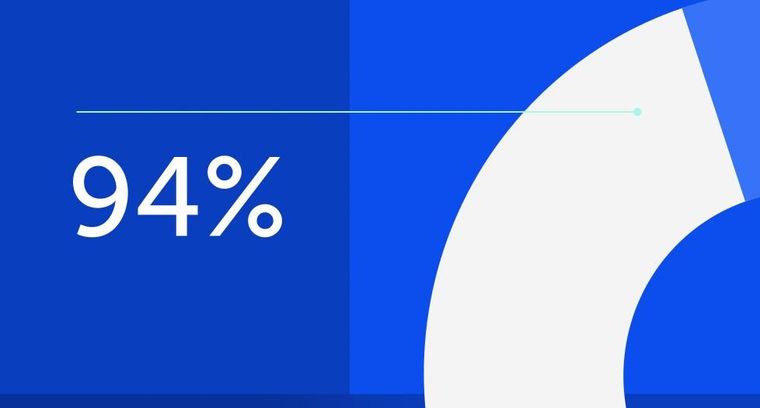
94% of researchers rate our articles as excellent or good
Learn more about the work of our research integrity team to safeguard the quality of each article we publish.
Find out more
ORIGINAL RESEARCH article
Front. Mar. Sci., 05 April 2022
Sec. Microbial Symbioses
Volume 9 - 2022 | https://doi.org/10.3389/fmars.2022.850984
This article is part of the Research TopicRising Stars in Microbial Symbioses: 2022View all 8 articles
Coral endolithic microbes can be an important nutrients support for hosts while under stresses. Previous studies have found that the endolithic microbial composition of a single coral species can be biogeographical diverse. However, the potential environmental factors, such as salinity, temperature, pH, and nutrient, that might influence the composition of the endolithic microbes remain unclear. In this study, we used both amplicon sequence variants (ASV) and a kmer-based taxonomic unit (KTU) to characterize the endolithic bacterial constitution of Isopora spp. located in the western Pacific Ocean—where it is subjected to the Kuroshio Current (in Okinawa, Japan and Green Island, Taiwan)—and the South China Sea (Dongsha Atoll). The endolithic bacterial community compositions showed a significant geographical difference, and we suggest that the ocean current and primary productivity are the most essential environmental factors influencing the bacterial communities in the skeleton of Isopora spp. In addition, our results showed that, technically, compared to ASV, bacterial composition based on KTU avoids extreme data, making it a suitable tool for explaining the associations between microbes and environmental factors.
Coral endolithic microbes are considered to be highly important because they play key roles in carbon and nitrogen cycles (Fine and Loya, 2002; Ricci et al., 2019; Yang et al., 2019; Pernice et al., 2020); their coral hosts can, in turn, utilize the nutrients by those endolithic microorganisms, such as the organic carbon synthesized by the green algae Ostreobium (Ferrer and Szmant, 1988; Fine and Loya, 2002). The abundant endolithic community is composed by bacteria, microeukaryotes and viruses (Ricci et al., 2019; Pernice et al., 2020), among them are eukaryotic algae Ostreobium (del Campo et al., 2017); the photoautotrophic cyanobacteria Plectonema terebrans and Mastigocoleus testarum (Fine and Loya, 2002; Tribollet and Golubic, 2005); and fungi (Tribollet and Golubic, 2005). Those microbes are able to form clear green layers in coral skeletons beneath coral tissues (Ricci et al., 2019; Pernice et al., 2020).
Isopora spp., common reef-building corals in the offshore of the western Pacific Ocean, have such a green layer in their skeleton (Yang et al., 2016; Yang et al., 2019; Yang et al., 2020). However, instead of finding aerobic microbes in coral skeletons, we found that the endolithic microbes in Isopora were dominated by anaerobes, such as Chlorobi, Chloroflexi and Deltaproteobacteria. These anaerobic bacteria in the green layer might involve in nitrogen fixation and sulfur cycles (Yang et al., 2019). In Yang et al. (2019)’s sample, the rarely-found photoautotrophic algae or fungi endolithic communities were speculated to be host-specific.
Ricci et al. (2021) recently found that the endolithic community of Isopora from the southern Great Barrier Reef, Australia, was dominated by the algae Ostreobium. In addition, the endolithic bacterial community of Isopora in Okinawa is mainly dominated by aerobic Bacteroidetes and Alphaproteobacteria (Yang et al., 2020). These observations suggest that the endolithic communities in the Isopora skeleton might be influenced by geographical factors. Nevertheless, which local environmental factors influence endolithic composition remains unclear.
The operational taxonomic unit (OTU) has been implemented for microbial ecology studies since molecular biology tools were applied to study microbial molecular taxonomy (Edgar, 2010; Edgar, 2013). The OTU analysis was performed by either de novo or reference-based clustering methods with a 97% sequence similarity threshold for defining an operational species (Stackebrandt and Goebel, 1994). However, the denoising methods unbiasedly detect amplicon sequence variants (ASVs) and have revolutionized OTU-based pipelines in recent years. The ASVs are distinguished by single nucleotide variants; thus, any unique ASV is reproducible through the denoising process (by processing the same sequence regions) (Rosen et al., 2012).
More and more studies have been implementing denoising pipelines using the DADA2 (Callahan et al., 2016), Deblur (Amir et al., 2017) or UNOISE (Edgar, 2016) algorithms to obtain unbiased taxonomic composition data. However, in contrast to the OTUs, the ultra-high resolution sequence variants lead to a sparse ASV abundance table with too-many-zeros. Some studies reclustered ASVs into OTUs with 97% sequence similarity to improve the explained variance in community diversity (Liu et al., 2020). The reclustering-OTU (e.g. the VSEARCH plug-in embedded in the QIIME2 pipeline) method still follows operational classification cutoffs (e.g. 97% similarity for species or 94% similarity for genus) (Youssef et al., 2012), but it is criticized for its universality.
