- 1Research Institute of Ecoscience, Ewha Womans University, Seoul, South Korea
- 2Department of Applied Statistics, Kyonggi University, Suwon, South Korea
- 3Division of Ecoscience, Ewha Womans University, Seoul, South Korea
The population divergence process of deep-sea vent invertebrates is driven by both biotic (e.g., dispersal during the larval stage) and abiotic factors such as deep-ocean currents, depth, and the geological setting of vents. However, little is known regarding the divergence of hydrothermal vent microorganisms. Therefore, our study sought to investigate the influence of geological and geographic factors on the divergence of symbiotic bacteria of Bathymodiolus vent mussels. The genetic differentiation patterns of symbionts were examined using next-generation sequencing DNA data in two ocean basins with distinct geological features: the slow-spreading Central Indian Ridge (CIR) and the fast- or superfast-spreading eastern Pacific Ridges. Our findings showed that the degree of differentiation of symbiont populations was geographically hierarchical: the highest between ocean basins, followed by inter-ridge sites between the East Pacific Rise and the Pacific Antarctic Ridge. The Easter Microplate intervening these two ridges acted as a biogeographic physical barrier for both symbionts and their host mussels. On a scale of intra-ridge, symbionts showed isolation by distance in the CIR but not in the eastern Pacific ridges. These contrasting genetic patterns relate to different ridge spreading rates determining most of the geological characteristics of mid-ocean ridges that affect the connectivity of vent habitats in space and time. At the intra-ridge geographic scale of the CIR, population divergence processes of both symbionts and hosts from separate three ridge segments were analyzed in detail using a genetic model of isolation with migration (IM). The phylogenetic topology of symbiont populations was congruent with the host populations, indicating the influence of common historical and physical constraints for habitats and dispersal between vents in the Central Indian Ridge. Collectively, our findings provide key insights into the dynamics of microbial population divergence in deep-sea vents.
Introduction
Studies on macroorganisms suggest that they share phylogeographic patterns with symbiotic microorganisms, although the spatiotemporal scale of these patterns may vary between the two organismic groups. The spatial distribution of microorganisms is mainly constrained by environmental factors, causing niche partitioning and geographical distance between habitats (De Wit and Bouvier, 2006; Hanson et al., 2012; Van Der Gast, 2015). However, the relative magnitude of the two factors remains controversial. Initially, environmental variables were considered the major factors that shape the biogeographical distribution of microorganisms, which supported Bass-Becking’s hypothesis: “everything is everywhere, the environment selects” (Finlay and Clarke, 1999). The influence of geographical distance was excluded based on the assumption that microorganisms have unlimited dispersal capability due to their extremely small sizes and massive population sizes. However, a growing body of evidence has suggested that bacterial populations are in fact affected by geographical distance, as demonstrated by studies on the distribution patterns of archaea populations and the phylogeny of hot spring cyanobacteria (Papke et al., 2003; Whitaker et al., 2003). Furthermore, the dispersal of endemic taxa inhabiting isolated habitats appears to be particularly affected by geographic distance (Louca, 2021).
The hydrothermal vent ecosystem is a representative isolated habitat with spatially and temporally discontinuous distribution along the mid-ocean ridge and back-arc basin. Nevertheless, the environmental variations of vent fields are considered important determinants of biogeographical patterns due to the close association between the chemistry of vents and bacterial metabolism. Previous studies have analyzed vent geochemistry and fluid chemistry to characterize their effect on the geographical structure of vent microorganisms by comparing community compositions at various spatial scales (Campbell et al., 2006; Flores et al., 2011; Anderson et al., 2015; Reveillaud et al., 2016; Meier et al., 2017). Interestingly, Huber et al. (2010) proposed that geographic isolation rather than the chemistry of diffuse flow regions affected the biogeographic patterns of Epsilonproteobacteria at a large spatial scale (>1,000 km). Likewise, the effect of geographic isolation was observed from the gill-associated symbiotic bacteria of Bathymodioline mussel, Idas modiolaeformis, collected from sunken wood and deep in the east Atlantic and Mediterranean (Laming et al., 2015). A comparison among several sites distributed broadly represented that the dissimilarity of bacterial composition was positively correlated with the geographical distance between habitats. The geographic distance was recently proven to be an essential factor for the divergence of the symbiotic bacteria of deep-sea vents: symbionts of northern Mid-Atlantic Ridge (MAR) Bathymodiolus mussels (Ücker et al., 2021) and symbionts of scaly-foot vent snails, Chrysomallon squamiferum, in the Indian Ocean Ridge (Lan et al., 2022). These few pioneering studies cast research questions about the connectivity and divergence of bacteria of deep-sea hydrothermal vents between mid-ocean ridge systems showing different dynamics of tectonic activities and geological features involving various geographic distance scales, geomorphological structures, and so on.
In the deep-sea hydrothermal vent ecosystem, most invertebrates rely on chemosynthetic bacteria harbored in the specialized organs for the absorption of key nutrients. Therefore, acquiring symbiotic bacteria is directly linked to the survival of host animals. Host animals acquire the bacterial symbiont from the environment (horizontal transmission) or directly from the parent through gametes (vertical transmission) or by a mixed transmission mode of them (leaky vertical transmission). Bathymodioline mussels inhabit deep-sea chemosynthetic environments and harbor sulfur-oxidizing and/or methane-oxidizing bacteria in their gills. The host mussels obtain their symbionts from the environment through horizontal transmission, which has been demonstrated by discrepancies between the phylogenic topology of hosts and symbionts, as well as genome size similarities between symbionts and free-living bacteria (Won et al., 2003; Won et al., 2008; Fontanez and Cavanaugh, 2014; Russell et al., 2020). Fontanez and Cavanaugh (2014) detected strains of symbiotic bacteria in seawater and biofilms, suggesting that the mussel symbionts are acquired from free-living bacterial populations with presumably larger gene pools. Recently, Franke et al. (2021) discovered that the acquisition of symbionts in bathymodioline mussels first occurs during metamorphosis and after settlement. Moreover, the cellular anatomy of Bathymodiolus septemdierum demonstrated that there are pathways that connect the internal bacteriocytes to the external environment (Ikuta et al., 2021). Therefore, we hypothesize that population genetic studies of the symbiotic bacteria of mussels, which constitute enriched samples of environmental free-living bacteria, can provide insights into the diverging process of microbial populations in the deep-sea hydrothermal vents.
Bathymodioline mussels inhabiting the central Indian and eastern Pacific Oceans harbor sulfur-oxidizing bacteria, and the symbiont communities of mussels are dominantly composed of a single phylotype (Won et al., 2008; Ho et al., 2017). The two regions have substantially different seafloor spreading rates, with the Central Indian Ridge (CIR) exhibiting slow spreading and the East Pacific Rise (EPR) and Pacific Antarctic Ridge (PAR) of the eastern Pacific Ocean exhibiting fast or ultrafast spreading. The geological characteristics of vents influence genetic diversity in a metapopulational context on a local scale and the geographical connectivity of vent animals on a regional scale (Vrijenhoek, 2010; Mullineaux et al., 2018). In the eastern Pacific Ocean, vent invertebrates exhibit genetically subdivided population structures due to the geographical barrier around the equator and the Easter Microplate on the southern EPR (Vrijenhoek, 2010). Bathymodioline mussels and their symbiotic bacteria also showed geographically subdivided distributions across the Easter Microplate boundary, suggesting that the physical barrier not only interrupted host dispersal but also affected its symbionts (Johnson et al., 2013; Ho et al., 2017). However, the spatial structure of vent populations along the CIR, particularly between the Onnuri and other vent fields, remains uncharacterized. The Onnuri field, located approximately 780 km north of the Dodo vent field, is the most recently discovered vent field along the CIR (Kim et al., 2020). To date, a total of five vent fields have been found along the CIR: the Kairei and Edmond vent fields near the Rodrigues Triple Junction (Van Dover et al., 2001), the Solitaire and Dodo vent fields in the north (Nakamura et al., 2012), and the Onnuri field in the northernmost region of the CIR (Kim et al., 2020). Previous studies based on mitochondrial genes revealed the high geographical connectivity of invertebrates among the Solitaire, Edmond, and Kairei (Beedessee et al., 2013; Chen et al., 2015; Sun et al., 2020; Zhou et al., 2022). The vent mussels of the Indian Ocean, Bathymodiolus marisindicus, exhibited high genetic connectivity within a more geographically extended range than other invertebrates, extending from the Solitaire vent in the CIR to the Longqi vent in the South West Indian Ridge (SWIR) (Sun et al., 2020).
Our study aimed to explore the population divergence of bacteria in a representative hydrothermal vent environment by comparing genetically differentiated patterns of vent bacteria under distinct geological settings: the slow-spreading CIR and the fast-spreading eastern Pacific (EPR and PAR). Most previous studies have compared the composition of taxa based on 16S rDNA sequences at broad taxonomic levels (e.g., genus or family levels) to detect the biogeographic patterns of microorganisms. However, a finer taxonomic resolution is needed to detect the effect of distance on microbial biogeographic patterns (Hanson et al., 2012). Here, we examined the genetic variation of mussel gill-associated sulfur-oxidizing bacteria belonging to the SUP05 clade based on amplicon sequences obtained by next-generation sequencing (NGS) of multiple protein-coding genes and 16S rRNA to achieve an acceptable resolution. We compared the genetic differentiation patterns of the symbiotic bacteria in a geographically hierarchical manner from different ocean basins, ridges, and vent fields. Finally, we explored the diverging processes of symbionts and their host mussels among three adjacent ridge segments of the CIR by applying a population divergence genetics model known as the isolation with migration (IM) model to the NGS DNA data of symbiont populations and PCR-sequenced DNA data of host populations.
Materials and methods
Sampling
Bathymodiolus marisindicus mussels were collected from four hydrothermal vent fields on the CIR (Table 1, Figure 1). The mussels were obtained from the Onnuri and the Solitaire vent fields using a video-guided hydraulic grab (Oktopus GmbH, Germany, Dive number: GTV1702, GTV1807, and GTV1809) and from the Edmond and Kairei vent fields using a remotely operated vehicle, Jason I (Dive number: JL301, JL296). All samples were frozen at −80°C on board, transported to the laboratory on dry ice, and stored at −80°C until required for downstream analyses.
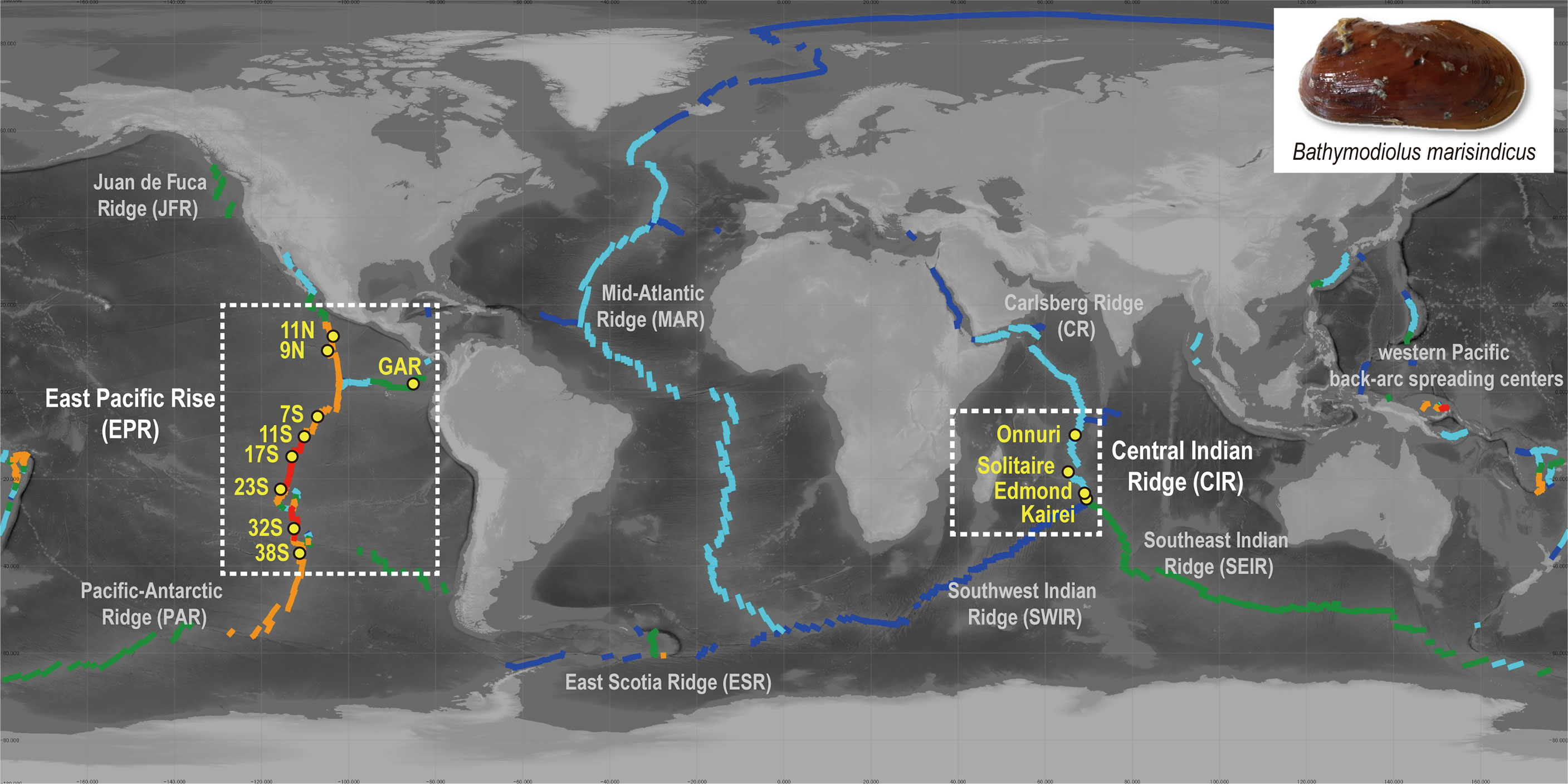
Figure 1 Distribution of sampling sites in this study and spreading rate of ridge axes. The colors of the ridge axes represent their spreading rate (Bird, 2003): ultra-slow (dark blue, <20 mm/year), slow (light blue, 20–50 mm/year), intermediate (green, 50–80 mm/year), fast (orange, 30–140 mm/year), and superfast (red, >140 mm/year). Map created with QGIS v. 3.12.3 using bathymetry data from the General Bathymetric Chart of the Oceans (GEBCO).
