- Sea Mammal Research Unit, Scottish Oceans Institute, University of St Andrews, St Andrews, United Kingdom
Understanding how marine predators find patchily distributed prey resources in a dynamic environment is key to identifying important ecological areas for ecosystem-level conservation management. However, the mechanisms underpinning important foraging areas often result from complex interactions between static and dynamic covariates (e.g. topography and currents). Modelling habitat associations with hydrodynamic processes is rarely useful when attempting to identify and characterise foraging areas across an individual’s foraging range. Investigating the influence of static habitat features on predator behaviour can provide a more tractable baseline understanding of habitat associations, upon which additional complexity can be added. Seabed gradient covariates (e.g. slope and aspect) are often used, yet such metrics are computed at singular user-defined resolutions, and provide limited ecological insight when used in isolation. Instead, categorising the seabed into geomorphological features may provide better characterisation of seabed structure. Here we explore the utility of a pattern recognition algorithm to delineate whole geomorphological features (“geomorphons”) on the seabed (e.g. valleys, ridges, footslopes) from bathymetry data, and examine the influence of geomorphology on marine predator habitat use. We demonstrate the potential application of this approach in a case study, examining the influence of geomorphons on the at-sea behaviour of a highly mobile predator inhabiting shelf seas: the grey seal (Halichoerus grypus). We analyse GPS tracking data from three seals tagged in the southern North Sea, an area with heterogeneous geomorphology. We use hidden Markov models (HMMs) to infer foraging and travelling behaviour and model the effect of different feature types on the probability of switching between states. All three seals showed an increased probability of transitioning from travelling to foraging when encountering slopes, footslopes and hollows, and foraging activity was concentrated at slopes on the fringes of the Dogger Bank. We hypothesise that such features may host prey aggregations, and/or lead to increased prey capture success. The results suggest the importance of such areas for grey seals in the southern North Sea, a region undergoing rapid and widespread anthropogenic habitat change. This method could be incorporated into future species distribution models to improve estimates of predator distribution, informing conservation management and marine spatial planning.
Introduction
Understanding how animals utilise their environment to optimise food intake and maximise fitness is a fundamental question for ecologists (Stephens and Krebs, 1986). Studying the intrinsic and extrinsic mechanisms which underpin the foraging behaviour of animals can provide insights into the functioning of ecosystems and ecologically important areas (Schmitz et al., 1997). It is particularly important to understand the processes which shape the foraging behaviour of high trophic level predators, as they play a vital role in the structure of marine and terrestrial communities through the top-down regulation of prey (Williams et al., 2004; Heithaus et al., 2008). Marine ecosystems are inherently dynamic and prey resources are often patchily distributed (Haury et al., 1978). Therefore, highly mobile predators (e.g. marine mammals, turtles, pelagic fish and seabirds) may adjust their foraging behaviour to maximise time in the most productive areas (Kie, 1999; Weimerskirch, 2007; Kirchner et al., 2018). Understanding how predators use environmental features to optimise foraging is necessary to allow ecologically important areas to be identified, and thus to inform marine spatial planning (Boyd and Murray, 2001; Hindell et al., 2020). Such areas are not easy to identify at sea because we cannot easily observe them, but spatial aggregations of predators can be used as indicators (Block et al., 2011; Montevecchi et al., 2012; Grecian et al., 2016; Hindell et al., 2020).
Observing the behaviour of highly mobile marine predators is challenging as they spend most or all of their time at sea, often far from land or underwater, and can cover large distances (Fedak et al., 2002; Luschi et al., 2003; Skomal et al., 2017). Animal-borne sensors allow for the collection of fine-scale data from which the movements of marine predators can be reconstructed (Wilmers et al., 2015). Such data can be analysed to infer behaviours (Patterson et al., 2008; Carter et al., 2016), to identify important foraging areas (Camphuysen et al., 2012), and to understand the environmental characteristics of these areas using ancillary oceanographic data from animal-borne tags (Lydersen et al., 2002; Boehme et al., 2008a; Boehme et al., 2008b). Locational data collected from animal-borne tags are frequently used to infer discrete behaviours, such as travelling (e.g. commuting to, from and between foraging sites) and Area-Restricted Search (ARS), which in many vagile species has been associated with foraging activity, i.e. searching for, capturing and handling prey (Kareiva and Odell, 1987; Pinaud and Weimerskirch, 2007). Identifying discrete behaviours from animal tracking data is commonly done by analysing aspects of track geometry, such as changes in step lengths (the distance between two consecutive locations) and turning angles through time (Boyd, 1996; Fauchald and Tverra, 2003; Barraquand and Benhamou, 2008). During ARS, an animal will typically exhibit large turning angles compared to while travelling, combined with a reduction in speed, as a result of searching for, encountering and responding to a food source or in response to environmental cues, for example in areas where prey was previously encountered (Kareiva and Odell, 1987). In recent years, hidden Markov models (HMMs) have emerged as a popular and flexible analytical tool which can be used to identify discrete movement modes (from which behaviours can be inferred) from animal movement data (Patterson et al., 2008; Langrock et al., 2012). The movements of many highly mobile marine predators, including pelagic fish (e.g. Patterson et al., 2009; Towner et al., 2016), seabirds (e.g. Pirotta et al., 2018; Clay et al., 2020) and marine mammals (e.g. Isojunno et al., 2017; Carter et al., 2020) have been investigated using tracking data and HMMs. A major advantage of HMMs over other movement models is that they can be used to investigate the mechanisms that shape animal behaviour through the inclusion of covariate effects, such as environmental variables, on state transition probabilities (Morales et al., 2004; Patterson et al., 2009; Photopoulou et al., 2020). In this way, the effects of encountering a specific feature or habitat type on the probability that an animal will switch from one state to another can be quantified (Morales et al., 2004).
Examining animal behaviour in relation to the variety of environmental conditions that an individual encounters whilst searching for prey can help us to understand what ultimately drives foraging decisions. A wide range of environmental factors are often considered in studies of marine predator habitat associations, including static covariates such as bathymetric depth, which can influence prey accessibility for benthic foragers (e.g., Burns et al., 2004), as well as dynamic covariates that may influence prey distribution, such as sea ice presence for polar species (e.g., Pagano and Williams, 2021) and sea surface temperature in seasonal seas (e.g., Georges et al., 2000; Speakman et al., 2020). Seabed topography (i.e. spatial variation in seabed terrain) can influence predator behaviour and distribution through a variety of complex mechanisms (Cox et al., 2018). These mechanisms may be indirect, for example static features in the open ocean such as seamounts are associated with increased primary productivity (Genin and Boehlert, 1985), creating predictable foraging conditions for marine predators (Morato et al., 2010; Maxwell et al., 2012; Letessier et al., 2019). Similarly, in shelf seas tidal currents moving over offshore banks and uneven terrain can lead to subsurface aggregations of small fish, either through increased plankton concentration (Embling et al., 2012), or the mechanical influence of internal waves (Embling et al., 2013). Such phenomena create spatially and temporally predictable foraging opportunities (Embling et al., 2012; Scott et al., 2013). Topography may have a more direct relationship to predator foraging behaviour; known foraging areas of bottlenose dolphins (Tursiops truncatus) in the Moray Firth (Northeast Scotland) are characterised by steep seabed gradients, which may act as a physical barrier against which to herd prey (Hastie et al., 2003; Bailey and Thompson, 2010). While the individual oceanographic components that characterise foraging sites (e.g. currents, water column stratification, tidal fronts) can be modelled at high resolution, processing and interpretation of such data can be challenging, often requiring specific expertise. Moreover, the hydrodynamic conditions that characterise foraging sites may vary spatially and temporally (e.g. across seasons). Capturing such complexities in models of predator-habitat association is challenging, particularly when studying a species across entire movement tracks rather than at isolated known foraging sites. Furthermore, although hydrodynamic covariates have been shown to be important for a range of species including some aerial and pelagic predators (Cox et al., 2018), the habitat associations of benthic predators may be better described by covariates relating to seabed characteristics. Such static metrics may prove more accessible and tractable indicators of important ecological areas, upon which dynamic covariates can later be added to investigate the specific mechanisms underpinning the importance of discrete areas. Singular metrics of topographic gradients, such as slope and aspect, are frequently used in such studies of marine predator habitat associations. An advantage of such metrics is that they can be computed across wide spatial extents, such as ocean basins. However, given the complexity of processes linking topography to prey described above, such metrics only provide limited ecological insight into predator-habitat associations, especially when used in isolation (Bouchet et al., 2015). Moreover, gradients are computed at a singular user-defined spatial scale, and do not provide insight into geological context or function. For example, it is impossible to determine the relative importance of areas near the top or bottom of a slope using gradient alone. Instead, classification of the seabed into features which have distinct geomorphological shapes provides more information about the underlying structure of the seabed (Bouchet et al., 2015).
In the terrestrial environment, geomorphology has been used to investigate the drivers of movements of wide-ranging species, such as elk (Cervus sp.), where the direction of movement was found to be dependent on the topography of the landscape (Kie et al., 2005). For snow leopards (Uncia uncia), geomorphology has also been found to influence behaviour, with features such as ridgelines and cliffs being used for both daytime resting and travelling (Jackson, 1996). In the marine environment, seabed geomorphology has been mapped for the global ocean for geological applications, categorising seabed features into a set of landforms (Harris et al., 2014), and a study has combined bathymetric data with seabed backscatter data from acoustic surveys to generate high resolution seabed classifications (“bathymorphons”) for a specific study area (Masetti et al., 2018). However, only a handful of studies have considered such data in the context of predator-habitat associations (Torres et al., 2013; Bouchet et al., 2020; Claro et al., 2020). These studies have generally investigated the influence of broadscale features, such as canyons, seamounts and plateaus on pelagic species, and the influence of topographic features on shelf sea predators remains largely unstudied using geomorphology. Moreover, the geomorphological dataset presented in Harris et al. (2014) is not resolved to identify discrete features in shelf seas. However, the “geomorphon” pattern recognition algorithm developed by Jasiewicz and Stepinski (2013) presents an opportunity to generate new geomorphological datasets from digital elevation model (DEM) or digital terrain model (DTM) data. Importantly, the algorithm is customisable such that a range of spatial resolutions can be explored, allowing the user to generate a landform map that is relevant to their research question and study area (Jasiewicz and Stepinski, 2013). Moreover, the pattern recognition process accounts for variation in the spatial magnitude of individual features, thus allowing features to be mapped at a range of scales (e.g. capturing both narrow and wide valleys). To our knowledge this approach has not been widely used in marine ecological studies, but when applied to bathymetry data, it provides an opportunity to investigate the potential influence of seabed topographic features on the behaviour of shelf sea predators at a spatial scale which is relevant to individual animals.
This study explores the utility of seabed geomorphological features as predictors of habitat use for highly mobile predators. Here we present a case study to: (i) employ the “geomorphon” algorithm to generate a dataset of seabed geomorphological features on a spatial scale that is relevant to the study species; (ii) examine whether inclusion of geomorphology as a covariate on state transition probabilities in a HMM applied to tracking data for three grey seals (Halichoerus grypus) in the southern North Sea improves the model fit over a null model (i.e. with no covariate effects); and (iii) consider to what extent this approach has the potential to provide ecological insights that will contribute information towards identifying important ecological areas for conservation management.