We performed an alternative ASV-reclustering method “KTU,” a kmer-based taxonomic unit, alignment-free clustering algorithm to aggregate sparse ASVs into convergent taxonomic units (i.e. KTUs) with the closest tetra nucleotide usage patterns, which reflect to a ‘genome signature’ of a species (Dick et al., 2009; Liu et al., 2022). KTU reclustering reduces the zero-inflation effect in the data structure of the microbial communities. Therefore, continuous quantitative microbial abundance could precisely fit the environmental factors. In addition, the KTU assembles the ASVs into the closest phylogenetic lineages with the same genus or species names; for example, KTU reclusters Lactobacillus ASVs into several close sub-lineages with over 99% sequence similarities (e.g. Fructilactobacillus spp., Levilactobacillus spp., Latilactobacillus spp., and Limosilactobacillus spp.) (Huang et al., 2018; Zheng et al., 2020; Liu et al., 2022). Thus, the reclustered features not only assembles close-phylogeny features but also improves the confidence of associations between microbiota compositions and continuous environmental/clinical factors compared to using raw ASV composition data.
In this study, we targeted Isopora spp. from three locations subjected to the Kuroshio Current (KC) in the western Pacific Ocean to investigate the environmental factors which might affect the endolithic bacterial diversity in the skeletons. In addition, we attempted to improve the correlation of bacterial community and key environmental factors by comparing the two amplicon feature detection methods (KTUs and ASVs).
Isopora colonies were sampled from three locations: Green Island (GI) (November 2015 at 22°75’N, 121°50’E and April 2017 at 22°40’N, 121°27’E) and Dongsha Atoll (DS) (July 2017 at 20°75’N, 116°75’E) in Taiwan, and Okinawa (OK) in Japan (October 2017 at 26°38’N, 127°51’E). The OK and DS sites are reef flat, GI site is at reef front. The location and depth of each sample are listed in Table 1.
Table 1 Information on Isopora spp. coral colonies used to characterize bacterial communities in different locations.
The collected samples were washed twice with filtered seawater and stored at -80°C until DNA extraction. The extraction procedure followed the method in Yang et al. (2016). Slurry samples from the green layers of the coral skeleton were transferred into clean 1.5 mL tubes. The total genomic DNA of all the samples were extracted using the DNeasy PowerSoil Kit (Qiagen, Maryland, US). All the PCR protocol were followed Yang et al. (2016). In brief, PCR was performed using two bacterial universal primers: 968F (5’-AACGCGAAGAACCTTAC-3’) and 1391R (5’-ACGGGCGGTGWGTRC-3’), designed for the bacterial V6-V8 hypervariable regions in the 16S ribosomal RNA gene, with 30 cycles of PCR with initial step of 94°C for 5 min, 94°C for 30 s, 52°C for 20 s, 72°C for 45 s, and finally 72°C for 10 min. Each PCR product was tagged using DNA tagging PCR (Chen et al., 2011), then sequenced using the Illumina Miseq 300 bp paired-end configuration.
The 16S rDNA amplicon sequences were processed using the Quantitative Insights Into Microbial Ecology 2 (QIIME 2) pipeline (version 2019.10) (Bolyen et al., 2019). The raw reads were first demultiplexed by cutadapt (version 1.15) (Martin, 2011), then the demultiplexed sequences were denoised using the DADA2 plugin of QIIME2 (Callahan et al., 2016). The qualified amplicon sequence variants (ASVs) were obtained via the denoising process with quality filtering (by truncating both ends of the reads to 235 bp) and chimera removal. ASV taxonomy was assigned using the classifier-consensus-vsearch plugin (Bokulich et al., 2018) against SILVA NR128 99% 16S rRNA gene sequences (Quast et al., 2013; Yilmaz et al., 2014). The ASV sequences were then re-clustered and aggregated using the “K-mer-based taxonomic (KTU) clustering algorithm” (https://github.com/poyuliu/KTU/) to refine the sparseness of the ASV abundance table. The KTU algorithm iteratively clustered ASV representative sequences with their tetra-nucleotide patterns (256 tetramer features, either frequencies or present scores; using the present-score option of KTU algorithm in the study) by partitioning around medoids (PAM, also called k-Medoids). A convergent KTU number was found by iteratively searching the maximum silhouette coefficient. Then we evaluated the KTU algorithm of the dataset by calculating the 1) ASV-to-KTU aggregating rate of each KTU, 2) sequence similarity within KTU and 3) within-KTU divergence based on the cosine distance. Finally, the KTUs were obtained by reclustering and used for subsequent analyses. R software (version 3.6.3) (R Core Team, 2015) was used for all data analyses. The ASV and KTU abundance tables were rarefied to the minimal read counts of the samples using the vegan R-package (31,024 reads per sample) (Oksanen et al., 2015). The unassigned taxon, chloroplast and mitochondria reads were removed from both the ASV and KTU abundance tables. The 52 removed chloroplast KTUs were then extracted to identify eukaryotic coral symbionts using Blastn (Camacho et al., 2009) (e value < 1e-5, identity ≥ 85%) by searching against the NCBI non-Redundant (NR) database.
The bacterial community analyses were conducted and visualized using the MARco R-package (Liu, 2021). A Kruskal-Wallis test in R software (R Core Team, 2015), with α=0.05, was used for all statistical analyses of group comparisons and Dunn’s test for post-hoc comparisons. Dissimilarities among microbial communities were measured by Bray-Curtis dissimilarity using a principal coordinates analysis (PCoA), and heterogeneity was tested using ADONIS (permutational multivariate analysis of variance using distance matrices). Alpha diversity indices were estimated by richness, Shannon’s index, Simpson’s index and Chao1 index. Pearson correlation analysis was performed to find the associations between factor analysis (FA) axes and PCoA axes. Spearman correlation analysis was performed to select FA axes-associated taxa, and taxa with p < 0.01 and in the top 2.5% coefficients (ρ) were selected. These taxa were visualized with a heatmap using the pheatmap R-package. An indicator species analysis was done with a chi-square test (Niemi et al., 1997); the indicator species were then identified by Pearson residuals > 5.
