- Department of Biological Sciences, Faculty of Science, Kuwait University, Safat, Kuwait
The marine waters of Kuwait are highly dynamic and strongly influenced by extreme environmental conditions and high levels of anthropogenic activities. This study explored the bacterioplankton community composition and diversity in Kuwait Bay and at four offshore sites close to urbanized coastal areas during two seasons, autumn (October) and spring (April). 16S rRNA amplicon sequencing detected higher bacterial diversity and richness in spring than in autumn. Also, bacterial community beta diversity revealed a significant seasonal partitioning between the two sampling periods but no clear spatial variation could be depicted. Alphaproteobacteria, Gammaproteobacteria, Cyanobacteria, Flavobacteriia, and Acidimicrobiia dominated the seasonal samples, and SARII clade Ia, SARII clade II, Synechococcus, and SUP05 cluster were among the most abundant taxa in the seasonal samples. However, the strong temporal shift in bacterial community composition was related to Synechococcus abundance. The prevailing physicochemical parameters displayed a significant influence on the bacterioplankton community composition, which was mainly driven by shifts in temperature and nutrient inputs. Furthermore, functional prediction by PICRUSt analysis revealed a relatively stable conserved functional profile in Kuwait’s waters. However, functional genes related to membrane transport were enriched in eutrophic spring waters, while photosynthetic genes were enriched in autumn. Our results provide in-depth insights into the temporal and spatial variations of bacterioplankton dynamics in Kuwait waters and highlight the strong seasonal influence of natural and anthropogenic stressors on their composition and predicted functional capabilities.
Introduction
Bacterioplankton is the keystone of the structure and function of marine ecosystems (Gilbert et al., 2009; Richa et al., 2017). Marine bacterioplankton include phototrophic cyanobacteria and chemotrophic primary producers in addition to secondary heterotrophic producers (Azam and Worden, 2004; Fuhrman et al., 2015). Marine bacterioplankton play a major role in biogeochemical processes and drive the global carbon flux and marine food web (Azam et al., 1983; Kirchman et al., 2010). Cyanobacteria are responsible for global primary productivity (Waterbury et al., 1986; Field et al., 1998; Partensky et al., 1999), while heterotrophic bacteria are important for the recycling of dissolved organic carbon and nutrients (Azam et al., 1983; Fuhrman et al., 2015).
Culture-based techniques were originally employed to determine the bacterioplankton community composition in aquatic habitats; however, owing to the limitations of these techniques, culture-independent approaches have been extensively exploited to gain a deeper understanding of marine biodiversity. High-throughput targeting of phylogenetic marker genes, such as the small subunit of the ribosomal RNA gene (16S rRNA) (Amann et al., 1995; Cottrell and Kirchman, 2000) is employed to directly explore the abundance and diversity of marine microbiomes from their natural aquatic environments. Metagenomic community 16S rRNA gene sequence analysis has expanded our knowledge of the spatial and temporal microbial abundance patterns and phylogenetic diversity present in oceanic (DeLong et al., 2006; Rusch et al., 2007; Campbell et al., 2011; Sunagawa et al., 2015), freshwater (Jones et al., 2009; Sommaruga and Casamayor, 2009; Arora-Williams et al., 2018), coastal (Ferrera et al., 2011; Bertrand et al., 2015; Teeling et al., 2016; Bryson et al., 2017; Chafee et al., 2018), and deep-sea environments (Sogin et al., 2006; Salazar et al., 2015).
Moreover, 16S rRNA gene survey studies have also been used to predict the metabolic activity and functional capabilities of bacterial communities as an alternative approach to shotgun metagenomics by mapping 16S rRNA data to a reference genome database (Langille et al., 2013). Although such an approach has many limitations, it is useful to roughly detect and correlate changes in the abundance of certain bacterial members with functional changes.
Metagenomic studies have also investigated the influence of environmental variables, such as temperature, the salinity gradient, dissolved oxygen, nutrient availability, and physical mixing, on bacterioplankton community structure (Bouvier and Del Giorgio, 2002; Fuhrman et al., 2015; Sunagawa et al., 2015). Metagenomic analysis has also revealed the global distribution patterns of marine bacterioplankton (Pommier et al., 2007), and time-series studies in various marine habitats have revealed that the composition of surface-water bacterial communities varies with recurrent temporal patterns and exhibits nonrandom spatial variation (Fuhrman et al., 2006; Sogin et al., 2006; Gilbert et al., 2009; Gilbert et al., 2012; Fuhrman et al., 2015). Taxonomic surveys have also revealed shifts in bacterioplankton communities in response to biotic and abiotic factors (Gilbert et al., 2012; Chow et al., 2013).
Kuwait’s coastal waters are located in the northernmost corner of the Arabian/Persian Gulf (hereinafter the Gulf) with a shoreline of approximately 500 km (Al-Yamani et al., 2004; Nicolaus et al., 2017). The Gulf is a unique shallow semi-enclosed hypersaline sea surrounded by arid-region countries (Sheppard et al., 2010). The temperature and salinity in the Gulf, including Kuwait’s marine waters, are extremely variable (Nasrallah et al., 2004; Al-Abdulghani et al., 2013). The seawater temperature in the gulf, including the coast of Kuwait, ranges from 10°C in winter to 36°C in summer (Al-Yamani et al., 2004; Nasrallah et al., 2004), while the salinity ranges from 36 ppt to 42 ppt (Al-Abdulghani et al., 2013; Al-Yamani et al., 2004). However, salinity usually fluctuates owing to freshwater input from the Shatt Al-Arab River at the northwest end, which creates a diluting factor along the Kuwait coast (Al-Yamani et al., 2004). Under pristine conditions, the gulf marine environment was originally oligotrophic; however, as a result of riverine nutrient inflow, the gulf waters are nutrient-rich (Al-Yamani et al., 2004). Also, anthropogenic activities have severely impacted the marine environment of the Gulf’s waters (Sheppard et al., 2010)
The subtropical waters of Kuwait are generally well-mixed, with a maximum depth of 30 m (Al-Yamani et al., 2004). However, water is subjected to several stressful factors, such as oil-based activities, industrialization, desalination plants, maricultural operations, and, more importantly, sewage discharge (Al-Ghadban et al., 2002). The general population in Kuwait is served by five main Sewage Treatment Plants (STP) and three smaller facilities (Devlin et al., 2015; Lyons et al., 2015). To date, the capacities of these plants have not been able to manage the rapid urbanization of Kuwait. Therefore, wastewater with various states of treatment is regularly discharged into the marine environment (Devlin et al., 2015; Al-Said et al., 2019) and is considered a major source of anthropogenic contamination in Kuwait’s waters, specifically in Kuwait Bay (Devlin et al., 2015). The semi-enclosed Kuwait Bay is influenced by the Shat Al-Arab River discharge and receives large amounts of contaminants from point sources of pollution near its coast. Furthermore, owing to the prevalent counterclockwise water circulation in the Gulf, Kuwait waters are contaminated with various pollutants, such as anthropogenic contaminants, trace metals, and petroleum hydrocarbons (Al-Ghadban et al., 2002; Al-Yamani et al., 2004; Sheppard et al., 2010; Devlin et al., 2015).
Extreme environmental variables and anthropogenic pollutants can control and modulate the Kuwaiti marine ecosystem. However, bacterioplankton community composition and diversity patterns in the Kuwaiti marine environment and the effect of physicochemical variables on bacterioplankton community structure in Kuwaiti waters have not been extensively characterized. Exploring the microbial community composition and distribution in Kuwaiti waters is key to understanding and predicting marine ecosystem function responses to changing environmental conditions. Very few studies have explored bacterioplankton communities in the Kuwaiti marine environment. Most of these studies were specifically focusing on the isolation of indigenous hydrocarbon-degrading bacteria from Kuwait waters (Radwan et al., 2005; Al-Awadhi et al., 2007; Mahmoud et al., 2009; Al-Mailem et al., 2010; Mahmoud et al., 2010; Al-Awadhi et al., 2012) and only one study explored the spatial and temporal variations in the bacterioplankton composition of the coastal waters of Kuwait (Almutairi, 2015). However, in this study, low-throughput 16S rRNA gene clones were used, which unfortunately did not cover the full bacterioplankton diversity present. In the current study, we aimed to address this knowledge gap by using 16S rRNA high-throughput amplicon sequencing to determine seasonal changes in the bacterioplankton assemblage composition, diversity, and function in Kuwaiti waters.
In this study, we selected Kuwait Bay and four offshore sites in close proximity to highly urbanized coastal areas. Surface water samples were collected from these sites in autumn (October) and spring (April). 16S rRNA amplicon sequencing was employed to explore bacterioplankton community profiles in the heavily anthropogenically impacted Kuwait Bay and offshore samples both spatially and temporally. Physicochemical variables were also measured during sampling to link the bacterioplankton composition with these variables. The results obtained from this study generated extensive 16S rRNA amplicon sequence foundational datasets indigenous to the Kuwaiti marine environment. Furthermore, they provide a comprehensive understanding of bacterioplankton community structure, spatiotemporal dynamics, and potential metabolic activities in the Kuwaiti marine ecosystem, and provide indications for the physicochemical variables that may modulate them.
Materials and Methods
Sampling and Filtration
Surface water samples at 1 m depth were collected from five sites twice, in October 2018 and April 2019 (Figure 1). The first site was located in the center of the highly urbanized and anthropogenically disturbed Kuwait Bay (KB) (47°57′272′′ E, 29°26′185′′ N, depth 12 m). Four offshore sites in close proximity to urbanized coastal areas and human activities were selected. These were the Messila (M) (48°20′6072′′ E, 29°29′5392′′ N, depth 15 m), Fintas (F) (48°32′3806′′ E, 29°15′1261′′ N, depth 15 m), Khiran (KH) (48°45′172′′ E, 28°68′131′′ N, depth 15 m), and Shuaiba (SH) (48°23′3611′′ E, 28°99′7722′′ N, depth 15 m) sites. The distances between sampling sites were 29 km (between KB and M sites), 8 km (between M and F sites), 20 km (between F and SH sites), and 34.7 km (between SH and KH sites). One liter of surface water samples was collected from each site in quadruplicate using a peristaltic pump. The water samples were kept in polyethylene bottles on ice and processed on the same day. In the laboratory, the water samples from each site were pooled and thoroughly mixed, and 1 L of water was filtered through a sterile 0.22 µm bottle top filter (Filtermax rapid; TPP Techno Plastic Products AG, Trasadingen, Switzerland). The filter was aseptically removed, placed in a sterile container, and stored at −80°C until further analysis.
