Marine Environment. ICES J. Mar. Sci. 65, 1520–1526. doi: 10.1093/icesjms/fsn155
Phillips R. A., Xavier J. C., Croxall J. P. (2003). Effects of Satellite Transmitters on Albatrosses and Petrels. Auk: Ornithol. Adv. 120, 1082–1090. doi: 10.1093/AUK/120.4.1082
Pinet P., Jaquemet S., Phillips R. A., Le Corre M. (2012). Sex-Specific Foraging Strategies Throughout the Breeding Season in a Tropical, Sexually Monomorphic Small Petrel. Anim. Behav. 83, 979–989. doi: 10.1016/j.anbehav.2012.01.019
Priddel D., Carlile N., Evans O., Evans B., McCoy H. (2010). A Review of the Seabirds of Phillip Island in the Norfolk Island Group. Notornis 57, 113–127.
Priddel D., Carlile N., Portelli D., Kim Y., O’Neill L., Bretagnolle V., et al (2014). Pelagic Distribution of Gould’s Petrel (Pterodroma Leucoptera): Linking Shipboard and Onshore Observations With Remote-Tracking Data. Emu - Austral Ornithol. 114, 360–370. doi: 10.1071/MU14021
Rabiner L. R. (1989). A Tutorial on Hidden Markov Models and Selected Applications in Speech Recognition. Proc. IEEE 77, 257–286. doi: 10.1109/5.18626
Raine A. F., Gjerdrum C., Pratte I., Madeiros J., Felis J. J., Adams J. (2021). Marine Distribution and Foraging Habitat Highlight Potential Threats at Sea for the Endangered Bermuda Petrel Pterodroma Cahow. Endanger. Species Res. 45, 337–356. doi: 10.3354/ESR01139
Ramírez I., Paiva V. H., Menezes D., Silva I., Phillips R. A., Ramos J. A., et al (2013). Year-Round Distribution and Habitat Preferences of the Bugio Petrel. Mar. Ecol. Prog. Ser. 476, 269–284. doi: 10.3354/meps10083
Ramos R., Carlile N., Madeiros J., Ramírez I., Paiva V. H., Dinis H. A., et al (2017). It is the Time for Oceanic Seabirds: Tracking Year-Round Distribution of Gadfly Petrels Across the Atlantic Ocean. Divers. Distrib. 23, 1794–1805. doi: 10.1111/ddi.12569
Ramos R., Ramírez I., Paiva V. H., Militão T., Biscoito M., Menezes D., et al (2016). Global Spatial Ecology of Three Closely-Related Gadfly Petrels. Sci. Rep. 6, 23447. doi: 10.1038/srep23447
Ramos R., Sanz V., Militão T., Bried J., Neves V. C., Biscoito M., et al (2015). Leapfrog Migration and Habitat Preferences of a Small Oceanic Seabird, Bulwer’s Petrel (Bulweria Bulwerii). J. Biogeogr. 42, 1651–1664. doi: 10.1111/jbi.12541
Rayner M., Carlile N., Priddel D., Bretagnolle V., Miller M., Phillips R., et al (2016). Niche Partitioning by Three Pterodroma Petrel Species During non-Breeding in the Equatorial Pacific Ocean. Mar. Ecol. Prog. Ser. 549, 217–229. doi: 10.3354/meps11707
Rayner M. J., Hauber M. E., Clout M. N., Seldon D. S., Van Dijken S., Bury S., et al (2008). Foraging Ecology of the Cook’s Petrel Pterodroma Cookie During the Austral Breeding Season: A Comparison of its Two Populations. Mar. Ecol. Prog. Ser. 370, 271–284. doi: 10.3354/meps07660
Rayner M., Taylor G., Phillips R. A., Sagar P. (2012). The Breeding Cycle, Year-Round Distribution and Activity Patterns of the Endangered Chatham Petrel (Pterodroma Axillaris). Emu - Austral Ornithol. 112, 107–116. doi: 10.1071/MU11066
R Core Team (2021) R: A Language and Environment for Statistical Computing. Available at: https://www.r-project.org/.
Roberts D. R., Bahn V., Ciuti S., Boyce M. S., Elith J., Guillera-Arroita G., et al (2017). Cross-Validation Strategies for Data With Temporal, Spatial, Hierarchical, or Phylogenetic Structure. Ecography 40, 913–929. doi: 10.1111/ecog.02881
Rodríguez A., Arcos J. M., Bretagnolle V., Dias M. P., Holmes N. D., Louzao M., et al (2019). Future Directions in Conservation Research on Petrels and Shearwaters. Front. Mar. Sci. 6. doi: 10.3389/fmars.2019.00094
Sánchez-Piñero F., Polis G. A. (2000). Bottom-Up Dynamics of Allochthonous Input: Direct and Indirect Effects of Seabirds on Islands. Ecology 81, 3117–3132. doi: 10.1890/0012-9658(2000)081[3117:BUDOAI]2.0.CO;2
Smith J. L., Mulder C. P. H., Ellis J. C. (2011). “Seabirds as Ecosystem Engineers: Nutrient Inputs and Physical Disturbance,” in Seabird Islands: Ecology, Invasion, and Restoration. Eds. Mulder C. P. H., Anderson W. B., Towns D. R., Bellingham P. J. (Oxford: Oxford University Press).
Soanes L. M., Arnould J. P. Y., Dodd S. G., Sumner M. D., Green J. A. (2013). How Many Seabirds do We Need to Track to Define Home-Range Area? J. Appl. Ecol. 50, 671–679. doi: 10.1111/1365-2664.12069
Spear L., Ainley D. G. (1993). Kleptoparasitism by Kermadec Petrels, Jaegers, and Skuas in the Eastern Tropical Pacific: Evidence of Mimicry by Two Species of Pterodroma. Auk: Ornithol. Adv. 110, 222–233.