Genomic DNA extraction and sequencing
The genomic DNA of the mussels was extracted from each specimen’s foot or mantle tissue using the Qiagen DNeasy Tissue kit (Qiagen Inc., Hilden, Germany). One mitochondrial gene (mtCOI) and two nuclear genes (EF1α and Col-1) were amplified using gene-specific primer sets with primer-specific PCR conditions previously designed for bathymodioline mussels (Supplementary Table S1). The PCR was performed in 20 μl volumes including 2 μl of 10× Taq polymerase buffer, 1.5 μl of 2.5 mM stock solution of dNTPs, 1.5 μl of each primer (10 μmol/l), 1 μl of 1 mg/ml bovine serum albumin, 1 μl of extracted DNA (30–150 ng), 0.625 units of IP Taq polymerase (Cosmo Genetech Inc., South Korea), and sterile H2O to reach the desired volume. The PCR products were purified using the Dr. Prep Kit (Cat. No. MK02020, MGmed, South Korea). The DNA sequences were then determined using a Big Dye Terminator V3.1 Cycle Sequencing Kit on an Applied Biosystems 3730xl DNA Analyzer (Applied Biosystems Inc., South Korea). All sequences from the host mussels were deposited in the GenBank database under accession numbers OK509086–OK509159 (mtCOI), OK524315–OK524440 (EF1a), and OK524441–OK524584 (Col).
The genomic DNA of symbiotic bacteria was extracted from the gill tissues of individual mussels using the Qiagen DNeasy Tissue Kit (Qiagen Inc., Hilden, Germany). We targeted single-copy genes including 16S rRNA and six functional genes with different functional categories used in previous studies on sulfur-oxidizing symbionts of deep-sea mussels of the genus Bathymodiolus: sulfur metabolism (dsrB), protein folding (dnaK), glycolysis (pfk, pgi, and pykF), transcription regulation (rpoD) (Fontanez and Cavanaugh, 2014; Ho et al., 2017). Libraries for Illumina-MiSeq sequencing were prepared via two-step PCR. First, fragments of seven genes were amplified using specific primer sets with the following conditions (Supplementary Table S2): 95°C for 3 min; 30 cycles at 95°C for 40 s, 50°C–55°C for 30 s, and 72°C for 90 s, and 72°C for 7 min. The 16S rRNA gene was amplified using a universal prokaryotic primer set targeting the V3 and V4 regions. Functional genes were amplified using a thiotroph-specific primer set designed based on the previously deposited sequences of B. marisindicus sulfur-oxidizing endosymbionts and newly generated sequences by PacBio RS II (unpublished data sequenced from gill tissue of specimen “S10” collected at the Solitaire field in 2017). In the second PCR, unique pairs of Illumina Nextera Indices (N7/S5) were attached to the end of the amplified fragments to separate individual hosts. After PCR purification using AMpure XP magnetic beads (Edwards, 2012), the amplicons were pooled at equal quantities (80 ng). Finally, the DNA of the symbiont communities was sequenced on an Illumina MiSeq sequencer (600PE) following a 2 × 300 bp paired-end protocol at the National Instrumentation Center for Environmental Management (NICEM) of Seoul National University (Seoul, Korea). The raw Illumina amplicon data were deposited in the GenBank database under accession number PRJNA769986.
The raw reads of the functional genes were processed using Cutadapt v. 2.3 (Martin, 2011) and the ‘DADA2’ R package taking into account the DADA2 algorithm designed to detect a fine-scale variation from both 454 and Illumina data with more accuracy (Rosen et al., 2015; Callahan et al., 2016). The paired-end reads were demultiplexed according to gene region, and primer sequences were trimmed using Cutadapt v. 2.3. The DADA2 R package was used for filtering by quality, denoising, merging paired reads, and removing chimeric sequences. These procedures were conducted using the default settings except when removing chimeric sequences to prevent excessive deletion of sequences, in which case the ‘minfoldParentOverAbundance (default = 2)’ option was modified to 4. Subsequently, the sequences with <90% similarity with other reads and single reads of each locus were excluded in subsequent analyses.
Raw 454 pyrosequencing data from gill-associated symbionts of bathymodiolin mussels at seven vent fields in the eastern Pacific Ocean were obtained from a previously published study (Ho et al., 2017). Our study analyzed four common loci (dnaK, pgi, pykF, and rpoD) used for molecular analyses of symbionts of the CIR. The sequencing data from the CIR and the eastern Pacific symbionts were obtained by two different sequencing techniques (Illumina MiSeq and 454 pyrosequencing platforms, respectively). We used the same pipeline, DADA2, for the NGS data process to minimize the potential bias caused by the usage of different denoising algorithms. The DADA2 pipeline was conducted with the appropriate settings for the pyrosequencing dataset to denoise gap errors in homopolymer sites and restrict the number of insertions that the 454 platform frequently generates (HOMOPOLYMER_GAP_PENALTY = −1, BAND_SIZE = 32). Likewise with the processing of the Illumina dataset, the sequences with <90% similarity and single reads of each locus were excluded in subsequent analyses. The pipelines for the analysis of raw sequence data from Illumina MiSeq and 454 pyrosequencing platforms are schematized in Supplementary Material S1.
Community analyses using the 16S rRNA gene
The diversity and relative abundance of each bacterial strains in the gill tissue of B. marisindicus were characterized by ribotyping of amplicons of the V3–V4 regions of the 16S rRNA gene. The raw reads were first quality-checked, after which low-quality (<Q25) reads were filtered using Trimmomatic v. 0.32 (Bolger et al., 2014). Afterward, the paired-end sequence data were merged with VSEARCH v. 2.13.4 (Rognes et al., 2016) with the default parameters. Primers were then trimmed with the alignment algorithm of Myers and Miller (1988) at a 0.8 similarity threshold. Non-specific amplicons that do not encode 16S rRNA were detected using nhmmer 4 in the HMMER software package v. 3.2.1 with hmm profiles. Unique reads were extracted, and redundant reads were clustered with the unique reads using VSEARCH. The EzBioCloud 16S rRNA database (Yoon et al., 2017) was used for taxonomic assignment followed by more precise pairwise alignment using VSEARCH. The cutoff values of sequence similarity were taken from Yarza et al. (2014). Chimeric reads were filtered based on a <97% similarity threshold via reference-based chimeric detection using the UCHIME algorithm (Edgar et al., 2011) and the non-chimeric 16S rRNA database from EzBioCloud. Finally, operational taxonomic units (OTUs) with single reads were omitted from further analysis. The pipelines for the analysis of the 16S rRNA raw sequence data are schematized in Supplementary Material S2.
Molecular analyses
Comparison of symbiont populations between the CIR and the eastern Pacific Ocean
The two datasets from the 454 and Illumina MiSeq platforms were normalized by adjusting the number of reads per population for each gene to minimize the bias caused by the large variations in read numbers, in addition to the process of the raw data using the same denoising algorithm, DADA2. The number of sequence reads of each gene from the CIR was normalized by randomly sampling among the processed NGS sequences from Illumina MiSeq and adjusting them to the average number of reads per gene for each population generated by 454 pyrosequencing from the EPR (i.e., the normalized number of sequence reads per gene for population of the CIR symbionts: dnaK, 5,213; pgi, 4,909; pykF, 6,031; rpoD, 7,260). The length of fragments for four genes was adjusted to overlapped sites after alignment using the Clustal Omega algorithm on the Geneious Prime 2022.1.1. Subsequently, we compared nucleotide diversity (π) between two datasets to evaluate the effect of different error rates on the level of genetic diversity of symbiont populations. The levels of genetic differentiation among populations were estimated using Dxy (Nei, 1987), the average number of pairwise nucleotide differences per site between symbiont populations, to avoid the effect of variation in the genetic diversity of populations (Cruickshank and Hahn, 2014). The Dxy of four loci (dnaK, pgi, pykF, and rpoD) were estimated according to the Jukes–Cantor substitution model in Arlequin v. 3.5 (Excoffier and Lischer, 2010).
Next, the correlation between genetic differentiation and geographical distances was determined via Mantel and Pearson correlation tests using the mean Dxy of the four loci according to Legendre and Fortin (2010). Geographic distances were estimated using the latitude/longitude distance calculator on the ‘National hurricane center and central pacific hurricane center’ website (https://www.nhc.noaa.gov/gccalc.shtml). For the populations in the eastern Pacific Ocean, a partial Mantel test was performed using the open-source software zt (Bonnet and Van De Peer, 2002), which reflected the geographical assignment according to the results of a previous study (Ho et al., 2017) to exclude the effect of physical barriers on gene flow across the Easter Microplates around 25°S: 11N, 9N, 7S, 11S, 17S, 23S, and 32S, 38S. Geographical separation was treated as the third matrix in which a value of zero or one was assigned depending on whether the populations belonged to the same or different clusters, respectively. The Mantel test was carried out using a custom R script for the CIR populations because the sample size was smaller than five, which is the minimum number of samples required by the zt software. The R scripts are included in the Supplementary Data uploaded on Figshare (https://doi.org/10.6084/m9.figshare.17701754.v5). Similarly, the Pearson correlation tests were carried out between populations within the EPR and PAR, respectively, and between populations of the CIR at the intra-ridge level. The correlation tests were conducted on Excel using the XLSTAT 2022.3. statistical and data analysis solution (Addinsoft, New York, USA).
Comparison of host and symbiont population on the CIR
We next examined the population genetics of the CIR samples, including host and symbiotic bacteria. As indicated in the sequencing section, the fragments of three genes (mtCOI, Col, and EF1a) for the host and seven genes (16S rRNA, dnaK, dsrB, pfk, pgi, pykF, and rpoD) for symbiotic bacteria were used. For the 16S rRNA gene, the reads assigned in the same OTU with ≥97% sequence similarity among the reads belonging to the genus Candidatus Thioglobus were used to observe genetic variation at the intraspecific level of bacteria. The reads were extracted using USEARCH v.11 (Edgar, 2010; Edgar, 2013).
For both hosts and symbionts, genetic diversity, analysis of molecular variance (AMOVA), and Dxy based on the Jukes–Cantor substitution model were determined using Arlequin v. 3.5 (Excoffier and Lischer, 2010). Median-joining networks of symbionts were generated with CYTOSCAPE v. 3.7.2 (Shannon et al., 2003) based on median-joining network tables (Bandelt et al., 1999) estimated from PopART v. 1.7 (Leigh and Bryant, 2015). To simplify the network, we excluded the private alleles found at only one location. The median-joining networks of hosts were constructed using PopART v. 1.7. The Mantel tests were conducted using the R script, and the Pearson correlation tests were performed using XLSTAT 2022. 3 (Addinsoft, New York, USA).
Neutrality test and estimation of demographic parameters using the IM model
Tajima’s D (Tajima, 1989) and Fu’s Fs (Fu, 1997) were estimated using 100 randomly sampled sequences from the dataset pooled from the same vent location regardless of the host. Because the 100 sequences per vent locality was a manageable amount of input data for running the IMa3 computer program when we attempted in this study, we tested the neutrality with those 100 sequences per gene per vent population (Figure 2B). The two neutrality tests assumed non-recombination. Thus, we performed a four-gamete test for each gene in DnaSP v. 5 (Librado and Rozas, 2009) and only the longest non-recombined DNA fragments of each locus were analyzed (dnaK, 146 bp; dsrB, 161 bp; pfk, 128 bp; pgi, 134 bp; pykF, 94 bp; rpoD, 146 bp).
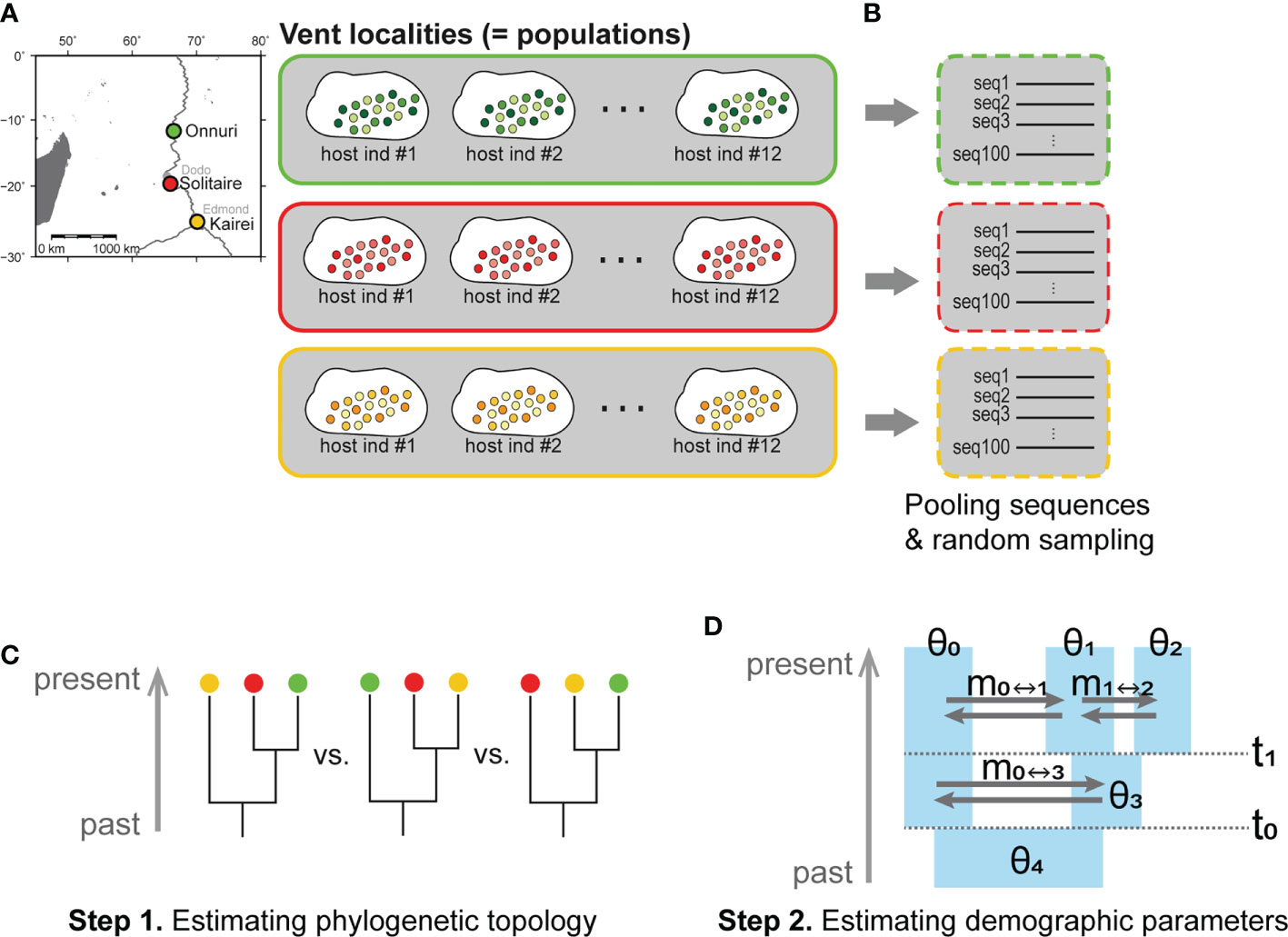
Figure 2 Hierarchical level of symbiont populations examined in this study and steps for IMa3 analyses. (A) Definition of a population in this study. The sequences from the same vent field were regarded as a population regardless of individual hosts. (B–D) Inference about demographic history based on the isolation with migration (IM) model using the IMa3 software: (B) Preparation of an input file, (C) Step 1. Estimation of phylogenetic topology, (D) Step 2. Analysis of demographic parameters scaled by mutation rate (θ, population size; m, migration rate; t, splitting time) (Hey et al., 2018).