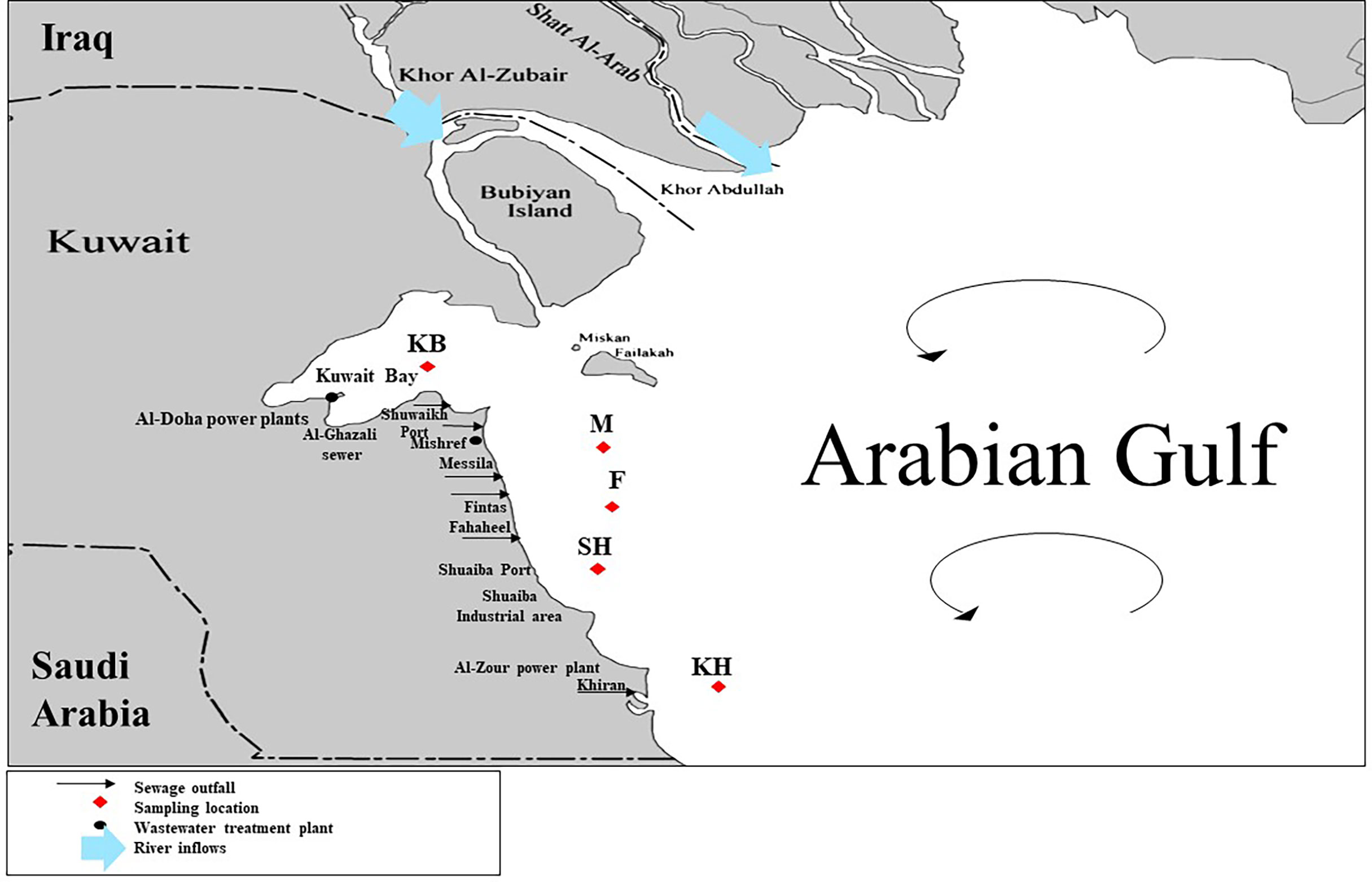
Figure 1 Kuwait map showing the locations of the five sampling sites: Kuwait Bay (KB), Messila (M), Fintas (F), Shuaibah (SH) and Khiran (KH). The map shows the predominant surface water circulation patterns in the Arabian Gulf.
Seawater Quality and Chemical Analysis
At each sampling site, environmental parameters such as turbidity, pH, dissolved oxygen (DO), conductivity, temperature, and salinity were measured using a water quality checker (Horiba LTD, Japan).
Fifty milliliters of the unfiltered water samples were used for nitrate and phosphate analysis using an ion chromatography meter. One liter of the unfiltered water sample was used for total petroleum hydrocarbon (TPH) analysis. The water samples were first extracted following the US-EPA 3510C (Update III, June 1997) method, and the extracted samples were analyzed by trace gas chromatography with FID (Thermo Finnigan) using the US-EPA 8015C (Rev. February 3, 2007) method.
Extraction of Environmental DNA and 16S rRNA Sequencing and Analysis
Total genomic DNA was extracted from the stored filters using a DNeasy PowerWater kit (Qiagen), following the manufacturer’s instructions. Each filter was cut in half and treated separately, and after environmental DNA elution, the DNA samples from the two parts of the same filter were combined. The quantity and quality of the extracted environmental DNA were checked using a NanoDrop Lite spectrophotometer (Thermo Fisher Scientific). Purified DNA extracts were then stored at -80°C until further analysis.
The 16S rRNA gene was amplified with universal primers 27f (5’-AGAGTTTGATCTGGCTCAG-3’) and 1492r (5’-TACGGYTACCTTGTTACGACTT-3’) (Lane, 1991) using ready-to-go PCR beads (GE Healthcare, UK). PCR was performed in 25 μL reaction mixtures (2.5 μM of each primer, ~2.5 μM of environmental DNA). The PCR conditions were as follows: initial denaturation at 95°C for 5 min, followed by 30 cycles of denaturation at 94°C (30 s), annealing at 52°C (30 s), and extension at 72°C (1 min), followed by a final extension at 72°C (10 min) (Lane, 1991). The PCR products were visualized using 1% gel electrophoresis. PCR was performed thrice for each sample, and the PCR products were pooled and purified using a PCR purification kit (Qiagen). The quantity and purity of the cleaned amplified DNA products were measured using a Qubit 2.0 fluorometer (Thermo Fisher Scientific) and the Qubit ds DNA High sensitivity assay kit (Thermo Fisher Scientific) following the manufacturer’s protocol.
The primers 27F (AGRGTTTGATCMTGGCTCAG; Vergin et al., 1998) and 519Rmod (GTNTTACNGCGGCKGCTG; Andreotti et al., 2011) targeting the V1-V3 variable region of the amplified products were used for amplicon sequencing using the bTEFAP® (MR DNA www.mrdnalab.com) method. Each sample was subjected to a single-step 30 cycle PCR using HotStarTaq Plus. A Master Mix kit (Qiagen, Valencia, CA) was used under the following conditions: 94°C for 3 min, followed by 30 cycles at 94°C for 30 s, 53°C for 40 s, and 72°C for 1 min, after which a final elongation step at 72°C for 5 min was performed. After PCR, all amplicon products from different samples were mixed in equal concentrations and purified using Agencourt Ampure beads (Agencourt Bioscience Corporation, MA, USA). The samples were sequenced using Illumina MiSeq chemistry, following the manufacturer’s protocols at Mr. DNA (USA). Raw sequencing reads were deposited in NCBI under Bioproject number PRJNA783270.
The obtained sequence data were processed using the QIIME 2 (Quantitative Insights into Microbial Ecology) software package (v2-2019.10) (Bolyen et al., 2019). Briefly, paired-end sequences imported into QIIME 2 were first demultiplexed using the qiime2 demux function. Barcodes, primers, and low-quality bases at the ends of both the forward and reverse primers were trimmed using the qiime2-dada2 plugin. The sequences were then quality filtered, dereplicated, merged, and chimera-checked to generate amplicon sequence variants (ASVs) using the qiime2-dada2 plugin. The taxonomic assignment of the ASVs was carried out using the qiime2 naive Bayes classifier trained on the Greengenes (v13_8) database with a 99% OTUs identity threshold. Following taxonomic classification, ASVs comprising singletons or those classified as chloroplasts or mitochondria were excluded from the downstream analysis. Individual abundant representative ASVs lacking taxonomic assignment at the genus level in the Greengenes database were further checked using the SILVA database.
Functional Predictions
The 16S rRNA sequence data were used for functional predictions using the Phylogenetic Investigation of Communities by Reconstruction of Unobserved States (PICRUSt) (Langille et al., 2013). The sequences were clustered against the Greengenes database v.13.5 using the closed-reference clustering approach in QIIME 2 (Version 2019.10) according to the online protocol. Functional predictions were performed in PICRUSt (Galaxy version 1.0.0) using the Kyoto Encyclopedia of Genes and Genomes (KEGG). The analyses were performed at three KEGG pathway hierarchical levels. The obtained data were statistically analyzed using STAMP (Statistical Analysis of Taxonomic and Functional Profiles, v.2.1.3) (Parks et al., 2014).
Statistical Analysis
One-way analysis of variance (ANOVA) was performed to check the dissimilarity in environmental parameters between samples and seasons using Excel.
Alpha and beta diversity analyses were performed in Qiime 2 and rarified to an even sampling depth of 12,682. Alpha diversity was estimated by determining Shannon’s diversity index (Shannon’s DI), Faith’s phylogenetic diversity (Faith’s PD), and the observed features of ASVs. The evenness was calculated using Pielou’s evenness (Pielou’s E). Beta diversity between samples was estimated based on Bray-Curtis dissimilarity distances and visualized via principal coordinate analysis (PCoA).
A linear discriminant analysis (LDA) effect size pipeline (LEFSe) (Segata et al., 2011; available at http://huttenhower.sph.harvard.edu/galaxy/), was used to identify significantly differentially distributed ASVs and also significantly differentially distributed pathways among groups. A threshold of 3.0 on the logarithmic LDA score was selected for discriminative features.
All statistical analyses were run in the statistical program R (version 3.1.2). Pairwise analysis of similarity (ANOSIM) based on Bray-Curtis dissimilarity between seasonal community groups was performed. Principal component analysis (PCA) was carried out to investigate the relationship between the physicochemical parameters and the distribution of the sampling sites in an ordination plot. Indicator species indicative of a particular group were identified using the indispecies package in R. Venn diagrams constructed using the VennDiagram package were performed to display ASVs shared between samples.
The associations between bacterial communities and physicochemical variables were explored by canonical correspondence analysis (CCA) using the ‘vegan’ package in R and the envfit parameter. The data were log (x+1) transformed prior to statistical analysis. Spearman’s correlation analysis was conducted to check the correlations of the top abundant taxa and environmental variables and the correlation of the abundant metabolic pathways and top abundant taxa and visualized in a heatmap using the pheatmap package in R.
Results
Overview of Hydro-Physicochemical Conditions
The physicochemical parameters measured from seasonal samples at the five sites are presented in Table 1. The recorded temperature had significant variations between the two seasons (P < 6.98 × 10-8), with higher temperatures in autumn. The average temperature in autumn was 30.3°C, while in spring it was 23.6°C. The overall temperature varied from 23.21°C (F-APR) to 30.59°C (M-OCT) with a mean of 26.9°C (± 3.5). In all samples, the pH varied from 8.17 to 8.49 with a mean of 8.36 (± 0.11) and was slightly higher in autumn than in spring. The overall water salinity of all samples ranged between 3.8% and 3.9%, while it varied slightly seasonally, with higher salinity in spring. Dissolved oxygen values ranged between 5.52 mg/mL and 10.13 mg/mL with a mean value of 8.47 (± 1.49) and higher values in autumn than in spring. The turbidity levels ranged between 0.42 NTU and 2.0 NTU and turbidity varied seasonally with slightly higher average turbidity in autumn. The nitrate concentration revealed significant seasonal variations (P < 2.6 × 10-8). Higher nitrate levels were observed in spring than in autumn, and the average nitrate concentrations were 1.15 mg/L and 10.87 mg/L in autumn and spring, respectively. The phosphate concentration also exhibited significant seasonal variation (P < 1.27 × 10-9), with higher concentrations in spring and below the detection limits for all autumn samples. The phosphate concentration in the spring season ranged between 8,582.99 mg/L (F-APR) and 9,788.95 mg/L (SH-APR), with an average of 8,971.4 mg/L. The TPH concentration also exhibited significant seasonal variation (P < 0.05), with higher concentrations in early autumn than in spring.
Principal component analysis (PCA) was performed to explore the influence of measured physicochemical parameters at the sampling sites on the distribution of the seasonal samples (Figure 2). The PCA biplot showed a clear seasonal separation between the samples. All spring samples clustered separately from autumn samples and PCA explained 78.9% of the total variance along the first two axes. The first axis (PC1) explained 56.5% of the variability and was positively correlated with salinity, nitrate, and phosphate levels, but negatively correlated with temperature, pH, TPH, DO, and turbidity. The second axis explained 22.4% of the variability and was positively correlated with turbidity, TPH, salinity, and temperature, but negatively correlated with pH, DO, nitrate, and phosphate levels.
