- 1Department of Chemistry, Ferdowsi University of Mashhad, Mashhad, Iran
- 2SRL-Environmental, LLC, Racine, WI, United States
Microalgae are considered as a promising feedstock for the production of valuable fatty acids. In this study, individual fatty acid profiles during the growth period of Chlorella vulgaris were investigated. The results showed that the quantity and the type of fatty acids changed with varying rates during the growth of microalgal cells. Interchanging the fatty acid profiles may provide some metabolic information as a complementary method to radiolabeling studies. For C. vulgaris, two unsaturated fatty acids, oleic and linoleic acids, were the major components. Constant concentration of palmitic and oleic acids shows that they may serve as precursors for longer-chain fatty acids. Higher concentration of palmitic rather than palmitoleic acid (about 16 to 34 times depending on the cultivation day) shows that palmitic acid production rate is higher than its conversion rate to longer-chain fatty acids. In fact, palmitoleic acid might have been partly converted to oleic and linoleic acids. The fatty acid content variations during the growth period are not linear and can be fitted to a Sigmoidal model with R2 value higher than 0.98 and low RMSD values (except for oleic and palmitic acid).
1 Introduction
Microalgae can be defined as photosynthetic microorganisms with unicellular or multicellular structures (Jalilian et al., 2020). These microorganisms attracted scientific attention due to their high growth rate and biomass productivities, and adaptability in a broad spectrum of climate conditions (Paliwal and Jutur, 2021; Rafa et al., 2021). Moreover, large-scale microalgae cultivation would be more economically feasible with integration of microalgae-based CO2 fixation, wastewater treatment, concurrent processing of high value-added products, and biofuel production (Molazadeh et al., 2019a; Singh et al., 2020). A variety of algal species exist in different ecosystems, both aquatic and terrestrial (Elegbede et al., 2017). In different environmental conditions, microalgae produce a wide range of high-value products such as proteins, lipids, pigments, and carbohydrates (Lari et al., 2016; Ram et al., 2019). Algal lipids are one of the important commercial compounds that can be used in various industries such as biofuels (Borowitzka and Moheimani, 2013), and pharmaceutical and nutritional products (Caporgno and Mathys, 2018; Xue et al., 2020). Regarding the algal biodiversity, their lipid content can reach up to 70% of dry biomass under optimized culture conditions (Paliwal et al., 2017). Furthermore, the lipid profile variation is as diverse as the algal biodiversity. The lipid molecules generally occur in two forms, polar and non-polar. In non-polar lipids, fatty acids (FAs) are attached to an uncharged head group such as glycerol (Xue et al., 2020). It is worth mentioning that some types of neutral lipids such as hydrocarbons, sterols, ketones, and pigments (carotenes and chlorophylls) do not include any FA groups. The polar lipid molecules are formed by bonding the FAs to a charged group. For instance, bonding FAs to the glycerol–phosphate complex produces a polar phospholipid molecule that is a major component of cell membranes (Lordan et al., 2017). The FAs usually range from 12 to 22 carbon atoms in length, which can be either saturated or unsaturated (Kabir et al., 2020). The length of carbon chain determines the FA properties (Lim et al., 2021). Some FAs are pharmacologically important especially omega 3 (O-3) and omega 6 (O-6) series such as eicosapentaenoic acid (EPA), docosahexaenoic acid (DHA), arachidonic acid (AA), and linoleic acid (LA) (Patel et al., 2022). Both O-3 and O-6 polyunsaturated FAs are believed to have several beneficial effects on cardiovascular diseases (Mata et al., 2010). In fact, consumption of O-3 and O-6 can decrease the total concentration of cholesterol in plasma (Hooper et al., 2018; Djuricic and Calder, 2021). Using O-3 and O-6 FAs during pregnancy has beneficial effects on fetal growth specifically for the central nervous system and cognitive function (Shrestha et al., 2020). Furthermore, essential FA deficiency also increases skin inflammations and prolongs wound treatment (Balić et al., 2020; Bilal et al., 2021). There are some FAs with no major medical applications and can be used in biodiesel production. Biodiesel is a methyl or ethyl ester derivative of FAs. The different types of FAs affect the biodiesel quality (Mondal et al., 2021). Some researchers advice algal lipid with high content of oleic and palmitic acids for biodiesel production (Amit and Ghosh, 2018) and some others recommend algal lipid with a mixture of saturated and unsaturated FAs (Talebi et al., 2014).
The types of produced FAs depend on the lipid metabolism of microalgae. Lipid metabolism usually has been studied using radiolabeled carbon sources such as 14CO2 and [14C]acetate (Harris and James, 1965a). The carbon sources can be incorporated into all types of FAs. However, 14CO2 can be incorporated into other components such as sugar and glycerol, which make it difficult to elucidate the metabolism. Acyl-glycerol was derived from de novo synthesis by acylation of glycerol 3-phosphate. Acetyl-CoA carboxylase (ACC) and a type II (dissociable) FA synthetase are two key enzymes involved in de novo fatty-acid synthesis (Nakamura and Li-Beisson, 2016). The final product of de novo synthesis is palmitic and stearic acids (Blasio and Balzano, 2021). Longer-chain FAs (LCFAs) can be produced by elongase enzymes that insert an ethyl group into the carbon chain (Qiu et al., 2020). Desaturase enzyme introduces double bonds in situations depending on the carbon chain length (Howling et al., 1968). Elongation and desaturation of carbon chains can be carried out in more than one way; therefore; the mechanism of the lipid conversion and the metabolism are very diverse and heterogeneous. Analysis of FA profiles during the growth period of algae may be regarded as a complementary method for evaluating the metabolism.
This work reports on the concentration, production rate, and the productivities of individual FAs in order to shed light on the metabolic pathways of the conversion of shorter-chain FAs to longer-chain FAs. Here, we determine the changes of FA profiles of Chlorella vulgaris in 5-day intervals at 5 growth periods. In the current literature, most articles report total FA productivities (Del Río et al., 2017; Kim et al., 2017; Park et al., 2018; Shen et al., 2020; Kim et al., 2021) and fewer articles report on individual FA productivities (Aussant et al., 2018; Ju et al., 2019; Thoisen et al., 2020) and specific rates of individual FA production as searched on Scopus, Web of Science, Science Direct, and Reaxys search engines. The novelty of this work is in the establishment of a correlation between algae biomass growth rate and the rate of individual FAME production. Furthermore individual FA concentrations in 5-day intervals were fitted to two kinetic models, Sigmoidal and Logarithmic, to find the changes in the trend of each FAs.
2 Methods and Materials
2.1 Cultivation of Chlorella vulgaris
C. vulgaris (ATCC ® 30821™) was cultivated in 2-L Erlenmeyer flasks with 1 L of BG11 culture medium (Rippka et al., 1979) mixed by way of air bubbling at the bottom of flask, at 25 ± 2°C, under a light intensity of 35 μmol photon m-2 s-1 with a 16:8 h light:dark photoperiod. The light intensity in some parts of the world is low especially in winter; we decided to evaluate the algae growth using a lower light intensity in order to understand the possibility of algae growth in parts of the world with low sunshine. The BG11 medium was composed of (per liter of distilled water) 1.50 g NaNO3, 20.0 mg Na2CO3, 36.0 mg CaCl2·2H2O, 7.5 mg MgSO4·7H2O, 6.0 mg (NH4)5[Fe (C6H4O7)2] (ferric ammonium citrate), 6.0 mg C3H5O(COOH)3 (citric acid), 1.0 mg EDTA, 40.0 mg K2HPO4.2H2O, and 1 ml of the trace elements stock solution. The trace elements stock solution contained 494.0 mg L−1 Co(NO3)2·6H2O, 79.0 mg L−1 CuSO4·5H2O, 39.0 mg L−1 NaMoO4·2H2O, 222.0 mg L−1 ZnSO4·7H2O, 1.810 g L−1 MnCl2·4H2O, and 2.860 g L−1 H3BO3. Sampling was performed at days 5, 10, 15, 20, and 25 of cultivation. The microalgae biomass was lyophilized (Buchi B-191, Switzerland) to make sure that the accuracy of FAME analysis is high enough.
