- 1School of Aquatic and Fishery Sciences, University of Washington, Seattle, WA, United States
- 2Cascadia Research Collective, Olympia, WA, United States
- 3Washington Department of Fish and Wildlife, Marine Mammal Investigations, Lakewood, WA, United States
- 4Biology Department, Portland State University, Portland, OR, United States
- 5Marine Mammal Institute, Oregon State University, Newport, OR, United States
- 6Conservation Biology Division, Northwest Fisheries Science Center, National Marine Fisheries Service, National Oceanic & Atmospheric Administration, Seattle, WA, United States
- 7The SeaDoc Society, UC Davis School of Veterinary Medicine, Karen C. Drayer Wildlife Health Center – Orcas Island Office, Eastsound, WA, United States
- 8The Whale Museum, Friday Harbor, WA, United States
- 9Makah Fisheries Management, Makah Tribe, Neah Bay, WA, United States
- 10Orca Network, Central Puget Sound Marine Mammal Stranding Network, Freeland, WA, United States
- 11Port Townsend Marine Science Center, Port Townsend, WA, United States
- 12Oregon Department of Fish and Wildlife, Salem, OR, United States
- 13Oregon Institute of Marine Biology, University of Oregon, Charleston, OR, United States
- 14Whatcom Marine Mammal Stranding Network, Lummi Island, WA, United States
- 15World Vets, Gig Harbor, WA, United States
- 16NOAA Fisheries, Protected Resources Division, West Coast Region, Seattle, WA, United States
- 17Seaside Aquarium, Seaside, OR, United States
- 18Marine-Med: Marine Research, Epidemiology, and Veterinary Medicine, Bothell, WA, United States
Studying patterns in marine mammal stranding cases can provide insight into changes in population health, abundance, and distribution. Cetaceans along the United States West coast strand for a wide variety of reasons, including disease, injury, and poor nutritional status, all of which may be caused by both natural and anthropogenic factors. Examining the potential drivers of these stranding cases can reveal how populations respond to changes in their habitat, notably oceanographic variability and anthropogenic activities. In this study, we aim to synthesize recent patterns in 1,819 cetacean strandings across 26 species in the Pacific Northwest from 2000 to 2019 to compare with previous findings. Additionally, we aim to quantify the effects of localized and basin-scale oceanographic conditions on monthly stranding cases for five focal species using generalized additive models in order to explore potential relationships between strandings and changes in biophysical features that could affect foraging conditions or other important physiological cues. Our results suggest that strandings of harbor porpoises, gray whales, humpback whales, Dall’s porpoises, and striped dolphins are correlated with certain environmental variables, including sea surface temperature, chlorophyll concentration, and the Pacific Decadal Oscillation depending on the species. While it remains challenging to identify the causal mechanisms that underlie these relationships for a given species or population based on its utilization of such a complex ecosystem, improving our understanding of periods of increased strandings can enhance our knowledge of how these species interact with their environment and assist conservation and management efforts. This study enhances the utility of stranding records over time beyond simply reporting trends and has broader applicability to other geographic regions amid global climate change.
Introduction
Over the past several decades, marine mammal stranding records have been used as an indicator of ocean and cetacean health (Gulland and Hall, 2007; Bogomolni et al., 2010; Bossart, 2011). Examining where, when, and how often marine mammals strand can provide insight into ecological behaviors, reproductive success (Norman et al., 2004; Pikesley et al., 2012), health status, the impacts of human activities (Warlick et al., 2018), and local species distribution and abundance (Evans et al., 2005; MacLeod et al., 2005). The presence of cetaceans is strongly influenced by changes in the marine environment via diverse and dynamic mechanisms, including changes in sea surface temperature, winds, or large-scale oceanographic oscillations that can, among other factors, shift the balance of nutrients and prey species abundance and distribution (Ballance et al., 2006). These changes can be amplified through the food web and may be exacerbated by bioaccumulation of pollutants or toxins from algal blooms, ultimately having noticeable effects on top predators. Here we investigated the possible effects of oceanographic variability on cetacean strandings by evaluating stranding records collected consistently and systematically from 2000 to 2019 in the Pacific Northwest.
As top predators, marine mammals may be particularly sensitive to alterations in oceanographic and climatic patterns (Moore, 2008; Evans et al., 2010). Recent studies have found correlations between long-term stranding trends and several indices of climatic variability, demonstrating how strandings may be used as bio-indicators of prevailing environmental conditions. Evans et al. (2005) observed that cetacean strandings in southeast Australia exhibited a periodicity coincident with regional wind patterns. Factors such as sea ice and the North Atlantic Oscillation have been found to correlate with strandings and mortality of certain pinniped and cetacean species in the Gulf of St. Lawrence, Canada (Johnston et al., 2012; Soulen et al., 2013; Truchon et al., 2013). El Niño conditions have corresponded with increased California sea lion (Zalophus californianus) strandings and fisheries interactions along the California coast (Keledjian and Mesnick, 2013). Berini et al. (2015) noted that pygmy sperm whale (Kogia breviceps) strandings in the southeast United States were positively correlated with sea surface temperatures, wind, and barometric pressure.
Environmental changes are acknowledged to be occurring on a global scale (IPCC, 2014), though the local realization of these changes is patchy and difficult to predict due to ecosystem complexity and spatial heterogeneity (Moore, 2008; Evans and Bjørge, 2013; Jacox et al., 2016). Because responses to environmental change are complex, variable, species-dependent, and often poorly understood, oceanographic features should be studied over varying scales (local, continental, and temporal), ecotypes, and cetacean species (Laidre et al., 2008; Evans and Bjørge, 2013; Truchon et al., 2013). Large-scale climate processes such as the El Niño–Southern Oscillation (ENSO) and Pacific Decadal Oscillation (PDO) have decade-scale effects on the biophysical conditions and food webs of the California Current and Salish Sea ecosystems (Newton, 1995; Mantua and Hare, 2002; Chavez et al., 2003; Hickey and Banas, 2003). During the zonal wind phase after the 1970’s, decreased upwelling and warming waters were observed in the California Current (McGowan et al., 1998), resulting in a simultaneous decline in primary productivity, zooplankton, pelagic fish, and seabirds. After 2000, sea surface temperatures cooled (Oviatt et al., 2015), suggesting that enhanced upwelling and productivity likely occurred, with increased zooplankton abundance noticed since the late 1990s (Lavaniegos and Ohman, 2007). In recent years, the California Current ecosystem experienced an “extreme marine heat wave” that became known as “the Blob”, where above average water temperatures persisted from 2014 to 2016, causing a wide range of changes in primary productivity, fish spawning, larval abundance, and marine wildlife health (Bond et al., 2015; Di Lorenzo and Mantua, 2016; Auth et al., 2017).
These anomalous oceanographic conditions along the United States West Coast, along with increasing ocean acidification and harmful algal blooms in the Pacific Northwest (Mote and Salathé, 2010; Mauger et al., 2015) can negatively impact marine mammal population dynamics through changes in the abundance and distribution of their prey, among other effects (McCabe et al., 2016; Santora et al., 2020). The United States Pacific Northwest encompasses coastal, inland, and estuarine waters extending from northern California through British Columbia, including the Salish Sea and the mouth of the Columbia River. It is an ecosystem that contains important feeding and breeding habitat for numerous marine mammal species in the eastern north Pacific and beyond, including gray whales (Eschrichtius robustus), humpback whales (Megaptera novaeangliae), endangered southern resident killer whales (Orcinus orca), and numerous smaller odontocete species (Calambokidis et al., 2015; Norman et al., 2018).
Studying the mechanisms that give rise to patterns in marine mammal strandings is complicated by numerous factors, one of which is that observed strandings are not necessarily a direct reflection of changes in actual mortality rates. While actual mortality rates may be changing over a given time period, observed changes in stranding cases may be more a product of seasonal changes in abundance and distribution (e.g., for migratory species such as large whales), interannual changes in abundance and distribution (e.g., population recovery or recolonization), changes in reporting protocols and capacity (e.g., less reporting in winter or inaccessible coastal areas), and changes in oceanographic conditions or the cause of strandings that may affect the likelihood of an individual washing onshore versus sinking at sea (Moore et al., 2020). Despite these caveats, examining changes in strandings over time provides important information for monitoring cetacean populations, tracking distribution or abundance trends, and examining emerging health or disease conditions, particularly in light of recently documented changes in oceanographic conditions on both local and regional scales (Pierce et al., 2007; Truchon et al., 2013; Sprogis et al., 2017). The goals of this study were to compare recent cetacean stranding patterns in the Pacific Northwest to those previously reported for 1930–2002 (Norman et al., 2004); examine strandings as a proxy for the changing prevalence of cetacean species within certain geographic areas; and to investigate possible relationships between spatiotemporal variation in cetacean strandings and oceanographic conditions. Understanding the complex mechanisms that affect marine mammal strandings with respect to changes in environmental conditions can provide useful insights into the health of cetacean populations and has broad applicability to other geographic regions amid global climate change.
Materials and Methods
Study Area and Stranding Data
We compiled all available Level A records of cetacean strandings (2000–2019) that are maintained by the U. S. NOAA Fisheries and its stranding response network members in Oregon and Washington. Strandings are detected by dedicated beach survey efforts (Huggins et al., 2015a) or reports from the public or field researchers, which are then verified by stranding network partners. Stranding reports are submitted regularly to NOAA’s national stranding database by network members and include basic data such as observation date, stranding location, and when determinable, age class, sex, status (dead or alive), species, evidence of injury or human interaction (HI), and level of postmortem condition. Level A data do not include the cause of the stranding event or mortality. Reports containing ambiguous species identification, regardless of source, were included in one of several ‘Unknown’ categories based on the level of information known.
With the exception of live free-swimming entangled animals, cases noted as involving human interactions were included in the total stranding counts reported in this study. Documented HI findings within this dataset included those that were unrelated to any apparent cause of stranding or mortality (e.g., healed entanglement wound), and determination of HI can be hindered by decomposition or inability to conduct a thorough external and internal examination. Though the inclusion of cases where HI likely contributed to the stranding could complicate the interpretation of correlations with environmental variables, incidents of HI could hypothetically be exacerbated by certain oceanographic conditions as has been documented for pinnipeds (Keledjian and Mesnick, 2013).
Approximately 15 percent of all cases during the study period were observed in a state of advanced decomposition and 6 percent of cases were missing this information. These cases were retained to maximize sample size and document total strandings over the study period, though this could introduce a small degree of bias in our results with respect to the seasonality of strandings (largely for harbor porpoise and gray whales, as they comprise approximately 50% and 25% of these more decomposed or unknown decomposition state cases, respectively.
Stranding records were aggregated by year, month, geographic state (OR/WA), and stranding region (inland Washington waters east of the western entrance to the Strait of Juan de Fuca versus the outer coast), similar to Warlick et al. (2018). This study region has a diverse coastline ranging from rocky intertidal zones to estuarine embayments and variable degrees of public access and development (e.g., residential and commercial districts, ports, fishing activity, shipping and ferry channels, and ecotourism). Seasons were defined as Spring: March-May; Summer: June-August; Fall: September-November; Winter: December-February. Strandings for all species were qualitatively summarized, though only five focal species were explored using statistical analyses.
Environmental Data
Local Oceanographic Conditions
Variables representing local oceanographic conditions were obtained from satellite data and reanalysis products using NOAA data server ERDDAP (Simons, 2019), and Copernicus Marine Environment Monitoring Service, spanning an area off the coasts of Oregon and Washington (42.0°N/123.0°W to 48.3°N/127.3°W; Figure 1). The spatio-temporal scope for environmental covariates was selected with the aim of being localized and relevant to the cetaceans and yet large enough to encompass likely foraging habitat and smooth over fine-scale anomalies. Sea surface temperature (SST, °C) and chlorophyll concentration were obtained from Aqua MODIS satellite sensor data (NOAA Southwest Fisheries Science Center Environmental Research Division (SWFSC ERD), 2019) and aggregated for monthly values for each year 2000–2019 across the study region. Data on mixed layer depth, sea surface height (an atmospheric index of climate that represents the average of variations in the highs and lows of the ocean’s surface; Oviatt et al., 2015), and meridional (north-south longitudinally-directed) and zonal (east-west latitudinally directed) winds were derived from the ARMOR3D L4 reanalysis data product (Mertz et al., 2019) and also aggregated at the monthly level. Changes in SST can be indicative of El Niño conditions that can also be associated with changing prey availability. Chlorophyll, mixed layer depth, and indices of wind velocity can be measures of the degree and strength of upwelling and primary production, which both affect the timing, amount, type, and diversity of available copepods and, after a certain amount of lag time, forage fish and other prey items (Peterson and Keister, 2003; Tian et al., 2004; Ainsworth et al., 2011; Oviatt et al., 2015; Santora et al., 2017). Sea surface height can be a measure of eddy strength, general ocean circulation, storm activity, and changes in atmospheric pressure, which can all alter the aforementioned prey indices.
