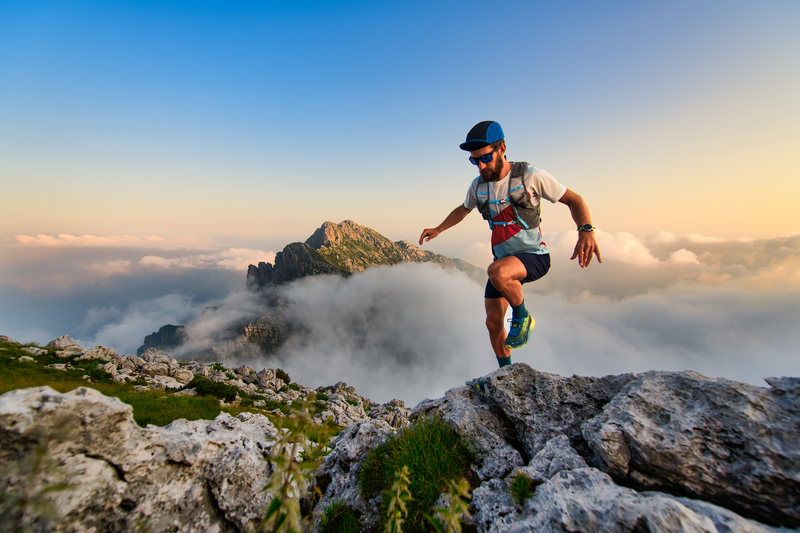
94% of researchers rate our articles as excellent or good
Learn more about the work of our research integrity team to safeguard the quality of each article we publish.
Find out more
ORIGINAL RESEARCH article
Front. Mar. Sci. , 13 January 2023
Sec. Marine Ecosystem Ecology
Volume 9 - 2022 | https://doi.org/10.3389/fmars.2022.1096594
This article is part of the Research Topic Seascape Ecology: from characterization to evaluation of state and change over time View all 10 articles
Accurate data on community structure is a priority issue in studying coastal habitats facing human pressures. The recent development of remote sensing tools has offered a ground-breaking way to collect ecological information at a very fine scale, especially using low-cost aerial photogrammetry. Although coastal mapping is carried out using Unmanned Aerial Vehicles (UAVs or drones), they can provide limited information regarding underwater benthic habitats. To achieve a precise characterisation of underwater habitat types and species assemblages, new imagery acquisition instruments become necessary to support accurate mapping programmes. Therefore, this study aims to evaluate an integrated approach based on Structure from Motion (SfM) photogrammetric acquisition using low-cost Unmanned Aerial (UAV) and Surface (USV) Vehicles to finely map shallow benthic communities, which determine the high complexity of coastal environments. The photogrammetric outputs, including both UAV-based high (sub-meter) and USV-based ultra-high (sub-centimetre) raster products such as orthophoto mosaics and Digital Surface Models (DSMs), were classified using Object-Based Image Analysis (OBIA) approach. The application of a supervised learning method based on Support Vector Machines (SVM) classification resulted in good overall classification accuracies > 70%, proving to be a practical and feasible tool for analysing both aerial and underwater ultra-high spatial resolution imagery. The detected seabed cover classes included above and below-water key coastal features of ecological interest such as seagrass beds, “banquettes” deposits and hard bottoms. Using USV-based imagery can considerably improve the identification of specific organisms with a critical role in benthic communities, such as photophilous macroalgal beds. We conclude that the integrated use of low-cost unmanned aerial and surface vehicles and GIS processing is an effective strategy for allowing fully remote detailed data on shallow water benthic communities.
