- 1Department of Geography, University of Victoria, Victoria, BC, Canada
- 2Institute of Ocean Sciences, Fisheries and Oceans Canada, Sidney, BC, Canada
- 3Pacific Biological Station, Fisheries and Oceans Canada, Nanaimo, BC, Canada
The Strait of Georgia, Canada, is an important region for numerous commercially and culturally important species (e.g., herring, salmon, and orcas), yet little is known about the links between lower trophic level (e.g. phytoplankton and zooplankton) phenology due to historical sampling gaps. Here, we present fourteen years (2003-2016) of data linking interannual variability in phytoplankton and zooplankton phenology in the Central Strait of Georgia, BC. Satellite-derived chlorophyll a (Chl a) data were used to calculate spring bloom dynamics (bloom initiation, bloom intensity, and bloom magnitude). Average spring bloom initiation occurred during the last week of March in the Central Strait of Georgia. Bloom initiation occurred in mid-to-late February/early March during “early” Chl a bloom years (2004, 2005, and 2015) whereas initiation did not occur until the end of April during “late” bloom years (2007, 2008). Spring Chl a bloom initiation was significantly correlated with the North Pacific Gyre Oscillation (NPGO; r = 0.75, p < 0.01) and spring sea surface temperature (SST; r = -0.70, p < 0.01); spring blooms occurred earlier during warm years. When all environmental variables were considered together, NPGO best explained variations in spring bloom initiation (Adj R2 = 0.53, p < 0.01) and bloom magnitude (Adj R2 = 0.57, p < 0.01), whereas stratification best explained variations in bloom intensity (Adj R2 = 0.38, p < 0.05). Early Chl a blooms were associated with high crustacean abundance (maximum of > 1000 ind m-3) but low biomass (37.5 mg m-3). Independent of the Chl a data, hierarchical cluster analysis revealed similar groupings of years for crustacean abundance data. Most notably, community composition in cluster Group 2 (2004, 2005, and 2015; early Chl a bloom years), was comprised of a higher proportion of small crustaceans (e.g. non-calanoid copepods) compared to the other cluster groups. To our knowledge, this study provides the first evidence linking early spring Chl a bloom timing to a shift in the crustacean community towards smaller taxa in response to multiple warm events in the Strait of Georgia. Our results show that early Chl a blooms may potentially result in a mismatch between phytoplankton and large energy-rich crustacean zooplankton, with lower abundances of the latter. In contrast, average Chl a bloom years were optimal for large-bodied euphausiids, whereas late Chl a blooms were a match for some crustaceans (e.g., medium calanoid copepods), but not others (e.g., large calanoid copepods and amphipods). We hypothesize that early bloom years may result in poorer feeding conditions for juvenile salmon and other predators in the region.
1 Introduction
Broadly defined, phenology is the study of the interannual variability in the timing of important life cycle events in response to changes in biotic and abiotic factors (Leith, 1974). In marine ecosystems, phenology is typically studied by examining the timing of peak abundances of key species or taxonomic groups (Mackas et al., 2013). The Match/Mismatch Hypothesis, put forth by Cushing (1969; Cushing, 1990), suggests that interannual variations in larval fish recruitment may be caused by the amount of overlap between their annual peak and the peak in their zooplankton prey. Both phytoplankton and zooplankton may show large year to year variability in response to variations in environmental drivers such as temperature, wind, and stratification (Mackas et al., 2012). Furthermore, warmer temperatures due to climate change are expected to result in shifts in zooplankton timing and community composition towards a community dominated by smaller species compared to historical norms (Richardson, 2008). Given that years with higher zooplankton biomass, particularly of large lipid-rich crustaceans, are generally thought to provide a better feeding environment for planktivorous fish and birds (Peterson and Schwing, 2003; Mackas et al., 2007, El Saabawi et al., 2013, Hipfner et al., 2020), shifts in the phenology of phytoplankton and zooplankton, and the resulting match/mismatch between these lower trophic levels, may have important consequences throughout the entire food web.
Studies investigating the match/mismatch between phytoplankton and fish phenology are fairly common in marine ecosystems. Platt et al. (2003) combined remote-sensing satellite data with long-term haddock recruitment data off the eastern continental shelf of Nova Scotia and showed that larval fish survival is dependent on the timing of the spring phytoplankton bloom. Similarly, Malick et al. (2015) found that spring bloom timing was significantly correlated with pink salmon productivity for Alaska and British Columbia populations. In addition, other studies have considered the match/mismatch of zooplankton phenology with respect to both higher and lower trophic levels. For example, Beaugrand et al. (2003) related changes in zooplankton biomass, timing, and community composition to a decline in Atlantic cod (Gadus morhua L.) recruitment in the North Sea, and Edwards and Richardson (2004) investigated changes in phenology in the North Sea across three trophic levels (from primary producers, through zooplankton, to fish larvae), revealing a mismatch between trophic levels in response to climate change.
Our study region, the Strait of Georgia (SoG), Canada, is a semi-enclosed basin between mainland British Columbia and Vancouver Island and is part of the larger waterbody known as the Salish Sea (Figure 1). This region provides important habitat and feeding grounds for numerous commercially and culturally important species such as Pacific hake, Pacific herring, Pacific salmon, and orcas. Spring bloom timing has been studied extensively in the SoG using satellite-derived methods (e.g., Gower et al., 2013; Schweigert et al., 2013; Jackson et al., 2015), biophysical models (Collins et al., 2009; Allen & Wolfe, 2013; Peña et al., 2016), and in situ chlorophyll a (Chl a) sampling (Halverson and Pawlowicz, 2013; Sastri et al., 2016). In addition, some studies have examined the link between the timing of the spring bloom and fish. For example, Chittenden et al. (2010) examined the effects of release time on migratory behaviour and survival of wild and hatchery-reared Coho salmon (Oncorhynchus kisutch) in relation to both phytoplankton and zooplankton blooms. Furthermore, Schweigert et al. (2013) examined Pacific herring (Clupea pallasi) and spring phytoplankton bloom phenology, determining that young of year herring had better growth and survival during years when spawning most closely matched phytoplankton bloom timing. More recently, Boldt et al. (2019) used the timing of the spring phytoplankton bloom and the peak availability of zooplankton prey to assess age-0 herring abundance and condition in the SoG.
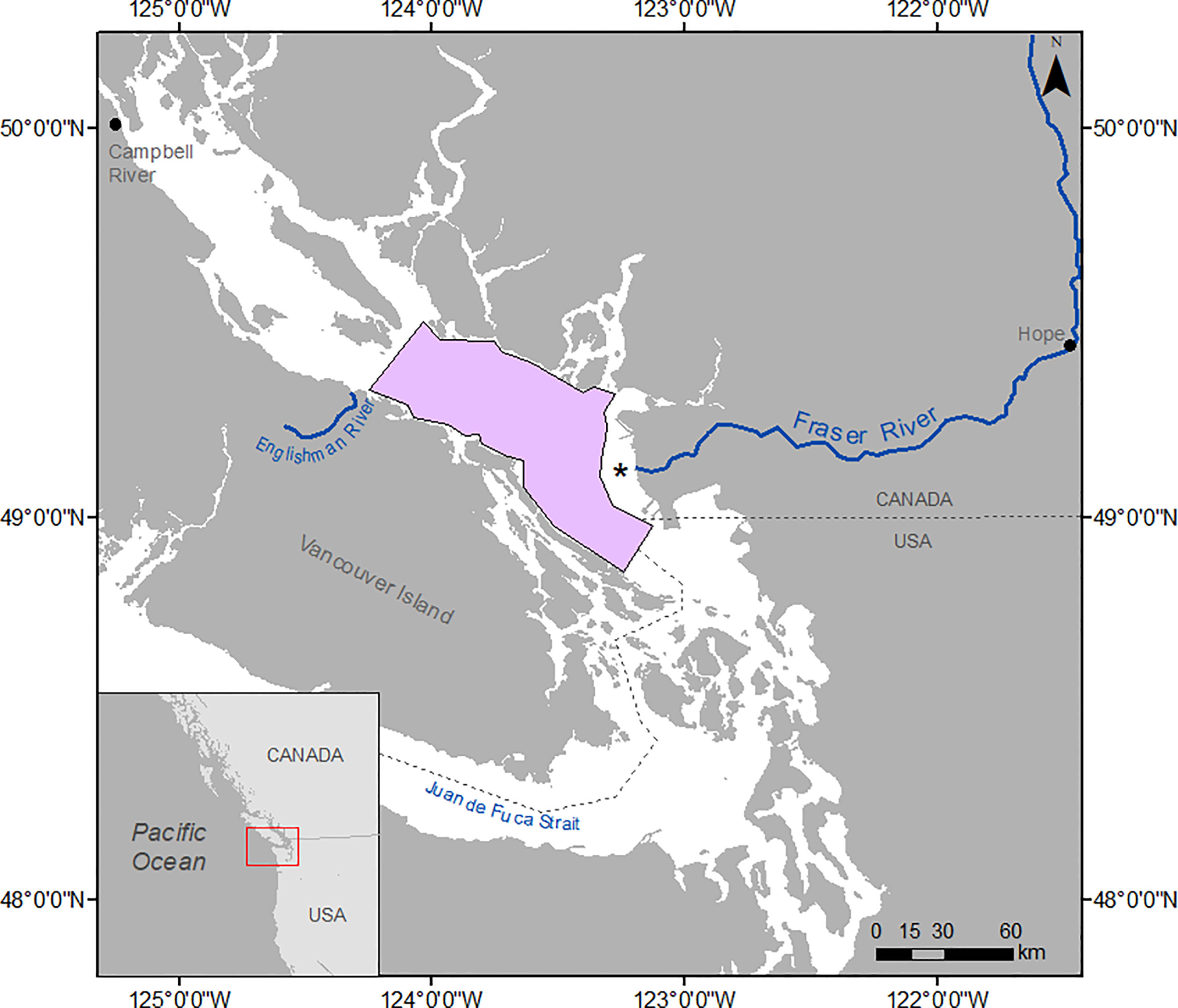
Figure 1 Map showing the Central Strait of Georgia (SoG), BC, Canada, study area (purple shading). Asterisk * indicates the high turbidity region near the mouth of the Fraser River that was masked during satellite retrievals.
While numerous studies in the Northeast Pacific have examined both interannual and decadal variability in zooplankton in response to environmental variables (e.g., Brodeur and Ware 1992; Mackas et al., 2001; Mackas et al., 2013; Li et al., 2013; Perry et al., 2021), only a few have considered zooplankton phenology. For example, Continuous Plankton Recorders (CPRs) have been used to examine zooplankton phenology for the Northeast Pacific region (Mackas et al., 2007; Batten and Mackas, 2009). However, studies on the link between phytoplankton and zooplankton phenology in the SoG are more limited (e.g. Yin et al., 1997; Mackas et al., 2013), likely due to the historical gaps in zooplankton sampling in the region (Mackas et al., 2013). Previous work has shown that interannual variability in zooplankton biomass in the SoG is predominantly related to decadal changes in the zooplankton signal that correlate with large-scale climate indices such as the North Pacific Gyre Oscillation (NPGO; Mackas et al., 2013) and the Pacific Decadal Oscillation (PDO, Perry et al., 2021). In general, cooler conditions in the SoG are known to be more favourable for large-bodied crustacean zooplankton taxa (Mackas et al., 2013); yet, the direct impacts of spring phytoplankton bloom timing and other bloom characteristics (e.g., bloom intensity, bloom magnitude) on the zooplankton community in this region remain unclear.
Here, we present the first long-term study linking interannual variability in both phytoplankton and zooplankton phenology in the Strait of Georgia, BC. This work is part of the Salish Sea Marine Survival Project (SSMSP), an initiative of the Pacific Salmon Foundation (Canada) and Long Live the Kings (USA), which aimed to address the declines in Coho and Chinook salmon that have been occurring since the 1980s in the Salish Sea (Preikshot et al., 2013). As such, we focus on crustaceans as they comprise the majority of the total zooplankton biomass in the SoG (Harrison et al., 1983; Mackas et al., 2013; Perry et al., 2021) and are a preferred food item for juvenile salmon (Neville & Beamish, 1999; Daly et al., 2010; Preikshot et al., 2010) and herring (Boldt et al., 2019). The main goal of this study was to examine the link between satellite-derived phytoplankton bloom dynamics and the abundance and biomass of the crustacean zooplankton community in the SoG during years with varying environmental conditions. Specifically, our objectives were to determine the match/mismatch in timing of crustacean zooplankton during early, average, and late Chl a bloom years. We examined both local and large-scale environmental drivers of Chl a bloom dynamics. Additionally, we used a cluster analysis approach to establish years with similar crustacean community composition, and subsequently compared bloom dynamics and environmental conditions between the clustering groups. Results from this study provide insight into how interannual variability in the phenology of key crustacean taxa may ultimately impact higher trophic levels in the region.
2 Methods
2.1 Study Area
The Strait of Georgia (SoG) has a surface area of approximately 6515 km2 with a maximum depth of over 400 m (Thomson, 1981), and is connected to open ocean waters at both its northern and southern ends. The main source of freshwater into the strait is the Fraser River, which plays an important role in stratification that varies with the seasonal influence of river input (Harrison et al., 1983). This influx of freshwater results in an estuarine-like circulation with surface waters (mostly) leaving the SoG via the Juan de Fuca Strait to the south and deep, nutrient-rich water being upwelled into the surface (Li et al., 2000; Pawlowicz et al., 2007). Our study focused on the Central SoG (Figure 1), as historical zooplankton sampling was most comprehensive in this region. Phytoplankton biomass in the Central SoG typically peaks in March (Peña et al., 2016), whereas zooplankton biomass has a more variable peak, occurring from late-spring to late-summer (Mackas et al., 2013). In addition, the Central SoG is one of the main regions associated with migrating juvenile salmon species (Beamish et al., 2012; Furey et al., 2015), with Coho and Chinook salmon typically entering the strait in mid-May (Beamish et al., 2010, Neville et al., 2015).
2.2 Satellite-Derived Chl a
2.2.1 Data Acquisition
Daily MODIS-Aqua imagery (Level 1A, 1 km2) from 2003-2016 were obtained from NASA’s Oceancolor web portal (https://oceancolor.gsfc.nasa.gov/). Details of satellite data processing are described in Suchy et al. (2019). Briefly, data were atmospherically corrected using SeaDAS (developed by the Ocean Biology Processing Group, OBPG) following regional methods outlined in Carswell et al. (2017) and applying the OC3M chlorophyll a (Chl a) algorithm (McClain et al., 2000). In addition, a mask (indicated by an asterisk in Figure 1) was applied to eliminate all satellite data from the region near the mouth of the Fraser River, as recommended in Komick et al. (2009), in order to minimize any error associated with the high turbidity known to occur in this region. Pixels were excluded with standard quality-control flags (e.g., contamination caused by straylight, high solar and sensor zenith angles). As a result, no data were available from mid-November to mid-February due to the position of the sun and extensive cloud cover during the winter months.
All Chl a products were spatially binned and mapped to a common grid at 1.1 km2 spatial resolution and transformed to a base 10 logarithm (Campbell, 1995). Missing pixels due to persistent cloud cover in the region were spatially interpolated using the Data Interpolating Empirical Orthogonal Functions (DINEOF) methodology (Beckers and Rixen, 2003) with considerations of the optimal temporal resolution of the input dataset as defined in Hilborn and Costa (2018). Implementation of the DINEOF method resulted in a daily spatially-continuous, gap-filled time series that was subsequently binned into 8-day “weeks”. Maps showing the seasonal and spatial variability in Chl a during the same years considered in this study are presented in Figures 8, 9 in Suchy et al. (2019). Although interannual variability in the spatial extent of Chl a was observed, Chl a concentrations during spring (mid-February to May), on average, were highest in the southern portion of the Central SoG near the mouth of the Fraser River. Warmer years exhibited widespread Chl a concentrations > 5 mg m-3 throughout the entire region, whereas Chl a blooms during colder years were more localized (Suchy et al., 2019).
2.2.2 Spring Bloom Phenology Metrics
Commonly used metrics for defining the start of the spring bloom in remote sensing studies include the date when chlorophyll concentrations first become 5% greater than the local annual median (e.g. Siegel et al., 2002; Henson et al., 2009) or selecting the date when chlorophyll concentrations first reach a pre-defined threshold concentration, e.g. 2 mg m-3 (Jackson et al., 2015). Given that our 8-day weekly Chl a data showed substantial variability from spring through to fall (Supplementary Table 1; and see Suchy et al., 2019), we chose a more conservative definition of spring bloom initiation. Spring bloom initiation was defined using a threshold value of the median + 5% Chl a concentration for a given year. Bloom initiation occurred when: i) the first week in our Chl a time series reached the threshold Chl a concentration, and ii) at least one of the two following weeks were >70% of the threshold value. This additional criterion was imposed to ensure that we did not prematurely assign bloom initiation to a given week in the Chl a time series if that week was followed by a long period of low Chl a concentrations. It should be noted that although using threshold values of 75% or 80% yielded the same results for all years except 2012 (Supplementary Table 2), we used the 70% value so as to not limit our ability to interpret bloom initiation in 2012. From these results, we calculated the mean spring bloom initiation week. Chl a bloom years were considered “early” or “late” if bloom initiation was greater than or equal to 1 standard deviation about the mean. In addition, we characterized spring bloom intensity and spring bloom magnitude using slightly modified definitions given in Friedland et al. (2015; Friedland et al., 2016) in order to account for the high variability in Chl a concentrations throughout the months considered in this study. Weeks with Chl a concentration above the median + 5% threshold concentration were considered to be under ‘bloom conditions’. Bloom intensity was then defined as the average Chl a concentrations across all weeks under bloom conditions over the course of the spring (mid-February to May), whereas spring bloom magnitude (a proxy for bloom duration) was defined as bloom intensity multiplied by the number of weeks under bloom conditions. Due to the dynamic nature of the region, it was not possible to clearly define the end of the bloom in a given year. Therefore, we focused on bloom dynamics during the spring only; the impacts of summer and autumn bloom conditions on crustacean zooplankton will be considered in future studies.