Grey seals are an ideal study species to explore the above research questions, as they are high trophic level predators inhabiting shelf seas of the North Atlantic. They regularly undertake multi-day foraging trips at sea, travelling up to hundreds of kilometres from terrestrial haulout sites, and thus are likely to encounter a wide range of topographic conditions (McConnell et al., 1999; Breed et al., 2009; Nowak et al., 2020). Moreover, their ecology has been studied in detail for decades (Thompson et al., 1991; McConnell et al., 1992; Russell et al., 2013), but understanding of the environmental mechanisms that underpin foraging behaviour remains incomplete. Grey seals have been model species for the development of animal movement HMM techniques (Jonsen et al., 2005; McClintock et al, 2012; Carter et al., 2020), and recent studies have begun to use HMMs to investigate the influence of environmental covariates on grey seal behaviour (van Beest et al., 2019; Nowak et al., 2020). However, no studies have examined how different static geomorphological features might influence their foraging decisions. The North Sea is particularly important habitat for this species, with the United Kingdom (UK) coast of the North Sea alone hosting ~25% of the global population (SCOS, 2020). Yet, this area is undergoing a process of intense change with the decommissioning of oil and gas infrastructure and the large-scale construction of wind farms, which overlap with seal habitat (Russell et al., 2014; Russell et al., 2016). Grey seals are protected in the UK under specific national legislation, as well as 2019 amendments to species and habitats regulations which retained the basic requirements of the European Union (EU) Habitats Directive in UK law after Brexit in 2021. As such, grey seal populations must still be maintained in “favourable conservation status”, in line with the EU Habitats Directive. Special Areas of Conservation (SACs) have been designated around the UK (Russell et al., 2019), yet these SACs are largely centred on coastal pupping and haulout sites and adjacent coastal areas. Moreover, the foraging distribution of grey seals may be hundreds of kilometres away from breeding and haulout sites (McConnell et al., 1999; Russell et al., 2013). Delineating important ecological areas offshore in the North Sea is therefore critical for effective marine spatial planning, and understanding the environmental characteristics of key foraging grounds is a research priority.
Methods
Tag Deployment and Study Area
The data used in this case study were from three adult grey seals tagged with Fastloc® GPS/GSM (Global System for Mobile Communications) devices (SMRU Instrumentation, UK) as part of a larger deployment in May 2015 (n = 20) at two haulout sites in Southeast England (Blakeney Point and Donna Nook) (see Russell, 2016). Seals were captured using hand nets, and anaesthetised with intravenous Zoletil100® (Virbac, France). The tagging procedure followed that of Sharples et al. (2012). Tags were glued to the fur on the back of the neck at the base of the skull using Loctite® 422™ cyanoacrylate instant adhesive (Henkel, UK); the tags then fall off by or during the annual moult. The tags collect positional information using Fastloc® GPS when the seal is at the surface or on land, as well as haul-out status using a wet/dry sensor. Data are stored in the buffer memory to be transmitted when the seal enters GSM range (McConnell et al., 2004). Table 1 outlines the tag deployment information for each seal.
The three individuals used in this case study were chosen as they had the widest ranging movements of the 20 tagged seals and thus were most likely to encounter a variety of different geomorphological features. The spatial extent of seal tracking data is shown in Figure 1. All three seals were either tagged in, or visited, haulout sites within the Humber Estuary SAC (Donna Nook) for which grey seals are a qualifying feature. The habitat covered by seal tracks encompasses much of the southwestern quadrant of the North Sea; an area of shallow but variable water depth (on average ~ 30 m deep; bathymetry shown in Figure 1). This area is predominantly sandy, featuring a large sandbank (Dogger Bank), rising to ~15 m below the surface, which separates seasonally stratified deeper waters of the northern North Sea from the shallower, mixed waters of the southern North Sea (Hill et al., 1993). This division of water bodies is punctuated by the Flamborough tidal mixing front, extending eastwards from the English coast around the fringes of the Dogger Bank (Hill et al., 1993). Dominant prey species for seals in this region include sandeels (Ammodytes spp.), flatfish, gadids and other benthic species associated with sandy substrates [e.g. dragonet (Callionymus lyra) and goby] (Wilson and Hammond, 2019).
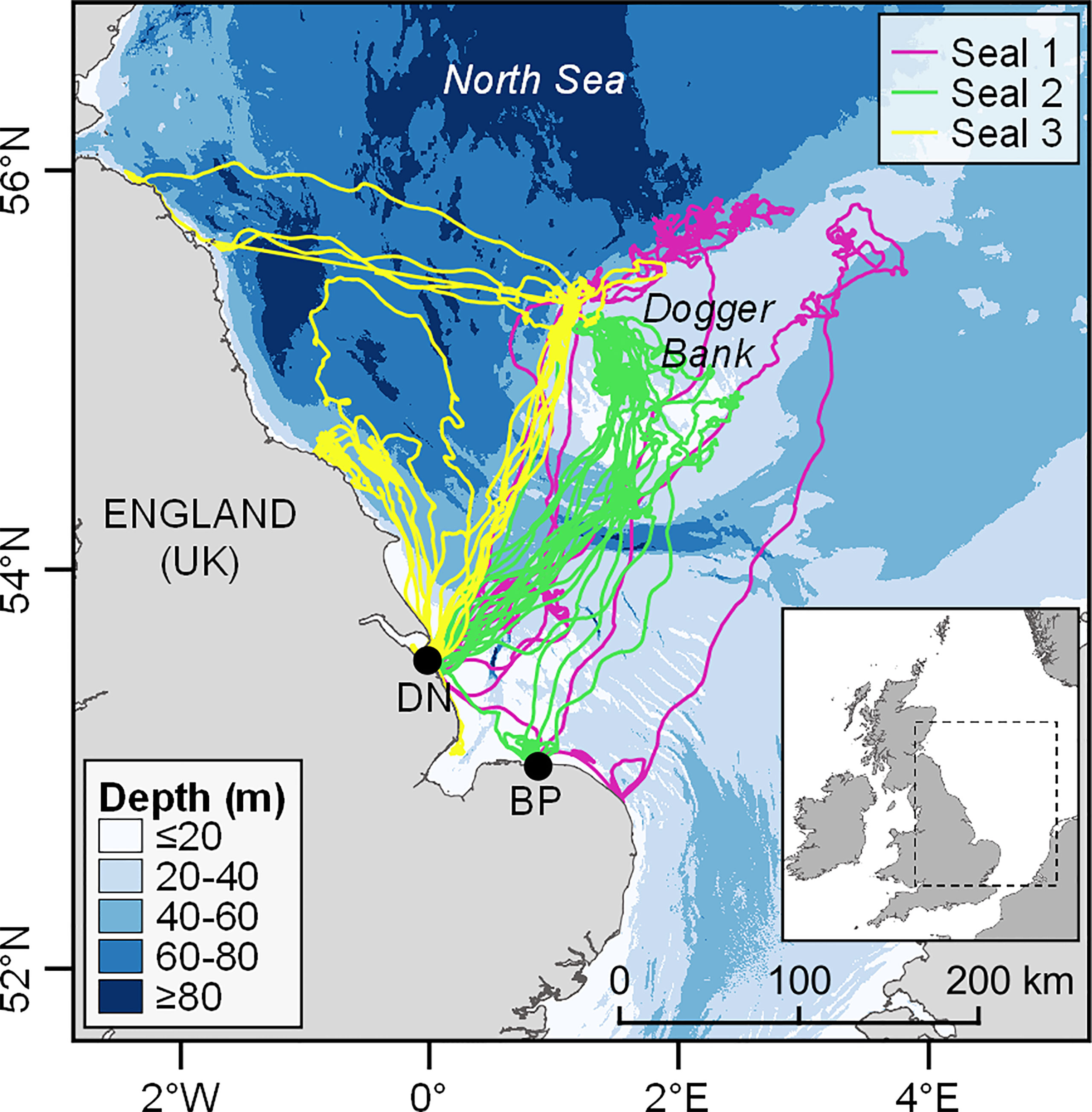
Figure 1 At-sea movements of the three tagged grey seals. Black dots indicate the tagging sites (DN = Donna Nook, BP = Blakeney Point). Bathymetry data: EMODnet Digital Bathymetry (DTM) (2016).
Processing of Movement Data
Location data for the three seals were cleaned to remove erroneous estimates following the protocol from Russell et al. (2015) using residual error threshold and number of satellites. Trips with < 10 observations were removed as they were unlikely to consist of foraging behaviour at sea and were more likely to be near-shore behaviour related to waiting for tidal haulout sites to become available (Thompson, 1989) (See Supplementary Material). Location data were regularised to a consistent time step of 1 hour using linear interpolation between pre- and post-location estimates, then any location that fell during a haul-out event was excluded, leaving only at-sea locations. For detail on the selection of a regularisation interval, see Supplementary Material. Any time interval for which there was a gap of > 2 hours between observed locations surrounding the interpolated point was flagged as ‘unreliable’ (Russell et al., 2015). These unreliable data points were included in the model, but the associated step lengths and turn angles were omitted. A state was assigned to these intervals by the HMM based on the Markov property, not on the state-dependent parameters, thus they did not influence the state distributions (Carter et al., 2020). See “Statistical Analysis” section below for HMM formulation. The location at the midpoint of the regularised time intervals was used for matching with environmental data (geomorphons; see below). The data were separated into trips, with the start of a trip identified by the end of a haul-out event, and the end of a trip when another haul-out event was initiated. Data within 10 km of the coast at mean high water were excluded using QGIS (QGIS Development Team, 2019) as it is impossible to distinguish between foraging and other near-shore behaviour (such as waiting for tidal haulout sites to become available) using location data.
Environmental Data
The “geomorphon” pattern recognition algorithm generates a raster layer of discrete landforms from DTM input data (Jasiewicz and Stepinski, 2013). A DTM for the North Sea was obtained from the European Marine Observation and Data Network (EMODnet Bathymetry Consortium, 2016) with a grid resolution of 250 m. The Geographic Resources Analysis Support System (GRASS GIS) (Neteler and Mitasova, 2007) extension r.geomorphon (Jasiewicz and Stepinski, 2013) was used to calculate the geomorphons in R (R Core Team, 2021). A raster was produced with grid cell values corresponding to one of the 10 landform elements which are most commonly recognisable in a typical terrestrial landscape (Figure 2). The algorithm requires three parameters to be set by the user, which can influence the geomorphology classification: (i) the maximum extent of a landform, known as the maximum lookup distance (this value needs to be relatively large for the landform elements across a range of sizes to be classified), (ii) the flatness threshold (degrees), below this value any terrain is classified as flat, and (iii) the spatial resolution of the DTM data (Stepinski and Jasiewicz, 2011). This method uses a line-of-sight principle (Yokoyama et al., 2002) for identifying the relationship of a central cell in the DTM raster with its neighbouring cells. This line-of-sight principle allows an incremental increase in lookup distance (up to the predefined maximum value) until minimum zenith and nadir angles along the eight compass directions are obtained between the central cell and the neighbouring cells (Stepinski and Jasiewicz, 2011). These minimum zenith and nadir angles are compared by the algorithm to identify if the central cell is higher, lower or the same elevation as its neighbours and this is converted into a ternary pattern which represents the geomorphon (Stepinski and Jasiewicz, 2011). There are, theoretically, 6561 possible topographic patterns, and in order to reduce this for mapping, the patterns are grouped into the ten most common landforms: flat, summit, ridge, shoulder, spur, slope, hollow, depression, valley and footslope (Jasiewicz and Stepinski, 2013) (Figure 2). The output of this algorithm is a raster with values for each grid cell corresponding to one of the ten common landform elements mentioned above. Importantly, the algorithm allows the final lookup distance for each cell to be determined automatically, and to be self-adaptive. This means that the geomorphons are mapped at a range of spatial extents, thus allowing for the recognition of landforms of a variety of sizes (e.g., identifying both narrow and broad valleys) (Stepinski and Jasiewicz, 2011). Moreover, the option to customise algorithm parameters allows the user to produce a landform map that is relevant to their research question, depending on the spatial extent and resolution of interest.