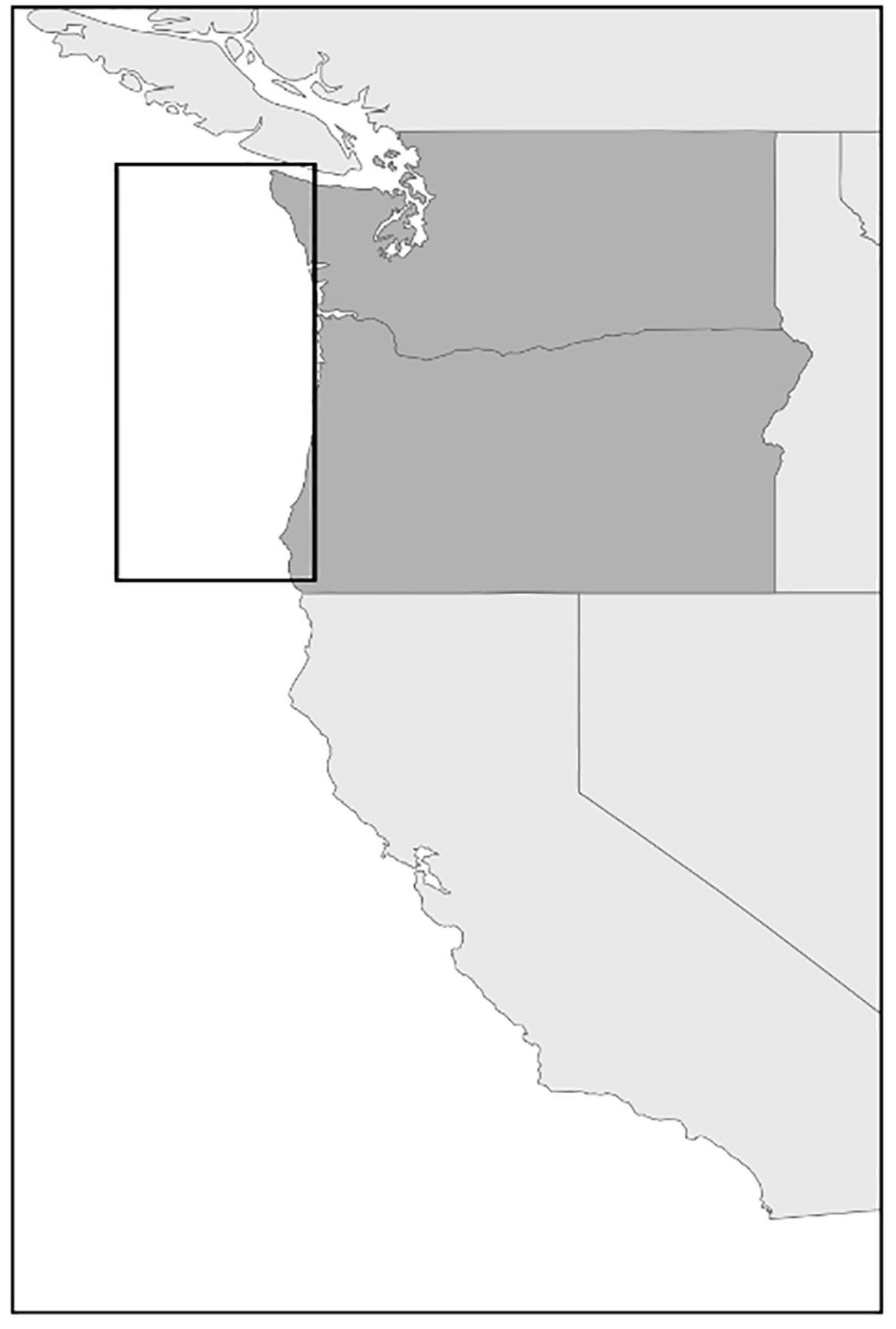
Figure 1. Map of the study area indicating Oregon and Washington in darker gray with the black rectangle representing the spatial extent from which localized and satellite data for oceanographic covariates were gathered.
Large-Scale Oceanographic Oscillations
Variables used to assess the effect of large-scale, climatic factors on strandings included the Multivariate El Niño Southern Oscillation index (MEI), the PDO, and the North Pacific Gyre Oscillation (NPGO), all of which were obtained from the California Current Integrated Ecosystem Assessment (IEA) project data portal at the monthly level (NOAA Integrated Ecosystem Assessment (IEA), 2019). The MEI describes El Niño conditions since it combines six meteorological measures over portions of the Pacific Ocean (Wolter and Timlin, 1993). Large positive MEI values indicate the occurrence of El Niño conditions, while large negative MEI values indicate La Niña conditions. The PDO represents a recurring pattern of climate variability (Mantua and Hare, 2002) with historical records strongly suggesting an association with salmon production (Beamish et al., 1999; Hare et al., 1999) and zooplankton production in the eastern North Pacific Ocean (Francis et al., 2003). The NPGO is largely associated with variation in sea surface height, salinity, and nutrient concentrations (Di Lorenzo et al., 2008). These local and basin-scale variables were included in this exploratory analysis because we hypothesized that changes in these dynamic landscape features could affect the distribution, abundance, and, availability of prey species or other factors that could directly affect survival, reproduction, or the probability of individuals washing onshore.
Statistical Analysis
To examine seasonal and interannual patterns in stranding cases and whether variability in monthly strandings correlated with oceanographic conditions, we fit generalized additive models (GAM; Hastie and Tibshirani, 1990),
ys,m,t ∼ Poisson(μs,m,t) (1)
log(μs,m,t) = β0s + f1(Yeart) + f2(Monthm) + fb(xm,t) (2)
where the number of stranding cases y for each species s in each month m and year t were assumed to be Poisson-distributed with the natural log of mean monthly cases μs,m,t being a function of intercept β0 and smooth functions f for year, month, and b number of environmental covariates x (Equations 1-2).
To account for the fact that the larger migratory species are only seasonally present in the study region, additional models examining strandings and environmental covariates at the annual level were conducted for gray and humpback whales, though only a limited number of covariates could be included simultaneously due to the smaller sample size. Additionally, models for harbor porpoises were conducted at the regional level (e.g., inland versus outer coast strandings) to account for potential differences in habitat use among the different ecosystems and harbor porpoise populations. Results, however, are largely reported for aggregate harbor porpoise strandings.
The GAM framework enables the examination of potentially non-linear relationships between strandings and oceanographic conditions (e.g., if there was an ideal temperature range for a given species below and/or above which strandings increased). The effective degrees of freedom (edf) is a measure of the degree of ‘wiggliness’ in that non-linear relationship, where an edf close to 1.0 would indicate that the relationship is in fact linear (values < 1.0 indicate shrinkage due to the null space penalization). The GAMs were fit with a Poisson distribution and a cyclic cubic spline to estimate the effect of month and a thin plate spline for all other variables using restricted maximum likelihood (REML) using the mgcv package (Wood, 2011) in the R statistical programming language (R Development Core Team, 2019).
Variable selection with respect to oceanographic covariates was conducted using null space penalization and removing variables with an edf less than 0.4. The final model was selected by minimizing the Akaike’s Information Criterion (AIC) (Akaike, 1973) in conjunction with biological relevance. Because many of the oceanographic variables are inter-related, we tested for collinearity between variables using a Pearson’s correlation coefficient and did not include highly correlated variables in the same models. The best models that included oceanographic variables were compared to null models and models with only year and month effects. P-values of smooth terms are largely approximate due to the challenge of comparing degrees of freedom between GAM models (Wood, 2011). Environmental conditions were considered in both real-time and one- to three-month lags. Longer lag times that may be ecologically realistic were not explored due to the constraints of examining a reasonable number of possible models. To qualitatively examine spatio-temporal stranding patterns across seasons and regions, hotspot maps were generated with a kernel density estimation (Gatrell et al., 1996) (‘geom_density2d’ function in the ggplot2 R package).
Methodological Limitations
All stranded animals, regardless of cause of death, were included in the analysis, though the cause of death was not included in GAM models. Although it could be illuminating to formally include cause of death in the analysis, the sample size would be limited due to lack of this information for most strandings. This study also has the added complexity of not being able to define the relationship between environmental conditions and health of the animal, nor how the environmental indices relate to habitat use, distribution patterns, or the likelihood of a carcass washing ashore.
Results
Over the study period (2000–2019), 1,819 cetacean strandings were recorded in Oregon (n = 613) and Washington (n = 1,206) (Tables 1, 2) across 26 species and a hybrid combination (Phocoena phocoena/Phocoenoides dalli). The number of strandings in Washington was further broken down by outer coast (n = 615) and inland waters (n = 591). Nearly all cases (96%) were identified to species level. The five most commonly stranded species were harbor porpoise, gray whale, Dall’s porpoise (Phocoenoides dalli), striped dolphin (Stenella coeruleoalba), and humpback whales. Mean annual strandings over the study period for harbor porpoises, gray whales, and Dall’s porpoise were 56, 10.4, and 5.5 cases per year, respectively, and combined represented approximately 79% of total strandings (Table 1). Striped dolphins were the most commonly stranded delphinid species (annual mean = 4.7; 3% of all total strandings), with 79% of reports in Oregon. Mean annual strandings for humpback whales, 3 per year, represented 2.5% of total stranding cases. Though sex could not be determined for approximately one-third of strandings, there was no significant difference between the number of males and females over the study period for any of the five most commonly stranded species. Greater than one-third (n = 437; 38%) of all harbor porpoise strandings were reported in the inland waters of Washington State, 33% (n = 377) along Washington’s outer coast, and the remainder (n = 322) in Oregon.
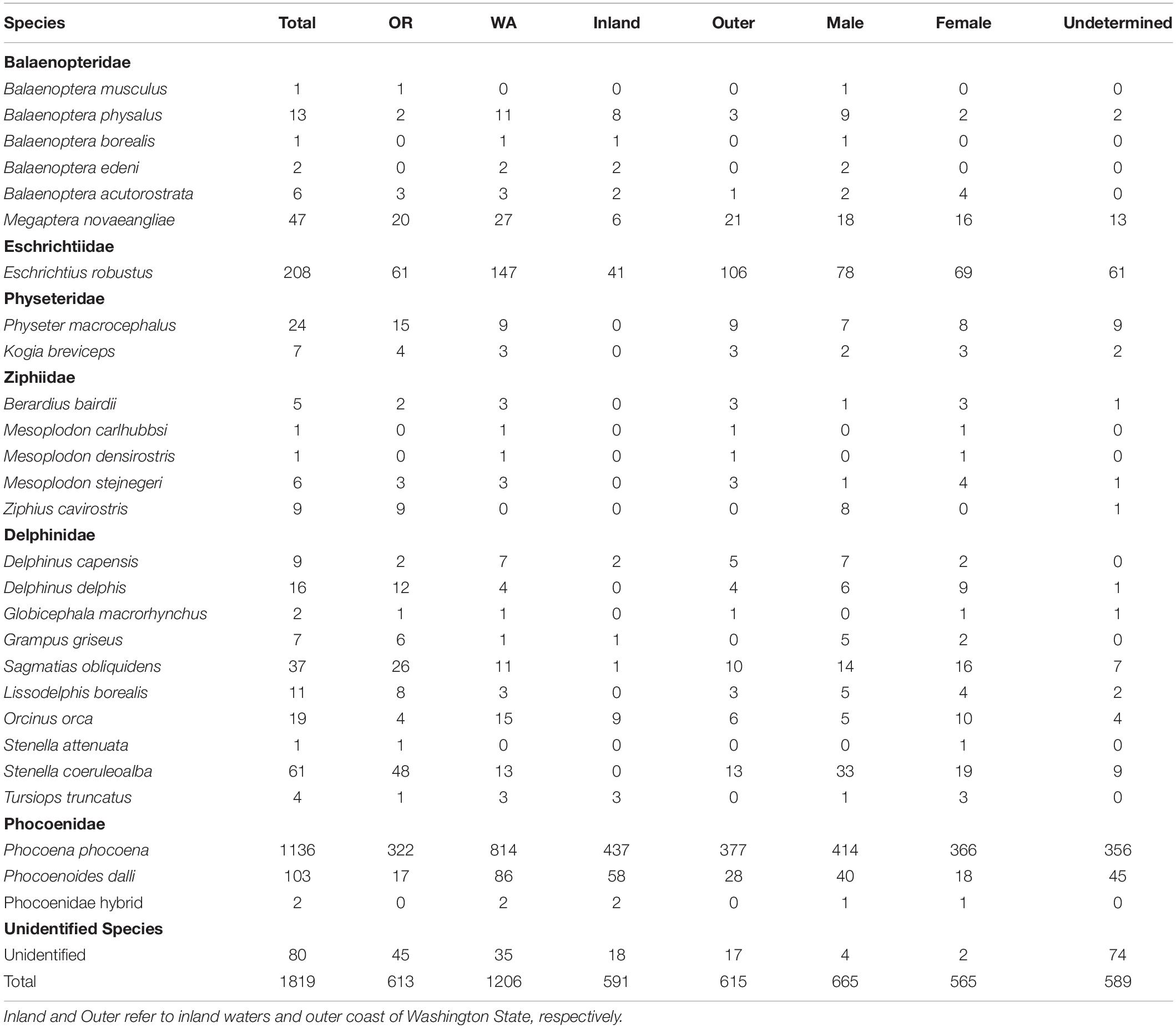
Table 1. Cetacean stranding cases in Oregon (OR) and Washington (WA) from 2000 to 2019 by species and sex.