The phylogenetic and demographic history was analyzed using the IMa3 program based on the IM model (Nielsen and Wakeley, 2001; Hey et al., 2018). The IM3 analyses were carried out for only three populations because the computational burden was rapidly increased for four-population analysis when applying our symbiont NGS data. The three vent localities, Onnuri, Solitaire, and Kairei, were chosen because they represent three adjacent but isolated ridge segments of the CIR north to south (Figure 2A). Because Edmond and Kairei vent fields are located within the same southernmost ridge segment, we chose Kairei because of its slightly higher nucleotide diversity (π) than Edmond (Supplementary Figure S1). As input data for IMa3 running, the same datasets with the neutrality test were used except for the dsrB gene (Hey and Wang, 2019). The size of the non-recombination block of dsrB for three-population IMa3 was 191 bp, slightly longer than that of the neutrality test (161 bp).
IM analyses were conducted through two steps (Figures 2C, D): 1) inference of phylogeny of three geographic populations, followed by 2) estimation of demographic parameters in step 1. We estimated the marginal posterior probability distribution of phylogenetic tree topologies for three symbiont populations from a Markov chain Montel Carlo (MCMC) simulation. The phylogeny of three symbiont populations with the maximum posterior probability was then chosen. In step 2, we conducted an MCMC simulation to estimate demographic parameters such as population sizes (θ), migration rates (m), and splitting times (t) of symbiont populations based on the estimated phylogeny from step 1. In each step, the Hasegawa–Kishino–Yano (HKY) substitution model was used for symbiont analyses. The inheritance scalar was set as 1.0 for the six symbiont-specific functional genes analyzed herein. The options for the MCMC simulations used for steps 1 and 2 are described in Supplementary Material S3. For each IMa3 analysis, several convergence diagnostics were monitored (Supplementary Material S4).
For the analysis of host mussels, every sequence of three loci obtained from Sanger sequencing (59 for mtCOI, 114 for Col-1, and 96 for Ef1a) was used. Once again, the longest non-recombined fragment of each nuclear gene was used. The IM analyses were performed following the same procedures as for the symbiotic bacteria through steps 1 and 2. The HKY substitution model was used for the symbiotic analyses using an inheritance scalar of 1.0 for two nuclear genes and 0.25 for the mtCOI gene. The details for MCMC simulations and convergence diagnostics are described in Supplementary Materials S3 and S4.
The estimated demographic parameters including population sizes, migration rates, and splitting times were converted to each corresponding demographic scale using the geometric mean of ML estimates of relevant mutation rate scalars calculated from a scalar histogram in IMa3 based on previously estimated mutation rates and generation times from previous studies. For symbionts, we used the substitution rate of four functional genes previously estimated by Ho et al. (2017) for synonymous substitutions of Bathymodiolus symbiotic bacteria on the EPR: dnaK (1.1–2.33 × 10-8 substitution/site/year), pgi (0.99–2.1 × 10-8/site/year), pykF (0.43–0.92 × 10-8 substitution/site/year), and rpoD (0.63–1.33 × 10-8 substitution/site/year). For hosts, we used the substitution rate for mtCOI (1.62 × 10-8 substitution/site/year) estimated for bathymodioline mussels based on fossil calibration (Lorion et al., 2013) and a substitution rate of 4.0 × 10-9 substitution/site/year for the two nuclear genes according to the estimate for the EF1a gene s brooding starfish belonging to the genus Leptasterias, which inhabits the North Pacific (Foltz et al., 2008). The generation time of the host was assumed as 1 year based on a histological study of bathymodioline mussels not only from the Mid-Atlantic Ridge (MAR) and the Gulf of Mexico but also from the South China sea at depths of more than 1,000 m (Colaço et al., 2006; Dixon et al., 2006; Tyler et al., 2007; Zhong et al., 2020). However, the population sizes of symbionts could not be converted to a demographic scale due to a lack of related references for the generation time of symbiotic bacteria.
Results
Composition of the bacterial community in the gill of B. marisindicus
A total of 5,776 to 26,558 gill-associated bacterial 16S rRNA sequence reads were obtained per host mussel from the CIR (Supplementary Table S3). The bacterial reads were identified as Gammaproteobacteria, most of which were classified as sulfur-oxidizing bacteria within the genus Candidatus Thioglobus (>80%). Most reads of sulfur-oxidizing bacteria had high sequence similarity (>99%). Next, a small number of reads were identified as bacteria within the genus Kistimonas of the family Endozoicomonadaceae regardless of the sampling location (Figure 3, Supplementary Table S3). Likewise, the Candidatus Endonucleobacter within the same family Endozoicomonadacea was detected broadly in the Onnuri, Solitaire, and Kairei fields despite the considerably low abundance (<1.28% of reads). Most of the reads were identified as Candidatus Endonucleobacter bathymodiolin based on sequence similarity >97%.
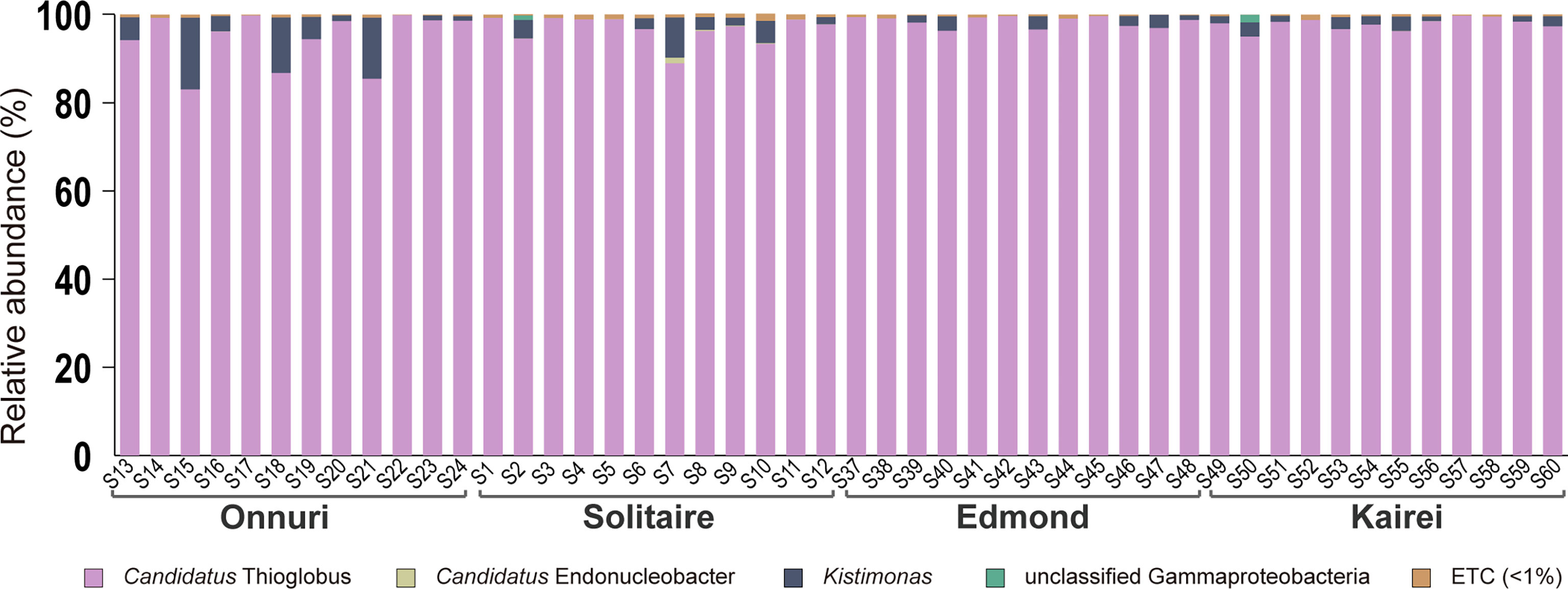
Figure 3 Community analyses based on the 16S rRNA gene of symbiotic bacteria in the gills of Bathymodiolus marisindicus. The reads are assigned at the genus level. The taxa with lower abundance <1% commonly in all host mussels were classified into ETC.
Genetic variation of symbiotic bacteria in the CIR and the eastern Pacific Ocean
The number of reads between Illumina and 454 pyrosequencing platforms was considerably different (mean number of reads per gen: Illumina MiSeq data = 94,946–144,842, 454 pyrosequencing = 4,939–7,260; Table 2, Supplementary Table S4). Before the further examination of the genetic divergence of symbiotic bacteria, the nucleotide diversity (π) of each vent locality was measured and compared to each other to check if the different platforms have led to a possible systematic underestimate or overestimate of the population genetic index of Dxy. The nucleotide diversities were considerably low in the vents of the eastern Pacific ridges, of which symbiont sequences were generated by 454 pyrosequencing, compared to those from the vents of the CIR of which sequences were generated by Illumina MiSeq (mean nucleotide diversity: eastern Pacific vents = 0.0015–0.0051, central Indian vents = 0.0031–0.0153; Supplementary Figure S1). However, two vent fields, Edmond and Kairei, from the southernmost ridge segment of the CIR were similarly low in nucleotide diversity and equivalent to those of the eastern Pacific vents. In contrast, the symbiont populations in Onnuri and Solitaire vent fields exhibited higher nucleotide diversity than others. The low nucleotide diversity in the eastern Pacific by the 454 pyrosequencing was contrary to the expectation of higher sequencing error rates of this platform. Therefore, the possible introduction of systematic overestimation or underestimation into the present study due to the difference in sequencing platforms was unlikely.
The symbiont populations exhibited a distinct geographical differentiation pattern between the two different ocean basins (Figure 4). The comparison between the CIR and the eastern Pacific Ocean showed the highest range of genetic differentiation Dxy (mean = 0.271–0.278; Figure 4; Supplementary Table S5) followed by the estimates between the East Pacific Rise (EPR) including the Galápagos Rift (GAR) and the Pacific Antarctic Ridge (PAR) symbiont populations (mean = 0.019–0.021). The Dxy values between CIR symbiont populations ranged from 0.007 to 0.017. Finally, the lowest Dxy values between symbiont populations within the same ridges (EPR including GAR, and PAR) in the eastern Pacific Ocean ranged from 0.002 to 0.004. The Mantel test indicated that the mean of genetic differentiation of symbionts in the CIR increased sharply and proportionally with the geographical distance, but this effect was not statistically significant (Mantel’s r = 0.912, P = 0.08). However, the Pearson test showed a statistically significant positive correlation between the two variables (Pearson’s r = 0.836, P = 0.011). Meanwhile, genetically homogeneous populations were observed in the same ridges in the eastern Pacific Ocean regardless of the geographic distance (partial Mantel’s r = 0.13, P = 0.268; Pearson’s r = 0.001, P = 0.871).
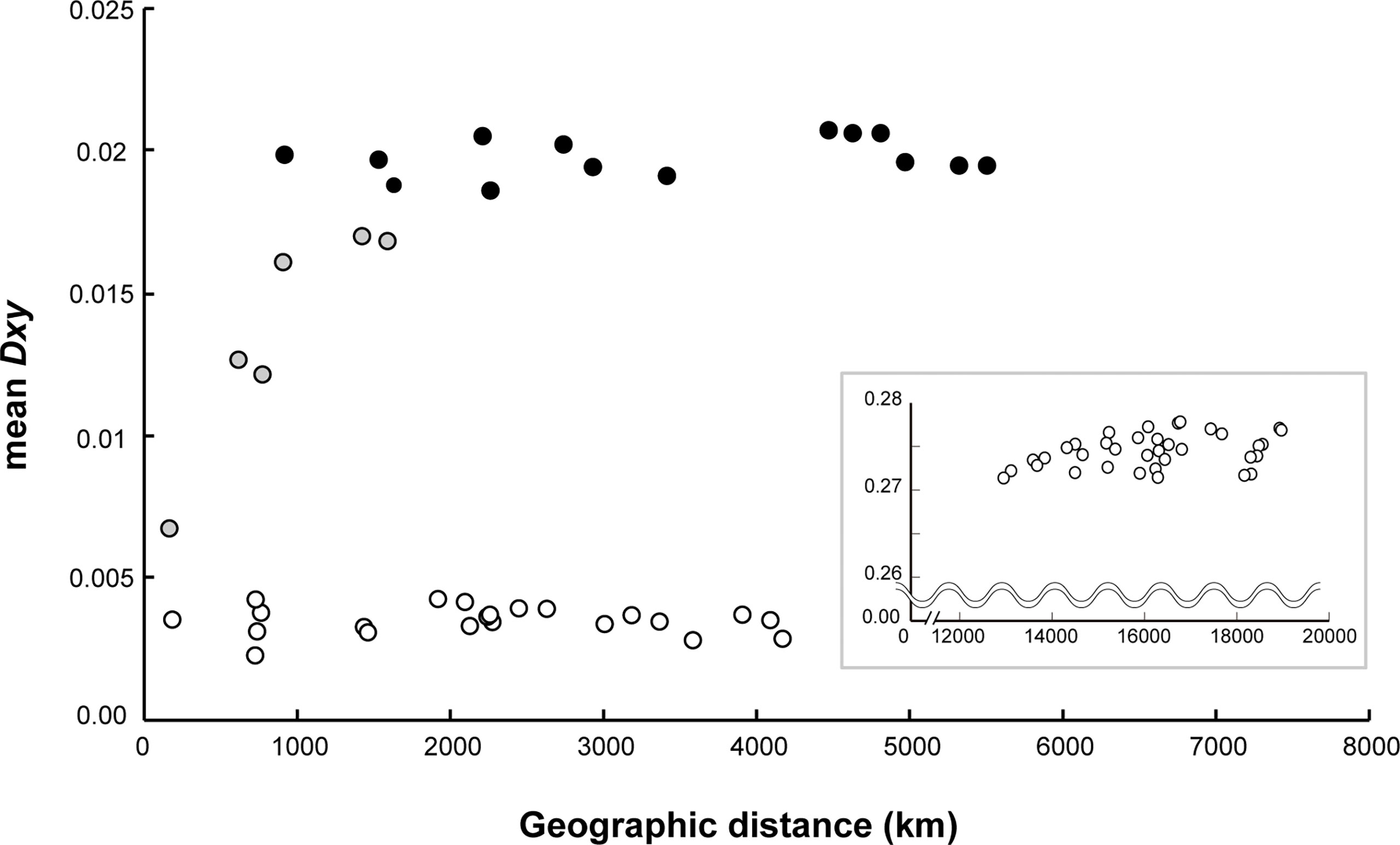
Figure 4 Correlation of average population divergence (Dxy) with geographic distance between the CIR and the eastern Pacific. The gray circles represent the comparison between vent fields within the CIR, and the white circles represent the comparison between vent fields within each region of the eastern Pacific, the EPR with the GAR, and the PAR. The black circles represent the comparison between the EPR and the PAR in the eastern Pacific. The graph in inset illustrates the comparison between the CIR and the eastern Pacific. This analysis included data collected for 30 years from 1990 at the GAR on the East Pacific Ocean to 2018 at the Onnuri vent field on the Indian Ocean.