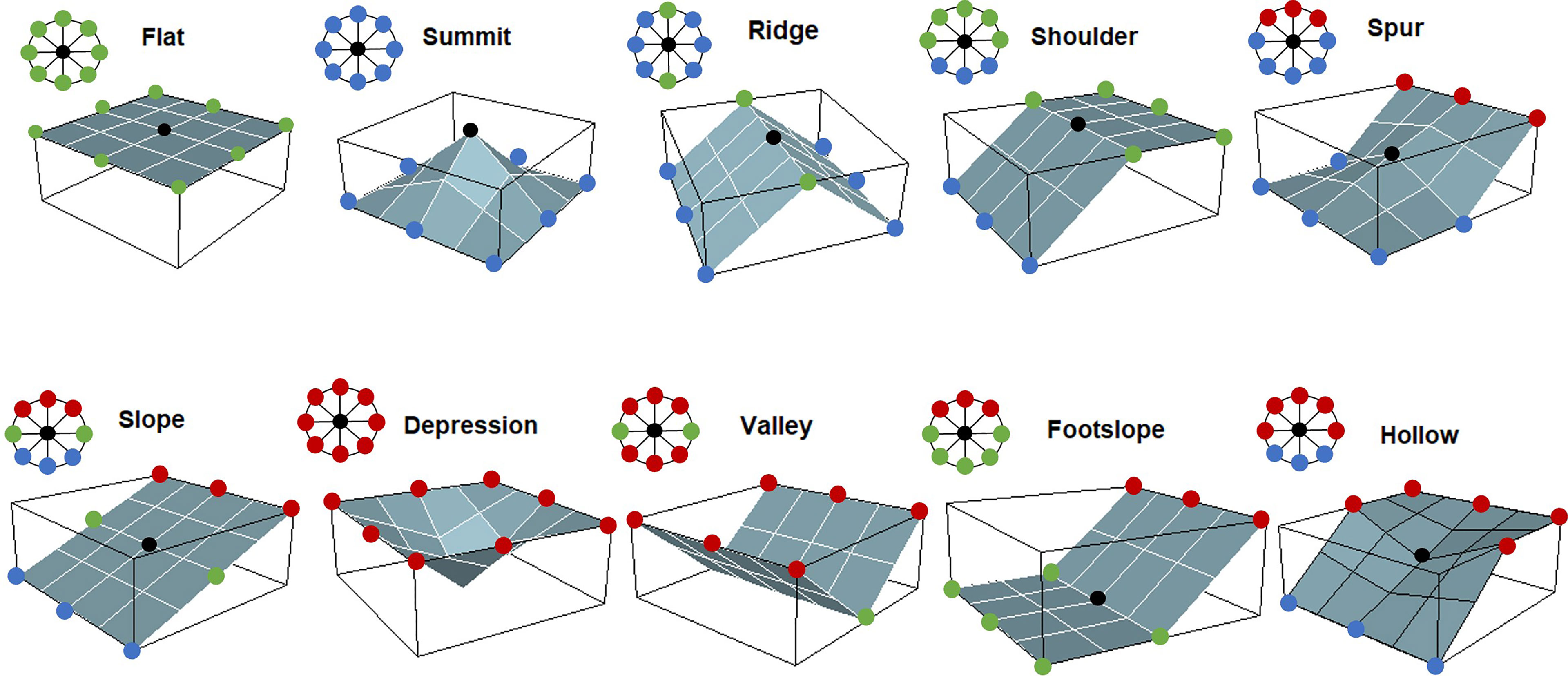
Figure 2 Representative 3D morphologies and their equivalent geomorphons (ternary patterns) for the 10 most common landform elements [after Figure 3 from Jasiewicz and Stepinski (2013)]. Coloured dots indicate if the cells surrounding the central cell (black) are higher (red), lower (blue) or of the same elevation value (green) as the central cell.
In order to find the most suitable terrain classification for investigating how geomorphological features influence grey seal movements, a range of values for the three parameters (lookup distance, flatness threshold and spatial resolution of the DTM) were trialled. A stepwise procedure was applied by creating a matrix encapsulating all possible combinations of four distinct values for each parameter, giving a total of 64 combinations. The values in the matrix were chosen as they provided a large range in the possible geomorphology rasters; it was unknown which geomorphological scale would be most biologically relevant. Four different bathymetry resolutions were trialled (250 m, 500 m, 1000 m and 2000 m), with four different lookup distances (5 cells, 15 cells, 40 cells and 60 cells) and four different flatness thresholds (0.1, 0.25, 0.5 and 0.7 degrees). Relatively large values of lookup distance and flatness threshold would result in a terrain classification from a higher and broader viewpoint than from smaller values; smaller values would give a classification of the terrain from a finer spatial resolution and thus finer detailed features (Stepinski and Jasiewicz, 2011). Because the final lookup distance is determined automatically, and is self-adaptive, larger values of lookup distance would allow the identification of landforms of a variety of sizes (Stepinski and Jasiewicz, 2011). Higher flatness thresholds produce maps that are flatter, and the influence of this threshold increases disproportionately with coarseness of the resolution of the terrain data. Thus, values of < 1 degree were used to avoid over-classification of flat areas (Stepinski and Jasiewicz, 2011). For each combination of parameter values, a geomorphology raster was created, resulting in 64 candidate rasters. For each candidate raster, geomorphons were extracted for each seal location, and included in a HMM as a covariate effect on state transition probabilities (see “Statistical Analysis” section below). The R package momentuHMM (McClintock and Michelot, 2018) was used to formulate the HMM. The best configuration of parameters for the pattern recognition algorithm was determined using model selection by Akaike Information Criterion (AIC) (Burnham and Anderson, 2002).
To allow comparison among individuals in the effect of geomorphology on behaviour, one individual (Seal 1) was used for defining the best parameters for the pattern recognition algorithm, as this seal covered the widest area and the largest variety of geomorphons (Figure 1). Thus, it was assumed that the scale of the geomorphology perceived by each seal would be similar. The values for the different parameters that produced the geomorphology raster with the lowest AIC score when used in the covariate HMM for Seal 1 were: a bathymetry resolution of 2000 m; a lookup distance of 15 cells and a flatness threshold of 0.1 degrees. This configuration produced the model with lowest AIC score compared to other parameter configurations (4.67 points lower than the next best model; see Supplementary Material Table S1). The resulting geomorphology raster is shown in Figure 3. This geomorphology raster was used for extracting the geomorphons for each location for each seal using the “raster” package (Hijmans and van Etten, 2021) in R.
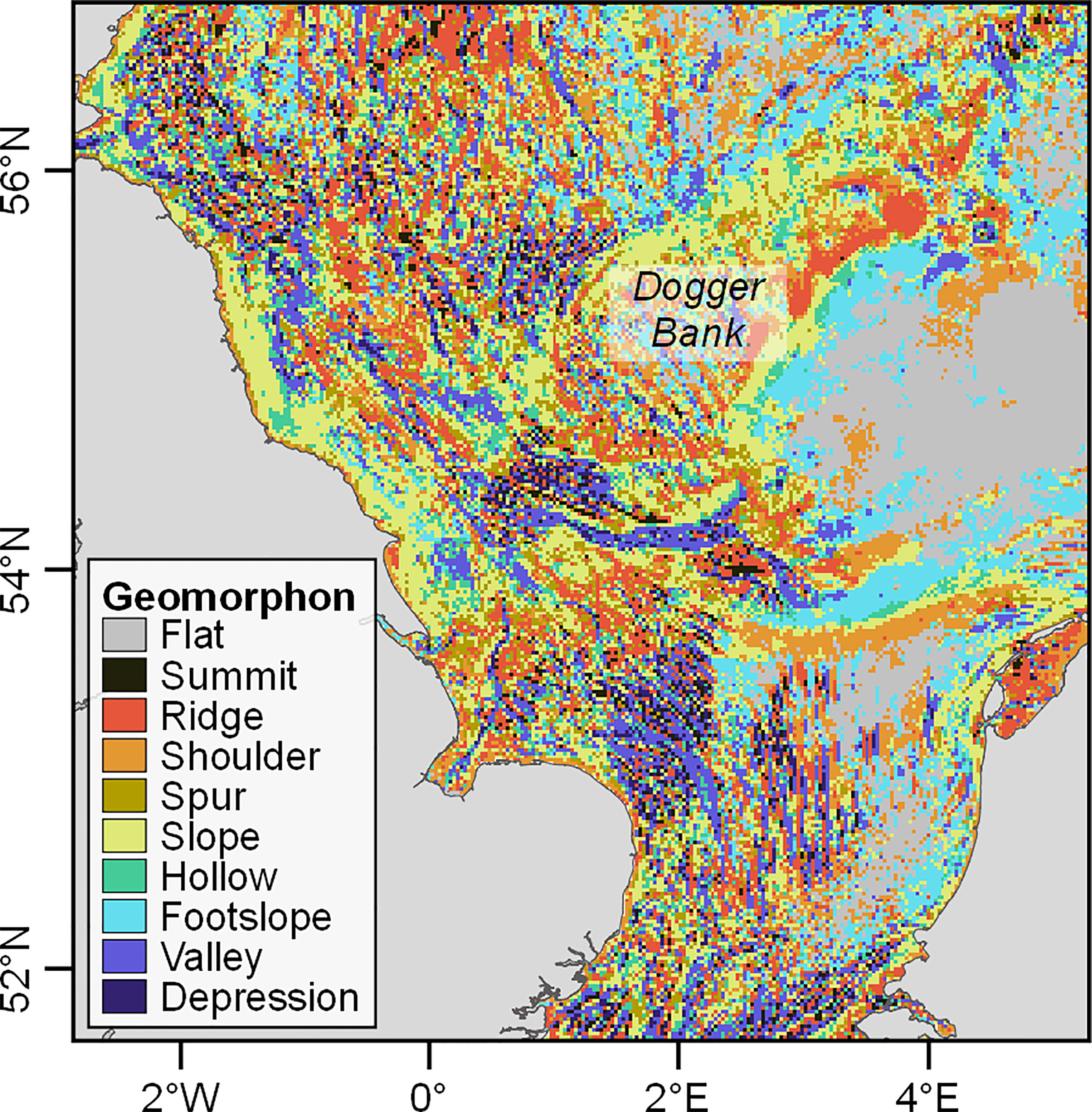
Figure 3 The geomorphology raster of the southern North Sea showing the distribution of the different geomorphons.
Statistical Analysis
To test the hypothesis that there is a relationship between seabed topography and seal behaviour, a two state HMM was fitted for each seal independently with a covariate effect of geomorphology (10 level factor) acting on state transition probabilities (covariate model). This covariate model was then compared to a two state HMM for each seal with no covariate effects (null model). The covariate model was deemed superior to the null model (no covariate effects) if it resulted in a reduction in AIC score of 2 points or more (Burnham and Anderson, 2002). The package momentuHMM (McClintock and Michelot, 2018) was used to formulate the models in R. HMMs were fitted with step lengths and change in bearing (turn angle) as the state-dependent data streams, in line with other studies of grey seal behaviour (Jonsen et al., 2005; Breed et al., 2009; Russell et al., 2015; Nowak et al., 2020). Models were fitted with two discrete movement states for each individual to provide a simple framework for testing the effect of geomorphology on behavioural state. The Viterbi algorithm was used to calculate the most probable state at each time step (Zucchini et al., 2016), and states were inferred as foraging or travelling based on prior knowledge of seal behaviour, and published examples of similar models (i.e. short step lengths and high turn angles = ARS (foraging), long step lengths and low turn angles = travelling) (Carter et al., 2020; Nowak et al., 2020). A Gamma distribution was assumed for the step lengths and a wrapped Cauchy distribution was assumed for the turn angles (see Carter et al., 2020 for mathematical notation). Starting values for the state-dependent parameters (mean and standard deviation for step lengths and the wrapped Cauchy concentration parameter for turn angles) must be specified for each state. A range of starting values for each state-dependent parameter were trialled until arriving at the global optimum for the negative log-likelihood (Michelot et al., 2016). Model validation checks were carried out by graphical checking of the pseudo-residuals (Zucchini et al., 2016), and no indication of issues relating to poor model fit were found. To facilitate a qualitative comparison of habitat use among individuals, the overall activity budgets (percentage of time spent foraging versus travelling) were estimated for each seal, alongside the percentage of total time spent per state at each geomorphon type.
