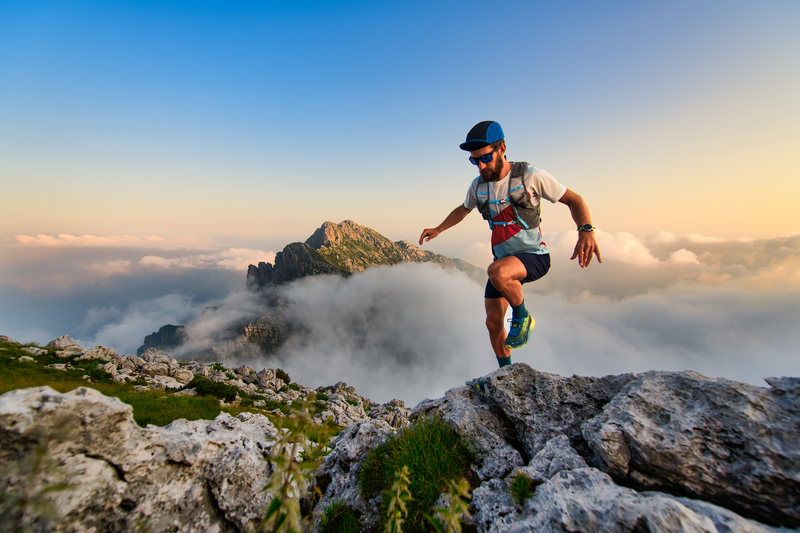
95% of researchers rate our articles as excellent or good
Learn more about the work of our research integrity team to safeguard the quality of each article we publish.
Find out more
ORIGINAL RESEARCH article
Front. Mar. Sci. , 27 April 2022
Sec. Marine Ecosystem Ecology
Volume 9 - 2022 | https://doi.org/10.3389/fmars.2022.640046
Algal blooms over the past years have caused considerable worldwide impacts on marine ecology, aquaculture, recreational activities, and human health. Therefore, there is an urgent need to develop indices for evaluating the nutritional status of seawater as a means of predicting algal blooms. A long-term water quality monitoring dataset from Dapeng Bay, Southern Taiwan, indicated that seawater dissolved oxygen (DO) concentrations and pH were significantly correlated with algal abundance. Using this dataset, we then developed a real-time trophic state index (RTSI) by (1) referring to the seawater nutrient grading system defined by Carlson’s index and (2) incorporating an algorithm based on the relationship between DO, pH, and eutrophication status. The RTSI was superior to contemporary indices in its simplicity, as no complicated nutrient or chlorophyll a (Chl a) measurements were required, and real-time data were displayed on a personal computer. The index is sensitive to changes in seawater quality that will be of aid to managers.
Lagoons are semi-enclosed seawater environments that are generally located between landmasses and sandy beaches (Boynton et al., 1996), thereby serving as a transition zone between land and sea (Tew et al., 2008). The calm and oftentimes nutrient-rich waters of a lagoon make for ideal nursery conditions for a wide variety of commercially important fish and invertebrates (Tew et al., 2008, 2010, 2014; Hsieh et al., 2010) and display a rich ecosystem (Araujo et al., 2015). Given the poor water exchange of lagoons, they are highly sensitive to exogenous inputs (Tew et al., 2010), especially nutrients (Pinckney et al., 2001; Hsieh et al., 2010; Tew et al., 2010, 2014). Nutrient influx results in eutrophication, which can stimulate primary production (Nixon, 1995; Pinckney et al., 2001; Heisler et al., 2008), and consequently jeopardize the ecosystem and the economic activities associated with it such as fisheries and tourism (Andersen et al., 2006). Eutrophication is typically assessed by measuring chlorophyll a (Chl a) levels in the water, though phytoplankton density may also serve as a proxy (Carlson, 1977; Cloern, 2001; Gupta, 2014).