2.2 Microalgae Growth
Trend for algal growth and biomass production were monitored using dry cell weight. In order to obtain total algal biomass in the culture media, 20.00 ± 0.03 ml of suspension were oven dried at 70°C for 24 h.
2.3 Lipid Extraction
Before extraction, grinding was performed on the lyophilized algal biomass to disrupt the cell walls (Zheng et al., 2011). Microscopic observation using an optical microscope (BH-2, Olympus, Japan) was used to confirm the cell wall disruption. After addition of 2 ml of methanol and 1 ml of hexane to 10.00 ± 0.01 mg of dried biomass, the samples were mixed by vortexing for 1 min. Subsequently, water was added and the suspension was centrifuged at 4,000 rpm for 10 min. The organic phase containing lipids was transferred to pre-weighed vials (Moradi-Kheibari et al., 2017). The procedure was performed in triplicate to achieve a comprehensive extraction. Then, the solvents were evaporated in an oven at 55°C for 24 h and lipid yields were calculated. Drying the lipid under nitrogen stream will cause the residual solvent to remain in the lipid content, and consequently bias the total lipid content. Therefore, to improve the accuracy in total lipid calculation, drying of lipid was performed using oven drying.
2.4 In Situ Transesterification and FAME Analysis
Transesterification reactions were performed by adding 500 μl of 2% v/v H2SO4 in methanol to 5.00 ± 0.01 mg of the lyophilized biomass harvested in 5-day intervals. The change in the production of individual FAME is negligible on a daily basis; therefore, we have performed the FAME analysis every 5 days. To make sure that the accuracy of FAME analysis is high enough, the biomass was lyophilized. Before transesterification, the cell wall disruption was confirmed by microscopic observations. The solution was then shaken at 240 rpm for 4 h at 60°C. The samples were cooled down to room temperature (22 ± 1°C) and 300 μl of hexane was added into the reaction vial and vortexed (XB982, Asda, UK) for 30 s. The phase separation was achieved by the addition of 600 μl of a 1% w/v NaCl and centrifuging the suspension at 3,000 rpm for 5 min (Sorvall RC 5C Plus, Newtown, CT). Finally, the hexane containing FAME was injected onto a GC-FID (Agilent 6890, Santa Clara, USA) instrument for FAME profiling.
An Hp88 capillary column (Agilent, Santa Clara, USA) (100 m, 0.25 mm I.D., film thickness 0.2 μm) was used to achieve reproducible separation. When the 30-m-long capillary column was used, the resolution of the 37-component FAME mixture was not acceptable. The chosen carrier gas was helium at a constant flow rate of 1.5 ml/min with a split ratio of 20:1. The amount of sample injected was 1 μl. The oven temperature program was 140°C for 5 min, rising to 240°C at 4°C/min, then held at 240°C for 5 min. Injector and detector temperatures were 260°C. Applied temperature programing (the inset in Figure 1) and an example chromatogram of FAMEs yielded from C. vulgaris is shown in Figure 1. A mix of 37-component FAME standard, Cat. No. 47885-U (Supelco, Bellefonte, USA) was injected with different dilutions and a calibration curve was constructed. For the algae biomass, after in situ transesterification and extraction of FAME, the GC-FID analysis was performed, and after retention time matching, the peak areas were used to calculate the amount of each FAMEs from the calibration curve.
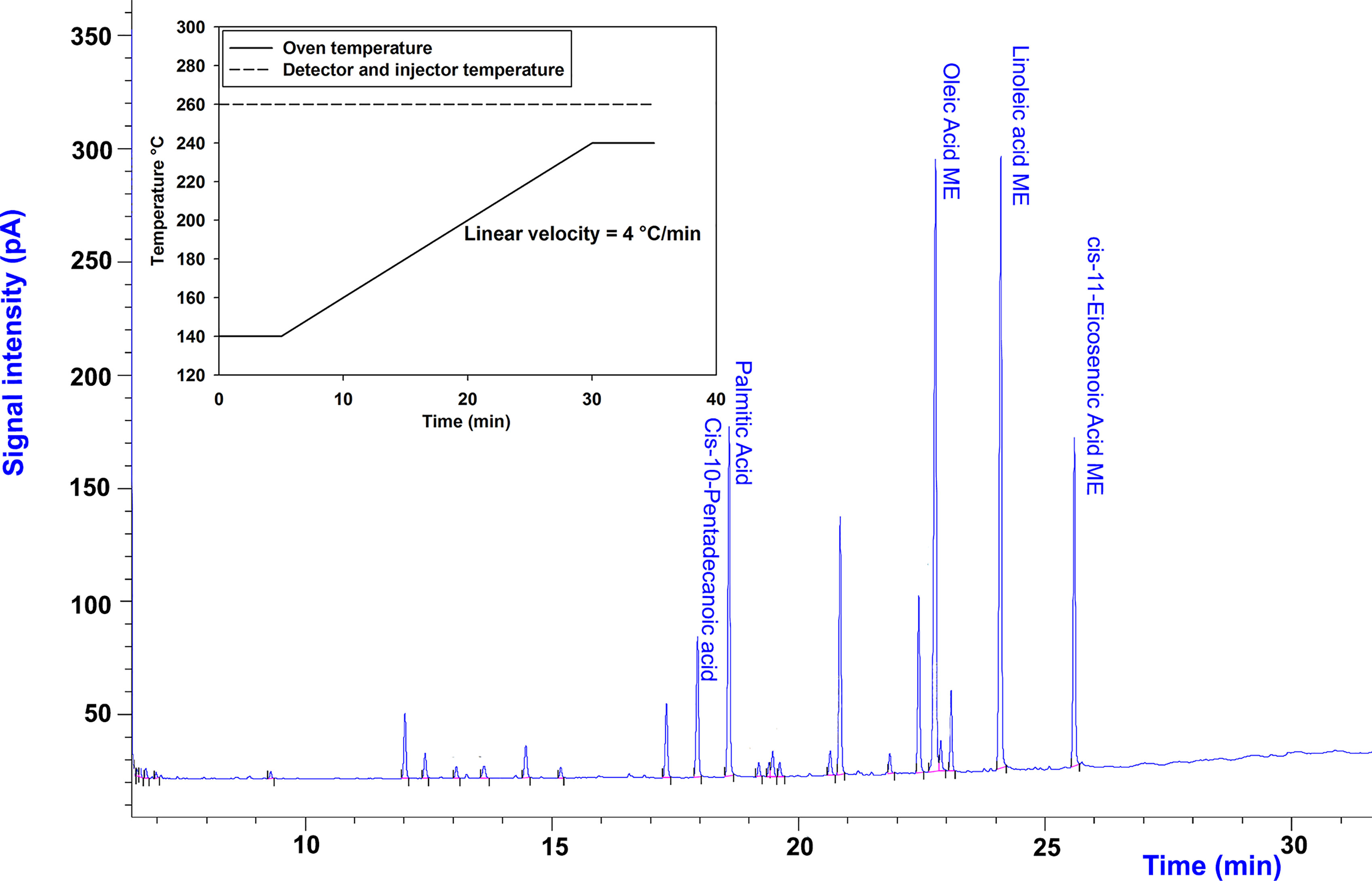
Figure 1 Temperature programing (inset) and GC-FID chromatogram of C. vulgaris on the 25th day of cultivation to obtain the GC-FID profiling of FAs.
2.5 Total Lipid Yield, Productivities, and Specific Rates of Individual FAME Production
Total lipid yield (Y%), productivities (P), and specific production rate of individual FAs (R) were calculated by equations 1, 2, and 3, respectively.
Where N1 and N2 are the concentration of components (g/L) at time t1 (day) and t2 (day), respectively.