Results
HMMs successfully identified two discrete movement states for each seal, inferred here as foraging and travelling (Supplementary Material Figure S5). Model selection by AIC score showed clear support for the covariate model (effect of geomorphons on state transition probabilities) over the null model (no covariate effects) for all three seals (Table 2). The AIC score of the covariate model was substantially lower than that of the null model for all three seals (ΔAIC = -17.3, -14.2 and -9.6 for Seals 1, 2 and 3 respectively; Table 2). Figure 4 shows the distribution of foraging locations for each seal, as inferred from the covariate model, colour-coded by the underlying geomorphology. Foraging sites for all seals occurred either within 50-70 km of the coast, or at sites approximately 200 km away from the haulout, overlapping with a variety of geomorphons (Figure 4). Foraging appeared to occur mostly on the fringes of the Dogger Bank for Seals 1 and 3, and on the bank itself for Seal 2. The foraging areas of Seal 3 appeared to overlap slightly with those of Seal 1, near the northern edge of the Dogger Bank (Figure 4). Foraging also occurred within 20 km of the coast for Seals 2 and 3 (Figure 4). The number and distribution of locations inferred as foraging was similar between the covariate model and the null model for all three seals. The total percentage of state assignments which agreed between the two models was 97.8% for Seal 1, 98.1% for Seal 2 and 91.3% for Seal 3.
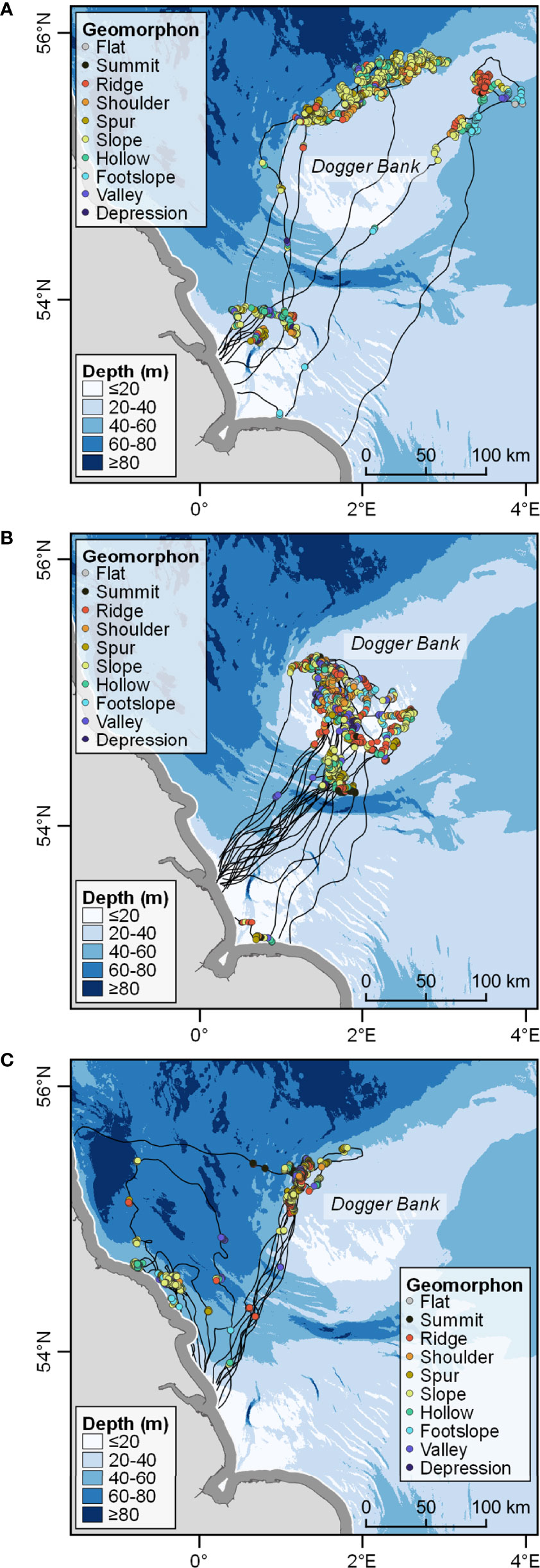
Figure 4 Movement of (A) Seal 1, (B) Seal 2 and (C) Seal 3 with foraging locations classified according to the geomorphology for each location and travelling indicated by black lines. The 10 km coastal buffer within which locations were excluded is shown in dark grey. All three seals foraged in the vicinity of the Dogger Bank.
According to the covariate model, the activity budgets were qualitatively similar among all three seals; Seal 1 was in the putative foraging state 63% of the time, versus 61% for Seal 2 and 59% for Seal 3. Figure 5A and Table 3 show the mean effect of different geomorphons on the probability of switching from travelling to foraging states with associated 95% confidence intervals (CIs). Figure 5B shows the percentage of time spent in each state at each of the geomorphons across the whole track for each seal, alongside the relative mean percentage of time seals are expected to be in the foraging state based on activity budgets. There was a positive effect of footslope, slope and hollow on the probability of transitioning from travelling to foraging for all three seals, but the respective effect sizes varied among individuals. While the largest effect size for Seal 1 was recorded for footslope (0.3, 0.16-0.51 95% CIs) (Table 3, Figure 5A), the seal only encountered this feature on 4.9% (83) of locations (Table 3), and the ratio of foraging to travelling states at this feature appeared to be proportional to the overall activity budget (Figure 5B). Slope was the most frequently encountered feature for all three seals, and Seal 1 appeared to show a disproportionately high amount of foraging at this feature type (Figure 5B). The largest effect sizes for Seal 2 were recorded for footslope (0.21, 0.1-0.38) and shoulder (0.3, 0.19-0.43) and the seal appeared to show a disproportionately high amount of foraging at these feature types. Seal 2 also encountered these two feature types more frequently than the other two seals. The largest effect sizes for Seal 3 were recorded for footslope (0.1, 0.03-0.29) and slope (0.09, 0.06-0.13). Seal 3 appeared to show a disproportionately high amount of foraging at ridges and shoulders, despite these features not having a large influence on the transition probability from travelling to foraging. There was no effect of flat on state transition probability for any of the seals, but the number of locations that overlapped with this feature type was low (Table 3).
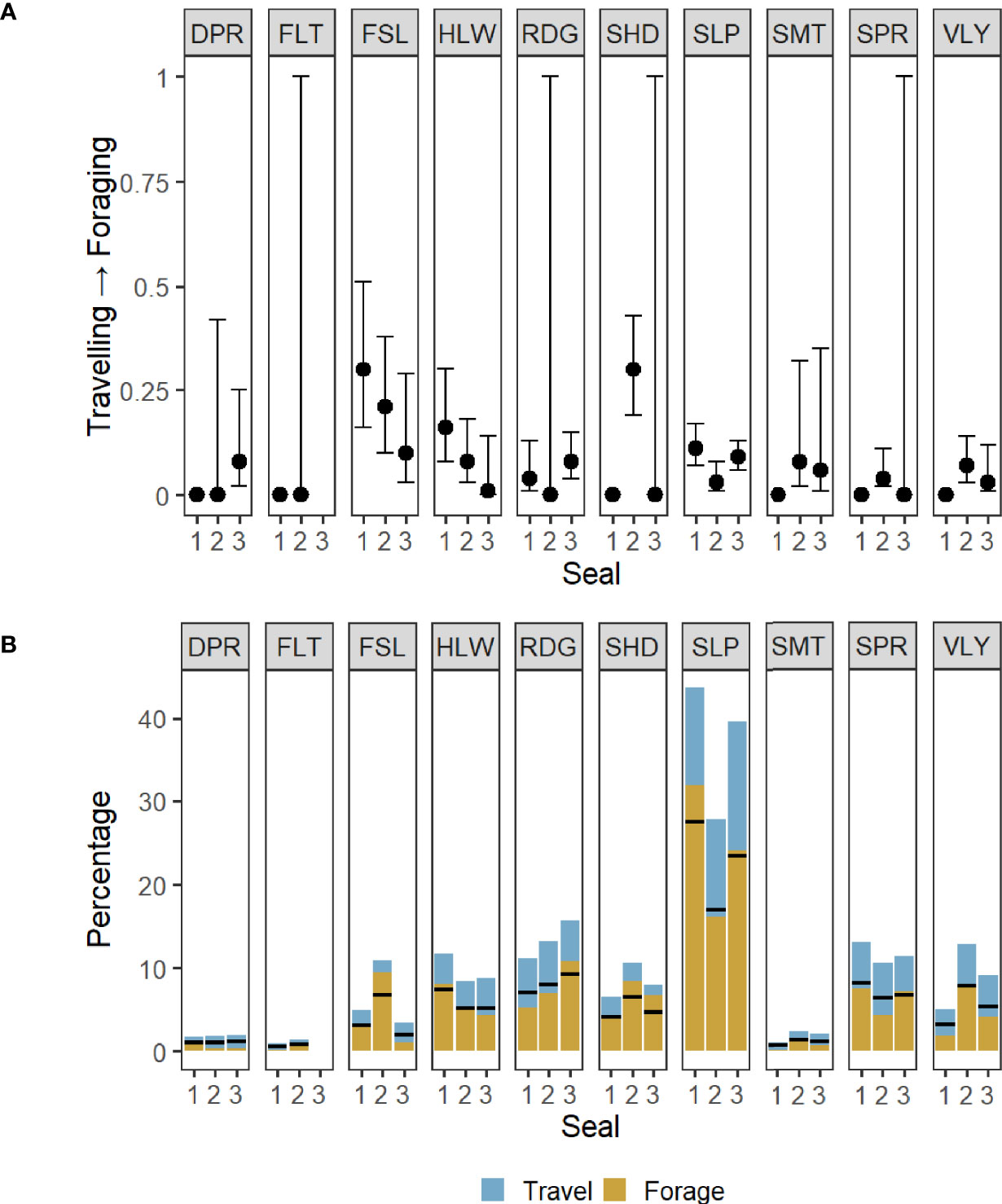
Figure 5 (A) Mean transition probabilities between behavioural states inferred from the states assigned by the covariate HMM with 95% confidence intervals. There was an increased likelihood of switching from travelling to foraging for all three seals in association with the following geomorphons: footslope (FSL), slope (SLP) and hollow (HLW). Where the confidence intervals are 0, seals never transitioned out of the state they were in upon encountering that feature. But where CIs are wide (0-1), although there may have been an influence of that feature on transitioning from foraging to travel, there was no instance of the seal transitioning out of travelling upon encountering that feature. (B) Percentage of total time (per seal) spent in foraging or travelling state at each feature type. Horizontal black bars show the relative mean percentage of time seals are expected to be in the foraging state based on activity budgets: Seal 1 = 63%, Seal 2 = 61%, Seal 3 = 59%. A disproportionately large amount of time was spent foraging over slope features for Seal 1, footslopes (FSL) and shoulders (SHD) for Seal 2, and ridges (RDG) and shoulders for Seal 3. For all feature type abbreviations see Table 3.