2.3 Zooplankton Data
Data were obtained from the Fisheries and Oceans Canada Institute of Ocean Sciences Zooplankton Database (version 9, data downloaded on 21 May 2020), a version of which is available on the Government of Canada Open Data Portal under Strait of Georgia zooplankton (https://open.canada.ca/data/en/dataset/2822c11d-6b6c-437e-ad65-85f584522adc).
2.3.1 Sampling
Zooplankton samples from 2003-2016 were collected as part of larger sampling programs that have been conducted in the SoG since 1996 by Fisheries and Oceans Canada (see Perry et al., 2021 for details). Sampling frequency has varied throughout the years; however, since 2015 sampling has been conducted approximately monthly from February to October (Table 1). Spatial coverage of the zooplankton sampling also varied between years, with a few core stations being consistently sampled throughout the time series (see Figure 1 in Perry et al., 2021). While the spatial coverage during the early part of our time series (i.e., prior to 2015) was mostly limited to these core stations, the spatial extent of zooplankton sampling has expanded throughout the Central SoG since 2015. For our study, only vertical net tow data from Scientific Committee on Oceanic Research (SCOR), Bongo, or ring nets with mouth diameters of approximately 50 cm and mesh sizes of approximately 250 µm were selected from the database. Typical vertical tow profiles were obtained from ~10 m above the bottom of the seafloor to the surface. Nets were retrieved at 1 m s-1, and calibrated flow meters were used to record the volumes filtered by each tow. Samples were preserved immediately in 10% sodium borate-buffered formalin in seawater. We focused our analyses on the deep (bottom depths deeper than 50 m) Central SoG as this region had the best temporal resolution over the time period of our study. In addition, we selected net tows that covered at least 70% or more of the water column to ensure that our samples included the diel and seasonal vertical migrating zooplankton taxa.
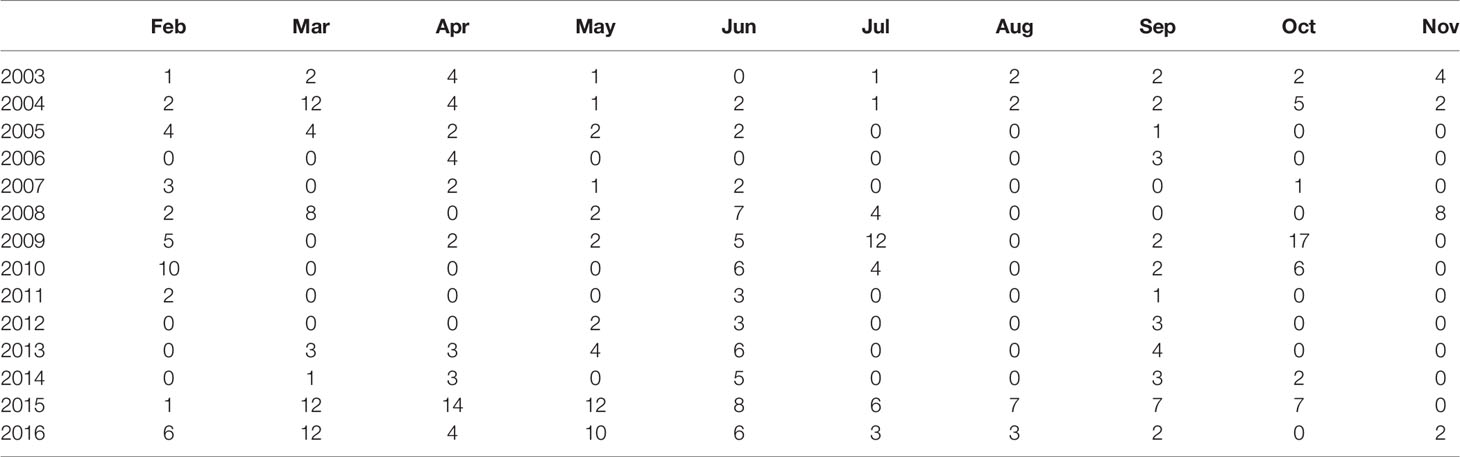
Table 1 Number of zooplankton samples collected in the Central Strait of Georgia, BC, by month from 2003-2016.
2.3.2 Taxonomic Analyses
In the laboratory, samples were examined using stereomicroscopes (Wild dissecting microscope up to 2013, and Zeiss SteREO Discovery 8 thereafter) and processed in two parts based on zooplankton size. Whole samples were first scanned for large (> 5 mm) or rare individuals, then split using a Folsom splitter to approximately 100 individuals > 5 mm. Plankton in these subsamples were identified to species, sex and stage where possible, and subsequently removed. The remaining subsample containing organisms < 5 mm was split to approximately 400 individuals which were identified to the lowest possible taxonomic classification and life history stage or size class. Biomass was calculated from the abundance data using the average biomass of individuals derived from measured or literature values. The average biomass values per individual taxa (species and life stage) are provided in the Open Data Portal listed above (see also Perry et al., 2021). Abundance and biomass data are presented as numbers or weight (dry mass; mg) per m3, respectively.
For taxonomic groupings, we followed those defined in Table S1 in Perry et al. (2021). Specifically, we focused on the following crustacean groups: hyperiid amphipods, small (prosome length < 1 mm) calanoid copepods, medium (prosome length 1-3 mm) calanoid copepods, large (prosome length > 3 mm) calanoid copepods, non-calanoid copepods, cladocerans, ostracods, decapods (larval crabs), and euphausiids. Daytime adult euphausiid abundance/biomass was multiplied by 3 to adjust for under sampling due to net avoidance (Mackas et al., 2013; Perry et al., 2021).
2.3.3 Crustacean Zooplankton Phenology
Due to inconsistent and patchy temporal sampling throughout our study period, the zooplankton data were insufficient to look at peak timing in individual years using common mathematical methods such as the central tendency (e.g. Edwards & Richardson, 2004) or the cumulative percentiles (e.g. Mackas et al., 2012) methods. Therefore, we calculated the climatological peak timing of crustacean abundance and biomass by taking the mean value for a given month over the course of our time series (2003-2016) and smoothing the data using simple spline curves. Additionally, we calculated similar climatologies using mean monthly crustacean abundance and biomass values for the “early”, “average”, and “late” years as defined by the Chl a bloom metrics in order to assess the match/mismatch between phytoplankton and crustacean zooplankton under different bloom conditions.
2.4 Environmental Variables
Annual values for large-scale climate indices and local environmental variables were calculated using the same datasets presented in Suchy et al. (2019) and are provided in the Supplementary material (Supplementary Table 3). Here, we considered the annual values of three large-scale climate indices: the Pacific Decadal Oscillation (PDO), the North Pacific Gyre Oscillation (NPGO), and the Southern Oscillation Index (SOI). In addition, we considered local environmental variables, averaged over late winter/spring months (January-May) in order to capture the conditions prior to, and during, the spring Chl a bloom. Sea surface temperature (SST) and photosynthetic active radiation (PAR), which were calculated from daily MODIS-Aqua (Level 2, 1 km2 spatial resolution) satellite products, were only available from mid-February to May. Daily average wind speed (km h-1) data were obtained from the Halibut Bank buoy in the Central SoG (Station 46146, 49°20′24″N 123°43′48″W; Environment and Climate Change Canada). Wind speed values were converted to wind speed cubed (m3 s-3; a proxy for wind stress). Fraser River peak discharge data at Hope, BC (Station 08MF005) were obtained from Environment and Climate Change Canada. Mean sea surface salinity (SSS), salinity averaged over 0-100 m, and a stratification parameter (Δσt; kg m−3) were obtained from CTD (Conductivity, Temperature, Depth) profiles collected by the Institute of Ocean Sciences, Fisheries and Oceans Canada, Sidney, BC. Stratification was calculated as the density difference between the surface (averaged over the top 10 m) and 50 m (see Suchy et al., 2019).
2.5 Statistical Analyses
Individual relationships between spring Chl a bloom dynamics (initiation, intensity, and magnitude) and environmental variables (large-scale climate indices and local drivers) were examined by creating a heatmap of Pearson Product Moment correlations in order to determine the environmental variables associated with Chl a bloom metrics. In addition, we considered all environmental variables together using forward selection stepwise regression (Blanchet et al., 2008) to determine which environmental variable(s) (explanatory variables) best explained each bloom dynamic metric (dependent variables). The explanatory variable with the highest significant correlation was added to the model first and sequential variables were added only if they reduced the Akaike Information Criterion (AIC). Only models that were statistically significant were considered and any variables showing multicollinearity, i.e., having a variance inflation factor (VIF) > 10, were removed. Multiple linear regression was then performed on the explanatory variable(s) represented in the model selected by the forward regression procedure. The percentage of variation explained by each model was presented as an adjusted R2 (Adj R2) value. We recognize that potential problems have been identified with the use of stepwise regression to reduce the number of variables in multivariate analyses (e.g. Whittingham et al., 2006). However, Smith (2018) has noted that stepwise regression performs poorest when there are a large number of potential predictors. In our case, we had only 9 predictors in our initial regression models, which is not a large number. In addition, our time series of 14 years was relatively short and not amenable to other statistical tests which separate the data series into training and test/validation segments.
Spearman Rank Order correlations were used to explore the relationships between total annual crustacean abundance and biomass with spring bloom dynamics to see if variability in Chl a bloom metrics had an impact on the crustacean community as a whole. Additionally, we examined these relationships with a subset of key crustacean taxa (the large calanoid copepod Neocalanus plumchrus, hyperiid amphipods, euphausiids, and decapods), all of which feed directly on phytoplankton for at least a part of their life cycle, and are, historically, preferred prey items for juvenile salmon (Neville & Beamish, 1999; Daly et al., 2010; Preikshot et al., 2010).
Independent of the satellite-derived Chl a data, we used crustacean abundance data to conduct hierarchical clustering using the Unweighted Pair-Group Method with arithmetic Averages (UPGMA) to examine the similarity of crustacean community composition across study years. Years with fewer than three months of zooplankton data (2006, 2011, and 2012) were excluded from this part of the analysis. Mean abundance and biomass for each of the dominant crustacean taxa was then calculated for each cluster revealed by the cluster analysis and one-way analysis of variance (ANOVA) was used to test the differences in mean abundance and biomass among the cluster groups. Lastly, non-parametric Kruskal-Wallis tests were used to compare the environmental drivers between the different clustering groups. All statistical analyses were performed using Sigmaplot® version 13.0, R version 3.5.1 (R Development Core Team, 2018) and Python 3.9 with the Jupyter environment (Kluyver et al., 2016).
3 Results
3.1 Satellite-Derived Chl a Bloom Phenology Dynamics
Mean spring bloom initiation over the duration of this study (2003-2016) was end of March (DOY 89; Figure 2). Earlier than average blooms (>1 SD about the mean) occurred in both 2005 and 2015 when the spring bloom began around February 18 (week of DOY 49-56). An early bloom (middle of the 8-day week fell on the 1 SD line) also occurred in early March (between DOY 65 and 72) in 2004. In contrast, 2007 and 2008 had later than average (>1 SD above the mean) spring bloom initiation (end of April; between DOY 113 and 120). The highest spring bloom intensities were observed in 2015 (14.1 mg m-3) and in 2007 (10.7 mg m-3); however, bloom intensity often showed substantial variability within a given year. In contrast, the lowest spring bloom intensity was observed in 2008 (6.2 mg m-3; Figure 3A). Bloom conditions persisted throughout most of the spring in 2005 (90% of weeks were under bloom conditions) and >60% of weeks were under bloom conditions in 2006, 2009, and 2015. On the other hand, 2007 and 2008 exhibited the lowest proportion of weeks (~20%) under spring bloom conditions due to the late bloom initiation during those years (Figure 3A). Spring bloom magnitude, which considers both bloom intensity and the number of weeks under bloom conditions, was highest (>60.0 mg m-3 8-day) in 2005, 2006, 2009, and 2015 (Figure 3B). Conversely, the lowest spring bloom magnitudes were observed in 2003, 2008, and 2011 with values of <25.0 mg m-3 8-day.
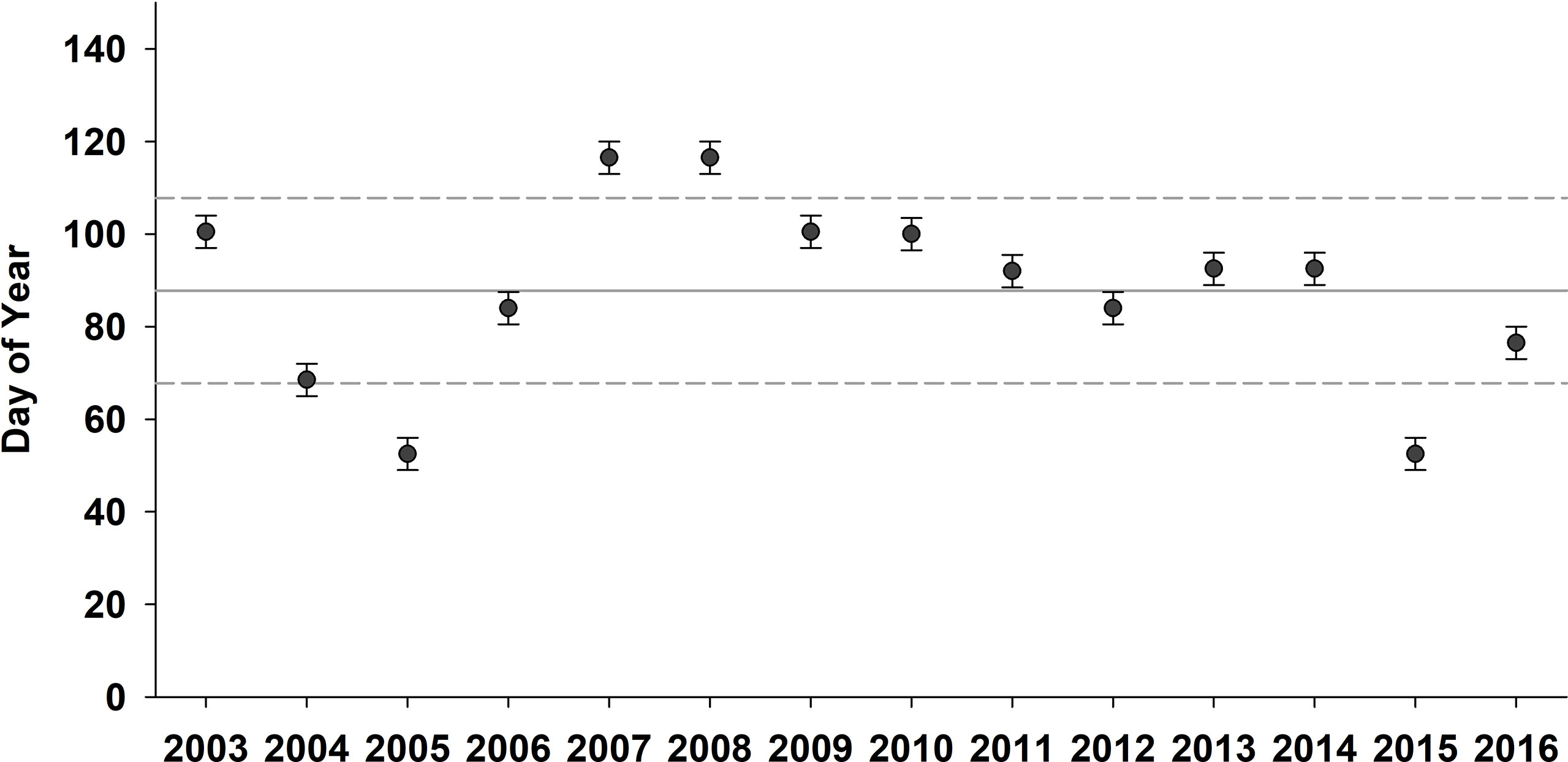
Figure 2 Satellite-derived spring Chl a bloom initiation from 2003-2016 in the Central Strait of Georgia, BC. Black dots indicate the middle of the 8-day week (day of year) with error bars representing +/- 3.5 days. Solid horizontal line is the mean bloom initiation date; dashed lines show the +/- 1 standard deviation used to define “early” vs. “late” blooms.