Temporal Distribution
Over the study period, combined strandings have increased over time, though this pattern has varied across species (Table 2). Harbor porpoise strandings increased throughout the 2000s and then peaked in 2012. Reports of stranded humpback whales and striped dolphins in the study area have increased since 2003 while Dall’s porpoise strandings have decreased and gray whale strandings have fluctuated interannually with little apparent directional trend during the study period (Figure 2).
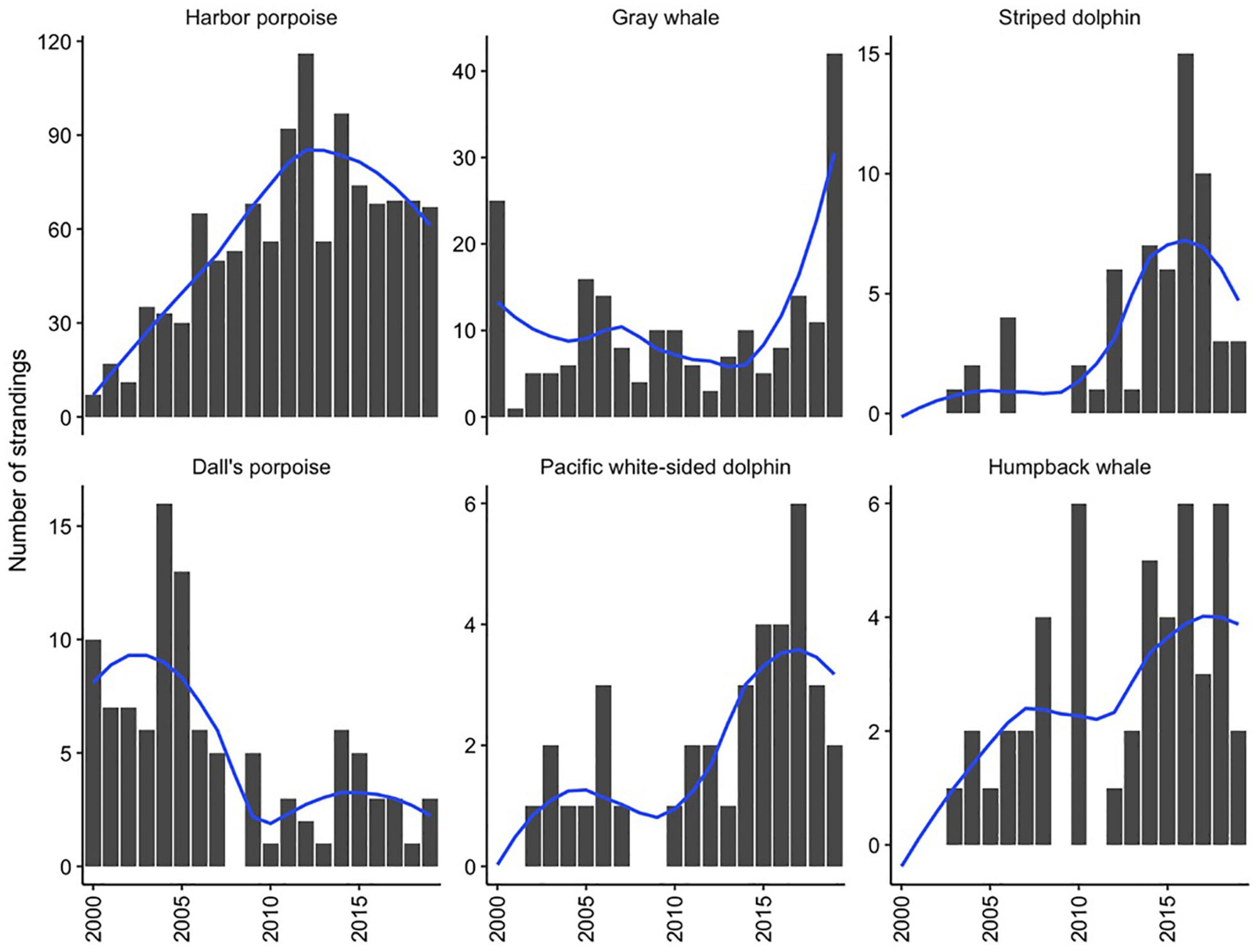
Figure 2. Total annual stranding cases and smoothed loess line for the six most commonly stranding species from 2000 to 2019 in Oregon and Washington. Note difference in scale of y-axes.
Overall, there were notable differences in the number of strandings per season, with fewer strandings across all species in the fall and winter (mean of 12–15 per year per season) compared with the spring and summer (mean of 28–36 per year per season). The effect of month in GAMs models was significant for four of the five focal species, indicating seasonal peaks in strandings between June-August for harbor porpoises and humpback whales, and an earlier peak in May for Dall’s porpoises and gray whales, though these patterns depended on the region (Figure 3).
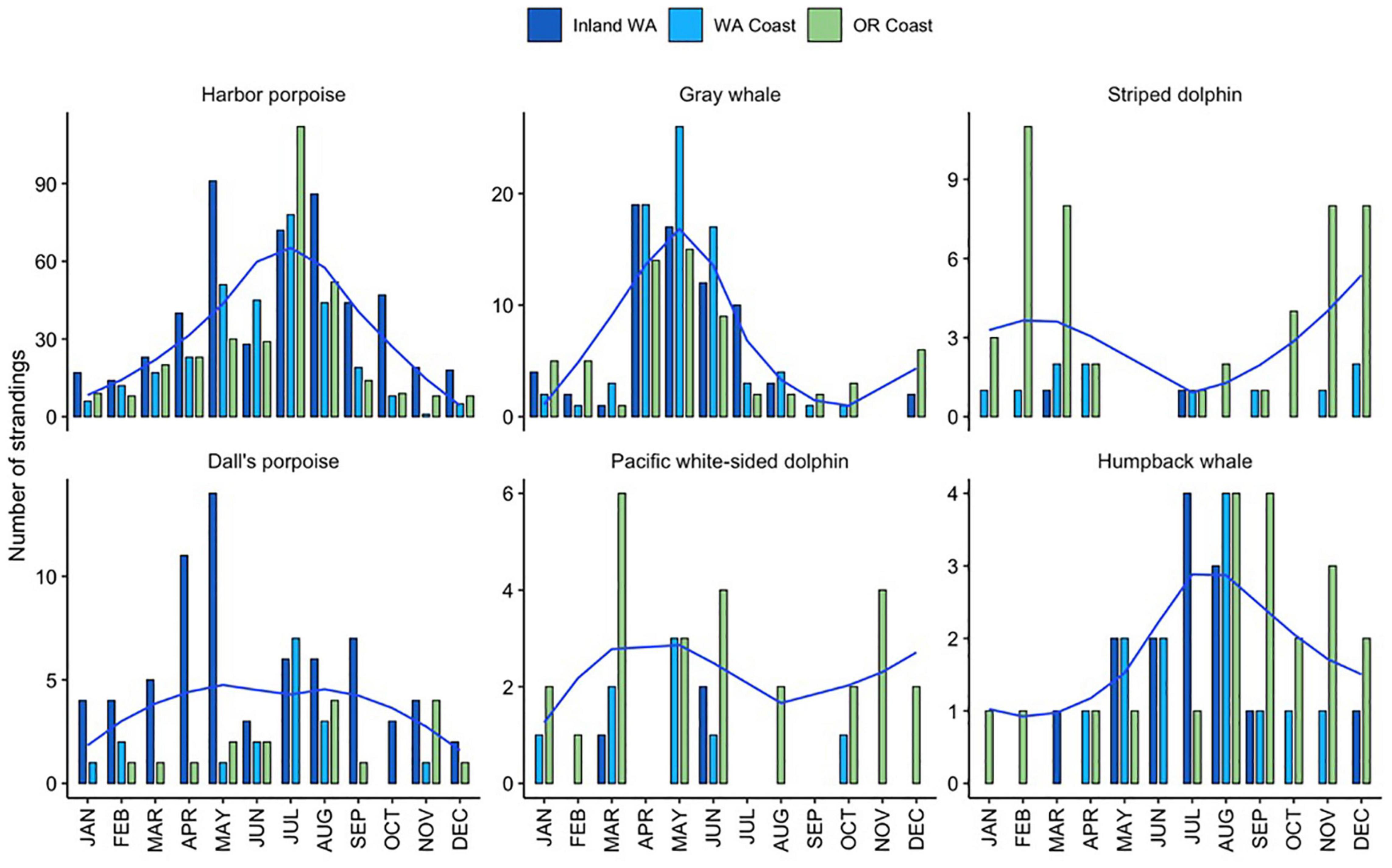
Figure 3. Total monthly regional stranding cases (bars) and smoothed loess line for total combined cases across regions (blue) for the six most commonly stranding species from 2000 to 2019 in Oregon and inland and coastal Washington.
Spatial Distribution
Overall, the number of strandings reported in inland Washington waters was higher than that reported along the coasts, though this was largely driven by harbor porpoise strandings. Other frequently stranded species exhibited different spatial trends, with a higher number of striped dolphins stranding in Oregon, Dall’s porpoise strandings clustering in inland Washington waters, and gray whales along the outer Washington coast (Figure 4). At the seasonal level, gray and humpback whale strandings were concentrated further south in Oregon on the outer coast during fall compared with other months when more strandings occurred in Washington (Figure 4). For harbor porpoises, cases were more frequently reported on the outer coast at their peak in the summer compared to being relatively more concentrated in inland waters the rest of the year.
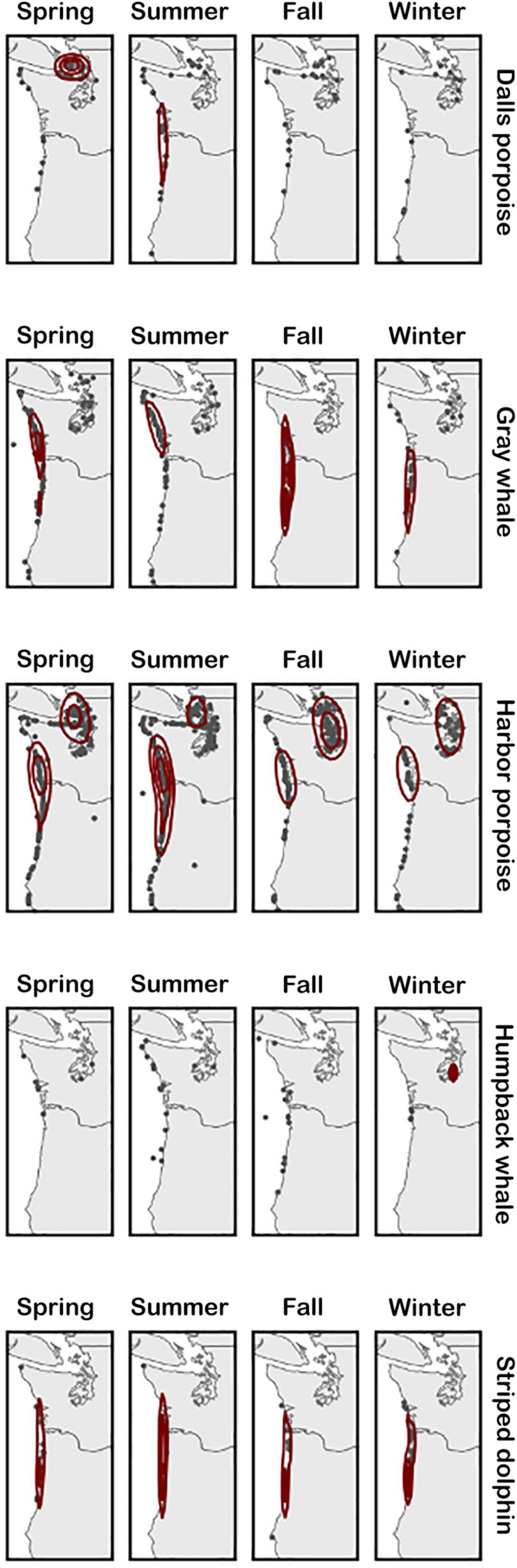
Figure 4. Seasonal distribution of stranding cases for each of the five most commonly stranding species. Kernel density estimation is calculated for each season and species separately, therefore contour lines are intended to show the spatial density of strandings in each panel relative to itself, not compared to others.
Oceanographic Conditions
To examine the effect of ocean conditions and interannual and seasonal patterns of stranding cases, GAMs were fit for the five most commonly stranded focal species: harbor porpoise, gray and humpback whales, Dall’s porpoise, and striped dolphin. For all five focal species, including environmental covariates improved model fit as evidenced by lower AIC values and higher deviance explained (Table 3).