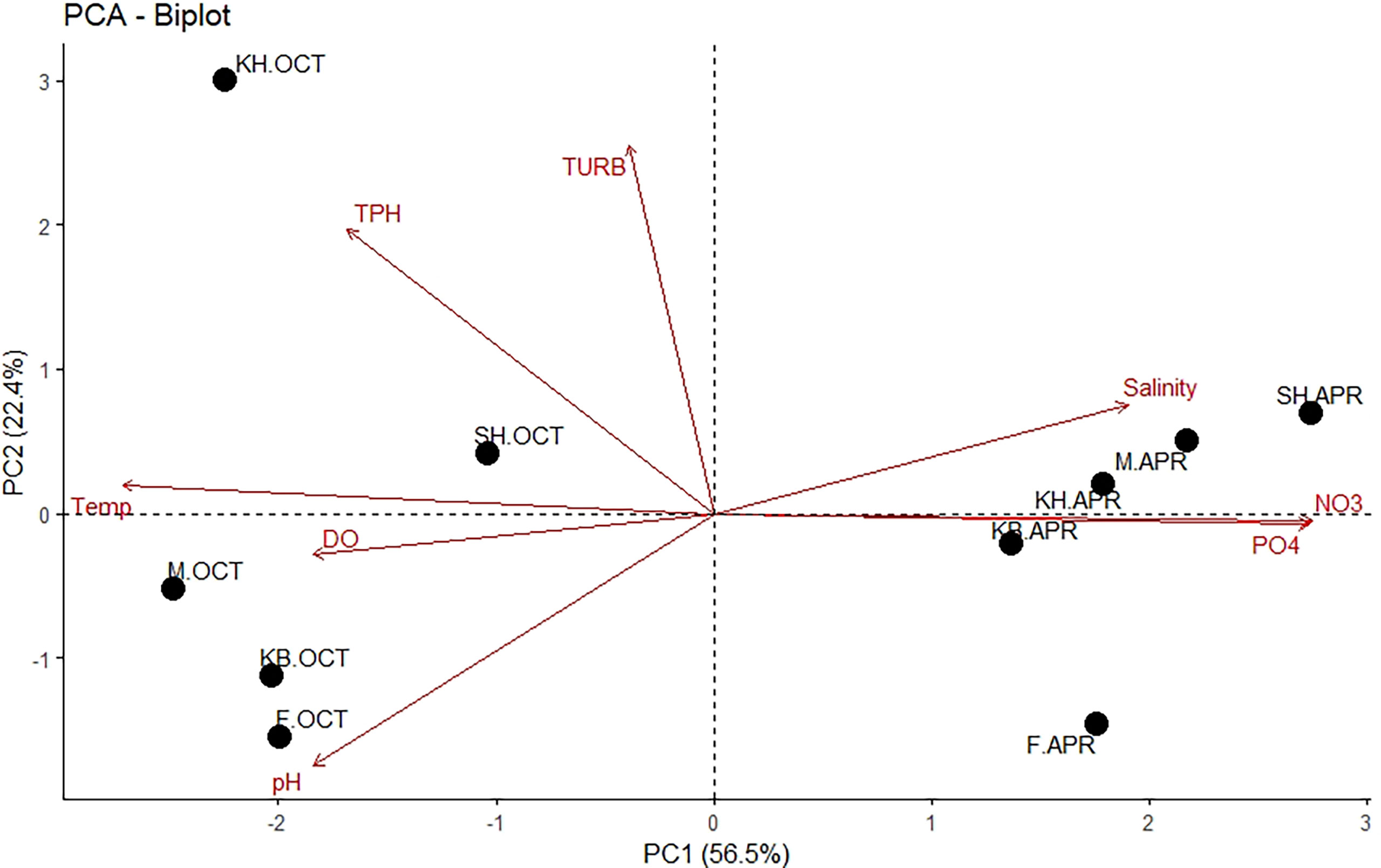
Figure 2 Principal Component Analysis (PCA) of the physicochemical variables measured at the sampling sites. The percentage of variability explained by axes is shown.
Overview of the Sequencing Data
A total of 230,725 high-quality reads were obtained for all the samples and clustered into 991 ASVs. The Shannon rarefaction curves for all samples plateaued, suggesting a sufficient representation of the overall bacterial diversity of the samples (Supplementary Figure 1). The number of ASVs, Shannon, and Pielou diversity indices, as well as the phylogenetic diversity (Faith’s PD) richness index per sample are summarized in Supplementary Table 1. The number of ASVs per site varied between 209 and 252 in autumn, and between 173 and 222 in spring. A Venn diagram was constructed to show the distribution of the total number of ASVs detected across all samples (Figure 3A). The analysis revealed that only 173 of the 991 ASVs were shared among the seasonal samples. In addition, 482 and 336 unique ASVs were identified in the autumn and spring samples, respectively, accounting for 73.5% and 66.0% of the total ASVs, respectively. The bacterial taxa shared among the sites (core microbiome) were represented by 55 ASVs in autumn as well as by 55 ASVs in spring (Supplementary Figure 2).
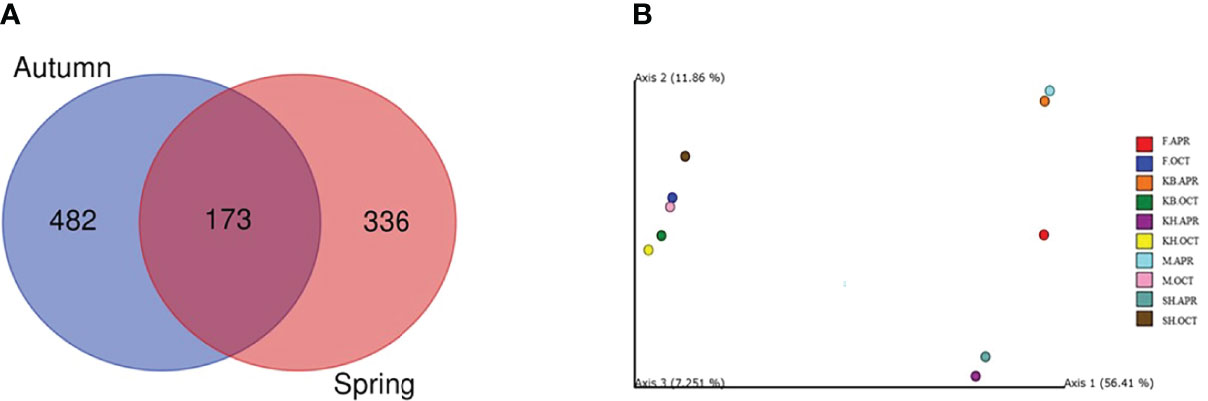
Figure 3 (A) Venn diagram showing the number of shared and unique ASVs between the seasonal samples. (B) PCoA analysis of sample beta diversity using Bray Curtis distance. Clustering of samples by month of sampling is observed.
In autumn, the Shannon and Pielou diversity measures were lower in Kuwait Bay and higher at Fintas and Shuaiba. However, in spring, the Shannon and Pielou diversity measures were higher in Kuwait Bay and lower at Khiran. Faith’s PD richness index was higher at Khiran and lower at Shuaiba in autumn, while the opposite was true in spring. All alpha diversity estimates exhibited a significant seasonal diversity (Supplementary Figure 3). The number of ASVs was significantly higher in autumn than in spring (P < 0.05). The alpha-diversity measures (Shannon and Pielou) were significantly higher in spring than that for autumn (P < 0.05), while Faith’s PD richness index was significantly higher (P < 0.05) in autumn. The beta diversity based on the Bray–Curtis distance between samples revealed a significant seasonal dissimilarity (P < 0.05) and clear separate clustering of autumn and spring samples (Figure 3B), along with the primary principal coordinate explaining 56.41% of the total variability. The autumn samples were grouped together, and higher variability was observed in the bacterial composition of spring samples. The ANOSIM R statistic also confirmed significant differences in the bacterial community composition of the seasonal samples (R =1; P < 0.01).
Bacterial Community Composition
The taxonomic classification identified 19 phyla, 37 classes, 73 orders, 101 families, and 132 taxa. The four most dominant phyla accounted for >87.9 of the ASVs in all samples, and the major phyla were Proteobacteria (49%), Cyanobacteria (16%), Bacteroidetes (16%), and Actinobacteria (7%) (Figure 4A). The relative abundance of Proteobacteria increased in spring, while that of Cyanobacteria increased considerably in autumn. Further classification at the class level revealed that the samples were dominated by five classes: Alphaproteobacteria (30%), Synechococcophycideae (16%), Gammaproteobacteria (14%), Flavobacteriia (13%), and Acidimicrobiia (6%).
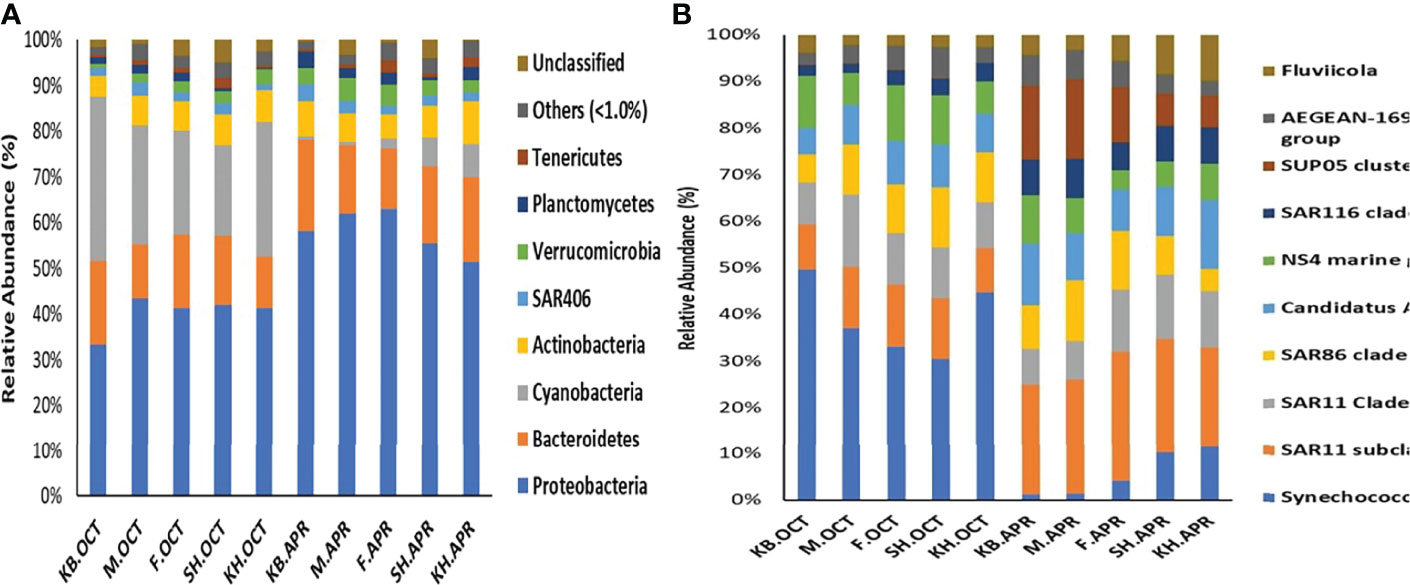
Figure 4 (A) The relative abundances of the bacterial community composition from the 10 samples at phylum and (B) The relative abundances of the top 10 abundant taxa from the 10 samples. Bacterial phylotypes with a relative abundance of < 1% were labeled as “others”.
Over 94% of the shared ASVs among autumn samples and 96% of the shared ASVs among spring samples belonged to the top 10 most abundant taxa. In addition, the top 10 abundant taxa with ASVs collapsed to the genus rank were present in all samples, but in different proportions (Figure 4B). To identify the differentially abundant bacterial groups indicative of seasonal samples, we performed indicator species analysis using taxa with at least 1% relative abundance in any sample. This analysis (Supplemental Table 2) identified 21 indicator species that were significantly associated with one of the seasons. Six indicator taxa were differentially abundant in autumn, and 15 indicator taxa were differentially abundant in spring. The majority were lower abundance taxa, and four of the top 10 abundant taxa were recognized as indicator taxa. Synechococcus was significantly differentially abundant in autumn, and the SAR11 clade Ia, SUP05 cluster, and SAR116 clade were significantly differentially abundant in spring. We also performed linear discriminant analysis effect size analysis (LEFSe), and the results showed that 22 taxa were differentially abundant between the seasonal samples (LDA >3.0) (Supplementary Figure 4). Similar to the indicator species results, most of the enriched taxa had low abundance, and three of the top 10 abundant taxa were considered differentially abundant. Synechococcus and NS4 marine group were enriched in autumn, while the SUP05 cluster was enriched in spring.
Association of the Bacterioplankton Community Composition With Physicochemical Variables
Canonical correspondence analysis (CCA) was performed to detect the specific environmental variables that could influence the bacterial community composition in the seasonal samples (Figure 5A). The CCA explained 52.11% of the variance. Based on the envfit parameter, Monte Carlo permutations (999 permutations) revealed that only TPH (P > 0.001), nitrate (P > 0.009), phosphate (P > 0.007), and temperature (P > 0.008) were significant determinants of the bacterial community composition (Supplementary Table 3). Nitrate and phosphates were determinants at all sites in spring, and the bacterial community was largely explained by temperature at all sites in autumn, except for Khiran.