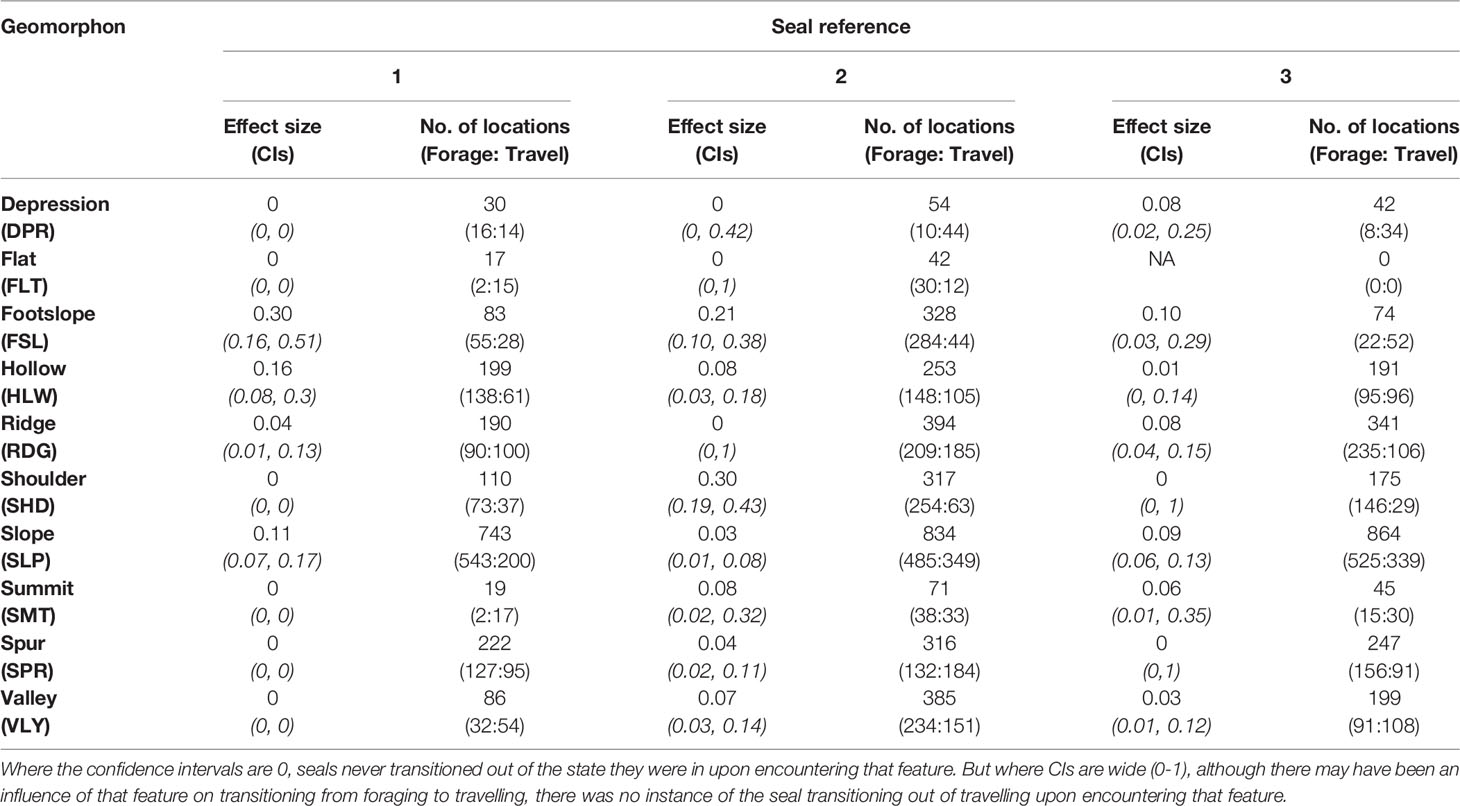
Table 3 Effect sizes with 95% confidence intervals (CIs) for the probabilities of transitioning between travelling and foraging for each seal for each geomorphon.
Discussion
This study aimed to explore the utility of a pattern recognition algorithm for classifying seabed geomorphological features (see Jasiewicz and Stepinski, 2013) to better understand the habitat use of mobile marine predators, using grey seals tagged in the southern North Sea as a case study. By modelling the seal movement data in a HMM framework with geomorphological features as a covariate acting on state transition probabilities, we found that geomorphological data improved the identification of behavioural states for the three seals studied, and offered new insights into foraging ecology which will aid the identification of important habitat.
Geomorphons impacted the state transitions for all three seals, providing evidence that seabed geomorphology does influence seal foraging behaviour. There were commonalities among the three individuals in the types of seabed geomorphology that had a positive influence on switching from travelling to foraging; slopes, footslopes and hollows were all associated with an increase in the probability of seals switching to foraging upon encountering them (Figure 5A). However, there was a mismatch between the feature types that were likely to increase the probability of switching from travelling to foraging, and feature types where the majority of foraging activity was concentrated. For example, Seal 3 was most likely to transition into ARS upon encountering footslopes and slopes compared to other feature types, but appeared to spend a disproportionately large amount of time foraging at ridges and shoulders (Figure 5). A seal travelling through the landscape towards an area of raised seabed, such as the Dogger Bank, is likely to encounter footslopes and slopes before arriving at shoulders or ridges (Figure 3). The mechanism underlying the influence of slopes and footslopes on the decision to switch to foraging may therefore be related to overspill of prey patches from good foraging habitat (i.e. ridges and shoulders) into surrounding areas. Similarly, the geomorphon with the largest influence on behaviour switching for Seal 1 was footslope, but the seal did not encounter this feature type frequently (only 4.9% of locations). HMMs are commonly used to understand the environmental drivers of foraging behaviour by examining covariate effects on state transition probabilities (Morales et al., 2004; Patterson et al., 2009; van Beest et al., 2019). However, our results highlight that the mechanisms that influence switching into foraging behaviour may be associated with different habitat characteristics to areas where foraging activity is concentrated. This is a key consideration when identifying important ecological areas for conservation management based on inference from covariate effects on state transitions in HMMs, particularly if the covariate is a categorical representation of habitat. We therefore recommend that habitat characteristics associated with state-switching behaviour should be considered in conjunction with the characteristics of areas where foraging activity is concentrated for a more complete understanding of the relationship between habitat and predator behaviour.
Slopes were the most frequently encountered feature type, associated with the greatest proportion of foraging activity, and had a positive effect on state transitions for all three seals (Figure 5). Slopes exposed to currents (e.g. on the fringes of the Dogger Bank where Seals 1 and 3 foraged) are known to produce tidal fronts and upwellings, leading to increased productivity and accumulation of plankton, and in turn, increased numbers of prey species (Ingram et al., 2007; Morato et al., 2010; Reisinger et al., 2018; Bouchet et al., 2020). Beyond the importance of slopes, there were differences among individuals in the variety of geomorphons that influenced the transition from travelling to foraging behaviour, and where foraging was concentrated (Figure 5). These differences between the seals could be caused by various factors. Firstly, individual preference has been shown to be an important factor in foraging behaviour of seals and other marine predators (Ostfeld, 1982; Tollit et al., 1998; Sargeant et al., 2005). Some individuals might have prior spatial memory of where certain geomorphological features exist, but different individuals might also have varying prey preferences and distinct prey capture strategies. Indeed, Carter et al. (2020) found that, for grey seal pups, foraging patches encountered on initial exploration after first leaving the natal colony are re-visited in subsequent directed foraging trips throughout early life, suggesting that spatial memory plays a key role in the development of foraging habitat preferences. Maxwell et al. (2012) also found that some individual northern elephant seals (Mirounga angustirostris) returned to the same seamount chain in the North Pacific Ocean on discrete trips, suggesting a maintained knowledge of profitable foraging areas. As well as differences in previous experience, sex differences in diet composition have been reported for grey seals in the Northwest Atlantic, which may relate to sex differences in body size and metabolic strategies (Beck et al., 2007). Sex-specific diet may translate to sex-specific associations with habitat. Given that we selected three seals which show reasonably discrete foraging areas for this case study, a further study on a larger dataset is required to examine possible sex differences in habitat associations, and if features used by all three seals studied here remain important at the population level.
Our results show that categorising seabed topography using geomorphological shapes can provide a tractable static covariate to investigate marine predator habitat use across the foraging range of an individual. This covariate may also provide further insight into predator-habitat associations when considered in studies focussed on discrete known foraging areas, such as the fringes of the Dogger Bank identified here for grey seals. Given the complexity of interactions between topography and tidal currents that underpin a wide variety of mechanisms affecting prey behaviour and distribution (Cox et al., 2018), geomorphons could be combined with hydrographic data, such as tidal currents and frontal intensity, to elucidate patterns of predator behaviour and space use at fine spatial and temporal scales. Hydrodynamic features resulting from an interplay between topography and currents can be modelled at increasingly fine resolution and included in models of predator habitat associations (Embling et al., 2012; Scott et al., 2013). However, such spatially and temporally discrete features applied to movement data across the individual’s foraging range are not likely to prove insightful. Moreover, although hydrodynamic features may provide important foraging habitat for aerial predators such as seabirds [as demonstrated by Scott et al. (2013)] and seals in tidally energetic coastal channels (Hastie et al., 2016), the role of tides may be less important for benthic foragers in the southern North Sea; our results show that individual grey seals remain in certain foraging patches across multiple tidal cycles during multi-day foraging trips (Figure 4). This suggests that other static components of habitat (such as the potential for certain topographic features to aggregate benthic prey) may be more influential drivers of behaviour than hydrodynamic processes for grey seals. However, an assumption about the movement data in this analysis was that the seals exhibited two behavioural states at sea. Seals routinely spend prolonged periods at the surface, which may be conflated with ARS when using location data in movement models (McClintock et al., 2013; Russell et al., 2015). This surface behaviour is likely related to digestion (Sparling et al., 2007), and thus probably occurs in foraging areas. While not accounting for resting therefore likely does not impact our findings, future studies of switching from foraging to resting in relation to tidal state may provide further insight into the temporal dynamics of habitat use. Exploring the influence of geomorphons on behaviour in conjunction with other static covariates is also an important avenue for future research. For example, although we have shown that encountering a certain feature type can influence the decision for seals to switch from foraging to travelling, the role of the environment in shaping how seals navigate to these features remains unclear. It is possible that other metrics such as depth gradients or changes in substrate type may be useful in elucidating how individuals navigate to fine-scale features. Moreover, combining geomorphons with information from other static covariates such as aspect in holistic habitat models may lead to better understanding of the functional significance of individual features.
The effect size of some of the geomorphons was small (< 0.1) and this could be related to the probability that a seal will encounter this feature type. For example, flat areas were not frequently encountered by any of the seals, and thus had no effect on foraging behaviour (Figure 5). However, large flat areas are present within the foraging range of the seals (Figure 3). To determine if certain features are preferentially selected or avoided, use of gemorphons could be modelled in a use-availability habitat preference framework (Aarts et al., 2008). Such models allow us to quantify if the use of a habitat type is proportionate to its availability within the environment accessible to the study animal (Aarts et al, 2008). However, a caveat of this approach is that it assumes that the individual has complete knowledge of their accessible environment, and thus chooses to go to certain areas despite the known existence of alternative habitat elsewhere. In reality this may not be the case. Nevertheless, combining insights from individual-focussed mechanistic studies such as ours with population level habitat preference models would be a valuable line of future investigation to understand the wider importance of different feature types and predict the location of important foraging areas.
Our case study has demonstrated the potential for this approach to provide useful information for conservation management. For example, the importance of the Dogger Bank is widely acknowledged for both commercial fisheries (Sell and Kröncke, 2013) and a range of predator species (Hamer et al., 2000; De Boer, 2010; Hammond et al., 2013; Gilles et al., 2016), yet the mechanisms influencing predator behaviour and distribution are less well understood. Our results suggest that slopes and slope-like features on and around the bank may be especially important for seals. The southern North Sea (and Dogger Bank in particular) is an area of rapid offshore wind development, with numerous large turbine arrays currently at various stages of planning, consenting, construction and operation. The impact of such developments on important prey species, and the implications for grey seal foraging habitat remain unknown. Russell et al. (2014) found that some individual harbour (Phoca vitulina) and grey seals repeatedly forage at established wind turbines and other anthropogenic structures, suggesting that novel foraging habitat is created due to artificial reef effects that arise from placing a solid structure in an otherwise sandy or soft sediment environment (Wright et al., 2020). However, artificial reefs may concentrate prey that would otherwise be distributed elsewhere, leading to increased competition and vulnerability to over-exploitation (Pickering and Whitmarsh, 1997). Moreover, seals are known to exhibit short-term avoidance of areas during pile driving for wind turbine installation (Russell et al., 2016), but it is unclear what the fitness consequences of such disturbance might be. The continued expansion of offshore anthropogenic structures in the North Sea may therefore have complex implications for marine predator foraging behaviour, as well as trophic dynamics and species interactions in the wider ecosystem. Further research is needed to understand the environmental characteristics of important foraging habitat, and the possible long-term consequences for predator populations of modifying such habitat.