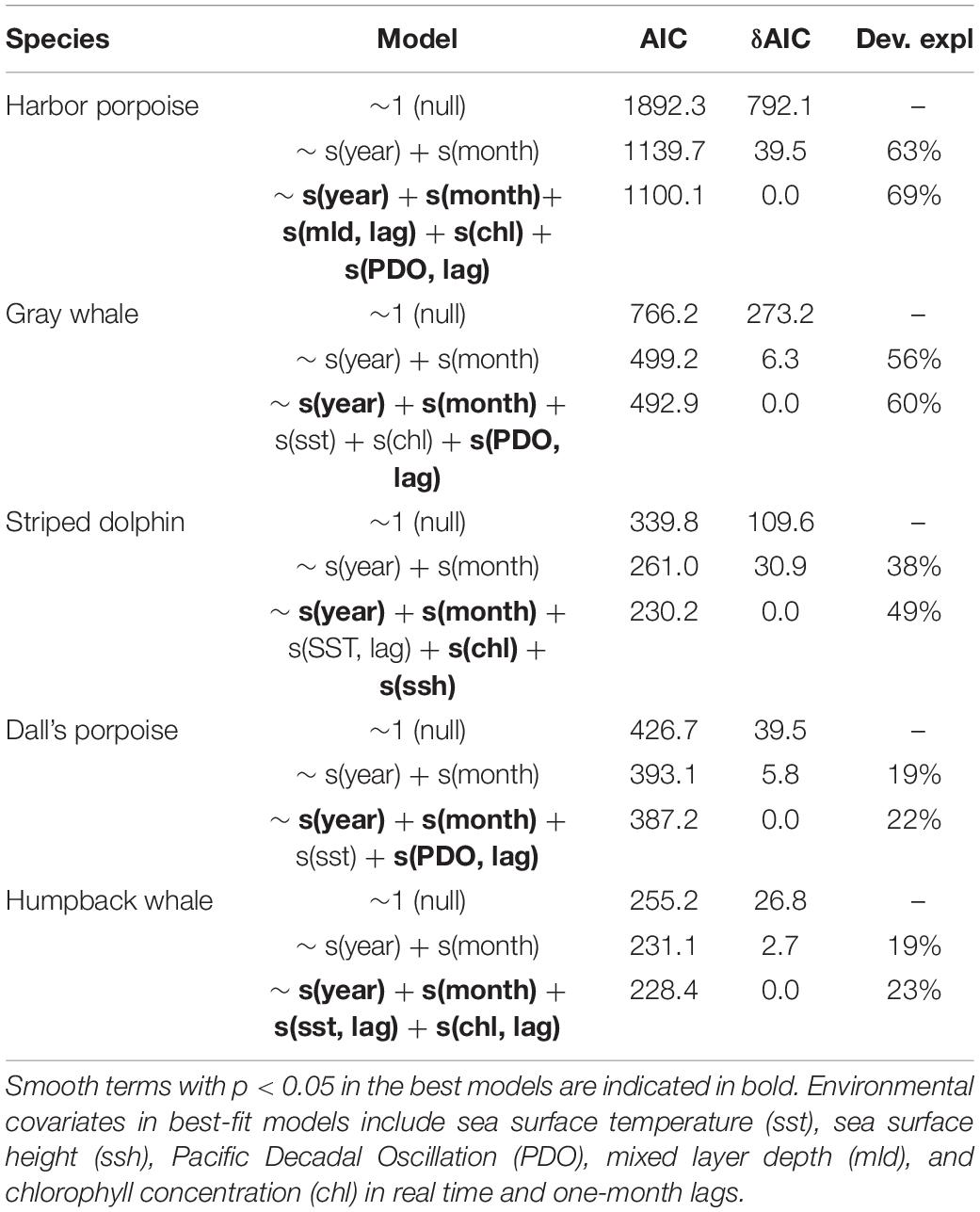
Table 3. Best models for monthly stranding counts for harbor porpoise, gray whale, Dall’s porpoise, and striped dolphin over the study period compared to null models and models without environmental covariates, including the effective degrees of freedom (edf) and p-values for smooth effect terms, Akaike Information Criterion (AIC) values, and deviance explained.
For harbor porpoise, the best model of aggregate region-wide strandings included smooth effects of year (edf = 5.1, p < 0.01), month (edf = 5.6, p < 0.01), chlorophyll (edf = 4.7, p < 0.01), and one-month lagged PDO (edf = 1.1, p < 0.1) and mixed layer depth (edf = 5.8, p < 0.01) (Figure 5). This model would predict increased strandings in late summer and under conditions with lower chlorophyll concentrations, smaller mixed layer depths, and positive phase PDO. Though not included in the best-fit model due to being correlated with chlorophyll concentration, meridional and zonal wind velocities were also strong predictors of monthly stranding cases. This best model with environmental covariates explained 69% deviance, higher than the other focal species most likely due to the higher sample size rather than a tighter association between strandings and oceanographic conditions. These environmental indices explained 71% of deviance for outer coast strandings, but only 42% of deviance for strandings that occurred in inland waters.
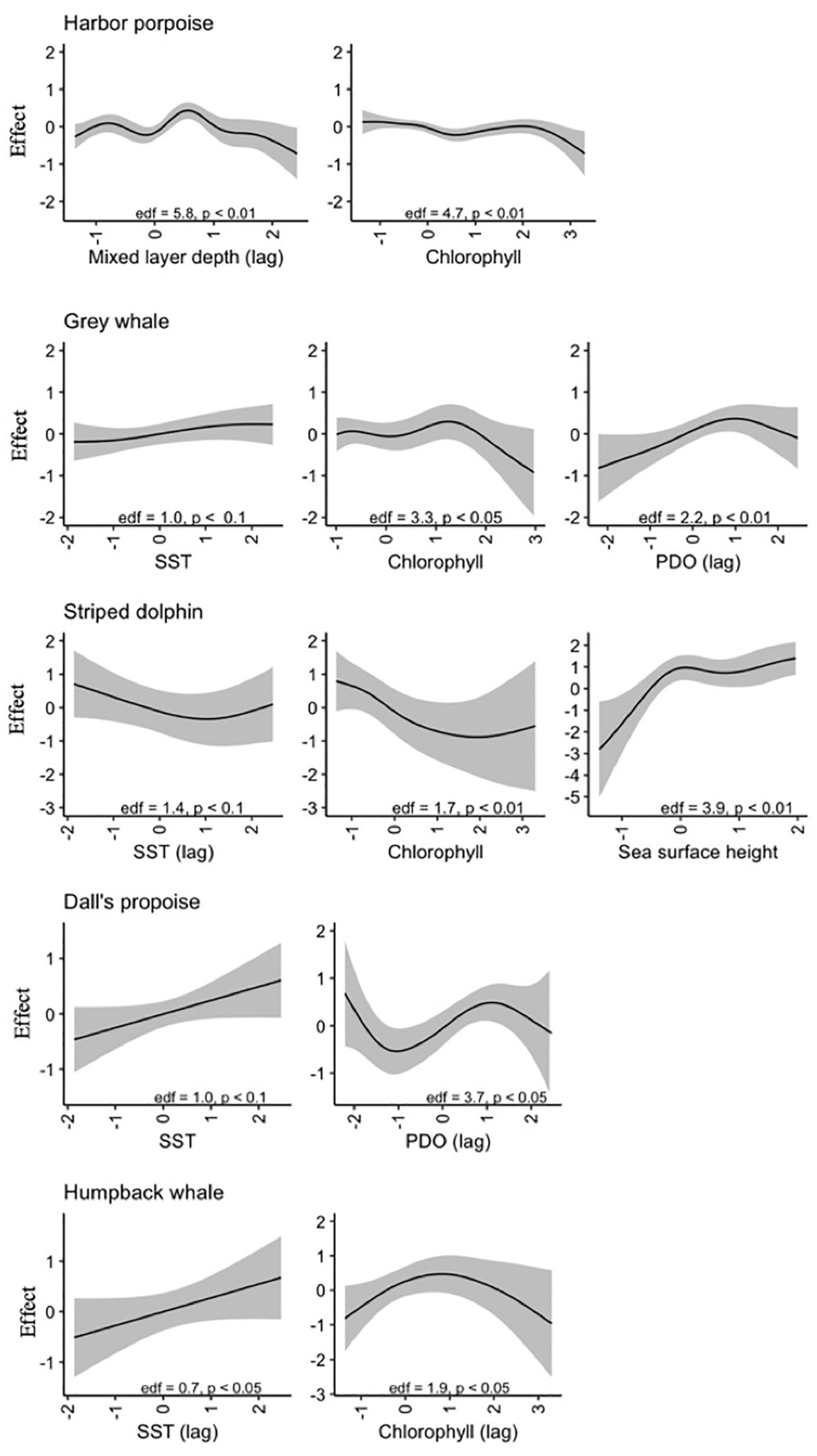
Figure 5. Estimated smooth effects of environmental variables for best-fit models of monthly stranding cases for each of five focal species, with effective degrees of freedom (edf), confidence intervals, and p-values indicated.
Monthly gray whale strandings were best explained by a model that included smooth terms for year (edf = 5.9, p < 0.01), month (edf = 5.4, p < 0.01), sea surface temperature (edf = 1.0, p < 0.1), chlorophyll (edf = 3.3, p < 0.05), and one-month lagged PDO (edf = 2.2, p < 0.01). This model would predict decreased strandings in conditions with lower temperatures, higher chlorophyll concentration, and negative phase PDO, with a seasonal peak in May (Figure 5). The addition of environmental covariates to the model contributed less to model fit compared to the other species (56% versus 60% deviance explained, Table 3). At the annual level, the best-fit model included smooth terms for MEI (edf = 6.5, p < 0.01) and year (edf = 1.9, p < 0.01), which explained 58% of deviance. Gray whale strandings were notably higher in 2000 and 2019 compared to all the years in between.
Striped dolphin strandings have exhibited an increasing trend (edf = 1.1, p < 0.01) and the best-fit model included smooth effects of month (edf = 3.2, p < 0.01), sea surface height (edf = 3.9, p < 0.01), chlorophyll (edf = 1.7, p < 0.01), and lagged sea surface temperature (edf = 1.4, p < 0.1) (Figure 3). This model would predict a higher number of cases in late winter-early spring or during months with increased sea surface height and lower temperatures and chlorophyll concentrations (Figure 5). This model with covariates explained more of the deviance (49%) and had a lower AIC value compared to the model with only an interannual effect (38% deviance, Table 3).
In the case of Dall’s porpoise strandings, strandings have decreased over the study period (edf = 1.0, p < 0.01) and the best model included smooth effects of month (edf = 1.5, p < 0.05) and lagged PDO (edf = 3.7, p < 0.05), and linear effects of SST (edf = 1.0, p < 0.5) (Figure 5). This best model would predict peak seasonal strandings during May-June, increased strandings with warmer temperatures and decreased strandings with negative-phase PDO values (Figure 5). Including environmental covariates improved model fit but the model overall has low explained deviance at 22% (Table 3).
Humpback whale strandings have increased in recent years. The best model for this species included a nearly linear effect of year (edf = 1.2, p < 0.05) and lagged SST (edf = 0.7, p < 0.05), and smooth effects of month (edf = 1.8, p < 0.05) and lagged chlorophyll (edf = 1.8, p < 0.05). This best model would predict peak strandings in summer and lower strandings in months after higher chlorophyll concentrations and lower temperatures (Figure 5). Including environmental covariates only marginally improved model fit (23% deviance explained) over the model with just annual and monthly effects (19% deviance explained) (Table 3). However, the best model fit to annual strandings included smooth terms for SST (edf = 0.8, p < 0.01) and chlorophyll (edf = 2.1, p < 0.05) and explained 57% of deviance.
Discussion
This study examined spatial, annual, and seasonal trends in cetacean stranding cases in the Pacific Northwest region of the United States with the aim of comparing these results to previously identified stranding patterns and to examine whether some variability in stranding cases can be explained by oceanographic conditions over the study period. Stranding reports for many species increased since 2000 and are notably higher per year than numbers reported by Norman et al. (2004). Incorporating environmental variables in GAMs improved model fit for all of the five most commonly stranded species (though some more notably than others), indicating that some variability in stranding cases can be explained by oceanographic conditions. The GAM framework is beneficial in this context because it allows for the estimation of non-linear effects of environmental covariates, which may most accurately reflect how species likely have optimum niche ranges for certain oceanographic conditions or ecosystem states that affect prey availability, foraging behavior, the prevalence of storms, or even disease. Our results indicate that it may be possible to identify relationships between oceanographic variability and patterns in strandings for some species. However, we cannot definitively identify the causal mechanisms behind these correlations with this framework, particularly due to our exploration of relatively short temporal lag times and the noise that exists in stranding patterns due to unmodeled changes in localized abundance and distribution of each species, which would be better suited for more focused future studies.
Interannual Variability
Our results suggest that the interannual variability of marine mammal stranding events over a 20-year period is associated with both regional and basin-scale oceanographic conditions, though patterns and correlations differ for each of the five most commonly stranded species (Table 2). The smaller number of monthly stranding cases for many species makes the identification of these correlations impossible for all species that stranded over the study period.