2.6 Kinetics of FAME production
Two non-linear mathematical models, namely, Logarithm and Sigmoidal models, were fitted to predict the FAME production by C. vulgaris using Sigmaplot 10.0. The experimental data are referred to the FA contents of C. vulgaris at different days of cultivation. Kinetics is the study of the rate of a process under controlled parameters. There are multiple variables influencing the growth rate of microalgae. Some parameters, such as light intensity, should follow a cycle of on:off, and this will hinder the possibility of algae kinetics study. We sampled microalgae for biomass measurement every fifth day. During these 5-day intervals, the average of 10 on:off cycles of light intensities is considered as a controlled parameter.
In summary, by prolonging the sampling rate, we have been able to have a preliminary assessment of overall kinetics of algae biomass and its correlation with the overall rate of individual FAME production.
The Logarithm model is a two-parameter model whereas the Sigmoidal model is a three-parameter model as shown below:
The parameters of t and C0 in Eq. 4 and a, b, and t0 in Eq. 5 will be determined by the models. The determination of these parameters would propose the kinetics of FAME production.
2.6.1 Statistical Analysis of FAME Production Kinetics
Coefficient of determination (R2), root mean square deviation (RMSD), and graph of experimental data versus predicted values of FAMEs can be used as statistical indicators to evaluate the reliability of the kinetics models.
The R2,, and RMSD can be calculated using the following equations (Lam et al., 2017):
Where n refers to the number of experimental observations, yexp and ycal refer to the FAME concentration yield from experimental data and from calculation, respectively, and refers to the average values of yexp.
3 Results and Discussion
3.1 Biomass and Total Lipid Analysis
During 25 days of batch culture, the biomass concentration, lipid content, and biomass and lipid productivities of C. vulgaris were determined and are depicted in Figure 2. As shown in Figure 2A, biomass concentration reaches 1,166 ± 9 mg L-1 after 25 days, which is approximately equal to the amount reported by Chen et al. (2021).
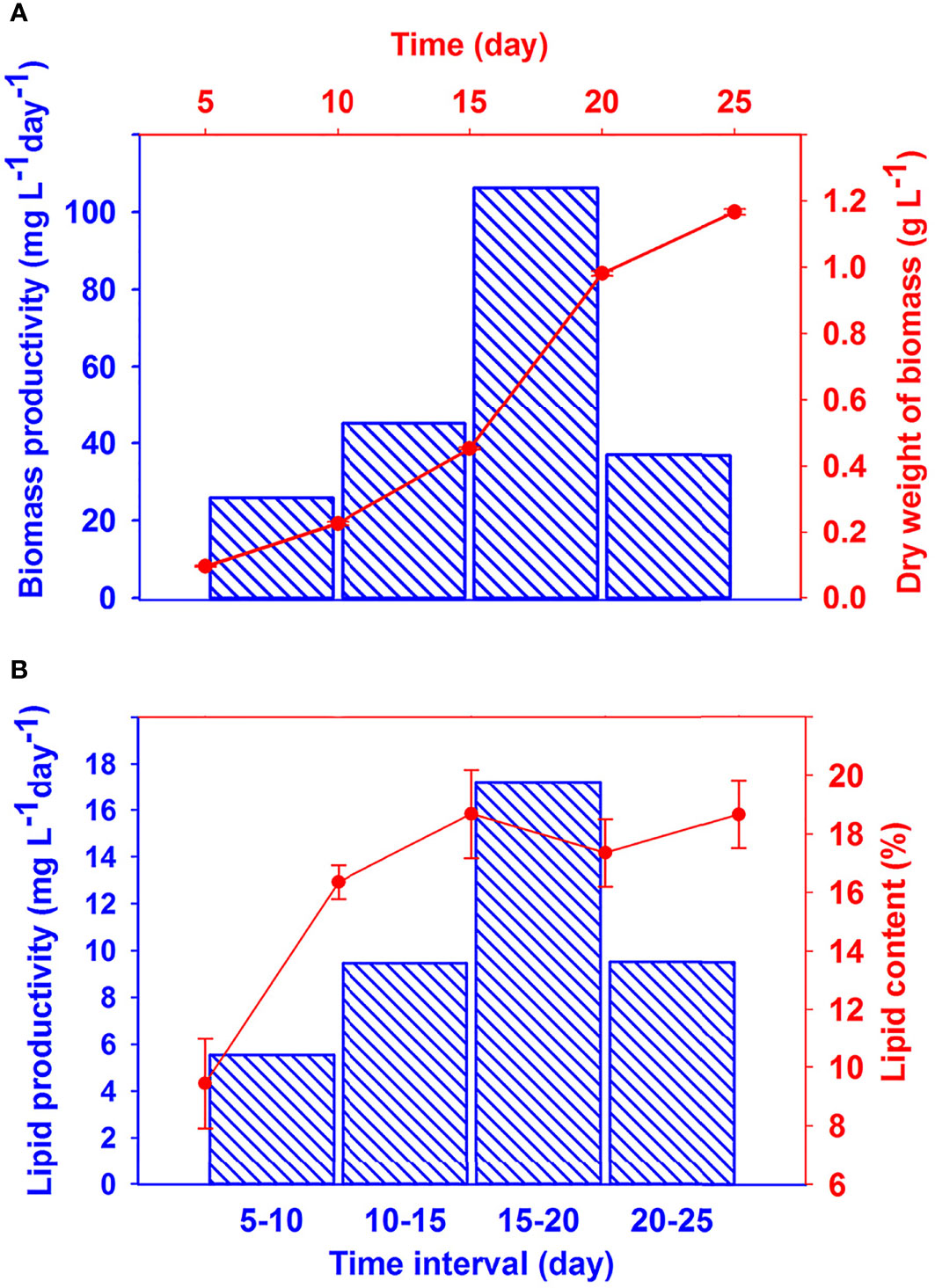
Figure 2 Biomass concentration and biomass productivity (A), and lipid content and lipid productivities (B) of C. vulgaris as a function of time. Top and right axes belong to the scatter plots and bottom and left axes belong to the bar charts.
The productivities were calculated in 5-day intervals to evaluate variations in these intervals. Biomass productivity, related to the growth rate, approached its maximum value at 15- to 20-day intervals (106 mg L−1 day−1). The lipid content after 15 days reached a constant value (19%). The results shown in Figures 2A, B indicate that lipid productivity has similar trend with the biomass productivity. This means that lipid productivity achieves its maximum in the 15- to 20-day interval (1.7 mg L−1 day−1). Lipid exponential growth rate starts from 5 to 10 days. This is usually when the nutrients have been depleted. This observation was reported in our group and elsewhere. For example, Taziki et al. (2015) reported that NO3- ion concentration depleted from 1,000 to 270 mg/L in 6 days. In another report, Molazadeh et al. (2019b) reported that nitrogen and phosphorus were significantly removed by C. vulgaris in 10 days.
High lipid productivity may not necessarily correlate with high lipid content (Griffiths and Harrison, 2009). In fact, both biomass productivity and lipid content should be considered. Although lipid productivity and lipid content are important, the lipid profiles determine the quality of lipid for biodiesel production and for usage on animal feedstock.