Eutrophication emerging from inland discharge (Paerl and Scott, 2010; Wang et al., 2015) can cause algal blooms (Smith, 2003; Heisler et al., 2008), which can fundamentally alter the ecosystem diversity, and function (Cloern, 2001; Islam and Tanaka, 2004). In the case of harmful algal blooms (HABs) and blue-green algal blooms in freshwater lakes [the latter of which releases toxic microcystins (Metcalf and Codd, 2009)], it can even impact tourism (Smith, 2003) and human health (Ulloa et al., 2017). Since detoxifying bodies of water is costly (Michalak, 2016), it is unfortunate that anthropogenic impacts are on the rise (Anderson et al., 2021). Global climate change and other human-associated factors have been associated with increases in the frequency, distribution, and economic impacts of HABs over the past two decades (Paerl and Paul, 2012; Rigosi et al., 2014; Anderson et al., 2021; Karlson et al., 2021), and management costs have risen commensurately (Ho and Michalak, 2015).
The occurrence of HABs is influenced by the phytoplankton assemblage, nutrient levels, temperature, and other abiotic parameters (Lv et al., 2014; Yang et al., 2016; Zhang et al., 2016). HABs are usually associated with abnormal seawater color, noxious odors, drops in dissolved oxygen (DO) content, death of aquatic organisms, and sometimes shellfish poisoning (Pitcher and Probyn, 2011; Reich et al., 2015; Mao et al., 2017). Specifically, poisons derived from some toxic dinoflagellates have caused epidemics in humans (Hoagland et al., 2014; Reich et al., 2015), fish, and birds (Fauquier et al., 2013; Brooks et al., 2016) and negatively impacted tourism (Brooks et al., 2016). The increasing risk from HABs has fueled research on developing a methodology for monitoring, and even attempting to control, HABs (Zhang et al., 2016; Ruiz-Ruiz et al., 2017; Page et al., 2018).
To evaluate the water quality of lakes and streams, the Carlson trophic state index (CTSI), which is calculated from the Secchi disc depth (SD) and concentrations of Chl a and total phosphorus (TP; Carlson, 1977), is routinely employed, though other indices have been proposed since this seminal work (Tew et al., 2014; Zhang et al., 2016; Page et al., 2018; Chen and Chen, 2021). A review of all published models and methods found that a reliable evaluation of water quality requires a professional sampling technician, 3–7 days of sample analysis, and at least one (but oftentimes more) analytical model (Ruiz-Ruiz et al., 2017). Given the speed at which data are generated and analyzed, the lack of real-time information has thwarted the efforts to reduce eutrophication impacts. Therefore, the aim of this study was to identify the key predictors of algal blooms by correlating data within a dataset obtained from Dapeng Bay, a highly impacted lagoon in Southern Taiwan with regular algal blooms. Specifically, Physicochemical parameters of seawater were analyzed using the National Institute of Environment Analysis (NIEA) methods, input into the CTSI model, and the results were compared with those of a novel, real-time monitoring system aimed at quickly and reliably assessing seawater quality parameters found to correlate strongly with algal blooms.
Dapeng Bay is a 3.5 km × 1.8 km lagoon (5.3 km2) located off Pingtung county, Southern Taiwan, which has featured in several prior studies (Hsieh et al., 2010; Tew et al., 2010, 2014). The only connection with the open ocean (Taiwan Strait) is through a narrow channel (1 km long, 138 m wide, and ∼2 m deep), and water residence time in the lagoon is consequently over 30 days, but varies during different monsoon conditions (Lee et al., 2020). There are five small drainages discharging into the lagoon, all of which contain aquaculture and farming wastewater with high amounts of nitrogenous and phosphorous nutrients (Hsieh et al., 2010; Tew et al., 2010). Though the total amount of discharge into the lagoon is low relative to the exchange rate with the Taiwan Strait, the nutrient levels are nevertheless high in Dapeng Bay (Tew et al., 2010; Meng et al., 2017), resulting in repeated algal blooms in the lagoon (Tew et al., 2010, 2014). Such algal blooms are usually associated with low DO and consequently mass fish mortality (Hsieh et al., 2010, 2012; Tew et al., 2010). During the period 2015–2016, three locations in the lagoon were selected for long-term, real-time automated water quality monitoring (Figure 1), which include a site close to the lagoon outlet (R1), a site in the middle of the lagoon (R2), and the most remote location from the lagoon outlet (R3). Based on our previous studies, these three continuous monitoring stations have been chosen according to the following reasons: (1) R1 station is the closest to the opening channel of the lagoon, where the circulation and flushing rate is the best among all; (2) R2 station is in the middle of the lagoon, where the circulation and flushing rate is moderate; and (3) R3 is the inner station, where the circulation and flushing rate is the poorest (Tew et al., 2014). Another ten stations (DP1–DP10) around the lagoon were also established for seasonal water quality monitoring.