Population genetic structure of host mussels and symbiotic bacteria on the CIR
Genetic diversity and divergence
Among all genes analyzed for symbiotic bacteria, the 16S rRNA gene of sulfur-oxidizing symbionts exhibited the lowest genetic diversity regardless of geographic location (H = 0.32–0.36, π = 0.0009–0.001; Table 2). The functional genes of symbionts showed similar genetic diversity values and patterns among all locations, except at the Edmond vent where both genetic and nucleotide diversity were relatively low (H = 0.47–0.64, π = 0.0001–0.0023; Table 2). The Onnuri and Solitaire symbionts had higher genetic diversity despite having fewer reads. A similar pattern of genetic diversity was also observed in the median-joining network (Figure 5A). For most of the six functional genes, the gene pool of the Edmond symbionts consisted of two or three dominant haplotypes with few variants from them. However, the Onnuri and Solitaire symbionts exhibited many haplotypes and different network shapes among different genes. The median-joining networks of symbionts demonstrated the genetic separation of the Onnuri population from the Edmond and Kairei populations (Figure 5A). Very few haplotypes were distributed widely among the four locations. However, the Solitaire symbionts shared haplotypes with all other three populations. The neutrality test of the four symbiont populations could not reject the null hypothesis of equilibrium population size and/or neutrality of markers about selection. After Bonferroni correction, all estimates of Tajima’s D and Fu’s Fs were statistically non-significant regardless of locations (Supplementary Table S6).
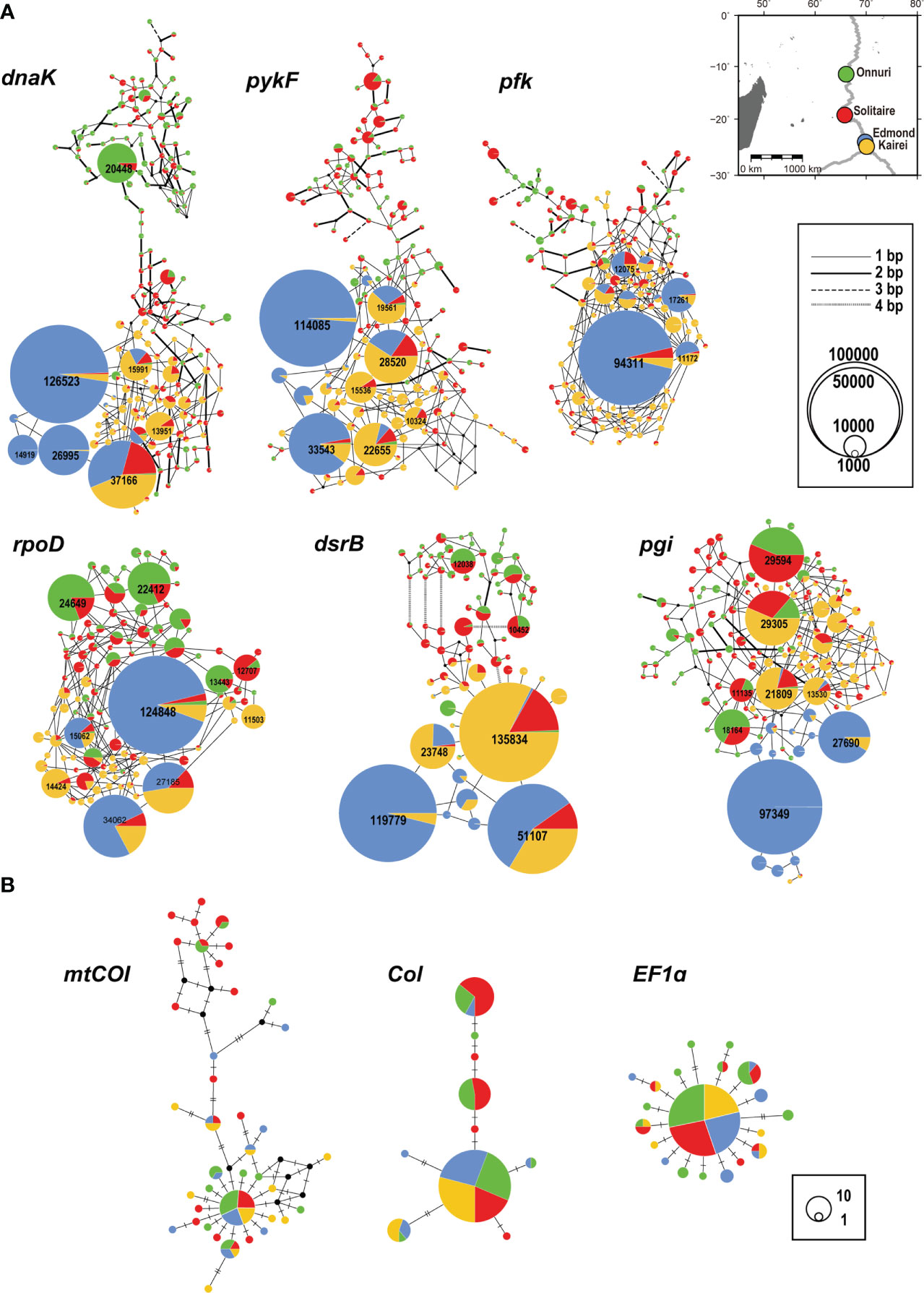
Figure 5 Median-joining networks of genes. (A) Symbiotic bacteria. (B) Host mussels. The colors indicate the geographical locality on the map in (A). The size of the circle represents the abundance of alleles. The black dot represents a hypothetical allele. (A) The network was drawn after eliminating private alleles. The number on the circle denotes the abundance of alleles >10,000. The thickness of the edges represents the number of substitutions between alleles. (B) The number of hatches denotes the number of substitutions between alleles.
The genetic variation of the host mussel populations differed depending on the used gene (Table 3). The mtCOI gene exhibited the highest genetic diversity, whereas the EF1a gene had the lowest (Table 3 and Figure 5B). Contrary to the high variation of genetic diversity among symbiont populations, the host mussels showed a similar level of genetic diversity independent of vent localities. The Onnuri population of mussels exhibited the highest genetic diversity (H = 0.63–0.96, π = 0.0021–0.0087).
The Dxy between symbiont populations demonstrated the influence of geographical distance on the genetic differentiation along the CIR (Figure 6A, Supplementary Table S7). As shown in Figure 4, which was drawn based on only four functional genes, all of the six functional genes generally exhibited an ascending pattern as the geographic distance between the vents increased. The Dxy estimates between the Edmond and Kairei vents on the same ridge segment were the lowest for most genes examined (Supplementary Table S7). The Dxy estimates between the Onnuri and the other vent fields were higher regardless of geographic distribution, except for the pgi and rpoD genes (0.030–0.032 for dnaK, 0.019–0.026 for dsrB, 0.020–0.023 for pfk, and 0.20–0.21 for pykF). The Mantel test identified a statistically significant positive correlation between each Dxy estimate for dnaK, pfk, and rpoD genes and geographical distance (Mantel’s r = 0.93, P = 0.04 for dnaK; Mantel’s r = 0.927, P = 0.04 for pfk; and Mantel’s r = 0.723, P = 0.04 for rpoD; Supplementary Table S8). In contrast, the Mantel test based on the other three genes showed no statistically significant correlation with geographical distance. The Pearson correlation test exhibited more statistically significant connections. The Dxy for four genes, dnaK, dsrB, pfk, and pykF, were correlated significantly with geographical distance (Pearson’s r = 0.864, P = 0.007 for dnaK; Pearson’s r = 0.862, P = 0.008 for dsrB; Pearson’s r = 0.862, P = 0.008 for pfk; and Pearson’s r = 0.678, P = 0.04 for pykF).
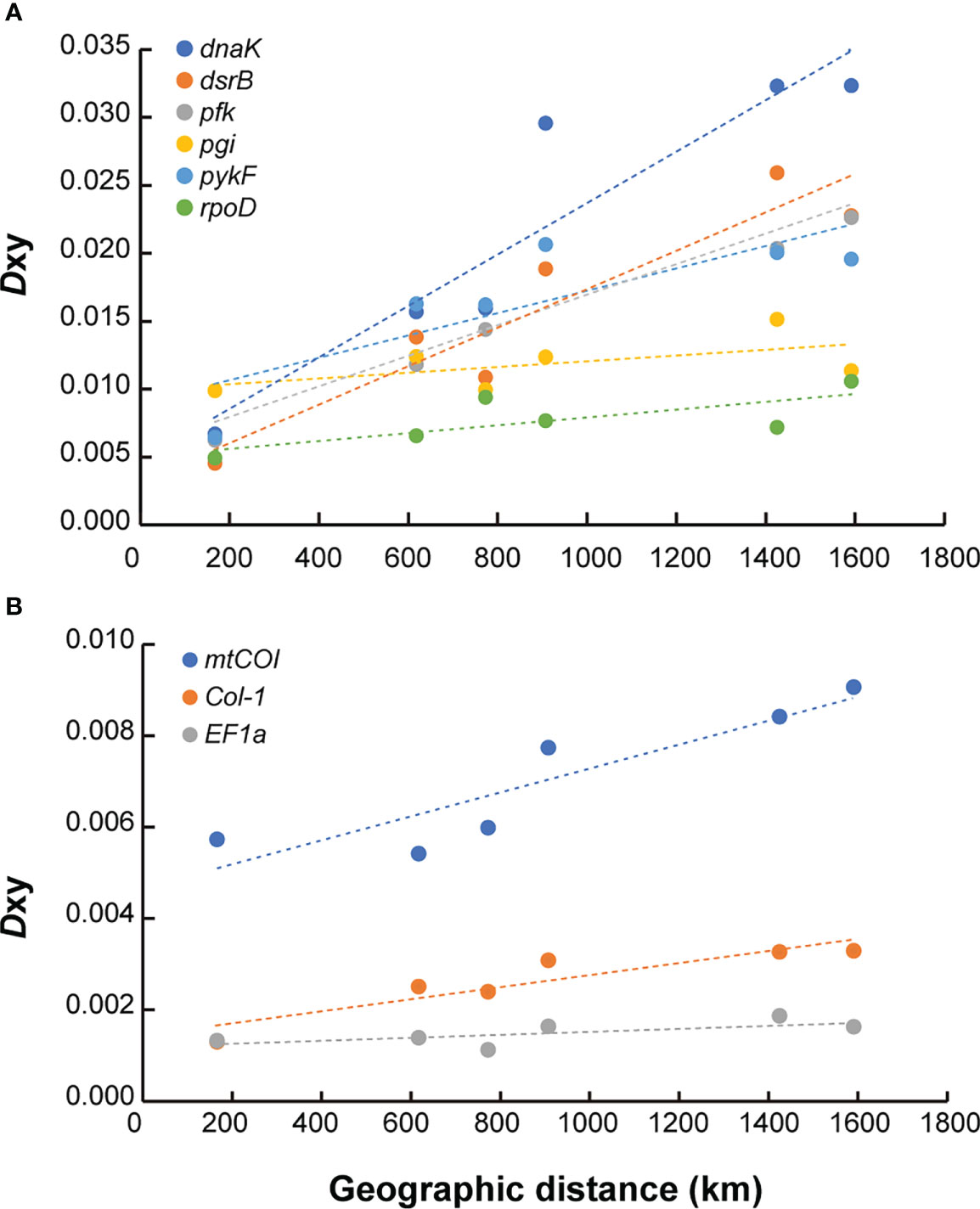
Figure 6 Correlation of population divergence (Dxy) with geographic distance for functional genes in the CIR. (A) Symbiotic bacteria. (B) Host mussels. The dashed lines represent the linear regression lines of each locus.
For the host mussels, the degree of genetic differentiation was relatively low than symbiont mussels (0.005–0.009 for mtCOI, 0.001–0.003 for Col-1, and 0.001–0.002 for EF1a). The mtCOI and Col-1 genes exhibited a consistently increasing pattern of genetic differentiation among populations with geographical distance (Figure 6B, Supplementary Table S7). According to the Mantel test, only the Dxy estimates of the mtCOI gene were significantly correlated with geographical distance (Mantel’s r = 0.905, P = 0.04), while the Pearson correlation test showed significant correlations between Dxy estimates of the Col-l gene, as well as mtCOI, with geographical distance (Pearson’s r = 0.842, P = 0.01 for Col-1; Pearson’s r = 0.818, P = 0.01 for mtCOI; Supplementary Table S8).
For symbiotic bacteria, the AMOVA results for six functional genes revealed the following hierarchical partitioning of genetic variation: among four symbiotic populations (28.64%–50.81%), among host individuals within populations (6.64%–7.88%), and among symbiont strains within individual hosts (42.56%–63.57%) (Supplementary Table S9). On the other hand, AMOVA of the 16S rRNA gene indicated that most of the genetic variations of the four populations was partitioned among symbiont strains within individuals (99.85%), then among individual hosts within populations (0.11%), and finally among the four populations (0.04%).