3.2 GC-FID Analysis of Fatty Acid Methyl Esters
FA compositions are determined by the analysis of methyl esters of FAs using gas chromatography with a flame ionization detector (GC-FID). The FA profile is a determining factor to incorporate algal lipid quality into biofuels or biotechnological applications. Microalgal lipids usually have FAs with 16 and 18 carbon chain lengths (Pushpakumari Kudahettige et al., 2018). Figure 3 shows 10 important FAME components of C. vulgaris sampled in 5 different 5-day intervals of growth period. These components can be categorized into 3 groups: high (higher than 1,500 mg per 100 g of dried biomass), intermediate (500 to 1,500 mg per 100 g of dried biomass), and low concentration (under 500 mg per 100 g of dried biomass). As depicted in Figure 3, C18:2n6c (linoleic acid) and C18:1n9c (oleic acid) are the dominant FAs with high concentration, which accounted for approximately 50% of total FA. C20:1 (cis-11-eicosenoic acid) and C16:0 (palmitic acid) are categorized as the intermediate concentration FAs and the other FAs fall into the low concentration category. These results are in agreement with the previous study (Hempel et al., 2012). The major saturated, monounsaturated and polyunsaturated FAs were C16:0 (palmitic acid), C18:1n9c (oleic acid), and C18:2n6c (linoleic acid), respectively. Trienoic FAs were not observed in this algae species. Furthermore, we observed no conversion of 16 and 18 carbon precursors to long-chain FAs with higher than 22 carbon chain length. Both total concentrations and the profiles of FAs have changed during the algal growth. Total FAME concentration at 5, 10, 15, 20, and 25 days are 4.58, 6.03, 7.71, 9.39, and 9.62 mg per 100 g of dried biomass, respectively. Although total lipid yield remains constant after the 15th day of cultivation, the total FA concentrations reached a constant value after the 20th day at the expense of consuming shorter-chain FAs to produce longer-chain FAs. Therefore, the best harvesting time to yield more total FAs is the 20th day of cultivation. Analyses of FA profiles in different days are very important but the literature usually has reported on the total lipids (Park et al., 2019; Lakshmikandan et al., 2020; Brindhadevi et al., 2021) or FA profile in 1 day of cultivation (Del Río et al., 2017; Kim et al., 2017; Shen et al., 2020), which does not provide any additional data about the metabolism.
Comparing the composition and the content of individual FAs in different days shows that the rates of change in mg of FAs per 100 g of dried biomass are different for almost all FAs (Figure 3). As shown in Figure 3, with increasing the growth time, long-chain FA content increases as well. Generally speaking, shorter-chain FAs act as a precursor for longer-chain FA synthesis (Harwood and Guschina, 2009; Blasio and Balzano, 2021). C16:0 (palmitic acid) and C18:0 (Stearic acid) are two FAs produced from de novo synthesis. The concentration of palmitic (about 1,000 mg per 100 g dried biomass) is much higher than stearic acid (approximately 40 mg per 100 g of dried biomass). A precursor–product relationship between stearic and oleic acids is demonstrated (Harris and James, 1965a). Low concentration and a decreasing trend of stearic acid corroborate the conversion of stearic to oleic acid. There is no major change in C18:1n9c (oleic acid) and C16:0 (palmitic acid) content while C18:2n6c (linoleic acid) changed from 713 to 2,599 (mg per 100 g of dried biomass). This significant change (364%) could be rationalized by hypothesizing that the shorter-chain FAs such as oleic and palmitic acid are converted to longer-chain FAs such as C20:1 (Guschina and Harwood, 2006; Harwood and Guschina, 2009). Oleic and palmitoleic acid are synthesized by desaturation of stearic acid and palmitic acid at the Δ9-position by desaturase enzyme. Howling et al. (1968) showed that the major conversion to dienoic acids was found with C18 precursor and had double bond particularly in the 9,12 positions to yield oleic and linoleic acids. Due to the aerobic nature of this enzyme, it requires molecular oxygen that is provided by the aeration (Harris and James, 1965b). Palmitoleic acid (C16:1) was in low concentration, which may indicate that it was consumed to produce oleic acid by an elongase enzyme. According to the previous radiolabeling studies (Harris and James, 1965a; Harris and James, 1965b) on C. vulgaris under aerobic condition, oleic acid is directly converted to linoleic acid. In this study, the concentration of linoleic acid increased from 713 to 2,599 mg per 100 g of dried biomass while the concentrations of the palmitic and oleic acid remained constant at approximately 1,000 and 2,000 mg per 100 g of dried biomass, respectively. We did not use organic carbon sources for algal cultivation. Therefore, according to the literature, unsaturated FAs such as oleic and linoleic acids are dominant when no organic carbon sources were used (Harris and James, 1965a). Addition of carbohydrate to the culture media results in elongation of carbon chain rather than desaturation (Harris and James, 1965a). As shown in Figure 3, cis configuration is much more dominant in FA profiles. For instance, oleic acid and linoleic acids reached 22.35% and 26.98% of total FAs on the 25th day of cultivation, i.e., almost 50% of total lipid is made of only these two FAs on day 25 of the growth period. This may be due to the stereospecific nature of desaturation. In fact, FA desaturase introduces a double bond into the FA chain mostly with cis configuration (Nakamura and Li-Beisson, 2016). Cis isomers have lower melting points and viscosities as compared with trans isomers, which is due to the low stacking ability of FA molecules. The lower melting point of cis isomers makes them more favorable for human consumption (Adu-Mensah et al., 2019). Furthermore, for production of high-quality biodiesel, the viscosity is an important parameter that should be low enough for biodiesel to flow well in engines (Bari et al., 2020; Hoang, 2021).
Specific rate of individual FAME production (R) is an intensive parameter, i.e., it does not depend on the concentration of FAME components. The FAMEs with higher (Eq. 3) ratios have a higher R value, which indicate higher changes in the concentration of the FAME component with time. Calculation of this parameter helps metabolic studies. In fact, the components with a low R value are consumed for the production of longer-chain FAs. This parameter was calculated in 5-day intervals. As shown in Figure 4A, at the start of the growth period (5–10 days), R has the highest values. It decreases with time so that the value reaches the minimum at 20- to 25-day intervals. Oleic and palmitic acid have the lowest R value in the 5- to 10-day interval. It can be concluded that FAME components with a lower R value such as oleic acid (C18:1n9c) and palmitic acid (C16:0) are involved in the production of long-chain FAs.
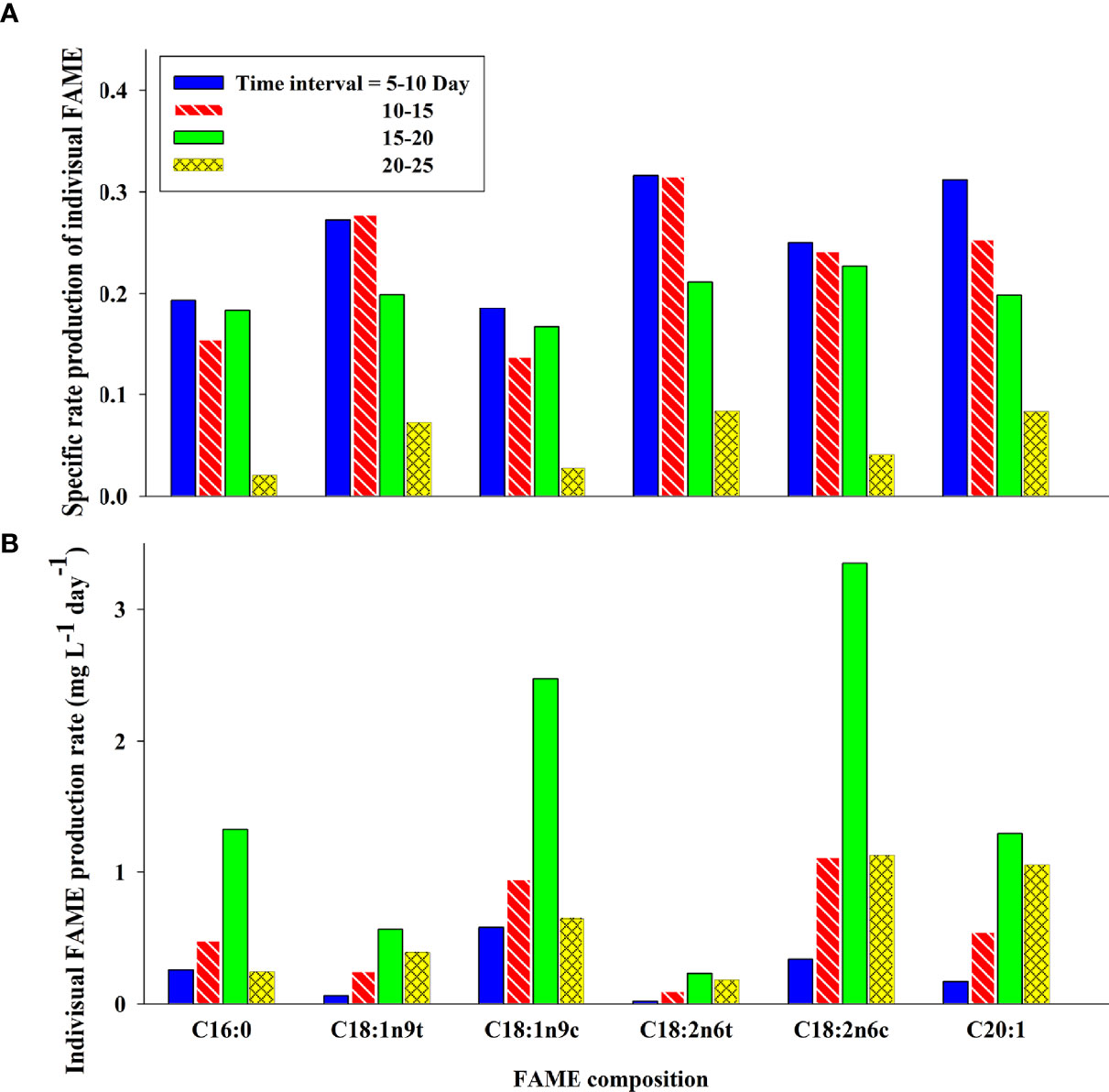
Figure 4 Specific rate of individual FA production (A) and productivity of individual FAs (B) of C. vulgaris as a function of time.