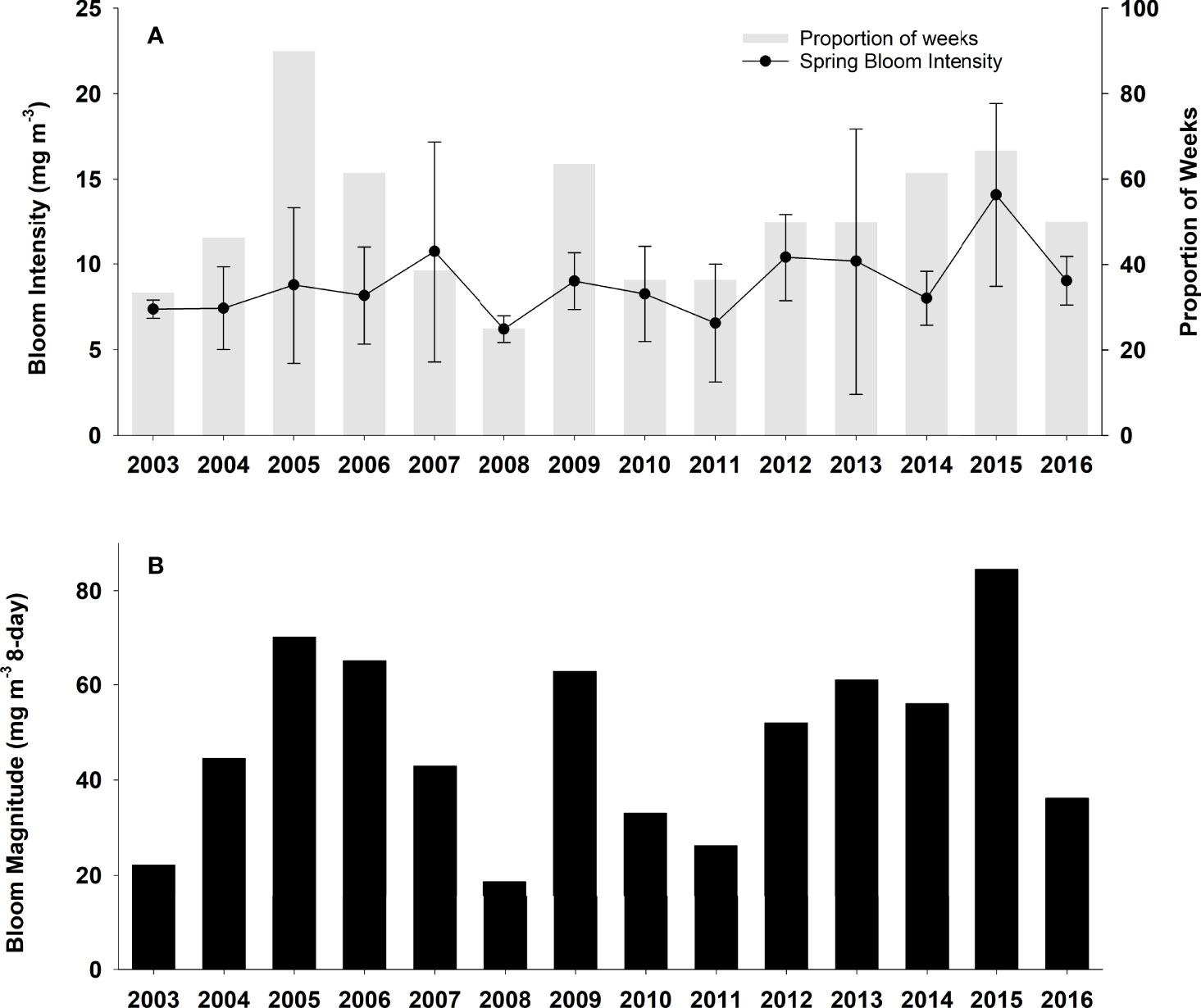
Figure 3 (A) Satellite-derived Chl a spring bloom intensity (line) and proportion of weeks under bloom conditions (grey bars) and (B) spring bloom magnitude (which considers the intensity plus the number of weeks in bloom conditions) from 2003 to 2016 in the Central Strait of Georgia, BC.
Monthly median Chl a climatology (2003-2016) peaked in April at 7.8 mg m-3 and remained on average > ~5.0 mg m-3 (range of 4.9 to 6.7 mg m-3) from May through to the end of November (Figures 4A, E). During early Chl a bloom years (2004, 2005, and 2015), an initial peak in Chl a was present at the beginning of our study period in February (11.2 mg m-3), followed by a secondary peak in April at 12.3 mg m-3 (Figures 4B, F). Average bloom years exhibited one main peak of Chl a (maximum value of 7.1 mg m-3) lasting from April through May (Figures 4C, G). In contrast, during late bloom years a Chl a peak was not observed until the beginning of May, remaining high (> 6.6 mg m-3) through to the end of July (Figures 4D, H). The highest Chl a concentrations during late bloom years were observed in Autumn (September to November; monthly median of > 8.0 mg m-3) and were likely due to exceptionally high Chl a concentrations that occurred in Autumn 2008 (see Suchy et al., 2019).
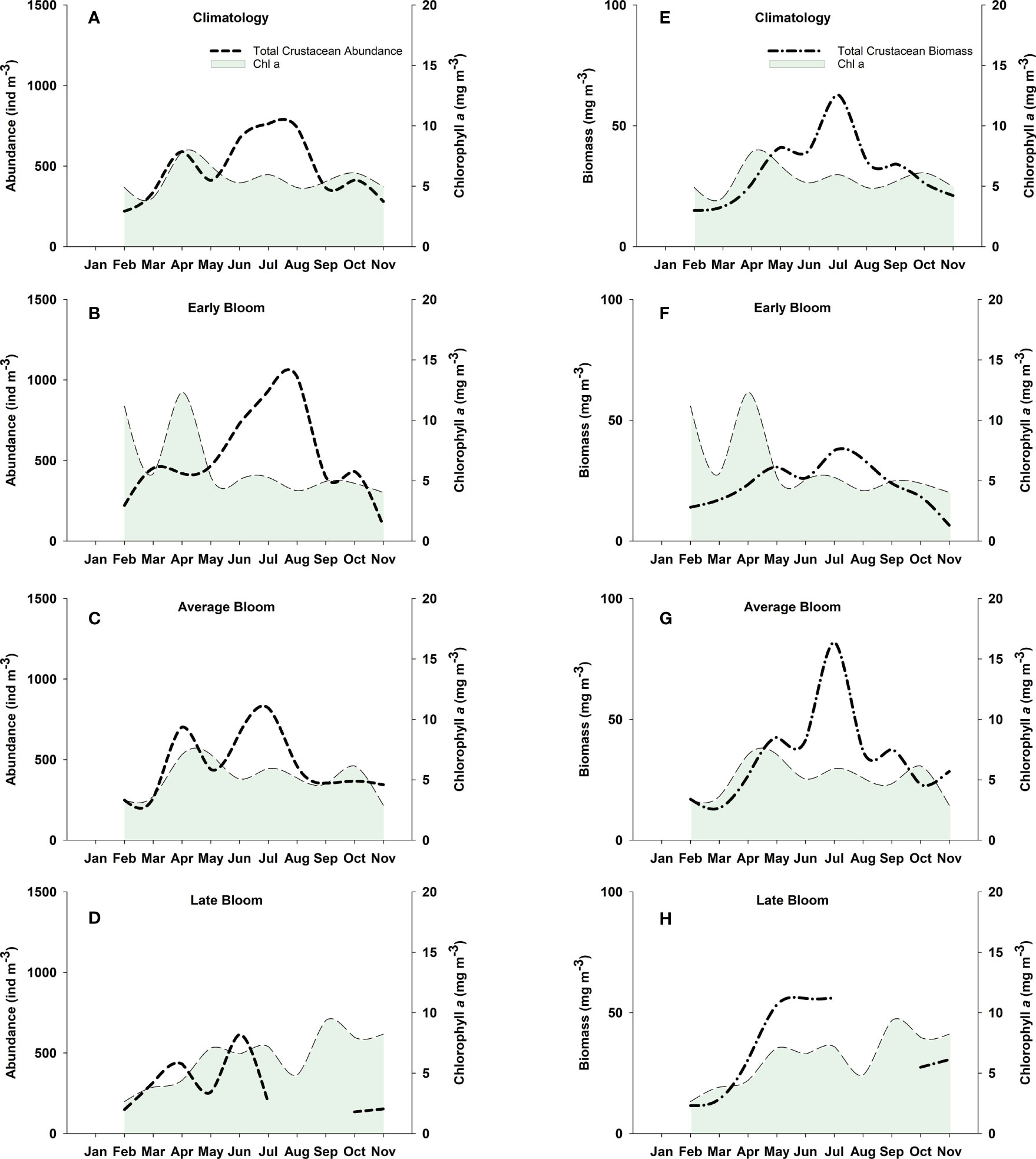
Figure 4 Monthly Chl a and total crustacean abundance (left panels) and biomass (right panels) during climatological years (2003-2016;A, E), and during early (2004, 2005, 2015; B, F), average (2003, 2006, 2009-2014, 2016;) (C,G), and late (2007, 2008; D, H) Chl a bloom years in the Central Strait of Georgia, BC.
3.2 Environmental Drivers of Chl a Bloom Dynamics
Individual relationships between bloom dynamics and environmental variables showed that spring bloom initiation was highly significantly correlated with NPGO (r = 0.75, p < 0.01) and SST (r = - 0.70, p < 0.01; Figure 5) meaning that earlier blooms occurred during warmer years in the SoG. When grouping previously-defined “cold” years (i.e., 2007-2012; see Suchy et al., 2019) and “warm” years (2003-2006 and 2013-2016) in the SoG, spring bloom initiation was significantly later during cold years (t-test, t = -2.7, df = 12, p = 0.009). In addition, spring bloom initiation was related to PAR (r = -0.56, p < 0.05) and wind (r = 0.54, p < 0.05), suggesting that increased light availability and weaker winds help to establish earlier bloom initiation in the SoG. Spring bloom intensity was positively correlated with stratification (r = 0.66, p < 0.05) and negatively related to SOI (r = -0.57, p < 0.05). Spring bloom magnitude was negatively related to both NPGO (r = -0.77, p < 0.01) and SOI (r = -0.65, p < 0.05), but showed no significant relationships with any of the local environmental variables. That said, spring bloom magnitude was significantly and negatively correlated with spring bloom initiation (r = -0.64, p < 0.05; Figure 5) meaning that higher spring bloom magnitudes occurred during years with the earliest blooms. The strongest correlations between local environmental variables and large scale climate indices were observed for SST and wind. SST was strongly correlated with PDO (r = 0.83, p < 0.01) and SOI (r = -0.72, p < 0.01), whereas wind was strongly correlated to NPGO (r = 0.74, p < 0.01) and PDO (r = -0.68, p < 0.01). When all environmental variables were considered together, forward selection stepwise regression revealed that NPGO best explained variations in spring bloom initiation (Adj R2 = 0.53, p < 0.01) and spring bloom magnitude (Adj R2 = 0.57, p < 0.01; Table 2). If large-scale climate indices were removed from the regression analysis for bloom initiation and only local environmental drivers were considered, spring bloom initiation was best explained by SST (Adj R2 = 0.44, p < 0.01; not shown). Spring bloom intensity was best explained by stratification (Adj R2 = 0.38, p < 0.05; Table 2).
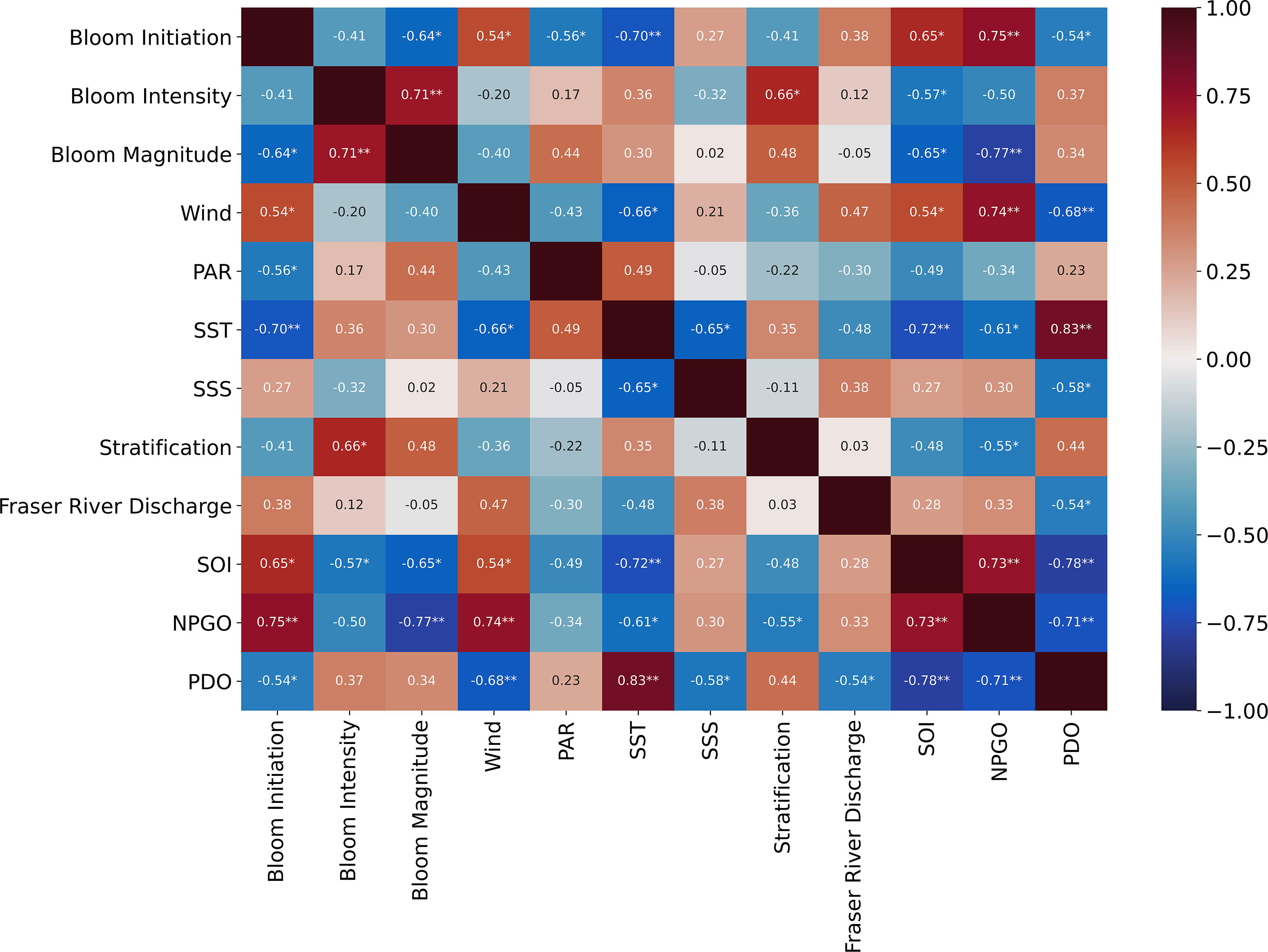
Figure 5 Heatmap showing Pearson Product Moment correlations between spring Chl a bloom dynamics (bloom initiation, bloom intensity, and bloom magnitude) and environmental drivers. Significant relationships at p < 0.05 are indicated with * and at p < 0.01 with **.
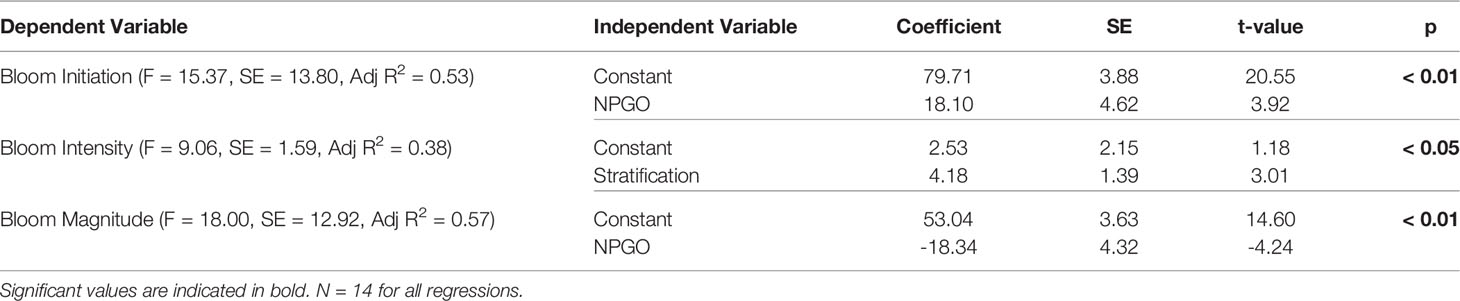
Table 2 Results of linear regressions and the significance of the models chosen by forward stepwise regression to describe the environmental variables influencing Chl a bloom dynamics.
