- 1Muséum national d’Histoire naturelle, Paris, France
- 2Laboratoire Ecologie, Systématique et Evolution, Université Paris-Saclay, CNRS, AgroParisTech, Ecologie Systématique et Evolution, Orsay, France
- 3Envirology SARL, Saint Père, France
- 4Rastoma, Réseau des acteurs de la sauvegarde des tortues marines en Afrique Centrale, Paris, France
- 5Istituto Superiore per la Protezione e la Ricerca Ambientale (ISPRA), Biodiversity Department, Roma, Italy
- 6Research Institute for Integrated Management of Coastal Areas, Universitat Politècnica de València, Gandía (Valencia), Spain
- 7Departament de Biologia Evolutiva, Ecologia i Ciències Ambientals and Institut de Recerca de la Biodiversitat (IRBio), Facultat de Biologia, Universitat de Barcelona, Barcelona, Spain
- 8Ethology Unit, Department of Biology, University of Pisa, Pisa, Italy
- 9Association Marineland, Antibes, France
- 10EcoOcéan Institut, Montpellier, France
- 11Aquarium La Rochelle, Centre d’Etudes et de Soins pour les Tortues Marines, Quai Louis Prunier, La Rochelle, France
- 12Centre d’Etude et de Sauvegarde des Tortues Marines de Méditerranée (CESTMed), Grau du Roi, France
- 13University of Sfax, Sfax Faculty of Sciences, Marine Biodiversity and Environment Laboratory, Sfax, Tunisia
- 14Centre for Ecology and Conservation, College of Life & Environmental Sciences, University of Exeter, Exeter, United Kingdom
- 15Department of Ecology, School of Biology, Aristotle University of Thessaloniki, Thessaloniki, Greece
- 16Société Herpétologique de France, Réseau Tortues Marines de Méditerranée Française, Paris, France
- 17CEFE, University of Montpellier, CNRS, EPHE-PSL University, IRD, Montpellier, France
- 18European Commission, Joint Research Centre (JRC), Ispra, Italy
- 19ALNITAK–Save The Med Foundation, Poligono Industrial de Marratxi, Balearic Islands, Spain
- 20Centro Internazionale in Monitoraggio Ambientale (CIMA) Research Foundation, Savona, Italy
- 21Marine Zoology Unit, Cavanilles Institute of Biodiversity and Evolutionary Biology, University of Valencia, Valencia, Spain
- 22Marine and Environmental Sciences Centre, Faculty of Sciences of the University of Lisbon, Lisbon, Portugal
- 23Institute of Marine Sciences-Okeanos, University of the Azores, Horta, Portugal
Environmental policies, including the European Marine Strategy Framework Directive (MSFD), generally rely on the measurement of indicators to assess the good environmental status (GES) and ensure the protection of marine ecosystems. However, depending on available scientific knowledge and monitoring programs in place, quantitative GES assessments are not always feasible. This is specifically the case for marine turtle species, which are listed under the Biodiversity Descriptor of the MSFD. Relying on an expert consultation, the goal of this study was to develop indicators and a common assessment approach to be employed by European Union Member States to evaluate the status of marine turtle populations in the frame of the MSFD. A dedicated international expert group was created to explore and test potential assessment approaches, in coherence with other environmental policies (i.e. Habitats Directive, OSPAR and Barcelona Conventions). Following a series of workshops, the group provided recommendations for the GES assessment of marine turtles. In particular, indicators and assessment methods were defined, setting a solid basis for future MSFD assessments. Although knowledge gaps remain, data requirements identified in this study will guide future data collection initiatives and inform monitoring programs implemented by EU Member States. Overall this study highlights the value of international collaboration for the conservation of vulnerable species, such as marine turtles.
1 Introduction
1.1 Marine turtle conservation in Europe: context and challenges
The ever-increasing anthropogenic pressures on marine environments, including the unsustainable use of ocean resources, pollution and the ongoing effects of climate change, have major impacts on biodiversity, altering marine ecosystems function and productivity (Jackson et al., 2001; Hoegh-Guldberg and Bruno, 2010; Halpern et al., 2015). Over the last decades, the observed degradation of marine environments, and the realization of its potential to backfire on human societies, have motivated multiple global initiatives, such as the Census of Marine Life (2000-2010), that have significantly increased our knowledge of the diversity, abundance and distribution of marine life worldwide (Ausubel et al., 2010; Visbeck, 2018). However, as more information becomes available, ensuring that newly acquired scientific knowledge is effectively translated into policy and management strategies has been one of the most pressing issues.
Linking ocean health to anthropogenic pressures to guide management is at the core of the Marine Strategy Framework Directive (MSFD) adopted in 2008 by European Union (EU) Member States (European Commission, 2008). The MSFD is the EU’s integrative management tool to protect European marine environments. Relying on an ecosystem-based approach, its main objective is to ensure that good environmental status (GES) is achieved and maintained through the assessment of 11 qualitative Descriptors describing the desired state of the environment (European Commission, 2008, Figure 1). Each Descriptor is associated with a set of criteria defining GES achievement for the state of the different ecosystem elements, and for the pressures and impacts deriving from anthropogenic activities and acting on the state. Similarly, indicators have been defined for each criterion to provide a measure of their status, generally relying on agreed threshold values (European Commission, 2017).
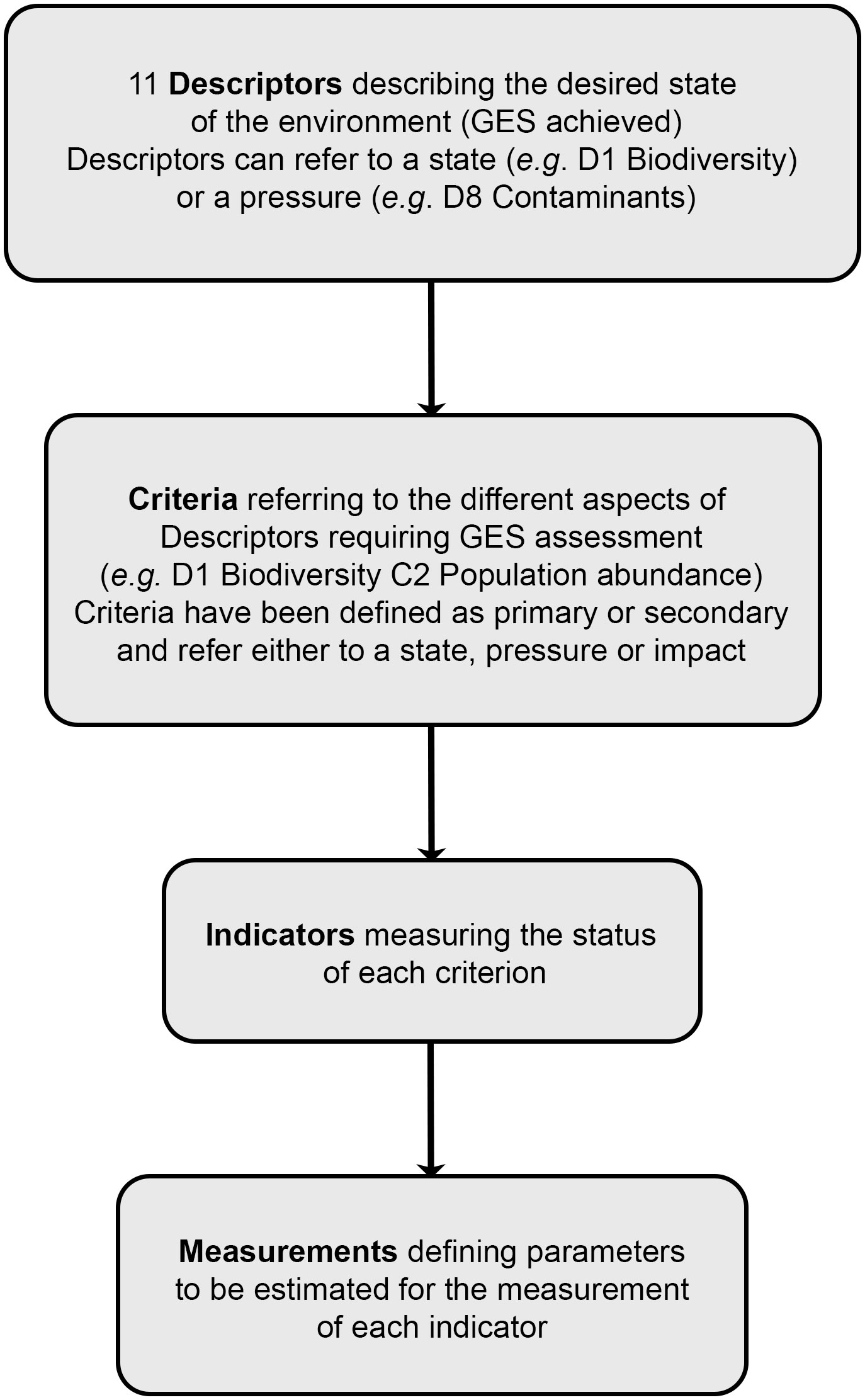
Figure 1 Flowchart illustrating the implementation structure of the Marine Strategy Framework Directive.
To optimize assessment at the EU level, the use of standardized assessment methods and consistent indicators by all member states is key to measure progress toward GES. However, such indicators have not always been clearly defined, resulting in inconsistent assessment methods (Palialexis et al., 2014; Palialexis and Boschetti, 2021). Disparities in assessment strategies (quantitative vs qualitative assessments) exist between, but also within, Descriptors. For instance, in the case of the Biodiversity Descriptor (Descriptor 1), unlike marine mammal, fish or bird species, no standardized assessment approaches or indicators have been agreed on at the EU or regional level for marine turtles, hindering quantitative assessments of the conservation status of these species.
Marine turtles are important components of marine ecosystems (Estes et al., 2016). In addition to their ecological importance, marine turtles have always had a high cultural and economic value to human societies (Frazier, 2005). Six out of the seven extent species frequent European waters, either as temporary visitors or, in the case of Mediterranean sub-populations of loggerhead and green turtles, as residents (Wallace et al., 2010a; Casale et al., 2018). All species occurring in European waters are listed in the International Union for Conservation of Nature’s (IUCN) Red List of threatened species (IUCN, 2020) and in the annexes of multiple European Directives (i.e. MSFD, Habitats Directive), global and Regional Sea Conventions (i.e. Bern and Bonn Conventions, Barcelona Convention, OSPAR) (Palialexis et al., 2018). To ensure the protection of marine turtle species, there is thus a clear need for precise assessment of their status, which should build upon different aspects of their complex life history and demography.
Marine turtles are highly mobile organisms that occupy distinct, and often, very distant habitats over different stages of their life cycle (Carr et al., 1978; Hays and Scott, 2013). Therefore, status assessments should ideally be carried out at the regional scale, requiring international collaboration. International initiatives are not only key for data sharing, but also for the development of standardized assessment strategies. For instance, such initiatives have led to the successful development of common indicators and standardized protocols to monitor and assess interactions between marine turtles and litter in the frame of the MSFD Marine Litter Descriptor (Descriptor 10) and other environmental policies, including the Barcelona Convention (Attia El Hili et al., 2018). A similar approach was applied to the MSFD Biodiversity Descriptor (Descriptor 1) at the scale of Macaronesia (Azores, Madeira and Canary Islands; Saavedra et al., 2018; Pipa et al., 2019).
Expanding on previous work, a two-year study was initiated in 2019 to develop common indicators and strategies for the assessment of marine turtle populations under MSFD Descriptor 1 at the EU scale. Relying on the consultation of an international group of experts and the analysis of shared datasets, the objectives of this study were to (1) define indicators to quantitatively assess the status of marine turtle populations, (2) propose standardized assessment methods taking into consideration the requirements of other relevant Directives and Conventions (i.e. Habitats Directive, OSPAR and Barcelona Conventions) and (3) identify data requirements for the assessment and monitoring of proposed indicators.
Here, we present the outcome of this study, specifically focusing on recommendations that resulted from the different expert workshops. Overall, we set the foundations for a common assessment framework and identify future priorities for marine turtle conservation in the frame of European Directives and Regional Sea Conventions.
1.2 Marine turtle species in EU waters
The species observed and their frequency generally varies between MSFD regions and subregions (Figure 2).
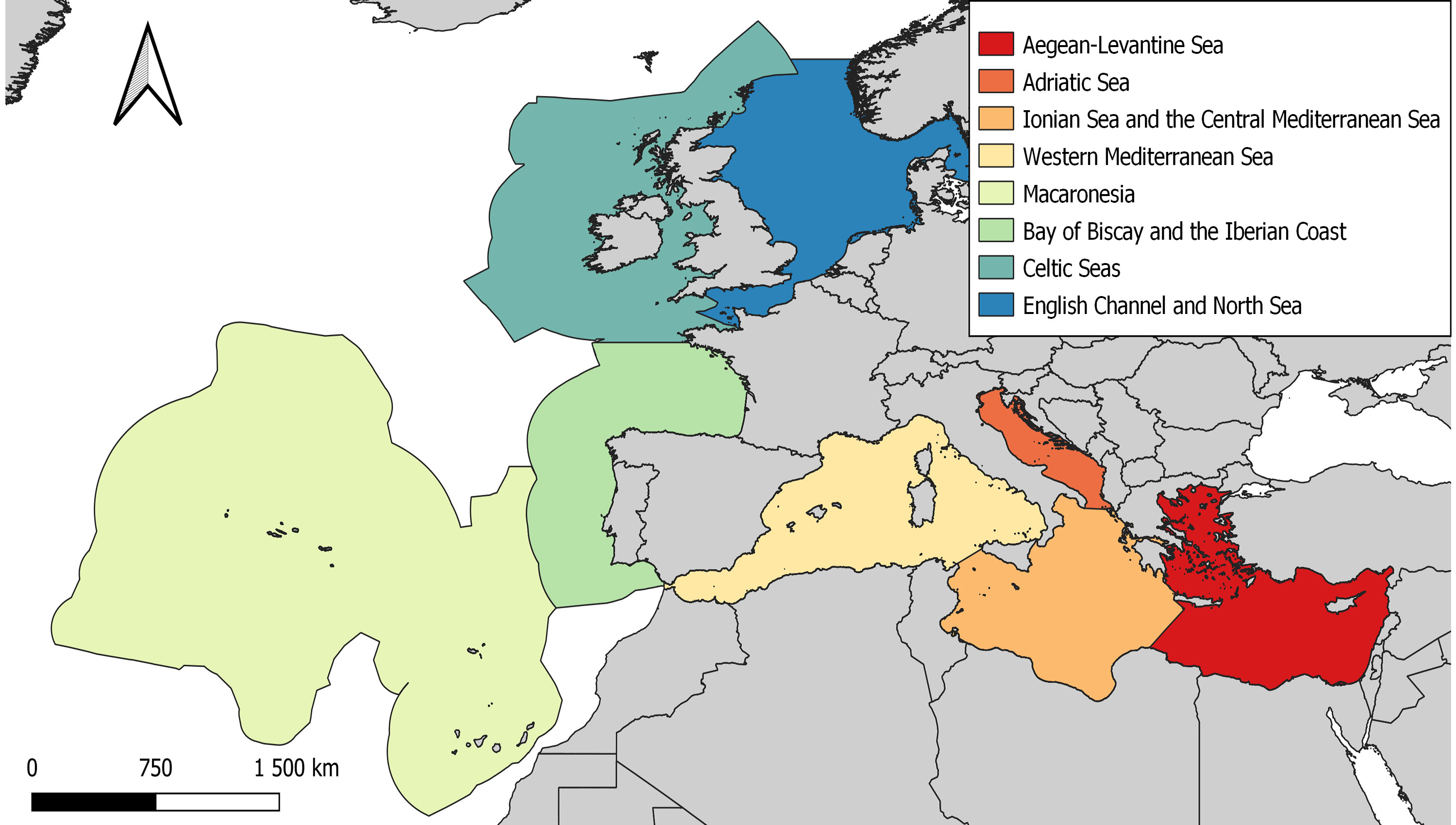
Figure 2 Map of MSFD subregions frequented by marine turtles. Shapefiles for this map were downloaded from the European Environment Agency website (www.eea.europa.eu).