FAME productivity (P) is directly related to the concentration of FAME components. This means that it is an extensive property, i.e., it depends on the biomass. Therefore, the components with higher concentration like oleic acid and linoleic acid have higher FAME productivities as compared to the other components. The productivity of FAs was influenced by the lipid productivity. This parameter was calculated in 5-day intervals to evaluate the variation of algal cells’ ability for FAME production in different time intervals. As depicted in Figure 4B, P values reach the maximum of 3.4 mg L−1 day−1 at the 15- to 20-day interval for linoleic acid. Comparing Figures 2 and 4 shows a correlation between the individual FAME production rate and the algal growth phases. The FA content and FAME productivity in addition to the lipid productivity are good indicators for industrial applications of this microalgae strain for biodiesel production.
3.3 Kinetics Study
Mathematical models are necessary to represent the complexity of molecular processes or the dynamics of metabolite production (Hasdemir et al., 2014; Saa and Nielsen, 2016). Metabolic pathways are highly sophisticated and, therefore, metabolic engineering strategies are often best described with metabolic models (Strutz et al., 2019). Moreover, using kinetic models, metabolite concentrations can be predicted as a function of time (Costello and Martin, 2018).
Two kinetic models were applied to predict the FAME production after in situ transesterification of C. vulgaris and the experimental values were used to validate the models. Experimental analysis of FAME is costly and time-consuming and it needs large sample volumes. Therefore, modeling of FAME production could save both time and capital.
Table 1 shows R2, RMSD (Eq. 6 and Eq. 8), and the constant values for two mathematical models (Eq. 4 and Eq. 5), which describe the FAME production kinetics of C. vulgaris.
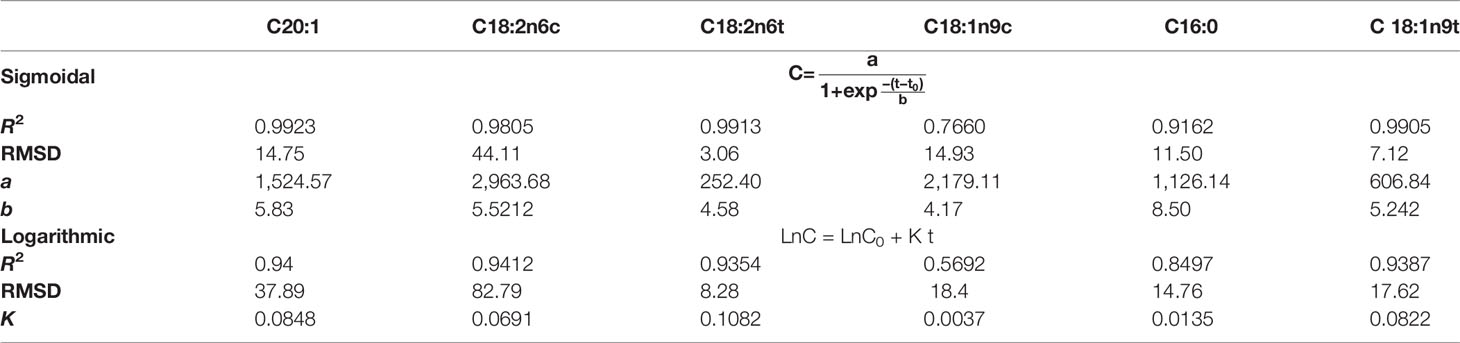
Table 1 Sigmoidal and logarithmic kinetics models for the prediction of FAME produced by C. vulgaris.
The coefficient of determination (R2) was applied as an indicator to demonstrate the preciseness of models for fitting the experimental data. The highest accuracy of a model is achieved when R2 is equal to one. RMSDs are usually applied to compare the accuracy of different models. A model with smaller RMSD represents more accuracy than a model with larger values. For most FAMEs (except for oleic and palmitic acid), Sigmoidal models gives a better fit (R2 > 0.98) and lower RMSD in comparison with the Logarithmic model. Figures 5A, B show the predicted results in comparison with the experimental values for Logarithmic and Sigmoidal models, respectively. It can be concluded that the Sigmoidal model shows great predictability for FAMEs production using C. vulgaris.
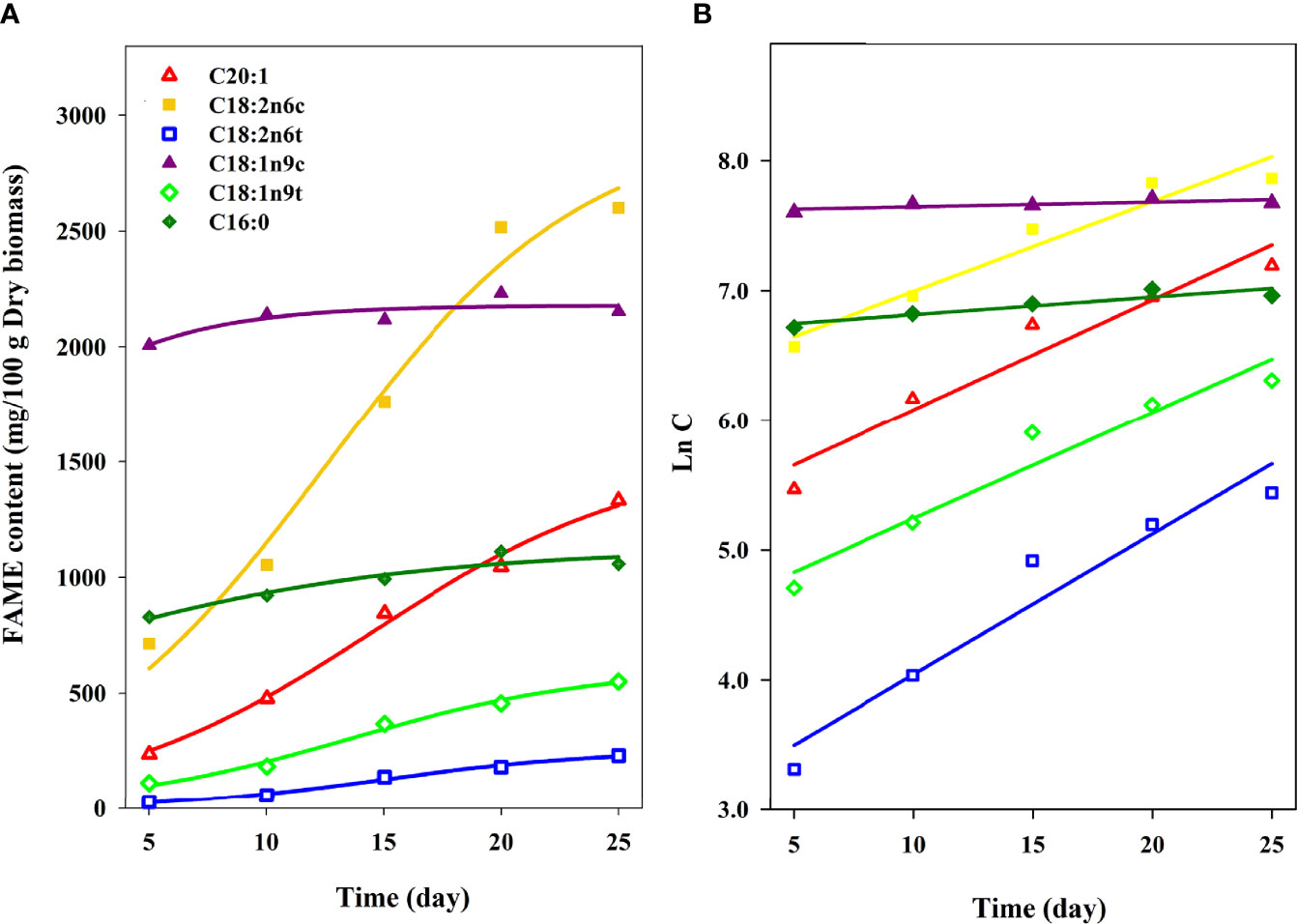
Figure 5 Comparison of experimental versus predicted values of important FAME components for (A) Sigmoidal and (B) Logarithmic models. Lines represent the predicted values and symbols represent the experimental values.