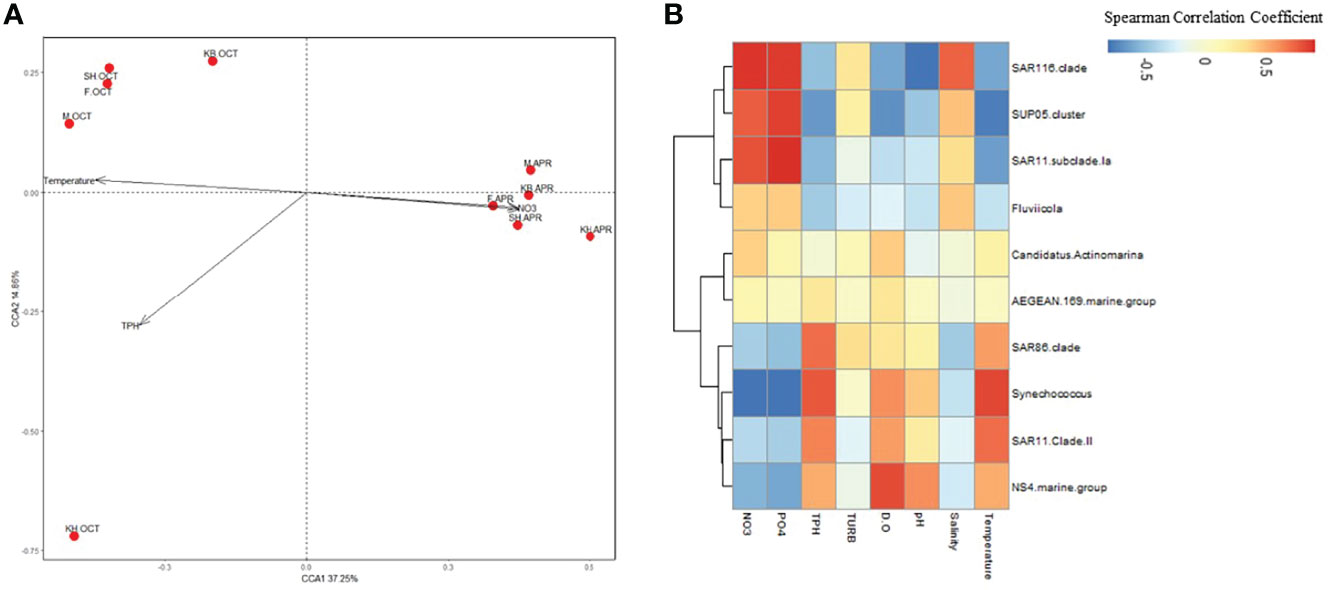
Figure 5 The association between physicochemical parameters and the bacterial community structure. (A) CCA ordination plot depicting the relationship between physicochemical parameters and bacterial community composition. (B) Heatmap of Spearman’s correlations between physicochemical variables and the top 10 abundant taxa. The color legend shows correlation coefficients.
Spearman’s correlation analysis was also used to examine the association between the bacterial community composition represented by the top 10 abundant taxa and the physicochemical parameters (Figure 5B). The analysis revealed contrasting correlation patterns with all tested parameters, except for turbidity. Furthermore, the taxa were grouped by their correlation coefficients with physicochemical variables into two clusters. Cluster I was associated with taxa that exhibited higher abundance in spring, while Cluster II taxa had higher abundance in autumn.
Within Cluster I, SAR11 subclade 1a, SUP05 cluster, and SAR116 clade exhibited strong significant positive correlations with nutrients and negative correlations with temperature, dissolved oxygen, and TPH. However, they displayed different correlations with salinity, except for SAR116 clade taxa, which displayed a strong positive correlation with salinity. Flaviicola, another member of Cluster I, exhibited positive correlations with salinity and nutrient levels and negative correlations with other variables.
Within Cluster II, Synechococcus spp. displayed strong and significant positive correlations with temperature and TPH as well as a good positive correlation with dissolved oxygen and a weak correlation with pH. However, Synechococcus was negatively correlated with salinity and had a significantly negative correlation with nutrients. Furthermore, the SAR86 clade and NS4 marine group taxa both exhibited positive correlations with temperature and TPH; however, the NS4 marine group taxa showed more positive correlations with pH and dissolved oxygen. In Cluster II, the AEGEAN-169 marine group taxa and Candidatus Actinomarina exhibited weak positive correlations with all physicochemical variables.
Functional Predictions of the Bacterioplankton Communities
To check the effect of seasonal differences in taxonomic composition on the metabolic functions across samples, we used 16S rRNA sequences and PICRUSt to explore the functional capacity of the bacterial communities inhabiting the different samples. At the first level of KEGG functional categories, PICRUSt analysis predicted basic physiological activities including metabolism, cellular processes, genetic information processing, and environmental information processing (Supplementary Figure 5). The PCA profiles of the functional pathways at the first level of KEGG functional categories showed grouping of same season samples and a slight separation between autumn and spring samples (Figure 6A). However, the differences in the mean proportions of the predicted pathways between the two seasons were less than 1% (Supplementary Figure 6) suggesting functional redundancy. In the second-tier KEGG functional category hierarchy, 27 pathways were identified; in the third-tier KEGG functional category hierarchy 223 functional orthologs were predicted. The LEFSe analysis of the predicted third-tier KEGG pathways identified two pathways, “transporters” and “ABC transporters,” which were enriched in spring samples, while the “photosynthesis proteins” pathway was enriched in autumn samples (Figure 6B).
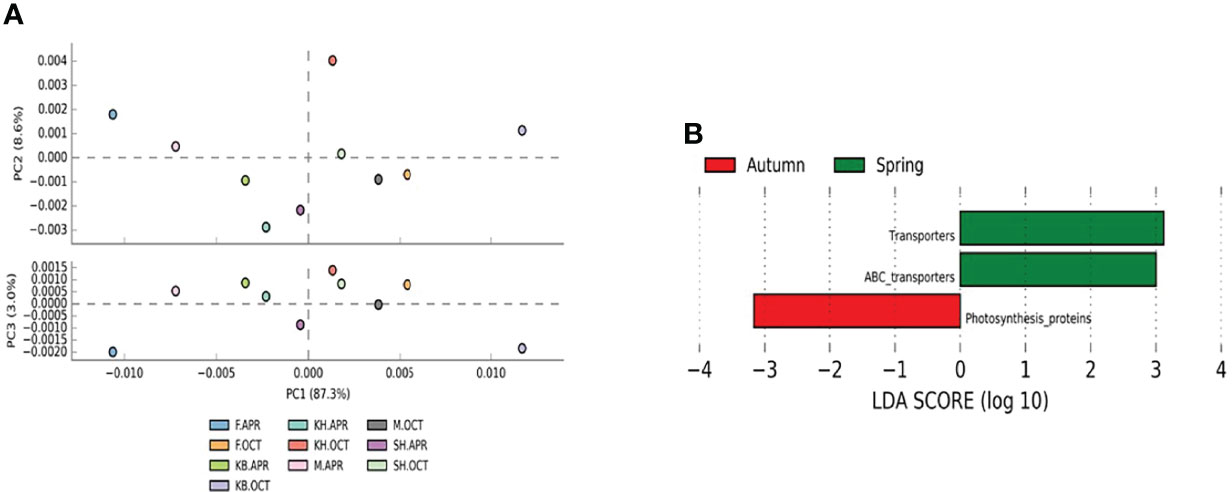
Figure 6 PICRUST prediction of functional profiling of the bacterioplankton communities based on 16S rRNA sequences for all samples. (A) Principal component analysis plot of distances between the seasonal sample pathway profiles. PC1 accounted for 87.3% and PC2 8.6% of the variation in the PICRUST predicted pathways between autumn and spring samples. (B) LEfSe analysis of the significantly differential abundant predicted KEGG categories at Level 3 between autumn and spring samples. Spring-enriched pathways are indicated with a positive LDA score (green), and pathways enriched in autumn with a negative score (red).
Spearman’s correlation analysis was performed to explore the association between the highly abundant third-tier KEGG functional category pathways and the top 10 abundant taxa (Supplementary Figure 7). The results showed that photosynthetic proteins displayed a significantly positive correlation with Synechococcus and SAR11 clade II and a negative correlation with the remaining taxa. On the other hand, transporters and ABC transporters revealed a significantly positive correlation with SAR11 subclade Ia, a positive correlation with the SUP05 cluster, and a negative correlation with Synechococcus.
Discussion
In this study, we used 16S rRNA amplicon sequencing to research the seasonal diversity of bacterioplankton community dynamics in Kuwait Bay and four sites along the Kuwait coastal shoreline. This allowed us to explore the spatiotemporal and functional diversity of bacterioplankton communities in the context of variable physicochemical parameters. Our results provide the first detailed insight into the microbial ecology of Kuwait’s waters and reveal strong temporal bacterioplankton patterns and differences in the microbial diversity of bacterioplankton. The general bacterioplankton community composition detected in Kuwait littoral waters and the most dominant ASVs that constituted the core microbiome in the surface waters of all examined sites were Proteobacteria, Cyanobacteria, Bacteroidetes, and Actinomycetes. This bacterioplankton composition is similar to those found in several marine and coastal environments (Giovannoni and Rappé, 2000; Gilbert et al., 2009; Gilbert et al., 2012; Sunagawa et al., 2015). However, the observed spatial distribution patterns of the bacterioplankton community among sites could be, as suggested by other studies, related to the environmental heterogeneity between sites (Quero and Luna, 2014; Liu et al., 2015).
Studies have shown that bacterioplankton communities at any specific space or time are frequently dominated by a few abundant taxa, while the majority of the remaining taxa are rare (Rappé et al., 2000; Sogin et al., 2006; Campbell et al., 2011; Gilbert et al., 2012). A large number of unique or rare ASVs with relatively low abundances (< 1%) were detected in the seasonal samples, thereby suggesting that these rare ASVs represent rare microbial diversity and thus account for most of the observed seasonal diversity between the autumn and spring samples. These rare ASVs could also be important for community dynamics and can be used as indicators of changes in the ecosystem status (Kirchman et al., 2010).
The bacterial alpha diversity and abundance of all major phyla, except cyanobacteria, increased in spring, when the conditions were highly eutrophic. The alpha diversity of bacterioplankton communities in coastal waters usually responds positively to increasing eutrophication levels (Dai et al., 2017). All of the most abundant taxa in this study have frequently been detected in the microbial communities of various marine (Morris et al., 2002; Cram et al., 2015) and coastal (Campbell et al., 2011; Gifford et al., 2014; Campbell et al., 2015) water environments and have been recently detected in heavily impacted polluted shoreline waters (Zimmer-Faust et al., 2021). The top 10 abundant taxa were present at all sites, but in various ratios, which is similar to the identified global patterns of bacterial diversity in surface waters (Pommier et al., 2007; Zinger et al., 2011). Our results showed that four of the top 10 abundant taxa could be recognized as indicator taxa for seasonal samples. Additionally, our results revealed contrasting correlation patterns with temperature, nutrient levels, and TPH, thereby suggesting a coherent response to the surrounding conditions. The patterns obtained mirrored the clear partitioning observed between the seasonal samples in both the PCA and CCA plots (Figures 2, 5A). This also confirms the beta-diversity measures and ANOSIM analysis results, which revealed significant compositional differences between seasonal samples.
Seasonal microbial succession and community dissimilarity in open water are usually linked to temperature and nutrient levels, mainly phosphorus and nitrogen (Treusch et al., 2009). Nutrient availability and resource partitioning can also influence niche structure (Church, 2009). Furthermore, several studies have shown that the partitioning of bacterioplankton communities and the observed shifts in relative abundance are potentially linked to the surrounding physicochemical environment and increased anthropogenic nutrient inputs (Perryman et al., 2011; Jeffries et al., 2016). Significant differences were observed in the nutrient concentrations of the seasonal samples. Several studies have indicated the influence of nutrients on the diversity, structure, and composition of bacterioplankton communities in marine ecosystems (Gilbert et al., 2009; Andersson et al., 2010; Gilbert et al., 2012; Bunse and Pinhassi, 2017). Freshwater input from the Shatt Al-Arab River, which usually occurs in spring, increases the nutrient levels (Al-Yamani et al., 2004). Additionally, temperature changes can also alter nutrient levels and freshwater inputs (Statham, 2012). Our results showed high nitrate and phosphate levels in spring and minimal levels in autumn. Similarly, Al-Said et al. (2019) also reported a temporal decrease in phosphate in Kuwaiti waters from winter-spring to summer-autumn. The high level of nutrients in spring could also be related to human-related activities, such as the release of industrial effluent and contaminants from desalination and power plants, and the discharge of domestic sewage into the sea (Al-Sarawi et al., 2015; Devlin et al., 2015). Historically, the discharge of large quantities of raw sewage into the sea through emergency outlets during the malfunction of the Mishref sewage station in 2009 caused a drastic reduction in water quality parameters, leading to a large increase in ammonia and phosphate levels and anthropogenic eutrophication in coastal waters (Al-Sarawi et al., 2015; Devlin et al., 2015; Al-Said et al., 2019).