Of the six marine turtle species frequenting the Mediterranean Sea, loggerhead and green turtles are the most commonly observed species (Casale et al., 2018). Although leatherback turtles are regularly reported in the Mediterranean, sightings of hawksbill, Kemp and olive ridley turtles remain rare (Laurent and Lescure, 1991; Casale et al., 2003; Tomás and Raga, 2007; Karaa et al., 2013; Revuelta et al., 2015; Bellido López et al., 2018). Mediterranean green turtles all belong to the same Regional Management Units (RMU; equivalent to sub-population) and frequent the eastern Mediterranean basin, where they nest (Wallace et al., 2010a; Casale et al., 2018). In the case of loggerheads, the Adriatic and Aegean-Levantine Seas are mostly frequented by individuals from the Mediterranean sub-population, while three sub-populations (Mediterranean, Northwest and Northeast Atlantic RMUs, Figure 3) co-occur in the Western and Central basins, with the abundances of individuals of Atlantic origin decreasing eastward (Maffucci et al., 2005; Carreras et al., 2006; Clusa et al., 2014; Loisier et al., 2021). Even though the number of sporadic nesting events in the western Mediterranean Sea has been increasing in the last few years (Maffucci et al., 2016; Carreras et al., 2018; Girard et al., 2021), nesting is almost entirely confined to the eastern Mediterranean basin (Margaritoulis et al., 2003; Casale et al., 2020b).
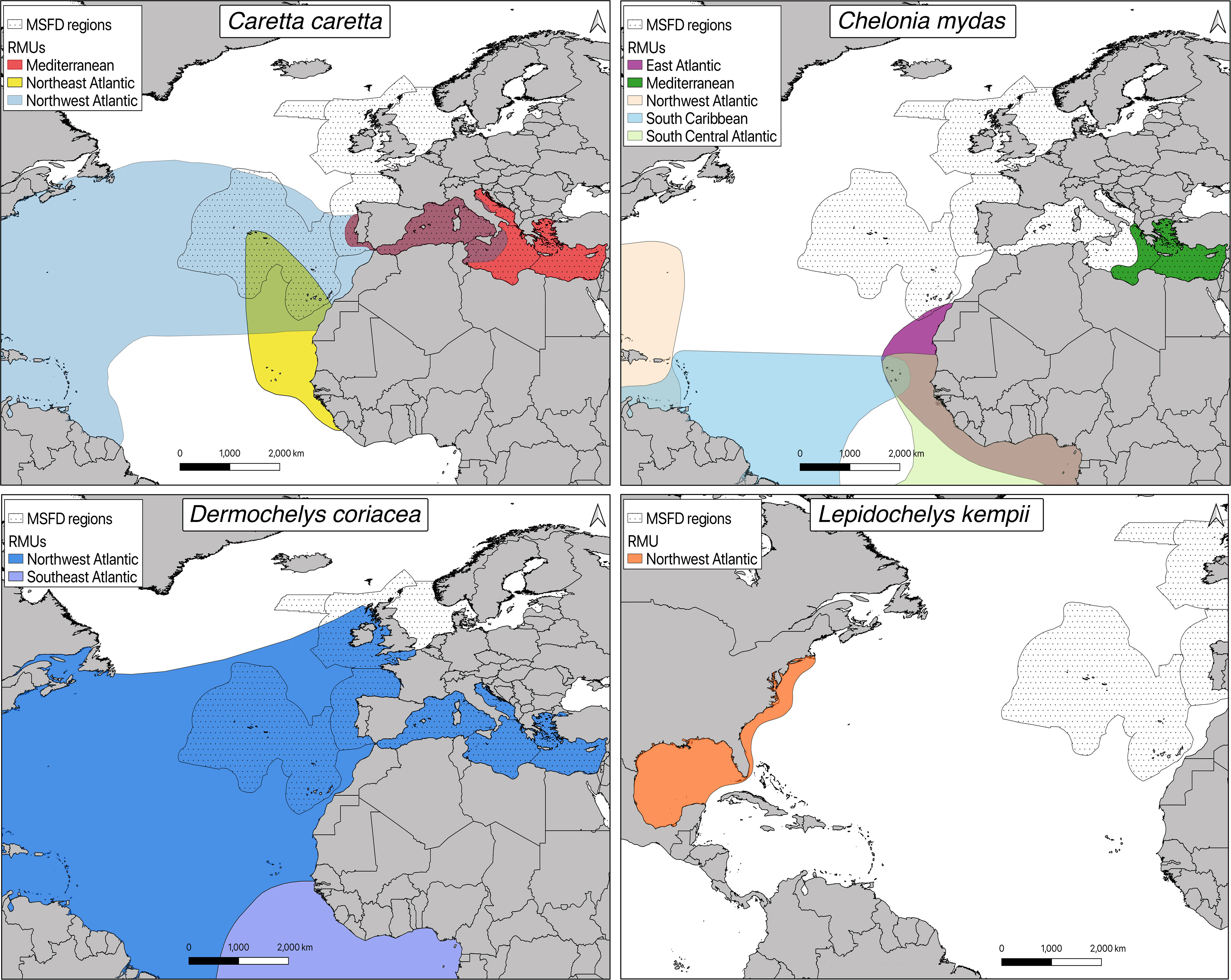
Figure 3 Maps representing the overlap between Regional Management Units (RMUs) for marine turtle species proposed for MSFD assessment and MSFD regions. Modified from Wallace et al., 2010a. RMU data were downloaded from the OBIS-SEAMAP website (http://seamap.env.duke.edu/swot; Kot et al., 2021).
In the case of the north-east Atlantic Ocean region, marine turtle species composition varies depending on the subregion considered. In the English Channel/North Sea, Celtic Seas and Bay of Biscay/Iberian coast subregions, leatherback turtles comprise the majority of observations, followed by loggerhead, Kemp’s ridley, and, more rarely, green turtles (Witt et al., 2007b). For the most part, recorded individuals are juveniles or subadults brought by current, travelling between, or occupying, north Atlantic foraging grounds (Witt et al., 2007b; Fossette et al., 2010; Avens and Dell’Amico, 2018). Conversely, the most abundant marine turtle species in Macaronesia is the loggerhead, followed by green turtle, which is primarily observed around the Canary Islands (Bolten, 2003; Carreras et al., 2014). Recorded individuals of both species are mainly juveniles originating from different Atlantic RMUs (Figure 3) that frequent foraging and developmental grounds off the Azores, Madeira and Canary islands (Bolten, 2003; Monzón-Argüello et al., 2018; Chambault et al., 2019). Other marine turtle species, including leatherback, hawksbill, Kemp’s ridley and olive ridley turtles are also occasionally observed in the Macaronesia subregion (Eckert, 2006; Varo-Cruz et al., 2017; Barcelos et al., 2021).
1.3 Overview of the policy landscape and stakeholders promoting marine turtle conservation in European waters
1.3.1 The Marine Strategy Framework Directive (MSFD)
The MSFD (2008/56/EC), adopted on 17 June 2008, aims to manage and protect marine environments across Europe (European Commission, 2008). More specifically, it aims to achieve and maintain GES such as “the environmental status of marine waters where these provide ecologically diverse and dynamic oceans and seas which are clean, healthy and productive”. In practice, GES is determined through the assessment of 11 qualitative Descriptors, and their associated criteria, related to ecosystems and human activities and pressures at the scales of pre-defined regions or sub-regions (European Commission, 2017; Figure 1).
Marine turtles are listed under the “Reptiles” species group of Descriptor 1 (D1), which states that “Biological diversity is maintained. The quality and occurrence of habitats and the distribution and abundance of species are in line with prevailing physiographic, geographic and climatic conditions”. As with other Descriptors, the status of marine turtle species must be assessed every six years, allowing member states to regularly update their marine strategy, as required by Article 17 of the MSFD (European Commission, 2008). Five criteria (four primary and one secondary) have been defined for assessment at the EU level (Table 1). Marine turtle species to be evaluated, on the other hand, are currently being selected at the national level.
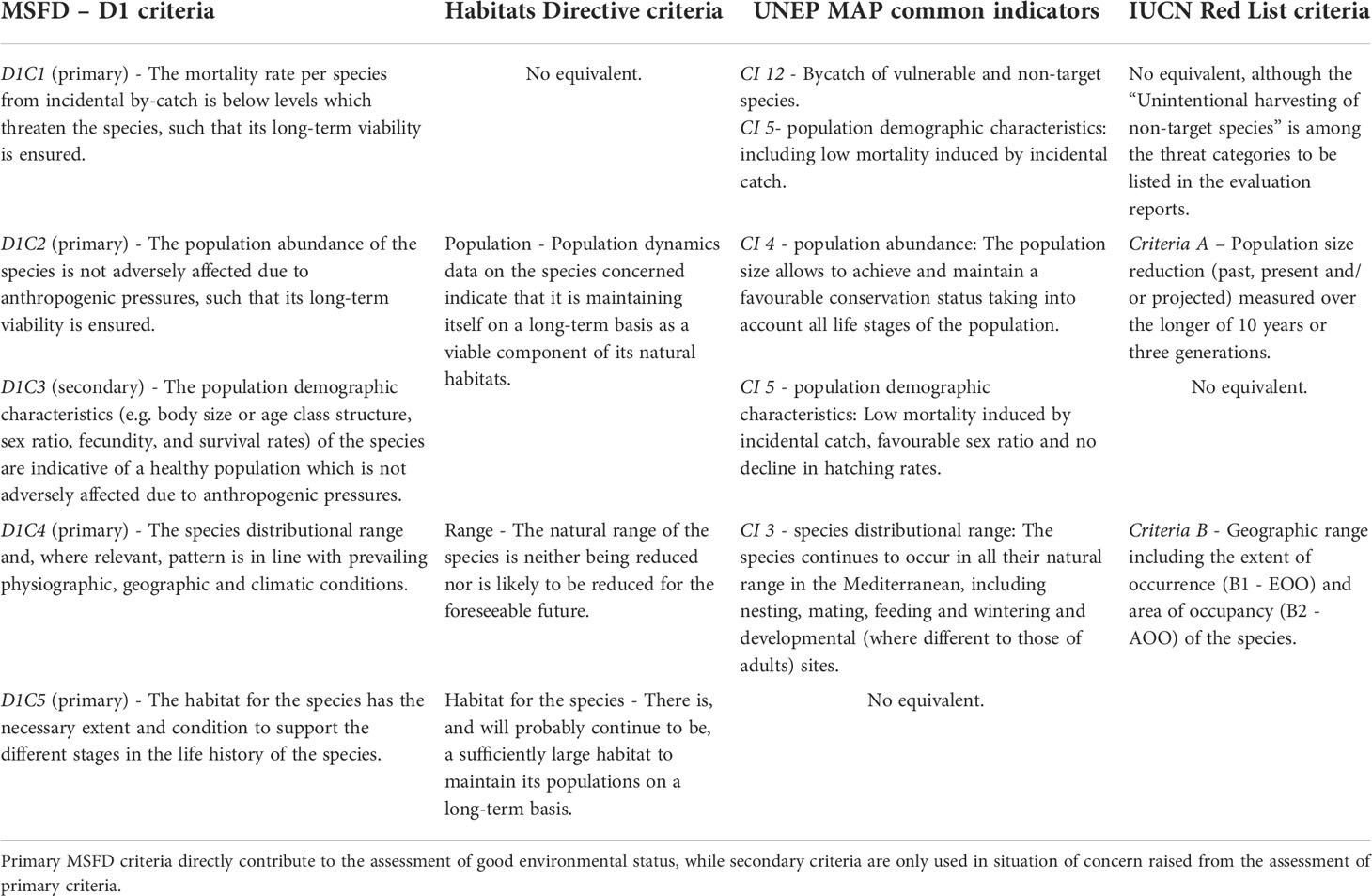
Table 1 Equivalence between MSFD Descriptor 1-Reptiles criteria (European Commission, 2017) and criteria/indicators used for assessment under the Habitats Directive, Barcelona Convention (UNEP MAP) and IUCN Red List.
Following an initial assessment in 2012, which set the baseline for subsequent evaluations, a first assessment was carried out in 2018 and a second is planned in 2024 (Figure 4). To date, no quantitative assessment of the status of marine turtle species has been carried out at the EU level (Palialexis et al., 2014; Palialexis and Boschetti, 2021).
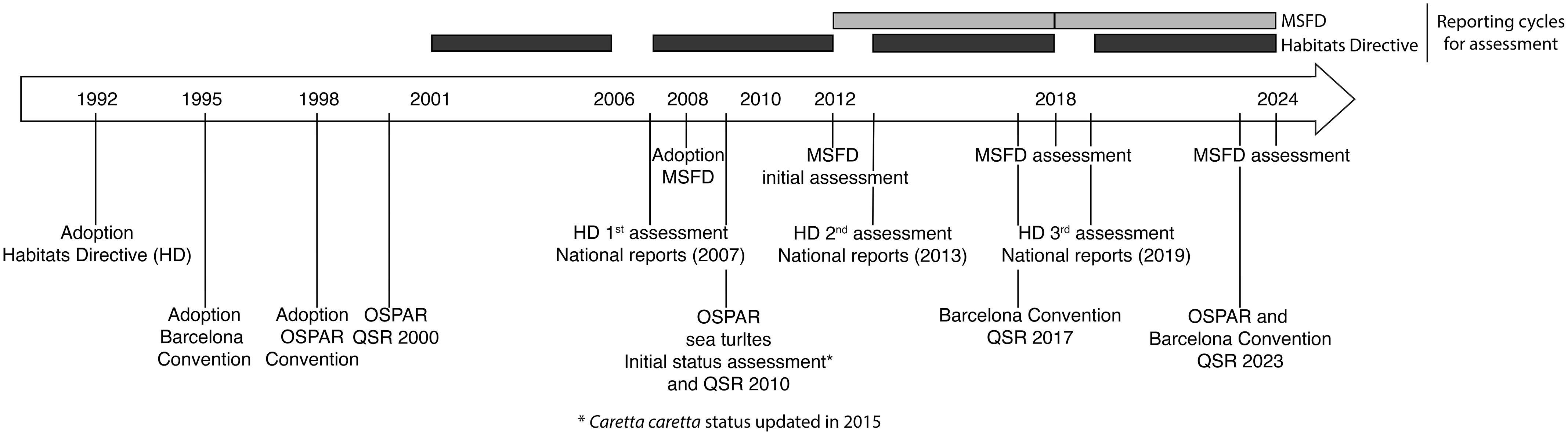
Figure 4 Timeline of reporting cycles and assessments under European Directives (Marine Strategy Framework Directive - MSFD, Habitats Directive - HD) and Regional Sea Conventions (OSPAR and Barcelona Convention). QSR refers to quality status reports.
1.3.2 The Habitats Directive
The HD (92/43/EEC) on the conservation of natural habitats and wild fauna and flora was adopted on 21 May 1992 (European Commission, 1992). Its overarching goal is to ensure that European habitats, and rare, threatened, or endemic species reach and remain at a favorable conservation status through the implementation of a wide range of conservation measures, including the establishment of an EU-wide Natura 2000 network of protected areas. As with the MSFD, Article 17 of the HD requires member states to assess the conservation status of species and habitats, and report on measures they have taken under the Directive every 6 years (Figure 4). At the end of each reporting period, member states must carry out the assessment in biogeographic and marine regions within their national territory following specific guidelines for harmonization (DG Environment, 2017). These national assessments are then aggregated to produce a final assessment at the European level. The timing of reporting under the HD does not entirely overlap with the MSFD, with three assessments published in 2007, 2013 and 2019, so one year after MSFD assessments.
Marine turtle species to be evaluated under the HD are listed in Annexes II (“Animal and plant species of community interest whose conservation requires the designation of special areas of conservation”) and IV (“Animal and plant species of community interest in need of strict protection”) of the Directive (European Commission, 1992). In particular, loggerhead (Caretta caretta) and green (Chelonia mydas) turtles are listed in Annex II, while five species are listed in Annex IV: Loggerhead, green, leatherback (Dermochelys coriacea), Kemp’s ridley (Lepidochelys kempii) and hawksbill (Eretmochelys imbricata) turtles. All criteria defined for the HD are equivalent to those of MSFD D1-Reptiles (Table 1) and can be evaluated across the same geographical areas. As with MSFD, so far, all assessments have been qualitative and mostly based on expert opinion.
1.3.3 The Barcelona Convention
The Barcelona Convention for the Protection of the Marine Environment and the Sustainable Development of the Coastal Areas of the Mediterranean was adopted in 1995, succeeding to the initial United Nations Environment Programme’s (UNEP) Mediterranean Action Plan (MAP) of 1975. Adopted by 22 Contracting Parties (21 countries and the EU), the main objectives of the Barcelona Convention are to protect marine and coastal environments by limiting pollution, and ensure the sustainable management of marine resources while strengthening solidarity between coastal states. To facilitate harmonization with the MSFD, the Integrated Monitoring and Assessment Programme of the Mediterranean Sea and Coast was adopted by Contracting Parties in 2016, providing a framework for integrated monitoring and assessment. As a result, common indicators and standard guidelines for monitoring these indicators have been developed. The first assessment was published in the quality status report of 2017, and a second is expected in 2023 (Figure 4).
All four common indicators relevant to marine turtles, are equivalent to MSFD D1-Reptiles criteria (Table 1). While only the loggerhead and green turtles were qualitatively assessed in 2017, all turtle species occurring in the Mediterranean Sea are listed in the Barcelona Convention.