Long-term increases in the number of reported strandings may result from changes in reporting effort due to increasing coastal activities, human habitation, public interest in marine mammals, as well as changes in the abundance and distribution of the species themselves (Huggins et al., 2015a; Segawa and Kemper, 2015; Prado et al., 2016; Pitchford et al., 2018; Warlick et al., 2018). Harbor porpoise are a good example of possible influences of changes in reporting effort and changes in abundance on the number of observed strandings.
An increasing trend in harbor porpoise strandings was documented until 2011–2012, at which time a plateau or decline in stranding reports was observed, continuing through 2019 (Figure 2). This notable rise in stranding cases over the study period, particularly in inland waters (Norman et al., 2004; Huggins et al., 2015b), likely reflects a greater presence of this species in inland Washington waters (i.e., Puget Sound), its seasonal distribution, and the growth of coastal and inland populations over this time period through mediation of anthropogenic factors such as fishery interactions and pollutants (Hanson, 2007; Smultea et al., 2015; Evenson et al., 2016; Jefferson et al., 2016). Similar increases in population growth were documented in California harbor porpoise stocks after protection from gill net fishery bycatch was implemented (Forney et al., 2020). Other factors potentially contributing to the increased harbor porpoise reports include changes in stranding response effort and the distribution and impact of human activities, which could similarly affect all species in this study.
While gray whale strandings were highly variable by year, the high number in 2000 and 2019 reflected larger trends in gray whale mortality along their entire range from Mexico to the Arctic. Gray whales have experienced two Unusual Mortality Events (UMEs) during the study period that remain undetermined: one occurring from 1999 to 2000 (Moore et al., 2001; Gulland et al., 2005) and one beginning in 2019 and extending throughout 2021 (NOAA Fisheries, 2021).
The number of Dall’s porpoise strandings significantly decreased until 2011, which could have been a change in population size or distribution. Anecdotal evidence has demonstrated fewer live sightings throughout inland Washington waters by individuals spending a large portion of their time on the water. However, recent abundance estimates have not been conducted to confirm this. Notable increases in stranding cases occurred for striped dolphins and humpback whales. Though striped dolphin abundance trends have varied interannually from 1991 to 2014 (Becker et al., 2012; Barlow, 2016), strandings significantly increased over the last 20 years in the Pacific Northwest compared to numbers previously reported (Norman et al., 2004), with a steep increase beginning in 2010–2011 (Figure 2). An increase in the number of stranded humpback whales occurred over the study period, with a particular uptick starting in 2008, likely reflecting increases in the population of the California-Oregon-Washington stock over the past 20–30 years, and a shift in habitat use such that more animals are now occupying northeastern Pacific Ocean waters (Barlow et al., 2011; Calambokidis et al., 2017; Calambokidis and Barlow, 2020).
Spatio-Temporal Trends
Spatio-temporal trends in stranding cases likely largely reflect the seasonal distribution and prevalence of each species based on life history characteristics such as calving requirements and migratory and foraging behavior. However, because such a large proportion of cases are reported by the public, strandings are more likely to be detected and reported from more densely populated areas with accessible beaches (inland waters) in warmer, drier, beach-going months (summer).
The majority (72%) of harbor porpoise strandings occurred in WA (particularly inland waters) and peaked seasonally in August, with a lesser peak in late spring, in contrast to a pattern of maximum strandings in summer and fall previously reported (Norman et al., 2004). Though cause of stranding and mortality is generally not discussed here, a noteworthy finding was that most of the fishery interactions documented in harbor porpoises were recorded in the fall months (September-November) and were spatially concentrated along the western shoreline of mid- to southern Puget Sound. These cases were confirmed with detailed examinations and elimination of other major contributory causes of death (Huggins et al., 2015b; NOAA Fisheries, unpublished data). However, it is important to interpret findings of HI on stranded animals with extreme caution, as strandings demonstrating signs of HI do not necessarily indicate that the injury or condition was the cause of stranding (e.g., could include cases with previously healed entanglement wounds) and it is often impossible to ascertain cause of death with certainty. Though many commercial and tribal salmon fisheries are highly regulated (Washington Department of Fish and Wildlife, 2020), interactions with other net-based fisheries may still exist on a smaller scale and may be underreported. HI cases are likely underestimated because evidence of human-caused injury and mortality can go undetected in strandings that are not necropsied or are in an advanced state of decomposition.
The proportion of harbor porpoise strandings occurring in inland WA versus the outer coast (55 vs. 45%, respectively) has remained relatively similar since those reported in Norman et al. (2004), and have followed similar peaks and troughs over the last 20 years. However, one notable difference is the seasonal peak in regional strandings. Prior to 2000, strandings peaked in late summer and early fall (Norman et al., 2004). In this study, we found that strandings increased in May (particularly for the outer WA coast) and peaked in July (for inland WA and OR coast) (Figure 3). This pattern can be validated by the recent higher observed live porpoise numbers in spring. The cause of this seasonal shift and the general rise in sightings remains unknown (Jefferson et al., 2016). Harbor porpoises are the most commonly stranded cetacean in the Pacific Northwest, as also noted in the timeframe studied in Norman et al. (2004), and variations in their stranding numbers could signify a change in health, mortality, abundance, and distribution, a shift in environmental conditions, or changes in the physiological or environmental causes of stranding that affect the likelihood of carcasses washing onshore.
While a small number of gray whales are present off the coasts of Oregon and Washington during all seasons of the year (Calambokidis et al., 2002), the primary eastern North Pacific gray whale population only migrates past Oregon and Washington in winter and spring (Pike, 1962; Rice and Wolman, 1971). Gray whale strandings peaked in May, coincident with the end of their northward migration past WA when their body reserves are most depleted after their seasonal fast in wintering areas off Mexico (Pike, 1962; Rice and Wolman, 1971). This pattern was also observed in years prior to 2000 (Norman et al., 2004). Although we found several environmental factors that may be related to gray whale strandings, gray whale migration timing is more likely driven by their biological clock and instincts rather than by environmental features (Guazzo et al., 2019).
As previously reported in Norman et al. (2004), Dall’s porpoise strandings were more commonly reported in Washington compared to Oregon. The relative proportion of strandings in inland Washington waters for this species decreased, which is explained by an overall decrease in the Dall’s porpoise population abundance in this region, while a simultaneous increase in harbor porpoise abundance occurred (Evenson et al., 2016). Shifts in prey abundance or distribution may help explain this shift in Dall’s abundance, and thus strandings, but further investigation is warranted.
Though striped dolphins have a widespread offshore distribution (Mangels and Gerrodette, 1994; Forney et al., 1995), little is known about the seasonality of their distribution and movement to contextualize our finding that fewer cases are reported in the summer compared to the fall and winter. Their abundance may vary from year to year and may be affected by oceanographic conditions, but long-term trends have not been identified (Becker et al., 2012; Barlow, 2016). Striped dolphins are rarely observed during coastwide cetacean surveys in the waters of Washington and Oregon and are thus considered a low abundance species in the region, but may seasonally shift into the area outside of the survey season (i.e., during winter) or due to anomalous warm waters. For example, the species may have undergone a temporary northern range expansion due to warm water incursions from events such as “the Blob” (Becker et al., 2012; Bond et al., 2015; Barlow, 2016). Of the striped dolphins stranded in WA and OR, most had neurological disease and a disproportionate number of them live stranded, suggesting that this increase of strandings may be disease related, with greater representation in Washington compared to prior years (Norman et al., 2004).
No range expansions were noted for the other species of cetaceans in this study; however, information on spatial and seasonal population trends are incomplete or lacking for many species (Carretta et al., 2020). Strandings of some species showed seasonal trends that likely reflect seasonal distribution, but these patterns may also be due to increased summer stranding response effort and public interest. On the other hand, the strandings of some species might not show seasonal trends because the species is not heavily distributed in the Pacific Northwest and therefore has a very small sample size, such as bottlenose dolphins (Tursiops truncatus) or sei (Balaenoptera borealis) and Bryde’s (Balaenoptera edeni) whales, all of which stranded in low numbers in this study (Table 1).
Oceanographic Variability
Though the power to detect an effect of oceanographic conditions on strandings for some species is relatively low due to small sample sizes, it is evident that a suite of environmental conditions may explain some of the variability in monthly stranding cases, particularly for harbor porpoises. The relationship between strandings and localized and basin-scale oceanographic conditions is likely different for each species due to the life history characteristics and population distributions within the region for each species (Salvadeo et al., 2010; Becker et al., 2019). Strandings for the five focal species in this analysis are likely affected by local and broad-scale conditions such as SST anomalies, wind variability, chlorophyll concentration (as a proxy for primary production), and the PDO and MEI through several mechanisms such as changes in geographical range, proximity to shore at time of death (Williams et al., 2011; Carretta et al., 2015), age structure, physiological health, and/or the availability of prey species (Cavole et al., 2016; Becker et al., 2019).
The degree to which changing ocean conditions affects cetacean health depends greatly on life history traits and a species’ ability to shift its distribution. Anomalous temperatures may affect cold-water species in the northern Pacific Ocean such as harbor and Dall’s porpoise (MacLeod, 2009) and encourage range shifts with resulting changes in stranding patterns. However, range shift options may be limited for resident communities such as Southern Resident killer whales, whose range is shared and potentially restricted by Northern Resident killer whales (Bigg, 1982). Those species that remain in warming waters may experience adverse outcomes such as changes in prey availability or increased potential exposure to anthropogenic contaminants, biotoxins, or infectious diseases that could impact fecundity and/or survival (Cavole et al., 2016).
The results of the GAMs suggest that after accounting for the monthly pattern in strandings, harbor porpoise cases were negatively correlated with lagged mixed layer depth and chlorophyll. Without a more in-depth examination of harbor porpoise body condition and the complex factors that affect the spatio-temporal availability of specific harbor porpoise prey items, for which data are not readily or consistently available, it is challenging to link these indices to immediate foraging conditions. However, this finding could indicate that conditions might be better after periods of higher chlorophyll (as a proxy for primary production) that potentially contributes to greater availability of biomass in the food web. It is important to remember that changes in biophysical features (such as temperature and chlorophyll) will not always induce similar ecosystem-level changes that reverberate up through the food web to ultimately affect cetacean health and distribution (Van der Putten et al., 2010). Additionally, certain environmental conditions such as increased wind velocity and storminess or heightened upwelling could affect carcass drift and/or the probability of observing stranded individuals (Moore et al., 2020). It is therefore difficult to use these results to predict how the ecosystem and its top predators will respond under future conditions or perturbations. It is also important to note that as some of these species increase toward local carrying capacities, population dynamics may become more sensitive to changes in environmental conditions where habitat resources may or may not become a limiting factor.
For gray whales, stranding cases were negatively correlated with chlorophyll concentration and positively correlated with SST and lagged PDO. As discussed earlier, however, gray whale mortality may more be a function of factors outside Oregon and Washington, where the inclusion of environmental variables improved model fit less than it did for the other species. The prominence of MEI as a significant explanatory variable for annual-level strandings reaffirms previous evidence for the connection between El Niño conditions and the elevated strandings during the 1999-2000 UME (Le Boeuf et al., 2000). To further examine the relationship between strandings and environmental conditions for this species, it could be beneficial to include abundance estimates and Arctic Sea ice coverage as model covariates to account for additional interannual variability in the number of stranding cases (Perryman et al., 2002, 2020). For example, in endangered western Pacific gray whales, sea ice coverage duration was examined in relation to foraging duration and growth rates. Approximately 77% of the variation in calf survival (range = 10–80%), was associated with feeding season duration while the mother was pregnant, leading to poor body condition (Gailey et al., 2020). Their results inferred that shorter foraging seasons led to decreased energy through limiting the number of foraging days. Presumably these individuals would not thrive and thus become more likely to die and strand. Similar to gray whales, humpback whale strandings also exhibited a relationship with lagged SST and chlorophyll concentration. The stronger correlation between these indices and strandings at the annual level likely suggests that other factors not robustly examined here contribute to fine-scale spatio-temporal patterns in strandings for highly migratory species.
Striped dolphin strandings exhibited a negative correlation with chlorophyll concentration and lagged SST. The latter makes particular sense given that striped dolphins frequent convergence zones and prefer warmer water (Archer, 2018). Strandings might therefore be more prevalent if individuals extended their range further into the Pacific Northwest in years and seasons with warmer conditions, making it more likely to see increased stranding cases. The positive correlation between striped dolphin strandings and sea surface height could be due to the fact that stronger currents might bring individuals onshore, but also could be due to the fact that higher sea surface height can co-occur with deeper thermoclines that reduce nutrient availability for uptake by organisms into the food web and ultimately may affect forage fish (Wilson and Adamec, 2001).
Dall’s porpoise strandings decreased over the study period and were positively correlated with SST and exhibited a non-linear relationship with lagged PDO. This pattern could be due to a temperature-induced spatio-temporal mismatch with their primary prey species or some other trophic cascade, ecological cue, or physiological sensitivity. While increased temperatures have been shown to coincide with increased strandings of marine mammal species in other areas around the world (Cavole et al., 2016), the mechanism in this case needs further investigation.