Coastal areas are vital for human activities and biological processes, constituting a critical interface between land and sea (Lakshmi and Rajagopalan, 2000). Although coastal areas cover only 10% of the earth’s surface area, they host over 60% of the world’s population and drive the growth of fisheries, infrastructure development and tourism, resulting in additional pressure on natural coastal ecosystems (Parravicini et al., 2012). Over half of the world’s coastal ecosystems can be considered under “moderate” or “high” threat from human development, implying an increased risk for benthic communities and associated species (Bryant et al., 1995; Dauvin et al., 2012; Morroni et al., 2020; Rakaj et al., 2021). Coastal areas are often composed of habitat mosaics, including terrestrial (above water) and marine (below water) features that play a key role in ecosystem functioning. For instance, in tropical environments, mangroves, coral, rocky reefs, and seagrasses usually form a continuum of shallow water habitats in which organisms spend parts of their early lifestages being nursery grounds for marine biota (Nagelkerken et al., 2015). Similarly, in temperate waters, coastal areas, including transitional waters (estuaries and lagoons), intertidal zone as well as shallow marine underwater habitats, are the result of the dynamic interactions between land and sea, resulting in very high level of productivity (Courrat et al., 2009). Shallow Mediterranean benthic habitats are generally dominated by seagrass meadows, macroalgal beds, sandy, and rocky substrates that form complex landscapes. This complexity influences the distribution of living organisms that are dispersed neither uniformly nor randomly but display various gradients or other types of spatial patterns due to microhabitat availability and resource utilisation (Letourneur et al., 2003). Considering such very highly localised and patchy diversity, how these organisms distribute spatially and temporally across habitats is thus relevant for understanding species ecology and for conservation purposes (Tait et al., 2021). Habitat availability and spatio-temporal segregation are often important underlying factors that explain the distribution of animal and vegetal assemblages along coastal environments (Harmelin-Vivien et al., 1995; Seytre and Francour, 2014; Ventura et al., 2015; Cheminée et al., 2017; Cheminée et al., 2021). One necessary step to comprehend these spatio-temporal dynamics in marine organism distribution consists in the characterization at appropriate spatial scales of the seascapes (Castellanos-Galindo et al., 2019). Furthermore, some species of seagrasses and macroalgae are among the most important marine ecosystem engineers, forming extended carpets and canopies which provide a wide range of ecological services such as primary production, carbon sequestration, nutrient recycling, dissipation of wave energy, and nursery habitats for many juvenile species (Heck et al., 2003; Sales et al., 2012; Cheminée et al., 2013; Cheminée et al., 2017; Morris et al., 2020). The increase of human activities such as coastal development, fisheries and marine traffics exacerbates the anthropogenic pressure on coastal ecosystems through pollution, alteration of sedimentary processes and habitat fragmentation, that together with climate change, strongly affect the distribution of seagrass meadows (Boudouresque et al., 2009) and macroalgal forests (Duarte et al., 2018), impacting, in turn, ecosystem functionig (Claudet and Fraschetti, 2010; Coll et al., 2012; Chand and Bollard, 2021). This dynamic scenario demands baseline information and adequate monitoring to understand the processes driving the ongoing ecological shifts. In this framework, solid spatial knowledge of habitat and species distribution over fine spatial and temporal scales is critical to support all stages of marine spatial planning, to provide scientific knowledge for decision-makers and guidance for the sustainable exploitation of marine resources (Levin et al., 2014; Martin et al., 2014; Fabbrizzi et al., 2020). Different technologies and methods based on remote sensing such as aerial imagery has been used for decades, and earth-observation satellites are now a staple of ecological monitoring globally (Tait et al., 2021). Spatial mapping of shallow-water benthic habitats using satellite data has been widely performed in temperate (Mumby and Edwards, 2002; Borfecchia et al., 2019) and tropical environments (Roelfsema and Phinn, 2010). New proprietary satellite systems (QuickBird, GeoEye-1, Ikonos, Worldview-4) offer up to 31 cm spatial resolution for panchromatic imagery (Alkan, 2018) and commercial aerial photography capable to reachup to 6 cm pixel area (Zhang and Hu, 2012). However, their use for ultra-fine scale ecological studies is still hindered by considerable limitations such as high costs per scene, revisit time, spatial resolution, and cloud cover which may negatively affect many applications for mapping shallow-water benthic environments (Anderson and Gaston, 2013). In this scenario, unmanned aerial vehicles (UAVs) represent a valuable tool for local scale monitoring thanks to their relatively low cost and high customizability, which allow fast and automatic data acquisition over difficult or dangerous areas to access (Hardin and Hardin, 2010). UAVs equipped with Inertial Measurement Units (IMU), GPS and RGB cameras can