Conclusion
This study found that incorporating seabed geomorphology into behavioural models improved predictions of habitat use by a marine predator in the southern North Sea and provided new insights into foraging behaviour. Although originally developed for terrestrial applications, we demonstrate how the geomorphon algorithm can be used to provide geomorphology datasets for shelf seas using bathymetry data, where other datasets (e.g. Harris et al., 2014) are not resolved to detect discrete seabed features. Metrics such as slope and aspect have been used to explore the environmental drivers of foraging in cetaceans (e.g. Hastie et al., 2003), seals (e.g. Maxwell et al., 2012) and seabirds (e.g. Suryan et al., 2006; Carpenter-Kling et al., 2020), and although these studies have provided some key insights into how predators exploit seabed terrain, habitat selection may be influenced by specific patterns beyond the presence and gradient of slope. Moreover, in highly heterogeneous environments such as shelf seas, a more complex representation of the seabed topography than simple gradient metrics may be necessary to understand its relationship to predator activity (Bouchet et al., 2020). Furthermore, where gradient computation requires a single user-defined scale to be set, the geomorphon algorithm allows flexibility in the size of mapped landforms, identifying landforms at the most appropriate scale for the habitat and study species (Stepinski and Jasiewicz, 2011). This provides a more tractable representation of the habitat, allowing better visualisation of the seabed terrain that individual animals encounter, and facilitating biological interpretation of movement models. When used in conjunction with other metrics, this approach may reveal key insights about scale-dependence of habitat associations and further our understanding of how predators interact with, and respond to, their environment. Our study highlights an avenue for future research to gain more ecological understanding of the mechanistic influence of seabed terrain on marine predator behaviour and distribution, and aid the identification and delineation of important habitats. Improving our understanding of what features characterise important predator foraging habitat is essential in assessing how marine ecosystems will be affected by changes caused by anthropogenic habitat modification, overfishing and climate change (McCauley et al., 2015). Moreover, better identification of important foraging areas for marine predators will lead to more effective marine spatial planning and conservation management for the wider ecosystem.
Data Availability Statement
Sources of environmental data are outlined in the methods section. Other data are available upon request from the authors. Requests to access these datasets should be directed to Dr Debbie Russell (dr60@st-andrews.ac.uk).
Ethics Statement
The animal study was reviewed and approved by the University of St Andrews Ethics Committee. Capture, handling and tagging of seals was carried out under UK Home Office project licence 60/4009 under the Animals (Scientific Procedures) Act 1986. The capture of seals was conducted under licence from the Marine Management Organisation (England) with necessary approvals from Natural England for work on designated sites.
Author Contributions
Designed the experiment: LB, DJFR, MIDC, HMEW. Collected the data: DJFR. Processed the data: HMEW, MIDC. Analysed the data: HMEW. Wrote the manuscript: HMEW and MIDC. Edited the manuscript: DJFR and LB. All authors reviewed the final version of the manuscript and gave consent for submission.
Funding
Department for Business, Energy, and Industrial Strategy (BEIS, formerly DECC) Offshore Energy Strategic Environmental Assessment programme funded the purchase of the tags and their deployment (OESEA-14-47) and supported HMEW (OESEA-20-118). DJFR was supported by NERC National Public Good- National Capability funding to SMRU (NE/R015007/1). MIDC was supported by project EcoSTAR, funded under NERC INSITE Phase II (grant no. NE/T010614/1). Funds for open access publication fees were from the research grants of supervisors at University of St Andrews.
Conflict of Interest
The authors declare that the research was conducted in the absence of any commercial or financial relationships that could be construed as a potential conflict of interest.
The reviewer PJB declared a shared affiliation, though no other collaboration, with the authors to the handling Editor.
Publisher’s Note
All claims expressed in this article are solely those of the authors and do not necessarily represent those of their affiliated organizations, or those of the publisher, the editors and the reviewers. Any product that may be evaluated in this article, or claim that may be made by its manufacturer, is not guaranteed or endorsed by the publisher.
Acknowledgments
We thank members of SMRU that were involved in fieldwork, particularly Simon Moss and Matt Bivins as well as SMRU Instrumentation and Hartley Anderson Ltd. for their support. We are grateful to the three reviewers that provided useful feedback and suggestions to improve the manuscript. Special thanks to Dr Bernie McConnell who shared his passion and curiosity for “what makes seals tick”, and first encouraged us to explore this technique.
Supplementary Material
The Supplementary Material for this article can be found online at: https://www.frontiersin.org/articles/10.3389/fmars.2022.818635/full#supplementary-material
References
Aarts G., MacKenzie M., McConnell B., Fedak M., Matthiopoulos J. (2008). Estimating Space-Use and Habitat Preference From Wildlife Telemetry Data. Ecography 31 (1), 140–160. doi: 10.1111/j.2007.0906-7590.05236.x
Bailey H., Thompson P. (2010). Effect of Oceanographic Features on Fine-Scale Foraging Movements of Bottlenose Dolphins. Marine Ecol. Prog. Ser. 418, 223–233. doi: 10.3354/meps08789
Barraquand F., Benhamou S. (2008). Animal Movements in Heterogeneous Landscapes: Identifying Profitable Places and Homogeneous Movement Bouts. Ecology 89 (12), 3336–3348. doi: 10.1890/08-0162.1
Beck C. A., Iverson S. J., Don Bowen W., Blanchard W. (2007). Sex Differences in Grey Seal Diet Reflect Seasonal Variation in Foraging Behaviour and Reproductive Expenditure: Evidence From Quantitative Fatty Acid Signature Analysis. J. Anim. Ecol. 76 (3), 490–502. doi: 10.1111/j.1365-2656.2007.01215.x
Block B. A., Jonsen I. D., Jorgensen S. J., Winship A. J., Shaffer S. A., Bograd S. J., et al. (2011). Tracking Apex Marine Predator Movements in a Dynamic Ocean. Nature 475 (7354), 86–90. doi: 10.1038/nature10082
Boehme L., Meredith M. P., Thorpe S. E., Biuw M., Fedak M. (2008a). Antarctic Circumpolar Current Frontal System in the South Atlantic: Monitoring Using Merged Argo and Animal-Borne Sensor Data. J. Geophysical Res.: Oceans 113 (C9), C09012. doi: 10.1029/2007JC004647
Boehme L., Thorpe S. E., Biuw M., Fedak M., Meredith M. P. (2008b). Monitoring Drake Passage With Elephant Seals: Frontal Structures and Snapshots of Transport. Limnol. Oceanography 53 (5.2), 2350–2360. doi: 10.4319/lo.2008.53.5_part_2.2350
Bouchet P. J., Letessier T. B., Caley M. J., Nichol S. L., Hemmi J. M., Meeuwig J. J. (2020). Submerged Carbonate Banks Aggregate Pelagic Megafauna in Offshore Tropical Australia. Front. Mar. Sci. 7. doi: 10.3389/fmars.2020.00530
Bouchet P. J., Meeuwig J. J., Salgado Kent C. P., Letessier T. B., Jenner C. K. (2015). Topographic Determinants of Mobile Vertebrate Predator Hotspots: Current Knowledge and Future Directions. Biol. Rev. 90 (3), 699–728. doi: 10.1111/brv.12130
Boyd I. L. (1996). Temporal Scales of Foraging in a Marine Predator. Ecology 77 (2), 426–434. doi: 10.2307/2265619
Boyd I. L., Murray A. W. A. (2001). Monitoring a Marine Ecosystem Using Responses of Upper Trophic Level Predators. J. Anim. Ecol. 70 (5), 747–760. doi: 10.1046/j.0021-8790.2001.00534.x
Breed G. A., Jonsen I. D., Myers R. A., Bowen W. D., Leonard M. L. (2009). Sex-Specific, Seasonal Foraging Tactics of Adult Grey Seals (Halichoerus Grypus) Revealed by State-Space Analysis. Ecology 90 (11), 3209–3221. doi: 10.1890/07-1483.1
Burnham K. P., Anderson D. R. (2002). “Model Selection and Multimodel Inference,” in A Practical Information-Theoretic Approach, 2 (New York: Springer).
Burns J. M., Costa D. P., Fedak M. A., Hindell M. A., Bradshaw C. J., Gales N. J., et al. (2004). Winter Habitat Use and Foraging Behavior of Crabeater Seals Along the Western Antarctic Peninsula. Deep Sea Res. Part II 51 (17-19), 2279–2303. doi: 10.1016/j.dsr2.2004.07.021
Camphuysen K. C., Shamoun-Baranes J., Bouten W., Garthe S. (2012). Identifying Ecologically Important Marine Areas for Seabirds Using Behavioural Information in Combination With Distribution Patterns. Biol. Conserv. 156, 22–29. doi: 10.1016/j.biocon.2011.12.024
Carpenter-Kling T., Reisinger R. R., Orgeret F., Connan M., Stevens K. L., Ryan P. G., et al. (2020). Foraging in a Dynamic Environment: Response of Four Sympatric Sub-Antarctic Albatross Species to Interannual Environmental Variability. Ecol. Evol. 10 (20), 11277–11295. doi: 10.1002/ece3.6766
Carter M. I. D., Bennett K. A., Embling C. B., Hosegood P. J., Russell D. J. F. (2016). Navigating Uncertain Waters: A Critical Review of Inferring Foraging Behaviour From Location and Dive Data in Pinnipeds. Movement Ecol. 4 (25), 1–20. doi: 10.1186/s40462-016-0090-9
Carter M. I. D., McClintock B. T., Embling C. B., Bennett K. A., Thompson D., Russell D. J. F. (2020). From Pup to Predator: Generalized Hidden Markov Models Reveal Rapid Development of Movement Strategies in a Naïve Long-Lived Vertebrate. Oikos 129 (5), 630–642. doi: 10.1111/oik.06853
Claro B., Pérez-Jorge S., Frey S. (2020). Seafloor Geomorphic Features as an Alternative Approach Into Modelling the Distribution of Cetaceans. Ecol. Inform 58, 101092. doi: 10.1016/j.ecoinf.2020.101092
Clay T. A., Joo R., Weimerskirch H., Phillips R. A., Den Ouden O., Basille M., et al. (2020). Sex-Specific Effects of Wind on the Flight Decisions of a Sexually Dimorphic Soaring Bird. J. Anim. Ecol. 89 (8), 1811–1823. doi: 10.1111/1365-2656.13267
Cox S. L., Embling C. B., Hosegood P. J., Votier S. C., Ingram S. N. (2018). Oceanographic Drivers of Marine Mammal and Seabird Habitat-Use Across Shelf-Seas: A Guide to Key Features and Recommendations for Future Research and Conservation Management. Estuar. Coast. Shelf Sci. 212, 294–310. doi: 10.1016/J.ECSS.2018.06.022
De Boer M. N. (2010). Spring Distribution and Density of Minke Whale Balaenoptera Acutorostrata Along an Offshore Bank in the Central North Sea. Marine Ecol. Prog. Ser. 408, 265–274. doi: 10.3354/meps08598
Embling C. B., Illian J., Armstrong E., van der Kooij J., Sharples J., Camphuysen K. C. J., et al. (2012). Investigating Fine-Scale Spatio-Temporal Predator-Prey Patterns in Dynamic Marine Ecosystems: A Functional Data Analysis Approach. J. Appl. Ecol. 49, 481–492. doi: 10.1111/j.1365-2664.2012.02114.x
Embling C. B., Sharples J., Armstrong E., Palmer M. R., Scott B. E. (2013). Fish Behaviour in Response to Tidal Variability and Internal Waves Over a Shelf Sea Bank. Prog. Oceanogr 117, 106–117. doi: 10.1016/j.pocean.2013.06.013
EMODnet Bathymetry Consortium (2016). Emodnet Digital Bathymetry (DTM). Marine Inf. Service. doi: 10.12770/c7b53704-999d-4721-b1a3-04ec60c87238
Fauchald P., Tveraa T. (2003). Using First-Passage Time in the Analysis of Area-Restricted Search and Habitat Selection. Ecology 84 (2), 282–288. doi: 10.1890/0012-9658(2003)084[0282:UFPTIT]2.0.CO;2
Fedak M., Lovell P., McConnell B., Hunter C. (2002). Overcoming the Constraints of Long Range Radio Telemetry From Animals: Getting More Useful Data From Smaller Packages. Integr. Comp. Biol. 42 (1), 3–10. doi: 10.1093/icb/42.1.3
Genin A., Boehlert G. W. (1985). Dynamics of Temperature and Chlorophyll Structures Above a Seamount: An Oceanic Experiment. J. Marine Res. 43 (4), 907–924. doi: 10.1357/002224085788453868
Georges J.-Y., Bonadonna F., Guinet C. (2000). Foraging Habitat and Diving Activity of Lactating Subantarctic Fur Seals in Relation to Sea-Surface Temperatures at Amsterdam Island. Marine Ecol. Prog. Ser. 196, 291–304. doi: 10.3354/meps196291
Gilles A., Viquerat S., Becker E. A., Forney K. A., Geelhoed S. C. V., Haelters J., et al. (2016). Seasonal Habitat-Based Density Models for a Marine Top Predator, the Harbor Porpoise, in a Dynamic Environment. Ecosphere 7 (6), e01367. doi: 10.1002/ecs2.1367
Grecian W. J., Witt M. J., Attrill M. J., Bearhop S., Becker P. H., Egevang C., et al. (2016). Seabird Diversity Hotspot Linked to Ocean Productivity in the Canary Current Large Marine Ecosystem. Biol. Lett. 12 (8), 20160024. doi: 10.1098/rsbl.2016.0024
Hamer K. C., Phillips R. A., Wanless S., Harris M. P., Wood A. G. (2000). Foraging Ranges, Diets and Feeding Locations of Gannets Morus Bassanus in the North Sea: Evidence From Satellite Telemetry. Marine Ecol. Prog. Ser. 200, 257–264. doi: 10.3354/meps200257
Hammond P. S., Macleod K., Berggren P., Borchers D. L., Burt L., Cañadas A., et al. (2013). Cetacean Abundance and Distribution in European Atlantic Shelf Waters to Inform Conservation and Management. Biol. Conserv. 164, 107–122. doi: 10.1016/j.biocon.2013.04.010
Harris P. T., Macmillan-Lawler M., Rupp J., Baker E. K. (2014). Geomorphology of the Oceans. Marine Geol. 352, 4–24. doi: 10.1016/j.margeo.2014.01.011
Hastie G. D., Russell D. J., Benjamins S., Moss S., Wilson B., Thompson D. (2016). Dynamic Habitat Corridors for Marine Predators; Intensive Use of a Coastal Channel by Harbour Seals Is Modulated by Tidal Currents. Behav. Ecol. Sociobiol. 70 (12), 2161–2174. doi: 10.1007/s00265-016-2219-7
Hastie G. D., Wilson B., Thompson P. M. (2003). Fine-Scale Habitat Selection by Coastal Bottlenose Dolphins: Application of a New Land-Based Video-Montage Technique. Can. J. Zool. 81 (3), 469–478. doi: 10.1139/z03-028
Haury L. R., McGowan J. A., Wiebe P. H. (1978). “Patterns and Processes in the Time-Space Scales of Plankton Distributions,” in Spatial Pattern in Plankton Communities. Ed. Steele J. H. (Boston, MA: Springer), 277–327.