The cosmopolitan SAR11 clade was consistently the most abundant in the dataset. The SAR11 clade comprises aerobic chemoheterotrophic free-living bacteria that usually favor oligotrophic conditions, but are also found in nutrient and organic matter-rich coastal waters (Rappé and Giovannoni, 2003; Wilhelm et al., 2007; Lami et al., 2009). The SAR11 clade normally exhibits higher abundance in spring following phytoplankton blooms (Treusch et al., 2009; Delpech et al., 2021). Owing to the high nutrient input and eutrophication, phytoplankton blooms, including macroalgae and harmful blooms, occur frequently in Kuwaiti waters, mostly during spring and autumn (Al-Yamani et al., 2012; Polikarpov et al., 2020). The abundance of the SAR11 clade increases in the presence of increased inorganic nutrient concentrations (Gilbert et al., 2012). Nutrient availability can also promote the growth of distinctive SAR11 ecotypes (Pinhassi and Berman, 2003), such as the observed higher abundance of SAR11 subclade Ia in spring in the presence of high nutrient levels. In contrast, the abundance of SAR11 clade II in autumn displayed a negative correlation with nutrients, suggesting the autumn phylotype’s differential preference to inhabit niches with lower nutrient levels. Consistent inverse abundance patterns for these two SAR11 clades have been previously observed (Salter et al., 2015) and seasonal variations in the abundance and diversity of SAR11 clades have been detected in tropical, subtropical, temperate, and freshwater environments (Logares et al., 2010; Chow et al., 2013; Heinrich et al., 2013; Vergin et al., 2013; Salter et al., 2015). Several studies have shown that temperature changes can alter bacterial metabolic activities and influence the structure and composition of bacterial communities (Gilbert et al., 2009; Kirchman et al., 2009; Gilbert et al., 2012). In addition, temporal variability in SAR11 clade abundance is mostly driven by temperature (Brown et al., 2012; Salter et al., 2015). SAR11 clades often exhibit higher abundance with increasing temperatures (Lefort and Gasol, 2013). However, our results showed that the two abundant SAR11 clades displayed contrasting correlations with temperature, suggesting their partitioning into different environmental niches.
Cyanobacteria was the second most abundant phylum in this study, and the overall patterns in the bacterial composition detected in this study were mostly related to the temporal shift in Cyanobacteria abundance in autumn. Synechococcus is globally dispersed throughout shallow temperate and tropical waters (Palenik et al., 2003; Flombaum et al., 2013). Our results are also in agreement with those of other studies that considered gulf surface water to be one of the world’s richest water bodies in terms of Picocyanobacteria, specifically Synechococcus (Al-Hasan et al., 2001; Radwan et al., 2005; Al-Bader et al., 2011). In this study, Synechococcus displayed a strong positive correlation with temperature. A previous study on Kuwait coastal waters confirmed that cyanobacteria abundance, mainly Synechococcus, increased with elevated temperature and that Synechococcus can still grow at 34°C (Al-Bader et al., 2011). In contrast, Synechococcus exhibited a significantly strong negative correlation with nutrients. Synechococcus generally consists of many distinctive lineages, each with a different niche preference. Studies have shown that some of these Synechococcus lineages are more abundant in coastal waters with a wide range of nitrate and phosphate levels (Scanlan et al., 2009). The high abundance of Synechococcus in autumn, despite the phosphate limitation, confirms earlier findings that N/P levels are not a limiting factor for Synechococcus growth in local waters (Al-Bader et al., 2011).
A higher abundance of Gammaproteobacteria is usually detected in anthropogenically disturbed regions (Cabral et al., 2016). The abundant member of this class, the SUP05 clade, exhibited a higher abundance in spring when the conditions were eutrophic. The SUP05 clade consists of abundant, cosmopolitan chemoautotrophic bacteria (Walsh et al., 2009; Wright et al, 2012; Shah et al., 2017) with an important role in carbon fixation, sulfur oxidation, and denitrification (Walsh et al., 2009; Mattes et al., 2013; Hawley et al., 2014). A recent study considered this clade a strong indicator of eutrophication in anthropogenically impacted bays on the coast of Vietnam (Kopprio et al., 2021). The SUP05 clade displayed a negative correlation with temperature and usually prefers lower temperatures for growth (Shah et al., 2017). The SUP05 clade displayed strong positive correlations with nitrate and phosphate levels. This clade is usually more abundant at the nitrite maxima of the oxygen minimum zones (Shah and Morris, 2015; Shah et al., 2017) and the euphotic zone of the gulf usually accumulates high levels of nitrite (Al-Yamani and Naqvi, 2019). In addition, the activities of this clade and the increase in organic matter remineralization can cause an increase in phosphate concentration (Lomnitz et al., 2016).
The Gulf is home to most of the world’s oil reserves; and most of the oil is marine-transported from shallow Gulf waters (Radwan et al., 2005; Al-Sarawi et al., 2015). Oil pollution is a persistent problem in the entire Gulf region, affecting both the biotic and abiotic features of the Gulf (Sheppard et al., 2010). Several studies have shown that oil contamination in Gulf water stimulates the growth of specialized hydrocarbon-degrading bacteria (Radwan et al., 2005; Mahmoud et al., 2009; Al-Mailem et al., 2013). Alphaproteobacteria and Gammaproteobacteria play major roles in oil degradation (Coulon et al., 2007; Mason et al., 2012). Although the CCA plot (Figure 5A) identified TPH as a major determinant of the bacterial communities in the seasonal samples, none of the samples revealed an association with TPH. In addition, all abundant taxa in spring exhibited negative correlations with TPH. A recent study showed that although the SUP05 clade was abundant in plume-derived hydrocarbon-degrading consortia, the purified isolate failed to degrade any of the tested hydrocarbons (Wang et al., 2020). Previous studies have found that picocyanobacterial isolates from the Gulf, including Synechococcus, can accumulate hydrocarbons and make them available to associated hydrocarbon-utilizing bacteria (Al-Hasan et al., 2001: Radwan et al., 2005). These studies concluded that these associated hydrocarbonoclastic bacteria are also important for enhancing Synechococcus growth (Radwan et al., 2005). Interestingly, the SAR86 clade, Synechococcus, and Flavobacteriaceae, which correlated positively with TPH, were recently detected in the microbial community along the oil pollution continuum in the Gulf (Rezaei Somee et al., 2021). Flavobacteriaceae are chemoorganotrophic bacteria, many of which degrade hydrocarbons (Kwon et al., 2006; Gutierrez et al., 2014; Hazen et al., 2016). The Flavobacteriaceae NS4 marine group displayed a negative correlation with nutrients, and the abundance of Flavobacteriaceae decreases in nutrient-enriched sites (Fodelianakis et al., 2014); this is in agreement with our findings. Also, a previous study showed that Flavobacteriaceae recovered from anthropogenically impacted estuaries exhibit a negative correlation with nutrients (Jeffries et al., 2016).
Salinity and dissolved oxygen are key factors shaping the structure and composition of microbial assemblages (Herlemann et al., 2011; Kirchman et al., 2017; Aldunate et al., 2018). However, the masked effect of these variables and the remaining environmental variables such as turbidity and pH, indicates, as suggested by many studies, that the local distribution of bacterial communities is regulated by a combination of several environmental variables (Quero and Luna, 2014; Liu et al., 2015; Richa et al., 2017); it also suggests that other unmeasured environmental factors and top-down pressures, such as grazing or viral lysis, influenced the structure of bacterial communities in the seasonal samples.
Investigating the metabolic potentials of the bacterial communities of the seasonal samples based on 16S rRNA sequences revealed that, although significant seasonal differences in the bacterial community composition existed between the samples, the distribution of the functional categories and variations in their metabolic potentials were similar. This suggests that many of the metabolic functions are shared between the bacterial communities of the seasonal samples and that these functional pathways are present throughout the water column, regardless of the season. Such functional redundancy or richness between seasonal samples could imply the presence of varied species diversity adjusted to a variety of niches (Sunagawa et al., 2015). Therefore, the absence of one member of the community does not eliminate the function provided by that member, as other members can also provide the function, suggesting the stability of the system. Furthermore, the LEfSe results suggested the enrichment of membrane transport genes, specifically ABC transporters, in the spring season. Transporters are ubiquitous in marine and coastal environments (Sowell et al., 2009; Poretsky et al., 2010) and their primary function in bacteria is to import carbon and energy sources, as well as the release of metabolites (Davidson and Chen, 2004). ABC transporters are essential for carbon and nutrient cycling in marine environments (Sowell et al., 2009) and are usually used to detect bacterial dissolved organic matter consumption patterns (Jiao and Zheng, 2011; Tang et al., 2012). In addition, the correlation analysis revealed a positive correlation of transporters and ABC transporters with the indicator taxa of the spring SAR11 subclade Ia and SUP05 cluster. In autumn, the LEfSe results showed the differential abundance of photosynthetic proteins, and the correlation analysis showed a strong positive correlation of photosynthetic proteins with indicator taxa of autumn Synechococcus. However, more in-depth studies are required to provide insight into the specific bacterial groups associated with the prevalent functional pathways and their role in the biogeochemical cycles in Kuwaiti waters. Overall, it should be noted that the PICRUSt analysis represents natural environmental 16S rRNA sequence data, where the predicted functions reflect environmental heterogeneity and ecosystem functioning (Staley et al., 2014; Yang et al., 2018). However, we are aware of the fact that the PICRUSt results could be skewed depending on primer bias, and the identified functions are limited by the availability of complete sequence genomes in the database. Nevertheless, the PICRUSt results highlight the bacterioplankton functional potential of an ecosystem, which should be verified with gene expression studies to link the functional genes of uncultured organisms to taxonomic identity.
In conclusion, herein we investigated for the first time the spatial, temporal, and functional potential of bacterioplankton communities in Kuwaiti waters. Our results identified a core bacterioplankton community and a significant seasonal shift in the bacterioplankton community structure. We also found that temperature, nutrient levels, and TPH were the major environmental drivers of the bacterial community structure. Our results also highlight the importance of niche specialization and resource partitioning in the surrounding habitat in stimulating the presence and prevalence of distinct phylotypes in local waters.
The absence of a major spatial distinction between Kuwait Bay and the offshore sites in the seasonal samples reflects the similarity of site habitats and suggests the dispersal of the bacterioplankton communities in local waters, which could be related to the prevalent anticlockwise circulation pattern in Kuwait waters. The movement of water masses, water mixing, and passive dispersal may promote the ubiquitous distribution of similar bacterioplankton taxa between sites. Furthermore, functional predictions indicated relatively stable seasonal functional profiles that maintained the functional consistency of the Kuwaiti water ecosystem. Overall, these baseline data provide the foundation for future microbial observatories and highlight the need for interannual studies to identify recurrent microbial community patterns and functional responses to eutrophication.
Data Availability Statement
The datasets presented in this study can be found in online repositories. The names of the repository/repositories and accession number(s) can be found below: https://www.ncbi.nlm.nih.gov/, PRJNA783270.
Author Contributions
All authors listed have made a substantial, direct, and intellectual contribution to the work, and approved it for publication.
Conflict of Interest
The authors declare that the research was conducted in the absence of any commercial or financial relationships that could be construed as a potential conflict of interest.
Publisher’s Note
All claims expressed in this article are solely those of the authors and do not necessarily represent those of their affiliated organizations, or those of the publisher, the editors and the reviewers. Any product that may be evaluated in this article, or claim that may be made by its manufacturer, is not guaranteed or endorsed by the publisher.
Acknowledgments
This study was supported by the college of graduate studies, Kuwait University. The authors would like to thank the Research Sector Projects Unit (RSPU), the National Unit for Environmental Research and Services (NUERS) and the Biotechnology Center for the support provided through projects GS02/01, SRUL01/13, GS 01/02 and GS02/11. The authors also appreciate the excellent assistance of the technical staff at RSPU, NUERS and the Biotechnology Center.