Phylogenies and IM model estimates of the CIR mussel hosts and symbionts
For three population analyses on IMa3, we chose one population per ridge segment of the CIR as explained in the Method section for representing each three ridge segments from north to south: Onnuri, Solitaire, and Kairei. Finally, Kairei was chosen instead of Edmond based on its slightly higher genetic diversity than Edmond hoping that Kairei represents more general features of the ridge segment than Edmond (Figure 5).
We inferred a population tree topology with the highest posterior probability of symbiotic bacteria and host mussels for the chosen three populations, Onnuri, Solitaire, and Kairei vent fields, on the CIR. The inferred phylogenetic tree topology of the host populations was similar to that of the symbiont populations (Table 4, Figure 7): the Onnuri population was identified as a phylogenetically distant group from the Solitaire and Kairei populations. However, the maximum a posteriori estimates of two splitting times among three symbiont populations slightly differed from those of the host populations (Figure 7, Supplementary Table S10 and S11). For the symbiotic bacteria, the splitting time between the Onnuri and ancestor populations and between the Solitaire and Kairei populations (t1) was estimated to be approximately 903 thousand years ago (Kya) (95% highest posterior density (HPD) interval: 702–903 Kya; Supplementary Table S12), which was an older event than the splitting of host populations (t1, host = 612 Kya, 95% HPD interval: 37–1,254 Kya; Supplementary Table S12). The splitting time between the Solitaire and Kairei populations (t0) was estimated to be also older for the symbiotic bacteria (approximately 742 Kya, 95% HPD interval: 398–893 Kya) than the host populations (approximately 75 Kya, 95% HPD interval: 13–904 Kya).
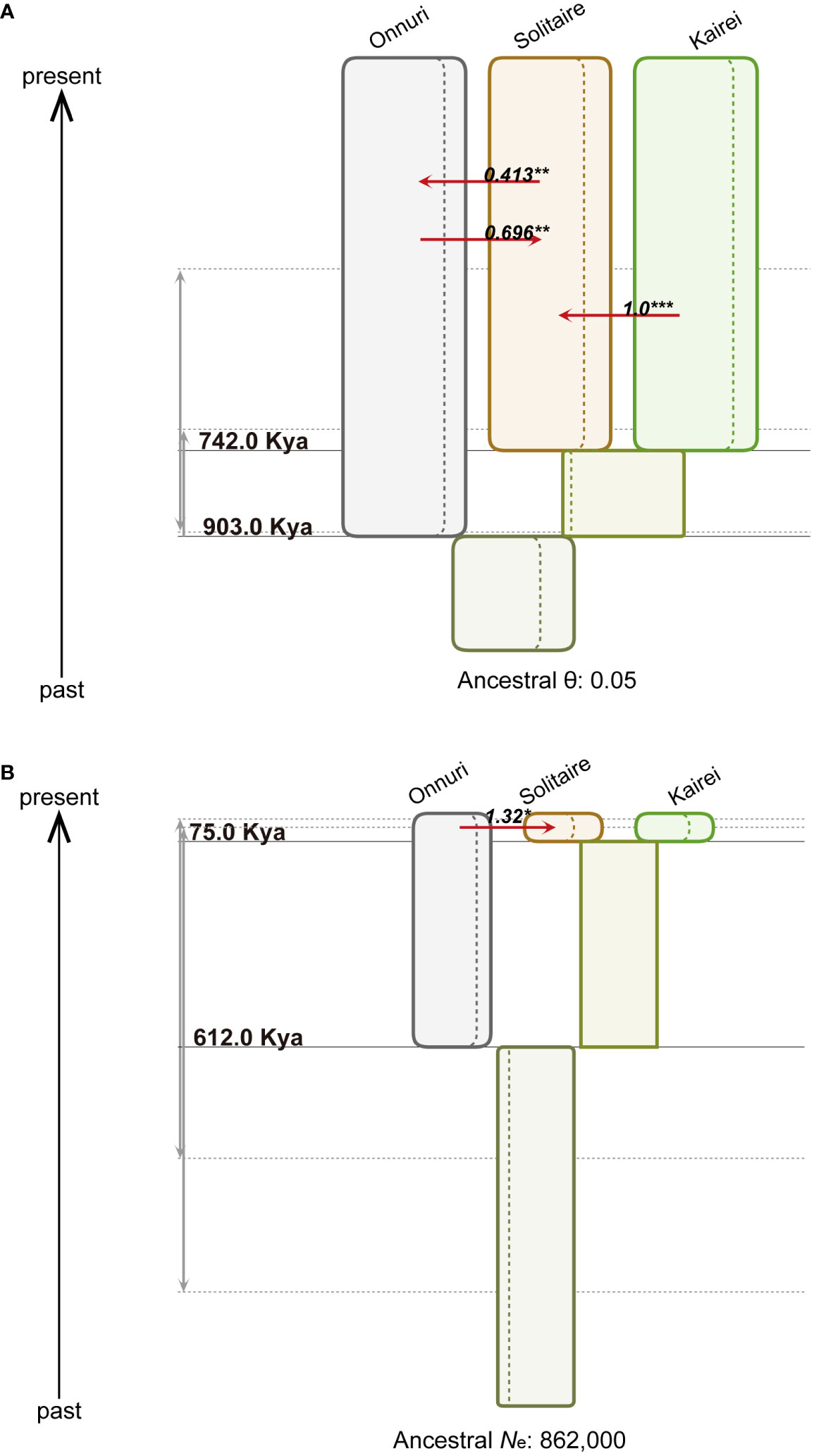
Figure 7 Demographic history of populations from three vent localities. The figures represent step 2 in Figure 2D. (A) Symbiotic bacteria. The width of the colored boxes is proportional to the maximum a posteriori estimates of the effective population size. The dashed lines within the boxes represent the 95% highest posterior density (HPD) intervals (Supplementary Table S10-12). The red arrow denotes the statistically significant population migration rate per generation (Nm for symbiont and 2Nm for host) using both marginal posterior distribution (* α = 0.05, ** α = 0.01, *** α = 0.001). The gray arrows represent 95% HPD intervals of each splitting time. For the symbiotic bacteria, the effective population size was scaled by mutation rate (θ) due to the uncertainty of the generation time of mussel symbiotic bacteria. (B) Host mussels. The figure description is the same as that for (A). The effective population size of hosts (Ne) was estimated assuming a generation time of one year.
The IMa3 analysis resulted in the degree and direction of gene flow among the extant symbiont populations. The bidirectional migration rates between the Onnuri and Solitaire fields were significantly different from zero (P < 0.01) according to a likelihood ratio test (Nm = 0.413 from the Solitaire to the Onnuri fields; Nm = 0.696 from the Onnuri to the Solitaire fields; Supplementary Table S12). On the other hand, the unidirectional migration rate from the Kairei to the Solitaire field (Nm = 1.00) was significant according to the likelihood ratio test (P < 0.001; Figure 7, Supplementary Table S12). Among host populations, only unidirectional migration was statistically significant from the Onnuri to the Solitaire field (2Nm = 1.32, P < 0.05; Figure 7, Supplementary Table S12).
For symbionts, the posterior means of population mutation parameters (θ) were commonly estimated close to 0.05 except for the ancestral population between the Solitaire and Kairei populations (approximately 0.027, 95% HPD interval: 0.0034–0.05; Supplementary Table S10). The mean of θ for the Onnuri population was approximately 0.047 (95% HPD interval: 0.041–0.05), which was similar to the mean of the Solitaire population (0.0461, 95% HPD interval: 0.04–0.05) and Kairei population (approximately 0.0465, 95% HPD interval: 0.04–0.05). The mean for the ancestor population of the three populations also exhibited a similar size of approximately 0.045 (95% HPD interval: 0.036–0.05). For the hosts, the posterior means of effective population size (N) of the three populations were estimated to be similarly large (Supplementary Table S12): 809,839 (95% HPD interval: 711,227–862,185) for the Onnuri population; 746,077 (95% HPD interval: 554,231–862,185) for the Solitaire population; and 770,342 (95% HPD interval: 605,125–862,185) for the Kairei population. The estimates of the ancestral populations were smaller, at approximately 505,692 (95% HPD interval: 0–862,185) for the ancestor between the Solitaire and Kairei populations and approximately 540,741 (95% HPD interval: 128,099–862,185) for the ancestor of the three populations.
Discussion
Geographical provincialism of deep-sea hydrothermal vent invertebrate species along the mid-ocean ridge systems is a common biological feature that we have learned since the first discovery of vent and its fauna in 1977 (Corliss et al., 1979; Bachraty et al., 2009; Rogers et al., 2012). Although the past oceanic exploration and comparative biological studies have revealed important regional geophysical and biological factors that lead to the isolation to various extents (Vrijenhoek, 2010), there is a significant knowledge gap about microorganisms in the deep sea. On the other hand, as our knowledge about the abiotic and biotic factors grows, the question of a more quantitative aspect of how long and to what extent specific abiotic barriers affect the connectivity of vent species becomes more interesting. Keeping this context in mind, we attempted to understand the diverging population processes of deep-sea hydrothermal vent animals and their symbiotic bacteria in time and space. To quantify population subdivisions in high resolution, we applied population genetic model-based approaches and comparisons of population DNA sequence data of vent mussels and their symbiotic bacteria from two ocean basins, the Central Indian Ocean and the eastern Pacific Ocean.
Gill-associated bacteria of Bathymodiolus marisindicus
To the best of our knowledge, our study is the first to evaluate the quantitative composition of gill-associated symbiotic bacteria of B. marisindicus. Our findings revealed that the mussels harbor symbiotic communities in which sulfur-oxidizing bacteria dominate (minimum >80%) regardless of the geographical distribution along the CIR, which is consistent with previous findings (Won et al., 2008; Fontanez and Cavanaugh, 2014). On the other hand, from the bacterial community of the gill tissue, we unexpectedly identified several strains belonging to the genus Kistimonas of the family Endozoicomonadaceae, typically found in shallow marine invertebrates such as starfish, dead clams, and sandworms (Choi et al., 2010; Lee et al., 2012; Ellis et al., 2019). The genus Kistimonas was recently observed in the gills of the deep-sea mussel Gigantidas haimaensis (Xu et al., 2019). Recently, other bacterial taxa of the family Endozoicomonadaceae have been identified from various marine mollusks globally (Cano et al., 2020). It suggests that Kistimonas sp. is also widely distributed in association with marine invertebrate animals at different ocean depths. Meanwhile, the Candidatus Endonucleobacter bathymodioli of the Endozoicomonadaceae is known as parasitic bacteria in nuclei of Bathymodioline mussels (Zielinski et al., 2009). Before our research, the parasites were found in bathymodioline mussels in the Gulf of Mexico, Atlantic, and eastern Pacific oceans (Zielinski et al., 2009). Interestingly, the parasites in that study were not observed in the symbiont-infected cell. Therefore, it is considered that the sulfur-oxidizing bacteria of gill tissue seem to protect the host mussel. This interpretation is supported by the antibacterial activity of mussel gill homogenates and the presence of related genes from gill tissue (Bettencourt et al., 2007). The very low abundance of the Candidatus Endonucleobacter bathymodioli compared to the sulfur-oxidizing bacteria is consistent with the expectation according to the hypothesis. Still, the protection mechanism is not known yet.
Nucleotide diversity of symbiont populations measured by the 454 and Illumina platforms
The fact that the two NGS DNA data sets of eastern Pacific and CIR were sequenced by the 454 and Illumina platforms, respectively, might cause a concern of systematic errors in the estimation of Dxy between vent localities due to the difference in platforms. Indeed, the two sequencing platforms used in the present study have different sequencing error rates. The Illumina sequencing generates fewer sequencing errors than the 454 platform (Loman et al., 2012). Despite the substantial differences in read length and sequencing process of the two platforms, however, the 454 and Illumina sequencing platforms showed reliable quantitative results about the abundances of genes and genotypes (Luo et al., 2012). The algorithm, DADA2, used for sequencing error correction in this study, was proven for its high performance in identifying more real variants and fewer false-positive sequence variants than other methods (Callahan et al., 2016). The result of nucleotide diversity (π) of symbionts in each vent locality indirectly dispels the concern (Supplementary Figure S1). The nucleotide diversities of the eastern Pacific symbiont populations assessed by the 454 platform were overall lower than those of the CIR that were by the Illumina platform, indicating that the suspected systematic errors cannot have affected our conclusion. In addition, the varying degrees of diversity estimated in the CIR mean that the Illumina platform did not seem to cause a systematic decrease or increase in the variation identification. Therefore, we conclude that the nucleotide diversity of populations and Dxy values between them reflect the actual attributes of the mussel symbionts in different mid-ocean ridges.
Influence of geological characteristics on the population divergence of symbionts
The comparison of genetic variation of vent mussel symbionts from the eastern Pacific and CIR allowed us to evaluate various factors influencing the population divergence of symbiotic bacteria. The level of genetic differentiation could be partitioned according to the following geographically hierarchical categories in decreasing order: 1) between oceans, the eastern Pacific, and CIR; 2) inter-ridge site between the EPR and PAR across the well-known physical barrier of Ester Microplate in the eastern Pacific; 3) intra-ridge vent fields in the CIR; and 4) intra-ridge vent fields in the EPR and PAR. These categories involve various geographical, geological, and hydrological features of mid-ocean ridges, such as distances between habitats, depths, ridge-axis offsets, transform faults, ridge spreading rates, microplates, and deep-oceanic currents, and the interaction of these factors.
First, the mussel symbiotic bacteria from the eastern Pacific and the CIR evolved independently for a long time. A phylogenetic tree based on 16S rRNA gene sequences showed that the two symbionts markedly diverged (Won et al., 2008). Accordingly, the Dxy estimates between the oceans are at least 10 times larger than the other subordinate comparisons. Secondly, at the inter-ridge scale of the eastern Pacific, the genetic differentiation was high. The Easter Microplate located at the boundary of the EPR and PAR was observed to impede the dispersal of diverse vent invertebrates and gave rise to a genetic isolation of several vent species (Guinot and Hurtado, 2003; Mateos et al., 2012; Johnson et al., 2013; Jang et al., 2016). The congruent vicariance among different taxonomic groups can be interpreted as a result of the orogeny of the Easter Microplate, 2.5–5.3 million years ago around the boundary of EPR and the PAR (Naar and Hey, 1991). Ho et al. (2017) reported that the EPR and PAR mussel symbionts exhibited complete geographical isolation independent of the hybridization of host species, B. thermophilus and B. antarcticus. For the first time, these previous studies (Johnson et al., 2013; Ho et al., 2017) indicated that the geomorphological process had driven the population divergence of both host animals and symbiotic bacteria in the eastern Pacific ridges.