deliver georeferenced imagery that can be processed by a plethora of Structure from Motion (SfM) photogrammetry software, opening new possibilities for the development of effective algorithms capable of producing ultra-high spatial resolution orthophoto mosaic and digital elevation models (DEMs). In fact, UAVs and SfM processing have been successfully applied for natural resource assessment and environmental monitoring of the coastal areas (Burns et al., 2015; Nikolakopoulos et al., 2017; Ventura et al., 2018; Burns et al., 2019; Taddia et al., 2019; Kabiri, 2020; Ventura et al., 2022). These products, other than playing a key role in assessing 3D habitat complexity and health conditions of specific biotopes such as biogenic reefs in tropical (Raoult et al., 2017; Burns et al., 2019; Fallati et al., 2020; Nieuwenhuis et al., 2022) and temperate (Zapata-Ramírez et al., 2013; Marre et al., 2019; Prado et al., 2020; Ventura et al., 2020) environnements, can also provide valuable information for fine-scale assessment and monitoring of seagrass and macroalgal beds limits, level of fragmentation, and restoration activities (Marre et al., 2020; Rende et al., 2020; Ventura et al., 2022). Moreover, UAVs provide a means to map the distribution and behaviour of many organisms in shallow aquatic environments that typically have high contrast against the substrate (Raoult and Gaston, 2018; Raoult et al., 2018; Williamson et al., 2021). Despite the recent extensive adoption of drones for marine research, this methodology still presents some constraints in providing a detailed analysis of the benthic community in terms of species assemblages. Underwater operator surveys must combine and integrate this form of remote-sensed imagery with biological data. For that purpose, Unmanned Surface Vehicles (USVs) can provide accurate information on habitat distribution in shallow waters, potentially overcoming underwater operator survey constraints through remote imagery acquisition.
This study aimed to employ two types of low-cost unmanned vehicles (UAVs and USVs) as integrated aerial and underwater acquisition tools for shallow coastal habitat mapping, combining SfM-based outputs consisting of aerial and underwater imagery of benthic assemblages. To analyse high and ultra-high spatial resolution maps, taxonomic identification by experts and supervised image classification to identify and provide information on the structure of distinct benthic habitats were employed within the same study area, according to the various levels of detail. Subsequently, we evaluated the accuracy of image classifications based on the Support Vector Machine (SVM) supervised learning algorithm to map shallow benthic habitats using both UAV and USV-based imagery. Finally, in this study we propose some general guidelines to encourage the adoption of this integrated methodological strategy in the mapping of local coastal habitats and associated biotopes and future applications for monitoring proposes.
The study area (3 Ha) is located north of Civitavecchia in the central Tyrrhenian Sea, Italy (11°44’2.233”E; 42°9’43.043”N). This is an important ecological area within a marine Site of Community Importance (SCI), “Fondali tra Punta S. Agostino e Punta Mattonara” and the natural monument “La Frasca” on the coastal side (Figure 1). This area included in a small bay, encompassing shallow sandy and rocky shorelines with gentle slope, is characterised by a rocky coast, halophilous vegetation and a healthy pinewood. The shallow infralittoral rocky bottoms are characterised at depths from 0.1 m up to 3 m by complex communities constituted by seagrass patches of Posidonia oceanica (L.) Delile, brown (Phaeophyta), red (Rhodophyta), and green (Chlorophyta) photophilous algal assemblages that host a large number of endemic species (Gravina et al., 2020). Considering this complex landscape, the shallow water and the high heterogeneity of benthic habitats, this area was selected to apply this integrated methodological approach encompassing aerial and underwater imagery acquisition using an unmanned aerial vehicle (UAV) and an unmanned surface vehicle (USV), respectively. Therefore, for a fine-scale characterisation inside the total mapped area, we identified two additional subplots to improve the image classification routine and detect specific cover classes characterizing the submerged benthic habitats. More specifically, for the UAV fine scale classification we chose an area with very high heterogeneity (microhabitat availability) and complex topographic characteristics (outcropping rocks) which allowed the presence of indicator species such as seagrasses, brown (Fucales) and green (Ulvales and Cladophorales) algae. For ultra-fine scale mapping and classification using USV a representative area of the deeper seabed displaying Posidonia oceanica patches and hard rocky substrata with photophilic algae was chosen (Figure 1).
Figure 1 The study area along the central Latium coast where shallow benthic habitats were mapped using low-cost unmanned aerial (UAV) and water surface vehicles (USV). The white and blue polygons represent the areas mapped for large and fine-scale classification using UAV-based imagery, whereas the red polygon identifies the area mapped by the USV for ultra-fine classification of benthic communities. The positions of checkpoints (CPs) and tape measure used for accuracy assessments are reported.