In general, notable changes in stranding patterns were observed around the years 2010–2012 for harbor and Dall’s porpoises, striped dolphins, humpback whales, and Pacific white-sided dolphins (Sagmatias obliquidens). The stranding trend for these species either increased or decreased around these years, during which La Niña events of a strong (2010–2011) and moderate (2011–2012) intensity occurred, coinciding with a cool phase PDO that resulted in cooler, more productive waters in the eastern tropical Pacific Ocean (NOAA Climate, 2019). Furthermore, 2012 also featured elevated strandings of Guadalupe fur seals (Arctocephalus townsendi) and sea turtles in Oregon.
The presence of the large, anomalously warm water mass (“the Blob”) that developed in 2013 and persisted through 2016 (Cavole et al., 2016; Gentemann et al., 2017; Peterson et al., 2017), resulted in a reduction in phytoplankton availability, which, in combination with elevated SST, caused significant alterations in zooplankton and marine invertebrate abundance and diversity along the United States West coast (Cavole et al., 2016; Peterson et al., 2017). Furthermore, decreased upwelling throughout the southern California Current led to decreased primary production, which likely reverberated throughout oceanic food webs to affect prey availability for top predators (Leising et al., 2015). Stranding trends were increasing over the 2014–2016 warm water event for harbor porpoise, striped dolphins, and humpback whales, with harbor porpoise stranding numbers in the inland waters starting to decrease around 2014 (Figure 2). The trend of decreasing stranded harbor porpoises starting in 2014 may have been due to the fact that at least one-third of total harbor porpoise strandings in the Pacific Northwest were represented by cases reported in Washington’s inland waters, where the effects of “the Blob” might have differed from those effects on the outer coast. Higher trophic level species such as seabirds, salmon, and marine mammals likely experienced poor feeding conditions during “the Blob” due to alterations in the lower trophic levels of the food web, such as lower lipid content of copepods and the forage fishes that depend on them, changing the overall composition of the marine food web within the California Current ecosystem (Peterson et al., 2017). Furthermore, habitat compression likely forced a larger portion of the regional humpback whale population to remain closer to shore, increasing the probability that a carcass would drift to shore where it would be more easily detected for a stranding report (Santora et al., 2020). Since dissipation of the warm water “Blob”, stranding numbers have continued to increase for striped dolphins and humpback whales, with other factors likely contributing to this increase such as population growth (Calambokidis et al., 2009, 2017) or changing prey availability.
Future Directions
The results of these analyses illustrate possible correlations between stranding cases and environmental variability for five focal species, though enhancements could be made to solidify our ability to make inferences about the specific mechanisms underlying these relationships. This is particularly true given that environmental conditions could be affecting the probability an animal washes onshore (Moore et al., 2020) rather than health and survival. First, including more direct measures of prey availability would clarify whether and to what degree physical oceanographic features themselves act on the health of individuals, or whether it is through bottom-up forcing that causes changes in the distribution and quality of preferred prey. Unfortunately, few reliable metrics for forage fish species abundance and biomass exist, and estimates that are available are generally from a discrete time and place, likely not reflecting prey availability over broad spatio-temporal scales corresponding with monthly stranding data. However, research aiming to model the synergistic effects of climate variability on food webs and fisheries have predicted that fisheries landings for many species could decline in response to the cumulative effects of shifting species distributions and the biophysical changes that have been observed (Brander, 2009; Ainsworth et al., 2011). Second, a state-space modeling framework could distinguish uncertainty in the ecological versus observation processes by estimating changes in the detection of stranded cetaceans, though measuring stranding response effort over time and space is challenging. Lastly, this project only considered the effects of oceanographic conditions in real-time and up to a three-month lag time, when realistically it is likely an accumulation of effects over several months or even years that ultimately creates situations where individuals may be more or less likely to become sick or malnourished, to the point of stranding onshore. Stochastic antecedent modeling and examining threshold effects (Large et al., 2013; Samhouri et al., 2017) could be future avenues of research that could improve our understanding of the effects of environmental variability by identifying and distinguishing between the strength, length, and pattern of the effect of oceanographic covariates.
Despite the uncertainties inherent in studying stranding data and environmental variability, particularly for migratory species like gray whales, our results do suggest that there are connections between stranding cases and oceanographic conditions for cetaceans, which has implications for future long-term climatic changes in the study area, the broader United States West coast, and around the world. Understanding the many ways that cetaceans respond to changes in the marine environment can improve our knowledge about wildlife health and can assist stranding network responders and natural resource managers. Ultimately, the global, regional, and local impacts of climate variability on marine mammal species are the sum of processes that act on individual animals. Each species possesses particular characteristics that govern its resilience to ecosystem dynamics. Studying correlations between marine mammal strandings and oceanographic conditions can improve our understanding of the impacts of climate change on wildlife health and can provide more specific information on the geographic range and distribution of wildlife diseases and anthropogenic impacts along the coast.
Conclusion
Cetacean strandings have increased in recent decades, likely reflecting a perpetually shifting combination of changes in population abundance, distribution, health, anthropogenic stressors, stranding response effort and capacity, and natural and climate-change induced variability in oceanographic conditions. Monitoring these long-term patterns can help disentangle the effects of increasing population size, range expansions, and periods of poorer health, as increased strandings do not necessarily signal worsening population status and in some cases could in fact suggest that a population was nearing carrying capacity. Cetacean strandings over the study period reveal seasonal and interannual variability that likely reflect the spatio-temporal distribution and abundance based on how a given species or population utilizes habitat within the Pacific Northwest. Despite the limitations of not incorporating direct information about cetacean abundance trends or forage fish availability, our study reveals notable correlations between certain environmental features and patterns in stranding cases for several species. This study can provide helpful insights into cetacean health in revealing how certain species may respond to ongoing environmental change throughout the region. This information is useful for researchers, federal agencies, and stranding responders studying the current and future health and recovery of these and other cetacean populations in ecosystems that are subject to human impacts and are exhibiting signs of environmental change.
Data Availability Statement
The raw data supporting the conclusions of this article will be made available by the authors, without undue reservation.
Author Contributions
AW conducted the statistical analysis and conceived of the project scope and design with SN. AW and SN were responsible for the drafting of the text and received extensive comment and review by all other authors. JLH, DL, DAD, DND, JR, JC, MH, JG, SJ, JO, JS, AA, MK, SB, SD, BC, SR, JH, VS, AE, CK, KW, and TB provided substantial data that was cleaned, organized, geo-referenced, reviewed, and collated from their respective stranding networks. All authors contributed to the article and approved the submitted version.
Conflict of Interest
The authors declare that the research was conducted in the absence of any commercial or financial relationships that could be construed as a potential conflict of interest.
Publisher’s Note
All claims expressed in this article are solely those of the authors and do not necessarily represent those of their affiliated organizations, or those of the publisher, the editors and the reviewers. Any product that may be evaluated in this article, or claim that may be made by its manufacturer, is not guaranteed or endorsed by the publisher.
Acknowledgments
This research would not be possible without the dedication and effort of all stranding response network members in the Pacific Northwest, including Cascadia Research Collective, Central Puget Sound Marine Mammal Stranding Network, Dungeness National Wildlife Refuge & Protection Island, Feiro Marine Life Center, Makah Tribe, Marine Animal Rescue Center, MaST Center Stranding Team, Olympic Coast National Marine Sanctuary, Oregon State University Marine Mammal Institute, Port Townsend Marine Science Center, Portland State University, San Juan County Marine Mammal Stranding Network, Seal Sitters, Seaside Aquarium, Sno-King Marine Mammal Response, The Whale Museum, Vashon Hydrophone Project, Washington Department of Fish and Wildlife Marine Mammal Investigations, and Whatcom Marine Mammal Stranding Network. Multiple stranding networks were recipients of United States Federal grants through the John H. Prescott Marine Mammal Rescue Assistance Grant Program, which have supported stranding response. Lauren De Maio with the NOAA Fisheries, Protected Resources Division West Coast Region assisted with stranding database queries. Open access publication fees were provided by the SeaDoc Society, a program of the University California Davis Karen C. Drayer Wildlife Health Center.
References
Ainsworth, C. H., Samhouri, J. F., Busch, D. S., Cheung, W. W. L., Dunne, J., and Oakey, T. A. (2011). Potential impacts of climate change on Northeast Pacific marine foodwebs and fisheries. ICES J. Mar. Sci. 68, 1217–1229. doi: 10.1093/icesjms/fsr043
Akaike, H. (1973). “Information theory and an extension of maximum likelihood principle,” in Proceedings of the Second International Symposium on Information Theory, eds B. N. Petrov and F. Csaki (Budapest: Akademiai Kiado), 267–281.
Archer, F. I. (2018). “Striped dolphin Stenella coeruleoalba,” in Encyclopedia of Marine Mammals, 3rd Edn, eds B. Würsig, J. G. M. Thewissen, and K. Kovacs (London: Academic Press), 954–956. doi: 10.1016/B978-0-12-804327-1.00251-X
Auth, T. D., Daly, E. A., Brodeur, R. D., and Fisher, J. L. (2017). Phenological and distributional shifts in ichthyoplankton associated with recent warming in the northeast Pacific Ocean. Glob. Change Biol. 24, 259–272. doi: 10.1111/gcb.13872
Ballance, L., Pitman, R. L., and Fiedler, P. C. (2006). Oceanographic influences on seabirds and cetaceans of the eastern tropical Pacific: a review. Prog. Oceanogr. 69, 360–390. doi: 10.1016/j.pocean.2006.03.013
Barlow, J. (2016). Cetacean Abundance in the California Current Estimated from Ship-Based Line-Transect Surveys in 1991-2014, Administrative Report LJ-16-01. La Jolla, CA: U.S. Department of Commerce, National Marine Fisheries Service, Southwest Fisheries Science Center.
Barlow, J., Calambokidis, J., Falcone, E. A., Baker, C. S., Burdin, A. M., Clapham, P. J., et al. (2011). Humpback whale abundance in the North Pacific estimated by photographic capture-recapture with bias correction from simulation studies. Mar. Mamm. Sci. 27, 793–818. doi: 10.1111/j.1748-7692.2010.00444.x
Beamish, R. J., Noakes, D. J., McFarlane, G. A., Klyashtorin, L., Ivanov, V. V., and Kurashov, V. (1999). The regime concept and natural trends in the production of Pacific salmon. Can. J. Fish. Aquat. Sci. 56, 516–526. doi: 10.1139/f98-200
Becker, E. A., Foley, D. G., Forney, K. A., Barlow, J., Redfern, J. V., and Gentemann, C. L. (2012). Forecasting cetacean abundance patterns to enhance management decisions. Endanger. Species Res. 16, 97–112. doi: 10.3354/esr00390
Becker, E. A., Forney, K. A., Redfern, J. V., Barlow, J., Jacox, M. G., Roberts, J. J., et al. (2019). Predicting cetacean abundance and distribution in a changing climate. Biodivers. Res. 25, 626–643. doi: 10.1111/ddi.12867
Berini, C. R., Kracker, L. M., and McFee, W. E. (2015). Modeling Pygmy Sperm Whale (Kogia breviceps, De Blainville 1838) Strandings Along the Southeast Coast of the United States from 1992 to 2006 in Relation to Environmental Factors. NOAA Technical Memorandum NOS NCCOS 203. Charleston, SC: NOAA Technical Memorandum, 44.
Bigg, M. A. (1982). An assessment of killer whale (Orcinus orca) stocks off Vancouver Island, British Columbia. Int. Whaling Comm. Rep. Committee 32, 655–666.
Bogomolni, A. L., Pugliares, K. R., Sharp, S. M., Patchett, K., Harry, C. T., LaRocque, J. M., et al. (2010). Mortality trends of stranded marine mammals on Cape Cod and southeastern Massachusetts, USA, 2000 to 2006. Dis. Aquat. Org. 88, 143–155. doi: 10.3354/dao02146
Bond, N. A., Cronin, M. F., Freeland, H., and Mantua, N. J. (2015). Causes and impacts of the 2014 warm anomaly in the NE Pacific. Geophys. Res. Lett. 42, 3414–3420. doi: 10.1002/2015GL063306
Bossart, G. D. (2011). Marine mammals as sentinel species for oceans and human health. Vet. Pathol. 48, 676–690. doi: 10.1177/0300985810388525
Brander, K. (2009). Impacts of climate change on fisheries. J. Mar. Syst. 79, 389–402. doi: 10.1016/j.jmarsys.2008.12.015
Calambokidis, J., and Barlow, J. (2020). Updated Abundance Estimates for Blue and Humpback Whales Along the U.S. West Coast Using Data Through 2018. U.S. Dep. Commer., NOAA Technical Memorandum NMFS-SWFSC-634. 20. Washington, DC: NOAA. doi: 10.25923/zrth-8n96
Calambokidis, J., Barlow, J., Flynn, K., Dobson, E., and Steiger, G. H. (2017). Update on Abundance, Trends, and Migrations of Humpback Whales Along the US West Coast. International Whaling Commission Paper SC/A17/NP/13. Cambridge: International Whaling Commission, 17.