The environmental factors of the sampling sites were retrieved from the Ocean Data Bank (ODB, Ocean Data Bank of the Ministry of Science and Technology, Republic of China; http://www.odb.ntu.edu.tw/); an exploratory factor analysis was conducted by the psych package in R. An “fa” function with varimax rotation was performed and three factors were extracted. The factor scores were extracted using a regression method.
The flow rates of currents in the western Pacific Ocean and the South China Sea were also retrieved from the ODB database. The average flow rates of U (the velocity toward east) and V (the velocity toward north) vectors were averaged based on the corresponding sampling months and depths; the averaged flow vectors were then placed onto a map of the western Pacific Ocean and the South China Sea.
A microbiota dataset of Kuroshio Current transects from eight stations between 121.72° and 123° E at 23.75° N east of Taiwan (Cheng et al., 2020) was retrieved from the NCBI Sequence Read Archive (SRA) database under the accession number PRJNA543843. The KC microbiota dataset was analyzed with the QIIME2 pipeline, with DADA2 denoising and reclustering ASVs with the KTU algorithm as in the pipeline mentioned above. The samples from the surface and deep chlorophyll maximum (DCM) layers of the maximum current subsets (sampling stations 2 and 3) were extracted for comparison to the current study.
The Illumina MiSeq platform generated a total of 2,655,526 high quality paired-end sequences, with an average of 912,519 non-chimeric reads, ranging from 31,024 to 64,034 reads/sample (median= 44,890 reads/sample; mean= 45,625.95 reads/sample) after DADA2 denoising. The QIIME2 pipeline coupling with KTU reclustering algorithm identified 3,927 ASVs and 1,938 KTUs (on average, 2.03 ASVs were clustered into each KTU; 98.86% mean sequence identity and 0.02 mean cosine divergence within KTUs; Figure S1). In general, the bacterial communities were different among the colonies from different locations (Figure 1). In both results of KTU and ASVs, Proteobacteria and Bacteroidetes were the dominant phyla in most of the samples (Figures 1A, B). In detail, Alphaproteobacteria occupied 31.55% of KTUs and 31.57% of ASVs; Gammaproteobacteria showed 10.18% of KTUs and 10.12% of ASVs; Cytophagia presented 22.57% KTUs and 22.47% of ASVs. Among them, Alphaproteobacteria was the most dominant class in GI and OK, but Cytophagia was the most dominant class in DS (Figures 1C, D).
Figure 1 Endolithic bacterial composition in Isopora spp. corals from three locations. (A) and (C) are bacterial phyla and classes, respectively, retrieved by KTU. (B, D) are bacterial phyla and class, respectively, retrieved by ASV. Location acronymous of samples: ES (Dongsha Atoll, Taiwan), DB (Green Island, Taiwan), GK (Green Island, Taiwan), WI (Okinawa, Japan), WS (Okinawa, Japan); please refer the Table 1 to see the detail sampling information.
The alpha diversity also showed a locational difference: the diversity from Dongsha (DS) was lower than those from Green Island (GI) and Okinawa (OK) (Figure S2). For the diversity indexes of KTU, the observed KTU (p=0.026) and Chao 1 (p=0.029) were significantly different among the three locations, while Shannon (p=0.054) and Simpson (p=0.082) did not show any significant difference. The pairwise Dunn’s test showed the diversity indexes—observed KTU (p=0.004), Chao 1 (p=0.0046) and Shannon diversity (p=0.0138)—were significantly different between DS and OK. The PCoA analysis of taxa composition for both KTU and ASV showed that the difference is more prominent when examining geological locations (Figure 2). In the PCoA of KTU, GI is separated from OK and DS, whereas in ASV, OK is separated from GI and DS. In summary, both ASV and KTU analyses demonstrated consistent biodiversity patterns, while the KTU results reflected higher associations with the environmental facts. Therefore, the following analyses were primarily based on KTU reclustering features, not raw ASV features.
Figure 2 Associations between the Isopora spp. endolithic microbiome and environment factors. (A) Geographical locations of three sampling sites (blue: Dongsha Atoll, DS; green: Green Island, GI; red: Bise-Okinawa, OK) in the western Pacific Ocean and South China Sea. (B–D) three components (factor axis, FA) for factor analysis of 14 environmental variables: (B) FA1, the Kuroshio Current and productivity factors; (C) FA2, the inorganic salts factors; (D) FA3, the minor local environmental factors. (E, I) the PCoA ordination of endolithic microbial beta diversity measured by Bray-Curtis dissimilarity using KTU and ASV, respectively. (F–H) and (J–L) the correlation analyses of KTU and ASV, respectively, with three FAs. Dash cubic lines represent the 95% confidence intervals of the correlations.
We investigated which ocean-geographical factors may affect bacterial compositions. Geological location is one of the main factors that causes the diversity in the bacterial community, and our further analysis of the other 14 environmental factors showed more specific correlations in bacterial compositions (Figure 2 and Table 1). Miscellaneous environmental factors were dimension reduced into three principal components (factor axes, FA). Among these, currents (current velocity, directions of the current: U. and V. component) and productivities (Chlorophyll a, phytoplankton, and primary production) explained 51.15% of FA1; inorganic factors (silicate, nitrate, salinity, temperature, and pH) explained 33.34% of FA2; and sampling depth explained 15.51% of FA3 (Figures 2B–D).
In the PCoA ordination of endolithic microbial beta diversity measured by Bray-Curtis dissimilarity using KTU (Figure 2E) and ASV (Figure 2I), PCoA1 and PCoA2 using KTU explained a higher ratio than they did using ASV. Even so, both KTU and ASV methods showed that bacterial communities were significantly separated by location. From both PCoA results, the PCoA1 separated OK from the other two locations, while PCoA2 separated GI from the other two locations.