High-resolution optical aerial images were acquired using a modified DJI Mavic 2 Pro quadcopter (Figure 2) in May 2022. This consumer-grade UAV is a lightweight (0.9 kg) and easy-to-carry drone equipped with a fully stabilised 3-axis gimbal Hasselblad L1D-20c camera with a 1-inch CMOS RGB sensor with a resolution capability of 5472 x 3648 (20 MP). Considering a GPS flight speed of 5 m/s and a flight height of 40 above mean sea level (AMSL), to maintain a sufficient overlap (>70%) between images, we took photos every 2 s by using the time-lapse mode with auto white balance and shutter priority (1/400 sec) to avoid motion blur in the acquired imagery. A circular polarizer (CPL) filter was used to minimise the effect of sun-glint. Even though the drone was equipped with a built-in GPS/GLONASS receiver, we mounted an additional GNSS antenna with Post Processing Kinematics (PPK) capabilities. With PPK, the acquired images can be georeferenced at centimetre-scale resolution and do not require a real-time connection between the base station and the rover. As the base station, we used Rinex 3.03 files downloaded from the nearby Continuously Operating Reference Station (CORS) of Civitavecchia of the Latium Permanent GNSS Network. GNSS Rinex files from the UAV (rover unit) and the base station (CORS) were post-processed with the Toposetter 2.0 app to obtain an accurate positioning of the images along the track of the pre-established autonomous flight path defined with the DJI Pilot app (https://www.dji.com/it/downloads/djiapp/dji-pilot ) running on a mobile device connected to the remote controller via Android debug bridge (ADB). Finally, the image coordinates were inspected spatially using RTKPLOT of RTKLib software to check the accuracy of post-processed coordinates (Takasu and Yasuda, 2009).
Figure 2 The two unmanned vehicles used in this research for mapping above and below water coastal habitats. (A, B) Mavic 2 pro (UAV) equipped with additional GNSS antenna for PPK and (C, D) Power Dolphin (USV) equipped with Gopro Hero 10 action camera.
Immediately after the UAV fight, high-resolution underwater images were acquired using the Power Dolphin (PowerVision Inc., Beijing, China) (Figure 2). This aquatic Unmanned Surface Vehicle (USV) was chosen based on its low-price range comparable to other consumer-level USVs. This device was operated by remote control and mobile device with the Vision+2 app, communicating through a built-in Wi-Fi signal. The Power Dolphin is a device that floats on the water surface and is propelled by two horizontal rear propellers. The built-in camera is mounted on the front of the USV with a user-adjustable tilt mechanism that can be oriented up and down in real-time using a remote control, independently of the direction of the USV (Diefenbacher, 2022). This device is also equipped with a GPS and depth echosounder, allowing to continuously collect depth data and reference coordinates. However, considering the low resolution of the built-in camera (1/2.3-inch CMOS 12 MP sensor), we decided to improve the acquisition of underwater imagery by adding a GoPro Hero 10 action camera with a 23 MP sensor. The camera was attached under Power Dolphin’s hull, pointing 90° downwards and acquiring photos every 1 sec. No protective housing was used, as this device is waterproof up to 10 m. The underwater imagery collected was then georeferenced using the onboard GPS and scaled during the photogrammetric processing using images representing the tape measure as a reference. The main features of the two systems used for imagery acquisition are reported in Table 1 in the supplementary material section.
Table 1 Residuals of the bundle adjustment transformation on seven checkpoints (CPs) and the total RMSE (cm).
The UAV- and USV-based imagery were processed using Agisoft Metashape v 1.8.1. With this low-cost SfM software package, 3D models and 2D raster products can be generated in a fully automated five-step process, comprising: (i) alignment of the photographs, (ii) calculation of a sparse point cloud, (iii) calculation of a dense 3D, (iv) polygonal mesh model generation and texture mapping, (v) generation of Digital Surface Models (DSMs) and orthorectification of the imagery (De Reu et al., 2013). Firstly, we performed the alignment of images with the parameter accuracy set to ‘high’. After the photoalignment, this initial bundle adjustment created sparse point clouds from overlapping digital images. The sparse point clouds included the position and orientation of each camera position and the 3D coordinates of all image features. The internal camera geometry was modelled by self-calibration during the bundle adjustment (Price et al., 2019). Subsequently, dense point clouds were built based on multi-view stereopsis (MVS) algorithms with high-quality and mild depth filtering. After filtering the dense point clouds according to points confidence (points with values less than three were removed), these were used for producing polygonal meshes and DSMs using an Inverse Distance Weighting (IDW) interpolation. Finally, the DSMs generated ortho-rectified RGB photomosaics of emerged and submerged habitats.