3.3 Crustacean Zooplankton Phenology
The climatological (2003-2016) main peak in mean crustacean abundance was observed from June to August with a maximum peak of 763 individuals (ind) m-3 occurring in July (Figure 4A). A smaller peak (590 ind m-3) was observed in the climatology in April, which occurred slightly before the main peak in Chl a. The highest peak in mean crustacean abundance (1021 ind m-3), mostly driven by the high abundances observed in 2015, occurred in August during early Chl a spring bloom years (Figure 4B). During average Chl a bloom years, crustacean abundance exhibited peaks in April and July (702 and 821 ind m-3, respectively; Figure 4C). Mean crustacean abundance was substantially lower in late Chl a bloom years wherein an initial peak was observed from March to April, followed by a high peak (616 ind m-3) of short duration in June (Figure 4D); however, limited samples were available from July to October during the late Chl a bloom years of 2007 and 2008 (Table 1).
The initial peak in crustacean biomass for the climatology occurred in May (41.1 mg m-3; Figure 4E), approximately one month after the peak in Chl a. Following this, the main peak in biomass occurred in July (62.9 mg m-3). Average Chl a bloom years showed a similar pattern as was observed in the climatology, which is expected given that average bloom years comprised the majority of the years used in the climatology calculations (Figure 4G). Early Chl a bloom years resulted in the lowest crustacean biomass (maximum mean biomass of 37.5 mg m-3 in July; Figure 4F). Crustacean biomass during late Chl a bloom years peaked from May to July (maximum biomass of 56.1 mg m-3), corresponding to the peak in Chl a during the same time period (Figure 4H).
In general, crustacean zooplankton showed varying phenological responses to Chl a bloom timing. Shifts in the timing of peak abundance was most evident for euphausiids, which peaked in April during early Chl a bloom years, mid-April during average bloom years, and in early May during late Chl a bloom years (Figure 6). Euphausiid abundance was highest during average bloom years with a peak of 102 ind m-3 compared to maximum peaks of 34 ind m-3 and 28 ind m-3 during early and late bloom years, respectively. A substantially higher abundance (>640 ind m-3) of non-calanoid copepods was observed during July and August of early Chl a bloom years compared to average and late bloom years which had peaks of 367 and 167 ind m-3, respectively (Figure 6). Similarly, small calanoid copepods exhibited a slightly higher peak (72 ind m-3) in July during early Chl a bloom years. In contrast, there was a higher abundance of medium-sized calanoid copepods (e.g. Metridia spp) during average and late bloom years compared to early Chl a bloom years. No notable changes between early and average Chl a bloom years were observed for either large calanoid copepods or hyperiid amphipods; however, amphipod abundance was noticeably lower in late Chl a bloom years (Figure 6).
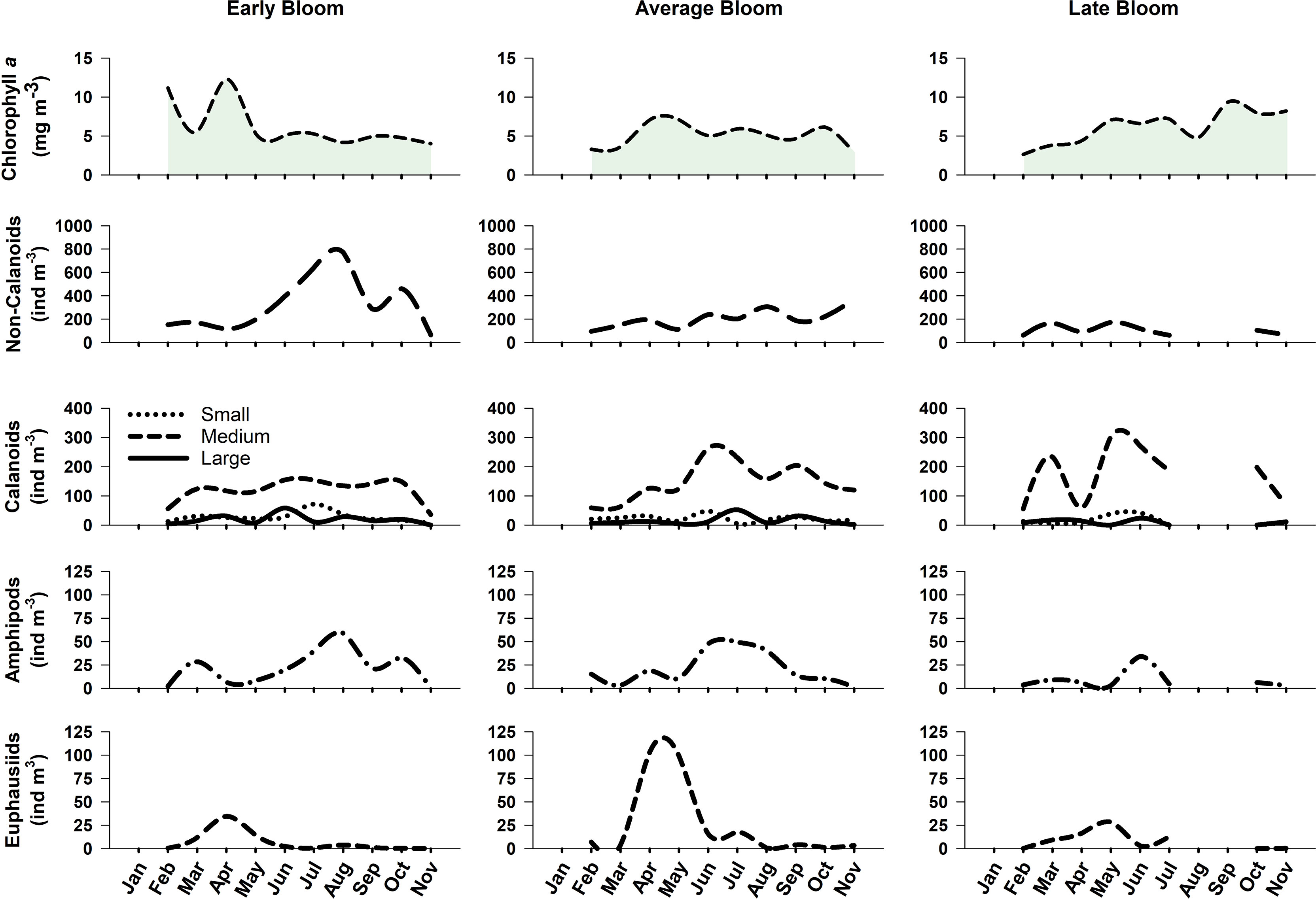
Figure 6 Total abundance (ind m-3) for non-calanoid and calanoid copepods, hyperiid amphipods, and euphausiids during early (2004, 2005, 2015; left panels), average (2003, 2006, 2009-2014, 2016; middle panels), and late (2007, 2008; right panels) Chl a bloom years in the Central Strait of Georgia, BC.
No significant relationships or trends were found between bloom phenology dynamics and mean annual crustacean abundance and biomass (Figure 7). However, of particular interest to this work were crustacean groups that act as a direct link between phytoplankton and higher trophic levels. Therefore, we examined these relationships with a subset of those crustacean taxa which feed directly on phytoplankton and are favoured dietary items of juvenile salmon (euphausiids, N. plumchrus, hyperiid amphipods, and decapod larvae). Results from this analysis revealed a nearly significant positive relationship between spring Chl a bloom initiation and mean annual crustacean biomass, i.e., the biomass of these key crustacean taxa tended to be higher with later bloom start dates (r = 0.50, p < 0.07; Figure 8B); however, no relationship was found between Chl a bloom initiation and the mean annual abundance of these key taxa (r = -0.22, p = 0.44; Figure 8A).
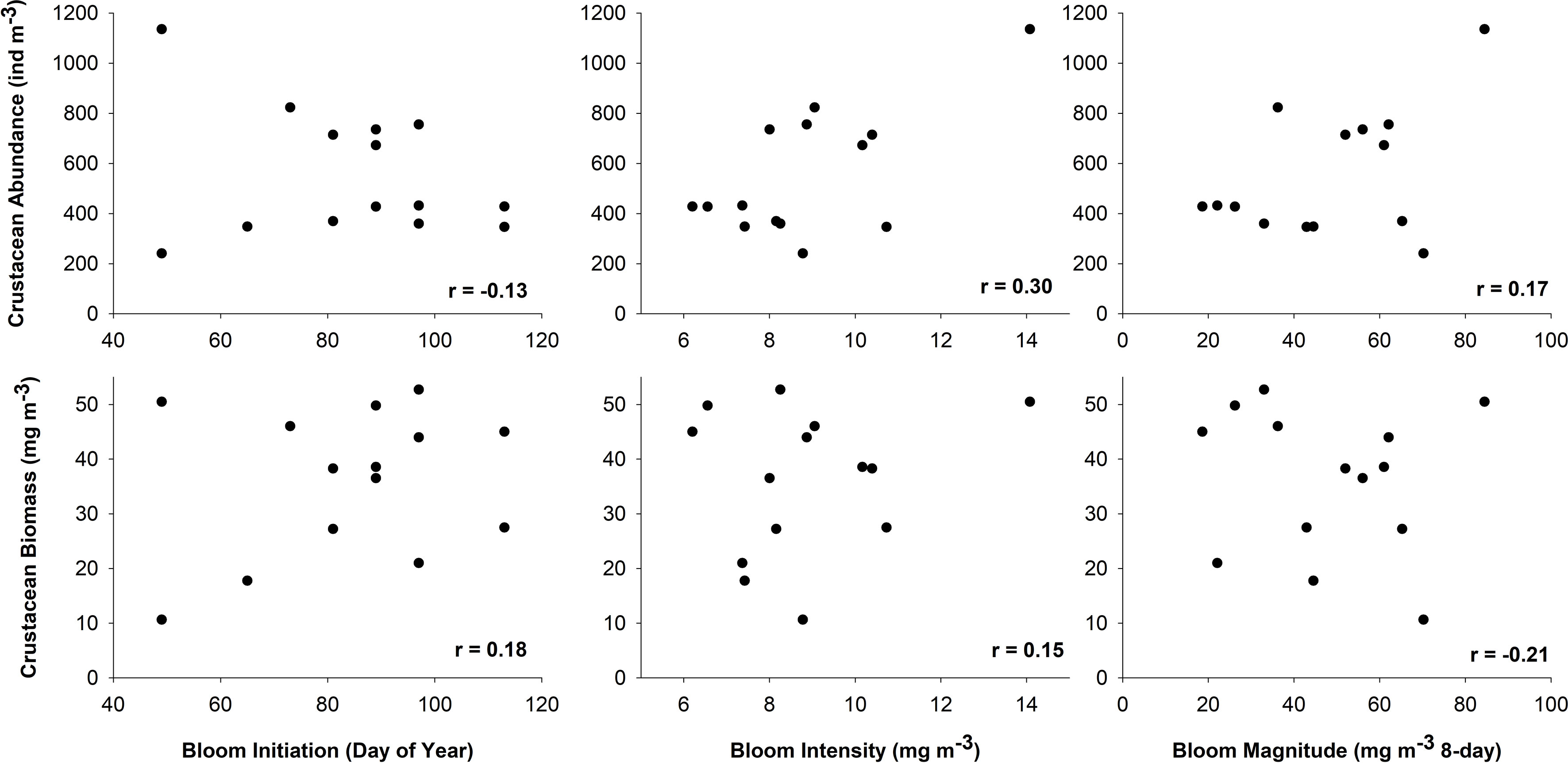
Figure 7 Relationships between annual abundance (top panels) and biomass (bottom panels) of crustacean zooplankton and bloom phenology dynamics from 2003-2016 in the Strait of Georgia, BC.
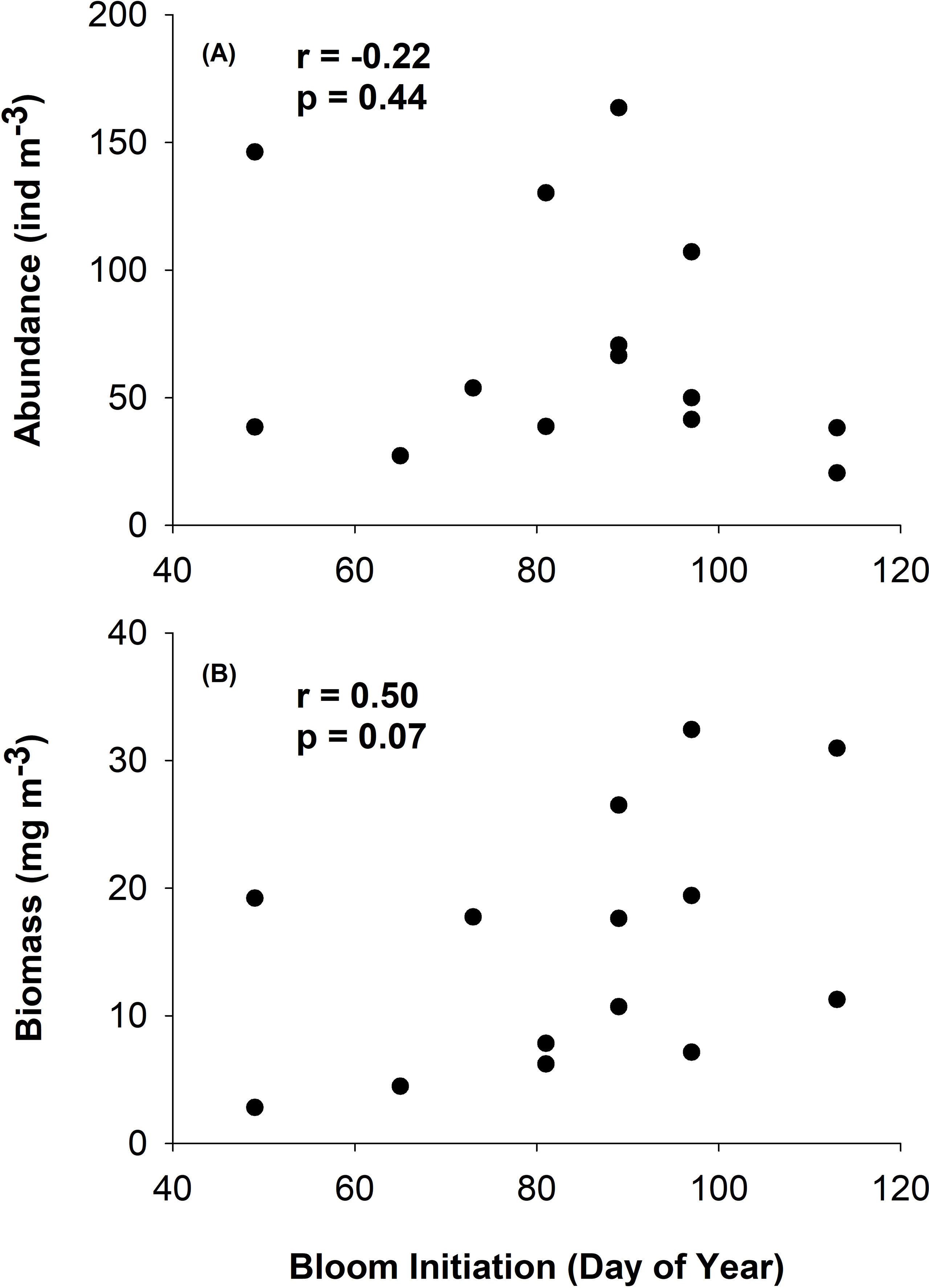
Figure 8 Relationship between annual abundance (A) and biomass (B) of key crustacean taxa (i.e., groups that constitute major dietary items for juvenile salmon which also feed directly on phytoplankton; euphausiids, hyperiid amphipods, decapods, and the large calanoid copepod Neocalanus plumchrus) and spring bloom start date in the Central Strait of Georgia, BC.
3.4 Crustacean Community Composition
Hierarchical clustering analysis revealed three main crustacean abundance groups (Figure 9). Group 1, the main group, included all of the “average” bloom years as defined by the satellite-derived Chl a bloom phenology, as well as 2008. Group 2 included 2004, 2005, and 2015, all of which were defined as the “early” Chl a bloom years. Lastly, 2007, one of the “late” Chl a bloom years, was separated from the other years into Group 3.