1.3.4 The OSPAR Convention
The Convention for the protection of the marine environment of the north-east Atlantic was officially adopted in 1998 by 15 governments and the EU, all of which committed to take all possible steps to prevent and eliminate pollution and to take necessary measures to protect marine environments from human activities and restore them when adversely affected (OSPAR Commission, 1992). To achieve these goals, Contracting Parties are required to undertake joint assessments at regular intervals and publish them under the form of quality status reports (Figure 4). As a result, reports were produced in 2000 and 2010 with another one planned in 2023 (Figure 4).
Whilst two marine turtle species are listed under the OSPAR convention (loggerhead and leatherback turtles), no indicators have been proposed for their assessment. However, background documents evaluating the conservation status and identifying priorities for the protection of the leatherback and loggerhead turtles were published in 2009 (loggerhead status updated in 2015; Figure 4). The conservation status of these two species has been re-assessed in 2021. As the OSPAR regions considered in these evaluations significantly overlap with the MSFD north-east Atlantic Ocean region, assessment results could contribute to the 2024 MSFD assessment.
1.3.5 The IUCN Red List
The IUCN Red List has been one of the world’s most comprehensive tools to evaluate the risk of global species extinction since its creation in 1964. Listed taxa are regularly assessed, ensuring that their conservation status remains up to date based on the latest scientific knowledge available.
All marine turtle species are listed in the IUCN Red List, going from “Least Concern” in the case of the Mediterranean loggerhead sub-population to “Critically Endangered” as, for instance, the Kemp’s ridley (IUCN, 2020). The IUCN status assessment of marine turtle species has been coordinated by the Marine Turtle Specialist Group (MTSG), whose chairmen have been in charge of nominating experts to carry out the assessments. The MTSG has defined RMUs for marine turtles delineating geographical areas used by homogeneous population segments of the species based on demography, distribution, movement and genetic data (Wallace et al., 2010a). Because they constitute independent conservation units, these RMUs have been used as the basis for status assessments. Since 2017, the MTSG has also been compiling national reports, provided by countries under its coordination, into regional reports summarizing all available data. These reports cover the 10 MTSG reporting regions and associated RMUs, and aim to facilitate Red List assessments. Five quantitative criteria have been defined to assess the conservation status of listed taxa (IUCN Standards and Petitions Committee, 2019), two of which are equivalent to MSFD D1-Reptiles criteria (Table 1).
1.3.6 Other relevant policy frameworks
Because by-catch represents the main threat to marine turtles in European waters (Wallace et al., 2010b), fishery policies also contribute to marine turtle conservation. The rules ensuring the sustainable management of the European fishing fleet are set by the Common Fishery Policy. In particular, the Data Collection Framework (DCF) established in 2000 within the Common Fishery Policy (EU, 2000) lists data that must be collected by the European fishing fleet, which include information on by-catch. By-catch data and statistics collected in the frame of the DCF are then used by Member States, but also intergovernmental organizations (International Council for the Exploration of the Sea, General Fisheries Commission for the Mediterranean) and regional fisheries management organizations (International Commission for the Conservation of Atlantic Tunas) to improve by-catch monitoring, assessment and mitigation.
2 Methodology
2.1 Creation of the expert group
To develop indicators and assessment approaches to evaluate the status of marine turtle populations as part of the MSFD, a two-year study, based on test analyses and an expert consultation, was launched in 2019 at the initiative of the French Ministry of Environment and French National Museum of Natural History. Experts with extensive experience either in the field of marine turtle research and/or with the assessment processes under the different EU Directives (MSFD, Habitats Directive) and Regional Sea Conventions (OSPAR, Barcelona) were invited to join the group. All invited experts were based in countries bordering MSFD subregions where marine turtles occur (Figure 2). In total, 29 experts from seven EU and non-member countries (EU: France, Spain, Italy, Portugal and Greece; non-EU: United Kingdom and Tunisia) have contributed to this effort.
2.2 Development of indicators and assessment approaches
In order to explore and identify appropriate indicators and assessment approaches, the expert group focused on answering the following questions for each MSFD D1 (Biodiversity) criterion: (1) are there any relevant indicators already used in other environmental policies; (2) are there any other potential indicators and can they be realistically measured; (3) what are the data, assessment and monitoring strategies required.
To cover exiting methodological gaps on the assessment of the different criteria, and further explore the potential use of new approaches, experts were first invited to share datasets to test different analytical methods. A total of 17 datasets collected at the national and regional levels were shared (see Supplementary Table 1 for details on datasets). Data included sightings from aerial surveys in the Atlantic Ocean and Mediterranean Sea, effort and sightings from dedicated monitoring programs using ferries as observation platform in the Mediterranean Sea, demographic data and observations recorded by the Mediterranean and Atlantic French stranding networks and satellite tracking data from the Mediterranean Sea.
Multiple analytical methods were then tested using these datasets (Supplementary Table 1), and results were presented during a first workshop organized in November 2019. Following presentations, potential indicators for the assessment of the different MSFD D1 criteria were discussed. Based on initial recommendations resulting from this workshop, additional analytical methods were tested in 2020. The applicability, repeatability and efficiency of these assessment methods, were then further evaluated by the expert group during two virtual workshops in September and October 2020.
All recommendations proposed during the different workshops were summarized in a report at the end of the two-year study and sent to all experts for validation.
Recommendations for which a consensus was reached are presented here.
3 Results and discussion
3.1 Selection of marine turtle species and relevant criteria for MSFD D1 assessment
Based on the frequency of occurrence of the different marine turtle species in European seas (cf. section 1.2), MSFD assessment should focus on different species depending on the region considered: loggerhead and green turtles in the Mediterranean Sea region, and leatherback, Kemp’s ridley, green and loggerhead turtles in the north-east Atlantic region.
In the Mediterranean Sea, loggerhead sub-populations should be individually assessed when possible. While other marine turtle species are present in the Mediterranean Sea and north-east Atlantic Ocean, they occur in significantly lower numbers than the above-mentioned species. Nevertheless, additional species may be considered for GES assessment in the future if they become more frequent (e.g. new reports of olive ridley turtles in the Mediterranean Sea and Atlantic Ocean).
Unlike the Mediterranean Sea, the margins of RMUs defined for Atlantic species (i.e loggerhead, leatherback, green and Kemp’s ridley turtles) only partially overlap with Atlantic MSFD subregions (Figure 3; Wallace et al., 2010a). Considering that RMUs are currently regarded as the best available units for evaluating the conservation status of marine turtle species, the north-east Atlantic region as defined in the MSFD does not in itself represent a biologically meaningful assessment unit for marine turtles. Therefore, the assessment approach to be employed for the north-east Atlantic Ocean should differ from that of the Mediterranean Sea. As criterion D1C1 (mortality rates from by-catch) is the only one that can be directly managed by EU Member States in this region (e.g. through the reduction of incidental captures), it should be the only criterion considered in the evaluation of marine turtle species in the north-east Atlantic region. Nevertheless, the abundance and distribution at sea of these species, along with the extent of foraging habitats in the north-east Atlantic should still be quantified to inform management strategies. In particular, abundance estimates at the RMU scale will be required to set threshold values for the assessment of D1C1. Moreover, in some cases, it may be relevant to assess additional criteria at the subregional level (Saavedra et al., 2018). For instance, D1C2 (population abundance) has been previously proposed for the assessment of loggerhead turtles in the Macaronesia subregion due to the importance of the area in their life cycle and the strong correlation between relative abundance at sea and nest counts in the main Atlantic rookeries (Saavedra et al., 2018; Vandeperre et al., 2019).
Specific recommendations for the assessment of the different D1 criteria are presented in the following sections.
3.2 Proposed indicators and assessment approaches
3.2.1 Mortality rate from by-catch (D1C1)
3.2.1.1 Proposed indicator definition
According to the GES Decision (European Commission, 2017), GES for D1C1 is achieved when “The mortality rate per species from incidental by-catch is below levels that threaten the species, such that its long-term viability is ensured”. An effective way to assess this criterion is thus to quantify the proportion of the population estimated to have died due to incidental by-catch. This indicator should be calculated for all assessed species in the Mediterranean and north-east Atlantic regions. When possible, the assessment should focus on life stages most vulnerable to by-catch (e.g. juvenile, subadult and adult loggerhead turtles larger than 20 cm in the case of the Mediterranean sub-population; Casale, 2011).
3.2.1.2 Indicator measurement and data requirements
Several parameters must be estimated to calculate the mortality rate from by-catch. A key parameter is the total by-catch per métier (group of fishing operations targeting a specific assemblage of species, using specific gear, during a precise period of the year and/or within a specific area; Deporte et al., 2012) per year, which includes a measure of fishing effort (Equation 1). Although less precise than a métier-based approach (Cambiè et al., 2020), by-catch rates per fishing gear per year can be used when data on métier are not available.
The fishing effort per métier/fishing gear can be obtained at the national (national fishery observer programs) and European (DCF) levels. Although several units exist to express fishing effort, the number of days at sea or number of fishing trips per fishing gear and per year are the most commonly reported by countries.
The by-catch rate represents the number of individuals caught per observed day and, as with fishing effort, can generally be obtained at the national and European levels. Although all fishing vessels are required to report by-catch to comply with the DCF, by-catch may be under-reported. Therefore, by-catch rates are generally more accurately estimated from data collected by observers onboard fishing vessels. However, observer programs generally only include vessels large enough to host fishery observers, impeding by-catch rate estimates for small vessels (smaller than 7 m) using this method. This is particularly problematic in the Mediterranean Sea where the fishing fleet mostly comprises small artisanal vessels (Sacchi, 2008). To overcome this issue, the Food and Agriculture Organization (FAO) of the United Nations has developed standardized questionnaires to be used in surveys at landing sites (FAO, 2019). Therefore, combining data collected through observer programs and questionnaire surveys, using the FAO standardized protocol, is likely the best solution to estimate by-catch rates. Nevertheless, other methods, for instance, relying on self-declarations by fishing crews using mobile applications, or other means, may also be used in complement to refine by-catch estimates.
Criterion D1C1, as defined in the GES Decision, refers to the mortality rate from by-catch, thus implying that only by-caught individuals reported as “dead” should be included in the by-catch rates calculation. Although it is likely the most easily implementable, this method does not account for delayed mortality of individuals released after being caught alive. Indeed, several studies have reported relatively high post-release mortality after by-catch for various types of fishing gear (Álvarez De Quevedo et al., 2013; Swimmer et al., 2014; Parga et al., 2020). Therefore, mortality rate calculations should not only include by-caught individuals reported dead, but also the number of released, live, individuals corrected using the best available estimate of post-release mortality.
Another key parameter to calculate the mortality rate from by-catch is population abundance, which is required to evaluate the proportion of the population estimated to have died from incidental capture. In the case of the Mediterranean region, population abundance of loggerhead (global abundance, including all sub-populations) and green turtles should be estimated as part of the assessment of D1C2 (section 3.2.2.). In the north-east Atlantic region, population estimates calculated for the different RMUs, and published in the literature, should be used (e.g. North-West Atlantic leatherback sub-population; The Northwest Atlantic Leatherback Working Group, 2019).
Finally, the assessment of D1C1 requires the definition of a threshold value to determine whether GES has been achieved. One of the most commonly used approaches to set thresholds for by-catch is the potential biological removal (PBR) approach. PBR represents the mortality limit beyond which further mortality would lead to depletion in the population (Wade, 1998). This approach was initially developed for marine mammals and heavily relies on demographic parameters. More recently, it has been applied to estimate by-catch mortality limits for Mediterranean loggerhead turtles (Casale and Heppell, 2016). PBR estimated in this study thus constitute a good starting point for the next MSFD assessment. As refined demographic models become available, these estimates could then be updated accordingly.
Overall, parameters used for the calculation of by-catch rates and in demographic models (estimation of population abundance and PBR) should be determined separately for juveniles and adults. Moreover, demographic parameters vary between sub-populations (Wallace et al., 2010a), and thus, the appropriate demographic models should be used.
3.2.1.3 Proposed assessment approach
The proposed assessment approach for criterion D1C1, based both on the trend in annual mortality rates from by-catch and a threshold value (PBR), is detailed in Table 2.

Table 2 Proposed definitions of the different environmental status categories (good, good based on low risk and bad) for MSFD D1C1 criterion.
3.2.2 Population abundance (D1C2)
3.2.2.1 Proposed indicator definition
The definition of GES for D1C2 states that “The population abundance of the species is not adversely affected due to anthropogenic pressures, such that its long-term viability is ensured” (European Commission, 2017). Marine turtles occupy two very distinct environments during their lifetime: the marine environment where they spend most of their lives and the terrestrial environment where they are born and where females come back to lay their eggs. Accordingly, criterion D1C2 should be assessed based on two parameters: the temporal trends in population abundance at sea and at nesting sites.
3.2.2.2 Indicator measurement and data requirements
Trends in marine turtle population abundance at sea or at nesting sites can be characterized using different methods. Historically, population abundances have been estimated from counts of nesting females or clutches on land. Because monitoring animals on land is cheaper and logistically easier than in the ocean, long time series of nesting activity data have been collected through beach monitoring programs worldwide (Stokes et al., 2014; Mazaris et al., 2017). For instance, in the Mediterranean Sea, close to 40 years of nesting activity data (number of clutches) are available for loggerhead nesting sites in Greece (Casale et al., 2020b). As a result, these long time series have been used to approximate trends in marine turtle population abundance and evaluate their conservation status (Mazaris et al., 2017). However, abundance estimates resulting from beach monitoring are typically based on clutches, used to estimate the number of turtles from demographic parameters (clutch frequency and remigration interval), and bear uncertainty that may undermine trend analysis (Matsinos et al., 2008; Esteban et al., 2017; Ceriani et al., 2019). Moreover, they are only based on a small fraction of the population (adult nesting females) and studies have suggested that at-sea monitoring should be included for more accuracy (Bjorndal et al., 2010).
Population abundance at sea can be estimated using distance sampling methods based on aerial and shipboard survey data, or a combination of the two. In these methods, the number of animals recorded along pre-defined transect lines is used to estimate total surface abundances and densities within the whole survey area (Thomas et al., 2010). Aerial surveys have been one of the most powerful and commonly used tools to estimate fauna abundance at sea (from jellyfish to marine mammals; cf. Lauriano et al., 2017) and have been suggested as one of the most robust approaches to gather information on marine turtle abundance and density (Casale and Heppell, 2016; Warden et al., 2017; Casale et al., 2018), as they allow estimations over large areas (Lauriano et al., 2011; Seminoff et al., 2014) and provide robust estimates (Panigada, 2021). In the Mediterranean Sea, a few countries (i.e. France, Italy, Spain, Croatia) organize surveys on a regular basis since 2009 (e.g. Lauriano et al., 2011; Laran et al., 2021). Additionally, the first large-scale collaborative survey, which covered most of the Mediterranean, was organized by the ACCOBAMS (Agreement for the Conservation of Cetaceans of the Black Sea, Mediterranean Sea, and Contiguous Atlantic Area) Survey Initiative in summer 2018 (Panigada, 2021). The analysis of these surveys have recently enabled the estimation of marine turtle densities over the entire Mediterranean Sea (Sparks and DiMatteo, 2020; Panigada, 2021). Finally, additional aerial surveys implementing the distance sampling methods are being organized by several countries to meet MSFD reporting requirements.
Shipboard surveys using standardized protocols can also be used effectively to estimate abundance at sea (Saavedra et al., 2018; Doreímus, 2021). In particular, opportunistic platforms are cost effective and can be used to collect seasonal data on marine turtle distribution and abundance at sea (Arcangeli et al., 2019; Vandeperre et al., 2019). While covering a limited area over fixed routes, data collected from ferries can provide valuable information on temporal trends in abundance at sea (Tepsich et al., 2020), especially if used in combination with aerial surveys. Moreover, based on the approach used for monitoring floating marine macro litter (Arcangeli et al., 2020), methods have been developed to sort individuals observed from ferry and aerial surveys into size classes, giving information on life stages (ISPRA, in press). Finally, other methods relying on sighting data collected by fishery observers from dedicated transects can allow for high observation effort over large areas. While constrained by the dynamic of the fishing activity, this method has been shown to represent a reliable alternative for estimating trends in abundance using model-based approaches (Vandeperre et al., 2019).