Orthophoto mosaics and DSMs generated in Metashape were exported as raster images (GeoTIFF format, in the reference system WGS84/UTM zone 33 N, EPSG:32633) and transferred to a geographical information system (GIS) using ArcMap 10.6 software (Esri, 2011) for subsequent Object-Based Image Classification (OBIA) processing. At this stage, classification methods based only on pixel information are time-consuming and limited due to the spectral similarities. Therefore, we reduced the pixel complexity by segmenting the orthophotos into more meaningful objects to speed up the aerial and underwater imagery classification. A means-shift (MS) algorithm was applied using the segment means-shift function in ArcMap. The MS is a non-parametric segmentation/clustering algorithm that uses the number of pixels and the Euclidean distance defined within a spectral space to segment an image (Lee et al., 2009). In our case, the spectral details, spatial details, and minimum segment size parameters were set to 20, 15 and 600, respectively. The spectral detail setting was used to discriminate between objects based on spectral signatures. In contrast, spatial detail was used to discriminate between objects based on the shape of the features to produce sharper segments (Gaw et al., 2019). A minimum mapping unit of 500 pixels (approximately 5 cm2) was chosen. After the segmentation process, we manually selected a set of image objects as training samples to train the Support vector machine (SVM) algorithm, which is a supervised machine learning classifier well adapted to solving non-linear, high dimensional space classifications that have become increasingly popular in remote sensing classification (Heumann, 2011; Pipaud and Lehmkuhl, 2017). Spectral reflectance signature files for both segmented UAV- and USV-based orthomosaics were generated after collecting training samples in the Training Sample Manager Toolbar. We manually selected a set of image objects as training samples to train the SVM algorithm. The SVM model uses each band’s mean and standard deviation to classify the image objects in the whole dataset.
For comparison purposes, a subset of 0.3 Ha of the UAV-based orthophoto mosaics was classified using SVM without running MS segmentation to avoid spectral smoothing and detect specific cover classes.
To optimise the results, we then performed a post-classification routine (Droppova, 2011) on all the classification outputs by removing some misclassified regions of pixels and small isolated objects. This task was carried out using the Spatial Analyst extension in ArcMap, which provides a set of generalisation tools for the post-classification processing task involving three main steps: a) filtering the classified output using the ‘Majority Filter’ tool; b) smoothing the ragged class boundaries and clumping the classes using the ‘Boundary Clean’ tool and, c) generalising classified output by reclassifying small, isolated regions of pixels to the nearest classes with the ‘Region group’, ‘Set Null’ and ‘Nibble’ tools).
We used GNSS coordinates of seven checkpoints (CPs) to assess the horizontal (x and y) and vertical (z) positional accuracy of UAV-based SfM products. For checkpoint (CPs) coordinates’ acquisition, an Emlid Reach RS+, a low-cost single-frequency (L1 - 1575.42 MHz) GNSS receiver, was employed. We used both small reflective target and natural features such as rocks that were easily detectable in the UAV imagery. The quality of the photogrammetric models based on the CPs residuals was computed as the difference between the position estimated through PPK and the coordinates of the manually surveyed CPs. We computed the Root Mean Square Error (RMSE) for each mapped area in the E, N, and U directions and 3D. Small values of the Root Mean Square Error (RMSE) indicate good image alignment processes and block adjustments resulting in accurate 3D point clouds, digital elevation models (DEMs), and orthophoto mosaics.
Before starting imagery acquisition, four metric tape measures were positioned on the seabed from 0.1 up to 2.5 m depth to have a constant reference distance during field operation and for scaling and estimating the accuracy of the underwater 3D models acquired with the USV. Snorkelers followed the tape measure during underwater video acquisition for ground truth data collection (Figure 1).
To assess the proportional accuracy of the underwater USV-based model, we compared the known dimensions of objects (lengths measured along the metric tape measures) to their estimated dimensions in the model. The measurement accuracy for each linear distance estimated from the 3D models was expressed as a percentage of difference (Young et al., 2017).