Calambokidis, J., Darling, J. D., Deecke, V., Gearin, P., Gosho, M., Megill, W., et al. (2002). Abundance, range and movements of a feeding aggregation of gray whales (Eschrichtius robustus) from California to southeastern Alaska in 1998. J. Cetacean Res. Manag. 4, 267–276. doi: 10.1016/b978-0-08-092372-7.50018-2
Calambokidis, J., Falcone, E., Douglas, A., Schlender, L., and Huggins, J. (2009). Photographic Identification of Humpback and Blue Whales off the U.S. West Coast: Results and Updated Abundance Estimates From 2008 Field Season. Final Report for Contract AB133F08SE2786 from Southwest Fisheries Science Center. 18. Available online at: https://www.cascadiaresearch.org/publications/photographic-identification-humpback-and-blue-whales-us-west-coast-results-and-updated doi: 10.21236/ADA539301 (accessed May 14, 2018).
Calambokidis, J., Steiger, G. H., Curtice, C., Harrison, J., Ferguson, M. C., Becker, E., et al. (2015). Biologically important areas for selected cetaceans within U.S. waters – west coast region. Aquat. Mamm. 41, 39–53. doi: 10.1578/AM.41.1.2015.39
Carretta, J. V., Danil, K., Chivers, S. J., Weller, D. W., Janiger, D. S., Berman-Kowalewski, M., et al. (2015). Recovery rates of bottlenose dolphin (Tursiops truncatus) carcasses estimated from stranding and survival rate data. Mar. Mamm. Sci. 32, 349–362. doi: 10.1111/mms.12264
Carretta, J. V., Forney, K. A., Oleson, E. M., Weller, D. W., Lang, A. R., Baker, J., et al. (2020). U.S. Pacific Marine Mammal Stock Assessments: 2019, U.S. Department of Commerce, NOAA Technical Memorandum NMFS-SWFSC-629. Available online at: https://media.fisheries.noaa.gov/dam-migration/2019_sars_pacific_508.pdf
Cavole, L. M., Demko, A. M., Diner, R. E., Giddings, A., Koester, I., Pagniello, C. M. L. S., et al. (2016). Biological impacts of the 2013–2015 warm-water anomaly in the Northeast Pacific: winners, losers, and the future. Oceanography 29, 273–285. doi: 10.5670/oceanog.2016.32
Chavez, F. P., Ryan, J., Lluch-Cota, S. E., and Ñiquen, M. (2003). From anchovies to sardines and back: multidecadal change in the Pacific Ocean. Science 299, 217–221. doi: 10.1126/science.1075880
Di Lorenzo, E., and Mantua, N. (2016). Multi-year persistence of the 2014/15 North Pacific marine heatwave. Nat. Clim. Change 6, 1042–1047. doi: 10.1038/NCLIMATE3082
Di Lorenzo, E., Schneider, N., Cobb, K. M., Chhak, K., Franks, P. J. S., Miller, A. J., et al. (2008). North Pacific Gyre Oscillation links ocean climate and ecosystem change. Geophys. Res. Lett. 35:L08607. doi: 10.1029/2007GL032838
Evans, K., Thresher, R., Warneke, R. M., Bradshaw, C. J. A., Pook, M., Thiele, D., et al. (2005). Periodic variability in cetacean strandings: links to large-scale climate events. Biol. Lett. 1, 147–150. doi: 10.1098/rsbl.2005.0313
Evans, P. G. H., and Bjørge, A. (2013). Impacts of climate change on marine mammals. Mar. Clim. Change Impacts Partnersh. Sci. Rev. 2013, 134–148. doi: 10.14465/2013.arc15.134-148
Evans, P. G. H., Pierce, G. J., and Panigada, S. (2010). Climate change and marine mammals. J. Mar. Biol. Assoc. U. K. 90, 1483–1487. doi: 10.1017/S0025315410001815
Evenson, J. R., Anderson, D., Murphie, B. L., Cyra, T. A., and Calambokidis, J. (2016). Disappearance and Return of Harbor Porpoise to Puget Sound: 20 Year Pattern Revealed from Winter Aerial Surveys. Technical Report. Olympia, WA: Washington Department of Fish and Wildlife, Wildlife Program and Cascadia Research Collective, 27.
Forney, K. A., Barlow, J., and Carretta, J. V. (1995). The abundance of cetaceans in California waters. Part II: aerial surveys in winter and spring of 1991 and 1992. Fish. Bull. 93, 15–26.
Forney, K. A., Moore, J. E., Barlow, J., Carretta, J. V., and Benson, S. R. (2020). A multidecadal Bayesian trend analysis of harbor porpoise (Phocoena phocoena) populations off California relative to past fishery bycatch. Mar. Mamm. Sci. Early View 37, 546–560. doi: 10.1111/mms.12764
Francis, R. C., Hare, S. R., Hollowed, A. B., and Wooster, W. S. (2003). Effects of interdecadal climate variability on the oceanic ecosystems of the NE Pacific. Fish. Oceanogr. 7, 1–21. doi: 10.1046/j.1365-2419.1998.00052.x
Gailey, G., Sychenko, O., Tyurneva, O., Yakovlev, Y., Vertyankin, V., van der Wolf, P., et al. (2020). Effects of sea ice on growth rates of an endangered population of gray whales. Sci. Rep. 10:1553. doi: 10.1038/s41598-020-58435-3
Gatrell, A. C., Bailey, T. C., Diggle, P. J., and Rowlingson, B. S. (1996). Spatial point pattern analysis and its application in geographical epidemiology. Trans. Inst. Br. Geogr. 21, 256–274. doi: 10.2307/622936
Gentemann, C. L., Fewings, M. R., and García-Reyes, M. (2017). Satellite sea surface temperatures along the west coast of the United States during the 2014–2016 northeast Pacific marine heat wave. Geophys. Res. Lett. 44, 312–319. doi: 10.1002/2016gl071039
Guazzo, R. A., Schulman-Janiger, A., Smith, M. H., Barlow, J., D’Spain, G. L., Rimington, D. B., et al. (2019). Gray whale migration patterns through the Southern California Bight from multi-year visual and acoustic monitoring. Mar. Ecol. Prog. Ser. 625, 181–203. doi: 10.3354/meps12989
Gulland, F. M. D., and Hall, A. J. (2007). Is marine mammal health deteriorating? Trends in the global reporting of marine mammal disease. EcoHealth 4, 135–150. doi: 10.1007/s10393-007-0097-1
Gulland, F. M. D., Pérez-Cortés, M. H., Urbán, R. J., Rojas-Bracho, L., Ylitalo, G., Weir, J., et al. (2005). Eastern North Pacific Gray Whale (Eschrichtius robustus) Unusual Mortality Event, 1999-2000. U.S. Dep. Commer., NOAA Technical Memorandum NMFS-AFSC-150. Washington, DC: NOAA, 33.
Hanson, M. B. (2007). “Seasonal movements and habitat use of Dall’s and harbor porpoises in the inland and coastal waters of Washington state as determined by radiotelemetry,” in Report of the National Marine Fisheries Service Workshop on Advancing Electronic Tag Technologies and Their Use in Stock Assessments. U.S. Dept. Commerce, NOAA Technical Memorandum NMFSF/SPO-82, eds P. Sheridan, J. W. Ferguson, and S. L. Downing (Washington, DC: NOAA), 53–54.
Hare, S. R., Mantua, N. J., and Francis, R. C. (1999). Inverse production regimes: Alaskan and West Coast Salmon. Fisheries 24, 6–14. doi: 10.1577/1548-8446(1999)024<0006:IPR>2.0.CO;2
Hastie, T., and Tibshirani, R. (1990) Exploring the nature of covariate effects in the proportional hazards model. Biometrics 46, 1005–1016. doi: 10.2307/2532444
Hickey, B. M., and Banas, N. S. (2003). Oceanography of the U.S. Pacific Northwest coastal ocean and estuaries with application to coastal ecology. Estuaries 26, 1010–1031. doi: 10.1007/BF02803360
Huggins, J. L., Oliver, J., Lambourn, D. M., Calambokidis, J., Diehl, B., and Jeffries, S. (2015a). Dedicated beach surveys along the central Washington coast reveal a high proportion of unreported marine mammal strandings. Mar. Mamm. Sci. 31, 782–789. doi: 10.1111/mms.12184
Huggins, J. L., Raverty, S. A., Norman, S. A., Calambokidis, J., Gaydos, J. K., Duffield, D. A., et al. (2015b). Increased harbor porpoise mortality in the Pacific Northwest, USA: understanding when higher levels may be normal. Dis. Aquat. Org. 115, 93–102. doi: 10.3354/dao02887
IPCC (2014). “Climate change 2014: synthesis report. Contribution of working groups I, II and III to the fifth assessment report of the Intergovernmental Panel on Climate Change,” in Report of the Intergovernmental Panel on Climate Change, eds Core Writing Team, R. K. Pachauri and L. A. Meyer (Geneva: IPCC).
Jacox, M. G., Hazen, E. L., Zaba, K. D., Rudnick, D. L., Edwards, C. A., Moore, A. M., et al. (2016). Impacts of the 2015–2016 El Niño on the California Current System: early assessment and comparison to past events. Geophys. Res. Lett. 43, 7072–7080. doi: 10.1002/2016GL069716
Jefferson, T. A., Smultea, M. A., Courbis, S. S., and Campbell, G. S. (2016). Harbor porpoise (Phocoena phocoena) recovery in the inland waters of Washington: estimates of density and abundance from aerial surveys, 2013–2015. Can. J. Zool. 94, 505–515. doi: 10.1139/cjz-2015-0236
Johnston, D. W., Bowers, M. T., Friedlaender, A. S., and Lavigne, D. M. (2012). The effects of climate change on harp seals (Pagophilus groenlandicus). PLoS One 7:e29158. doi: 10.1371/journal.pone.0029158
Keledjian, A., and Mesnick, S. L. (2013). The impacts of El Niño conditions on California sea lion (Zalophus californianus) fisheries interactions: predicting spatial and temporal hotspots along the California coast. Aquat. Mamm. 39, 221–232. doi: 10.1578/AM.39.3.2013.221
Laidre, K. L., Stirling, I., Lowry, L., Wiig, Ø., Heide-Jørgensen, M. P., and Ferguson, S. H. (2008). Quantifying the sensitivity of Arctic marine mammals to climate-induced habitat change. Ecol. Appl. 18(Suppl), S97–S125. doi: 10.1890/06-0546.1
Large, S. I., Fay, G., Friedland, K. D., and Link, J. S. (2013). Defining trends and thresholds in responses of ecological indicators to fishing and environmental pressures. ICES J. Mar. Sci. 70, 755–767. doi: 10.1093/icesjms/fst067
Lavaniegos, B. E., and Ohman, M. D. (2007). Coherence of long-term variations of zooplankton in two sectors of the California Current System. Prog. Oceanogr. 75, 42–69. doi: 10.1016/j.pocean.2007.07.002
Le Boeuf, B. U., Pérez-Cortés, M. H., Urbán, R. J., Mate, B. R., and Ollervides, U. F. (2000). High gray whale mortality and low recruitment in 1999: potential causes and implications. J. Cetacean Res. Manag. 2, 85–99.
Leising, A. W., Schroeder, I. D., Bograd, S. J., Abell, J., Durazo, R., Gaxiola-Castro, G., et al. (2015). State of the California Current 2014–15: impacts of the warm-water “Blob.”. CalCOFI Rep. 56, 31–68.
MacLeod, C. D. (2009). Global climate change, range changes and potential implications for the conservation of marine cetaceans, a review and synthesis. Endanger. Species Res. 7, 125–136. doi: 10.3354/esr00197
MacLeod, C. D., Bannon, S. M., Pierce, G. J., Schweder, C., Learmonth, J. A., Herman, J. S., et al. (2005). Climate change and the cetacean community of north-west Scotland. Biol. Conserv. 124, 477–483. doi: 10.1016/j.biocon.2005.02.004
Mangels, K. F., and Gerrodette, T. (1994). Report of Cetacean Sightings During a Marine Mammal Survey in the Eastern Pacific Ocean and the Gulf of California Aboard the NOAA Ships McArthur and David Starr Jordan July 28 – November 6, 1993. U.S. Dep. Commer., NOAA Technical Memorandum NMFS-SWFSC-221. Washington, DC: NOAA, 88.
Mantua, N. J., and Hare, S. R. (2002). The Pacific decadal oscillation. J. Oceanogr. 58, 35–44. doi: 10.1023/A:1015820616384
Mauger, G. S., Casola, J. H., Morgan, H. A., Strauch, R. L., Jones, B., Curry, B., et al. (2015). State of Knowledge: Climate Change in Puget Sound. Report prepared for the Puget Sound Partnership and the National Oceanic and Atmospheric Administration. Climate Impacts Group, University of Washington, Seattle. Available online at: https://cig.uw.edu/resources/special-reports/ps-sok/ (accessed August 2, 2018).