Lastly, population abundances at sea and nesting sites can also be quantified using mathematical models. A variety of models, including deterministic or stochastic structured (age- or stage- based) population models (Caswell, 2001) and individual-based models, (DeAngelis and Mooij, 2005) have been developed and successfully used to estimate population abundance and inform management decisions (Crouse et al., 1987; Chaloupka, 2002; Mazaris et al., 2005; Casale and Heppell, 2016). Characterizing temporal trends in abundance using demographic models has several advantages compared to using statistical methods relying entirely on observation data. First, these models can produce abundance estimates for each individual life stage of any given sub-population, accounting for differences in demographic parameters between RMUs. Moreover, the effects of anthropogenic pressures, such as by-catch, can be included into the models, allowing the calculation of mortality limits (section 3.2.1.2). Finally, demographic models can be used to make projections under different anthropogenic pressure or climate scenarios (Mazaris and Matsinos, 2006). However, a lot of data are required to estimate the demographic parameters feeding these models. Therefore, approaches relying on field observations and population modelling methods are highly complementary.
3.2.2.3 Proposed assessment approach
As detailed in section 3.2.2.1, the assessment of criterion D1C2 should be based on trends in population abundances both at sea and nesting sites, estimated considering different analytical methods (observation-based and modelling approaches; Table 3). Due to high interannual variability in abundance estimates (Hays, 2000; Solow et al., 2002), it has been suggested that long time series of at least 10 years of data are optimal to detect trends in population abundance (Mazaris et al., 2017; Piacenza et al., 2019). For this reason, the longest time series available should be used for the assessment (Table 3). While such long time series are available for nesting sites, observation effort at sea has usually been less constant, limiting the length and number of time series that can be used for assessment. Therefore, until longer time series become available, trends in abundance at sea should be characterized over the 6-year MSFD reporting period (Table 3).

Table 3 Proposed definitions of the different environmental status categories (good, good based on low risk and bad) for MSFD D1C2 criterion.
3.2.3 Population demographic characteristics (D1C3)
Criterion D1C3 has been defined as a secondary criterion, meaning that it should only be included in the assessment process either to complement primary criteria or when, for a particular criterion, the marine environment is at risk of not achieving or maintaining GES (European Commission, 2017). In this case, the status of D1C3 will be considered as good when “The population demographic characteristics (e.g. body size or age class structure, sex ratio, fecundity, and survival rates) of the species are indicative of a healthy population that is not adversely affected due to anthropogenic pressures” (European Commission, 2017). Although considered as secondary in the GES Decision, D1C3 is in fact a key criterion. As mentioned in section 3.2.2.2, the proper estimation of demographic parameters such as fecundity or survival rates is essential to the development of demographic models, and thus central to the assessments of primary criteria D1C1 and D1C2 (sections 3.2.1 & 3.2.2).
While, further work is needed to effectively assess criterion D1C3, approaches relying on field data, genetic studies and modelling were discussed. Specifically, an approach based on the evaluation of the adult/juvenile ratio, estimated from size distribution data (“reaction norm of the size of sexual maturity” method; Girondot et al., 2021) was considered as particularly promising and is currently under development.
3.2.4 Population distributional range (D1C4)
3.2.4.1 Proposed indicator definition
Commission Decision (EU) 2017/848 indicates that good status for criterion D1C4 is achieved when “The species distributional range and, where relevant, pattern is in line with prevailing physiographic, geographic and climatic conditions” (European Commission, 2017). Based on this definition, the proposed assessment method relies on the proportion of change in the observed distributional range of the species between reporting cycles. Because marine turtles occupy different habitats throughout their life cycle (Musick and Limpus, 1997; Bowen and Karl, 2007), distributional range estimations should be life stage-specific. In addition, the distributional range varies seasonally (Lauriano et al., 2011; Luschi and Casale, 2014; Blasi and Mattei, 2017; Arcangeli et al., 2019), and thus, different seasons should be assessed separately.
3.2.4.2 Indicator measurement and data requirements
The observed distributional range of the species can be estimated using data collected through monitoring programs at sea and at nesting sites. In the case of nesting sites, beach monitoring provides the data required for the assessment of distributional range on land. At sea, aerial and shipboard (including opportunistic platforms such as ferries) distance sampling surveys (see section 3.2.2.2 for details) represent the best source of data for the estimation of the species distributional range. Additionally, observations recorded by stranding networks (Dimitriadis et al., 2022) and citizen science programs (Casale et al., 2020a) can complement aerial and shipboard surveys.
Multiple statistical modelling approaches, relying on various data sources and algorithms, can be employed to map the distributional range of the species. For instance, kernel density estimation or distance sampling combined with kriging methods (based on the number of turtles observed per km of effort per cell) can be used to calculate marine turtle densities and map the distribution of these densities (Box 1). Based on obtained distribution maps, the extent of the observed distributional range can be quantified and spatial shifts in this distribution identified (Box 1).
Box 1. Estimation of the extent of distribution and shift over time using geospatial statistics (kriging).
Quantifying and mapping marine megafauna densities can be challenging due to spatially inconsistent observation effort and the scarcity of sightings. The geostatistical method of Kriging, or spatial interpolation, allows the estimation of parameter values in areas where there is a lack of prospecting effort or even outside the sampled area (Baillargeon, 2005; Oliver and Webster, 2013). To map the observed distribution of marine turtles in the north-western Mediterranean Sea, observation data collected aboard ferries by the Fixed Line Transect Mediterranean Network (FLT MedNet) along 9-10 routes were used (Arcangeli et al., 2019). These data were divided into two time periods (2013-2015 and 2016-2018) of equivalent observation effort during the summer season (April to October). Number of sightings and effort per cell on a regular 50km x 50km grid were summed by period, and used to calculate linear encounter rates per effort (sighting/km). A specific Kriging system was then applied to assess counts variability under a Poisson distribution assumption and to interpolate encounter rates (Monestiez et al., 2006). To characterize spatial shifts between the two studied periods, a universal kriging approach was employed (Bellier et al., 2009, 2010). Although not presented here, encounter rates can be converted into densities (number of individuals per km2) and uncertainties calculated and mapped (David et al. submitted).
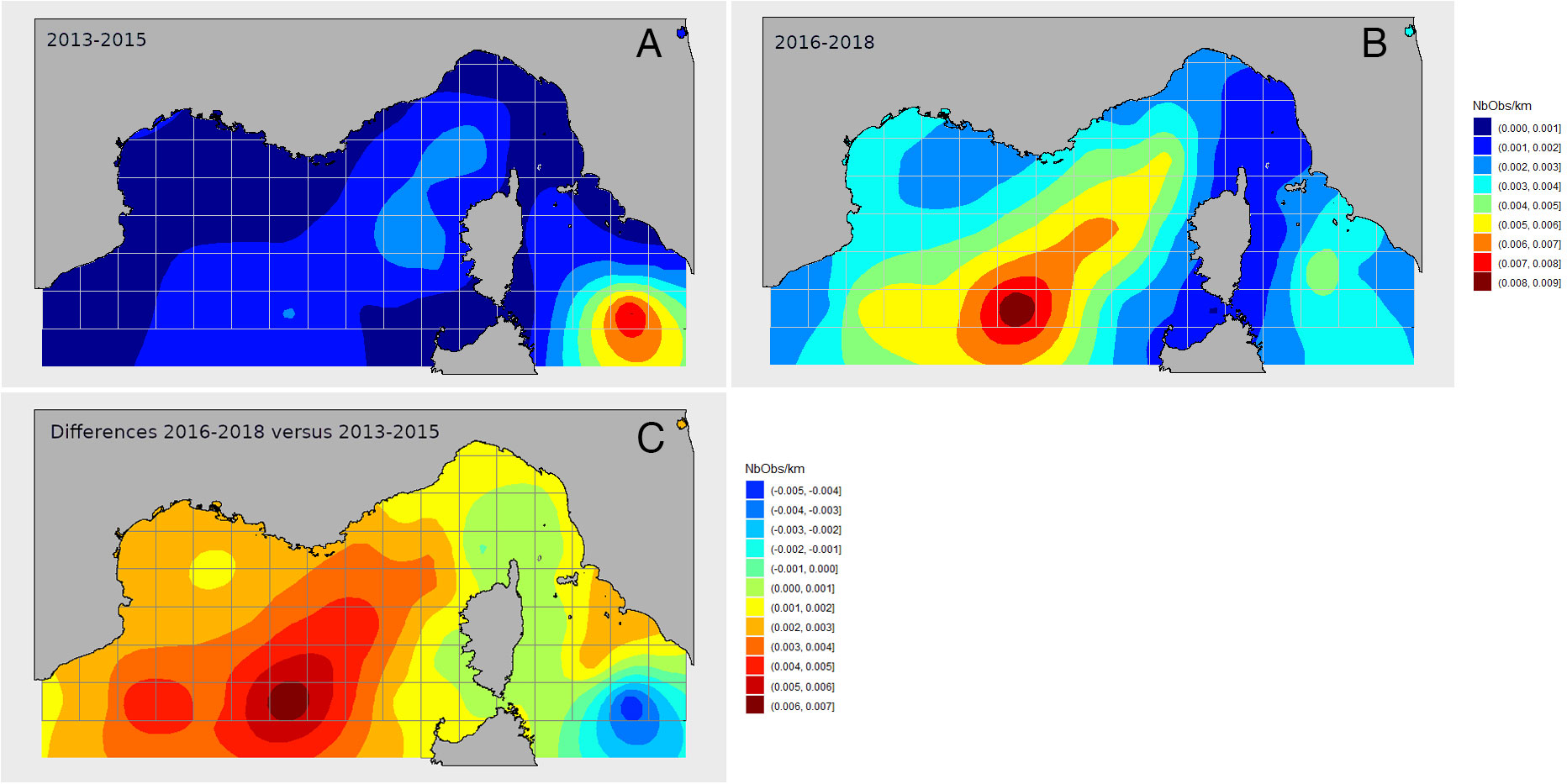
Box 1 Figure 1 (A, B) Distribution of the encounter rate of hard-shelled turtles estimated using kriging models under a Poisson distribution assumption. The color scale indicates the estimated number of sightings per km of effort in summer (April to October) during the (A) 2013-2015 and (B) 2016-2018 time periods. (C) Differences in the distribution of the summer encounter rate of hardshelled turtles between the two periods, 2013-2015 and 2016-2018. The color scale indicates differences in the number of sightings per km of effort. Based on data from the Fixed Line Transect Mediterranean Network. Data source: Arcangeli et al., 2019; Supplementary Table 1.
Results suggested that encounter rates in the north-western Mediterranean Sea were low (0.1 to 0.9 per 100 km; Figures 1A, B). Moreover, a significant spatial shift in distribution was observed between the two studied periods (Figure 1C). For both periods, areas with the highest encounter rates were located east of the Corsica and Sardinia islands (north of the Tyrrhenian Sea), and off the French continental coast, in the deep part of the basin.
3.2.4.3 Proposed assessment approach
Following the different workshops, experts agreed that available knowledge does not currently allow the quantitative assessment of D1C4. Marine turtles are highly mobile organisms and any change in their environment and prey distribution, either natural or human-caused, may significantly affect their distribution (Polovina et al., 2000; Polovina et al., 2001; Luschi et al., 2003; Hawkes et al., 2007; Revelles et al., 2007; Witt et al., 2007a; Witherington et al., 2011). In particular, climate change is expected to have a major impact on marine turtle distribution and the suitability of nesting habitats (Witt et al., 2010; Pike, 2013; Almpanidou et al., 2016; Monsinjon et al., 2019; Patrício et al., 2021). Consequently, variations in the observed distributional range are particularly difficult to interpret. To account for this difficulty, an option is to consider that GES is achieved when the distributional range remains stable between reporting cycles. Nevertheless, a better understanding of environmental and anthropogenic drivers of marine turtle distribution will be necessary to finalize assessment approaches.
3.2.5 Extent of suitable habitat (D1C5)
3.2.5.1 Proposed indicator definition
The status of criterion D1C5 has been defined as good when “The habitat for the species has the necessary extent and condition to support the different stages in the life history of the species” (European Commission, 2017). This definition explicitly indicates that, as with D1C4, life stages should be individually assessed. Furthermore, because marine turtles may occupy different habitats in different seasons (section 3.2.4.1), a seasonal assessment is also required for criterion D1C5. To best fit the definition of GES for D1C5, assessing changes in the extent of suitable habitats for the species between reporting cycles has been proposed.
3.2.5.2 Indicator measurement and data requirements
While terrestrial habitats are only used for nesting, distinct habitats for marine turtles have been identified at sea. In the Mediterranean Sea, foraging, developmental and wintering habitats used by loggerhead and green turtles have been delineated (Casale et al., 2018) and should be considered in the assessment of D1C5.
Several modelling approaches, relying on different types of data can be applied to estimate the extent of suitable habitats. At sea, suitable habitats can be effectively mapped using ensemble of ecological niche models parameterized with species presence records derived from field observations or satellite tagging data, which have the advantage of providing precise geographic location and information on the size/life stage and possibly sex of the tracked individuals (Box 2). Moreover, tracking data include information on individual behaviours, which can be accounted for in species distribution models (Chambault et al., 2021). Ecological modelling approaches based on locations derived from tracking studies and climatic datasets have already been used successfully to generate maps of suitable foraging grounds for adult and juvenile loggerheads in the Mediterranean (Almpanidou et al., 2021; Chatzimentor et al., 2021). Whereas ecological niche approaches based on a combination of different models have been shown to provide more robust results (Araújo and New, 2007), single-model approaches can also be applied for the assessment of D1C5.
Box 2. Ensemble niche models applied to determine the suitability and extent of important Caretta caretta foraging areas (Almpanidou et al., 2021; Chatzimentor et al., 2021)
A climatic niche modeling framework has been recently applied to spatially delineate key foraging habitats for adult (Almpanidou et al., 2021) and juvenile (Chatzimentor et al., 2021) loggerheads in the Mediterranean basin. Satellite tracking data for more than 150 individuals, derived from the literature, were georeferenced and digitalized. Focal points, indicative of foraging locations, were extracted and used as input locations for a number of algorithms (e.g. Generalized Additive Models, Random Forests) that were applied to explore sea-scape suitability. Predictors included a number of bioclimatic variables produced based on sea surface temperature data at a spatial resolution of less than 10km2. Bathymetric maps have also been used to delineate neritic and oceanic foraging grounds. In order to avoid model overfitting and counterbalance the uncertainty inherent to the choice of the algorithm applied, an ensemble modeling framework was used. A number of statistical test (e.g. AUC, TSS) were applied to assess performance of the models. Moreover, in the study of Almpanidou et al. (2021) novel field observations from different locations across the Mediterranean were used to validate the accuracy of spatial predictions.
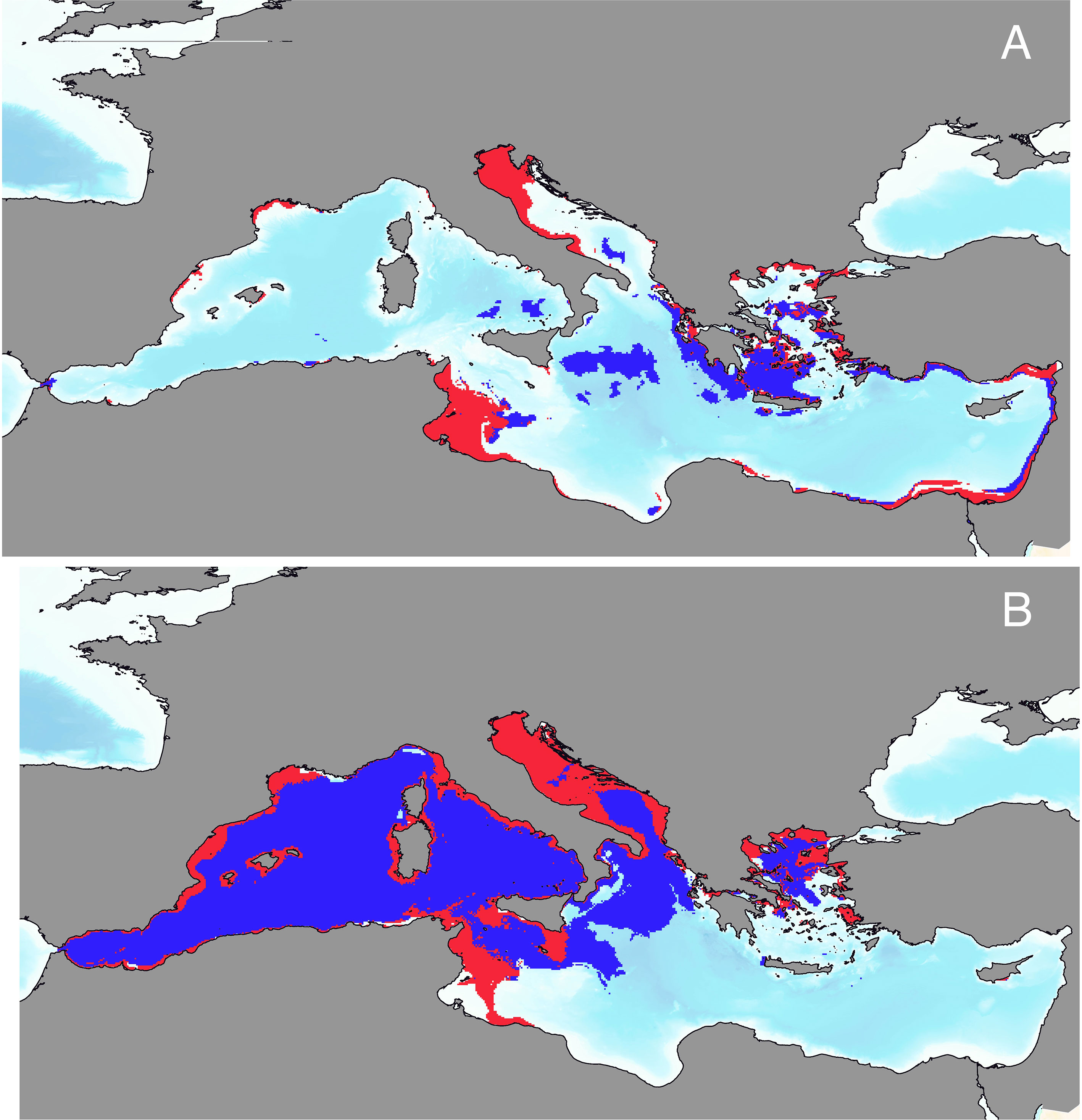
Box 2 Figure 1 Potential habitat distribution of (A) adult and (B) juvenile loggerhead sea turtles as projected by final ensemble models. Distributions have been binarized based on the threshold of maximization of the total sum of squares (TSS). Neritic areas of potential presence are represented in red, while oceanic areas of potential presence are in blue. Data source: Almpanidou, et al. 2021; Chatzimentor et al., 2021.