Figure 1. Long-term real-time monitoring sites (R1–R3) and seasonal survey stations (DP1–DP10) of water trophic levels in Dapeng Bay, Southern Taiwan. The five arrows represent the small drainage channels. The hollow arrow represents the inlet-outlet to the sea.
Long-term, real-time monitoring of water quality at the three primary sites (R1–R3) was achieved via a multi-parameter sonde (YSI-6600-V2, YSI EXO 2), a wireless transmission system, and a data receiving and analysis base (Figure 2). The sonde simultaneously measured DO saturation (%) and content (mg/L), conductivity, specific conductance, temperature, salinity, pH, and turbidity. The water quality data were sent back to the monitoring base every 30 min, and the sonde was calibrated every 2 weeks according to the manufacturer’s recommendations. Furthermore, data were periodically compared with those analyzed in the laboratory using the NIEA 102.51C guidelines.1 At the other 10 stations, water was collected every 3 months for 2 years. Temperature, salinity, DO, pH, and transparency were measured in situ with an Ocean Seven 304 CTD (temperature and salinity), a pH meter (Hach, Sension 1), and a YSI Pro ODO DO meter. Oxygen saturation percentages were converted to oxygen concentrations as suggested by Chen (1981).
Surface water samples (30 cm below the surface) were collected with Niskin samplers, stored at 4°C during transport to the laboratory, and later analyzed for Chl a and phytoplankton composition and abundance. To determine the Chl a, water samples were first filtered through GF/F filter paper (Whatman, United Kingdom) and desiccated in the dark at −20°C before extraction in 10 ml of 90% acetone in complete darkness for 20 h (Lobban et al., 1988). After centrifugation at 1000 × g for 15 min, Chl a in the supernatant was measured with a Turner (model 450) fluorometer. For the analysis of phytoplankton, 1 L samples were fixed immediately with Lugol’s solution and neutralized with formalin to a final concentration of 4%, concentrated by settling to 30 ml in the laboratory (Tew et al., 2010), and the phytoplanktons were then counted and identified to the lowest possible taxon in Utermöhl sedimentation chambers under an inverted microscope at ×400 magnification. All analytical procedures followed the quality assurance (QA) and quality control (QC) guidelines set forth by the Environmental Protection Administration of Taiwan, and the data obtained from the real-time monitoring system were compared to those obtained from these standardized methods.
Each dataset was examined for normality with Minitab14, and data were ln-transformed when they were not normally distributed. Simple correlation (Pearson’s correlation coefficient) was used to determine the relationship between Chl a content, phytoplankton abundance, oxygen saturation, and pH.
To develop a trophic state index that could be evaluated in real time, we examined the relative precision deviation (RPD; %) between the data from the real-time system and the standard methods (Table 1). Except for Chl a content (>90% RPD values), the RPDs of the temperature, salinity, pH, and oxygen saturation measurements were low (<2% in some cases), implying that the real-time monitoring system could provide highly reliable data on those four parameters. Therefore, we chose pH and DO saturation, instead of directly using real-time Chl a, to develop a quick and reliable prediction method. Given that Chl a is a major indicator for the trophic state of water, developing a correlation model using reliable data to evaluate Chl a is needed when applying a real-time monitoring system.
Table 1. The relative precision deviation (RPD) of data from the real-time monitoring system and standard methods developed by the Environmental Protection Administration, Taiwan.
The correlation between seasonal Chl a concentration and phytoplankton abundance from the 10 stations was examined in 2015 and 2016 (Figure 3), and these two parameters were positively and significantly correlated (R2 = 0.45, p < 0.001, n = 80). Algal photosynthesis in the water system could change water pH in different ways. During the light cycle, algal consumption of CO2 generally results in elevated pH. However, during algal blooms, high levels of algal respiration lead to CO2 release and a consequent decrease in pH (Mao et al., 2017). From our data, both pH and oxygen saturation displayed significant positive correlations with Chl a concentration (Figure 4; R2 = 0.15, p < 0.001 and R2 = 0.07, p < 0.05, respectively) across 2015 and 2016.