According to the correlation analyses of KTU with three FAs (Figures 2F–H) and the correlation analyses of ASV with three FAs (Figures 2J–L), FA1 and FA3 showed a significantly positive correlation with the PCoA2, whereas FA2 showed a positive correlation with the PCoA1. Both KTU and ASV analyses showed that the current and productivity had high correlation with the Isopora endolithic bacterial community (in Figure 2F, KTU: FA1 R=0.5, p=0.026; in Figure 2J, ASV: FA1 R=0.63, p=0.003). Variables associated with FA2 and FA3 had higher correlation in KTU than ASV—in FA2, based on KTU, R=0.4, p=0.081 (Figure 2G), based on ASV, R=0.34, p=0.142 (Figure 2K); in FA3, based on KTU, R=0.48, p=0.031 (Figure 2H), and based on ASV, R=0.35, p=0.128 (Figure 2L). In addition, the correlation analyses of four alpha diversities with three FAs showed that alpha diversities were positively correlated with FA2, negatively correlated with FA3, and not correlated with FA1 (Table 2).
We compared the top 2.5% of bacterial taxa that were correlated with three factors in the three locations (Figure 3) and found that the same bacterial species in all three locations showed different relationships to the factors. For example, Endozoicomonas had a stronger positive association with factors of current and productivities only in GI. Additionally, OK yielded the most bacteria with positive associations to factors (Figures 3A–C). Although the results based on ASV (Figures 3D–F) showed a similar pattern to the results based on KTU, the ASV results showed fewer factors correlated with taxa than did the KTU results. For instance, there were 27 KTUs correlated with FA2, but only 13 ASVs correlated with FA2. Even most correlated ASVs were assigned to the same taxa, which are just the intra-species variants. Instead, the correlated KTUs reflected more independent taxa associated with environmental factors.
Figure 3 Heatmaps of three FAs correlated with the top taxa at the three locations. (A) FA1 (Kuroshio Current and productivity factors)-correlated KTUs. (B) FA2 (inorganic salts factors)-correlated KTUs. (C) FA3 (minor local environmental factors)-correlated KTUs. The top 2.5% KTU high Spearman correlation coefficients, P < 0.01 were selected for visualizing. Heatmap of three FAs correlated with the top taxa in the three locations. (D) FA1 (Kuroshio Current and productivity factors)-correlated ASVs. (E) FA2 (inorganic salts factors)-correlated ASVs. (F) FA3 (minor local environmental factors)-correlated ASVs.
According to our above-mentioned analysis of factors, FA1 factors were more related to bacterial composition in the Isopora skeletons than FA2 and FA3. In the map showing the average flow rate of currents in the western Pacific Ocean and South China Sea (Figure S3), both GI and OK were located in the mainstream of the KC, but DS was located in a branch current; in addition, GI was subjected to the KC more than OK (Figure S3).
To investigate the commonality of KC and Isopora endolithic bacteria, we retrieved a seawater microbiota dataset (Cheng et al., 2020) of the KC mainstream in the open sea of eastern Taiwan. Comparing the bacteria in seawater of the KC and dominant endolithic bacteria in the coral skeleton at all three locations, we found that overlapped indicator species in DS and the KC showed lower abundances in other two locations. Overlapped indicator species of GI and the KC showed a lower abundance in DS, but higher abundance in OK. Similarly, overlapped indicator species of OK and the KC showed lower abundance in DS, but higher abundance in GI (Figure S4).
To identify the anaerobes in coral skeletons, we consulted the taxonomy information of anaerobes and their physiological-metabolic potentials from Bergey’s Manual of Systematic Bacteriology. OK had the highest average relative abundance of anaerobes (20.43%), followed by GI (13.36%) and DS (9.43%) (Table 3). Furthermore, environmental factors showed different correlations to anaerobic and aerobic endoliths. In Figure 2, the FAs represent three features of environments (FA1 represents current velocity and productivities, FA2 represents inorganic nutrients or salts, and FA3 represents minor local environmental factors). Among these features, FA1 describes factors at a large spatial scale, whereas FA2 and FA3 are local features. According to the relationship between FAs and endolithic anaerobes, FA1 (r=-0.17), FA2 (r=0.29) and FA3 (r=0.0013) were positively correlated to the endolithic anaerobes. This result indicated that the endolithic composition in the Isopora skeleton may have stronger relationships to local environments when the large spatial scales of geographic difference are partialized from compounded environmental factors.
However, even though amplicon sequencing targets the 16S rRNA gene of prokaryotes, the organelle rDNA, including mitochondrial and chloroplast, from mixed coral symbionts, can be sequenced. Therefore, we did not directly analyze the endolithic eukaryotic composition (e.g. 18S rDNA); we still captured the 16S rDNA of endolithic algae in the coral skeleton. Then we found that the endolithic algae in GI are different from those in DS and OK (Figures S5A, C). Moreover, the endolithic algae composition was correlated with the environmental factors of the Kuroshio Current and productivity (FA1; r=-0.72, P < 0.001; Figure S5B).
Past studies have confirmed that microbial composition is influenced by light intensity, oxygen concentration, inorganic nutrient and pH in the microenvironment of the coral skeletons (Pernice et al., 2020; Chen et al., 2021). The internal structure of coral skeletons depends on the coral genus or species, suggesting that endolithic microbes have host specificity. In addition, the environment that corals are located in can change the morphology and texture of the skeleton (Tambutté et al., 2015), driving the microenvironment where microbes live (Yang et al., 2020). In this study, we processed the ASV features with KTU re-clustering and then identified the latent coral-microbe-environment interactive factors, such as concentrations of inorganic chemicals at the sampling sites.
Isopora corals were widely distributed in the warm, open ocean ecosystem, and lacked apparent physical barriers. However, their endosymbiotic bacterial compositions varied among populations of different locations. To identify variations in the endolithic microbiome based on ocean-geographical factors, we conducted amplicon sequence analyses with both high-resolution methods—ASV and KTU.