The analyses revealed that potential foraging areas for adult loggerheads were mainly distributed in the eastern and central parts of the Mediterranean, with most of them located within the 200m isobath. Projected suitable habitats for adults covered about 15% of the total surface of the basin. Conversely, areas identified as potential suitable foraging grounds for juveniles covered an extensive part of the western basin comprising both neritic and oceanic sites.
Other modelling approaches, based on observation data collected from aerial and shipboard surveys could also represent effective tools to estimate the extent of suitable habitat at sea (Box 3). As detailed in section 3.2.2, when pooled together, aerial (Box 3A) and shipboard surveys (Box 3B) cover large spatial and temporal scales, and can be used to parameterize large scale habitat suitability models (Virgili et al., 2019). Moreover, habitat selection can vary for overwintering and reproductive purposes. Therefore, seasonal ecological niches should also be investigated using multi-variable modelling approaches able to subset the selected environment by the species (Box 3B).
Box 3. Estimation of the extent of suitable habitat using observation data collected from aerial surveys and ferry routes.
Box A. Predicting habitat preferences from aerial surveys using Density Surface Modelling
Density Surface Modelling (DSM; Hedley and Buckland, 2004; Miller et al., 2013) consists in smoothing abundance or density spatially using the output of Distance Sampling methods (Buckland et al., 2001; Thomas et al., 2010) and environmental covariates (e.g., depth, distance to the coast, sea temperature, salinity). Such a spatial model (fitted using Generalized Additive Models) allows for mapping abundance or density while correcting for detection uncertainty. Here observations of hard-shelled turtles in the Western Mediterranean from line transect distance sampling aerial surveys conducted in 2011-2012 (Marine Mammals Aerial Survey: SAMM), 2018 (ACCOBAMS Survey Initiative: ASI), and 2019 (SAMM) were used. Transects were split in 5-km segments for which the number of individuals was quantified. Using animal-observer perpendicular distances from SAMM, a probability of detection function was fitted, and the average probability of detection (p = 0.63) and the Effective Strip Width (ESW = 128.6 m after the 5% highest distances were discarded as per Buckland et al. 2001) were used as offset terms in DSMs. As exact animal-observer distances were not available from ASI, the detection probability was assumed to be similar to SAMM (aircrafts and procedures were similar). Based on the ecology of marine turtles, 10 candidate environmental covariates were selected: (1) Average Sea Surface Temperature (SST) and (2) its standard deviation, (3) average Net Primary Production (NPP), (4) average Sea Level Anomaly (SLA), (5) average Eddy Kinetic Energy (EKE), (6) average Sea Surface Salinity (SSS), (7) average depth, (8) average slope, (9) closest distance to coast and (10) to 200-m isobath (see Supplementary Table 2 for details on data source and original spatiotemporal resolution). To identify the most important environmental variables, DSMs were fitted with a negative binomial distribution for every possible combination of parameters, and models with the highest deviance explained (>99th percentile) were considered as equivalent candidates. Among those, the model with the fewest parameters was chosen. Longitude and latitude were then added to the selected model as additional covariates to account for unexplained spatial patterns. The analysis was conducted using the ‘dsm’ R package (Miller et al., 2013).
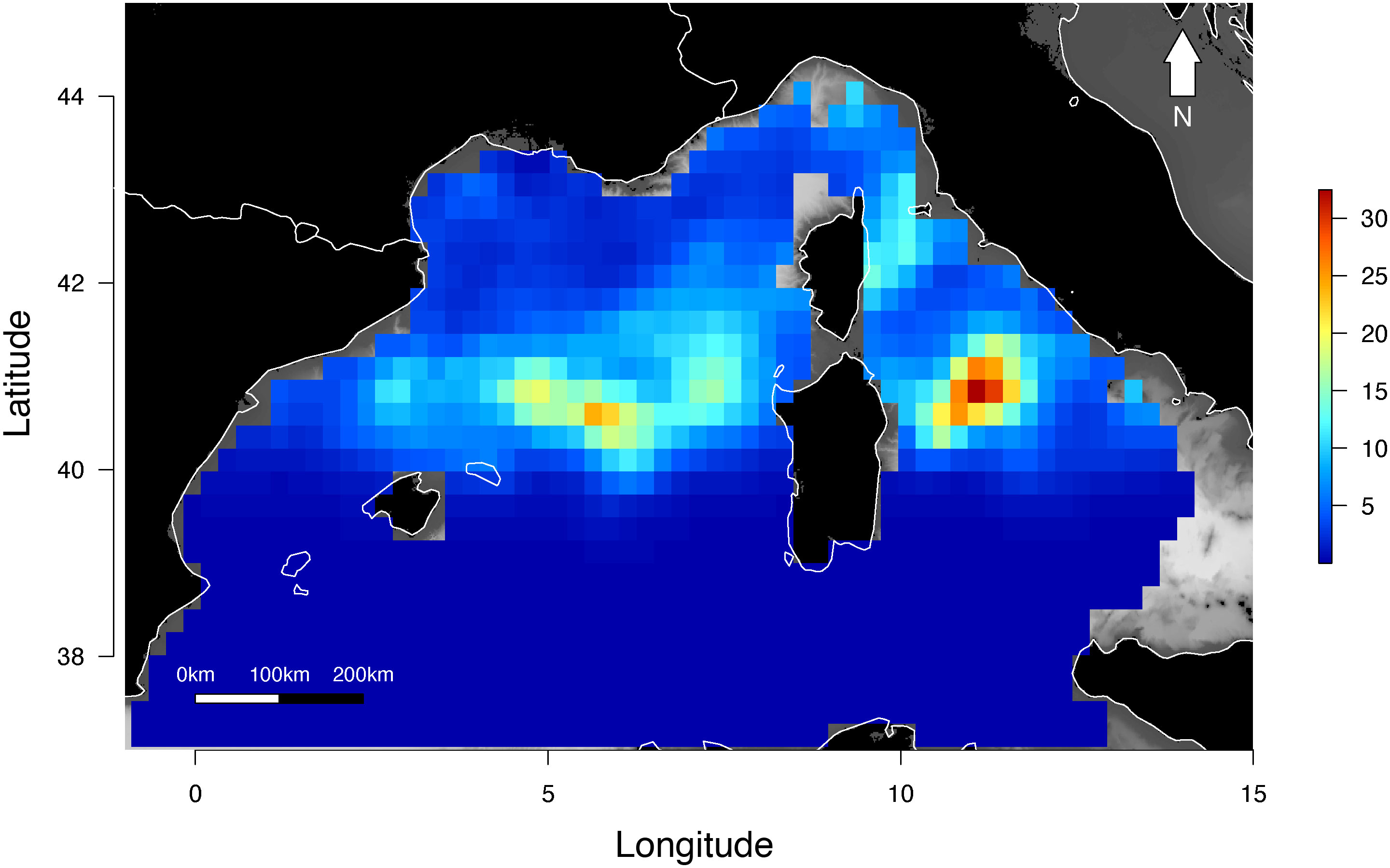
Box 3 Figure 1 Extent of preferred habitat estimated using Density Surface Modelling. The color scale indicates the estimated number of individuals in spring/summer averaged over 2012-2018. Data source: Panigada, 2021; Laran et al., 2021; Supplementary Table 1.
Results suggest that the following variables are important components of the habitat of hard-shelled sea turtles in the Western Mediterranean: average SST and its standard deviation, average SLA, average NPP, average SSS, depth and closest distance to coast (deviance explained = 38.8%). Longitude and latitude slightly improved model fit (deviance explained = 42.6%). Based on this model, abundances were predicted monthly (over 2012-2018) on a 0.25°×0.25° grid. Over spring and summer, turtles mostly aggregated in the Tyrrhenian sea (between mainland Italy and Sardinia) and in the Balearic sea, and were nearly absent below 40°N. Predictions for autumn and winter were not presented because of important data gaps.
Box 3B. Seasonal ecological niches of loggerhead turtles in the Adriatic and Ionian Seas characterized from ferry data (Zampollo et al., 2022)
The ecological niches of loggerheads were recently investigated for turtles with body size > 20 cm during the winter (October – March, W) and breeding-nesting (April – September, BN) periods in order to i) characterize spatial use within the central-southern Adriatic (AS) and north-western Ionian Sea (IS) during both seasons, ii) identify environmental predictors characterizing the habitat selection, and iii) predict the potential suitability of these seas (Zampollo et al., 2022). Data were systematically collected from December 2014 to February 2018, along fixed line transects using ferries as a platform of observation. Nine environmental predictors were selected: bathymetry, slope, distance from shore, mean sea surface temperature (SST), SST min, SST max, SST yearly excursion range, April SST, Particulate Organic Carbon (POC) and Chl-a. Maxent models (Phillips and Dudík, 2008) were used to extrapolate the suitable habitat from presence-only data and predict the suitability over unmonitored regions. The level of distribution overlap between W and BN predicted niche was calculated using the Schoener’s D index (Schoener, 1968).
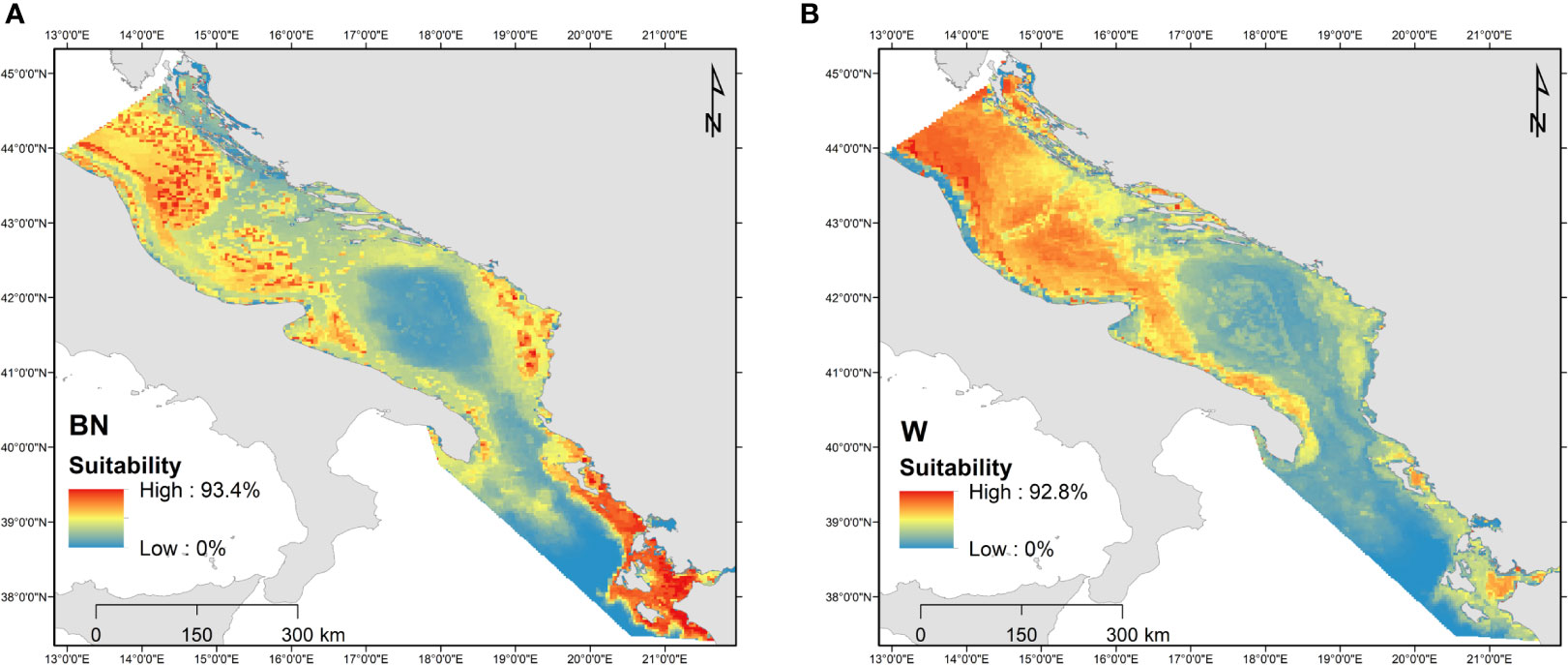
Box 3 Figure 2 Habitat suitability maps obtained from Maxent during (A) the breeding-nesting (BN) and (B) winter (W) periods. Data source: Zampollo et al., 2022; Supplementary Table 1.
In the central-southern Adriatic Sea, the predicted distribution of loggerhead turtles during W or NB periods did not largely change. In the north-western Ionian Sea, on the other hand, niches differed between the W and BN periods. Loggerheads selected their habitats based on different environmental variables depending on the Sea and season considered. In particular, SST affected habitat selection differently: extreme cold temperatures defined the spatial use within the AS during W and BN, while extreme warm temperatures contributed to identify suitable areas during BN in the IS. This study confirmed previous descriptions of the Adriatic Sea as an important foraging ground, and highlight the importance of considering seasonality when estimating habitat suitability.
Finally, in terrestrial habitats, modeling approaches that include factors affecting the suitability of nesting beaches (e.g. humidity, vegetation, erosion/accretion processes, topography, temperature etc.; Wood and Bjorndal, 2000; Fuentes et al., 2010; Kelly et al., 2017; Patrício et al., 2021), should be considered. For instance, models relying on temperature data can be applied to assess the suitability of a nesting beach at fine spatiotemporal resolution through the prediction of incubation duration, sex ratio, and hatching success (Hays et al., 2017; Monsinjon et al., 2019; Laloë et al., 2020). Over large spatiotemporal scales, nest temperatures can be predicted using microclimate models (Fuentes and Porter, 2013; Bentley et al., 2020) or data-driven correlative approaches via air temperature and sea surface temperature (Girondot and Kaska, 2015; Monsinjon et al., 2017; Monsinjon et al., 2019; Turkozan et al., 2021).
Overall, the effectiveness of the proposed modelling approaches depends on the careful selection of environmental predictors. A large array of potential predictors has been identified (e.g. bioclimatic variables, net primary production, sea surface salinity, depth etc.) and should be tested to determine which combinations of predictors are the most effective in predicting habitat suitability. Moreover, assessing GES is a rather complex, multidimensional process that requires quantitative inputs for multiple parameters. Acknowledging that data availability is often limited, multi-species modelling and marine ecosystem models could support assessment approaches if well parameterized and properly described. In addition to observation and environmental data used to parameterize the models, data collected through independent sources (e.g. observations recorded by stranding networks and citizen science programs) must ideally be used to validate model outputs (Pinto et al., 2016).
3.2.5.3 Proposed assessment approach
The proposed approach to determine GES for criterion D1C5 relies entirely on the trend in the extent of suitable habitats (foraging, developmental, wintering and nesting habitats) detected between reporting cycles (Table 4).

Table 4 Proposed definitions of the different environmental status categories (good, good based on low risk and bad) for MSFD D1C5 criterion.
3.2.6 Integration methods
Integration methods for MSFD assessments have been developed and proposed at the EU level for fish and seabird GES criteria and indicators (Dierschke et al., 2021). Unlike other components of Descriptor 1, D1-Reptiles is composed of a single species group. Therefore, integration is only necessary at the species, criteria, and when relevant, sub-population, life stage and seasonal levels. Considering the small number of species, sub-populations and life stages considered, the use of the One Out All Out approach for integration at all levels is recommended. That means, for instance, that the status of all assessed species should be good for the entire species group to achieve GES.