Next, the symbionts of intra-ridge on the CIR exhibited a high degree of differentiation with geographical distance in contrast with the symbiont of intra-ridge sites on the eastern Pacific. In fragmented habitats such as deep-sea hydrothermal vents, the geographical distribution of habitats and life history features of species related to dispersal capability could be the main factors that affect genetic connectivity. This notion suggests that the different geographical distributions of vent habitats must have led to the contrasting genetic differentiation patterns between the eastern Pacific and CIR if we accept that the two symbionts may have similar mobility because they belong to the same taxa, Candidatus Thioglobus.
The seafloor spreading rates of ridges are relevant to the geomorphological features, including the distribution pattern of vent habitats along the ridge axes. Contrary to the relatively linear topology of the fast-spreading ridge, disconnection of adjacent ridges by lateral offset frequently occurs, and an extensive depth range often occurs along the slow-spreading ridge. Therefore, the geomorphological feature in the slow-spreading ridge tends to decrease the genetic connectivity of vent organisms and leads to population subdivision and even speciation (Van Dover et al., 2002; Vrijenhoek, 2010). For example, the Blanco Transform Fault (TF) in the Juan de Fuca Ridge, with approximately 450 km offset in the northeast Pacific, contributed to the genetic separation of the vent tubeworm, Ridgeia piscesae (Young et al., 2008), and the speciation of vent limpets of the genus Lepetodrilus between the northern and southern regions (Johnson et al., 2006). The bathymodioline mussels in the MAR also exhibited a substantial genetic subdivision reaching interspecies levels between the northern and southern MAR across numerous transform faults around the equator, including the largest Romanche TF with an offset of approximately 935-km width and 7-km depth (Van Der Heijden et al., 2012). Likewise, the disconnection of ridge axes by transform faults was observed in the CIR; the Argo and Marie Celeste transform faults between the Onnuri and Solitaire fields offset the ridge by about 100 and 200 km, respectively, and up to 5 km in depth (Pak et al., 2017). The ridge segmentation of the ridge also appears to interrupt the geographical connectivity of symbiotic bacteria along the CIR, especially between the Onnuri and the other fields. The details of population structure of the CIR organisms will be discussed with the genetic variation of host mussels below.
At the intra-ridge scale, the relatively distant vent habitats along the slow-spreading ridge seem to decrease the connectivity of vent organisms. Geological modeling based on the global survey dataset for the past 35 years estimated the frequency of vent field in the fast-spreading EPR up to three- or fourfold higher than the slow-spreading CIR (vent frequency per 100 km = 0.76–1.33 for CIR and 1.79–3.91 for EPR) (Beaulieu et al., 2015). The geological model and the present results predict that isolation by distance (IBD) becomes apparent in a slow-spreading ridge along with ridge-axis offsets by transform faults. For instance, the genetic variation of symbiotic bacteria observed in the slow-spreading MAR supports this hypothesis. The composition of symbiont assemblages of bathymodioline mussel, I. modiolaeformis, exhibited a statistically significant correlation with geographic distance (Laming et al., 2015).
A recent study has proved that about 13% of total genetic variation on symbionts of bathymodioline mussels distributed along the northern MAR is determined by geographical distance alone (Ücker et al., 2021). If the factor of geographic distance in that study was combined with other factors of environment and host species, the accountability for the total genetic variation increased by about 45%. Therefore, all findings above indicate that we should consider various factors, including the interaction with the host and natural selection related to the chemical and physical environment, to comprehensively understand the population divergence process of symbiotic bacteria.
Population divergence processes of mussel hosts and their symbionts in the CIR
On a regional scale, the present study examined the genetic diversity and connectivity of the host mussels, B. marisindicus, and their symbiotic bacteria along the CIR, including the recently discovered Onnuri field. Hosts’ genetic diversity was similar among vent fields and significantly higher at the Onnuri. Similarly, the genetic diversity of symbionts was higher at Onnuri and Solitaire than at Edmond and Kairei. To our interests, a similar pattern of population genetic variation was detected in the symbiotic bacteria of scaly-foot snail, Chrysomallon squamiferum, between the Solitaire and Kairei vent fields (Lan et al., 2022). The Edmond mussel symbionts were genetically homogeneous much more than other populations. The genetic diversity of symbionts with the free-living stage is associated with the environmental pool (Ansorge et al., 2019). Frequent habitat turnover by tectonic activity reduces genetic diversity by recurrent bottleneck and founder effect (Wade and Mccauley, 1988; Mccauley, 1991). The loss of genetic diversity of the Edmond mussel symbiont could be attributable to recurrent extinction/recolonization events of bacterial communities or small population size in the first place due to potentially low habitability. In this regard, it is notable that there is circumstantial evidence that the mussel patches in Edmond were very scarce (personal observation during 2001 exploration, which is reported in Van Dover et al., 2001). Additionally, the difference in depths of vent habitats is also a potential factor for the connectivity between vents’ low genetic diversity of the Edmond symbionts. Table 1 shows that the depth difference between Edmond and the two nearby adjacent vents, Solitaire and Kairei, ranges from 650 to 800 m. The oceanic currents flowing at different depths may reduce the gene flow between vents with different depths, increasing geographical isolation by disruption of dispersal (Fujio and Imasato, 1991; Young et al., 2008). The neutrality tests with Tajima’s D and Fu’s Fs statistics refute any significant demographic changes in the four symbiont populations in the CIR, which indicates that the symbiont populations from every vent field including Edmond are in selectively neutral and/or in equilibrium of population size (Supplementary Table S6).
The AMOVA results indicated that symbionts were genetically heterogeneous at the intra-host level and homogeneous at the inter-host level within the same vent field regardless of the examined genes (Supplementary Table S9). Wentrup et al. (2014) suggested two hypotheses regarding the source of symbionts during the development of host mussels: 1) continuous acquisition from the environment and 2) self-infection from own older gill tissues. However, these two alternatives may not be mutually exclusive and could be mixed. Several studies supported the self-infection scenario based on the high genetic variation of symbionts at the inter-host level even within the same fields (Ho et al., 2017; Picazo et al., 2019). On the other hand, the homogeneity of symbionts at the inter-host level observed in B. marisindicus is similar to the pattern of genetic variation observed from the genomic data of the sulfur-oxidizing symbionts of Bathymodiolus mussels in the MAR (Ansorge et al., 2019). These findings suggest that symbiont populations may be intermixed among adjacent host individuals within the same vents by repeated release to and uptake from the environment.
The multi-locus genetic data of symbionts revealed a relatively high level of genetic differentiation among three populations in the Onnuri, Solitaire, and Kairei vent fields (Supplementary Table S7). In contrast, the geographically adjacent Edmond and Kairei symbionts showed relatively low differentiation. The present IM analyses revealed the population divergence order and times from a common ancestral population to the extant three populations representing the three ridge segments of the CIR. To our interest, the population tree topology of symbiotic bacteria was identical to that of the host mussels, although the time depths are different. The northernmost Onnuri mussel populations were phylogenetically distant from the Solitaire and Kairei populations. Meanwhile, the population divergence topologies of host mussel and symbiotic bacteria are consistent with the biogeographic province of vent fauna of the Indian Ocean (Zhou et al., 2022). To our interest, Zhou et al. (2022) reported a summary of faunal similarity in a dendrogram in which a subset of CIR populations reveals an identical tree topology with the present mussel hosts and symbiont populations. Remarkably, the faunal similarity was largely explainable by solely along-ridge distances between vents, about 47.5%. Another relevant finding came out from a phylogeographic study of the scaly-foot snails of the Indian Ocean (Lan et al., 2022), which showed a relatively close relationship between the Solitaire and Kairei symbiont populations compared to the distant populations in the Southwest Indian Ridge (SWIR) and the northernmost Carlsberg ridge. These two studies again emphasizes the strong effect of geographical distance on the divergence of vent invertebrate hosts and their symbiotic bacteria in the Indian Ocean ridges with ultra- to slow-spreading rates. Finally, the consistent pattern of the present study and the previous biogeographic studies altogether indicate the influence of common historical and physical constraints on the population divergence processes of vent invertebrate hosts and their symbionts in the CIR.
According to geochronological analysis, the age of the earliest measurable hydrothermal activity in the Kairei vent field was estimated to be approximately 95 Kya (Wang et al., 2012). From the IM analysis, the host populations of Kairei and Solitaire have undergone the divergence of approximately 75 Kya (95% highest posterior density (HPD) interval: 13–904 Kya). Despite the broad interval of HPD, the highest value is considerably close to the age of the first vent activity measured by isotope, which indicates that the tectonic activity in Kairei may be connected to the divergence of an ancestral mussel population of the Kairei and Solitaire populations. In the present IMa analysis, we could not resolve narrowly how earlier the Onnuri population diverged from the most recent common ancestral population than the subsequent splitting of the Solitaire and Kairei populations. Due to the broad intervals of HPD for the splitting times, the comparison of the divergence between the symbionts and host mussels is difficult. Nevertheless, the coincident pattern of geographical connectivity from the spatially and taxonomically more broad-scaled biogeographic studies suggests that the Onnuri mussel and symbiont populations have diverged substantially from the other populations for a long time.
Overall, both the symbiont and host populations are common in that genetic differentiation increases with geographical distance between habitats along the CIR. The IM analysis provided another insight into migration patterns among populations after divergence of an ancestral population to the descending two populations. We could not detect any significant gene flow between the branches of ancestral populations in both host and symbiont populations. We could only detect significant gene flow in some terminal branches, which implies a sort of secondary contact after divergence in both host and symbiont for a while. The secondary contact after isolation is often observed in deep-sea vent mussels, such as the secondary integration between B. azoricus and B. puteoserpentis in MAR (Breusing et al., 2017) and between B. thermophilus and B. antarcticus in the eastern Pacific (Johnson et al., 2013). In the IM analysis of symbionts, the northward gene flow from the southern two symbiont populations is consistent with the direction of the deep oceanic current at an approximately 2,500-m depth, which was estimated based on geostrophic circulation models (Reid, 2003). The northward migration of symbionts from Kairei to Solitaire was the highest (Nm = 1), indicating that the Kairei population provides genetic variation to Solitaire. The degree of nucleotide diversity at each CIR population agrees well with this convey of genetic variation (Supplement Figure S1), showing a higher level in the receiving populations, Onnuri and Solitaire. On the other hand, the southward gene flow from the Onnuri to Solitaire vents was also detected in the host mussel. It is difficult to interpret this gene flow from Onnuri to Solitaire due to a lack of related environmental information in this area. Considering the daily and seasonal fluctuations of deep oceanic currents, long-distance dispersal of hosts and symbionts may be possible (Murty et al., 2001). Despite the similarities in the phylogenetic tree topologies, the different migration patterns between the hosts and symbionts might be related to different life-history traits and dispersal capabilities of them. The host populations exhibited a higher level of southward gene flow from the Onnuri to Solitaire vents (2Nm >1), which was much more significant than the estimates of the symbiont populations. Bathymodiolin mussels have long-distance dispersal capability due to the long duration of their planktonic larval stage (Lutz et al., 1980; Arellano and Young, 2009; Breusing et al., 2016) and vertical dispersal (Arellano et al., 2014). Therefore, the different patterns and extent of gene flow between the symbionts and hosts indicate that they disperse among vent localities independently.
Conclusion
Our study sought to identify the connection of divergence of microbial populations and geographic features including the distance between vent localities of the ridge system. To this end, we compared orthologous genes of symbiotic bacteria of vent mussels from the central Indian Ocean and the eastern Pacific. Their ridges vary in spreading rates, and as a result, they have a difference in the frequency of active vent habitats for animals and microorganisms. Under this frame of comparison, we could identify several key factors for the divergence of mussel symbionts. First, the degree of differentiation of symbiont populations was hierarchical concerning geographical distance and geophysical barriers for dispersal. At the scale of the ocean basin, the farther geographically, the more differentiated between vent localities. The highest divergence comes first between ocean basins and second between the East Pacific Rise and Pacific Antarctic Ridge. Apart from the distance factor, a substantial geophysical structure such as the Easter Microplates exhibiting a swollen bottom of the sea floor between the EPR and PAR played a long-term barrier to symbionts between the neighboring ridges. The seafloor geological and hydrological features along the ridge axes in the eastern Pacific strongly influenced diverging population processes of both host mussels and their symbiotic bacteria. At the intra-ridge scale, however, contrasting genetic differentiation patterns were observed; the CIR with a slow-spreading rate showed a significant pattern of isolation by distance within 1,600 km, while the eastern Pacific ridges, both EPR and PAR, with superfast- or fast-spreading rates, exhibited an absence of isolation by distance even over 4,000 km. These contrasting patterns result from the denseness of vent habitats, which ultimately relates to the spreading rates of the ridges. Finally, on a more local scale, the application of the population genetic model, isolation with migration, using a large amount of sequence data of symbionts and their host mussels allowed us to unravel their diverging processes in time and space in the CIR. The similar topology of phylogenies between host mussels and symbiotic bacteria among the three representative ridge segments in the CIR also highlights the importance of geological history and habitat configuration along the ridge axis as essential factors for population divergence in both hosts and symbionts. The present study of genetic variation of mussel symbionts at diverse spatial and time scales provides insights into the geographic distribution and genetic divergence processes of microbial populations in deep-sea hydrothermal vents.
Data availability statement
The sequence data presented in the study are deposited in the GenBank repository, accession number OK509086–OK509159; OK524315–OK524584; and PRJNA769986.
Author contributions
S-JJ and Y-JW conceived and designed the study and collected samples. S-JJ conducted the laboratory experiments. S-JJ and SJ analyzed the raw NGS data using the DADA2 pipeline. S-JJ and YC participated in the examination of demographic parameters using the isolation with migration (IM) model. YC wrote the R scripts for MCMC diagnostics to test the statistical convergence of the IMa3 results. S-JJ drafted the manuscript, and Y-JW and YC made substantial improvements. All authors contributed to the article and approved the submitted version.
Funding
This research was part of a project titled ‘Understanding the deep-sea biosphere on seafloor hydrothermal vents in the Indian Ridge (No. 20170411)’ funded by the Ministry of Oceans and Fisheries, Korea. YC was supported by the National Research Foundation of Korea (NRF) grant funded by the Korean government (MSIT) (No. 2021R1C1C1011250).