4 Conclusions
Most researchers focus on the total lipid and/or FA profiling of microalgae. In this work, we have reported on determining FA profile and specific production rates of individual FAs in different days. Constant or low specific growth production rate of a FA such as stearic and palmitic acid indicate their role as precursors for longer-chain fatty acids. Saturated and long-chain fatty acids can be obtained by desaturase and elongase enzymes. Due to the stereospecific characterization of desaturase enzymes, cis configuration such as oleic and linoleic acids are more dominant. The growth rates of individual fatty acids were fitted using two models, and it was confirmed that the Sigmoidal model provides a better fit for all fatty acids except for oleic and palmitic acid. Total lipid yield remained constant after the 15th day of cultivation, whereas the total FA concentrations reached a plateau after the 20th day. We concluded that lower-chain FAs act as precursors to produce longer-chain FAs. FA profiles in different days were compared to calculate the rate of individual FAs production for the growth period. Although the literature usually reports on the total lipid or FA profile in 1 day of cultivation, to the best of our knowledge, this is the first report focusing on the specific rates of individual FAs.
In this study, the concentration of linoleic acid increased from 713 to 2,599 mg per 100 g of dried biomass while concentrations of the palmitic and oleic acid remained constant at approximately 1,000 and 2,000 mg per 100 g of dried biomass, respectively. Our results show that oleic acid and palmitic acid have the lowest R value in the 5- to 10-day interval. We concluded that FAME components with lower R values such as oleic acid (C18:1n9c) and palmitic acid (C16:0) are involved in the production of long-chain FAs. The higher-concentration FAMEs such as oleic acid and linoleic acid have higher productivities.
Data Availability Statement
The raw data supporting the conclusions of this article will be made available by the authors, without undue reservation.
Author Contributions
NM-K: Data collection, sample analysis, writing—original draft, and data interpretation. HA: Conceptualization, data analysis, funding acquisition, project supervision, and data validation. SL: Conceptualization and writing—review and editing. All authors contributed to the article and approved the submitted version.
Funding
The authors appreciate the financial support from the ATF committee and Ferdowsi University of Mashhad (grant numbers of 3/29836).
Conflict of Interest
Author Stephen Lyon is employed by SRL-Environmental, LLC.
The remaining authors declare that the research was conducted in the absence of any commercial or financial relationships that could be construed as a potential conflict of interest.
Publisher’s Note
All claims expressed in this article are solely those of the authors and do not necessarily represent those of their affiliated organizations, or those of the publisher, the editors and the reviewers. Any product that may be evaluated in this article, or claim that may be made by its manufacturer, is not guaranteed or endorsed by the publisher.
Acknowledgments
NM-K thanks Mehdi Zanghaee for GC analysis of FAs.
References
Adu-Mensah D., Mei D., Zuo L., Zhang Q., Wang J. (2019). A Review on Partial Hydrogenation of Biodiesel and its Influence on Fuel Properties. Fuel 251, 660–668. doi: 10.1016/j.fuel.2019.04.036
Amit, Ghosh U. K. (2018). An Approach for Phycoremediation of Different Wastewaters and Biodiesel Production Using Microalgae. Environ. Sci. Poll. Res. 25, 18673–18681. doi: 10.1007/s11356-018-1967-5
Aussant J., Guihéneuf F., Stengel D. B. (2018). Impact of Temperature on Fatty Acid Composition and Nutritional Value in Eight Species of Microalgae. Appl. Microbiol. Biotechnol. 102, 5279–5297. doi: 10.1007/s00253-018-9001-x
Balić A., Vlašić D., Žužul K., Marinović B., Bukvić Mokos Z. (2020). Omega-3 Versus Omega-6 Polyunsaturated Fatty Acids in the Prevention and Treatment of Inflammatory Skin Diseases. Int. J. Mol. Sci. 21, 741–766. doi: 10.3390/ijms21030741
Bari S., Hossain S. N., Saad I. (2020). A Review on Improving Airflow Characteristics Inside the Combustion Chamber of CI Engines to Improve the Performance With Higher Viscous Biofuels. Fuel 264, 116769. doi: 10.1016/j.fuel.2019.116769
Bilal M., Qindeel M., Nunes L. V., Duarte M. T. S., Ferreira L. F. R., Soriano R. N., et al. (2021). Marine-Derived Biologically Active Compounds for the Potential Treatment of Rheumatoid Arthritis. Mar. Drugs 19, 10. doi: 10.3390/md19010010
Blasio M., Balzano S. (2021). Fatty Acids Derivatives From Eukaryotic Microalgae, Pathways and Potential Applications. Front. Microbiol. 12. doi: 10.3389/fmicb.2021.718933
Borowitzka M. A., Moheimani N. R. (2013). Algae for Biofuels and Energy (Dordrecht: Springer). doi: 10.1007/978-94-007-5479-9
Brindhadevi K., Mathimani T., Rene E. R., Shanmugam S., Chi N. T. L., Pugazhendhi A. (2021). Impact of Cultivation Conditions on the Biomass and Lipid in Microalgae With an Emphasis on Biodiesel. Fuel 284, 119058. doi: 10.1016/j.fuel.2020.119058
Caporgno M. P., Mathys A. (2018). Trends in Microalgae Incorporation Into Innovative Food Products With Potential Health Benefits. Front. Nutr. 5. doi: 10.3389/fnut.2018.00058
Chen X., Yang Y., Lu Q., Sun X., Wang S., Li Q., et al. (2021). The Influence of Light Intensity and Organic Content on Cultivation of Chlorella Vulgaris in Sludge Extracts Diluted With BG11. Aquacul. Int. 29, 2131–2144. doi: 10.1007/s10499-021-00740-3
Costello Z., Martin H. G. (2018). A Machine Learning Approach to Predict Metabolic Pathway Dynamics From Time-Series Multiomics Data. NPJ Syst. Biol. Appl. 4, 19. doi: 10.1038/s41540-018-0054-3
Del Río E., García-Gómez E., Moreno J., G. Guerrero M., García-González M. (2017). Microalgae for Oil. Assessment of Fatty Acid Productivity in Continuous Culture by Two High-Yield Strains, Chlorococcum Oleofaciens and Pseudokirchneriella Subcapitata. Algal. Res. 23, 37–42. doi: 10.1016/j.algal.2017.01.003
Djuricic I., Calder P. C. (2021). Beneficial Outcomes of Omega-6 and Omega-3 Polyunsaturated Fatty Acids on Human Health: An Update for 2021. Nutrients 13, 2421. doi: 10.3390/nu13072421