Supplementary Material
The Supplementary Material for this article can be found online at: https://www.frontiersin.org/articles/10.3389/fmars.2022.838101/full#supplementary-material
References
Al-Abdulghani E., El-Sammak A., Al-Sarawi M. (2013). Environmental Assessment of Kuwait Bay: An Integrated Approach. J. Coast. Conserv. 17, 445–462. doi: 10.1007/s11852-013-0242-7
Al-Awadhi H., Al-Mailem D., Dashti N., Khanafer M., Radwan S. S. (2012). Indigenous Hydrocarbon-Utilizing Bacterioflora in Oil-Polluted Habitats in Kuwait, Two Decades After the Greatest Manmade Oil Spill. Arch. Microbiol. 194, 689–705. doi: 10.1007/s00203-012-0800-7
Al-Awadhi H., Sulaiman R. H., Mahmoud H. M., Radwan S. S. (2007). Alkaliphilic and Halophilic Hydrocarbon-Utilizing Bacteria From Kuwaiti Coasts of the Arabian Gulf. Appl. Microbiol. Biotech. 77 (1), 183–186. doi: 10.1007/s00253-007-1127-1
Al-Bader D., Rayan R., Mahmood H., Al-Hasan R., Bobby L., Eliyas M. (2011). Comparison of the Seasonal Diversity of Planktonic Cyanobacteria in Clear and Turbid Shallow Coastal Waters of Kuwait Using Culture-Independent Techniques. Kuwait J. Sci. Eng. 38, 163–183.
Aldunate M., de la Iglesia R., Bertagnolli A., Ulloa O. (2018). Oxygen Modulates Bacterial Community Composition in the Coastal Upwelling Waters Off Central Chile. Deep Sea Res. Part II: Top. Stud. Oceanogr. 156, 68–79. doi: 10.1016/j.dsr2.2018.02.001
Al-Ghadban A. N., Al-Majed N., Al-Muzaini S. (2002). The State of Marine Pollution in Kuwait: Northern Arabian Gulf. Technology 8, 7–26.
Al-Hasan R. H., Khanafer M., Eliyas M., Radwan S. S. (2001). Hydrocarbon Accumulation by Picocyanobacteria From the Arabian Gulf. J. Appl. Microbiol. 91, 533–540. doi: 10.1046/j.1365-2672.2001.01414.x
Al-Mailem D. M., Eliyas M., Radwan S. S. (2013). Oil-Bioremediation Potential of Two Hydrocarbonoclastic, Diazotrophic Marinobacter Strains From Hypersaline Areas Along the Arabian Gulf Coasts. Extremophiles 17, 463–470. doi: 10.1007/s00792-013-0530-z
Al-Mailem D. M., Sorkhoh N. A., Al-Awadhi H. H., Eliyas M., Radwan S. S. (2010). Biodegradation of Crude Oil and Pure Hydrocarbons by Extreme Halophilic Archaea From Hypersaline Coasts of the Arabian Gulf. Extremophiles 14, 321–328. doi: 10.1007/s00792-010-0312-9
Almutairi A. (2015). Spatial–temporal Variations and Diversity of the Bacterioplankton Communities in the Coastal Waters of Kuwait. Mar. Pollut. Bull. 100, 699–709. doi: 10.1016/j.marpolbul.2015.09.016
Al-Said T., Naqvi S. W. A., Ahmed A., Madhusoodhanan R., Fernandes L., Kedila R., et al. (2019). Heterotrophic Consumption May Mask Increasing Primary Production Fuelled by Anthropogenic Nutrient Loading in the Northern Arabian/Persian Gulf. Mar. Pollut. Bull. 148, 30–46. doi: 10.1016/j.marpolbul.2019.07.054
Al-Sarawi H. A., Jha A. N., Al-Sarawi M. A., Lyons B. P. (2015). Historic and Contemporary Contamination in the Marine Environment of Kuwait: An Overview. Mar. Pollut. Bull. 100 (2), 621–628. doi: 10.1016/j.marpolbul.2015.07.052
Al-Yamani F., Bishop J., Ramadhan E., Al-Husaini M., Al-Ghadban A. (2004). Oceanographic Atlas of Kuwait’s Waters (Kuwait: Kuwait Institute for Scientific Research).
Al-Yamani F., Naqvi S. W. A. (2019). Chemical Oceanography of the Arabian Gulf. Deep-Sea Res. II 161, 72–80. doi: 10.1016/j.dsr2.2018.10.003
Al-Yamani F., Saburova M., Polikarpov I. (2012). A Preliminary Assessment of Harmful Algal Blooms in Kuwait’s Marine Environment. Aquat. Ecosyst. Health Manage. 15 (sup1), 64–72. doi: 10.1080/14634988.2012.679450
Amann R. I., Ludwig W., Schleifer K. H. (1995). Phylogenetic Identification and In Situ Detection of Individual Microbial Cells Without Cultivation. Microbiol. Rev. 59 (1), 143–169. doi: 10.1128/mr.59.1.143-169.1995
Andersson A., Riemann L., Bertilsson S. (2010). Pyrosequencing Reveals Contrasting Seasonal Dynamics of Taxa Within Baltic Sea Bacterioplankton Communities. ISME J. 4, 171–181. doi: 10.1038/ismej.2009.108
Andreotti R., Perez, de Leon A. A., Dowd S. E., Guerrero F. D., Bendele K. G., et al. (2011). Assessment of Bacterial Diversity in the Cattle Tick Rhipicephalus (Boophilus) Microplus Through Tag-Encoded Pyrosequencing. BMC Microbiol. 11, 6. doi: 10.1186/1471-2180-11-6
Arora-Williams K., Olesen S. W., Scandella B. P., Delwiche K., Spencer S. J., Myers E. M., et al. (2018). Dynamics of Microbial Populations Mediating Biogeochemical Cycling in a Freshwater Lake. Microbiome 6 (1), 165. doi: 10.1186/s40168-018-0556-7
Azam F., Fenchel T., Field J. G., Gray J. S., Meyer-Reil L. A., Thingstad F. (1983). The Ecological Role of Water - Column Microbes in the Sea. Mar. Ecol. Prog. Ser. 10, 257–263. doi: 10.3354/meps010257
Azam F., Worden A. Z. (2004). Microbes, Molecules, and Marine Ecosystems. Science 303 (5664), 1622–1624. doi: 10.1126/science.1093892
Bertrand E. M., McCrow J. P., Moustafa A., Zheng H., McQuaid J. B., Delmont T. O., et al. (2015). Phytoplankton-Bacterial Interactions Mediate Micronutrient Colimitation at the Coastal Antarctic Sea Ice Edge. Pro. Natl. Acad. Sci. U.S.A. 112 (32), 9938–9943. doi: 10.1073/pnas.1501615112
Bolyen E., Rideout J. R., Dillon M. R., Bokulich N. A., Abnet C. C., Abnet C. C., et al. (2019). Reproducible, Interactive, Scalable and Extensible Microbiome Data Science Using QIIME 2. Nat. Biotech. 37, 852–857. doi: 10.1038/s41587-019-0209-9
Bouvier T. C., Del Giorgio P. A. (2002). Compositional Changes in Free-Living Bacterial Communities Along a Salinity Gradient in Two Temperate Studies. Limno. Oceano. 47, 453–470. doi: 10.4319/lo.2002.47.2.0453
Brown M. V., Lauro F. M., DeMaere M. Z., Muir L., Wilkins D., Thomas T., et al. (2012). Global Biogeography of SAR11 Marine Bacteria. Mol. Sys. Biol. 8, 595. doi: 10.1038/msb.2012.28
Bryson S., Li Z., Chavez F., Weber P. K., Pett-Ridge J., Hettich R. L., et al. (2017). Phylogenetically Conserved Resource Partitioning in the Coastal Microbial Loop. ISME J. 11 (12), 2781–2792. doi: 10.1038/ismej.2017.128
Bunse C., Pinhassi J. (2017). Marine Bacterioplankton Seasonal Succession Dynamics. Trends. Microbiol. 25, 494–505. doi: 10.1016/j.tim.2016.12.013
Cabral L., Júnior G. V. L., Pereira de Sousa S. T., Dias A. C. F., Lira Cadete L., Andreote F. D., et al. (2016). Anthropogenic Impact on Mangrove Sediments Triggers Differential Responses in the Heavy Metals and Antibiotic Resistomes of Microbial Communities. Environ. Pollut. 216, 460–469. doi: 10.1016/j.envpol.2016.05.078
Campbell A. M., Fleisher J., Sinigalliano C., White J. R., Lopez J. V. (2015). Dynamics of Marine Bacterial Community Diversity of the Coastal Waters of the Reefs, Inlets, and Wastewater Outfalls of Southeast Florida. Microbiol. Open 4 (3), 390–408. doi: 10.1002/mbo3.245
Campbell B. J., Yu L., Heidelberg J. F., Kirchman D. L. (2011). Activity of Abundant and Rare Bacteria in a Coastal Ocean. Proc. Natl. Acad. Sci. U.S. A. 108, 12776–12781. doi: 10.1073/pnas.1101405108
Chafee M., Fernàndez-Guerra A., Buttigieg P. L., Gerdts G., Eren A. M., Teeling H., et al. (2018). Recurrent Patterns of Microdiversity in a Temperate Coastal Marine Environment. ISME J. 12, 237–252. doi: 10.1038/ismej.2017.165
Chow C. E. T., Sachdeva R., Cram J. A., Steele J. A., Needham D. M., Patel A., et al. (2013). Temporal Variability and Coherence of Euphotic Zone Bacterial Communities Over a Decade in the Southern California Bight. ISME J. 7, 2259–2273. doi: 10.1038/ismej.2013.122
Church M. J. (2009). “Resource Control of Bacterial Dynamics in the Sea,” in Microbial Ecology of the Oceans. Ed. Kirchman D. L. (NJ, USA: Wiley & Sons Inc).