Acknowledgments
We thank all the scientists and operation teams who participated in the 2017 and 2018 ISABU cruise to the CIR for their help and assistance on board. We are grateful to Dr. Robert C. Vrijenhoek and Shannon B. Johnson for their help in receiving the specimens of the Edmond and Kairei vents. Lastly, we thank the reviewers for their helpful comments.
Conflict of interest
The authors declare that the research was conducted in the absence of any commercial or financial relationships that could be construed as a potential conflict of interest.
Publisher’s note
All claims expressed in this article are solely those of the authors and do not necessarily represent those of their affiliated organizations, or those of the publisher, the editors and the reviewers. Any product that may be evaluated in this article, or claim that may be made by its manufacturer, is not guaranteed or endorsed by the publisher.
Supplementary Material
The Supplementary Material for this article can be found online at: https://www.frontiersin.org/articles/10.3389/fmars.2022.845965/full#supplementary-material. All supplementary files in this study are available online at: https://doi.org/10.6084/m9.figshare.17701754
References
Anderson R. E., Sogin M. L., Baross J. A. (2015). Biogeography and ecology of the rare and abundant microbial lineages in deep-sea hydrothermal vents. FEMS Microbiol. Ecol. 91, 1–11. doi: 10.1093/femsec/fiu016
Ansorge R., Romano S., Sayavedra L., Porras M.Ã.G., Kupczok A., Tegetmeyer H. E., et al. (2019). Functional diversity enables multiple symbiont strains to coexist in deep-sea mussels. Nat. Microbiol. 4, 2487–2497. doi: 10.1038/s41564-019-0572-9
Arellano S. M., Van Gaest A. L., Johnson S. B., Vrijenhoek R. C., Young C. M. (2014). Larvae from deep-sea methane seeps disperse in surface waters. Proc. R. Soc. B.: Biol. Sci. 281, 20133276. doi: 10.1098/rspb.2013.3276
Arellano S. M., Young C. M. (2009). Spawning, development, and the duration of larval life in a deep-sea cold-seep mussel. Biol. Bull. 216, 149–162. doi: 10.1086/BBLv216n2p149
Bachraty C., Legendre P., Desbruyeres D. (2009). Biogeographic relationships among deep-sea hydrothermal vent faunas at global scale. Deep. Sea. Res. Part I.: Oceanographic. Res. Papers. 56, 1371–1378. doi: 10.1016/j.dsr.2009.01.009
Bandelt H.-J., Forster P., Röhl A. (1999). Median-joining networks for inferring intraspecific phylogenies. Mol. Biol. Evol. 16, 37–48. doi: 10.1093/oxfordjournals.molbev.a026036
Beaulieu S. E., Baker E. T., German C. R. (2015). Where are the undiscovered hydrothermal vents on oceanic spreading ridges? Deep. Sea. Res. Part II.: Topical. Stud. Oceanography. 121, 202–212. doi: 10.1016/j.dsr2.2015.05.001
Beedessee G., Watanabe H., Ogura T., Nemoto S., Yahagi T., Nakagawa S., et al. (2013). High connectivity of animal populations in deep-sea hydrothermal vent fields in the central Indian ridge relevant to its geological setting. PloS One 8, e81570. doi: 10.1371/journal.pone.0081570
Bettencourt R., Roch P., Stefanni S., Rosa D., Colaço A., Santos R. S. (2007). Deep sea immunity: unveiling immune constituents from the hydrothermal vent mussel bathymodiolus azoricus. Mar. Environ. Res. 64, 108–127. doi: 10.1016/j.marenvres.2006.12.010
Bird P. (2003). An updated digital model of plate boundaries. Geochemistry. Geophysics. Geosystems. 4, 1027. doi: 10.1029/2001GC000252
Bolger A. M., Lohse M., Usadel B. (2014). Trimmomatic: a flexible trimmer for illumina sequence data. Bioinformatics 30, 2114–2120. doi: 10.1093/bioinformatics/btu170
Bonnet E., Van De Peer Y. (2002). Zt: A sofware tool for simple and partial mantel tests. J. Stat. software. 7, 1. doi: 10.18637/jss.v007.i10
Breusing C., Biastoch A., Drews A., Metaxas A., Jollivet D., R. R, et al. (2016). Biophysical and population genetic models predict the presence of “Phantom” stepping stones connecting mid-Atlantic ridge vent ecosystems. Curr. Biol. 26, 2257–2267. doi: 10.1016/j.cub.2016.06.062
Breusing C., Vrijenhoek R. C., Reusch T. B. (2017). Widespread introgression in deep-sea hydrothermal vent mussels. BMC evolutionary. Biol. 17, 1–10. doi: 10.1186/s12862-016-0862-2
Callahan B. J., Mcmurdie P. J., Rosen M. J., Han A. W., Johnson A. J. A., Holmes S. P. (2016). DADA2: high-resolution sample inference from illumina amplicon data. Nat. Methods 13, 581. doi: 10.1038/nmeth.3869
Campbell B. J., Engel A. S., Porter M. L., Takai K. (2006). The versatile ϵ-proteobacteria: key players in sulphidic habitats. Nat. Rev. Microbiol. 4, 458–468. doi: 10.1038/nrmicro1414
Cano I., Ryder D., Webb S. C., Jones B. J., Brosnahan C. L., Carrasco N., et al. (2020). Cosmopolitan distribution of endozoicomonas-like organisms and other intracellular microcolonies of bacteria causing infection in marine mollusks. Front. Microbiol. 11, 577481. doi: 10.3389/fmicb.2020.577481
Chen C., Copley J. T., Linse K., Rogers A. D. (2015). Low connectivity between ‘scaly-foot gastropod’(Mollusca: Peltospiridae) populations at hydrothermal vents on the southwest Indian ridge and the central Indian ridge. Organisms. Diversity Evol. 15, 663–670. doi: 10.1007/s13127-015-0224-8
Choi E. J., Kwon H. C., Sohn Y. C., Yang H. O. (2010). Kistimonas asteriae gen. nov., sp. nov., a gammaproteobacterium isolated from asterias amurensis. Int. J. systematic. evolutionary. Microbiol. 60, 938–943. doi: 10.1099/ijs.0.014282-0
Colaço A., Martins I., Laranjo M., Pires L., Leal C., Prieto C., et al. (2006). Annual spawning of the hydrothermal vent mussel, Bathymodiolus azoricus, under controlled aquarium, conditions at atmospheric pressure. J. Exp. Mar. Biol. Ecol. 333, 166–171. doi: 10.1016/j.jembe.2005.12.005
Corliss J. B., Dymond J., Gordon L. I., Edmond J. M., Von Herzen R. P., Ballard R. D., et al. (1979). Submarine thermal springs on the Galapagos rift. Science 203, 1073–1083. doi: 10.1126/science.203.4385.1073
Cruickshank T. E., Hahn M. W. (2014). Reanalysis suggests that genomic islands of speciation are due to reduced diversity, not reduced gene flow. Mol. Ecol. 23, 3133–3157. doi: 10.1111/mec.12796
De Wit R., Bouvier T. (2006). ‘Everything is everywhere, but, the environment selects’; what did baas becking and beijerinck really say? Environ. Microbiol. 8, 755–758. doi: 10.1111/j.1462-2920.2006.01017.x
Dick G. J. (2019). The microbiomes of deep-sea hydrothermal vents: distributed globally, shaped locally. Nat. Rev. Microbiol. 17, 271–283. doi: 10.1038/s41579-019-0160-2
Dixon D. R., Lowe D. M., Miller P. I., Villemin G. R., Colaço A., Serrão-Santos R., et al. (2006). Evidence of seasonal reproduction in the Atlantic vent mussel Bathymodiolus azoricus, and an apparent link with the timing of photosynthetic primary production. J. Mar. Biol. Assoc. United. Kingdom. 86, 1363–1371. doi: 10.1017/S0025315406014391
Edgar R. C. (2010). Search and clustering orders of magnitude faster than BLAST. Bioinformatics 26, 2460–2461. doi: 10.1093/bioinformatics/btq461
Edgar R. C. (2013). UPARSE: highly accurate OTU sequences from microbial amplicon reads. Nat. Methods 10, 996–998. doi: 10.1038/nmeth.2604
Edgar R. C., Haas B. J., Clemente J. C., Quince C., Knight R. (2011). UCHIME improves sensitivity and speed of chimera detection. Bioinformatics 27, 2194–2200. doi: 10.1093/bioinformatics/btr381
Edwards D. (2012) PCR purification: AMPure and simple. Available at: http://www.keatslab.org/blog/pcrpurificationampureandsimple.
Ellis J. C., Thomas M. S., Lawson P. A., Patel N. B., Faircloth W., Hayes S. E., et al. (2019). Kistimonas alittae sp. nov., a gammaproteobacterium isolated from the marine annelid alitta succinea. Int. J. systematic. evolutionary. Microbiol. 69, 235–240. doi: 10.1099/ijsem.0.003137
Excoffier L., Lischer H. E. L. (2010). Arlequin suite ver 3.5: a new series of programs to perform population genetics analyses under Linux and windows. Mol. Ecol. Resour. 10, 564–567. doi: 10.1111/j.1755-0998.2010.02847.x
Finlay B. J., Clarke K. J. (1999). Ubiquitous dispersal of microbial species. Nature 400, 828–828. doi: 10.1038/23616
Flores G. E., Campbell J. H., Kirshtein J. D., Meneghin J., Podar M., Steinberg J. I., et al. (2011). Microbial community structure of hydrothermal deposits from geochemically different vent fields along the mid-Atlantic ridge. Environ. Microbiol. 13, 2158–2171. doi: 10.1111/j.1462-2920.2011.02463.x
Foltz D., Nguyen A., Kiger J., Mah C. L. (2008). Pleistocene speciation of sister taxa in a north pacific clade of brooding sea stars (Leptasterias). Mar. Biol. 154, 593–602. doi: 10.1007/s00227-008-0952-9
Fontanez K. M., Cavanaugh C. M. (2014). Evidence for horizontal transmission from multilocus phylogeny of deep-sea mussel (Mytilidae) symbionts. Environ. Microbiol. 16, 3608–3621. doi: 10.1111/1462-2920.12379
Franke M., Geier B., Hammel J. U., Dubilier N., Leisch N. (2021). Coming together–symbiont acquisition and early development in deep-sea bathymodioline mussels. Proc. R. Soc. B. 288, 20211044. doi: 10.1098/rspb.2021.1044
Fu Y.-X. (1997). Statistical tests of neutrality of mutations against population growth, hitchhiking and background selection. Genetics 147, 915–925. doi: 10.1093/genetics/147.2.915
Fujio S., Imasato N. (1991). Diagnostic calculation for circulation and water mass movement in the deep pacific. J. Geophysical. Research.: Oceans. 96, 759–774. doi: 10.1029/90JC02130
Guinot D., Hurtado L. A. (2003). Two new species of hydrothermal vent crabs of the genus Bythograea from the southern East pacific rise and from the Galapagos rift (Crustacea decapoda brachyura bythograeidae). Comptes. Rendus. Biologies. 326, 423–439. doi: 10.1016/S1631-0691(03)00126-4
Hanson C. A., Fuhrman J. A., Horner-Devine M. C., Martiny J. B. (2012). Beyond biogeographic patterns: processes shaping the microbial landscape. Nat. Rev. Microbiol. 10, 497–506. doi: 10.1038/nrmicro2795
Hey J., Chung Y., Sethuraman A., Lachance J., Tishkoff S., Sousa V. C., et al. (2018). Phylogeny estimation by integration over isolation with migration models. Mol. Biol. Evol. 35, 2805–2818. doi: 10.1093/molbev/msy162
Hey J., Wang K. (2019). The effect of undetected recombination on genealogy sampling and inference under an isolation-with-migration model. Mol. Ecol. Resour. 19, 1593–1609. doi: 10.1111/1755-0998.13083
Ho P.-T., Park E., Hong S. G., Kim E.-H., Kim K., Jang S.-J., et al. (2017). Geographical structure of endosymbiotic bacteria hosted by Bathymodiolus mussels at eastern pacific hydrothermal vents. BMC evolutionary. Biol. 17, 121. doi: 10.1186/s12862-017-0966-3
Huber J. A., Cantin H. V., Huse S. M., Mark Welch D. B., Sogin M. L., Butterfield D. A. (2010). Isolated communities of epsilonproteobacteria in hydrothermal vent fluids of the Mariana arc seamounts. FEMS Microbiol. Ecol. 73, 538–549. doi: 10.1111/j.1574-6941.2010.00910.x
Ikuta T., Amari Y., Tame A., Takaki Y., Tsuda M., Iizuka R., et al. (2021). Inside or out? clonal thiotrophic symbiont populations occupy deep-sea mussel bacteriocytes with pathways connecting to the external environment. ISME. Commun. 1, 1–4. doi: 10.1038/s43705-021-00043-x
Jang S.-J., Park E., Lee W.-K., Johnson S. B., Vrijenhoek R. C., Won Y.-J. (2016). Population subdivision of hydrothermal vent polychaete Alvinella pompejana across equatorial and Easter microplate boundaries. BMC evolutionary. Biol. 16, 1–15. doi: 10.1186/s12862-016-0807-9
Johnson S. B., Yong C. R., Jones W. J., Warén A., Vrijenhoek R. C. (2006). Migration, isolation, and speciation of hydrothermal vent limpets (Gastropoda; Lepetodrilidae) across the Blanco Transform Fault. Biol. Bull 210, 140–157. doi: 10.2307/4134603
Johnson S. B., Won Y.-J., Harvey J. B., Vrijenhoek R. C. (2013). A hybrid zone between Bathymodiolus mussel lineages from eastern pacific hydrothermal vents. BMC evolutionary. Biol. 13, 1–18. doi: 10.1186/1471-2148-13-21
Kim J., Son S. K., Kim D., Pak S. J., Yu O. H., Walker S. L., et al. (2020). Discovery of active hydrothermal vent fields along the central Indian ridge, 8–12°S. Geochemistry. Geophysics. Geosystems. 21, e2020GC009058. doi: 10.1029/2020GC009058
Laming S. R., Szafranski K. M., Rodrigues C. F., Gaudron S. M., Cunha M. R., Hilário A., et al. (2015). Fickle or faithful: the roles of host and environmental context in determining symbiont composition in two bathymodioline mussels. PloS One 10, e0144307. doi: 10.1371/journal.pone.0144307