Regarding the comparison between KTU- and ASV-based microbiome analyses, the amplicon denoising methods correct sequencing errors, improve taxonomic resolution, and replace the traditional OTU clustering methods for microbiome analysis (Rosen et al., 2012). However, the high resolution of sequence features is a double-edged sword for ASV-based microbiome analysis. The high resolution ASVs aggravate the zero-inflation effect of sparse microbiome data. Here, we used the KTU re-clustering algorithm to aggregate trivial ASV features in the dataset. The KTU clustering method successfully calibrated ASV features in closed systems (gastrointestinal tracts, bioreactor, and sourdough microbiome) in previous studies (Liu et al., 2022). Since the KTU features are clustered by their tetra-nucleotide patterns, which represents the resolution of variation in sequences at global-wise scale rather than the local variation in nucleotides, we suggest that this method is applicable for open systems, such as soil, lake, and ocean microbiomes.
There were 3,927 ASVs identified and clustered into 1,938 KTUs in this study. Data explanation efficacy, such as multiple environmental factors correlation to microbial composition, was improved after re-clustering (Figure 2). The explanation percentage of the first two coordinates of beta diversity increased from 19.31% (ASV) to 22.88% (KTU). Both the ASV and KTU were significantly correlated to FA1, with medium-high correlation coefficients. This indicates that the ocean currents (Kuroshio) and productivity (represented as FA1) would be the major physical factors analyzed in this study that contribute to the Isopora-microbes interactions in geographical differentiation. The re-clustering method would reduce zero-inflation in sequence-based microbial community surveys, thus truly reflecting environmental associations (Lin et al., 2020). Even if the ASV improves the resolution of amplicon variants, it also eliminates the gradient variations of biological dynamics by over zero-inflation. It is prone to false correlations or significant differences caused by extreme values (Liu et al., 2022). Moreover, in any microbiome-associated study, we suggested that both data types of the microbiome and environmental factors would be better as a continuous distribution rather than discrete.
According to previous studies (Yang et al., 2016; Yang et al., 2019; Yang et al., 2020), Isopora spp. have a dense and non-porous skeletal structure. Therefore, it forms an anaerobic microenvironment to the symbiotic bacteria. The abundance of anaerobic endolithic bacteria in coral skeletons and the average relative abundance of anaerobes were different in the three locations.
In this study, we conducted KTU re-clustering to aggregate the sparse data on the coral skeleton microbiome. The results showed significant correlations between microbial composition and broad- and local-scale environmental factors, including the ocean currents, productivity, composition of inorganic salts, and local environment factors. We provide comprehensive results that illustrate the local topographical differences and environmental factors related to endolithic microbial diversity and constitution.
According to our results based on both KTU and ASV, water current velocity and primary productivity are positively correlated to PCoA2, which effectively distinguished bacterial compositions between samples from the reef flat (DS and OK) and from other places. Cyronak et al. (2020) found that shallow reef systems (e.g. reef flat) exhibit wider changes in environmental conditions, including pH and temperature. In addition, the topography of lagoon-like areas decides the current velocity, which influence the dissolved organic matter in the seawater (Vanwonterghem and Webster, 2020). These factors may result in changes to the seawater carbonation chemistry, which likely influences the coral skeleton properties and microbe niches inside. Therefore, we suggest that the local topology of reefs influences the endolithic microbial composition in coral skeletons.
In addition to the seawater chemistry of reef flat environments, sampling depths may influence the endolithic microbial composition in the coral skeleton. Regarding our results, the minor local environmental factors (FA3)—including depth—showed a positive correlation with PCoA2, which may echo the influence from the local topology of reefs. Furthermore, bacterial communities and chloroplast compositions in DS and OK are closer to each other than they are to GI. Samples from DS and OK are from a shallower environment (1-5 meters) than those in GI. Corals at shallower depths are subjected to wider tidal changes, light intensity and dissolved oxygen in seawater (Cyronak et al., 2020). Hence Isopora may experience a stronger light intensity and oxygen concentration in their skeletons, promoting the growth of endolithic green algae and photoautotrophic bacteria.
The KC strongly influences the regional hydroclimate by creating temperature, salinity, and pH gradients from tropical to subtropical and temperate zones (Dai, 1991; Abbot et al., 2003). In our previous studies, we found that latitude may be one of the factors influencing microbial composition in tissues of a coral species that was sampled along the KC (Woo et al., 2017; Yang et al., 2017; Yang et al., 2020). In this study, PCoA1, which was positively correlated with FA2, distinguished between the tropical (DS and GI) and the subtropical sampling sites (OK). Additionally, FA2, correlated with the top bacterial taxa, showed that the taxa from DS and GI are more similar. Hence, we suggest that the latitude of the sampling site along the KC (Figure S4) may also be a factor deciding the endolithic microbial composition. However, unlike coral tissues associated with bacterial richness—which are lower at higher latitudes (Pollock et al., 2018; Yang et al., 2020)—in the present study, coral skeleton-associated bacterial richness was higher at higher latitudes. Further investigations combining spatial and temporal conditions are needed to elucidate the role of latitude in the bacterial composition of coral skeletons.
To determine whether endolithic microbial compositions are influenced by the KC, we compared the bacterial constitutions in the coral skeleton and seawater from the KC mainstream in our study to those of Cheng et al. (2020). In this study, bacteria in the GI that were highly correlated with FA1 were Pseudomonas, Rhodobacteraceae, Sva0996, and bacteria in the OK that were highly correlated to FA1 were Rhodospirillaceae, Coxiella, Rhodopirellula, and Sva0996. These bacteria overlapped the indicator species among the KC. In addition, both the alpha diversities and overlapped bacterial taxa were higher in the locations in the KC mainstream (GI and OK) than the KC branch (Dongsha). Hence, we suggested that the dominant bacterial taxa in the KC may be involved in the microbial composition in the coral skeleton.