A confusion matrix for each mapped area (from large-scale UAV-based to ultra-fine scale USV-based imagery) was calculated to evaluate the accuracy of the final classifications, including (i) producer’s accuracy, (ii) user’s accuracy; (iii) overall accuracy (OA); and (iv) the Kappa Index of Agreement (KIA). The confusion matrices were built using GNSS-based ground truth data points collected by snorkelers along the tape measure and randomly placed accuracy assessment points (distinct from the training sample areas). An expert classified each point manually by visually inspecting the original true colour orthophotos. Due to the ultra-high spatial resolution, visual photo interpretation could be considered very reliable for assessing the accuracy of thematic maps (Lechner et al., 2012).
The whole study area (3 Ha) was mapped after a flight time of 25’35’’ from a height of 40 m, leading to the acquisition of 339 aerial images (Supplementary Material Figure 2). After PPK, all the UAV GPS antenna positions showed a high ambiguity-fixed solutions percentage (Q1 = 100%, Supplementary Material Figure 1), resulting in a planimetric precision estimated after bundle adjustment at each CPs of 5.8 cm. In contrast, the altimetric precision was 11.1 cm (Table 1). The orthophoto mosaics and DSM showed a spatial resolution of 1.3 cm/pix and 1.6 cm/pix, respectively (Figures 3A, B). The 3D mesh model of the mapped with the UAV area is available at the following link: https://skfb.ly/oBEEL
Figure 3 (A) High spatial resolution UAV-based RGB orthophoto mosaic and (B) Digital surface models (DSM) with depth contour interval at 50 cm.
The high-resolution imagery allowed for a fine-scale characterisation of the site after MS segmentation and SVM classification (Figure 4). In fact, eleven cover classes encompassing both above and below the sea surface features are identified with an OA and KIA accuracy value of 0.77 and 0.75%, respectively (Supplementary Material Table 1). Among these, five major seabed cover classes representing broad benthic community-level categories were identified: Posidonia oceanica patches, soft bottoms with Cymodocea nodosa, hard bottoms with photophilic algae, hard bottoms with photophilic algae mixed with seagrass detritus (dead leaves and rhizomes), and sand. Significant seabed cover misclassification errors involved spectral confusion among classes with very similar spectral signatures, such as ‘beach’ class constituted by fine and wet sand (with a total of 35.6% of samples interpreted as coarse sand and banquette), hard bottoms with photophilic algae (with a total of 27.3% of samples interpreted as hard bottoms with photophilic algae with detritus and sand). Posidonia oceanica was classified with more than 90% user and producer accuracy, while Cymodocea nodosa reported only a 60% user accuracy value.
Figure 4 Thematic map showing the eleven cover classes identified after MS segmentation and SVM classification of the UAV-based high spatial resolution orthophoto mosaic.
Inside the selected subarea of 0.3 ha, the SVM classification was performed on the raw RGB orthomosaics without applying MS segmentation. This choice implied a longer computation time (+3h 20’) to train SVM, classify, and generalise classified raster outputs. However, working at pixel levels, without smoothing spectral signatures during segmentation, allowed a more detailed classification of the benthic habitats by splitting the two classes (‘hard bottoms with photophilic algae’ and ‘hard bottoms with photophilic algae and seagrass detritus’) into four additional cover classes with high ecological interest (Figures 5, 6). In fact, hard bottoms with photophilic algae can be distinguished by water depth (0-50 cm and > 50 cm) and according to the presence of algal assemblages dominated by Fucales brown algae of the genus Cystoseira spp., or green algae (Cladophorales and Ulvales). Seagrass cover classes represented by Posidonia oceanica and Cymodocea showed higher user and producer accuracy values than the large-scale classification carried out after MS segmentation. The other benthic cover classes displayed comparable values of percentage cover, with only slightly lower OA and KIA values (0.75 and 0.71, respectively) due to the misclassification of rocks covered by Cystoseira spp. As shallow rocks with other photophilic algae (represented by the ‘hard bottoms with photophilic algae (0- 50 cm)’ class) (Supplementary Material Table 2).
Figure 5 Comparison between large and fine scale UAV-based thematic maps. (A) UAV-based orthomosaics; (B) UAV-based large scale classification results after MS segmentation and SVM classification; (C) UAV-based fine scale classification results after SVM classification carried out without MS segmentation.
Figure 6 Bar plots reporting the percentage cover of each benthic cover class of UAV-based fine scale classification reported in Figure 4C.