Lan Y., Sun J., Chen C., Wang H., Xiao Y., Perez M., et al. (2022). Endosymbiont population genomics sheds light on transmission mode, partner specificity, and stability of the scaly-foot snail holobiont. ISME. J., 16, 2132-2143. doi: 10.1038/s41396-022-01261-4
Lee J., Shin N.-R., Lee H.-W., Roh S. W., Kim M.-S., Kim Y.-O., et al. (2012). Kistimonas scapharcae sp. nov., isolated from a dead ark clam (Scapharca broughtonii), and emended description of the genus Kistimonas. Int. J. systematic. evolutionary. Microbiol. 62, 2865–2869. doi: 10.1099/ijs.0.038422-0
Legendre P., Fortin M. J. (2010). Comparison of the mantel test and alternative approaches for detecting complex multivariate relationships in the spatial analysis of genetic data. Mol. Ecol. Resour. 10, 831–844. doi: 10.1111/j.1755-0998.2010.02866.x
Leigh J. W., Bryant D. (2015). POPART: full-feature software for haplotype network construction. Methods Ecol. Evol. 6, 1110–1116. doi: 10.1111/2041-210X.12410
Librado P., Rozas J. (2009). DnaSP v5: a software for comprehensive analysis of DNA polymorphism data. Bioinformatics 25, 1451–1452. doi: 10.1093/bioinformatics/btp187
Loman N. J., Misra R. V., Dallman T. J., Constantinidou C., Gharbia S. E., Wain J., et al. (2012). Performance comparison of benchtop high-throughput sequencing platforms. Nat. Biotechnol. 30, 434–439. doi: 10.1038/nbt.2198
Lorion J., Kiel S., Faure B., Kawato M., Ho S. Y., Marshall B., et al. (2013). Adaptive radiation of chemosymbiotic deep-sea mussels. Proc. R. Soc. B.: Biol. Sci. 280, 20131243. doi: 10.1098/rspb.2013.1243
Louca S. (2021). The rates of global bacterial and archaeal dispersal. ISME. J., 16, 159-167. doi: 10.1038/s41396-021-01069-8
Luo C., Tsementzi D., Kyrpides N., Read T., Konstantinidis K. T. (2012). Direct comparisons of illumina vs. Roche 454 sequencing technologies on the same microbial community DNA sample. PloS One 7, e30087. doi: 10.1371/journal.pone.0030087
Lutz R., Jablonski D., Rhoads D., Turner R. (1980). Larval dispersal of a deep-sea hydrothermal vent bivalve from the Galapagos rift. Mar. Biol. 57, 127–133. doi: 10.1007/BF00387378
Martin M. (2011). Cutadapt removes adapter sequences from high-throughput sequencing reads. EMBnet. J. 17, 10–12. doi: 10.14806/ej.17.1.200
Mateos M., Hurtado L. A., Santamaria C. A., Leignel V., Guinot D. (2012). Molecular systematics of the deep-sea hydrothermal vent endemic brachyuran family bythograeidae: a comparison of three Bayesian species tree methods. PloS One 7, e32066. doi: 10.1371/journal.pone.0032066
Mccauley D. E. (1991). Genetic consequences of local population extinction and recolonization. Trends Ecol. Evol. 6, 5–8. doi: 10.1016/0169-5347(91)90139-O
Meier D. V., Pjevac P., Bach W., Hourdez S., Girguis P. R., Vidoudez C., et al. (2017). Niche partitioning of diverse sulfur-oxidizing bacteria at hydrothermal vents. ISME. J. 11, 1545. doi: 10.1038/ismej.2017.37
Mullineaux L. S., Metaxas A., Beaulieu S. E., Bright M., Gollner S., Grupe B. M., et al. (2018). Exploring the ecology of deep-sea hydrothermal vents in a metacommunity framework. Front. Mar. Sci. 5, 49. doi: 10.3389/fmars.2018.00049
Murty V., Savin M., Babu V. R., Suryanarayana A. (2001). Seasonal variability in the vertical current structure and kinetic energy in the central Indian ocean basin. Deep. Sea. Res. Part II.: Topical. Stud. Oceanography. 48, 3309–3326. doi: 10.1016/S0967-0645(01)00043-1
Myers E. W., Miller W. (1988). Optimal alignments in linear space. Bioinformatics 4, 11–17. doi: 10.1093/bioinformatics/4.1.11
Naar D. F., Hey R. (1991). Tectonic evolution of the Easter microplate. J. Geophysical. Research.: Solid. Earth 96, 7961–7993. doi: 10.1029/90JB02398
Nakamura K., Watanabe H., Miyazaki J., Takai K., Kawagucci S., Noguchi T., et al. (2012). Discovery of new hydrothermal activity and chemosynthetic fauna on the central Indian ridge at 18–20 s. PloS One 7, e32965. doi: 10.1371/journal.pone.0032965
Nielsen R., Wakeley J. (2001). Distinguishing migration from isolation: a Markov chain Monte Carlo approach. Genetics 158, 885–896. doi: 10.1093/genetics/158.2.885
Pak S.-J., Moon J.-W., Kim J., Chandler M. T., Kim H.-S., Son J., et al. (2017). Widespread tectonic extension at the central Indian ridge between 8°S and 18°S. Gondwana. Res. 45, 163–179. doi: 10.1016/j.gr.2016.12.015
Papke R. T., Ramsing N. B., Bateson M. M., Ward D. M. (2003). Geographical isolation in hot spring cyanobacteria. Environ. Microbiol. 5, 650–659. doi: 10.1046/j.1462-2920.2003.00460.x
Picazo D. R., Dagan T., Ansorge R., Petersen J. M., Dubilier N., Kupczok A. (2019). Horizontally transmitted symbiont populations in deep-sea mussels are genetically isolated. ISME. J. 13, 2954–2968. doi: 10.1038/s41396-019-0475-z
Reid J. L. (2003). On the total geostrophic circulation of the Indian ocean: Flow patterns, tracers, and transports. Prog. Oceanography. 56, 137–186. doi: 10.1016/S0079-6611(02)00141-6
Reveillaud J., Reddington E., Mcdermott J., Algar C., Meyer J. L., Sylva S., et al. (2016). Subseafloor microbial communities in hydrogen-rich vent fluids from hydrothermal systems along the mid-Cayman rise. Environ. Microbiol. 18, 1970–1987. doi: 10.1111/1462-2920.13173
Rogers A. D., Tyler P. A., Connelly D. P., Copley J. T., James R., Larter R. D., et al. (2012). The discovery of new deep-sea hydrothermal vent communities in the southern ocean and implications for biogeography. PloS Biol. 10, e1001234. doi: 10.1371/journal.pbio.1001234
Rognes T., Flouri T., Nichols B., Quince C., Mahe F. (2016). VSEARCH: a versatile open source tool for metagenomics. PeerJ 4, e2584. doi: 10.7717/peerj.2584
Rosen M. J., Davison M., Bhaya D., Fisher D. S. (2015). Fine-scale diversity and extensive recombination in a quasisexual bacterial population occupying a broad niche. Science 348, 1019–1023. doi: 10.1126/science.aaa4456
Russell S. L., Pepper-Tunick E., Svedberg J., Byrne A., Ruelas Castillo J., Vollmers C., et al. (2020). Horizontal transmission and recombination maintain forever young bacterial symbiont genomes. PloS Genet. 16, e1008935. doi: 10.1371/journal.pgen.1008935
Shannon P., Markiel A., Ozier O., Baliga N. S., Wang J. T., Ramage D., et al. (2003). Cytoscape: a software environment for integrated models of biomolecular interaction networks. Genome Res. 13, 2498–2504. doi: 10.1101/gr.1239303
Sun J., Zhou Y., Chen C., Kwan Y. H., Sun Y., Wang X., et al. (2020). Nearest vent, dearest friend: biodiversity of tiancheng vent field reveals cross-ridge similarities in the Indian ocean. R. Soc. Open Sci. 7, 200110. doi: 10.1098/rsos.200110
Tajima F. (1989). Statistical method for testing the neutral mutation hypothesis by DNA polymorphism. Genetics 123, 585–595. doi: 10.1093/genetics/123.3.585
Tyler P., Young C. M., Dolan E., Arellano S. M., Brooke S. D., Baker M. (2007). Gametogenic periodicity in the chemosynthetic cold-seep mussel “Bathymodiolus” childressi. Mar. Biol. 150, 829–840. doi: 10.1007/s00227-006-0362-9
Ücker M., Ansorge R., Sato Y., Sayavedra L., Breusing C., Dubilier N. (2021). Deep-sea mussels from a hybrid zone on the mid-Atlantic ridge host genetically indistinguishable symbionts. ISME. J. 15, 3076–3083. doi: 10.1038/s41396-021-00927-9
Van Der Gast C. J. (2015). Microbial biogeography: the end of the ubiquitous dispersal hypothesis? Environ. Microbiol. 17, 544–546. doi: 10.1111/1462-2920.12635
Van Der Heijden K., Petersen J. M., Dubilier N., Borowski C. (2012). Genetic connectivity between north and south mid-Atlantic ridge chemosynthetic bivalves and their symbionts. PloS One 7, e39994. doi: 10.1371/journal.pone.0039994
Van Dover C. L., German C., Speer K. G., Parson L., Vrijenhoek R. (2002). Evolution and biogeography of deep-sea vent and seep invertebrates. Science 295, 1253–1257. doi: 10.1126/science.1067361
Van Dover C. L., Humphris S. E., Fornari D., Cavanaugh C. M., Collier R., Goffredi S. K., et al. (2001). Biogeography and ecological setting of Indian ocean hydrothermal vents. Science 294, 818–823. doi: 10.1126/science.1064574
Vrijenhoek R. C. (2010). Genetic diversity and connectivity of deep-sea hydrothermal vent metapopulations. Mol. Ecol. 19, 4391–4411. doi: 10.1111/j.1365-294X.2010.04789.x
Wade M. J., Mccauley D. E. (1988). Extinction and recolonization: their effects on the genetic differentiation of local populations. Evolution 42, 995–1005. doi: 10.1111/j.1558-5646.1988.tb02518.x
Wang Y., Han X., Jin X., Qiu Z., Ma Z., Yang H. (2012). Hydrothermal activity events at kairei field, central Indian ridge 25°S. Resource. Geology. 62, 208–214. doi: 10.1111/j.1751-3928.2012.00189.x
Wentrup C., Wendeberg A., Schimak M., Borowski C., Dubilier N. (2014). Forever competent: deep‐sea bivalves are colonized by their chemosynthetic symbionts throughout their lifetime. Environ. Microbiol. 16, 3699–3713. doi: 10.1111/1462-2920.12597
Whitaker R. J., Grogan D. W., Taylor J. W. (2003). Geographic barriers isolate endemic populations of hyperthermophilic archaea. Science 301, 976–978. doi: 10.1126/science.1086909
Won Y.-J., Hallam S. J., O'mullan G. D., Pan I. L., Buck K. R., Vrijenhoek R. C. (2003). Environmental acquisition of thiotrophic endosymbionts by deep-sea mussels of the genus Bathymodiolus. Appl. Environ. Microbiol. 69, 6785–6792. doi: 10.1128/AEM.69.11.6785-6792.2003
Won Y.-J., Jones W. J., Vrijenhoek R. C. (2008). Absence of cospeciation between deep-sea mytilids and their thiotrophic endosymbionts. J. Shellfish. Res. 27, 129–138. doi: 10.2983/0730-8000(2008)27[129:AOCBDM]2.0.CO;2
Xu T., Feng D., Tao J., Qiu J.-W. (2019). A new species of deep-sea mussel (Bivalvia: Mytilidae: Gigantidas) from the south China Sea: morphology, phylogenetic position, and gill-associated microbes. Deep. Sea. Res. Part I.: Oceanographic. Res. Papers 146, 79-90. doi: 10.1016/j.dsr.2019.03.001
Yarza P., Yilmaz P., Pruesse E., Glöckner F. O., Ludwig W., Schleifer K.-H., et al. (2014). Uniting the classification of cultured and uncultured bacteria and archaea using 16S rRNA gene sequences. Nat. Rev. Microbiol. 12, 635–645. doi: 10.1038/nrmicro3330
Yoon S.-H., Ha S.-M., Kwon S., Lim J., Kim Y., Seo H., et al. (2017). Introducing EzBioCloud: a taxonomically united database of 16S rRNA gene sequences and whole-genome assemblies. Int. J. systematic. evolutionary. Microbiol. 67, 1613-1617. doi: 10.1099/ijsem.0.001755
Young C., Fujio S., Vrijenhoek R. (2008). Directional dispersal between mid-ocean ridges: deep-ocean circulation and gene flow in Ridgeia piscesae. Mol. Ecol. 17, 1718–1731. doi: 10.1111/j.1365-294X.2008.03609.x
Zhong Z., Wang M., Chen H., Zheng P., Li C. (2020). Gametogenesis and reproductive traits of the cold-seep mussel Gigantidas platifrons in the south China Sea. J. Oceanology. Limnology. 38, 1304–1318. doi: 10.1007/s00343-020-0027-4
Zhou Y., Chen C., Zhang D., Wang Y., Watanabe H. K., Sun J., et al. (2022). Delineating biogeographic regions in Indian ocean deep-sea vents and implications for conservation. Diversity Distributions. doi: 10.1111/ddi.13535
Keywords: symbiotic bacteria, Bathymodioline mussel, population divergence, seafloor spreading rate, Central Indian Ridge, Eastern Pacific Ocean
Citation: Jang S-J, Chung Y, Jun S and Won Y-J (2022) Connectivity and divergence of symbiotic bacteria of deep-sea hydrothermal vent mussels in relation to the structure and dynamics of mid-ocean ridges. Front. Mar. Sci. 9:845965. doi: 10.3389/fmars.2022.845965
Received: 30 December 2021; Accepted: 13 September 2022;
Published: 03 October 2022.
Edited by:
Pei-Yuan Qian, Hong Kong University of Science and Technology, Hong Kong SAR, ChinaReviewed by:
Adam Michael Reitzel, University of North Carolina at Charlotte, United StatesSébastien Duperron, Muséum National d’Histoire Naturelle, France
Copyright © 2022 Jang, Chung, Jun and Won. This is an open-access article distributed under the terms of the Creative Commons Attribution License (CC BY). The use, distribution or reproduction in other forums is permitted, provided the original author(s) and the copyright owner(s) are credited and that the original publication in this journal is cited, in accordance with accepted academic practice. No use, distribution or reproduction is permitted which does not comply with these terms.
*Correspondence: Yong-Jin Won, won@ewha.ac.kr