Interestingly, compared to the bacterial taxa in DS and OK, we found a higher relative abundance of bacteria related to the sulfur cycle in the GI, such as Deltaproteobacteria, Chlorobi and Firmicutes. This finding echoes our previous results, that Chlorobi, Deltaproteobacteria and Firmicutes were dominant in coral skeletons (Yang et al., 2016; Yang et al., 2019; Chen et al., 2021). Unlike the other two locations—which are reef islands—the GI is a volcanic island. There are hot springs close to the island’s shore, and the hot spring water and seawater mix (Zheng et al., 2017). Although the exact sulfide concentration of seawater samples is unknown, a previous study found that the seawater surrounding volcanic islands and the host spring environment have higher concentrations of sulfide (Chen et al., 2005). This may explain why sulfur reducers and sulfur oxidizers are consistently dominant taxa in the skeletons of GI corals. Although further geochemistry data is needed, we suggest that the geological substance of the locations may also play a role in deciding the microbial composition in coral skeletons.
The influence of the environment on the microbial composition in coral is complex, especially for coral endolithic communities. Here, by investigating the relationship between microbial composition and locational environmental factors, we expand on the knowledge of potential biotic and abiotic factors that correlate with the microbial constitution in skeletons of Isopora from different locations. Although endolithic microbes may show host specificity because of the different skeleton structures of different hosts, endolithic microbes in Isopora spp. were mainly related to water currents, Chlorophyll a, phytoplankton and primary production in the surrounding water. In addition, using the KTU algorithm to re-cluster over-sparse sequence variants, we retrieved a continuous, not discrete, distribution of microbiome and environmental factors, showing gradient variations in the biological dynamics that were eliminated by ASV algorithm. Therefore, we suggest that KTU algorithm reclustering is an effective method for analyzing the relationships between microbial diversity and environment.
The datasets presented in this study can be found in online repositories. The names of the repository/repositories and accession number(s) can be found below: NCBI [accession: PRJNA686201].
P-YL: data analysis and manuscript writing (result and discussion). S-YY: sampling (Dongsha), data analysis, and manuscript writing. C-YL: performed molecular experiment. NW: sampling (Okinawa). SP: sampling (Green Island). S-SY: data analysis. HY: sampling (Okinawa). S-LT: conceived of the idea and research design. S-HY: conceived of the idea, manuscript writing, and research design. All authors contributed to the article and approved the submitted version.
This work was funded by the Ministry of Science and Technology (MOST) of Taiwan through grants to S-LT No. 108-2621-B-001-005-MY3 and S-HY no. 109-2621-B-002 -005 -MY3.
The authors declare that the research was conducted in the absence of any commercial or financial relationships that could be construed as a potential conflict of interest.
All claims expressed in this article are solely those of the authors and do not necessarily represent those of their affiliated organizations, or those of the publisher, the editors and the reviewers. Any product that may be evaluated in this article, or claim that may be made by its manufacturer, is not guaranteed or endorsed by the publisher.
We thank Dr. Chaolun Allen Chen for assisting sampling, and Mr. Noah Last of Third Draft Editing for editing the manuscript’s language. We thank Green Island Marine Research Station at Green island (Taiwan) and Sesoko Station at Sesoko island (Okinawa).
The Supplementary Material for this article can be found online at: https://www.frontiersin.org/articles/10.3389/fmars.2022.850984/full#supplementary-material
Abbot P., Celuzza S., Dyer I., Gomes B., Fulford J., Lynch J. (2003). Effects of East China Sea Shallow-Water Environment on Acoustic Propagation. IEEE J. Ocean. Eng. 28 (2), 192–211. doi: 10.1109/JOE.2003.811901
Amir A., McDonald D., Navas-Molina J. A., Kopylova E., Morton J. T. (2017). Deblur Rapidly Resolves Single-Nucleotide Community Sequence Patterns. MSystems 2 (2), e00191-16. doi: 10.1128/mSystems.00191-16
Bokulich N. A., Kaehler B. D., Rideout J. R., Dillon M., Bolyen E., Knight R., et al. (2018). Optimizing Taxonomic Classification of Marker-Gene Amplicon Sequences With QIIME 2’s Q2-Feature-Classifier Plugin. Microbiome 6 (1), 1–17. doi: 10.1186/s40168-018-0470-z
Bolyen E., Rideout J. R., Dillon M. R., Bokulich N. A., Abnet C. C., Al-Ghalith G. A., et al. (2019). Reproducible, Interactive, Scalable and Extensible Microbiome Data Science Using QIIME 2. Nat. Biotechnol. 37 (8), 852–857. doi: 10.1038/s41587-019-0209-9
Callahan B. J., McMurdie P. J., Rosen M. J., Han A. W., Johnson A. J. A., Holmes S. P. (2016). DADA2: High-Resolution Sample Inference From Illumina Amplicon Data. Nat. Methods 13 (7), 581–583. doi: 10.1038/nmeth.3869
Camacho C., Coulouris G., Avagyan V., Ma N., Papadopoulos J., Bealer K., et al. (2009). BLAST+: Architecture and Applications. BMC Bioinform. 10 (1), 1–9. doi: 10.1186/1471-2105-10-421
Cheng W. H., Lu H. P., Chen C. C., Jan S., Hsieh C. H. (2020). Vertical Beta-Diversity of Bacterial Communities Depending on Water Stratification. Front. Microbiol. 11. doi: 10.3389/fmicb.2020.00449
Chen C. P., Tseng C. H., Chen C. A., Tang S. L. (2011). The Dynamics of Microbial Partnerships in the Coral Isopora Palifera. ISME J. 5 (4), 728–740. doi: 10.1038/ismej.2010.151
Chen Y. H., Yang S. H., Tandon K., Lu C. Y., Chen H. J., Shih C. J., et al. (2021). Potential Syntrophic Relationship Between Coral-Associated Prosthecochloris and its Companion Sulfate-Reducing Bacterium Unveiled by Genomic Analysis. Microb. Genom. 7 (5), 000574. doi: 10.1099/mgen.0.000574
Chen C. T. A., Zeng Z., Kuo F. W., Yang T. F., Wang B. J., Tu Y. Y. (2005). Tide-Influenced Acidic Hydrothermal System Offshore NE Taiwan. Chem. Geol. 224 (1-3), 69–81. doi: 10.1016/j.chemgeo.2005.07.022
Cyronak T., Takeshita Y., Courtney T. A., DeCarlo E. H., Eyre B. D., Kline D. I., et al. (2020). Diel Temperature and pH Variability Scale With Depth Across Diverse Coral Reef Habitats. Limnol. Oceanogr. Lett. 5 (2), 193–203. doi: 10.1002/lol2.10129
Dai C. F. (1991). Reef Environment and Coral Fauna of Southern Taiwan. Atoll Res. Bull. 354, 1–24. doi: 10.5479/si.00775630.354.1
del Campo J., Pombert J. F., Šlapeta J., Larkum A., Keeling P. J. (2017). The ‘Other’ Coral Symbiont: Ostreobium Diversity and Distribution. ISME J. 11, 296–299. doi: 10.1038/ismej.2016.101
Dick G. J., Andersson A. F., Baker B. J., Simmons S. L., Thomas B. C., Yelton A. P., et al. (2009). Community-Wide Analysis of Microbial Genome Sequence Signatures. Genome Biol. 10, R85. doi: 10.1186/gb-2009-10-8-r85
Edgar R. C. (2010). Search and Clustering Orders of Magnitude Faster Than BLAST. Bioinformatics 26 (19), 2460–2461. doi: 10.1093/bioinformatics/btq461
Edgar R. C. (2013). UPARSE: Highly Accurate OTU Sequences From Microbial Amplicon Reads. Nat. Methods 10 (10), 996–998. doi: 10.1038/nmeth.2604
Edgar R. C. (2016). UNOISE2: Improved Error-Correction for Illumina 16S and ITS Amplicon Sequencing. BioRxiv, 081257. doi: 10.1101/081257
Ferrer L. M., Szmant A. M. (1988). “Nutrient Regeneration by the Endolithic Community in Coral Skeletons,” in Proceedings of the 6th International Coral Reef Symposium, vol. 2. (Townsville, Australia: AIMS), 1–4.