4 Conclusions and future directions
The international cooperative effort initiated in 2019 resulted in a set of specific recommendations for the assessment of the different MSFD D1-Reptiles criteria. Quantitative indicators, associated data requirements and assessment strategies were proposed for all four D1 primary criteria. While the assessment of certain criteria such as D1C1, D1C2 and D1C5 may be feasible in the short term (some data already available, established monitoring program and/or validated assessment methods), key data and knowledge gaps remain to be addressed to refine assessment strategies and allow for the quantitative assessment of all criteria (including D1C4 and secondary criterion D1C3) and species in the Mediterranean and north-east Atlantic regions.
4.1 Addressing data and knowledge gaps
The recommendations presented in section 3, which are based on expert opinion and on state-of-the-art scientific knowledge, provide the ideal assessment framework by accounting for the different aspects of the complex life history of marine turtle species. However, the proposed assessment methods rely on modelling approaches that require large and diverse datasets. As a result, at this stage, quantitative assessments may not be feasible for all species as some are rarer and less studied (e.g. Kemp’s ridley in the north-east Atlantic Ocean) than others (e.g. loggerheads in the Mediterranean Sea) or do not have established monitoring programs. Moreover, existing geographical and temporal biases in sampling effort are likely to affect assessments at regional scales. For instance, in the Mediterranean Sea, more data are available for neritic and oceanic environments in the western compared to eastern basin, and thus, not all countries will be able to provide the same level of assessment. Similarly, more data are generally collected in the summer than in the winter, complicating the interpretation of temporal trends. Consequently, increasing sampling efforts for data-poor species, seasons and geographical areas should be considered a priority.
Additionally, certain existing datasets can be challenging to get access to. This is particularly true for data on fishing effort, necessary for the estimation of total bycatch as part of D1C1. For example, vessel monitoring system data, which track large fishing vessels, are often heavily protected due to privacy issues and difficult to access. Therefore, effort should be made at the national and international levels to facilitate the collection, access and dissemination of these types of data, which are required to carry out GES assessments for the MSFD and other environmental policies.
In summary, key data requirements identified in the present study include by-catch records (including actual mortality rates and effort), tracking data, observations at sea (aerial surveys, shipboard observation platforms) and at nesting sites, and demographic data. In particular, the estimation of demographic parameters has been set as a priority. Although criterion D1C3 on population demographic characteristics has been defined as secondary, it is in fact central to the assessment of D1-Reptiles. While no assessment approach has been proposed for D1C3, datasets required to estimate demographic parameters were identified. In addition to sampling methods mentioned in section 3, telemetry studies, mark-recapture methods including genetic fingerprinting and data collection by stranding networks and monitoring programs, should be specifically prioritized to estimate key demographic parameters (i.e. survival rate of different life stages, remigration intervals, number of clutches per year, sex ratio etc.).
Overall, several of the data collection frameworks and assessment approaches described in this study are the same as those already implemented for the assessment of marine mammal species under the MSFD (Palialexis et al., 2019). Specifically, aerial and shipboard surveys have been used to assess the distribution (D1C4) and abundance (D1C2) of cetacean populations (based on OSPAR common indicator M4b; OSPAR Commission, 2019). Similarly, data on by-catch and fishing effort collected by fishery observer programs have been used for the evaluation of harbor porpoise by-catch (based on OSPAR common indicator M6; e.g. intermediate assessment OSPAR, 2018). The fact that both data on marine turtle and mammals (as well as other megafauna) are collected by fishery observer programs and during aerial surveys will greatly facilitate MSFD assessment for marine turtles.
Moreover, by identifying data requirement for MSFD assessment, this study sets targets for future data collection, informing monitoring programs implemented by member states. The MSFD does provide the framework for EU Member States to update their monitoring programs to cover any data gap, and encourages joint regional monitoring initiatives (Palialexis et al., 2021). Therefore, even if some of the proposed approaches may not be feasible for the next MSFD assessment in 2024, it is likely that they will be in the future.
In addition to filling data gaps, facilitating data sharing will be key for future GES assessments. This can be done through collaborative initiatives, such as the one presented here, which can provide a formal framework for data sharing. However, making data publicly accessible using online data repositories and/or platforms such as the Ocean Biodiversity Information System (sea turtle data: https://seamap.env.duke.edu/species/948946) remains the best way to support sea turtle conservation and should be prioritized when possible.
Meanwhile, EU Member States can use alternative methods, based on available observations (at sea and, when relevant, at nesting sites) and demographic data. For example, in the absence of effort data, the distributional range of the species (D1C4) could be assessed based on presence data, instead of estimated densities. Similarly, alternative methods, adapted to data-poor environments and species, could be used to set thresholds. Specifically, methods that are less data hungry than the PBR approach have been developed to estimate by-catch mortality limits, and could be used to set thresholds for D1C1 (Punt et al., 2020). Moreover, mortality limits could be set based on existing demographic models. For instance, population models developed for loggerhead turtles suggest that annual mortality rates of juveniles larger than 40 cm CCL (curved carapace length) and adults should be less than 0.2 for the population to maintain itself over the long term (Crouse et al., 1987; Heppell et al., 2003; Casale and Heppell, 2016). Finally, in the case of threatened and/or declining sub-populations, such as leatherback turtles in the Atlantic Ocean, conservative threshold values (e.g. 0.1% of the lowest population estimate; ICES, 2020) could be also used until PBRs can be estimated. These methods, that do not necessarily rely on modelling, would be particularly useful to countries with limited funds and research infrastructures.
4.2 Optimizing harmonization between environmental policies
In Europe, marine turtles are covered by different policies, all of which having their own assessment frameworks, indicators and operational objectives (section 1.3). As a result, assessments under these policies are generally based on different reporting periods and carried out by different experts. To tackle inconsistencies between assessment approaches and facilitate harmonization between environmental policies, significant efforts have been made to better synchronize reporting cycles and areas, and to develop common indicators or optimize their reuse for other policies. In the case of marine turtles, although the number of criteria/indicators differ between policies (no indicators proposed for OSPAR), existing indicators are relatively equivalent (Palialexis et al., 2019). Moreover, assessment areas considered in these policies overlap relatively well, with Regional Sea Conventions generally covering most of MSFD and HD assessment areas. Therefore, the basis for a good harmonization between assessment approaches already exists.
To ensure harmonization, having a common expert group contributing to the different European Directives and Regional Sea Conventions, as well as to commissions such as the Species Survival Commission of the IUCN (which includes the MTSG), is particularly important. Overall, the value of having an international expert group focusing on marine turtle conservation, and involved in the evaluation process under the different relevant environmental policies, goes beyond the design of standardized assessment and monitoring methods. Due to the high mobility of marine turtles, international collaborations are the only way toward the development of comprehensive and effective management strategies.
4.3 Next step
The present study contributed to improving the assessment process for marine turtle species under MSFD Descriptor 1. Now that data requirements have been identified, a similar approach as the one presented here could be employed to develop standardized monitoring programs for the MSFD and other environmental policies. On a broader scale, recommendations regarding priorities for data collection, assessment and monitoring should align with ongoing global initiatives. In particular, proposed methods must be in line with effort carried out by the MTSG including the IUCN status assessments of marine turtle species, the update of RMUs and the future designation of Important Marine Turtle Areas.
Overall, the framework applied in the present study (i.e. expert gathering, consultation, common modeling efforts etc.) could be adopted for other species of conservation interest. While we acknowledge that indicators and thresholds should reflect biological, behavioral and population dynamics features of a target species, the approach presented here could offer a baseline for delineating conservation priorities and status assessments for other MSFD Descriptors.
In conclusion, this collaborative effort has provided a solid basis for advancing our knowledge of marine turtles and supporting their conservation. As the European Commission recently adopted the EU Biodiversity Strategy for 2030, aiming to protect 30% of European waters within the next decade, the ability to adequately assess the status of charismatic marine megafauna, such as marine turtles, will be key to determine whether conservation goals are met. Overall, the MSFD, and now the UN Decade of Ocean Science for Sustainable Development (2021-2030), provide frameworks facilitating international initiatives working at the science-policy interface, such as the one presented here. In the future, similar initiatives including decision-makers and managers in addition to scientists will likely be central to strengthening the management and protection of the oceans.
Data availability statement
The data analyzed in this study were obtained from various sources (cf. Supplementary Table 1). Requests to access these datasets should be directed to data owners listed in Supplementary Table 1.
Author contributions
FC designed the study. FC and AG initiated the creation of the sea turtle expert group. FG, FC and AG led the expert group and organized the workshops. All authors contributed to the development of recommendations. JM, AA, LD and AM prepared the case studies and FG wrote the first draft of the manuscript. All authors contributed to the article and approved the submitted version.
Funding
This study was funded by the French Ministry of Environment (MTES-MNHN Conventions n°2102636187 (2019) and n°2102994526 (2020)). DM acknowledges support from the European Union’s Horizon 2020 research and innovation programme under the Marie Skłodowska-Curie grant agreement n°794938. The work of ADM was supported by the Hellenic Foundation for Research and Innovation (H.F.R.I.) under the “First Call for H.F.R.I. Research Projects to support Faculty members and Researchers and the procurement of high-cost research equipment grant” (Project Number: 2340). FV was supported by the Investigator Programme of the Fundação para a Cîencia e Tecnologia (FCT, CEECIND/03469/2017, CEECIND/03426/2020).
Acknowledgments
We would like to thank all the experts for making this work possible. We are particularly grateful to experts who accepted to share datasets and to those who participated in the different workshops and provided valuable input regarding MSFD assessment strategies and indicators.
Conflict of interest
Authors AG and JM were employed by Envirology SARL.
The remaining authors declare that the research was conducted in the absence of any commercial or financial relationships that could be construed as a potential conflict of interest.
Publisher’s note
All claims expressed in this article are solely those of the authors and do not necessarily represent those of their affiliated organizations, or those of the publisher, the editors and the reviewers. Any product that may be evaluated in this article, or claim that may be made by its manufacturer, is not guaranteed or endorsed by the publisher.
Supplementary material
The Supplementary Material for this article can be found online at: https://www.frontiersin.org/articles/10.3389/fmars.2022.790733/full#supplementary-material
References
Almpanidou V., Schofield G., Kallimanis A. S., Türkozan O., Hays G. C., Mazaris A. D. (2016). Using climatic suitability thresholds to identify past, present and future population viability. Ecol. Indic. 71, 551–556. doi: 10.1016/j.ecolind.2016.07.038
Almpanidou V., Tsapalou V., Cardona L., Claro F., Hostetter P., Kaska Y., et al. (2021). Foraging grounds of loggerhead sea turtles across the Mediterranean Sea: key sites and hotspots of risk. Biodivers. Conserv. 31, 143–160. doi: 10.1007/s10531-021-02326-0
Álvarez De Quevedo I., Félix M. S., Cardona L. (2013). Mortality rates in by-caught loggerhead turtle Caretta caretta in the Mediterranean Sea and implications for the Atlantic populations. Mar. Ecol. Prog. Ser. 489, 225–234. doi: 10.3354/meps10411
Araújo M. B., New M. (2007). Ensemble forecasting of species distributions. Trends Ecol. Evol. 22, 42–47. doi: 10.1016/j.tree.2006.09.010
Arcangeli A., David L., Aguilar A., Atzori F., Borrell A., Campana I., et al. (2020). Floating marine macro litter: Density reference values and monitoring protocol settings from coast to offshore. Results from the MEDSEALITTER project. Mar. pollut. Bull. 160, 111647. doi: 10.1016/j.marpolbul.2020.111647
Arcangeli A., Maffucci F., Atzori F., Azzolin M., Campana I., Carosso L., et al. (2019). Turtles on the trash track: Loggerhead turtles exposed to floating plastic in the Mediterranean Sea. Endanger. Species Res. 40, 107–121. doi: 10.3354/ESR00980
Attia El Hili H., Bradai M. N., Camedda A., Chaieb O., Claro F., Darmon G., et al. (2018). Pilot and feasibility studies for the implementation of littr impact indicators in the MSFD and RCSs OSPAR-macaronesia, HELCOM and Barcelona 158. INDICIT Deliv. n° D.2.5 Act. 2, dir. INDICIT Consort. Available at: https://www.patrinat.fr/fr/atom/760.
Ausubel J. H., Trew Crist D., Waggoner P. E. (2010). First census of marine life 2010. Highlights of a decade of discovery (Census of Marine Life), 64.
Avens L., Dell’Amico F. (2018). Evaluating viability of sea turtle foraging populations at high latitudes: age and growth of juveniles along the French Atlantic coast. Endanger. Species Res. 37, 25–36. doi: 10.3354/esr00913
Baillargeon S. (2005). Le krigeage: revue de la théorie et application à l’interpolation spatial de données de précipitations Vol. 137 (Laval: Mémoire maîtrise, Univ).
Barcelos L. M. D., Michielsen G., Sérgio B., Oliveira S., Barreiros J. P. (2021). First record of the olive ridley sea turtle, lepidochelys olivacea (Eschscholtz 1829), in the azores islands, northeastern atlantic ocean (testudines, cheloniidae). Herpetol. Notes 14, 371–373.
Bellido López J., Torreblanca E., Baez J. C., Camiñas J. (2018). Sea Turtles in the eastern margin of the north Atlantic: the northern ibero-Moroccan gulf as an important neritic area for sea turtles. Mediterr. Mar. Sci. 19, 662–672. doi: 10.12681/mms.15835
Bellier E., Certain G., Planque B., Monestiez P., Bretagnolle V. (2010). Modelling habitat selection at multiple scales with multivariate geostatistics: an application to seabirds in open sea. Oikos 119, 988–999. doi: 10.1111/j.1600-0706.2009.17808.x
Bellier E., Monestiez P., Guinet C. (2009). “Geostatistical modelling of wildlife populations: A non-stationary hierarchical model for count data,” in GeoENV VII – geostatistics for environmental applications. Eds. Atkinson P. M., Lloyd C. D. (Dordrecht: Springer Netherlands), 1–12. doi: 10.1007/978-90-481-2322-3_1
Bentley B. P., Kearney M. R., Whiting S. D., Mitchell N. J. (2020). Microclimate modelling of beach sand temperatures reveals high spatial and temporal variation at sea turtle rookeries. J. Therm. Biol. 88, 102522. doi: 10.1016/j.jtherbio.2020.102522
Bjorndal K. A., Bowen B. W., Chaloupka M., Crowder L. B., Heppell S. S., Al E. (2010). Assessment of sea-turtle status and trends: integrating demography and abundance. Washingt. Natl. Acad. Press 174. doi: 10.17226/12889
Blasi M. F., Mattei D. (2017). Seasonal encounter rate, life stages and main threats to the loggerhead sea turtle (Caretta caretta) in the aeolian archipelago (southern thyrrenian Sea). Aquat. Conserv. Mar. Freshw. Ecosyst. 27, 617–630. doi: 10.1002/aqc.2723
Bolten A. B. (2003). “Active swimmers – passive drifters: the oceanic juvenile stage of loggerheads in the Atlantic system,” in Loggerhead Sea turtles. Eds. Bolten A. B., Witherington B. E. (Washington DC, United States: Smithsonian Institution Press), 63–78.
Bowen B. W., Karl S. A. (2007). Population genetics and phylogeography of sea turtles. Mol. Ecol. 16, 4886–4907. doi: 10.1111/j.1365-294X.2007.03542.x
Buckland S. T., Anderson D. R., Burnham K. P., Laake J. L., Borchers D. L., Thomas. L. (2001). Introduction to distance sampling: Estimating abundance of biological populations Vol. 448 (Oxford, UK: Oxford Uni).
Cambiè G., Jribi I., Cambera I., Vagnoli G., Freggi D., Casale P. (2020). Intra-gear variation in sea turtle bycatch: Implications for fisheries management. Fish. Res. 221, 105405. doi: 10.1016/j.fishres.2019.105405
Carr A., Carr M., Meylan A. (1978). The ecology and migrations of sea turtles. 7, the West Caribbean green turtle colony. Bull. Am. Museum Nat. Hist. 162, 46.