Heithaus M. R., Frid A., Wirsing A. J., Worm B. (2008). Predicting Ecological Consequences of Marine Top Predator Declines. Trends Ecol. Evol. 23 (4), 202–210. doi: 10.1016/j.tree.2008.01.003
Hijmans R. J., van Etten J. (2021). “Raster: Geographic Data Analysis and Modeling,” in R Package Version 3, 4–13. Available at: http://CRAN.R-project.org/package=raster.
Hill A. E., James I. D., Linden P. F., Matthews J. P., Prandle D., Simpson J. H., et al. (1993). Dynamics of Tidal Mixing Fronts in the North Sea. Philos. Trans. R. Soc. A Phys. Sci. Eng. 343, 431–446. doi: 10.1098/rsta.1993.0057
Hindell M. A., Reisinger R. R., Ropert-Coudert Y., Hückstädt L. A., Trathan P. N., Bornemann H., et al. (2020). Tracking of Marine Predators to Protect Southern Ocean Ecosystems. Nature 580 (7801), 87–92. doi: 10.1038/s41586-020-2126-y
Ingram S. N., Walshe L., Johnston D., Rogan E. (2007). Habitat Partitioning and the Influence of Benthic Topography and Oceanography on the Distribution of Fin and Minke Whales in the Bay of Fundy, Canada. J. Marine Biol. Assoc. United Kingdom 87 (1), 149–156. doi: 10.1017/S0025315407054884
Isojunno S., Sadykova D., DeRuiter S., Cure C., Visser F., Thomas L., et al. (2017). Individual, Ecological, and Anthropogenic Influences on Activity Budgets of Long-Finned Pilot Whales. Ecosphere 8 (12), e02044. doi: 10.1002/ecs2.2044
Jackson R. M. (1996). Home Range, Movements and Habitat Use of Snow Leopard (Uncia Uncia) in Nepal (London:University of London). Ph.D. Thesis.
Jasiewicz J., Stepinski T. F. (2013). Geomorphons – A Pattern Recognition Approach to Classification and Mapping of Landforms. Geomorphology 182, 147–156. doi: 10.1016/j.geomorph.2012.11.005
Jonsen I. D., Flemming J. M., Myers R. A. (2005). Robust State-Space Modelling of Animal Movement Data. Ecology 86 (11), 2874–2880. doi: 10.1890/04-1852
Kareiva P., Odell G. (1987). Swarms of Predators Exhibit “Preytaxis” If Individual Predators Use Area-Restricted Search. Am. Nat. 130 (2), 233–270. doi: 10.1086/284707
Kie J. G. (1999). Optimal Foraging and Risk of Predation: Effects on Behavior and Social Structure in Ungulates. J. Mammalogy 80 (4), 1114–1129. doi: 10.2307/1383163
Kie J. G., Ager A. A., Bowyer R. T. (2005). Landscape-Level Movements of North American Elk (Cervus Elaphus): Effects of Habitat Patch Structure and Topography. Landscape Ecol. 20 (3), 289–300. doi: 10.1007/s10980-005-3165-3
Kirchner T., Wiley D. N., Hazen E. L., Parks S. E., Torres L. G., Friedlaender A. S. (2018). Hierarchical Foraging Movement of Humpback Whales Relative to the Structure of Their Prey. Marine Ecol. Prog. Ser. 607, 237–250. doi: 10.3354/meps12789
Langrock R., King R., Matthiopoulos J., Thomas L., Fortin D., Morales J. M. (2012). Flexible and Practical Modelling of Animal Telemetry Data: Hidden Markov Models and Extensions. Ecology 93 (11), 2336–2342. doi: 10.1890/11-2241.1
Letessier T. B., Mouillot D., Bouchet P. J., Vigliola L., Fernandes M. C., Thompson C., et al. (2019). Remote Reefs and Seamounts Are the Last Refuges for Marine Predators Across the Indo-Pacific. PloS Biol. 17 (8), e3000366. doi: 10.1371/journal.pbio.3000366
Luschi P., Hays G. C., Papi F. (2003). A Review of Long-Distance Movements by Marine Turtles, and the Possible Role of Ocean Currents. Oikos 103 (2), 293–302. doi: 10.1034/j.1600-0706.2003.12123.x
Lydersen C., Nøst O. A., Lovell P., McConnell B. J., Gammelsrød T., Hunter C., et al. (2002). Salinity and Temperature Structure of a Freezing Arctic Fjord—Monitored by White Whales (Delphinapterus Leucas). Geophysical Res. Lett. 29 (23), 34–31. doi: 10.1029/2002GL015462
Masetti G., Mayer L. A., Ward L. G. (2018). A Bathymetry- and Reflectivity-Based Approach for Seafloor Segmentation. Geosciences 8, 14. doi: 10.3390/geosciences8010014
Maxwell S. M., Frank J. J., Breed G. A., Robinson P. W., Simmons S. E., Crocker D. E., et al. (2012). Benthic Foraging on Seamounts: A Specialized Foraging Behavior in a Deep-Diving Pinniped. Marine Mammal Sci. 28 (3), E333. doi: 10.1111/j.1748-7692.2011.00527.x
McCauley D. J., Pinsky M. L., Palumbi S. R., Estes J. A., Joyce F. H., Warner R. R. (2015). Marine Defaunation: Animal Loss in the Global Ocean. Science 347 (6219), 1255641. doi: 10.1126/science.1255641
McClintock B. T., King R., Thomas L., Matthiopoulos J., McConnell B. J., Morales J. M. (2012). A General Discrete-Time Modelling Framework for Animal Movement Using Multistate Random Walks. Ecol. Monogr. 82, 335–349. doi: 10.1890/11-0326.1
McClintock B. T., Michelot T. (2018). Momentuhmm: R Package for Generalized Hidden Markov Models of Animal Movement. Methods Ecol. Evol. 9 (6), 1518–1530. doi: 10.1111/2041-210X.12995
McClintock B. T., Russell D. J., Matthiopoulos J., King R. (2013). Combining Individual Animal Movement and Ancillary Biotelemetry Data to Investigate Population-Level Activity Budgets. Ecology 94 (4), 838–849. doi: 10.1890/12-0954.1
McConnell B., Beaton R., Bryant E., Hunter C., Lovell P., Hall A. (2004). Phoning Home-A New GSM Mobile Phone Telemetry System to Collect Mark-Recapture Data. Marine Mammal Sci. 20 (2), 274–283. doi: 10.1111/j.1748-7692.2004.tb01156.x
McConnell B. J., Chambers C., Nicholas K. S., Fedak M. A. (1992). Satellite Tracking of Grey Seals (Halichoerus Grypus). J. Zool. 226 (2), 271–282. doi: 10.1111/j.1469-7998.1992.tb03839.x
McConnell B. J., Fedak M. A., Lovell P., Hammond P. S. (1999). Movements and Foraging of Grey Seals in the North Sea. J. Appl. Ecol. 36 (4), 573–590. doi: 10.1046/j.1365-2664.1999.00429.x
Michelot T., Langrock R., Patterson T. A. (2016). Movehmm: An R Package for the Statistical Modelling of Animal Movement Data Using Hidden Markov Models. Methods Ecol. Evol. 7 (11), 1308–1315. doi: 10.1111/2041-210X.12578
Montevecchi W. A., Hedd A., Tranquilla L. M., Fifield D. A., Burke C. M., Regular P. M., et al. (2012). Tracking Seabirds to Identify Ecologically Important and High Risk Marine Areas in the Western North Atlantic. Biol. Conserv. 156, 62–71. doi: 10.1016/j.biocon.2011.12.001
Morales J. M., Haydon D. T., Frair J., Holsinger K. E., Fryxell J. M. (2004). Extracting More Out of Relocation Data: Building Movement Models as Mixtures of Random Walks. Ecology 85 (9), 2436–2445. doi: 10.1890/03-0269
Morato T., Hoyle S. D., Allain V., Nicol S. J. (2010). Seamounts Are Hotspots of Pelagic Biodiversity in the Open Ocean. Proc. Natl. Acad. Sci. U. S. A. 107, 9707–9711. doi: 10.1073/pnas.0910290107
Neteler M., Mitasova H. (2007). Open Source GIS: A GRASS GIS Approach (3rd Ed.) (New York: Springer Verlag).