Fine M., Loya Y. (2002). Endolithic Algae: An Alternative Source of Photoassimilates During Coral Bleaching. Proc. R. Soc. Lond. Ser. B: Biol. Sci. 269 (1497), 1205–1210. doi: 10.1098/rspb.2002.1983
Huang C. H., Li S. W., Huang L., Watanabe K. (2018). Identification and Classification for the Lactobacillus Casei Group. Front. Microbiol. 9, 1974. doi: 10.3389/fmicb.2018.01974
Lin D., Lacey E. A., Bach B. H., Bi K., Conroy C. J., Suvorov A., et al. (2020). Gut Microbial Diversity Across a Contact Zone for California Voles: Implications for Lineage Divergence of Hosts and Mitonuclear Mismatch in the Assembly of the Mammalian Gut Microbiome. Mol. Ecol. 29 (10), 1873–1889. doi: 10.1111/mec.15443
Liu P. Y. (2021). Poyuliu/MARco: MARco: Microbiome Analysis RcodeDB (Version V1.0). (Taipei, Taiwan: Zenodo) doi: 10.5281/zenodo.4589898
Liu P. Y., Cheng A. C., Huang S. W., Lu H. P., Oshida T., Liu W., et al. (2020). Body-Size Scaling is Related to Gut Microbial Diversity, Metabolism and Dietary Niche of Arboreal Folivorous Flying Squirrels. Sci. Rep. 10 (1), 1–12. doi: 10.1038/s41598-020-64801-y
Liu P. Y., Yang S. H., Yang S. Y. (2022). KTU: K-Mer Taxonomic Units Improve the Biological Relevance of Amplicon Sequence Variant Microbiota Data. Methods Ecol. Evol. 13 (3), 560–568. doi: 10.1111/2041-210X.13758
Martin M. (2011). Cutadapt Removes Adapter Sequences From High-Throughput Sequencing Reads. EMBnet J. 17 (1), 10–12. doi: 10.14806/ej.17.1.200
Niemi G. J., Hanowski J. M., Lima A. R., Nicholls T., Weiland N. (1997). A Critical Analysis on the Use of Indicator Species in Management. J. Wildl. Manage. 61 (4), 1240. doi: 10.2307/3802123
Oksanen J., Blanchet F. G., Kindt R., Legendre P., Minchin P. R., O’hara R. B., et al. (2015) Vegan: Community Ecology Package. R Package Version 2.2-1. Available at: http://CRAN.Rproject.org/package=vegan2015.
Pernice M., Raina J. B., Rädecker N., Cárdenas A., Pogoreutz C., Voolstra C. R. (2020). Down to the Bone: The Role of Overlooked Endolithic Microbiomes in Reef Coral Health. ISME J. 14 (2), 325–334. doi: 10.1038/s41396-019-0548-z
Pollock F. J., McMinds R., Smith S., Bourne D. G., Willis B. L., Medina M., et al. (2018). Coral-Associated Bacteria Demonstrate Phylosymbiosis and Cophylogeny. Nat. Commun. 9 (1), 1–13. doi: 10.1038/s41467-018-07275-x
Quast C., Pruesse E., Yilmaz P., Gerken J., Schweer T., Yarza P., et al. (2013). The SILVA Ribosomal RNA Gene Database Project: Improved Data Processing and Web-Based Tools. Nucleic Acids Res. 41 (D1), D590–D596. doi: 10.1093/nar/gks1219
R Core Team. (2015). R: A Language and Environment for Statistical Computing. (Vienna, Austria: R Foundation for Statistical Computing).