Images were acquired from the surface using the PowerDolphin USV to map an area of 150 m2. The dimensions of the underwater objects (tape measure) rendered in the mesh model of the seabed matched strongly with their actual dimensions with a mean procedural accuracy for the length of 98.08% ± 0.02, resulting in a total error of 0.017 m. The generated orthomosaics (Figure 7A) and DSM showed an ultra-high spatial resolution of 1.3 and 2.2 mm/pixel, respectively. Through hill-shade map, a fine terrain representation based on topographic shielding factors for improving seabed morphology visualisation was generated using elevation data (Figure 7B). The 3D mesh model of the mapped area with the USV is available at the following link: https://skfb.ly/oBEHt
Figure 7 (A) The orthophoto mosaic derived from USV-based underwater imagery and (B) seabed morphology visualised through the hill shading map. The areas covered by Posidonia patches are missing due to significant errors during the DSM generation.
The SVM classification allowed the identification of five cover classes that implied a more detailed definition of the benthic habitat variability compared to the UAV-based classification. The benthic cover was mainly represented by hard calcareous bottoms covered by a carpet of photophilic red (52%) and brown algae (33%) (Figures 8, 9). Inside the broad cover class ‘hard bottom with photophilic algae’ previously identified also by UAV-based imagery, we mapped at the species level the arborescent algal cover (Figure 10), mainly represented by red algae (Jania rubens, Corallinales) and brown algae (Padina pavonica, Halopteris scoparia and Dictyota dichotoma). After MS segmentation, we reported lower per-class accuracies than other classifications, resulting in OA and KIA values of 73.4% and 0.61, respectively (Supplementary Material Table 2). Significant seabed cover misclassification errors involved spectral confusion among small sandy patches (with 53.6% of samples interpreted as brown and red algae) and brown algae (with a total of 53% of samples classified as red algae). In addition, the tape measure was classified as brown algae (Padina pavonica) due to its white colour. Similarly, to other classification method, Posidonia oceanica is identified in most cases with both user and producer accuracy values of > 80%.
Figure 8 Comparison between large scale UAV-based and ultra-fine scale USV-based thematic maps. (A) USV-based underwater orthomosaics over imposed on the UAV-based aerial orthomosaics; (B) UAV-based large scale classification results after MS segmentation and SVM classification; (C) USV-based ultra-fine classification results after SVM classification and MS segmentation.
Figure 9 Bar plots reporting the percentage cover of each benthic cover class of USV-based ultra-fine classification reported in Figure 7C.
Figure 10 (A) Detailed view of the USV-based underwater orthophoto mosaic in which the shrubby algal assemblage is visible and (B) the results after MS segmentation and SVM classification.
This study demonstrated that integrating consumer-grade unmanned aerial and surface vehicles (UAV and USV) is an effective tool for the characterization and mapping of shallow benthic habitats at fine and ultra-fine scales. Coastal benthic habitats, such as shallow rocky bottoms and seagrass beds, are among the most heavily anthropogenically-impacted marine ecosystems and are also among the most productive in terms of ecosystem functioning implying rigorous monitoring programs (O’Connor, 2013). Seagrass and seaweed are sensitive to changes in the ecosystem, making them valuable indicators of ecosystem health (Dokulil, 2003). The type, distribution, and condition of algal assemblages can drive water quality (pH, dissolved oxygen, suspended sediment) and local benthic and fish assemblages health (food resources, habitat), making the quantification of these species very useful in identifying mechanisms responsible for changes at both community and ecosystem-level (Airoldi et al., 1995; Benedetti-Cecchi and Cinelli, 1995; Kislik et al., 2020). In this context, the identification and fine mapping of canopy-forming species is a critical point in monitoring actions among coastal areas, which often exhibit significant habitat heterogeneity, even on a small scale, and are subject to various anthropogenic stressors (agricultural run-off and discharge of sewage, sedimentary alteration). Although numerous studies have already focused on the dynamics of seagrasses under the impact of human activities and have defined seagrass distribution and temporal trends on a large scale (Duarte, 2002; Telesca et al., 2015; Chefaoui et al., 2018), only a few have associated spatial, temporal and structural local data to these changes (Leriche et al., 2006; Pergent-Martini et al., 2022). To answer this question, UAV platforms can provide high spatial resolution at a frequency greater than traditional methods such as satellites, piloted aircraft, and LiDAR. We reported how some algal taxa could be detected directly as a good proxy for monitoring environmental quality changes (Cystoeira spp. Cladophorales and Ulvales) after