Figure 3. Correlation analysis between seasonal chlorophyll a (Chl a) concentration and phytoplankton abundance in Dapeng Bay (2015–2016).
Figure 4. Correlation analysis of chlorophyll a (Chl a) concentration vs. oxygen saturation (circle) and pH (triangle) in Dapeng Bay (2015–2016).
When we compared the CTSI calculated from the 2-year dataset with Chl a and phytoplankton abundance, we also found significant correlations (Figure 5; R2 = 0.44, p < 0.01 for Chl a; R2 = 0.51, p < 0.01 for phytoplankton). The CTSI defines four nutrient levels: oligotrophic (CTSI = 30–40), mesotrophic (CTSI = 40–50), eutrophic (CTSI = 50–70), and hyper-eutrophic (CTSI > 70). Therefore, we used CTSIs of 40, 50, and 70 as references to calculate the corresponding Chl a concentration using the regression equations obtained in Figure 5. Then, the calculated Chl a value was then converted to its corresponding pH and oxygen saturation with the correlation obtained in Figure 4. The corresponding Chl a concentration, oxygen saturation, and pH against the CTSI are shown in Table 2. Given that the Chl a concentration calculated from the CTSI was consistent with in situ algal blooming in Dapeng Bay, we defined an index called the real-time trophic state index (RTSI) by combining the parameters of oxygen saturation and pH obtained from a real-time monitoring system to classify trophic status (Table 3). The same idea has been applied in developing the river pollution index (Liou et al., 2004). For the RTSI calculation, we administered 1 point when oxygen saturation ≤ 114% and 1 point when pH ≤ 8.29, resulting in an RTSI ≤ 2, which we classified as oligotrophic. At oxygen saturation levels between 114 and 116% and pH between 8.29 and 8.30, 3 points were given to each parameter, resulting in 2 < RTSI ≤ 4, which we classified as mesotrophic. At oxygen saturation levels between 116 and 129% and pH between 8.30 and 8.41, 5 points were given to each parameter, respectively, resulting in 4 < RTSI ≤ 6, which we classified as eutrophic. At oxygen saturation levels >129% and pH > 8.41, 7 points were given to each parameter, respectively, resulting in RTSI > 6, which we classified as hypereutrophic (Table 3).
Figure 5. Correlation analysis of (A) chlorophyll a (Chl a) concentration and (B) phytoplankton abundance against the Carlson Trophic State Index (CTSI, Carlson, 1977) in Dapeng Bay (2015–2016).
Table 2. The corresponding values of chlorophyll a (Chl a), oxygen saturation (%), and pH to the Carlson trophic state index (CTSI) based on the water quality data obtained from Dapeng Bay (2015–2016).
Table 3. The index scores of oxygen saturation (%) and pH, and the conversion table between the real-time trophic state index (RTSI) and the Carlson trophic state index (CTSI), corresponding to the trophic status of the lagoon.
To verify the applicability of the RTSI, we compared the eutrophication categories defined by CTSI and RTSI. For the site R1 dataset, the RTSI and CTSI gave similar classifications in 21,609 out of 34,108 water samples (63%). Agreement percentages were similar at sites R2 (20,450 out of 34,244 datasets = 60%) and R3 (20,403 out of 33,241 = 61%). Seasonal survey data from sites DP1, DP6, and DP9, which were close to sites R1, R2, and R3, respectively, were also used to compare the CTSI and RTSI classifications. Among 24 datasets, 91.7% of the RTSI were in agreement with CTSI, suggesting that the RTSI is as good as CTSI in predicting the trophic status of a water body.