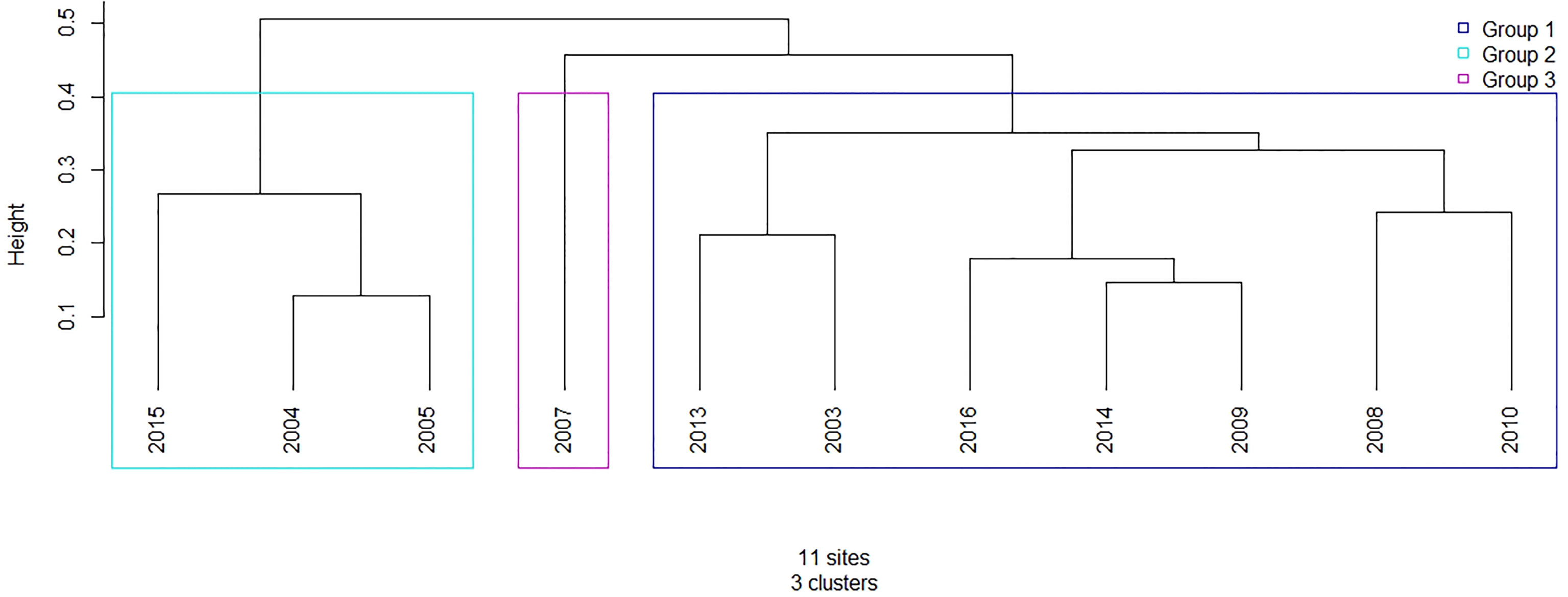
Figure 9 Cluster dendrogram for the Central Strait of Georgia crustacean community abundance from 2003-2016 using the Unweighted Pair-Group Method using the arithmetic Averages (UPGMA) method for hierarchical clustering. Years with fewer than 3 months of data (2006, 2011, 2012) were excluded from analysis.
When examining mean abundance and biomass for the crustacean taxa in each of the groups identified by the cluster analysis, mean crustacean abundance was comparable for Groups 1 and 2 (609.0 and 574.0 ind m-3, respectively) whereas mean abundance for Group 3 was substantially lower at 347.0 ind m-3, possibly due to a lack of August and September samples which may have biased the mean abundance values low (Figure 10). Non-calanoid copepods were more abundant in the years associated with Group 2 (i.e., early Chl a bloom years; Figure 10). Group 1, which corresponded with average Chl a bloom years, had a slightly higher abundance of medium-sized calanoid copepods and larger crustaceans, e.g. euphausiids and amphipods, compared to Group 2. Furthermore, euphausiids and amphipods were more abundant in Groups 1 and 2 compared to Group 3. As a result, mean crustacean biomass was highest in Group 1, i.e., 40.3 mg m-3 compared to 26.1 and 27.7 mg m-3 observed for Groups 2 and 3, respectively. The high biomass in Group 1 compared to the other groups was particularly evident for euphausiids, decapods, and large calanoid copepods (Figure 10). No significant relationships were found between cluster groups for total abundance, total biomass, or any of the individual taxa, with the exception of small calanoid copepods which was significantly different between the three cluster groups [one-way ANOVA, F(1,8) = 8.03, p = 0.02]. However, the power of these analyses was often below the desired power of 0.80 due to the small number of years in Groups 2 and 3, which potentially impacted our ability to detect statistically significant differences between groups.
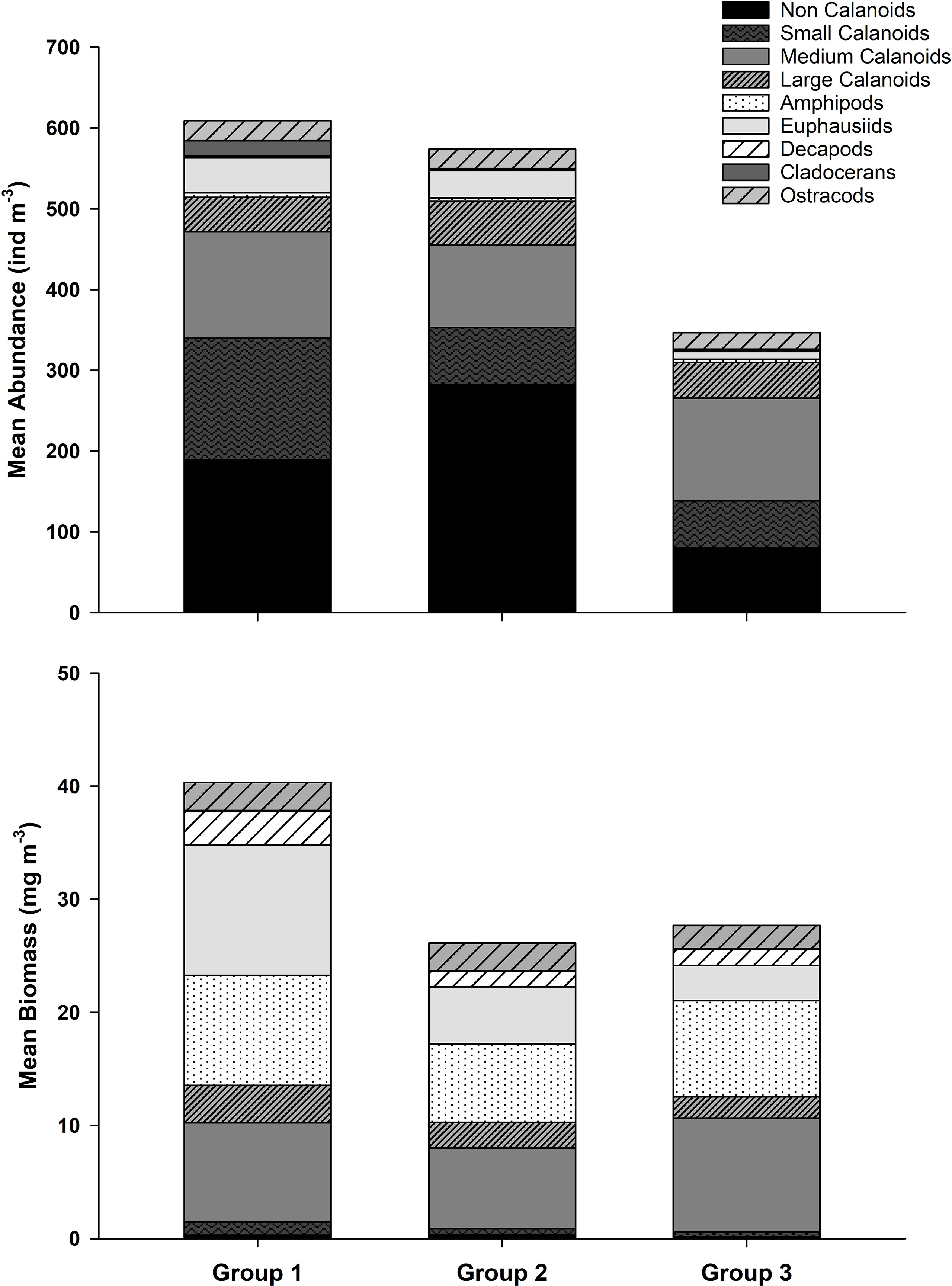
Figure 10 Mean abundance and biomass of the main crustacean taxa in Groups 1, 2, and 3 from the clustering analysis for the Central Strait of Georgia from 2003-2016. Years with fewer than 3 months of data (2006, 2011, 2012) were excluded from the analysis.
3.5 Environmental Drivers of Crustacean Community Composition
A comparison of large-scale climate indices, environmental drivers, and Chl a bloom phenology dynamics between the years grouped in the cluster analysis revealed that the spring Chl a bloom start date was the only variable significantly different between the three groups (Kruskal-Wallis rank sum test, chi-squared = 7.16, df=2, p < 0.03; Figure 11). Chl a bloom initiation occurred earlier during years associated with Group 2, followed by Group 1, then Group 3. Given that only 11 years were used in this part of the analysis, we also note variables that were significantly different between cluster groups at alpha = 0.10. Specifically, SOI (Kruskal-Wallis rank sum test, chi-squared = 6.03, df=2, p < 0.05, PAR (Kruskal-Wallis rank sum test, chi-squared = 5.63, df=2, p < 0.06, and wind (Kruskal-Wallis rank sum test, chi-squared = 5.63, df=2, p < 0.06) were all significantly different between the three groups identified in the cluster analysis. These results suggest that differences in crustacean community composition were associated with spring Chl a bloom start date, negative values of SOI (warm phase conditions), as well as the with key variables responsible for bloom initiation (light availability and wind).
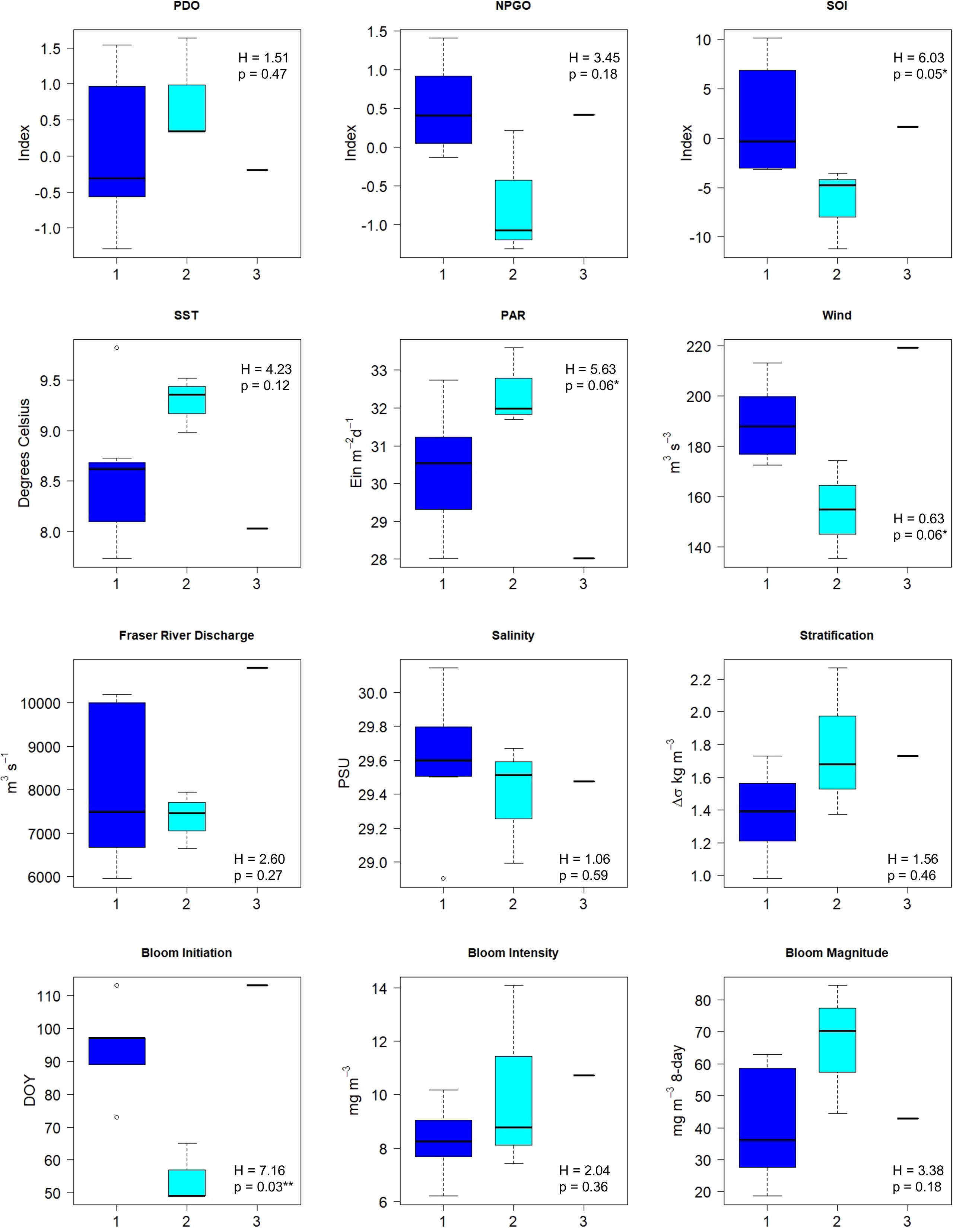
Figure 11 A comparison of large-scale climate indices, environmental parameters, and bloom dynamics between clustering groups for the Central Strait of Georgia crustacean community from 2003-2016. Group 1= dark blue boxplots, Group 2= light blue boxplots, Group 3= black lines representing a single year. P-values and H-statistics were determined using non-parametric Kruskal-Wallis test. D.f. = 2 for each test; **=significant at alpha=0.05, *=significant at alpha=0.10.
4 Discussion
4.1 Satellite-Derived Chl a Bloom Dynamics
4.1.1 Spring Bloom Timing
Spring bloom initiation throughout our study (2003-2016) occurred, on average, during the last week of March (DOY 89; middle of the 8-day week). These results differ slightly from Schweigert et al. (2013) who used SeaWiFS level 3 data from 1997-2010 to show that satellite-derived bloom initiation, defined as either the first annual date when two consecutive mean Chl a values were greater than the annual median + 5% or the first date when a selected threshold of 6 mg m-3 occurred, for the entire SoG typically occurred between DOY 60 and 80. However, our results were well within the spring bloom timing (defined as the peak in chlorophyll biomass) of mid-March to mid-April in the Central SoG identified by Bornhold (2000). Furthermore, Jackson et al. (2015) showed that Chl a climatology typically begins to increase in March in the SoG, however a peak in Chl a was not observed in their study until May, possibly due to the fact that they considered the entire SoG region as a whole, over different climatological years (1997-2010), using different satellite data and processing methods. Our results were also consistent with the long-term mean spring bloom start date (defined as the maximum phytoplankton concentration when nitrate was depleted) in the Central SoG of March 25 (DOY 84) determined by a one-dimensional biophysical model (Allen and Wolfe, 2013) despite the fact that our study considered a larger spatial scale (i.e., the entire Central SoG instead of a single sampling station) and a different range of years (2003-2016 in our study compared to 1968-2010 presented in Allen and Wolfe, 2013). Similarly, Peña et al. (2016) used a three-dimensional coupled biophysical model to determine that the spring bloom typically occurred between April 2 and 15, only slightly later than what we report in our study. Although Peña et al. (2016) also defined the spring bloom as the peak in phytoplankton biomass, and not initiation per se, their model did reveal that phytoplankton biomass begins to increase in February and typically reaches concentrations > 5 mg m-3 in March, consistent with our findings for bloom initiation. Whereas the previous examples are consistent with spring phytoplankton bloom timing in the Central SoG being around the end of March, they differ in their details, in part because they used different metrics (i.e., bloom initiation, peak in phytoplankton biomass, initial drawdown of nutrients, etc.), over different spatial and temporal scales, even within the same relatively small study region.
Despite the various methods used to characterize bloom timing in the SoG, the “early” (late February/early March) Chl a bloom years defined by this study (2004, 2005, 2015) were corroborated by results from other studies. In situ surface Chl a samples previously determined that the 2005 bloom began during the third week of February in the Central SoG (Halverson and Pawlowicz, 2013). In addition, 2005 and 2015 were designated as early bloom years in the Central SoG based on high resolution surface measurements of chlorophyll fluorescence from ferry box sampling (Sastri et al., 2016) and a coupled bio-physical model (Collins et al., 2009; Allen et al., 2016). In fact, 2015 was found to be the second earliest bloom (the earliest being late February 2005) as measured by the ferry systems in the Central SoG since 2001 (Sastri et al., 2016). The “late” bloom years defined in this study (2007, 2008) did not always agree well with previous studies. For example, observation data of in situ Chl a indicated that the bloom in 2007 occurred between April 2-8 (DOY 92-98; Sastri et al., 2016), whereas modeled bloom timing for 2007 revealed a slightly later bloom data of April 10 (DOY 100; Allen and Wolfe, 2013), which was closer to our observed value of the week beginning with DOY 113 (end of April). In contrast, coarser resolution (standard SeaWiFS and MODIS) satellite-derived chlorophyll data from 1997-2010 suggested an early bloom (February-March) in 2007 (Thomson et al., 2012); however, they noted that the 2008 bloom was delayed relative to their observed early bloom of 2007. Similarly, Gower et al. (2013) determined relatively early but more localized Chl a blooms in both 2007 (mid-February) and 2008 (mid-March) using the fluorescence line height approach for MODIS satellite imagery. Discrepancies in results between this study and other satellite-based studies may be due to the use of region-specific algorithms for atmospheric correction and the models used to retrieve chlorophyll data in the present study (see Carswell et al., 2017; Suchy et al., 2019).