Cottrell M. T., Kirchman D. L. (2000). Community Composition of Marine Bacterioplankton Determined by 16S rRNA Gene Clone Libraries and Fluorescence In Situ Hybridization. Appl. Environ. Microbiol. 66 (12), 5116–5122. doi: 10.1128/aem.66.12.5116-5122.2000
Coulon F., McKew B. A., Osborn A. M., McGenity T. J., Timmis K. N. (2007). Effects of Temperature and Biostimulation on Oil-Degrading Microbial Communities in Temperate Estuarine Waters. Environ. Microbiol. 9, 177–186. doi: 10.1111/j.1462-2920.2006.01126.x
Cram J. A., Chow C. E. T., Sachdeva R., Needham D. M., Parada A. E., Steele J. A., et al. (2015). Seasonal and Interannual Variability of the Marine Bacterioplankton Community Throughout the Water Column Over Ten Years. ISME J. 9, 563–580. doi: 10.1038/ismej.2014.153
Dai W., Zhang J., Tu Q., Deng Y., Qiu Q., Xiong J. (2017). Bacterioplankton Assembly and Interspecies Interaction Indicating Increasing Coastal Eutrophication. Chemosphere 177, 317–325. doi: 10.1016/j.chemosphere.2017.03.034
Davidson A. L., Chen J. (2004). ATP-Binding Cassette Transporters in Bacteria. Annu. Rev. Biochem. 73, 241–268. doi: 10.1146/annurev.biochem.73.011303.073626
DeLong E. F., Preston C. M., Mincer T., Rich V., Hallam S. J., Frigaard N. U., et al. (2006). Community Genomics Among Stratified Microbial Assemblages in the Ocean’s Interior. Science 311, 496–503. doi: 10.1126/science.1120250
Delpech L. M., Vonnahme T. R., McGovern M., Gradinger R., Præbel K., Poste A. E. (2021). Terrestrial Inputs Shape Coastal Bacterial and Archaeal Communities in a High Arctic Fjord (Isfjorden, Svalbard). Front. Microbiol. 12. doi: 10.3389/fmicb.2021.614634
Devlin M. J., Massoud M. S., Hamid S. A., Al-Zaidan A., Al-Sarawi H., Al-Enezi M., et al. (2015). Changes in the Water Quality Conditions of Kuwait's Marine Waters: Long Term Impacts of Nutrient Enrichment. Mar. Pollut. Bull. 100, 607–620. doi: 10.1016/j.marpolbul.2015.10.022
Ferrera I., Gasol J. M., Sebastián M., Hojerová E., Koblížek M. (2011). Comparison of Growth Rates of Aerobic Anoxygenic Phototrophic Bacteria and Other Bacterioplankton Groups in Coastal Mediterranean Waters. Appl. Environ. Microbiol. 77 (21), 7451–7458. doi: 10.1128/AEM.00208-11
Field C. B., Behrenfeld M. J., Randerson J. T., Falkowski P. (1998). Primary Production of the Biosphere: Integrating Terrestrial and Oceanic Components. Science 281 (5374), 237–240. doi: 10.1126/science.281.5374.237
Flombaum P., Gallegos J. L., Gordillo R. A., Rincón J., Zabala L. L., Jiao N., et al. (2013). Present and Future Global Distributions of the Marine Cyanobacteria Prochlorococcus and Synechococcus. Proc. Natl. Acad. Sci. U.S.A. 110, 9824–9829. doi: 10.1073/pnas.1307701110
Fodelianakis S., Papageorgiou N., Pitta P., Kasapidis P., Karakassis I., Ladoukakis E. D., et al. (2014). The Pattern of Change in the Abundances of Specific Bacterioplankton Groups is Consistent Across Different Nutrient-Enriched Habitats in Crete. Appl. Environ. Microbiol. 80, 3784–3792. doi: 10.1128/AEM.00088-14
Fuhrman J. A., Cram J. A., Needham D. M. (2015). Marine Microbial Community Dynamics and Their Ecological Interpretation. Nat. Rev. Microbiol. 13 (3), 133–146. doi: 10.1038/nrmicro3417
Fuhrman J. A., Hewson I., Schwalbach M. S., Steele J. A., Brown M. V., Naeem S. (2006). Annually Reoccurring Bacterial Communities are Predictable From Ocean Conditions. Proc. Natl. Acad. Sci. U.S.A. 103, 13104–13109. doi: 10.1073/pnas.0602399103
Gifford S. M., Sharma S., Moran M. A. (2014). Linking Activity and Function to Ecosystem Dynamics in a Coastal Bacterioplankton Community. Front. Microbiol. 5, 185. doi: 10.3389/fmicb.2014.00185
Gilbert J. A., Field D., Swift P., Newbold L., Oliver A., Smyth T. E. (2009). The Seasonal Structure of Microbial Communities in the Western English Channel. Environ. Microbiol. 11, 3132–3139. doi: 10.1111/j.1462-2920.2009.02017.x
Gilbert J. A., Steele J. A., Caporaso J. G., Steinbrück L., Reeder J., Temperton B., et al. (2012). Defining Seasonal Marine Microbial Community Dynamics. ISME J. 6, 298–308. doi: 10.1038/ismej.2011.107
Giovannoni S. J., Rappé M. S. (2000). “Evolution, Diversity, and Molecular Ecology of Marine Prokaryotes,” in Microbial Ecology of the Oceans. Ed. Kirchman D. L. (New York, N.Y: Wiley & Sons, Inc.), 47–84.
Gutierrez T., Rhodes G., Mishamandani S., Berry D., Whitman W. B., Nichols P. D., et al. (2014). Polycyclic Aromatic Hydrocarbon Degradation of Phytoplankton-Associated Arenibacter Spp. And Description of Arenibacter Algicola Sp. Nov., an Aromatic Hydrocarbon-Degrading Bacterium. Appl. Environ. Microbiol. 80, 618–628. doi: 10.1128/AEM.03104-13
Hawley A. K., Brewer H. M., Norbeck A. D., Paša-Tolić L., Hallam S. J. (2014). Metaproteomics Reveals Differential Modes of Metabolic Coupling Among Ubiquitous Oxygen Minimum Zone Microbes. PNAS 111, 11395–11400. doi: 10.1073/pnas.1322132111
Hazen T. C., Prince R. C., Mahmoudi N. (2016). Marine Oil Biodegradation. Environ. Sci. Technol. 50, 2121–2129. doi: 10.1021/acs.est.5b03333
Heinrich F., Eiler A., Bertilsson S. (2013). Seasonality and Environmental Control of Freshwater SAR11 (LD12) in a Temperate Lake (Lake Erken, Sweden). Aquat. Microb. Ecol. 70, 33–44. doi: 10.3354/ame01637
Herlemann D., Labrenz M., Jürgens K. (2011). Transitions in Bacterial Communities Along 257 the 2000 Km Salinity Gradient of the Baltic Sea. ISME J. 5, 1571–1579. doi: 10.1038/ismej.2011.41
Jeffries T. C., Schmitz Fontes M. L., Harrison D. P., Van-Dongen-Vogels V., Eyre B. D., Ralph P. J., et al. (2016). Bacterioplankton Dynamics Within a Large Anthropogenically Impacted Urban Estuary. Front. Microbiol. 6. doi: 10.3389/fmicb.2015.01438
Jiao N. Z., Zheng Q. (2011). The Microbial Carbon Pump: From Genes to Ecosystems. Appl. Environ. Microbiol. 77, 7439–7444. doi: 10.1128/AEM.05640-11
Jones S. E., Newton R. J., McMahon K. D. (2009). Evidence for Structuring of Bacterial Community Composition by Organic Carbon Source in Temperate Lakes. Environ. Microbiol. 11 (9), 2463–2472. doi: 10.1111/j.1462-2920.2009.01977.x
Kirchman D., Cottrel M., DiTullio G. (2017). Shaping of Bacterial Community Composition and Diversity by Phytoplankton and Salinity in the Delaware Estuary, USA. Aqu. Microb. Ecol. 78 (2), 93–106. doi: 10.3354/ame01805
Kirchman D. L., Cottrell M. T., Lovejoy C. (2010). The Structure of Bacterial Communities in the Western Arctic Ocean as Revealed by Pyrosequencing of 16S rRNA Genes. Environ. Microbiol. 12, 1132–1143. doi: 10.1111/j.1462-2920.2010.02154.x
Kirchman D. L., Morán X. A. G., Ducklow H. (2009). Microbial Growth in the Polar Oceans—Role of Temperature and Potential Impact of Climate Change. Nat. Rev. Microbiol. 7, 451–459. doi: 10.1038/nrmicro2115
Kopprio G. A., Luyen N. D., Duc T. M., Ha T. H., Gärdes A. (2021). Carrageenophyte-Attached and Planktonic Bacterial Communities in Two Distinct Bays of Vietnam: Eutrophication Indicators and Insights on Ice-Ice Disease. Ecol. Indic. 121, 107067. doi: 10.1016/j.ecolind.2020.107067
Kwon K. K., Lee H. S., Jung H. B., Kang J. H., Kim S. J. (2006). Yeosuana Aromativorans Gen. Nov., Sp. Nov., a Mesophilic Marine Bacterium Belonging to the Family Flavobacteriaceae, Isolated From Estuarine Sediment of the South Sea, Korea. Int. J. Syst. Evol. Microbiol. 56, 727–732. doi: 10.1099/ijs.0.64073-0
Lami R., Cottrell M. T., Campbell B. J., Kirchman D. L. (2009). Light-Dependent Growth and Proteorhodopsin Expression by Flavobacteria and SAR11 in Experiments With Delaware Coastal Waters. Environ. Microbiol. 11 (12), 3201–3209. doi: 10.1111/j.1462-2920.2009.02028.x
Lane D. J. (1991). “16s/23s rRNA Sequencing,” in Nucleic Acid Techniques in Bacterial Systematics. Eds. Stackebrandt E., Goodfellow M. (Chichester: John Wiley and Sons), pp 115–pp 175.
Langille M. G. I., Zaneveld J., Caporaso J. G., McDonald D., Knights D., Reyes J. A., et al. (2013). Predictive Functional Profiling of Microbial Communities Using 16S rRNA Marker Gene Sequences. Nat. Biotechnol. 31, 814–821. doi: 10.1038/nbt.2676
Lefort T., Gasol J. M. (2013). Global-Scale Distributions of Marine Surface Bacterioplankton Groups Along Gradients of Salinity, Temperature, and Chlorophyll: A Meta-Analysis of Fluorescence In Situ Hybridization Studies. Aquat. Microb. Ecol. 70, 111–130. doi: 10.3354/ame01643
Liu J., Fu B., Yang H., Zhao M., He B., Zhang X. H. (2015). Phylogenetic Shifts of Bacterioplankton Community Composition Along the Pearl Estuary: The Potential Impact of Hypoxia and Nutrients. Front. Microbiol. 6. doi: 10.3389/fmicb.2015.00064
Logares R., Brate J., Heinrich F., Shalchian-Tabrizi K., Bertilsson S. (2010). Infrequent Transitions Between Saline and Fresh Waters in One of the Most Abundant Microbial Lineages (SAR11). Mol. Biol. Evol. 27 (2), 347–357. doi: 10.1093/molbev/msp239
Lomnitz U., Sommer S., Dale A. W., Löscher C. R., Noffke A., Wallmann K., et al. (2016). Benthic Phosphorus Cycling in the Peruvian Oxygen Minimum Zone. Biogeosciences; 13, 1367–1386. doi: 10.5194/bg-13-1367-2016
Lyons B. P., Devlin M. J., Hamid S. A. A., Al-Otiabi A. F., Al-Enezi M., Massoud M. S., et al. (2015). Microbial Water Quality and Sedimentary Faecal Sterols as Markers of Sewage Contamination in Kuwait. Mar. Pollut. Bull. 100, 689–698. doi: 10.1016/j.marpolbul.2015.07.043
Mahmoud H., Al-Hasan R., Khanafer M., Radwan S. (2010). A Microbiological Study of the Self-Cleaning Potential of Oily Arabian Gulf Coasts. Enviro. Sci. Pollu. Res. 17 (2), 383–391. doi: 10.1007/s11356-009-0099-3
Mahmoud H. M., Al-Hasan R. H., Sorkhoh N. A., Eliyas M., Radwan S. S. (2009). Attenuation of Oil Pollutants in the Arabian Gulf Water by Bacteria Naturally Associated With Live Fish. Int. Biodeterior. Biodegrad. 63, 615–620. doi: 10.1016/j.ibiod.2009.04.005
Mason O., Hazen T., Borglin S., Chain P., Dubinsky E., Fortney J. L., et al. (2012). Metagenome, Metatranscriptome and Single-Cell Sequencing Reveal Microbial Response to Deepwater Horizon Oil Spill. ISME J. 6 (9), 1715–1727. doi: 10.1038/ismej.2012.59
Mattes T. E., Nunn B. L., Marshall K. T., Proskurowski G., Kelley D. S., Kawka O. E., et al. (2013). Sulfur Oxidizers Dominate Carbon Fixation at a Biogeochemical Hot Spot in the Dark Ocean. ISME J. 7 (12), 2349–2360. doi: 10.1038/ismej.2013.113
Morris R. M., Rappé M. S., Connon S. A., Vergin K. L., Siebold W. A., Carlson C. A., et al. (2002). SAR11 Clade Dominates Ocean Surface Bacterioplankton Communities. Nature 420 (6917), 806–810. doi: 10.1038/nature01240
Nasrallah H. A., Nieplova E., Ramadan E. (2004). Warm Season Extreme Temperature Events in Kuwait. J. Arid. Environ. 56, 357–371. doi: 10.1016/S0140-1963(03)00007-7