The trophic state of the aquatic environment is crucial for the formation of algal blooms (Heisler et al., 2008; Rigosi et al., 2014). Understanding the trophic status of a water body can provide an early warning of the pressures and problems confronted by the aquatic ecosystem (Saluja and Garg, 2017; Sruthy et al., 2021). Although algal blooming has occurred worldwide, no consensus has been achieved about the main causes of this phenomenon, suggesting the need for more survey locations and intensive sampling (O’Neill et al., 2015). However, previous methods developed to monitor the eutrophication of lake or coastal water usually lack real-time information (Tew et al., 2014; Page et al., 2018; Chen and Chen, 2021). In addition, the published models and methods also suggest that a reliable evaluation of the trophic state of an aquatic environment requires a professional sampling technician, 3–7 days of sample analysis, and sometimes more than one model (Ruiz-Ruiz et al., 2017). Thus, there exists the need for rapid real-time monitoring of aquatic ecosystems. However, since the algal communities vary among different environments, the equations established in this study and the coefficients in the RTSI will vary. Thus, data must be accumulated and the coefficients must be calculated in order to establish an RTSI suitable for each local environment.
Whether the RTSI is a better predictor of algal blooms than the CTSI remains to be tested. Since high deviations usually occur using a Chl a sensor, and pH or DO data alone is insufficient in predicting the trophic state of an aquatic environment, the RTSI that uses both pH and oxygen saturation data seems promising. If such results are ultimately shown, then we may soon possess the capacity to monitor the likelihood of algal bloom formation in real time, thereby ensuring that managers are promptly informed as to where mitigative actions could be taken.
The original contributions presented in the study are included in the article/supplementary material, further inquiries can be directed to the corresponding authors.
KT and P-JM conceived the presented idea. P-JM developed the theory and performed the computations. C-CC and J-TW verified the analytical methods and wrote the manuscript. C-YH assembled and analyzed the preliminary data. KT, P-JM, and H-YH supervised the findings of this work. All authors discussed the results and contributed to the final manuscript.
This study was financially supported by grants from the Ministry of Science and Technology of Taiwan (MOST 106-2611-M-291-006 and MOST 107-2611-M-291-001 to P-JM; MOST 110-2611-M-003-002 to C-CC) and Dapeng Bay National Scenic Area Administration (to P-JM and KT).
The authors declare that the research was conducted in the absence of any commercial or financial relationships that could be construed as a potential conflict of interest.
All claims expressed in this article are solely those of the authors and do not necessarily represent those of their affiliated organizations, or those of the publisher, the editors and the reviewers. Any product that may be evaluated in this article, or claim that may be made by its manufacturer, is not guaranteed or endorsed by the publisher.
We would like to thank Anderson Mayfield and John Shea for their critical comments on the manuscript as well as thorough proofreading of the English.
Andersen, J. H., Schluter, L., and Ærtebjerg, G. (2006). Coastal eutrophication: recent developments in definitions and implications for monitoring strategies. J. Plankton Res. 28, 621–628. doi: 10.1093/plankt/fbl001
Anderson, D. M., Fensin, E., Gobler, C. J., Hoeglund, A. E., Hubbard, K. A., Kulis, D. M., et al. (2021). Marine harmful algal blooms (HABs) in the United States: history, current status and future trends. Harmful Algae 102:101975. doi: 10.1016/j.hal.2021.101975