Carreras C., Monzón-Argüello C., López-Jurado L. F., Calabuig P., Bellido J. J., Castillo J. J., et al. (2014). Origin and dispersal routes of foreign green and kemp’s ridley turtles in Spanish Atlantic and Mediterranean waters. Amphibia-Reptilia 35, 73–86. doi: 10.1163/15685381-00002929
Carreras C., Pascual M., Tomás J., Marco A., Hochsheid S., Castillo J. J., et al. (2018). Sporadic nesting reveals long distance colonisation in the philopatric loggerhead sea turtle (Caretta caretta). Sci. Rep. 8, 1–14. doi: 10.1038/s41598-018-19887-w
Carreras C., Pont S., Maffucci F., Sanfe M., Aguilar A. (2006). Genetic structuring of immature loggerhead sea turtles (Caretta caretta) in the Mediterranean Sea reflects water circulation patterns. Mar. Biol. 149, 1269–1279. doi: 10.1007/s00227-006-0282-8
Casale P. (2011). Sea Turtle by-catch in the Mediterranean. Fish Fish. 12, 299–316. doi: 10.1111/j.1467-2979.2010.00394.x
Casale P., Broderick A. C., Camiñas J. A., Cardona L., Carreras C., Demetropoulos A., et al. (2018). Mediterranean Sea turtles : current knowledge and priorities for conservation and research. Endanger. Species Res. 36, 229–267. doi: 10.3354/esr00901
Casale P., Ciccocioppo A., Vagnoli G., Rigoli A., Freggi D., Tolve L., et al. (2020a). Citizen science helps assessing spatio-temporal distribution of sea turtles in foraging areas. Aquat. Conserv. Mar. Freshw. Ecosyst. 30, 123–130. doi: 10.1002/aqc.3228
Casale P., Heppell S. S. (2016). How much sea turtle bycatch is too much? a stationary age distribution model for simulating population abundance and potential biological removal in the Mediterranean. Endanger. Species Res. 29, 239–254. doi: 10.3354/esr00714
Casale P., Hochscheid S., Kaska Y., Panagopoulou A. (2020b). Sea Turtles in the Mediterranean region: MTSG annual regional report 2020. Rep. IUCN-SSC Mar. Turtle Specialist Group 331.
Casale P., Nicolosi P., Freggi D., Turchetto M., Argano R. (2003). Leatherback turtles (Dermochelys coriacea) in italy and in the mediterranean basin. Herpetol. J. 13, 135–139.
Caswell H. (2001). Matrix population models: construction, analysis, and interpretation Vol. 722 (Sunderland, Massachusetts: Sinauer Associates).
Ceriani S. A., Casale P., Brost M., Leone E. H., Witherington B. E. (2019). Conservation implications of sea turtle nesting trends: elusive recovery of a globally important loggerhead population. Ecosphere 10, 19. doi: 10.1002/ecs2.2936
Chaloupka M. (2002). Stochastic simulation modelling of southern great barrier reef green turtle population dynamics. Ecol. Modell. 148, 79–109. doi: 10.1016/S0304-3800(01)00433-1
Chambault P., Baudena A., Bjorndal K. A., Santos M. A. R., Bolten A. B., Vandeperre F. (2019). Swirling in the ocean: Immature loggerhead turtles seasonally target old anticyclonic eddies at the fringe of the north Atlantic gyre. Prog. Oceanogr. 175, 345–358. doi: 10.1016/j.pocean.2019.05.005
Chambault P., Hattab T., Mouquet P., Bajjouk T., Jean C., Ballorain K., et al. (2021). A methodological framework to predict the individual and population-level distributions from tracking data. Ecography (Cop.). 44, 1–12. doi: 10.1111/ecog.05436
Chatzimentor A., Almpanidou V., Doxa A., Dimitriadis C., Mazaris A. D. (2021). Projected redistribution of sea turtle foraging areas reveals important sites for conservation. Clim. Change Ecol. 2, 100038. doi: 10.1016/j.ecochg.2021.100038
Clusa M., Carreras C., Pascual M., Gaughran S. J., Piovano S., Giacoma C., et al. (2014). Fine − scale distribution of juvenile Atlantic and Mediterranean loggerhead turtles (Caretta caretta) in the Mediterranean Sea. Mar. Biol. 161, 509–519. doi: 10.1007/s00227-013-2353-y
Crouse D. T., Crowder L. B., Caswell H. (1987). A stage-based population model for loggerhead Sea turtles and implications for conservation. Ecology 68, 1412–1423. doi: 10.2307/1939225
David L., Roul M., Belhadjer A., Monestiez P., Arnal C., Bourcoud M.-O., et al. Evolution of the fin whale and striped dolphin populations over 25 years in the north-Western Mediterranean Sea, submitted.
DeAngelis D. L., Mooij W. M. (2005). Individual-based modeling of ecological and evolutionary processes. Annu. Rev. Ecol. Evol. Syst. 36, 147–168. doi: 10.1146/annurev.ecolsys.36.102003.152644
Deporte N., Ulrich C., Mahévas S., Demanèche S., Bastardie F. (2012). Regional métier definition: a comparative investigation of statistical methods using a workflow applied to international otter trawl fisheries in the north Sea. ICES J. Mar. Sci. 69, 331–342. doi: 10.1093/icesjms/fsr197
DG Environment (2017). Reporting under article 17 of the habitats directive: Explanatory notes and guidelines for the period 2013-2018 Vol. 188 (Brussel).
Dierschke V., Kreutle A., Häubner N., Magliozzi C., Bennecke S., Bergström L., et al. (2021). Integration methods for the MSFD biodiversity assessments Vol. 45 (Luxembourg: EUR 30656 EN, Publications Office of the European Union), JRC124613, ISBN: ISBN 978-92-79-33990-8. doi: 10.2760/4751
Dimitriadis C., Mazaris A. D., Katsanevakis S., Iosifakis A., Spinos E., Kalli E., et al. (2022). Stranding records and cumulative pressures for sea turtles as tools to delineate risk hot spots across different marine habitats. Ocean Coast. Manage. 217, 106017. doi: 10.1016/j.ocecoaman.2021.106017
Doreímus G. (2021). Suivi de la distribution de la mégafaune marine en Manche, Atlantique et Méditerranée en 2020 Vol.42 (La Rochelle, France: Rapport scientifique de l’Observatoire PELAGIS).
Eckert S. A. (2006). High-use oceanic areas for Atlantic leatherback sea turtles (Dermochelys coriacea) as identified using satellite telemetered location and dive information. Mar. Biol. 149, 1257–1267. doi: 10.1007/s00227-006-0262-z
Esteban N., Mortimer J. A., Hays G. C. (2017). How numbers of nesting sea turtles can be overestimated by nearly a factor of two. Proc. R. Soc B Biol. Sci. 284, 7. doi: 10.1098/rspb.2016.2581
Estes J. A., Heithaus M., McCauley D. J., Rasher D. B., Worm B. (2016). Megafaunal impacts on structure and function of ocean ecosystems. Annu. Rev. Environ. Resour 41, 83–116. doi: 10.1146/annurev-environ-110615-085622
EU (2000). Council regulation (EC) no 1543/2000 of 29 June 2000 establishing a community framework for the collection and management of the data needed to conduct the common fisheries policy Off. J. Eur. Union L 176 Vol. 19, 1–16.
European Commission (1992). COUNCIL DIRECTIVE 92/43/EEC of 21 may 1992 on the conservation of natural habitats and of wild fauna and flora. Off. J. Eur. Union L/206, 7–50.
European Commission (2008). Directive 2008/56/EC of the European parliament and the council establishing a framework for community action in the field of marine environmental policy (Marine strategy framework directive). Off. J. Eur. Union L/164, 19–40.
European Commission (2017). COMMISSION DECISION (EU) 2017/848 of 17 may 2017 laying down criteria and methodological standards on good environmental status of marine waters and specifications and standardised methods for monitoring and assessment, and repealing decision 2010/477/EU. Off. J. Eur. Union L/125, 43–74.
FAO (2019). Monitoring the incidental catch of vulnerable species in Mediterranean and black Sea fisheries: Methodology for data collection. FAO Fish. Aquac. Tech. Pap. Rome FAO. 640, 106.
Fossette S., Hobson V. J., Girard C., Calmettes B., Gaspar P., Georges J., et al. (2010). Spatio-temporal foraging patterns of a giant zooplanktivore, the leatherback turtle. J. Mar. Syst. 81, 225–234. doi: 10.1016/j.jmarsys.2009.12.002
Frazier J. (2005). Marine turtles: The role of flagship species in interactions between people and the Sea. Mast 4, 5–38.
Fuentes M. M. P. B., Dawson J. L., Smithers S. G., Hamann M., Limpus C. J. (2010). Sedimentological characteristics of key sea turtle rookeries: potential implications under projected climate change. Mar. Freshw. Res. 61, 464–473. doi: 10.1071/MF09142
Fuentes M. M. P. B., Porter W. P. (2013). Using a microclimate model to evaluate impacts of climate change on sea turtles. Ecol. Modell. 251, 150–157. doi: 10.1016/j.ecolmodel.2012.12.020
Girard F., Catteau S., Gambaiani D., Gérigny O., Sénégas J. B., Moisson P., et al. (2021). Shift in demographic structure and increased reproductive activity of loggerhead turtles in the French Mediterranean Sea revealed by long-term monitoring. Sci. Rep. 11, 12. doi: 10.1038/s41598-021-02629-w
Girondot M., Kaska Y. (2015). Nest temperatures in a loggerhead nesting beach in Turkey is more determined by sea surface than air temperature. J. Therm. Biol. 47, 13–18. doi: 10.1016/j.jtherbio.2014.10.008
Girondot M., Mourrain B., Chevallier D., Godfrey M. H. (2021). Maturity of a giant: age and size reaction norm for sexual maturity for Atlantic leatherback turtles. Mar. Ecol. 42, e12631. doi: 10.1111/maec.12631
Halpern B. S., Frazier M., Potapenko J., Casey K. S., Koenig K., Longo C., et al. (2015). Spatial and temporal changes in cumulative human impacts on the world’s ocean. Nat. Commun. 6, 1–7. doi: 10.1038/ncomms8615
Hawkes L. A., Broderick A. C., Coyne M. S., Godfrey M. H., Godley B. J. (2007). Only some like it hot - quantifying the environmental niche of the loggerhead sea turtle. Divers. Distrib. 13, 447–457. doi: 10.1111/j.1472-4642.2007.00354.x
Hays G. C. (2000). The implications of variable remigration intervals for the assessment of population size in marine turtles. J. Theor. Biol. 206, 221–227. doi: 10.1006/jtbi.2000.2116
Hays G. C., Mazaris A. D., Schofield G., Laloë J.-O. (2017). Population viability at extreme sex-ratio skews produced by temperature-dependent sex determination. Proc. R. Soc B Biol. Sci. 284, 20162576. doi: 10.1098/rspb.2016.2576
Hays G. C., Scott R. (2013). Global patterns for upper ceilings on migration distance in sea turtles and comparisons with fish, birds and mammals. Funct. Ecol. 27, 748–756. doi: 10.1111/1365-2435.12073
Hedley S. L., Buckland S. T. (2004). Spatial models for line transect sampling. J. Agric. Biol. Environ. Stat. 9, 181–199. doi: 10.1198/1085711043578
Heppell S. S., Snover M. L., Crowder L. B. (2003). “Sea Turtle population ecology,” in The biology of sea turtles, vol. 2 . Eds. Lutz P. L., Musick J. A., Wyneken J. (Boca Raton: CRC Press), 275–306.
Hoegh-Guldberg O., Bruno J. F. (2010). The impact of climate change on the world’s marine ecosystems. Sci. (80-.). 328, 1523–1528. doi: 10.1126/science.1189930
ICES (2020). EU Request on emergency measures to prevent bycatch of common dolphin (Delphinus delphis) and Baltic proper harbour porpoise (Phocoena phocoena) in the northeast Atlantic. ICES Spec. Req. Advice. Northeast Atl. ecoregions 21.
ISPRA. Fixed line transect Mediterranean monitoring network - monitoring protocol for data collection on species, in press. Technical annex I.
IUCN (2020) The IUCN red list of threatened species. version 2020-3. Available at: https://www.iucnredlist.org.
IUCN Standards and Petitions Committee (2019). Guidelines for using the IUCN red list categories and criteria. version 14 Vol. 113 (Standards and Petitions Committee).
Jackson J. B. C., Kirby M. X., Berger W. H., Bjorndal K. A., Botsford L. W., Bourque B. J., et al. (2001). Historical overfishing and the recent collapse of coastal ecosystems. Sci. (80-.). 293, 629–637. doi: 10.1126/science.1059199
Karaa S., Jribi I., Bouain A., Girondot M., Bradaіö M. N. (2013). On the occurrence of leatherback turtles Dermochelys coriacea (Vandelli 1761), in Tunisian waters (Central Mediterranean Sea). Herpetozoa 26, 65–75.
Kelly I., Leon J., Gilby B., Olds A., Schlacher T. (2017). Marine turtles are not fussy nesters: a novel test of small-scale nest site selection using structure from motion beach terrain information. PeerJ 5, e2770. doi: 10.7717/peerj.2770
Kot C. Y., Fujioka E., DiMatteo A. D., Wallace B. P., Hutchinson B. J., Cleary J., et al. (2021). The state of the world’s Sea turtles online database: Data provided by the SWOT team and hosted on OBIS-SEAMAP (Oceanic Society, Conservation International, IUCN Marine Turtle Specialist Group (MTSG), and Marine Geospatial Ecology Lab, Duke University). Available at: http://seamap.env.duke.edu/swot.
Laloë J.-O., Monsinjon J., Gaspar C., Touron M., Genet Q., Stubbs J., et al. (2020). Production of male hatchlings at a remote south pacific green sea turtle rookery: conservation implications in a female-dominated world. Mar. Biol. 167, 70. doi: 10.1007/s00227-020-03686-x
Laran S., Nivière M., Genu M., Doreímus G., Serre S., Spitz J., et al. (2021). Distribution et abondance de la mégafaune marine lors des campagnes SAMM cycle I et II en Méditerranée Vol. 76 (La Rochelle, France: Observatoire Pelagis (UMS 3462, La Rochelle Universiteí/CNRS). Rapport final& OFB.
Laurent L., Lescure J. (1991). Hawksbill turtles in the Mediterranean Sea. Mar. Turt. Newsl. 54, 12–13.
Lauriano G., Panigada S., Casale P., Pierantonio N., Donovan G. P. (2011). Aerial survey abundance estimates of the loggerhead sea turtle Caretta caretta in the pelagos sanctuary, northwestern Mediterranean Sea. Mar. Ecol. Prog. Ser. 437, 291–302. doi: 10.3354/meps09261
Lauriano G., Pierantonio N., Kell L., Cañadas A., Donovan G., Panigada S. (2017). Fishery-independent surface abundance and density estimates of swordfish (Xiphias gladius) from aerial surveys in the central Mediterranean Sea. Deep Sea Res. Part II Top. Stud. Oceanogr. 141, 102–114. doi: 10.1016/j.dsr2.2017.04.019
Loisier A., Savelli M. P., Arnal V., Claro F., Gambaiani D., Sénégas J. B., et al. (2021). Genetic composition, origin and conservation of loggerhead sea turtles (Caretta caretta) frequenting the French Mediterranean coasts. Mar. Biol. 168, 15. doi: 10.1007/s00227-021-03855-6
Luschi P., Casale P. (2014). Movement patterns of marine turtles in the Mediterranean Sea: A review. Ital. J. Zool. 81, 478–495. doi: 10.1080/11250003.2014.963714
Luschi P., Sale A., Mencacci R., Hughes G. R., Lutjeharms J. R. E., Papi F. (2003). Current transport of leatherback sea turtles (Dermochelys coriacea) in the ocean. Proc. R. Soc B Biol. Sci. 270, 129–132. doi: 10.1098/rsbl.2003.0036
Maffucci F., Corrado R., Palatella L., Borra M., Marullo S., Hochscheid S., et al. (2016). Seasonal heterogeneity of ocean warming: A mortality sink for ectotherm colonizers. Sci. Rep. 6, 1–9. doi: 10.1038/srep23983
Maffucci F., Kooistra W. H. C. F., Bentivegna F. (2005). Natal origin of loggerhead turtles, Caretta caretta, in the neritic habitat off the Italian coasts, central Mediterranean. Biol. Conserv. 7, 3–9. doi: 10.1016/j.biocon.2005.08.009
Margaritoulis D., Argano R., Baran I., Bentivegna F., Bradai M. N., Camiñas J. A., et al. (2003). “Loggerhead turtles in the Mediterranean: Present knowledge and conservation perspectives,” in Biology and conservation of loggerhead sea turtles. Eds. Bolten A., Witherington B. (Washington D.C: Smithsonian Institution Press), 175–198.