Elegbede I., Matemilola S., Kies F., Fadeyi O., Saba A., De Los Rios P., et al. (2017). Risk Analysis and Development of Algae Biofuel From Aquatic and Terrestrial Systems. Energy Proc. 128, 324–331. doi: 10.1016/j.egypro.2017.08.320
Griffiths M., Harrison S. L. (2009). Lipid Productivity as a Key Characteristic for Choosing Algal Species for Biodiesel Production. J. Appl. Phycol. 21, 493–507. doi: 10.1007/s10811-008-9392-7
Guschina I. A., Harwood J. L. (2006). Lipids and Lipid Metabolism in Eukaryotic Algae. Prog. Lipid Res. 45, 160–186. doi: 10.1016/j.plipres.2006.01.001
Harris R. V., James A. T. (1965a). The Fatty Acid Metabolism of Chlorella Vulgaris. Biochim. Biophys. Acta 106, 465–473. doi: 10.1016/0005-2760(65)90063-9
Harris R. V., James A. T. (1965b). Linoleic and Alpha-Linolenic Acid Biosynthesis in Plant Leaves and Green Alga. Biochim. Biophys. Acta 106, 456–464. doi: 10.1016/0005-2760(65)90062-7
Harwood J. L., Guschina I. A. (2009). The Versatility of Algae and Their Lipid Metabolism. Biochimie 91, 679–684. doi: 10.1016/j.biochi.2008.11.004
Hasdemir D., Hoefsloot H. C. J., Westerhuis J. A., Smilde A. K. (2014). How Informative is Your Kinetic Model?: Using Resampling Methods for Model Invalidation. BMC Syst. Biol. 8, 61. doi: 10.1186/1752-0509-8-61
Hempel N., Petrick I., Behrendt F. (2012). Biomass Productivity and Productivity of Fatty Acids and Amino Acids of Microalgae Strains as Key Characteristics of Suitability for Biodiesel Production. J. Appl. Phycol. 24, 1407–1418. doi: 10.1007/s10811-012-9795-3
Hoang A. T. (2021). Prediction of the Density and Viscosity of Biodiesel and the Influence of Biodiesel Properties on a Diesel Engine Fuel Supply System. J. Mar. Eng. Technol. 20, 299–311. doi: 10.1080/20464177.2018.1532734
Hooper L., Al-Khudairy L., Abdelhamid A. S., Rees K., Brainard J. S., Brown T. J., et al. (2018). Omega-6 Fats for the Primary and Secondary Prevention of Cardiovascular Disease. Cochrane Database Syst. Rev. 7, Cd011094. doi: 10.1002/14651858.CD011094.pub3
Howling D., Morris L. J., James A. T. (1968). The Influence of Chain Length on the Dehydrogenation of Saturated Fatty Acids. Biochim. Biophys. Acta (BBA) - Lipids Lipid Metab. 152, 224–226. doi: 10.1016/0005-2760(68)90027-1
Jalilian N., Najafpour G. D., Khajouei M. (2020). Macro and Micro Algae in Pollution Control and Biofuel Production – A Review. ChemBioEng. Rev. 7, 18–33. doi: 10.1002/cben.201900014
Ju J.-H., Oh B.-R., Ko D.-J., Heo S.-Y., Lee J.-J., Kim Y.-M., et al. (2019). Boosting Productivity of Heterotrophic Microalgae by Efficient Control of the Oxygen Transfer Coefficient Using a Microbubble Sparger. Algal. Res. 41, 101474. doi: 10.1016/j.algal.2019.101474
Kabir F., Gulfraz M., Raja G. K., Inam-Ul-Haq M., Awais M., Mustafa M. S., et al. (2020). Screening of Native Hyper-Lipid Producing Microalgae Strains for Biomass and Lipid Production. Renewable Energy 160, 1295–1307. doi: 10.1016/j.renene.2020.07.004
Kim Z. H., Kim K., Park H., Lee C. S., Nam S. W., Yim K. J., et al. (2021). Enhanced Fatty Acid Productivity by Parachlorella Sp., a Freshwater Microalga, via Adaptive Laboratory Evolution Under Salt Stress. Biotechnol. Biopro. Eng. 26, 223–231. doi: 10.1007/s12257-020-0001-1
Kim Z. H., Park Y.-S., Ryu Y.-J., Lee C.-G. (2017). Enhancing Biomass and Fatty Acid Productivity of Tetraselmis Sp. In Bubble Column Photobioreactors by Modifying Light Quality Using Light Filters. Biotechnol. Biopro. Eng. 22, 397–404. doi: 10.1007/s12257-017-0200-6
Lakshmikandan M., Murugesan A. G., Wang S., Abomohra A. E.-F., Jovita P. A., Kiruthiga S. (2020). Sustainable Biomass Production Under CO2 Conditions and Effective Wet Microalgae Lipid Extraction for Biodiesel Production. J. Clean. Prod. 247, 119398. doi: 10.1016/j.jclepro.2019.119398
Lam M. K., Yusoff M. I., Uemura Y., Lim J. W., Khoo C. G., Lee K. T., et al. (2017). Cultivation of Chlorella Vulgaris Using Nutrients Source From Domestic Wastewater for Biodiesel Production: Growth Condition and Kinetic Studies. Renewable Energy 103, 197–207. doi: 10.1016/j.renene.2016.11.032
Lari Z., Moradi-Kheibari N., Ahmadzadeh H., Abrishamchi P., Moheimani N. R., Murry M. A. (2016). Bioprocess Engineering of Microalgae to Optimize Lipid Production Through Nutrient Management. J. Appl. Phycol. 28, 3235–3250. doi: 10.1007/s10811-016-0884-6
Lim J. H. K., Gan Y. Y., Ong H. C., Lau B. F., Chen W.-H., Chong C. T., et al. (2021). Utilization of Microalgae for Bio-Jet Fuel Production in the Aviation Sector: Challenges and Perspective. Renewable Sustain. Energy Rev. 149, 111396. doi: 10.1016/j.rser.2021.111396
Lordan R., Tsoupras A., Zabetakis I. (2017). Phospholipids of Animal and Marine Origin: Structure, Function, and Anti-Inflammatory Properties. Mol. (Basel. Switzerland). 22, 1964. doi: 10.3390/molecules22111964
Mata T. M., Martins A. A., Caetano N. S. (2010). Microalgae for Biodiesel Production and Other Applications: A Review. Renewable Sustain. Energy Rev. 14, 217–232. doi: 10.1016/j.rser.2009.07.020
Molazadeh M., Ahmadzadeh H., Pourianfar H. R., Lyon S., Rampelotto P. H. (2019a). The Use of Microalgae for Coupling Wastewater Treatment With CO2 Biofixation. Front. BioEng. Biotechnol. 7. doi: 10.3389/fbioe.2019.00042
Molazadeh M., Danesh S., Ahmadzadeh H., Pourianfar H. R. (2019b). Influence of CO2 Concentration and N:P Ratio on Chlorella Vulgaris-Assisted Nutrient Bioremediation, CO2 Biofixation and Biomass Production in a Lagoon Treatment Plant. J. Taiwan. Insti. Chem. Engr. 96, 114–120. doi: 10.1016/j.jtice.2019.01.005
Mondal M., Khan A. A., Halder G. (2021). Estimation of Biodiesel Properties Based on Fatty Acid Profiles of Chlamydomonas Sp. BTA 9032 and Chlorella Sp. BTA 9031 Obtained Under Mixotrophic Cultivation Conditions. Biofuels 12, 1175–1181. doi: 10.1080/17597269.2019.1600453
Moradi-Kheibari N., Ahmadzadeh H., Hosseini M. (2017). Use of Solvent Mixtures for Total Lipid Extraction of Chlorella Vulgaris and Gas Chromatography FAME Analysis. Biopro. Biosyst. Eng. 40, 1363–1373. doi: 10.1007/s00449-017-1794-y
Nakamura Y., Li-Beisson Y. (2016). Lipids in Plant and Algae Development (Cham: Springer International Publishing). doi: 10.1007/978-3-319-25979-6