Araujo, L. R., Lopes, P. M., Santangelo, J. M., Esteves, F. D., and Bozelli, R. L. (2015). Long-term dynamics of the zooplankton community during large salinity fluctuations in a coastal lagoon. Mar. Freshwater Res. 66, 352–359. doi: 10.1071/mf14083
Boynton, W. R., Hagy, J. D., Murray, L., Stokes, C., and Kemp, W. M. (1996). A comparative analysis of eutrophication patterns in a temperate coastal lagoon. Estuaries 19, 408–421. doi: 10.2307/1352459
Brooks, B. W., Lazorchak, J. M., Howard, M. D. A., Johnson, M. V. V., Morton, S. L., Perkins, D. A. K., et al. (2016). Are harmful algal blooms becoming the greatest inland water quality threat to public health and aquatic ecosystems? Environ. Toxicol. Chem. 35, 6–13. doi: 10.1002/etc.3220
Carlson, R. E. (1977). A trophic state index for lakes1. Limnol. Oceanogr. 22, 361–369. doi: 10.4319/lo.1977.22.2.0361
Chen, C. K., and Chen, Y. C. (2021). Detection of chlorophyll fluorescence as a rapid alert of eutrophic water. Water Supply ws2021388. doi: 10.2166/ws.2021.388
Chen, C. T. (1981). “Oxygen solubility in seawater,” in ‘Solubility Data Series, vol. 7, Oxygen and Ozone’, ed. R. Battino (Oxford: Pergamon Press), 41–55. doi: 10.1016/b978-0-08-023915-6.50009-3
Cloern, J. E. (2001). Our evolving conceptual model of the coastal eutrophication problem. Mar. Ecol. Prog. Ser. 210, 223–253. doi: 10.3354/meps210223
Fauquier, D. A., Flewelling, L. J., Maucher, J. M., Keller, M., Kinsel, M. J., Johnson, C. K., et al. (2013). Brevetoxicosis in seabirds naturally exposed to Karenia brevis blooms along the central west coast of Florida. J. Wildl. Dis. 49, 246–260. doi: 10.7589/2011-09-270
Heisler, J., Glibert, P. M., Burkholder, J. M., Anderson, D. M., Cochlan, W., Dennison, W. C., et al. (2008). Eutrophication and harmful algal blooms: a scientific consensus. Harmful Algae 8, 3–13. doi: 10.1016/j.hal.2008.08.006
Ho, J. C., and Michalak, A. M. (2015). Challenges in tracking harmful algal blooms: a synthesis of evidence from Lake Erie. J. Great Lakes Res. 41, 317–325. doi: 10.1016/j.jglr.2015.01.001
Hoagland, P., Jin, D., Beet, A., Kirkpatrick, B., Reich, A., Ullmann, S., et al. (2014). The human health effects of Florida Red Tide (FRT) blooms: an expanded analysis. Environ. Int. 68, 144–153. doi: 10.1016/j.envint.2014.03.016
Hsieh, W. C., Chen, C. C., Shiah, F. K., Hung, J. J., Chiang, K. P., Meng, P. J., et al. (2012). Community metabolism in a tropical lagoon: carbon cycling and autotrophic ecosystem induced by a natural nutrient pulse. Environ. Eng. Sci. 29, 776–782. doi: 10.1089/ees.2011.0252
Hsieh, W. C., Lee, H. J., Tew, K. S., Lin, C., Fan, K., and Meng, P. J. (2010). Estimating nutrient budgets in a coastal lagoon. Chin. Sci. Bull. 55, 484–492. doi: 10.1007/s11434-009-0436-4
Islam, M. S., and Tanaka, M. (2004). Impacts of pollution on coastal and marine ecosystems including coastal and marine fisheries and approach for management: a review and synthesis. Mar. Pollut. Bull. 48, 624–649. doi: 10.1016/j.marpolbul.2003.12.004
Karlson, B., Andersen, P., Arneborg, L., Cembella, A., Eikrem, W., John, U., et al. (2021). Harmful algal blooms and their effects in coastal seas of Northern Europe. Harmful Algae 102:101989. doi: 10.1016/j.hal.2021.101989
Lee, H. J., Meng, P. J., Chen, C. C., and Tew, K. S. (2020). Monsoon effects on the residence time of a coastal lagoon in southwestern Taiwan. Estuar. Coast. Shelf Sci. 233:106535. doi: 10.1016/j.ecss.2019.106535
Liou, S. M., Lo, S. L., and Wang, S. H. (2004). A generalized water quality index for Taiwan. Environ. Monit. Assess. 96, 35–52. doi: 10.1023/b:emas.0000031715.83752.a1
Lobban, C. S., Chapman, D. J., and Kremer, B. P. (1988). Experimental Phycology: A Laboratory Manual. Cambridge: Cambridge University Press.