4.1.2 Environmental Drivers of Bloom Dynamics
A detailed discussion of environmental drivers of phytoplankton variability in the SoG based on the same satellite-derived Chl a data used in this study is presented in Suchy et al. (2019). Results from this previous work suggested that local drivers (i.e., Fraser River discharge), rather than large-scale climate indices, influence spatial and temporal variability of chlorophyll concentrations in the Central SoG. However, examination of the environmental drivers of Chl a bloom dynamics in this study, as opposed to chlorophyll concentrations per se, revealed that spring Chl a bloom initiation was strongly positively related to NPGO and SOI and negatively correlated with SST and PDO (Figure 5); warmer years tended to have earlier blooms whereas colder years exhibited average or late blooms. This general pattern of early initiation of the spring bloom associated with warmer SST has been found in numerous other studies (e.g., Sasaoka et al., 2011; Henson et al., 2013; Zhao et al., 2013; Hunter-Cevera et al., 2016). In contrast to our results, Allen & Wolfe (2013) found no significant relationship between modeled spring bloom date and temperature in the Central SoG; yet their results did show a weak relationship between bloom initiation and NPGO (r = 0.36, p = 0.05), which supports our findings. The warm phase conditions (-NPGO, -SOI, +PDO; Suchy et al., 2019) observed during early bloom years in the SoG were also associated with increased light levels (PAR) and weaker winds during late winter/early spring, both of which are known to impact bloom initiation in the SoG (Yin et al., 1997; Collins et al., 2009; Allen & Wolfe, 2013).
Warm-phase conditions may have direct and indirect effects on phytoplankton. For example, higher SST can result in earlier blooms if increased temperatures also act to increase the photosynthetic (Henson et al., 2006) or cell division (Hunter-Cevera et al., 2016) rates of phytoplankton cells. Alternatively, warmer atmospheric temperatures may indirectly impact Chl a concentrations during the spring by causing earlier snowmelt and increased freshwater runoff, resulting in increased water column stratification (Yin et al., 1997; Foreman et al., 2001; Ji et al., 2007; Suchy et al., 2019). Stratification in the SOG typically peaks in the summer (June or July) coincident with the peak in Fraser River discharge (Suchy et al., 2019). Chiba et al. (2008) found that warmer conditions during the winter in the Oyashio region resulted in strong stratification in the spring, which prompted earlier bloom timing. We found no significant relationships between spring bloom initiation and either SSS, Fraser River discharge, or stratification; however, spring bloom intensity, the average Chl a concentration over weeks under bloom conditions, was significantly related to stratification (Adj R2 = 0.38, p < 0.05; Table 2). These results further support our previous findings that increased stratification in the SoG during late winter/early spring as a result of warm-phase conditions contributes to early bloom formation and high Chl a concentrations compared to colder years (Suchy et al., 2019). Furthermore, warmer years with earlier Chl a blooms subsequently resulted in a higher spring bloom magnitude, a finding that has previously been reported for both the Grand Banks of Newfoundland (Zhao et al., 2013) the US Northeast Shelf (Friedland et al., 2015), the North Atlantic (Friedland et al., 2016), as well as on a global scale (Racault et al., 2012; Friedland et al., 2018). While no direct relationship was found between SST and bloom magnitude in our study, variability in spring bloom magnitude (our proxy for bloom duration) was best explained by NPGO (Adj R2 = 0.57, p < 0.01; Table 2). Specifically, years associated with -NPGO (warmer years) were characterized by weaker spring winds (Figure 5), likely allowing blooms to persist for a longer duration, and thus resulting in the observed higher bloom magnitudes.
4.2 Phytoplankton and Zooplankton in the Central Strait of Georgia
4.2.1 Climatological/Average Bloom Years
The climatological seasonal zooplankton cycle in the SoG is known to have a late-spring to late-summer peak and a winter/early-spring minimum (Mackas et al., 2013). Our results for the climatology (and average Chl a bloom years) showed that the maximum peaks in both abundance and biomass of total crustaceans occurred in July (Figure 4). The initial peak in crustacean abundance, however, occurred just prior to April and at the same time as the first peak in Chl a. This initial peak in crustacean abundance is likely attributed to the high numbers of juvenile crustaceans (e.g., euphausiids, copepod nauplii, and the copepodite stages included in the medium calanoid copepod grouping), which feed predominantly in the upper water column in response to rapid increases in phytoplankton. Euphausiid development cues are known to be strongly coupled with phytoplankton biomass (Ross & Quetin 2000) and their reproductive cycles have previously been shown to be triggered by episodic phytoplankton blooms (Ji et al., 2010). Results from our study indeed revealed a tight coupling between euphausiid abundance and Chl a biomass, wherein the peak timing of euphausiids corresponded to the main peak in Chl a regardless of Chl a bloom timing (Figure 6). Furthermore, there was an initial smaller peak in total crustacean biomass in May (Figure 4E), which is consistent with the findings from previous studies in this region that determined zooplankton biomass typically peaks about one month after the maximum phytoplankton biomass is reached (e.g., Stockner et al., 1977; Peña et al., 2016).
The seasonal patterns we observed in monthly median Chl a concentrations varied between early, average, and late Chl a bloom years. While our results indicate that bloom concentrations during late Chl a bloom years were associated with colder temperatures, lower light levels and stronger winds (which can cause extensive vertical mixing), zooplankton grazing may also disrupt or delay phytoplankton blooms. As such, the timing and composition of the zooplankton community is an important factor contributing to Chl a bloom dynamics given that high abundances of zooplankton grazers prior to a bloom initiation may inhibit its development (Parsons et al., 1966; Siegel et al., 2002). Below we discuss the synchrony in phytoplankton and zooplankton phenology during early and late Chl a bloom years in order to better understand what constitutes a match vs. mismatch year for lower trophic levels in the Central SoG.
4.2.2 Early Bloom Years
Median monthly Chl a concentrations were > 5 mg m-3 from February through April during early bloom years with a notable decrease in Chl a concentrations during March (Figures 4B, F). A decline in Chl a concentrations in the spring bloom in the SoG was previously shown to coincide with increased zooplankton biomass during a time when surface nitrate was still available, thus indicating that grazing, as opposed to nutrient limitation, was the cause of the decline (Peña et al., 2016). We suspect that this may be the case during the early bloom years in this study as the decline in Chl a in March coincided with an increase in the abundance of non-calanoid copepods, medium calanoid copepods, and amphipods that resulted from an earlier shift in the initial peak abundances of these groups (Figure 6). Variability in cohort timing of N. plumchrus, the copepodite stages of which are included in our medium calanoid copepod group, is known to be positively correlated with spring surface temperature anomalies in the Northeast Pacific (Mackas et al., 2012). Mackas et al. (1998) previously observed that the peak zooplankton biomass during “warm”, “normal”, and “cold years” at Ocean Station Papa in the North Pacific was distinctly early (and even higher) during years when the surface layers were anomalously warm. Our results support these findings and suggest that the shift in timing of herbivorous species may have contributed to the observed decrease in Chl a biomass during the spring of early years. While early bloom years were associated with the highest crustacean abundance observed in our study (> 1000 ind m-3 during August; Figure 4B), this high abundance was mainly attributed to smaller-bodied non-calanoid copepods (Figures 6, Figure 10). Overall, the low biomass values observed during these years suggest that early Chl a blooms cause a mismatch for phytoplankton and larger crustacean zooplankton in the Central SoG.
4.2.3 Late Bloom Years
Chl a concentrations remained < 5 mg m-3 from February through April during late bloom years and were subsequently higher (5 to 10 mg m-3) during summer and autumn compared to early and average bloom years (Figures 4D, H). Somewhat surprisingly, the earliest peak in total crustacean biomass during our study was observed during May to June for late Chl a bloom years (Figure 4H) coinciding with the time period when Chl a concentrations reached > 5 mg m-3. This main peak in crustacean biomass during late Chl a bloom years was lower, but of a longer duration, relative to average bloom years. Friedland et al. (2015) suggested that grazing pressure of zooplankton on phytoplankton may be higher during late bloom years when zooplankton populations have had sufficient time to develop prior to the bloom start, thus resulting in smaller Chl a blooms. Total crustacean abundance initially peaked in March during late bloom years in our study and was largely driven by a high abundance of medium-sized calanoid copepods (Figure 6). Therefore, it is possible that late Chl a blooms may have experienced heavier grazing pressure by zooplankton already present in the surface waters, thus preventing Chl a concentrations from increasing during the spring of those years (Siegel et al., 2002; Zhao et al., 2013). That said, even though crustacean abundance was lowest during late Chl a bloom years (Figure 4D), crustacean biomass was still relatively high when 2007 and 2008 were considered together (maximum biomass of 56.1 mg m-3; Figure 4H). Our results are in contrast to a study from nearby Rivers Inlet, BC, which found that the phenology of five main copepod species was delayed when the spring bloom was later (Tommasi et al., 2021); however, the authors note that phenological responses to environmental variables may be region-specific. In addition, our study considers larger taxonomic groupings and a longer time period (i.e., 14 years compared to the 3 years of observations in Tommasi et al., 2021). It is also important to mention that while our satellite-derived metrics defined both 2007 and 2008 as late Chl a bloom years, these years showed marked differences in both spring bloom intensity and magnitude, which were higher in 2007 compared to 2008 (Figure 3B), as well as in crustacean abundance and biomass, which was lower in 2007 relative to 2008 (Figure 8). Furthermore, the lack of summer samples in 2007 and 2008 may have biased our zooplankton-related phenology results for late Chl a bloom years and likely resulted in lower annual crustacean abundance and biomass estimates than would be observed with higher temporal sampling resolution.
4.3 Crustacean Community Composition
4.3.1 Cluster Analysis
One of the most notable findings of this study came from the clustering analysis of crustacean community composition. Our hierarchical clustering results based on crustacean abundance data, alone, clearly separated zooplankton communities associated with early bloom years (Group 2; 2004, 2005, 2015; Figure 9) as defined by the satellite-derived Chl a bloom phenology. In contrast, the main group (Group 1) included average Chl a bloom years, whereas Group 3 separated out the late bloom year of 2007 (but not 2008). Analysis of the crustacean taxa comprising each of these groups revealed a higher proportion of non-calanoid copepods in the years associated with Group 2 (i.e., early Chl a bloom years). These results are somewhat expected given that higher temperatures, associated with the early bloom years in this study, tend to favour smaller zooplankton taxa in the SoG (Mackas et al., 2013). However, smaller copepods such as non-calanoid and small calanoid copepods are not an ideal food item for juvenile salmon (Preikshot et al., 2010), which suggests that early bloom years may negatively impact the quality of zooplankton prey available for higher trophic levels.
In contrast, average Chl a bloom years associated with Group 1 had a slightly higher relative abundance of medium calanoid copepods and larger crustaceans, e.g. euphausiids and amphipods, resulting in the highest overall biomass (40.3 mg m-3) of all three groups (Figure 10). This higher proportion of large crustaceans, particularly large-bodied copepods with higher lipid content, is indicative of better food quality available for higher trophic levels (Preikshot et al., 2010; Mackas et al., 2013). As was observed by Tommasi et al. (2013), we observed a shift in community composition resulting in a substantially lower abundance of euphausiids during the late bloom year of 2007 (Figure 10). Coupled with the fact that overall crustacean abundance was substantially lower in 2007, this contributed to low overall biomass values for Group 3 that were comparable to the low biomass values observed during the early Chl a bloom years comprising Group 2 (26.1 and 27.7 mg m-3 for Groups 2 and 3, respectively). However, given our poor sampling resolution in 2007, any potential impacts of crustacean zooplankton as food, in terms of both quantity and quality, on higher trophic levels during late Chl a bloom years, remains unclear.
4.3.2 Environmental Drivers of Crustacean Community Composition
Previous studies have extensively examined the link between variations in zooplankton anomalies in relation to both large-scale climate indices and local factors in the SoG (Li et al., 2013; Mackas et al., 2013; Perry et al., 2021). Mackas et al. (2013) determined that zooplankton biomass from 1990-2010 was strongly related to NPGO, particularly on decadal scales; however, the authors highlighted that relationships between environmental variables and individual zooplankton taxa are often weak and confounded by the presence of multicollinearity amongst the environmental variable themselves in this region. More recently, Perry et al. (2021) found that zooplankton biomass anomaly trends from 1996-2018 were related to sea surface salinity, PDO, and peak spring phytoplankton bloom date. Given the consideration of these relationships elsewhere, we focused instead on determining the environmental variables associated with changes in crustacean community composition by comparing large-scale climate indices, environmental drivers, and bloom dynamics between the years grouped in our zooplankton cluster analysis. Results from this analysis indicated that spring bloom initiation was significantly different between clustering years (p < 0.05; Figure 11). In addition, SOI and two key variables associated with phytoplankton bloom formation, PAR and wind, were significantly different between clustering years at alpha = 0.10. Negative SOI values (warm-phase El Niño conditions), weak winds, and high PAR values likely contributed to early Chl a bloom initiation and were associated with the crustacean communities clustered into Group 2 (years 2004, 2005, and 2015; Figure 11). Previous studies have linked SOI to changes in the phytoplankton community (an increase in heterotrophic and autotrophic dinoflagellates) (Pospelova et al., 2010), the zooplankton community (Haro-Garay and Soberanis, 2008; Li et al., 2013), as well as to salmon production in the SoG (Beamish et al., 1997). The main El Niño events (negative values of SOI) during this study occurred from 2003 to mid-2005, and then again from mid-2014 to 2016 (Suchy et al., 2019), thus encompassing the early Chl a bloom years as defined by both satellite-derived metrics and zooplankton cluster analysis. Further evidence of these warm-phase conditions is revealed by the observed differences in NPGO and SST (non-significant) between the three cluster groups (highest SST and lowest NPGO values during years associated with Group 2; Figure 11).
Warming conditions are known to result in a shift in phytoplankton community composition, ultimately resulting in smaller size classes of zooplankton (Richardson, 2008). For example, El Niño events have been shown to result in earlier spring blooms (Yin et al., 1997; Yoo et al., 2008; Sasaoka et al., 2011) and may result in a shift of the phytoplankton assemblage to smaller phytoplankton cells (nanoflagellates; Harris et al., 2009). In addition, shifts from diatom-dominated to dinoflagellate-dominated communities have been linked to negative NPGO periods in the California Current System (Fischer et al., 2020). Although phytoplankton community composition data were not available during the years of our time series, HPLC data previously analyzed for this region showed that there was a higher proportion of smaller phytoplankton cells (i.e., haptophytes) compared to diatoms in the Central SoG during spring of the early bloom year 2015 (Nemcek et al., 2020). In terms of zooplankton, Haro-Garay & Soberanis (2008) determined that the low abundances of N. plumchrus and E. pacifica during the 1997 El Niño year were attributed to an early spring bloom which supports our results. Given that we have previously shown that El Niño years are associated with high Fraser River discharge in the SoG (Suchy et al., 2019), it is plausible that warmer conditions, increased stratification, and potentially higher nutrient limitation, may result in a shift in both phytoplankton and zooplankton sizes towards smaller species (Kamykowski and Zentara, 2005; Richardson, 2008) during warm-phase El Niño conditions. Future data are needed in order to fully elucidate these shifts by comparing both the phytoplankton and zooplankton communities simultaneously, as well as the nutrient concentrations, during El Niño versus La Niña years in the SoG. To our knowledge, this study provides the first evidence linking earlier spring bloom timing to a shift in the crustacean community towards smaller taxa in response to multiple warm-phase events in the SoG. In contrast, strong winds and low PAR values during the cold-phase La Niña year of 2007 resulted in the late spring bloom start date associated with Group 3. Previous analyses of zooplankton community variability from 1990-2010 in the SoG (including a portion of the data used in the present study) also indicated that 2007 was the largest outlier in zooplankton ordinations, and that the low zooplankton biomass during this year was related to strong winter winds (Mackas et al., 2013). Furthermore, Perry et al. (2021) determined that there was a significant shift in zooplankton trends between 2006 and 2007 over the time period 1996 to 2018. Therefore, results from these longer-term studies support our findings that the late Chl a bloom year of 2007 had anomalously low crustacean biomass despite our lack of summer zooplankton sampling.
4.4 Implications for Higher Trophic Levels
Our results partially support the findings of Schweigert et al. (2013) who determined that the earliest and latest phytoplankton bloom initiation dates were associated with smaller herring year classes in the SoG by providing evidence of a potential shift to smaller crustacean zooplankton taxa of poorer food quality during early Chl a bloom years. Previous studies have shown that variations in zooplankton timing may impact the amount of energy available for fish and seabird predators in the NE Pacific (Mackas et al., 2007). Variability of zooplankton biomass has been shown to be an important contributing factor to poor salmon recruitment within the SoG (Mackas et al., 2013) and interannual variations in zooplankton have recently been linked to early marine survivals of Chinook, and returns of Coho salmon, in the region (Perry et al., 2021). Moreover, age-0 herring condition in the SoG has been shown to increase when adult herring spawning closely matches the peak spring phytoplankton bloom, suggesting that feeding condition during this time would better match peaks in the availability of larger zooplankton prey (Boldt et al., 2019). Although no relationships were found between the annual total crustacean abundance or biomass and spring bloom dynamics (Figure 7), our results did show an important, positive relationship (r = 0.50, p = 0.07; Figure 8) between spring bloom start date and the mean annual biomass of the key crustacean taxa relevant to juvenile salmon diets, i.e., the biomass of these key taxa was higher during later Chl a bloom years.