Matsinos Y., Mazaris A., Pantis J. (2008). Evaluating the effect of varying clutch frequency in nesting trend estimation of sea turtles. Amphibia-Reptilia 29, 361–369. doi: 10.1163/156853808785111968
Mazaris A. D., Fiksen Ø., Matsinos Y. G. (2005). Using an individual-based model for assessment of sea turtle population viability. Popul. Ecol. 47, 179–191. doi: 10.1007/s10144-005-0220-5
Mazaris A. D., Matsinos Y. G. (2006). An individual based model of sea turtles: Investigating the effect of temporal variability on population dynamics. Ecol. Modell. 194, 114–124. doi: 10.1016/j.ecolmodel.2005.10.006
Mazaris A. D., Schofield G., Gkazinou C., Almpanidou V., Hays G. C. (2017). Global sea turtle conservation successes. Sci. Adv. 3, 1–7. doi: 10.1126/sciadv.1600730
Miller D. L., Burt M. L., Rexstad E. A., Thomas L. (2013). Spatial models for distance sampling data: Recent developments and future directions. Methods Ecol. Evol. 4, 1001–1010. doi: 10.1111/2041-210X.12105
Monestiez P., Dubroca L., Bonnin E., Durbec J.-P., Guinet C. (2006). Geostatistical modelling of spatial distribution of Balaenoptera physalus in the northwestern Mediterranean Sea from sparse count data and heterogeneous observation efforts. Ecol. Modell. 193, 615–628. doi: 10.1016/j.ecolmodel.2005.08.042
Monsinjon J., Jribi I., Hamza A., Ouerghi A., Kaska Y., Girondot M. (2017). Embryonic growth rate thermal reaction norm of Mediterranean Caretta caretta embryos from two different thermal habitats, Turkey and Libya. Chelonian Conserv. Biol. 16, 172–179. doi: 10.2744/CCB-1269.1
Monsinjon J. R., Wyneken J., Rusenko K., López-Mendilaharsu M., Lara P., Santos A., et al. (2019). The climatic debt of loggerhead sea turtle populations in a warming world. Ecol. Indic. 107, 105657. doi: 10.1016/j.ecolind.2019.105657
Monzón-Argüello C., Cardona L., Calabuig P., Camacho M., Crespo-Picazo J. L., García-Párraga D., et al. (2018). Supplemental feeding and other anthropogenic threats to green turtles (Chelonia mydas) in the canary islands. Sci. Total Environ. 621, 1000–1011. doi: 10.1016/j.scitotenv.2017.10.126
Musick J., Limpus C. (1997). “Habitat utilization and migration in juvenile sea turtles,” in The biology of sea turtles, vol. 432 . Eds. Lutz P., Musick J. (Boca Raton: CRC Press).
Oliver M. A., Webster R. (2013). A tutorial guide to geostatistics: Computing and modelling variograms and kriging. CATENA 113, 56–69. doi: 10.1016/j.catena.2013.09.006
OSPAR (2018) Harbour porpoise bycatch. intermediate assessment. Available at: https://oap.ospar.org/en/ospar-assessments/intermediate-assessment-2017/biodiversity-status/marine-mammals/harbour-porpoise-bycatch/.
OSPAR Commission (1992). Convention for the protection of the marine environment of the north-east Atlantic. OSPAR Conv. 33.
OSPAR Commission (2019). CEMP guideline M4. common indicator: Abundance and distribution at the relevant spatio-temporal scale of cetacean species regularly present (M4), Vol. 9, 10.
Palialexis A., Boschetti S. (2021). Review and analysis of member states’ 2018 reports descriptor 1: Species biological diversity, EUR 30664 EN Vol. 167 (Luxembourg: Publications Office of the European Union), JRC 124085ISBN 978-92-76-34256-4. doi: 10.2760/27700
Palialexis A., Cardoso A. C., Somma F. (2018). JRC’s reference lists of MSFD species and habitats, EUR 29125 EN Vol. 39 (Luxembourg Publications Office of the European Union), JRC110960. doi: 10.2760/79418
Palialexis A., Connor D., Damalas D., Gonzalvo J., Micu D., Mitchel I., et al. (2019). Indicators for status assessment of species, relevant to MSFD biodiversity descriptor Vol. 66 (Luxembourg: Publications Office of the European Union), JRC117126, ISBN: ISBN 978-92-76-09156-1. EUR 29820 EN. doi: 10.2760/282667
Palialexis A., Kousteni V., Boicenco L., Enserink L., Pagou K., Zweifel U. L., et al. (2021). Monitoring biodiversity for the EU marine strategy framework directive: Lessons learnt from evaluating the official reports. Mar. Policy 128, 104473. doi: 10.1016/j.marpol.2021.104473
Palialexis A., Tornero V., Barbone E., Gonzalez D., Hanke G., Cardoso A. C., et al. (2014). In-depth assessment of the EU member states’ submissions for the marine strategy framework directive under articles 8, 9 and 10 Vol. 31 (Luxembourg: Publications Office of the European Union), JRC88072, ISBN: ISBN 978-92-79-35273-7. EUR 26473. doi: 10.2760/794186
Panigada S., Boisseau O., Canadas A., Lambert C., Laran S., McLanaghan R., et al (2021). Estimates of abundance and distribution of cetaceans, marine mega-fauna and marine litter in the Mediterranean Sea from 2018-2019 surveys. (Monaco: ACCOBAMS - ACCOBAMS Survey Initiative Project, Monaco), 177.
Parga M. L., Crespo-Picazo J. L., Monteiro D., García-Párraga D., Hernandez J. A., Swimmer Y., et al. (2020). On-board study of gas embolism in marine turtles caught in bottom trawl fisheries in the Atlantic ocean. Sci. Rep. 10, 1–9. doi: 10.1038/s41598-020-62355-7
Patrício A., Hawkes L., Monsinjon J., Godley B., Fuentes M. (2021). Climate change and marine turtles: recent advances and future directions. Endanger. Species Res. 44, 363–395. doi: 10.3354/esr01110
Phillips S. J., Dudík M. (2008). Modeling of species distributions with maxent: new extensions and a comprehensive evaluation. Ecography (Cop.). 31, 161–175. doi: 10.1111/j.0906-7590.2008.5203.x
Piacenza S. E., Richards P. M., Heppell S. S. (2019). Fathoming sea turtles: monitoring strategy evaluation to improve conservation status assessments. Ecol. Appl. 29, 1–16. doi: 10.1002/eap.1942
Pike D. A. (2013). Climate influences the global distribution of sea turtle nesting. Glob. Ecol. Biogeogr. 22, 555–566. doi: 10.1111/geb.12025
Pinto C., Thorburn J. A., Neat F., Wright P. J., Wright S., Scott B. E., et al. (2016). Using individual tracking data to validate the predictions of species distribution models. Divers. Distrib. 22, 682–693. doi: 10.1111/ddi.12437
Pipa T., Atchoi E., Silva C., Bécares J., Gil M., Freitas L., et al. (2019). Applying a sub-regional coherent and coordinated approach to the monitoring and assessment of marine biodiversity in macaronesia for the second cycle of the MSFD,” in MISTICSEAS II final technical report (TRWP1), vol. 339.
Polovina J. J., Howell E., Kobayashi D. R., Seki M. P. (2001). The transition zone chlorophyll front, a dynamic global feature defining migration and forage habitat for marine resources. Prog. Oceanogr. 49, 469–483. doi: 10.1016/S0079-6611(01)00036-2
Polovina J. J., Kobayashi D. R., Parker D. M., Seki M. P., Balazs G. H. (2000). Turtles on the edge: Movement of loggerhead turtles (Caretta caretta) along oceanic fronts, spanning longline fishing grounds in the central north pacific 1997-1998. Fish. Oceanogr. 9, 71–82. doi: 10.1046/j.1365-2419.2000.00123.x
Punt A. E., Siple M. C., Francis T. B., Hammond P. S., Heinemann D., Long K. J., et al. (2020). Can we manage marine mammal bycatch effectively in low-data environments? J. Appl. Ecol. 58, 1–12. doi: 10.1111/1365-2664.13816
Revelles M., Isern-Fontanet J., Cardona L., San Félix M., Carreras C., Aguilar A. (2007). Mesoscale eddies, surface circulation and the scale of habitat selection by immature loggerhead sea turtles. J. Exp. Mar. Bio. Ecol. 347, 41–57. doi: 10.1016/j.jembe.2007.03.013
Revuelta O., Carreras C., Domènech F., Gozalbes P., Tomás J. (2015). First report of an olive ridley (Lepidochelys olivacea) in the Mediterranean Sea. Mediterr. Mar. Sci. 16, 346–351. doi: 10.12681/mms.1131
Saavedra C., Santos M. B., Valcarce P., Freitas L., Silva M., Pipa T., et al. (2018). MISTICSEAS II - macaronesian roof report, Vol. 116.
Sacchi J. (2008). Impact des techniques de pêche sur l’environnement en méditerranée Vol. 62 (Rome: Commission Générale des pêches pour la Méditerranée).
Schoener T. W. (1968). The anolis lizards of bimini: Resource partitioning in a complex fauna. Ecology 49, 704–726. doi: 10.2307/1935534
Seminoff J. A., Eguchi T., Carretta J., Allen C. D., Prosperi D., Rangel R., et al. (2014). Loggerhead sea turtle abundance at a foraging hotspot in the eastern pacific ocean: Implications for at-sea conservation. Endanger. Species Res. 24, 207–220. doi: 10.3354/esr00601
Solow A. R., Bjorndal K. A., Bolten A. B. (2002). Annual variation in nesting numbers of marine turtles: the effect of sea surface temperature on re-migration intervals. Ecol. Lett. 5, 742–746. doi: 10.1046/j.1461-0248.2002.00374.x
Sparks L. M., DiMatteo A. D. (2020). Loggerhead Sea turtle density in the Mediterranean Sea. NUWC-NPT Tech. Rep. 360, 77.
Stokes K. L., Fuller W. J., Glen F., Godley B. J., Hodgson D. J., Rhodes K. A., et al. (2014). Detecting green shoots of recovery: The importance of long-term individual-based monitoring of marine turtles. Anim. Conserv. 17, 593–602. doi: 10.1111/acv.12128
Swimmer Y., Empey Campora C., Mcnaughton L., Musyl M., Parga M. (2014). Post-release mortality estimates of loggerhead sea turtles (Caretta caretta) caught in pelagic longline fisheries based on satellite data and hooking location. Aquat. Conserv. Mar. Freshw. Ecosyst. 24, 498–510. doi: 10.1002/aqc.2396
Tepsich P., Schettino I., Atzori F., Azzolin M., Campana I., Carosso L., et al. (2020). Trends in summer presence of fin whales in the western mediterranean sea region: New insights from a long-term monitoring program. PeerJ 8, 1–27. doi: 10.7717/peerj.10544
The Northwest Atlantic Leatherback Working Group (2019)Dermochelys coriacea (Northwest Atlantic ocean subpopulation). In: The IUCN red list of threatened species 2019 (Accessed January 25, 2022). e.T46967827A83327767.
Thomas L., Buckland S. T., Rexstad E. A., Laake J. L., Strindberg S., Hedley S. L., et al. (2010). Distance software: Design and analysis of distance sampling surveys for estimating population size. J. Appl. Ecol. 47, 5–14. doi: 10.1111/j.1365-2664.2009.01737.x
Tomás J., Raga J. A. (2007). Occurrence of kemp’s ridley sea turtle (Lepidochelys kempii) in the Mediterranean. Mar. Biodivers. Rec. 1, 2. doi: 10.1017/S1755267207006409
Turkozan O., Almpanidou V., Yılmaz C., Mazaris A. D. (2021). Extreme thermal conditions in sea turtle nests jeopardize reproductive output. Clim. Change 167, 30. doi: 10.1007/s10584-021-03153-6
Vandeperre F., Parra H., Pham C. K., Machete M., Santos M., Bjorndal K. A., et al. (2019). Relative abundance of oceanic juvenile loggerhead sea turtles in relation to nest production at source rookeries: implications for recruitment dynamics. Sci. Rep. 9, 1–12. doi: 10.1038/s41598-019-49434-0
Varo-Cruz N., Cejudo D., Calabuig P., Herrera R., Urioste J., Monzón-Argüello C. (2017). Records of the hawksbill Sea turtle (Eretmochelys imbricata) in the canary islands. Mar. Turt. Newsl. 154, 1–6.
Virgili A., Authier M., Boisseau O., Cañadas A., Claridge D., Cole T., et al. (2019). Combining multiple visual surveys to model the habitat of deep-diving cetaceans at the basin scale. Glob. Ecol. Biogeogr. 28, 300–314. doi: 10.1111/geb.12850
Visbeck M. (2018). Ocean science research is key for a sustainable future. Nat. Commun. 9, 1–4. doi: 10.1038/s41467-018-03158-3
Wade P. R. (1998). Calculating limits to the allowable human-caused mortality of cetaceans and pinnipeds. Mar. Mammal Sci. 14, 1–37. doi: 10.1111/j.1748-7692.1998.tb00688.x
Wallace B. P., DiMatteo A. D., Hurley B. J., Finkbeiner E. M., Bolten A. B., Chaloupka M. Y., et al. (2010a). Regional management units for marine turtles: A novel framework for prioritizing conservation and research across multiple scales. PLoS One 5, 1–11. doi: 10.1371/journal.pone.0015465
Wallace B. P., Lewison R. L., Mcdonald S. L., Mcdonald R. K., Kot C. Y., Kelez S., et al. (2010b). Global patterns of marine turtle bycatch. Conserv. Lett. 3, 131–142. doi: 10.1111/j.1755-263X.2010.00105.x
Warden M. L., Haas H. L., Richards P. M., Rose K. A., Hatch J. M. (2017). Monitoring trends in sea turtle populations: Walk or fly? Endanger. Species Res. 34, 323–337. doi: 10.3354/esr00855
Witherington B., Hirama S., Mosier A. (2011). Barriers to Sea turtle nesting on Florida (United states) beaches: Linear extent and changes following storms. J. Coast. Res. 27, 450–458. doi: 10.2112/JCOASTRES-D-09-00146.1
Witt M. J., Broderick A. C., Johns D. J., Martin C., Penrose R., Hoogmoed M. S., et al. (2007a). Prey landscapes help identify potential foraging habitats for leatherback turtles in the NE Atlantic. Mar. Ecol. Prog. Ser. 337, 231–243. doi: 10.3354/meps337231
Witt M. J., Hawkes L. A., Godfrey M. H., Godley B. J., Broderick A. C. (2010). Predicting the impacts of climate change on a globally distributed species: The case of the loggerhead turtle. J. Exp. Biol. 213, 901–911. doi: 10.1242/jeb.038133
Witt M. J., Penrose R., Godley B. J. (2007b). Spatio-temporal patterns of juvenile marine turtle occurrence in waters of the European continental shelf Marine Biology 151, 873–885. doi: 10.1007/s00227-006-0532-9
Wood D. W., Bjorndal K. A. (2000). Relation of temperature, moisture, salinity, and slope to nest site selection in loggerhead Sea turtles. Copeia 1, 119–128. doi: 10.1643/0045-8511(2000)2000[0119:ROTMSA]2.0.CO;2
Keywords: MSFD, sea turtles, good environmental status, indicators, Mediterranean Sea, north-east Atlantic Ocean
Citation: Girard F, Girard A, Monsinjon J, Arcangeli A, Belda E, Cardona L, Casale P, Catteau S, David L, Dell’Amico F, Gambaiani D, Girondot M, Jribi I, Lauriano G, Luschi P, March D, Mazaris AD, Miaud C, Palialexis A, Sacchi J, Sagarminaga R, Tepsich P, Tomás J, Vandeperre F and Claro F (2022) Toward a common approach for assessing the conservation status of marine turtle species within the european marine strategy framework directive. Front. Mar. Sci. 9:790733. doi: 10.3389/fmars.2022.790733
Received: 07 October 2021; Accepted: 20 September 2022;
Published: 10 October 2022.
Edited by:
Maria Grazia Pennino, Spanish Institute of Oceanography (IEO), SpainReviewed by:
Letizia Marsili, University of Siena, ItalyMarta Coll, Institute of Marine Sciences, Spanish National Research Council (CSIC), Spain
Copyright © 2022 Girard, Girard, Monsinjon, Arcangeli, Belda, Cardona, Casale, Catteau, David, Dell’Amico, Gambaiani, Girondot, Jribi, Lauriano, Luschi, March, Mazaris, Miaud, Palialexis, Sacchi, Sagarminaga, Tepsich, Tomás, Vandeperre and Claro. This is an open-access article distributed under the terms of the Creative Commons Attribution License (CC BY). The use, distribution or reproduction in other forums is permitted, provided the original author(s) and the copyright owner(s) are credited and that the original publication in this journal is cited, in accordance with accepted academic practice. No use, distribution or reproduction is permitted which does not comply with these terms.
*Correspondence: Andreas Palialexis, QW5kcmVhcy5wYWxpYWxleGlzQGVjLmV1cm9wYS5ldQ==
†Present address: Jonathan Monsinjon, Institut Français de Recherche pour l’Exploitation de la Mer (Ifremer), Délégation Océan Indien (DOI), Le Port, France