Lv, H., Yang, J., Liu, L. M., Yu, X. Q., Yu, Z., and Chiang, P. C. (2014). Temperature and nutrients are significant drivers of seasonal shift in phytoplankton community from a drinking water reservoir, subtropical China. Environ. Sci. Pollut. Res. 21, 5917–5928. doi: 10.1007/s11356-014-2534-3
Mao, X. D., Kuang, C. P., Gu, J., Kolditz, O., Chen, K., Zhang, J. L., et al. (2017). Analysis of chlorophyll-a correlation to determine nutrient limitations in the coastal waters of the Bohai Sea, China. J. Coast. Res. 33, 396–407. doi: 10.2112/jcoastres-d-16-00023.1
Meng, P. J., Tew, K. S., Hsieh, H. Y., and Chen, C. C. (2017). Relationship between magnitude of phytoplankton blooms and rainfall in a hyper-eutrophic lagoon: a continuous monitoring approach. Mar. Pollut. Bull. 124, 897–902. doi: 10.1016/j.marpolbul.2016.12.040
Metcalf, J. S., and Codd, G. A. (2009). Cyanobacteria, neurotoxins and water resources: are there implications for human neurodegenerative disease? ALS 10, 74–78. doi: 10.3109/17482960903272942
Michalak, A. M. (2016). Study role of climate change in extreme threats to water quality. Nature 535, 349–350. doi: 10.1038/535349a
Nixon, S. W. (1995). Coastal marine eutrophication: a definition, social causes, and future concerns. Ophelia 41, 199–219. doi: 10.1080/00785236.1995.10422044
O’Neill, K., Schreider, M., McArthur, L., and Schreider, S. (2015). Changes in the water quality characteristics during a macroalgal bloom in a coastal lagoon. Ocean Coast. Manag. 118, 32–36. doi: 10.1016/j.ocecoaman.2015.04.020
Paerl, H. W., and Paul, V. J. (2012). Climate change: links to global expansion of harmful cyanobacteria. Water Res. 46, 1349–1363. doi: 10.1016/j.watres.2011.08.002
Paerl, H. W., and Scott, J. T. (2010). Throwing fuel on the fire: synergistic effects of excessive nitrogen inputs and global warming on harmful algal blooms. Environ. Sci. Technol. 44, 7756–7758. doi: 10.1021/es102665e
Page, T., Smith, P. J., Beven, K. J., Jones, I. D., Elliott, J. A., Maberly, S. C., et al. (2018). Adaptive forecasting of phytoplankton communities. Water Res. 134, 74–85. doi: 10.1016/j.watres.2018.01.046
Pinckney, J. L., Paerl, H. W., Tester, P., and Richardson, T. L. (2001). The role of nutrient loading and eutrophication in estuarine ecology. Environ. Health Perspect. 109, 699–706. doi: 10.1289/ehp.01109s5699
Pitcher, G. C., and Probyn, T. A. (2011). Anoxia in southern Benguela during the autumn of 2009 and its linkage to a bloom of the dinoflagellate Ceratium balechii. Harmful Algae 11, 23–32. doi: 10.1016/j.hal.2011.07.001
Reich, A., Lazensky, R., Faris, J., Fleming, L. E., Kirkpatrick, B., Watkins, S., et al. (2015). Assessing the impact of shellfish harvesting area closures on neurotoxic shellfish poisoning (NSP) incidence during red tide (Karenia brevis) blooms. Harmful Algae 43, 13–19. doi: 10.1016/j.hal.2014.12.003
Rigosi, A., Carey, C. C., Ibelings, B. W., and Brookes, J. D. (2014). The interaction between climate warming and eutrophication to promote cyanobacteria is dependent on trophic state and varies among taxa. Limnol. Oceanogr. 59, 99–114. doi: 10.4319/lo.2014.59.1.0099
Ruiz-Ruiz, T. M., Arreola-Lizarraga, J. A., Morquecho, L., Mendez-Rodriguez, L. C., Martinez-Lopez, A., and Mendoza-Salgado, R. A. (2017). Detecting eutrophication symptoms in a subtropical semi-arid coastal lagoon by means of three different methods. Wetlands 37, 1105–1118. doi: 10.1007/s13157-017-0944-y
Saluja, R., and Garg, J. K. (2017). Trophic state assessment of Bhindawas Lake, Haryana, India. Environ. Monit. Assess. 189:32. doi: 10.1007/s10661-016-5735-z
Smith, V. H. (2003). Eutrophication of freshwater and coastal marine ecosystems - A global problem. Environ. Sci. Pollut. Res. 10, 126–139. doi: 10.1065/espr2002.12.142