Spear L. B., Ainley D. G. (1997). Flight Speed of Seabirds in Relation to Wind Speed and Direction. Ibis 139, 234–251. doi: 10.1111/j.1474-919X.1997.tb04621.x
Spear L. B., Ainley D. G., Walker W. A. (2007). Foraging Dynamics of Seabirds in the Eastern Tropical Pacific Ocean (Norman: Cooper Ornithological Society).
Spear L. B., Ballance L. T., Ainley D. G. (2001). Response of Seabirds to Thermal Boundaries in the Tropical Pacific: The Thermocline Versus the Equatorial Front. Mar. Ecol. Prog. Ser. 219, 275–289. doi: 10.3354/meps219275
Stapp P., Polis G. A., Piñero F. S. (1999). Stable Isotopes Reveal Strong Marine and El Niño Effects on Island Food Webs. Nature 401, 467–469. doi: 10.1038/46769
Taylor G. A., Elliott G. P., Walker K. J. (2020). Year-Round Distribution, Breeding Cycle, and Activity of White-Headed Petrels (Pterodroma Lessonii) Nesting on Adams Island, Auckland Islands. Notornis 67, 369–386.
Telford R. J., Birks H. J. B. (2009). Evaluation of Transfer Functions in Spatially Structured Environments. Quat. Sci. Rev. 28, 1309–1316. doi: 10.1016/j.quascirev.2008.12.020
Thompson D. R. (2007). “Air-Breathing Visitors to Seamounts: Importance of Seamounts to Seabirds,” in Seamounts: Ecology, Conservation and Management, vol. 12 . Eds. Pitcher T. J., Morato T., Hart P. J. B., Clark M. R., Haggan N., Santos R. S. (Oxford: Blackwell), 245–251.
Thuiller W., Georges D., Gueguen M., Engler R., Breiner F. (2021) Biomod2: Ensemble Platform for Species Distribution Modeling. Available at: https://CRAN.R-project.org/package=biomod2.
Thuiller W., Lafourcade B., Engler R., Araújo M. B. (2009). BIOMOD – a Platform for Ensemble Forecasting of Species Distributions. Ecography 32, 369–373. doi: 10.1111/j.1600-0587.2008.05742.x
Valavi R., Elith J., Lahoz-Monfort J. J., Guillera-Arroita G. (2019). blockCV: An R Package for Generating Spatially or Environmentally Separated Folds for K-Fold Cross-Validation of Species Distribution Models. Methods Ecol. Evol. 10, 225–232. doi: 10.1111/2041-210X.13107
Ventura F., Granadeiro J. P., Padget O., Catry P. (2020). Gadfly Petrels Use Knowledge of the Windscape, Not Memorized Foraging Patches, to Optimize Foraging Trips on Ocean-Wide Scales. Proc. R. Soc Lond. Ser. B 287, 20191775. doi: 10.1098/rspb.2019.1775
Warham J. (1996). The Behaviour, Population Biology and Physiology of the Petrels (San Diego: Academic Press).
Waugh S. M., Filippi D. P., Kirby D. S., Abraham E., Walker N. (2012). Ecological Risk Assessment for Seabird Interactions in Western and Central Pacific Longline Fisheries. Mar. Policy 36, 933–946. doi: 10.1016/j.marpol.2011.11.005
Waugh S. M., Griffiths J. W., Poupart T. A., Filippi D. P., Rogers K., Arnould J. Y. P. (2018). Environmental Factors and Fisheries Influence the Foraging Patterns of a Subtropical Seabird, the Westland Petrel (Procellaria Westlandica), in the Tasman Sea. Condor: Ornithol. Appl. 120, 371–387. doi: 10.1650/CONDOR-17-179.1
Weber S. B., Richardson A. J., Brown J., Bolton M., Clark B. L., Godley B. J., et al (2021). Direct Evidence of a Prey Depletion “Halo” Surrounding a Pelagic Predator Colony. Proc. Natl. Acad. Sci. 118, e2101325118. doi: 10.1073/pnas.2101325118
Weimerskirch H. (2007). Are Seabirds Foraging for Unpredictable Resources? Deep Sea Res. Part II 54, 211–223. doi: 10.1016/j.dsr2.2006.11.013
Keywords: seabirds, seabird conservation and management, High Seas, Australia, Pterodroma petrels, at-sea distribution, foraging behavior, species distribution modeling (SDM)
Citation: Halpin LR, Mott R, Clay TA, Humphries GRW, Chatwin TA, Carlile N and Clarke RH (2022) Predicting the Foraging Habitats of Sympatrically Breeding Gadfly Petrels in the South Pacific Ocean. Front. Mar. Sci. 9:853104. doi: 10.3389/fmars.2022.853104
Received: 12 January 2022; Accepted: 29 April 2022;
Published: 14 June 2022.
Edited by:
Jorge M. Pereira, University of Coimbra, PortugalReviewed by:
Matt Rayner, Auckland Museum, New ZealandIngrid L Pollet, Acadia University, Canada
Federico De Pascalis, University of Milan, Italy
Copyright © 2022 Halpin, Mott, Clay, Humphries, Chatwin, Carlile and Clarke. This is an open-access article distributed under the terms of the Creative Commons Attribution License (CC BY). The use, distribution or reproduction in other forums is permitted, provided the original author(s) and the copyright owner(s) are credited and that the original publication in this journal is cited, in accordance with accepted academic practice. No use, distribution or reproduction is permitted which does not comply with these terms.
*Correspondence: Luke R. Halpin, Luke.Halpin@gmail.com