Ricci F., Fordyce A., Leggat W., Blackall L. L., Ainsworth T., Verbruggen H. (2021). Multiple Techniques Point to Oxygenic Phototrophs Dominating the Isopora Palifera Skeletal Microbiome. Coral Reefs 40 (2), 275–282. doi: 10.1007/s00338-021-02068-z
Ricci F., Rossetto Marcelino V., Blackall L. L., Kühl M., Medina M., Verbruggen H. (2019). Beneath the Surface: Community Assembly and Functions of the Coral Skeleton Microbiome. Microbiome 7 (1), 1–10. doi: 10.1186/s40168-019-0762-y
Rosen M. J., Callahan B. J., Fisher D. S., Holmes S. P. (2012). Denoising PCR-Amplified Metagenome Data. BMC Bioinform. 13 (1), 1–16. doi: 10.1186/1471-2105-13-283
Stackebrandt E., Goebel B. M. (1994). Taxonomic Note: A Place for DNA-DNA Reassociation and 16S rRNA Sequence Analysis in the Present Species Definition in Bacteriology. Int. J. Syst. Evol. Microbiol. 44 (4), 846–849. doi: 10.1099/00207713-44-4-846
Tambutté E., Venn A. A., Holcomb M., Segonds N., Techer N., Zoccola D., et al. (2015). Morphological Plasticity of the Coral Skeleton Under CO 2-Driven Seawater Acidification. Nat. Commun. 6 (1), 1–9. doi: 10.1038/ncomms8368
Tribollet A., Golubic S. (2005). Cross-Shelf Differences in the Pattern and Pace of Bioerosion of Experimental Carbonate Substrates Exposed for 3 Years on the Northern Great Barrier Reef, Australia. Coral Reefs 24 (3), 422–434. doi: 10.1007/s00338-005-0003-7
Vanwonterghem I., Webster N. S. (2020). Coral Reef Microorganisms in a Changing Climate. Iscience 23 (4), 100972. doi: 10.1016/j.isci.2020.100972
Woo S., Yang S. H., Chen H. J., Tseng Y. F., Hwang S. J., De Palmas S., et al. (2017). Geographical Variations in Bacterial Communities Associated With Soft Coral Scleronephthya Gracillimum. PloS One 12 (8), e0183663. doi: 10.1371/journal.pone.0183663
Yang S. H., Lee S. T., Huang C. R., Tseng C. H., Chiang P. W., Chen C. P., et al. (2016). Prevalence of Potential Nitrogen-Fixing, Green Sulfur Bacteria in the Skeleton of Reef-Building Coral Isopora Palifera. Limnol. Oceanogr. 61 (3), 1078–1086. doi: 10.1002/lno.10277
Yang S. Y., Lu C. Y., Tang S. L., Das R. R., Sakai K., Yamashiro H., et al. (2020). Effects of Ocean Acidification on Coral Endolithic Bacterial Communities in Isopora Palifera and Porites Lobata. Front. Mar. Sci. doi: 10.3389/fmars.2020.603293
Yang S. Y., Tseng C. H., Huang C. R., Chen C. P., Tandon K., Lee S. T. M., et al. (2017). Long-Term Survey Is Necessary to Reveal Various Shifts of Microbial Composition in Corals. Front. Microbiol. 8, 1094. doi: 10.3389/fmicb.2017.0109
Yang S. H., Tandon K., Lu C. Y., Wada N., Shih C. J., Hsiao S. S. Y., et al. (2019). Metagenomic, Phylogenetic, and Functional Characterization of Predominant Endolithic Green Sulfur Bacteria in the Coral Isopora Palifera. Microbiome 7 (1), 1–13. doi: 10.1186/s40168-018-0616-z
Yilmaz P., Parfrey L. W., Yarza P., Gerken J., Pruesse E., Quast C., et al. (2014). The SILVA and “All-Species Living Tree Project (LTP)” Taxonomic Frameworks. Nucleic Acids Res. 42 (D1), D643–D648. doi: 10.1093/nar/gkt1209
Youssef N. H., Ashlock-Savage K. N., Elshahed M. S. (2012). Phylogenetic Diversities and Community Structure of Members of the Extremely Halophilic Archaea (Order Halobacteriales) in Multiple Saline Sediment Habitats. Appl. Environ. Microbiol. 78 (5), 1332–1344. doi: 10.1128/AEM.07420-11
Zheng J., Wittouck S., Salvetti E., Franz C., Harris H. M. B., Mattarelli P., et al. (2020). A Tax-Onomic Note on the Genus Lactobacillus: Description of 23 Novel Genera, Emended Description of the Genus Lactobacillus Beijerinck 1901, and Union of Lactobacillaceae and Leuconostocaceae. Int. J. Syst. Evol. Microbiol. 70, 2782–2858. doi: 10.1099/ijsem.0.004107
Keywords: Isopora, endolithic bacteria, KTU, Kuroshio Current, marine environmental factors
Citation: Liu P-Y, Yang S-Y, Lu C-Y, Wada N, De Palmas S, Yeh S-S, Yamashiro H, Tang S-L and Yang S-H (2022) Ocean Currents May Influence the Endolithic Bacterial Composition in Coral Skeletons. Front. Mar. Sci. 9:850984. doi: 10.3389/fmars.2022.850984
Received: 08 January 2022; Accepted: 14 March 2022;
Published: 05 April 2022.
Edited by:
Yoshitomo Kikuchi, National Institute of Advanced Industrial Science and Technology (AIST), JapanReviewed by:
Francesco Ricci, University of New South Wales, AustraliaCopyright © 2022 Liu, Yang, Lu, Wada, De Palmas, Yeh, Yamashiro, Tang and Yang. This is an open-access article distributed under the terms of the Creative Commons Attribution License (CC BY). The use, distribution or reproduction in other forums is permitted, provided the original author(s) and the copyright owner(s) are credited and that the original publication in this journal is cited, in accordance with accepted academic practice. No use, distribution or reproduction is permitted which does not comply with these terms.
*Correspondence: Shan-Hua Yang, c2hhbmh1YUBudHUuZWR1LnR3
†ORCID: Po-Yu Liu, orcid.org/0000-0003-1290-0850
Sung-Yin Yang, orcid.org/0000-0001-7431-406X
Shan-Hua Yang, orcid.org/0000-0002-1999-5269
‡These authors share first authorship
Disclaimer: All claims expressed in this article are solely those of the authors and do not necessarily represent those of their affiliated organizations, or those of the publisher, the editors and the reviewers. Any product that may be evaluated in this article or claim that may be made by its manufacturer is not guaranteed or endorsed by the publisher.
Research integrity at Frontiers
Learn more about the work of our research integrity team to safeguard the quality of each article we publish.