While these results further suggest that early bloom years are less favourable in terms of providing ideal feeding conditions for higher trophic levels, there were two years that stood out as notable exceptions to the general trend observed. For example, unlike other early Chl a bloom years, 2015 exhibited relatively high crustacean biomass throughout the year (Figure 8), coupled with the strongest bloom intensity observed throughout the study period (Figure 3A). In contrast, the mean biomass of the key crustacean groups was relatively low during the late bloom year of 2007 compared to the other late Chl a bloom year 2008. These results suggest that late Chl a blooms may result in a match scenario for at least some of the historically-preferred juvenile salmon dietary groups (e.g., hyperiid amphipods, large calanoid copepods, and decapod larvae). Furthermore, late Chl a bloom years exhibited a high abundance of medium-sized calanoid copepods (Figure 6), suggesting a potential match for predators such as juvenile herring which preferentially feed on medium copepods such as Metridia pacifica (Boldt et al., 2019). However, given that only two years were classified as late Chl a bloom years over the 14 years considered in this study, future analyses of longer time series are needed in order to further elucidate the impact of late bloom years on the crustacean community.
It is important to highlight that no relationships were found between crustacean biomass and either spring bloom intensity or bloom magnitude, parameters indicative of the amount of food available to grazers throughout the spring. These results suggest that food is likely rarely, if ever, limiting in the surface waters of the Central SoG during the main growing season for zooplankton (i.e., spring, summer, and autumn). Unlike other regions in the world that exhibit a single spring peak in Chl a (e.g., the North Atlantic), Chl a in the SoG peaks multiple times throughout the growing season (Schweigert et al., 2013; Suchy et al., 2019). The suspected lack of food limitation was especially evident for the climatology and early bloom years wherein Chl a concentrations were rarely observed to be < 5 mg m-3, thus indicating that zooplankton were not likely experiencing a shortage of food. In fact, the 8-day weekly Chl a concentrations used to generate our monthly values rarely decreased below 2 mg m-3 once the spring bloom was initiated and, if values < 2 mg m-3 did occur, it was never for two or more consecutive weeks (see Suchy et al., 2019). Furthermore, average and late bloom years exhibited Chl a concentrations only slightly below 5 mg m-3 at the beginning or end of the sampling period. Nevertheless, many of the dominant crustaceans, including the taxa considered in this study, are known to feed omnivorously for at least part of their life cycle. Therefore, it is reasonable to assume that even during a period of low phytoplankton biomass, many species could switch to omnivorous feeding in order to meet their nutritional requirements for growth and survival (El-Sabaawi et al., 2009).
4.5 Data Limitations
Satellite-based Chl a studies compromise information on the vertical distribution of phytoplankton in exchange for data at large spatial-temporal scales. Therefore, one important caveat in the interpretation of results from this study is that our satellite-derived Chl a measurements represent only surface Chl a biomass. Although we use these surface values as a proxy to represent the food potentially available throughout the euphotic zone, caution must still be taken when interpreting the results. Nevertheless, during the time frame of this study, a preliminary comparison with in situ Chl a data showed no instances of deep-water chlorophyll maxima that were not also reflected in the surface satellite measurements (see Suchy et al., 2019). In addition, although used widely as a proxy, Chl a is not always the optimal indicator of phytoplankton biomass (Chittenden et al., 2010; Behrenfeld & Boss, 2014; Behrenfeld et al., 2015) and thus may not effectively represent what zooplankton are actually feeding on (Ji et al., 2010; Friedland et al., 2015). Furthermore, our spring bloom initiation metric was based on annual median Chl a values calculated over the time period for which satellite data were available (mid-February to mid-November). We note that median Chl a values may be slightly lower if we had considered Chl a concentrations during the winter months; however, low Chl a values were observed in February and November of some years and were thus included in our calculations (Supplementary Table 1). More importantly, the time period we considered was consistent across years which helped to minimize any bias in our results due to a lack of winter Chl a values. We also note that while it is possible that bloom conditions may have occurred prior to mid-February during some years, high Chl a concentrations early in the year are more likely to be localized or confined to nearby inlets (Gower et al., 2013) compared to the widespread bloom across the Central SoG represented here.
In contrast to the surface Chl a measurements, our zooplankton data span the entire vertical range of the water column from just above the bottom of the seafloor to the surface. Therefore, our study attempts to correlate parameters captured on two different vertical scales. That said, many zooplankton undertake diel vertical migrations, and seasonal variability in zooplankton is typically more pronounced in the surface layers where juvenile stages are feeding and developing (Mackas et al., 2013). It is thus reasonable to observe a relationship between these lower trophic levels. In addition, zooplankton sampling using vertical net hauls has a variety of its own limitations. Specifically, certain species (e.g., euphausiids) are known to exhibit net avoidance (Mackas et al., 2013; Perry et al., 2021) and our use of a 250 µm mesh net may result in an under-sampling of smaller zooplankton species, particularly the naupliar and juvenile stages of smaller crustacean taxa. As mentioned, the zooplankton dataset used in this study suffered from limited temporal coverage during some years, a discussion of which can be found in Perry et al. (2021), which affected our interpretation of the impact of late Chl a blooms on the crustacean zooplankton community.
4.6 Conclusions
To our knowledge, this is the first study to examine the link between satellite-derived Chl a bloom dynamics to zooplankton phenology over a long (14 year) time period in the Strait of Georgia, BC. The satellite-derived Chl a data revealed that the spring bloom occurs, on average, during the last week of March in the Central SoG. Early blooms (years 2004, 2005, and 2015) occurred in mid-to-late February/early March during warm-phase conditions (-NPGO, -SOI, +SST, +PDO) and were characterized by higher SST and higher bloom magnitudes. In contrast, average to late bloom years exhibited lower SST and lower bloom magnitude. The monthly median climatology indicated that Chl a peaks in April and typically remains > 5 mg m-3 until November. Although the peak timing of Chl a varied between early, average, and late years, Chl a concentrations were rarely below 5 mg m-3, thus suggesting that food for zooplankton is likely not limiting in the Central SoG once the spring bloom has been initiated. Early bloom years were associated with a shift in the composition of the crustacean community, with a higher prevalence of smaller non-calanoid copepods and a decrease in larger crustaceans (large calanoid copepods, euphausiids, and amphipods). Both early and late Chl a blooms may potentially result in a mismatch between phytoplankton and larger crustacean zooplankton phenology, thus resulting in lower abundances of large, energy-rich crustaceans and lower overall biomass available to higher trophic levels. We hypothesize that this may result in poorer feeding conditions for juvenile salmon and other predators. This work highlights the need for long-term frequent zooplankton sampling programs that include estimates of lower trophic level productivity, in addition to standard abundance and biomass data, in order to understand how phenological match/mismatches affect the transfer of energy between phytoplankton and zooplankton. Combined with accurate region-specific models, these future efforts will help to further elucidate the match/mismatch of zooplankton timing relative to their phytoplankton prey and the potential impacts on higher trophic levels in the SoG.
Data Availability Statement
Publicly available datasets were analyzed in this study. These data can be found here: https://open.canada.ca/data/en/dataset/2822c11d-6b6c-437e-ad65-85f584522adc.
Author Contributions
KS, MC, IP, KY, and MG contributed to the design of the study. KY, MG provided taxonomic identification for the zooplankton dataset. KS performed the data analyses. KS wrote the first draft of the manuscript. All authors revised, read, and approved the submitted versions.
Conflict of Interest
The authors declare that the research was conducted in the absence of any commercial or financial relationships that could be construed as a potential conflict of interest.
Publisher’s Note
All claims expressed in this article are solely those of the authors and do not necessarily represent those of their affiliated organizations, or those of the publisher, the editors and the reviewers. Any product that may be evaluated in this article, or claim that may be made by its manufacturer, is not guaranteed or endorsed by the publisher.
Acknowledgments
This is publication Number 63 from the Salish Sea Marine Survival Project (https://marinesurvivalproject.com). This work was made possible with support from the Pacific Salmon Foundation, the Marine Environmental Observation Prediction and Response (MEOPAR) network, and the Canadian Space Agency (FAST 18FAVICB09). Funding to KS was provided by a Mitacs Accelerate Award with matching funds from the Pacific Salmon Foundation. We thank the two reviewers whose comments significantly improved this manuscript.
Supplementary Material
The Supplementary Material for this article can be found online at: https://www.frontiersin.org/articles/10.3389/fmars.2022.832684/full#supplementary-material
References
Allen S. E., Latornell D. J., Olson E., Pawlowicz R. (2016). Timing of the Spring Phytoplankton Bloom in the Strait of Georgia 2015 and 2016 in State of the Physical, Biological, and Selected Fishery Resources of Pacific Canadian Marine Ecosystems. Can. Tech. Rep. Fish. Aquat. Sci. 3179, 147–152.
Allen S. E., Wolfe M. A. (2013). Hindcast of the Timing of the Spring Phytoplankton Bloom in the Strait of Georgia 1968–2010. Prog. Oceanogr. 115, 6–13. doi: 10.1016/j.pocean.2013.05.026
Batten S. D., Mackas D. L. (2009). Shortened Duration of the Annual Neocalanus Plumchrus Biomass Peak in the Northeast Pacific. Marine Ecol. Prog. Ser. 393, 189–198. doi: 10.3354/meps08044
Beamish R. J., Mahnken C., Neville C. M. (1997). Hatchery and Wild Production of Pacific Salmon in Relation to Large-Scale, Natural Shifts in the Productivity of the Marine Environment. ICES J. Mar. Sci. 54, 1200–1215. doi: 10.1016/S1054-3139(97)80027-6
Beamish R. J., Sweeting R. M., Lange K. L., Noakes D. J., Preikshot D., Neville C. M. (2010). Early Marine Survival of Coho Salmon in the Strait of Georgia Declines to Very Low Levels. Mar. Coast. Fish. 2(1), 424–39. doi: 10.1016/j.pocean.2013.05.020
Beamish R. J., Neville C., Sweeting R., Lange K. (2012). The Synchronous Failure of Juvenile Pacific Salmon and Herring Production in the Strait of Georgia in 2007 and the Poor Return of Sockeye Salmon to the Fraser River in 2009. Mar. Coast. Fish: Dynam. Manage Ecosystem Sci. 2 (1), 434–29. doi: 10.1016/j.pocean.2013.05.020
Beaugrand G., Brander K. M., Lindley J. A., Souissi S., Reid P. C. (2003). Plankton Effect on Cod Recruitment in the North Sea. Nature 426, 661–663. doi: 10.1038/nature02164
Beckers J. M., Rixen M. (2003). EOF Calculations and Data Filling From Incomplete Oceanographic Datasets. J. Atmospheric Oceanic Technol. 20, 1839–1856. doi: 10.1175/1520-0426(2003)020<1839:ECADFF>2.0.CO;2
Behrenfeld M. J., Boss E. S. (2014). Resurrecting the Ecological Underpinnings of Ocean Plankton Blooms. Annu. Rev. Marine Sci. 6, 167–194. doi: 10.1146/annurev-marine-052913-021325
Behrenfeld M. J., O’Malley R. T., Boss E. S., Westberry T. K., Graff J. R., Halsey K. H., et al. (2015). Revaluating Ocean Warming Impacts on Global Phytoplankton. Nat. Climate Change 6, 323–330. doi: 10.1038/nclimate2838
Blanchet F. G., Legendre P., Borcard D. (2008). Forward Selection of Explanatory Variables. Ecology 89 (9), 2623–2632. doi: 10.1890/07-0986.1
Boldt J. L., Thompson M., Rooper C. N., Hay D. E., Schweigert J. F., Quinn T. J. II, et al. (2019). Bottom-Up and Top-Down Control of Small Pelagic Forage Fish: Factors Affecting Age-0 Herring the Strait of Georgia, British Columbia. Marine Ecol. Prog. Ser. 617-618, 53–66. doi: 10.3354/meps12485
Bornhold E. A. (2000). Interannual and Interdecadal Patterns in Timing and Abundance of Phytoplankton and Zooplankton in the Central Strait of Georgia, BC: With Special Reference to Neocalanus Plumchrus. MSc Thesis (Vancouver, BC, Canada: University of British Columbia), 132.
Brodeur R. D., Ware D. M. (1992) Long-term Variability in Zooplankton Biomass in the Subarctic Pacific Ocean Fish. Oceanogr., 1(1), 32–38. doi: 10.1111/j.1365-2419.1992.tb00023.x
Campbell J. W. (1995). The Lognormal Distribution as a Model for Bio-Optical Variability in the Sea. J. Geophysical Res. 100, 13,237–13,254. doi: 10.1029/95JC00458
Carswell T., Costa M., Young E., Komick N., Gower J., Sweeting R. (2017). Evaluation of MODIS-Aqua Atmospheric Correction and Chlorophyll Products of Western North American Coastal Waters Based on 13 Years of Data. Remote Sens. 9 (1063), 1–24. doi: 10.3390/rs9101063
Chiba S., Aita M. N., Tadokoro K., Saino T., Sugisaki H., Nakata K. (2008). From Climate Regime Shifts to Lower-Trophic Level Phenology: Synthesis of Recent Progress in Retrospective Studies of the Western North Pacific. Prog. Oceanogr. 77, 112–126. doi: 10.1016/j.pocean.2008.03.004
Chittenden C. M., Jensen J. L. A., Ewart D., Anderson S., Balfry S., Downey E., et al. (2010). Recent Salmon Declines: A Result of Lost Feeding Opportunities Due to Bad Timing? PLoS One 5 (8), e12423. doi: 10.1371/journal.pone.0012423
Collins A. K., Allen S. E., Pawlowicz R. (2009). The Role of Wind in Determining the Timing of the Spring Bloom in the Strait of Georgia. Can. J. Fish. Aquat. Sci. 66, 1597–1616. doi: 10.1139/F09-071
Cushing D. H. (1969). The Regularity of the Spawning Season of Some Fishes. J. Cons Int. Explor. Mer 33, 81–92. doi: 10.1093/icesjms/33.1.81
Cushing D. H. (1990). Plankton Production and Year-Class Strength in Fish Populations: An Update of the Match/Mismatch Hypothesis. Adv. Mar. Biol. 26, 249–293. doi: 10.1016/S0065-2881(08)60202-3
Daly E., Benkwitt C., Brodeur R., Litz M., Copeman L. (2010). Fatty Acid Profiles of Juvenile Salmon Indicate Prey Selection Strategies in Coastal Marine Waters. Mar. Biol. 157, 1975–1987. doi: 10.1007/s00227-010-1466-9
Edwards M., Richardson A. J. (2004). Impact of Climate Change on Marine Pelagic Phenology and Trophic Mismatch. Nature 430, 881–884. doi: 10.1038/nature02808
El-Sabaawi R., Dower J. F., Kainz M., Mazumder A. (2009). Interannual Variability in Fatty Acid Composition of the Copepod Neocalanus Plumchrus in the Strait of Georgia, British Columbia. Mar. Ecol. Prog. Ser. 382, 151–161. doi: 10.3354/meps07915
Fischer A. D., Hayashi K., McGaraghan A., Kudela R. M. (2020). Return of the “Age of Dinoflagellates” in Monterey Bay: Drivers of Dinoflagellate Dominance Examined Using Automated Imaging Flow Cytometry and Long-Term Time Series Analysis. Limnol Oceanogr. 65, 2125–2141. doi: 10.1002/lno.11443
Foreman M. G. G., Lee D. K., Morrison J., Macdonald ,. S., Barnes D., Williams I. V. (2001). Simulations and Retrospective Analyses of Fraser Watershed Flows and Temperatures. Atmosphere-Ocean 39 (2), 89–105. doi: 10.1080/07055900.2001.9649668
Friedland K. D., Leaf R. T., Kane J., Tommasi D., Asch R. G., Rebuck N., et al. (2015). Spring Bloom Dynamics and Zooplankton Biomass Response on the US Northeast Continental Shelf. Continental Shelf Res. 102, 47–61. doi: 10.1016/j.csr.2015.04.005
Friedland K. D., Mouw C. B., Asch R. G., Ferreira A. S. A., Henson S., Hyde K. J. W., et al. (2018). Phenology and Time Series Trends of the Dominant Seasonal Phytoplankton Bloom Across Global Scales. Global Ecol. Biogeography 27, 551–569. doi: 10.1111/geb.12717
Friedland K. D., Record N. R., Asch R. G., Kristiansen T., Saba V. S., Drinkwater K. F., et al. (2016). Seasonal Phytoplankton Blooms in the North Atlantic Linked to the Overwintering Strategies of Copepods. Elementa 4: 000099. doi: 10.12952/journal.elementa.000099
Furey N. B., Vincent S. P., Hinch S. G., Welch D. W. (2015). Variability in Migration Routes Influences Early Marine Survival of Juvenile Salmon Smolts. PLoS One 10 (10): e0139269. doi: 10.1371/journal.pone.0139269
Gower J., King S., Statham S., Fox R., Young E. (2013). The Malaspina Dragon: A Newly-Discovered Pattern of the Early Spring Bloom in the Strait of Georgia, British Columbia, Canada. Prog. Oceanogr. 115, 181–188. doi: 10.1016/j.pocean.2013.05.024
Halverson M. J., Pawlowicz R. (2013). High-Resolution Observations of Chlorophyll-a Biomass From an Instrumented Ferry: Influence of the Fraser River Plume From 2003 to 2006. Continental Shelf Res. 59, 52–64. doi: 10.1016/j.csr.2013.04.010
Haro-Garay M. J., Soberanis L. H. (2008). Dominance Shift of Zooplankton Species Composition in the Central Strait of Georgia, British Columbia During 1997. Hidrobiológica 18, 53–60.