Sruthy, G. S., Priya, K. L., Madhu, A. M., Chellappan, S., Adarsh, S., and Haddout, S. (2021). Fuzzy logic approach for the assessment of trophic state of water bodies. Ecol. Eng. 169:106314. doi: 10.1016/j.ecoleng.2021.106314
Tew, K. S., Chou, W. R., Chuang, S. F., He, C. C., Liao, J. H., and Fang, L. S. (2008). Comparison of the decapod communities in an anthropogenic impacted lagoon and its adjacent coastal waters. Hydrobiologia 607, 1–10. doi: 10.1007/s10750-008-9328-x
Tew, K. S., Meng, P. J., Glover, D. C., Wang, J. T., Leu, M. Y., and Chen, C. C. (2014). Characterising and predicting algal blooms in a subtropical coastal lagoon. Mar. Freshwater Res. 65, 191–197. doi: 10.1071/mf13029
Tew, K. S., Meng, P. J., Lee, H. J., Ye, Y. X., Kuo, J., Fang, L. S., et al. (2010). Dynamics of phytoplankton and picoplankton over a tidal cycle in a subtropical lagoon. Chin. Sci. Bull. 55, 2522–2528. doi: 10.1007/s11434-010-4107-2
Ulloa, M. J., Alvarez-Torres, P., Horak-Romo, K. P., and Ortega-Izaguirre, R. (2017). Harmful algal blooms and eutrophication along the mexican coast of the Gulf of Mexico large marine ecosystem. Environ. Dev. 22, 120–128. doi: 10.1016/j.envdev.2016.10.007
Wang, J. L., Zhang, Y. J., Yang, F., Cao, X. M., Bai, Z. Q., Zhu, J. X., et al. (2015). Spatial and temporal variations of chlorophyll-a concentration from 2009 to 2012 in Poyang Lake, China. Environ. Earth Sci. 73, 4063–4075. doi: 10.1007/s12665-014-3691-x
Yang, J., Lv, H., Yang, J., Liu, L. M., Yu, X. Q., and Chen, H. H. (2016). Decline in water level boosts cyanobacteria dominance in subtropical reservoirs. Sci. Total Environ. 557, 445–452. doi: 10.1016/j.scitotenv.2016.03.094
Zhang, Y. L., Shi, K., Liu, J. J., Deng, J. M., Qin, B. Q., Zhu, G. W., et al. (2016). Meteorological and hydrological conditions driving the formation and disappearance of black blooms, an ecological disaster phenomena of eutrophication and algal blooms. Sci. Total Environ. 569, 1517–1529. doi: 10.1016/j.scitotenv.2016.06.244
Keywords: algal bloom, nutrients, trophic state index, water quality, Taiwan
Citation: Chen C-C, Wang J-T, Huang C-Y, Hsieh H-Y, Tew KS and Meng P-J (2022) Developing a Real-Time Trophic State Index of a Seawater Lagoon: A Case Study From Dapeng Bay, Southern Taiwan. Front. Mar. Sci. 9:640046. doi: 10.3389/fmars.2022.640046
Received: 10 December 2020; Accepted: 25 February 2022;
Published: 27 April 2022.
Edited by:
Angel Borja, Technological Center Expert in Marine and Food Innovation (AZTI), SpainReviewed by:
Béchir Béjaoui, Institut National des Sciences et Technologies de la Mer, TunisiaCopyright © 2022 Chen, Wang, Huang, Hsieh, Tew and Meng. This is an open-access article distributed under the terms of the Creative Commons Attribution License (CC BY). The use, distribution or reproduction in other forums is permitted, provided the original author(s) and the copyright owner(s) are credited and that the original publication in this journal is cited, in accordance with accepted academic practice. No use, distribution or reproduction is permitted which does not comply with these terms.
*Correspondence: Kwee Siong Tew, dGV3a3NAbm1tYmEuZ292LnR3; Pei-Jie Meng, cGptZW5nQGdtcy5uZGh1LmVkdS50dw==
†These authors have contributed equally to this work
Disclaimer: All claims expressed in this article are solely those of the authors and do not necessarily represent those of their affiliated organizations, or those of the publisher, the editors and the reviewers. Any product that may be evaluated in this article or claim that may be made by its manufacturer is not guaranteed or endorsed by the publisher.
Research integrity at Frontiers
Learn more about the work of our research integrity team to safeguard the quality of each article we publish.