Paliwal C., Jutur P. P. (2021). Dynamic Allocation of Carbon Flux Triggered by Task-Specific Chemicals is an Effective non-Gene Disruptive Strategy for Sustainable and Cost-Effective Algal Biorefineries. Chem. Eng. J. 418, 129413. doi: 10.1016/j.cej.2021.129413
Paliwal C., Mitra M., Bhayani K., Bharadwaj S. V. V., Ghosh T., Dubey S., et al. (2017). Abiotic Stresses as Tools for Metabolites in Microalgae. Biores. Technol. 244, 1216–1226. doi: 10.1016/j.biortech.2017.05.058
Park H., Jung D., Lee J., Kim P., Cho Y., Jung I., et al. (2018). Improvement of Biomass and Fatty Acid Productivity in Ocean Cultivation of Tetraselmis Sp. Using Hypersaline Medium. J. Appl. Phycol. 30, 2725–2735. doi: 10.1007/s10811-018-1388-3
Park S., Nguyen T. H. T., Jin E. (2019). Improving Lipid Production by Strain Development in Microalgae: Strategies, Challenges and Perspectives. Biores. Technol. 292, 121953. doi: 10.1016/j.biortech.2019.121953
Patel A., Desai S. S., Mane V. K., Enman J., Rova U., Christakopoulos P., et al. (2022). Futuristic Food Fortification With a Balanced Ratio of Dietary ω-3/ω-6 Omega Fatty Acids for the Prevention of Lifestyle Diseases. Trends Food Sci. Technol. 120, 140–153. doi: 10.1016/j.tifs.2022.01.006
Pushpakumari Kudahettige N., Pickova J., Gentili F. G. (2018). Stressing Algae for Biofuel Production: Biomass and Biochemical Composition of Scenedesmus Dimorphus and Selenastrum Minutum Grown in Municipal Untreated Wastewater. Front. Energy Res. 6. doi: 10.3389/fenrg.2018.00132
Qiu X., Xie X., Meesapyodsuk D. (2020). Molecular Mechanisms for Biosynthesis and Assembly of Nutritionally Important Very Long Chain Polyunsaturated Fatty Acids in Microorganisms. Prog. Lipid Res. 79, 101047. doi: 10.1016/j.plipres.2020.101047
Rafa N., Ahmed S. F., Badruddin I. A., Mofijur M., Kamangar S. (2021). Strategies to Produce Cost-Effective Third-Generation Biofuel From Microalgae. Front. Energy Res. 9. doi: 10.3389/fenrg.2021.749968
Ram S., Paliwal C., Mishra S. (2019). Growth Medium and Nitrogen Stress Sparked Biochemical and Carotenogenic Alterations in Scenedesmus Sp. CCNM 1028. Biores. Technol. Rep. 7, 100194. doi: 10.1016/j.biteb.2019.100194
Rippka R., Deruelles J., Waterbury J. B., Herdman M., Stanier R. Y. (1979). Generic Assignments, Strain Histories and Properties of Pure Cultures of Cyanobacteria. Microbiology 111, 1–61. doi: 10.1099/00221287-111-1-1
Saa P. A., Nielsen L. K. (2016). Construction of Feasible and Accurate Kinetic Models of Metabolism: A Bayesian Approach. Sci. Rep. 6, 29635. doi: 10.1038/srep29635
Shen X.-F., Gao L.-J., Zhou S.-B., Huang J.-L., Wu C.-Z., Qin Q.-W., et al. (2020). High Fatty Acid Productivity From Scenedesmus Obliquus in Heterotrophic Cultivation With Glucose and Soybean Processing Wastewater via Nitrogen and Phosphorus Regulation. Sci. Total. Environ. 708, 134596. doi: 10.1016/j.scitotenv.2019.134596
Shrestha N., Sleep S. L., Cuffe J. S. M., Holland O. J., Perkins A. V., Yau S. Y., et al. (2020). Role of Omega-6 and Omega-3 Fatty Acids in Fetal Programming. Clin. Exp. Pharmacol. Physiol. 47, 907–915. doi: 10.1111/1440-1681.13244
Singh R., Paliwal C., Nesamma A. A., Narula A., Jutur P. P. (2020). Nutrient Deprivation Mobilizes the Production of Unique Tocopherols as a Stress-Promoting Response in a New Indigenous Isolate Monoraphidium Sp. Front. Mar. Sci. 7. doi: 10.3389/fmars.2020.575817
Strutz J., Martin J., Greene J., Broadbelt L., Tyo K. (2019). Metabolic Kinetic Modeling Provides Insight Into Complex Biological Questions, But Hurdles Remain. Curr. Opin. Biotechnol. 59, 24–30. doi: 10.1016/j.copbio.2019.02.005
Talebi A. F., Tohidfar M., Bagheri A., Lyon S. R., Salehi-Ashtiani K., Tabatabaei M. (2014). Manipulation of Carbon Flux Into Fatty Acid Biosynthesis Pathway in Dunaliella Salina Using AccD and ME Genes to Enhance Lipid Content and to Improve Produced Biodiesel Quality. Biofuel. Res. J. 1, 91–97. doi: 10.18331/brj2015.1.3.6
Taziki M., Ahmadzadeh H., Murry M. A. (2015). Growth of Chlorella Vulgaris in High Concentrations of Nitrate and Nitrite for Wastewater Treatment. Curr. Biotechnol. 4, 1–7. doi: 10.2174/2211550104666150930204835
Thoisen C., Pedersen J. S., Jørgensen L., Kuehn A., Hansen B. W., Nielsen S. L. (2020). The Effect of Cell Density on Biomass and Fatty Acid Productivity During Cultivation of Rhodomonas Salina in a Tubular Photobioreactor. Aquacul. Res. 51, 3367–3375. doi: 10.1111/are.14672
Xue Z., Yu Y., Yu W., Gao X., Zhang Y., Kou X. (2020). Development Prospect and Preparation Technology of Edible Oil From Microalgae. Front. Mar. Sci. 7. doi: 10.3389/fmars.2020.00402
Zheng H., Yin J., Gao Z., Huang H., Ji X., Dou C. (2011). Disruption of Chlorella Vulgaris Cells for the Release of Biodiesel-Producing Lipids: A Comparison of Grinding, Ultrasonication, Bead Milling, Enzymatic Lysis, and Microwaves. Appl. Biochem. Biotechnol. 164, 1215–1224. doi: 10.1007/s12010-011-9207-1
Keywords: Chlorella vulgaris (C. vulgaris), fatty acid profiling, FAME differentiation, total lipid, FAME kinetics models
Citation: Moradi-Kheibari N, Ahmadzadeh H and Lyon SR (2022) Correlation of Total Lipid Content of Chlorella vulgaris With the Dynamics of Individual Fatty Acid Growth Rates. Front. Mar. Sci. 9:837067. doi: 10.3389/fmars.2022.837067
Received: 16 December 2021; Accepted: 21 March 2022;
Published: 25 April 2022.
Edited by:
Shailesh Kumar Patidar, Central University of Rajasthan, IndiaReviewed by:
Chetan Paliwal, International Centre for Genetic Engineering and Biotechnology, IndiaXupeng Cao, Dalian Institute of Chemical Physics (CAS), China
Copyright © 2022 Moradi-Kheibari, Ahmadzadeh and Lyon. This is an open-access article distributed under the terms of the Creative Commons Attribution License (CC BY). The use, distribution or reproduction in other forums is permitted, provided the original author(s) and the copyright owner(s) are credited and that the original publication in this journal is cited, in accordance with accepted academic practice. No use, distribution or reproduction is permitted which does not comply with these terms.
*Correspondence: Hossein Ahmadzadeh, aC5haG1hZHphZGVoQHVtLmFjLmly