Harrison P. J., Fulton J. D., Taylor F. J. R., Parson T. R. (1983). Review of the Biological Oceanography of the Strait of Georgia: Pelagic Environment. Can. J. Fish. Aquat. Sci. 40, 1064–1094. doi: 10.1139/f83-129
Harris S. L., Varela D. E., Whitney F. W., Harrison P. J. (2009). Nutrient and Phytoplankton Dynamics Off the West Coast of Vancouver Island During the 1997/98 ENSO Event. Deep-Sea Res. II 56, 2487–2502. doi: 10.1016/j.dsr2.2009.02.009
Henson S., Cole H., Beaulieu C., Yool A. (2013). The Impact of Global Warming on Seasonality of Ocean Primary Production. Biogeosciences 10, 4357–4369. doi: 10.5194/bg-10-4357-2013
Henson S. A., Dunne J. P., Sarmiento J. L. (2009). Decadal Variability in North Atlantic Phytoplankton Blooms. J. Geophys. Res.: Oceans 114, C04013. doi: 10.1029/2008JC005139
Henson S. A., Robinson I., Allen J. T., Waniek J. J. (2006). Effect of Meteorological Conditions on Interannual Variability in Timing and Magnitude of the Spring Bloom in the Irminger Basin, North Atlantic. Deep Sea Res. Part I 53, 1601–1615. doi: 10.1016/j.dsr.2006.07.009
Hilborn A., Costa M. (2018). Applications of DINEOF to Satellite-Derived Chlorophyll-a From a Productive Coastal Region. Remote Sens. 10 (9), 1449. doi: 10.3390/rs10091449
Hipfner J. M., Galbraith M., Bertram D. F., Green D. J. (2020). Basin-Scale Oceanographic Processes, Zooplankton Community Structure, and Diet and Reproduction of a Sentinel North Pacific Seabird Over a 22-Year Period. Prog. Oceanogr 182, 102290. doi: 10.1016/j.pocean.2020.102290
Hunter-Cevera K. R., Neubert M. G., Olson R. J., Solow A. R., Shalapyonok A., Sosik H. M. (2016). Physiological and Ecological Drivers of Early Spring Blooms of a Coastal Phytoplankter. Science 354, 326–329. doi: 10.1126/science.aaf8536
Jackson J. J., Thomson R. E., Brown L. N., Willis P. G., Borstad G. A. (2015). Satellite Chlorophyll Off the British Columbia Coast 1997-2010. J. Geophysical Res.: Oceans 120, 4709–4728. doi: 10.1002/2014JC010496
Ji R. B., Davis C. S., Chen C. S., Townsend D. W., Mountain D. G., Beardsley R. C. (2007). Influence of Ocean Freshening on Shelf Phytoplankton Dynamics. Geophys. Res. Lett. 34, L24607. doi: 10.1029/2007GL032010
Ji R., Edwards M., Mackas D. L., Runge J. A., Thomas A. C. (2010). Marine Plankton Phenology and Life History in a Changing Climate: Current Research and Future Directions. J. Plankton Res. 32, 1355–1368. doi: 10.1093/plankt/fbq062
Kamykowski D., Zentara J. (2005). Changes in World Ocean Nitrate Availability Through the 20th Century. Deep Sea Res. I 52, 1719–1744. doi: 10.1016/j.dsr.2005.04.007
Kluyver T., Ragan-Kelley B., Pérez F., Granger B., Bussonnier M., Frederic J., et al. (2016). “Jupyter Notebooks – a Publishing Format for Reproducible Computational Workflows,” in Positioning and Power in Academic Publishing: Players, Agents and Agendas, vol. 760 . Eds. Loizides F., Scmidt B. (Netherlands: IOS Press), 87–90.
Komick N. M., Costa M. P. F., Gower J. (2009). Bio-Optical Algorithm Evaluation for MODIS for Western Canada Coastal Waters: An Exploratory Approach Using in Situ Reflectance. Remote Sens. Environ. 113 (4), 794–804. doi: 10.1016/j.rse.2008.12.005
Li M., Gargett A., Denman K. (2000). What Determines Seasonal and Interannual Variability of Phytoplankton and Zooplankton in Strongly Estuarine Systems? Appl. to Semi-enclosed Estuary Strait Georgia Juan Fuca Strait. Estuarine Coastal Shelf Sci. 50, 467–488. doi: 10.1006/ecss.2000.0593
Li L., Mackas D., Hunt B., Schweigert J., Pakhomov E., Perry R. I., et al. (2013). Zooplankton Communities in the Strait of Georgia, British Columbia, Track Large-Scale Climate Forcing Over the Pacific Ocean. Prog. Oceanogr. 115, 90–102. doi: 10.1016/j.pocean.2013.05.025
Mackas D. L., Goldblatt R., Lewis A. G. (1998). Interdecadal Variation in Developmental Timing of Neocalanus Plumchrus Populations at Ocean Station P in the Subarctic North Pacific. Can. J. Fisheries Aquat. Sci. 55, 1878–1893. doi: 10.1139/f98-080
Mackas D. L., Thomson R. E., Galbraith M. (2001). Changes in the Zooplankton Community of the British Columbia Continental Margin, 1985-1999, and Their Covariation With Oceanographic Conditions. Can. J. Fish. Aquat. Sci. 58 (4), 685–702. doi: 10.1139/f01-009
Mackas D. L., Batten S., Trudel M. (2007). Effects on Zooplankton of a Warmer Ocean: Recent Evidence From the Northeast Pacific. Prog. Oceanogr. 75, 223–252. doi: 10.1016/j.pocean.2007.08.010
Mackas D. L., Greve W., Edwards M., Chiba S., Tadokoro K., Eloire D., et al. (2012). Changing Zooplankton Seasonality in a Changing Ocean: Comparing Time Series of Zooplankton Phenology. Prog. Oceanogr. 97–100, 31–62. doi: 10.1016/j.pocean.2011.11.005
Mackas D., Galbraith M., Faust D., Masson D., Young K., Shaw W., et al. (2013). Zooplankton Time Series From the Strait of Georgia: Results From Year-Round Sampling at Deep Water Locations 1990-2010. Prog. Oceanogr. 115, 129–159. doi: 10.1016/j.pocean.2013.05.019
Malick M. J., Cox S. P., Mueter F. J., Peterman R. M. (2015). Linking Phytoplankton Phenology to Salmon Productivity Along a North-South Gradient in the Northeast Pacific Ocean. Can. J. Fish. Aquat. Sci. 72, 697–708. doi: 10.1139/cjfas-2014-0298
McClain C. R., Ainsworth E. J., Barnes R. A., Eplee R. E., Patt F. S., Robinson W. D., et al. (2000). SeaWiFS Postlaunch Calibration and Validation Analyses, Part 1; Technical Report 2000-206892 (Greenbelt, MD, USA: NASA Goddard Space Flight Center).
Nemcek N., Hennekes M., Perry I. (2020). Seasonal Dynamics of the Phytoplankton Community in the Salish Sea From HPLC Measurements 2015-2019 in State of the Physical, Biological, and Selected Fishery Resources of Pacific Canadian Marine Ecosystems in 2019. (Eds.) Boldt, J.L., Javorski, A., and Chandler P.C. Can. Tech. Rep. Fish. Aquat. Sci. 3179, 169–173.
Neville C. M., Beamish R. J. (1999). “Comparison of the Diets of Ocean Age 0 Hatchery and Wild Chinook Salmon. (NPAFC Doc. 435),” in Department of Fisheries and Oceans, Pacific Biological Station. Ed. Nanaimo B. C.(Canada).
Neville C. M., Beamish R. J., Chittenden C. M. (2015). Poor Survival of Acoustically-Tagged Juvenile Chinook Salmon in the Strait of Georgia, British Columbia, Canada. Trans. Am. Fish. Soc. 144 (1), 25–33. doi: 10.1080/00028487.2014.954053
Parsons T. R., Giovando L. F., LeBrasseur R. J. (1966). The Advent of the Spring Bloom in the Eastern Subarctic Pacific Ocean. J. Fish. Res. Board Canada 23 (4), 539–546. doi: 10.1139/f66-045
Pawlowicz R., Riche O., Halverson M. (2007). The Circulation and Residence Time of The Strait of Georgia Using a Simple Mixing-Box Approach. Atmosphere-Ocean 45 (4), 173–193. doi: 10.3137/ao.450401
Peña M. A., Masson D., Callendar W. (2016). Annual Plankton Dynamics in a Coupled Physical-Biological Model of the Strait of Georgia, British Columbia. Prog. Oceanogr. 146, 58–74. doi: 10.1016/j.pocean.2016.06.002
Perry R. I., Young K., Galbraith M., Chandler P., Velez-Espino A., Baillie S. (2021). Zooplankton Variability in the Strait of Georgia, Canada, and Relationships With the Marine Survivals of Chinook and Coho Salmon. PLoS One 16, e0245941. doi: 10.1371/journal.pone.0245941
Peterson W. T., Schwing F. B. (2003). A New Climate Regime in Northeast Pacific Ecosystems. Geophys. Res. Lett. 30, 1896. doi: 10.1029/2003GL017528
Platt T., Fuentes-Yaco C., Frank K. T. (2003). Spring Algal Bloom and Larval Fish Survival. Nature 423, 398–399. doi: 10.1038/423398b
Pospelova V., Esenkulova S., Johannessen S. C., O'Brien M. C., Macdonald R. W. (2010) Organic-Walled Dinoflagellate Cyst Production, Composition and Flux From 1996 to 1998 in the Central Strait of Georgia (BC, Canada): A Sediment Trap Study Mar Micropaleontol, 75(1–4), 17–37. doi: 10.1016/j.marmicro.2010.02.003
Preikshot D., Beamish R. J., Neville C. M. (2013). A Dynamic Model Describing Ecosystem-Level Changes in the Strait of Georgia From 1960 to 2010. Prog. Oceanogr. 115, 28–40. doi: 10.1016/j.pocean.2013.05.020
Preikshot D., Beamish R. J., Sweeting R. M. (2010). Changes in the Diet Composition of Juvenile Sockeye Salmon in the Strait of Georgia From the 1960s to the Early 21st Century. NPAFC Doc, Vol. 1285. 17. Fisheries and Oceans Canada. Nanaimo (Canada).
Racault M. F., Quéré C., Buitenhuis E., Sathyendranath S., Platt T. (2012). Phytoplankton Phenology in the Global Ocean. Ecol. Indic. 14, 152–163. doi: 10.1016/j.ecolind.2011.07.010
R Development Core Team (2018). R: A Language and Environment for Statistical Computing (Vienna: R Foundation for Statistical Computing).
Richardson A. J. (2008). In Hot Water: Zooplankton and Climate Change. ICES J. Mar. Sci. 65, 279–295. doi: 10.1093/icesjms/fsn028
Ross R. M., Quetin L. B. (2000). In: Reproduction in Euphausiacea (ed) Krill Biology, Ecology and Fisheries. Fisheries and Aquatic Resources Series. Blackwell Science, Cambridge, p 150–81
Sasaoka K., Chiba S., Saino T. (2011). Climatic Forcing and Phytoplankton Phenology Over the Subarctic North Pacific From 1998 to 2006, as Observed From Ocean Color Data. Geophys. Res. Lett. 38, L15609. doi: 10.1029/2011GL048299
Sastri A., Dewey R., Mihaly S., Pawlowicz R. (2016). Deep Water and Sea-Surface Properties in the Strait of Georgia During 2015: Ferries and Cabled Instruments in State of the Physical, Biological, and Selected Fishery Resources of Pacific Canadian Marine Ecosystems in 2015. (Eds.) Chandler, P.C., King, S.A., and Perry, R.I. Can. Tech. Rep. Fish. Aquat. Sci. 3179, 142–146.
Schweigert J. F., Thompson M., Fort C., Hay D. E., Therriault ,. T. W., Brown L. N. (2013). Factors Linking Pacific Herring (Clupea Pallasi) Productivity and the Spring Plankton Bloom in the Strait of Georgia, British Columbia, Canada. Prog. Oceanogr. 115, 103–110. doi: 10.1016/j.pocean.2013.05.017
Siegel D. A., Doney S. C., Yoder J. A. (2002). The North Atlantic Spring Phytoplankton Bloom and Sverdrup’s Critical Depth Hypothesis. Science 296, 730–733. doi: 10.1126/science.1069174
Stockner J. G., Cliff D. D., Buchanan D. B. (1977). Phytoplankton Production and Distribution in Howe Sound, British Columbia: A Coastal Marine Embayment-Fjord Under Stress. J. Fish. Res. Board Canada 34, 907–919. doi: 10.1139/f77-142
Suchy K. D., Le Baron N., Hilborn A., Perry R. I., Costa M. (2019). Influence of Environmental Drivers on Spatio-Temporal Dynamics of Satellite-Derived Chlorophyll a in the Strait of Georgia. Prog. Oceanogr. 176, 102134. doi: 10.1016/j.pocean.2019.102134
Thomson R. E. (1981). Oceanography of the British Columbia Coast. Can. Spec. Publ. Fish. Aquat. Sci. 56, p. 291
Thomson R. E., Beamish R. J., Beacham T. D., Trudel M., Whitfield P. H., Hourston R. A. S. (2012). Anomalous Ocean Conditions may Explain the Recent Extreme Variability in Fraser River Sockeye Salmon Production. Mar. Coastal. Fish. 4, 415–437. doi: 10.1080/19425120.2012.675985
Tommasi D., Hunt B. P. V., Pakhomov E. A. (2021). Differential Response of Distinct Copepod Life History Types to Spring Environmental Forcing in Rivers Inlet, British Columbia, Canada. PeerJ 9, e12238. doi: 10.7717/peerj.12238
Tommasi D. A. G., Routledge R. D., Hunt B. P. V., Pakhomov E. A. (2013). The Seasonal Development of the Zooplankton Community in a British Columbia (Canada) Fjord During Two Years With Different Spring Bloom Timing. Marine Biol. Res. 9, 129–144. doi: 10.1080/17451000.2012.708044
Whittingham M. J., Stephens P. A., Bradbury R. B., Freckleton R. P. (2006). Why do We Still Use Stepwise Modelling in Ecology and Behaviour? J. Anim. Ecol. 75 (5), 1182–1189. doi: 10.1111/j.1365-2656.2006.01141.x
Yin K., Harrison P. J., Goldblatt R. H., St. John M. A., Beamish R. J. (1997). Factors Controlling the Timing of the Spring Bloom in the Strait of Georgia Estuary, British Columbia, Canada. Can. J. Fish. Aquat. Sci. 54, 1985–1995. doi: 10.1139/f97-106
Yoo S., Batchelder H. P., Peterson W. T., Sydeman W. J. (2008). Seasonal, Interannual and Event Scale Variation in North Pacific Ecosystems. Prog. Oceanogr. 77 (2-3), 155–181. doi: 10.1016/j.pocean.2008.03.013
Keywords: remote sensing, chlorophyll a, crustacean zooplankton, match/mismatch, phenology, Strait of Georgia, Salish Sea, spring bloom
Citation: Suchy KD, Young K, Galbraith M, Perry RI and Costa M (2022) Match/Mismatch Between Phytoplankton and Crustacean Zooplankton Phenology in the Strait of Georgia, Canada. Front. Mar. Sci. 9:832684. doi: 10.3389/fmars.2022.832684
Received: 10 December 2021; Accepted: 21 April 2022;
Published: 26 May 2022.
Edited by:
Martin Edwards, Plymouth Marine Laboratory, United KingdomReviewed by:
Daria Martynova, Zoological Institute (RAS), RussiaRebecca G. Asch, East Carolina University, United States
Copyright © 2022 Suchy, Young, Galbraith, Perry and Costa. This is an open-access article distributed under the terms of the Creative Commons Attribution License (CC BY). The use, distribution or reproduction in other forums is permitted, provided the original author(s) and the copyright owner(s) are credited and that the original publication in this journal is cited, in accordance with accepted academic practice. No use, distribution or reproduction is permitted which does not comply with these terms.
*Correspondence: Karyn D. Suchy, a2FyeW5kc3VjaHlAZ21haWwuY29t
†Present address: Karyn D. Suchy, Department of Earth, Ocean and Atmospheric Sciences, University of British Columbia, Vancouver, BC, Canada