Nicolaus E. E. M., Wright S. R., Barry J., Bolam T. P. C., Ghareeb K., Ghaloom M., et al. (2017). Spatial and Temporal Analysis of the Risks Posed by Total Petroleum Hydrocarbon and Trace Element Contaminants in Coastal Waters of Kuwait. Mar. Pollut. Bull. 120, 422–427. doi: 10.1016/j.marpolbul.2017.04.031
Palenik B., Brahamsha B., Larimer F. W., Land M., Hauser L., Chain P., et al. (2003). The Genome of a Motile Marine Synechococcus. Nature, 424, 1037–1042. doi: 10.1038/nature01943
Parks D. H., Tyson G. W., Hugenholtz P., Beiko R. G. (2014). STAMP: Statistical Analysis of Taxonomic and Functional Profiles. Bioinformatics 30, 3123–3124. doi: 10.1093/bioinformatics/btu494
Partensky F., Hess W. R., Vaulot D. (1999). Prochlorococcus, a Marine Photosynthetic Prokaryote of Global Significance. Microbiol. Mol. Biol. Rev. 63 (1), 106–127. doi: 10.1128/MMBR.63.1.106-127
Perryman S. E., Rees G. N., Walsh C. J., Grace M. R. (2011). Urban Storm Water Run Off Drives Denitrifying Community Composition Through Changes in Sediment Texture and Carbon Content. Microb. Ecol. 61, 932–940. doi: 10.1007/s00248-011-9833-8
Pinhassi J., Berman T. (2003). Differential Growth Response of Colony Forming Alpha- and Gamma-Proteobacteria in Dilution Culture and Nutrient Addition Experiments From Lake Kinneret (Israel), the Eastern Mediterranean Sea, and the Gulf of Eilat. Appl. Environ. Microbiol. 69, 199–211. doi: 10.1128/AEM.69.1.199-211.2003
Polikarpov I., Saburova M., Al-Yamani F. (2020). Decadal Changes in Diversity and Occurrence of Microalgal Blooms in the NW Arabian/Persian Gulf. Deep-Sea Res. Ii. Top. Stud. Oceanogr. 179, 104810. doi: 10.1016/j.dsr2.2020.104810
Pommier T., Canback B., Riemann L., Bostrom K. H., Simu K., Lundberg P., et al. (2007). Global Patterns of Diversity and Community Structure in Marine Bacterioplankton. Mol. Ecol. 16, 867–880. doi: 10.1111/j.1365-294X.2006.03189.x
Poretsky R. S., Sun S., Mou X., Moran M. A. (2010). Transporter Genes Expressed by Coastal Bacterioplankton in Response to Dissolved Organic Carbon. Environ. Microbiol. 12, 616–627. doi: 10.1111/j.1462-2920.2009.02102.x
Quero G. M., Luna G. M. (2014). Diversity of Rare and Abundant Bacteria in Surface Waters of the Southern Adriatic Sea. Mar. Genomics 17, 9–15. doi: 10.1016/j.margen.2014.04.002
Radwan S. S., Al-Hasan R. H., Ali N., Salamah S., Khanafer M. (2005). Oil-Consuming Microbial Consortia Floating in the Arabian Gulf. Int. Biodeter. Biodegrad. 56, 28–33. doi: 10.1016/j.ibiod.2005.03.007
Rappé M. S., Giovannoni S. J. (2003). The Uncultured Microbial Majority. Annu. Rev. Microbiol. 57, 369–394. doi: 10.1146/annurev.micro.57.030502.090759
Rappé M. S., Vergin K., Giovannoni S. J. (2000). Phylogenetic Comparisons of a Coastal Bacterioplankton Community With Its Counterparts in Open Ocean and Freshwater Systems. FEMS Microbiol. Ecol. 33, 219–232. doi: 10.1111/j.1574-6941.2000.tb00744.x
Rezaei Somee M., Dastgheib S. M. M., Shavandi M., Ghanbari Mamam L., Amoozegar M. A., Mershas M. (2021). Distinct Microbial Community Along the Chronic Oil Pollution Continuum of the Persian Gulf Converge With Oil Spill Accidents. Sci. Rep. 11, 11316. doi: 10.1038/s41598-021-90735-0
Richa K., Balestra C., Piredda R., Benes V., Borra M., Passarelli A., et al. (2017). Distribution, Community Composition, and Potential Metabolic Activity of Bacterioplankton in an Urbanized Mediterranean Sea Coastal Zone. Appl. Environ. Microbiol. 83 (17), 1– 17. doi: 10.1128/AEM.00494-17
Rusch D. B., Halpern A. L., Sutton G., Heidelberg K. B., Williamson S., Yooseph S., et al. (2007). The Sorcerer II Global Ocean Sampling Expedition: Northwest Atlantic Through Eastern Tropical Pacific. PloS Biol. 5, 398–431. doi: 10.1371/journal.pbio.0050077
Salazar G., Cornejo-Castillo F. M., Benítez-Barrios V., Nuez E. F., Álvarez-Salgado X. A., Duarte C. M., et al. (2015). Global Diversity and Biogeography of Deep-Sea Pelagic Prokaryotes. ISME J. 10, 596–608. doi: 10.1038/ismej.2015.137
Salter I., Galand P. E., Fagervold S. K., Lebaron P., Obernosterer I., Oliver M. J., et al. (2015). Seasonal Dynamics of Active SAR11 Ecotypes in the Oligotrophic Northwest Mediterranean Sea. ISME J. 9, 347–360. doi: 10.1038/ismej.2014.129
Scanlan D. J., Ostrowski M., Mazard S., Dufresne A., Garczarek L., Hess W. R., et al. (2009). Ecological Genomics of Marine Picocyanobacteria. Microbiol. Mol. Biol. Rev. 73, 249 –2299. doi: 10.1128/MMBR.00035-08
Segata N., Izard J., Waldron L., Gevers D., Miropolsky L., Garrett W. S., et al. (2011). Metagenomic Biomarker Discovery and Explanation. Genome Biol. 12, R60. doi: 10.1186/gb-2011-12-6-r60
Shah V., Chang B. X., Morris R. M. (2017). Cultivation of a Chemoautotroph From the SUP05 Clade of Marine Bacteria That Produces Nitrite and Consumes Ammonium. ISME J. 11, 263–271. doi: 10.1038/ismej.2016.87
Shah V., Morris R. M. (2015). Genome Sequence of “Candidatus Thioglobus Autotrophica” Strain EF1, a Chemoautotroph From the SUP05 Clade of Marine Gammaproteobacteria. Genome Announc. 3. doi: 10.1128/genomeA.01156-15
Sheppard C., Al-Husiani M., Al-Jamali F., Al-Yamani F., Baldwin R., Bishop J., et al. (2010). The Gulf: A Young Sea in Decline. Mar. Pollut. Bull. 60, 13–38. doi: 10.1016/j.marpolbul.2009.10.017
Sogin M. L., Morrison H. G., Huber J. A., Mark Welch D., Huse S. M., Neal P. R. (2006). Microbial Diversity in the Deep Sea and the Underexplored ‘Rare Biosphere’. Proc. Natl. Acad. Sci. U.S.A. 103, 12115–12120. doi: 10.1073/pnas.0605127103
Sommaruga R., Casamayor E. O. (2009). Bacterial 'Cosmopolitanism' and Importance of Local Environmental Factors for Community Composition in Remote High-Altitude Lakes. Freshw. Biol. 55 (5), 994–1005. doi: 10.1111/j.1365-2427.2008.02146.x
Sowell S., Wilhelm L., Norbeck A., Lipton M. S., Nicora C. D., Barofsky D. F., et al. (2009). Transport Functions Dominate the SAR11 Metaproteome at Low-Nutrient Extremes in the Sargasso Sea. ISME J. 3, 93–105. doi: 10.1038/ismej.2008.83
Staley C., Gould T. J., Wang P., Phillips J., Cotner J. B., Sadowsky M. J. (2014). Core Functional Traits of Bacterial Communities in the Upper Mississippi River Show Limited Variation in Response to Land Cover. Front. Microbiol. 5, 414. doi: 10.3389/fmicb.2014.00414
Statham P. J. (2012). Nutrients in Estuaries—An Overview and the Potential Impacts of Climate Change. Sci. Tot. Environ. 434, 213–227. doi: 10.1016/j.scitotenv.2011.09.088
Sunagawa S., Coelho L. P., Chaffron S., Kultima J. R., Labadie K., Salazar G., et al. (2015). Structure and Function of the Global Ocean Microbiome. Science 348 (6237), 1261359. doi: 10.1126/science.1261359
Tang K., Jiao N., Liu K., Zhang Y., Li S. (2012). Distribution and Functions of TonB-Dependent Transporters in Marine Bacteria and Environments: Implications for Dissolved Organic Matter Utilization. PloS One 7 (7), e41204. doi: 10.1371/journal.pone.0041204
Teeling H., Fuchs B. M., Bennke C. M., Krüger K., Chafee M., Kappelmann, et al. (2016). Recurring Patterns in Bacterioplankton Dynamics During Coastal Spring Algae Blooms. eLife 5, e11888. doi: 10.7554/eLife.11888
Treusch A. H., Vergin K. L., Finlay L. A., Donatz M. G., Burton R. M., Carlson C. A., et al. (2009). Seasonality and Vertical Structure of Microbial Communities in an Ocean Gyre. ISME J. 3 (10), 1148–1163. doi: 10.1038/ismej.2009.60
Vergin K. L., Beszteri B., Monier A., Cameron T. J., Temperton B., Treusch A. H., et al. (2013). High-Resolution SAR11 Ecotype Dynamics at the Bermuda Atlantic Time-Series Study Site by Phylogenetic Placement of Pyrosequences. ISME J. 7 (7), 1322–1332. doi: 10.1038/ismej.2013.32
Vergin K. L., Urbach E., Stein J. L., DeLong E. F., Lanoil B. D., Giovannoni S. J. (1998). Screening of a Fosmid Library of Marine Environmental Genomic DNA Fragments Reveals Four Clones Related to Members of the Order Planctomycetales. Appl. Environ. Microbiol. 64, 3075–3078. doi: 10.1128/AEM.64.8.3075-3078.1998
Walsh D. A., Zaikova E., Howes C. G., Song Y. C., Wright J. J., Tringe S. G., et al. (2009). Metagenome of a Versatile Chemolithoautotroph From Expanding Oceanic Dead Zones. Science 326 (5952), 578–582. doi: 10.1126/science.1175309
Wang W., Li Z., Zeng L., Shao Z. (2020). The Oxidation of Hydrocarbons by Diverse Heterotrophic and Mixotrophic Bacteria That Inhabit Deep-Sea Hydrothermal Ecosystems. ISME J. 14, 1994–2006. doi: 10.1038/s41396-020-0662-y
Waterbury J. B., Watson S. W., Valois F. W., Franks D. G. (1986). Biological and Ecological Characterization of the Marine Unicellular Cyanobacteria Synechococcus. Can. J. Fish. Aqua. Sci. 214, 71–120.
Wilhelm L. J., Tripp H. J., Givan S. A., Smith D. P., Giovannoni S. J. (2007). Natural Variation in SAR11 Marine Bacterioplankton Genomes Inferred From Metagenomic Data. Biol. Dir. 2, 27. doi: 10.1186/1745-6150-2-27
Wright J. J., Konwar K. M., Hallam S. J. (2012). Microbial Ecology of Expanding Oxygen Minimum Zones. Nat. Rev. Microbiol. 10, 381–394. doi: 10.1038/nrmicro2778
Yang Y., Gao Y., Huang X., Ni P., Wu Y., Deng Y., et al. (2018). Adaptive Shifts of Bacterioplankton Communities in Response to Nitrogen Enrichment in a Highly Polluted River. Environ. Pollut. 245, 290–299. doi: 10.1016/j.envpol.2018.11.002
Zimmer-Faust A. G., Steele J. A., Xiong X., Staley C., Griffith M., Sadowsky M. J., et al. (2021). A Combined Digital PCR and Next Generation DNA-Sequencing Based Approach for Tracking Nearshore Pollutant Dynamics Along the Southwest United States/Mexico Border. Front. Microbiol. 12. doi: 10.3389/fmicb.2021.674214
Keywords: Kuwait marine system, anthropogenic impacts, environmental microbiology, bacterioplankton, Arabian gulf
Citation: Ismail N and Almutairi A (2022) Bacterioplankton Community Profiling of the Surface Waters of Kuwait. Front. Mar. Sci. 9:838101. doi: 10.3389/fmars.2022.838101
Received: 17 December 2021; Accepted: 28 March 2022;
Published: 19 April 2022.
Edited by:
Wael Ahmed Ismail, Arabian Gulf University, BahrainReviewed by:
Patricia M. Valdespino-Castillo, Lawrence Berkeley National Laboratory, United StatesJonathan Douglas Van Hamme, Thompson Rivers University, Canada
Wael Samir El-Sayed, Ain Sham University, Egypt
Copyright © 2022 Ismail and Almutairi. This is an open-access article distributed under the terms of the Creative Commons Attribution License (CC BY). The use, distribution or reproduction in other forums is permitted, provided the original author(s) and the copyright owner(s) are credited and that the original publication in this journal is cited, in accordance with accepted academic practice. No use, distribution or reproduction is permitted which does not comply with these terms.
*Correspondence: Awatef Almutairi, YS5hbG11dGFpcmlAa3UuZWR1Lmt3
†These authors have contributed equally to this work and share first authorship