McCabe, R. M., Hickey, B. M., Kudela, R. M., Lefebvre, K. A., Adams, N. G., Bill, B. D., et al. (2016). An unprecedented coastwide toxic algal bloom linked to anomalous ocean conditions. Geophys. Res. Lett. 43, 10366–10376. doi: 10.1002/2016GL070023
McGowan, J., Cayan, D., and Dorman, L. (1998). Climate-ocean variability and ecosystem response in the Northeast Pacific. Science 281, 210–217. doi: 10.1126/science.281.5374.210
Mertz, F., Mulet, S., Verbrugge, N., and Nardelli, B. B. (2019). For Global Ocean Multi Observation products MULTIOBS_GLO_PHY_REP_015_002 23 (accessed February, 2021).
Moore, M. J., Mitchell, G. H., Rowles, T. K., and Early, G. (2020). Dead cetacean? beach, bloat, float, sink. Front. Mar. Sci. 7:333. doi: 10.3389/fmars.2020.00333
Moore, S. E. (2008). Marine mammals as ecosystem sentinels. J. Mammal. 89, 534–540. doi: 10.1644/07-MAMM-S-312R1.1
Moore, S. E., Urban, R. J., Perryman, W. L., Gulland, F., Perez-Cortes, M. H., Wade, P. R., et al. (2001). Are gray whales hitting ‘K’ hard? Mar. Mamm. Sci. 17, 954–958. doi: 10.1111/j.1748-7692.2001.tb01310.x
Mote, P. W., and Salathé, E. P. Jr. (2010). Future climate in the Pacific Northwest. Clim. Change 102, 29–50. doi: 10.1007/s10584-010-9848-z
Newton, J. A. (1995). “El Niño weather conditions reflected in Puget Sound temperatures and salinities,” in Proceedings of Puget Sound Research ’95, Vol. 2, Bellevue, Washington, ed E. Robichaud (Olympia, WA: Puget Sound Water Quality Authority), 979–991.
NOAA Climate (2019). Climate. Available online at: https://www.noaa.gov/climate (accessed May 23, 2019).
NOAA Fisheries (2021). Available online at: https://www.fisheries.noaa.gov/national/marine-life-distress/2019-2021-gray-whale-unusual-mortality-event-along-west-coast-and (accessed June 1, 2021).
NOAA Integrated Ecosystem Assessment (IEA) (2019). California Integrated Ecosystem. Assessment. Available online at: https://www.integratedecosystemassessment.noaa.gov/regions/california-current-region/index.html
NOAA Southwest Fisheries Science Center Environmental Research Division (SWFSC ERD) (2019). Aqua MODIS Satellite Sensor Data. Available online at: https://coastwatch.noaa.gov/cw_html/OceanColor_NRT_MODIS_Aqua.html
Norman, S. A., Bowlby, C. E., Brancato, M. S., Calambokidis, J., Duffield, D., Gearin, P. J., et al. (2004). Cetacean strandings in Oregon and Washington between 1930 and 2002. J. Cetacean Res. Manag. 6, 87–100.
Norman, S. A., Hanson, M. B., Huggins, J., Lambourn, D., Calambokidis, J., Cottrell, P., et al. (2018). Conception, fetal growth, and calving seasonality of harbor porpoise (Phocoena phocoena) in the Salish Sea waters of Washington, USA, and southern British Columbia, Canada. Can. J. Zool. 96, 566–575. doi: 10.1139/cjz-2017-0155
Oviatt, C., Smith, L., McManu, M. C., and Hyde, K. (2015). Decadal patterns of westerly winds, temperatures, ocean gyre circulations and fish abundance: a review. Climate 3, 833–857. doi: 10.3390/cli3040833
Perryman, W. L., Donahue, M. A., Perkins, P. C., and Reilly, S. B. (2002). Gray whale calf production 1994-2000: are observed fluctuations related to changes in seasonal ice cover? Mar. Mamm. Sci. 18, 121–144. doi: 10.1111/j.1748-7692.2002.tb01023.x
Perryman, W. L., Joyce, T., Weller, D. W., and Durban, J. W. (2020). Environmental factors influencing eastern North Pacific gray whale calf production 1994–2016. Mar. Mamm. Sci. 37, 1–5. doi: 10.1111/mms.12755
Peterson, W. T., and Keister, J. E. (2003). Interannual variability in copepod community composition at a coastal station in the northern California Current: a multivariate approach. Deep Sea Res. Part II Top. Stud. Oceanogr. 50, 2499–2517. doi: 10.1016/S0967-0645(03)00130-9
Peterson, W. T., Fisher, J. L., Strub, P. T., Du, X., Risien, C., Peterson, J., et al. (2017). The pelagic ecosystem in the Northern California Current off Oregon during the 2014–2016 warm anomalies within the context of the past 20 years. J. Geophys. Res. Oceans 122, 7267–7290. doi: 10.1002/2017JC012952
Pierce, G. J., Santos, M. B., Smeenk, C., Saveliev, A., and Zuur, A. F. (2007). Historical trends in the incidence of strandings of sperm whales (Physeter macrocephalus) on North Sea coasts: an association with positive temperature anomalies. Fish. Res. 87, 219–228. doi: 10.1016/j.fishres.2007.06.001
Pike, G. C. (1962). Migration and feeding of the gray whale (Eschrichtius gibbosus). J. Fish. Res. Board Canada 19, 815–838. doi: 10.1139/f62-051
Pikesley, S. K., Witt, M. J., Hardy, T., Loveridge, J., Loveridge, J., Williams, R., et al. (2012). Cetacean sightings and strandings: evidence for spatial and temporal trends? J. Mar. Biol. Assoc. U. K. 92, 1809–1820. doi: 10.1017/S0025315411000464
Pitchford, J. L., Garcia, M., Pulis, E. E., Ambert, A. M., Heaton, A. J., and Solangi, M. (2018). Gauging the influence of increased search effort on reporting rates of bottlenose dolphin (Tursiops truncatus) strandings following the Deepwater Horizon oil spill. PLoS One 13:e0199214. doi: 10.1371/journal.pone.0199214
Prado, J. H. F., Mattos, P. H., Silva, K. G., and Secchi, E. R. (2016). Long-term seasonal and interannual patterns of marine mammal strandings in subtropical western south Atlantic. PLoS One 11:e0146339. doi: 10.1371/journal.pone.0146339
R Development Core Team (2019). R: A Language and Environment for Statistical Computing. Vienna: R Foundation for Statistical Computing.
Rice, D. W., and Wolman, A. A. (1971). The Life History and Ecology of the Gray Whale (Eschrichtius rabustus), Special Publication No. 3. Stillwater, OK: The American Society of Mammalogists. doi: 10.5962/bhl.title.39537
Salvadeo, C. J., Lluch-Belda, D., Gómez-Gallardo, A., Urbán-Ramírez, J., and MacLeod, C. D. (2010). Climate change and a poleward shift in the distribution of the Pacific white-sided dolphin in the northeastern Pacific. Endanger. Species Res. 11, 13–19. doi: 10.3354/esr00252
Samhouri, J. F., Andrews, K. S., Fay, G., Harvey, C. J., Hazen, E. L., Hennessey, S. M., et al. (2017). Defining ecosystem thresholds for human activities and environmental pressures in the California Current. Ecosphere 8:e01860. doi: 10.1002/ecs2.1860
Santora, J., Hazen, E., Schroeder, I., Bograd, S., Sakuma, K., and Field, J. (2017). Impacts of ocean climate variability on biodiversity of pelagic forage species in an upwelling ecosystem. Mar. Ecol. Prog. Ser. 580, 205–220. doi: 10.3354/meps12278
Santora, J., Mantua, N. J., Schroeder, I. D., Field, J. C., Hazen, E. L., Brograd, S. J., et al. (2020). Habitat compression and ecosystem shifts as potential links between marine heatwave and record whale entanglements. Nat. Commun. 11:536. doi: 10.1038/s41467-019-14215-w
Segawa, T., and Kemper, C. (2015). Cetacean strandings in South Australia (1881–2008). Aust. Mammal. 37, 51–66. doi: 10.1071/AM14029
Smultea, M. A., Jefferson, T. A., Courbis, S., Campbell, G., and Hopkins, J. (2015). Marine Mammal Aerial Surveys Conducted in the Inland Puget Sound Waters of Washington, Summer 2013-Spring 2015. Draft Final Report. Prepared for Commander, U.S. Pacific Fleet. San Diego, CA: Naval Facilities Engineering Command Pacific, Pearl Harbor.
Soulen, B. K., Cammen, K., Schultz, T. F., and Johnston, D. W. (2013). Factors affecting harp seal (Pagophilus groenlandicus) strandings in the northwest Atlantic. PLoS One 8:e68779. doi: 10.1371/journal.pone.0068779
Sprogis, K. R., Christiansen, F., Wandres, M., and Bejder, L. (2017). El Niño Southern Oscillation influences the abundance and movements of a marine top predator in coastal waters. Glob. Change Biol. 24, 1085–1096. doi: 10.1111/gcb.13892
Tian, Y., Akamine, T., and Suda, M. (2004). Modeling the influence of oceanic-climatic changeson the dynamics of Pacific saury in the northwestern Pacific using a life cycle model. Fish. Oceanogr. 13(Suppl. 1), 125–137. doi: 10.1111/j.1365-2419.2004.00314.x
Truchon, M.-H., Measures, L., L’Hérault, V., Brêthes, J.-C., Galbraith, P. S., Harvey, M., et al. (2013). Marine mammal strandings and environmental changes: a 15-year study in the St. Lawrence ecosystem. PLoS One 8:e59311. doi: 10.1371/journal.pone.0059311
Van der Putten, W. H., Macel, M., and Visser, M. E. (2010). Predicting species distribution and abundance responses to climate change: why it is essential to include biotic interactions across trophic levels. Philos. Trans. R. Soc. B 365, 2025–2034. doi: 10.1098/rstb.2010.0037
Warlick, A. J., Duffield, D. A., Lambourn, D. M., Jeffries, S. J., Rice, J. M., Gaydos, J. K., et al. (2018). Spatio-temporal characterization of pinniped strandings and human interaction cases in the Pacific Northwest, 1991-2016. Aquat. Mamm. 44, 299–318. doi: 10.1578/AM.44.3.2018.299
Washington Department of Fish and Wildlife (2020). Commercial Puget Sound salmon fishery regulations and maps. Available online at: https://wdfw.wa.gov/fishing/commercial/salmon/rules (accessed December 11, 2020).
Williams, R., Gero, S., Bejder, L., Calambokidis, J., Kraus, S. D., Lusseau, D., et al. (2011). Underestimating the damage: interpreting cetacean carcass recoveries in the context of the Deepwater Horizon/BP incident. Conserv. Lett. 4, 228–233. doi: 10.1111/j.1755-263X.2011.00168.x
Wilson, C., and Adamec, D. (2001). Correlations between surface chlorophyll and sea surface height in the tropical Pacific during the 1997-1999 El Niño–Southern Oscillation event. J. Geophys. Res. 106, 31175–31188. doi: 10.1029/2000JC000724
Wolter, K., and Timlin, M. S. (1993). “Monitoring ENSO in COADS with a seasonally adjusted principal component index,” in Proceedings of the 17th Climate Diagnostics Workshop, NOAA/N MC/CAC, NSSL, Oklahoma Climate Survey, CIMMS and the School of Meteorology (Norman, OK: University of Oklahoma).
Keywords: cetacean, marine mammal, harbor porpoise, strandings, oceanographic variability, climate variability, generalized additive model (GAM)
Citation: Warlick AJ, Huggins JL, Lambourn DM, Duffield DA, D’Alessandro DN, Rice JM, Calambokidis J, Hanson MB, Gaydos JK, Jeffries SJ, Olson JK, Scordino JJ, Akmajian AM, Klope M, Berta S, Dubpernell S, Carlson B, Riemer S, Hodder J, Souze V, Elsby A, King C, Wilkinson K, Boothe T and Norman SA (2022) Cetacean Strandings in the US Pacific Northwest 2000–2019 Reveal Potential Linkages to Oceanographic Variability. Front. Mar. Sci. 9:758812. doi: 10.3389/fmars.2022.758812
Received: 15 August 2021; Accepted: 27 January 2022;
Published: 02 March 2022.
Edited by:
Vitor H. Paiva, University of Coimbra, PortugalReviewed by:
Andrew M. Fischer, University of Tasmania, AustraliaWen-Ta Li, Pangolin International Biomedical Consultant Ltd., Taiwan
Helene Peltier, UMS 3462 Systèmes d’Observation pour la Conservation des Mammifères et Oiseaux Marins, France
Lemnuel De Vera Aragones, University of the Philippines Diliman, Philippines
Copyright © 2022 Warlick, Huggins, Lambourn, Duffield, D’Alessandro, Rice, Calambokidis, Hanson, Gaydos, Jeffries, Olson, Scordino, Akmajian, Klope, Berta, Dubpernell, Carlson, Riemer, Hodder, Souze, Elsby, King, Wilkinson, Boothe and Norman. This is an open-access article distributed under the terms of the Creative Commons Attribution License (CC BY). The use, distribution or reproduction in other forums is permitted, provided the original author(s) and the copyright owner(s) are credited and that the original publication in this journal is cited, in accordance with accepted academic practice. No use, distribution or reproduction is permitted which does not comply with these terms.
*Correspondence: Amanda J. Warlick, amandajwarlick@gmail.com