Nowak B. V. R., Bowen W. D., Whoriskey K., Lidgard D. C., Flemming J. M., Iverson S. J. (2020). Foraging Behaviour of a Continental Shelf Marine Predator, the Grey Seal (Halichoerus Grypus), Is Associated With in Situ, Subsurface Oceanographic Conditions. Movement Ecol. 8 (1), 1–14. doi: 10.1186/s40462-020-00225-7
Ostfeld R. S. (1982). Foraging Strategies and Prey Switching in the California Sea Otter. Oecologia 53 (2), 170–178. doi: 10.1007/BF00545660
Pagano A. M., Williams T. M. (2021). Physiological Consequences of Arctic Sea Ice Loss on Large Marine Carnivores: Unique Responses by Polar Bears and Narwhals. J. Exp. Biol. 224 (Suppl_1), jeb228049. doi: 10.1242/jeb.228049
Patterson T. A., Basson M., Bravington M. V., Gunn J. S. (2009). Classifying Movement Behaviour in Relation to Environmental Conditions Using Hidden Markov Models. J. Anim. Ecol. 78 (6), 1113–1123. doi: 10.1111/j.1365-2656.2009.01583.x
Patterson T. A., Thomas L., Wilcox C., Ovaskainen O., Matthiopoulos J. (2008). State–Space Models of Individual Animal Movement. Trends Ecol. Evol. 23 (2), 87–94. doi: 10.1016/j.tree.2007.10.009
Photopoulou T., Heerah K., Pohle J., Boehme L. (2020). Sex-Specific Variation in the Use of Vertical Habitat by a Resident Antarctic Top Predator. Proc. R. Soc. B 287 (1937), 20201447. doi: 10.1098/rspb.2020.1447
Pickering H., Whitmarsh D. (1997). Artificial Reefs and Fisheries Exploitation: A Review of the “Attraction Versus Production” Debate, the Influence of Design and Its Significance for Policy. Fish Res. 31, 39–59. doi: 10.1016/S0165-7836(97)00019-2
Pinaud D., Weimerskirch H. (2007). At-Sea Distribution and Scale-Dependent Foraging Behaviour of Petrels and Albatrosses: A Comparative Study. J. Anim. Ecol. 76 (1), 9–19. doi: 10.1111/j.1365-2656.2006.01186.x
Pirotta E., Edwards E. W., New L., Thompson P. M. (2018). Central Place Foragers and Moving Stimuli: A Hidden-State Model to Discriminate the Processes Affecting Movement. J. Anim. Ecol. 87 (4), 1116–1125. doi: 10.1111/1365-2656.12830
QGIS Development Team. (2019). Qgis Geographic Information System (Open Source Geospatial Foundation Project). Available at: http://qgis.osgeo.org.
R Core Team. (2021). R: A Language and Environment for Statistical Computing (Vienna, Austria: R Foundation for Statistical Computing).
Reisinger R. R., Raymond B., Hindell M. A., Bester M. N., Crawford R. J., Davies D., et al. (2018). Habitat Modelling of Tracking Data From Multiple Marine Predators Identifies Important Areas in the Southern Indian Ocean. Divers. Distrib. 24 (4), 535–550. doi: 10.1111/ddi.12702
Russell D. J. F., Brasseur S. M. J. M., Thompson D., Hastie G. D., Janik V. M., Aarts G., et al. (2014). Marine Mammals Trace Anthropogenic Structures at Sea. Curr. Biol. 24 (14), R638–R639. doi: 10.1016/j.cub.2014.06.033
Russell D. J. F., Hastie G. D., Thompson D., Janik V. M., Hammond P. S., Scott-Hayward L. A., et al. (2016). Avoidance of Wind Farms by Harbour Seals Is Limited to Pile Driving Activities. J. Appl. Ecol. 53 (6), 1642–1652. doi: 10.1111/1365-2664.12678
Russell D. J. F., McClintock B. T., Matthiopoulos J., Thompson P. M., Thompson D., Hammond P. S., et al. (2015). Intrinsic and Extrinsic Drivers of Activity Budgets in Sympatric Grey and Harbour Seals. Oikos 124 (11), 1462–1472. doi: 10.1111/oik.01810
Russell D. J. F.. (2016). “Movements of Grey Seals that Haul Out on the UK Coast of the Southern North Sea”. in Sea Mammal Research Unit Report to the Department of Energy and Climate Change (OESEA-14-17), pp. 18.
Russell D. J. F., McConnell B., Thompson D., Duck C., Morris C., Harwood J., et al. (2013). Uncovering the Links Between Foraging and Breeding Regions in a Highly Mobile Mammal. J. Appl. Ecol. 50 (2), 499–509. doi: 10.1111/1365-2664.12048
Russell D. J. F., Morris C., Duck C., Thompson D., Hiby L. (2019). Monitoring Long-Term Changes in UK Grey Seal Pup Production. Aquat. Conserv. Mar. Freshw. Ecosyst 29, 24–39. doi: 10.1002/aqc.3100
Sargeant B. L., Mann J., Berggren P., Krützen M. (2005). Specialization and Development of Beach Hunting, A Rare Foraging Behavior, by Wild Bottlenose Dolphins (Tursiops Sp.). Can. J. Zool. 83 (11), 1400–1410. doi: 10.1139/z05-136
Schmitz O. J., Beckerman A. P., O’Brien K. M. (1997). Behaviorally Mediated Trophic Cascades: Effects of Predation Risk on Food Web Interactions. Ecology 78 (5), 1388–1399. doi: 10.1890/0012-9658(1997)078[1388:BMTCEO]2.0.CO;2
SCOS (2020). Scientific Advice on Matters Related to the Management of Seal Populations. Main Advice Rep. 2020 http://www.smru.standrews.ac.uk/files/2021/06/SCOS-2020.pdf.
Scott B. E., Webb A., Palmer M. R., Embling C. B., Sharples J. (2013). Fine Scale Bio-Physical Oceanographic Characteristics Predict the Foraging Occurrence of Contrasting Seabird Species; Gannet (Morus Bassanus) and Storm Petrel (Hydrobates Pelagicus). Prog. Oceanogr. 117, 118–129. doi: 10.1016/j.pocean.2013.06.011
Sell A. F., Kröncke I. (2013). Correlations Between Benthic Habitats and Demersal Fish Assemblages—A Case Study on the Dogger Bank (North Sea). J. Sea Res. 80, 12–24. doi: 10.1016/j.seares.2013.01.007
Sharples R. J., Moss S. E., Patterson T. A., Hammond P. S. (2012). Spatial Variation in Foraging Behaviour of a Marine Top Predator (Phoca Vitulina) Determined by a Large-Scale Satellite Tagging Program. PloS One 7 (5), e37216. doi: 10.1371/journal.pone.0037216
Skomal G. B., Braun C. D., Chisholm J. H., Thorrold S. R. (2017). Movements of the White Shark Carcharodon Carcharias in the North Atlantic Ocean. Marine Ecol. Prog. Ser. 580, 1–16. doi: 10.3354/meps12306
Sparling C. E., Georges J.-Y., Gallon S. L., Fedak M., Thompson D. (2007). How Long Does A Dive Last? Foraging Decisions by Breath-Hold Divers in a Patchy Environment: A Test of a Simple Model. Anim. Behav. 74 (2), 207–218. doi: 10.1016/j.anbehav.2006.06.022
Speakman C. N., Hoskins A. J., Hindell M. A., Costa D. P., Hartog J. R., Hobday A. J., et al. (2020). Environmental Influences on Foraging Effort, Success and Efficiency in Female Australian Fur Seals. Sci. Rep. 10 (1), 1–16. doi: 10.1038/s41598-020-73579-y
Stepinski T. F., Jasiewicz J. (2011). Geomorphons – A New Approach to Classification of Landforms. Proc. Geomorphometry 2011, 109–112.
Suryan R. M., Sato F., Balogh G. R., Hyrenbach K. D., Sievert P. R., Ozaki K. (2006). Foraging Destinations and Marine Habitat Use of Short-Tailed Albatrosses: A Multi-Scale Approach Using First-Passage Time Analysis. Deep Sea Res. Part II 53 (3-4), 370–386. doi: 10.1016/j.dsr2.2006.01.012
Thompson P. M. (1989). Seasonal Changes in the Distribution and Composition of Common Seal (Phoca Vitulina) Haul-Out Groups. J. Zool. 217 (2), 281–294. doi: 10.1111/j.1469-7998.1989.tb02488.x
Thompson D., Hammond P. S., Nicholas K. S., Fedak M. A. (1991). Movements, Diving and Foraging Behaviour of Grey Seals (Halichoerus Grypus). J. Zool. 224 (2), 223–232. doi: 10.1111/j.1469-7998.1991.tb04801.x
Tollit D. J., Black A. D., Thompson P. M., Mackay A., Corpe H. M., Wilson B., et al. (1998). Variations in Harbour Seal Phoca Vitulina Diet and Dive-Depths in Relation to Foraging Habitat. J. Zool. 244 (2), 209–222. doi: 10.1111/j.1469-7998.1998.tb00026.x
Torres L. G., Smith T. D., Sutton P., Macdiarmid A., Bannister J., Miyashita T. (2013). From Exploitation to Conservation: Habitat Models Using Whaling Data Predict Distribution Patterns and Threat Exposure of an Endangered Whale. Divers. Distrib 19, 1138–1152. doi: 10.1111/ddi.12069
Towner A. V., Leos-Barajas V., Langrock R., Schick R. S., Smale M. J., Kaschke T., et al. (2016). Sex-Specific and Individual Preferences for Hunting Strategies in White Sharks. Funct. Ecol. 30 (8), 1397–1407. doi: 10.1111/1365-2435.12613
van Beest F. M., Mews S., Elkenkamp S., Schuhmann P., Tsolak D., Wobbe T., et al. (2019). Classifying Grey Seal Behaviour in Relation to Environmental Variability and Commercial Fishing Activity – A Multivariate Hidden Markov Model. Sci. Rep. 9 (1), 5642. doi: 10.1038/s41598-019-42109-w
Weimerskirch H. (2007). Are Seabirds Foraging for Unpredictable Resources? Deep Sea Res. Part II 54 (3-4), 211–223. doi: 10.1016/j.dsr2.2006.11.013
Williams T. M., Estes J. A., Doak D. F., Springer A. M. (2004). Killer Appetites: Assessing the Role of Predators in Ecological Communities. Ecology 85 (12), 3373–3384. doi: 10.1890/03-0696
Wilmers C. C., Nickel B., Bryce C. M., Smith J. A., Wheat R. E., Yovovich V. (2015). The Golden Age of Bio-Logging: How Animal-Borne Sensors Are Advancing the Frontiers of Ecology. Ecology 96 (7), 1741–1753. doi: 10.1890/14-1401.1
Wilson L. J., Hammond P. S. (2019). The Diet of Harbour and Grey Seals Around Britain: Examining the Role of Prey as a Potential Cause of Harbour Seal Declines. Aquat. Conserv. 29, 71–85. doi: 10.1002/aqc.3131
Wright S. R., Lynam C. P., Righton D. A., Metcalfe J., Hunter E., Riley A., et al. (2020). Structure in a Sea of Sand: Fish Abundance in Relation to Man-Made Structures in the North Sea. ICES J. Marine Sci. 77 (3), 1206–1218. doi: 10.1093/icesjms/fsy142
Yokoyama R., Shirasawa M., Pike R. J. (2002). Visualizing Topography by Openness: A New Application of Image Processing to Digital Elevation Models. Photogrammetric Eng. Remote Sens. 68 (3), 257–266.
Keywords: hidden Markov models (HMMs), movement ecology, North Sea, geomorphons, grey seal (Halichoerus grypus), satellite telemetry and tracking
Citation: Wyles HME, Boehme L, Russell DJF and Carter MID (2022) A Novel Approach to Using Seabed Geomorphology as a Predictor of Habitat Use in Highly Mobile Marine Predators: Implications for Ecology and Conservation. Front. Mar. Sci. 9:818635. doi: 10.3389/fmars.2022.818635
Received: 19 November 2021; Accepted: 18 March 2022;
Published: 19 April 2022.
Edited by:
Tommy Clay, University of California, United StatesReviewed by:
James Waggitt, Bangor University, United KingdomPhil J Bouchet, University of St Andrews, United Kingdom
Jan Marcin Weslawski, Polish Academy of Sciences, Poland
Copyright © 2022 Wyles, Boehme, Russell and Carter. This is an open-access article distributed under the terms of the Creative Commons Attribution License (CC BY). The use, distribution or reproduction in other forums is permitted, provided the original author(s) and the copyright owner(s) are credited and that the original publication in this journal is cited, in accordance with accepted academic practice. No use, distribution or reproduction is permitted which does not comply with these terms.
*Correspondence: Matt I. D. Carter, bWlkY0BzdC1hbmRyZXdzLmFjLnVr; Hannah M. E. Wyles, aHc1OUBzdC1hbmRyZXdzLmFjLnVr