refining the classification approach used for UAV imagery. We could detect, using the complete information available at the pixel level, the most critical algal assemblages among a limited shallow area with a relatively good level of accuracy. These sensing instruments may even provide quasi-continuous temporal coverage that results in a detailed description of the ecological dynamics that characterise most coastal species with fast development and short life cycles (e.g., algal blooms). We demonstrated that low-altitude UAV imagery is powerful in detecting and quantifying submerged vegetation over shallow areas characterised by fair water clarity and can be integrated into monitoring programs where recurrent sampling and mapping are needed. Therefore, as reported in other studies (Duffy et al., 2018; Yang et al., 2020), UAV-based imagery is a flexible, low-cost, and time-effective technique for monitoring intertidal and shallow water marine vegetation. Nevertheless, the acquisition methods carried out with a single instrument (e.g., UAV) show resolution limits that cannot be exceeded (e.g., species recognition and organism counting). Therefore, to achieve a precise characterization of the benthic assemblages, an integrated use of several acquisition instruments is necessary. Therefore, the integration of USV with consumer-grade UAV for photogrammetric acquisition makes it possible to characterise complex benthic communities at a broader resolution scale (from large to ultra-fine). The use of the USV for benthic habitat mapping can be considered an emerging tool to be implemented in monitoring plans since classification accuracy decreases with water depth, particularly in water deeper than 3 m (Shintani and Fonstad, 2017), limiting the use of UAV-based optical data to a narrow stretch of coast. Aerial images cannot capture the taxonomic detail with the same accuracy as in-situ surveys, whereas USV-based data can provide comparable results regarding species identification. This has the advantage of adding accurate spatial information that can help address other research questions and monitoring goals.
However, without using an image segmentation approach, there are several technical challenges that scholars need to be fully aware of, including extensive processing time to train the classifiers and define the training sample, as well as the long and complex post-processing routine linked to more ‘salt-and-pepper’ artefacts due to spurious pixel classifications (Figure 11). Therefore, for extensive scale assessment where broad classification is needed, it is advisable to reduce the amount of computer memory available per processing unit by smoothing the spectral complexity of UAV imagery, before training the classifier. This step becomes mandatory for the imagery acquired using the USV due to their ultra-high spatial resolution. Even though after MS segmentation of USV-based imagery, small spectral difference among similar classes are too smoothed, making impossible to distinguish species with similar colours (e.g., Dictyotales from Sphacelariales), we performed a very detailed habitat characterisation of the deeper rocky bottoms by identifying the patchy algal assemblages. The OBIA processing applied in ArcMap can be substituted with other approaches available in the eCognition software (Trimble Geospatial Imaging, Munich, Germany), which has a powerful multi-resolution algorithm for image segmentation capable of reducing computational complexity and processing times compared to MS segmentation (Fu et al., 2013). Moreover, the lengthy trial-and-error processing time required to configure the suitable parameters for image segmentation can be reduced by enabling a more statistically-based solution for selecting scale parameters (Drăguţ et al., 2014).
Figure 11 Processing times (in hours) of the main steps used in this work to classify high (UAV-based) and ultra-high (USV-based) imagery.
Another primary aspect to investigate during monitoring campaigns is the presence of organisms with a key role in benthic communities. Research on target species, such as herbivorous, deposit-feeders or filter-feeders, is critical for the development of conservation and management measures of coastal areas since they play a vital role in the sea floor dynamics (Boncagni et al., 2019; Morroni et al., 2020; Grosso et al., 2022; Pensa et al., 2022). When the research is focused on benthic organisms living in shallow rocky-bottom areas, data collection through SCUBA diving and snorkelling has traditionally been the most widely chosen method. However, among recent benthic sampling techniques, remote photographic recording methods offer a variety of advantages by reducing the time spent by divers underwater and logistics costs (Piazza et al., 2019). More specifically, photogrammetric outputs such as 2D plots or transects cropped from large orthophotos can be usable in software specifically dedicated to the elaboration of benthic images (e.g., PhotoQuad, Seascape, CPC, ImageJ), enabling the analysis and comparison of defined seabed areas to investigate changes in the benthic communities over time (Piazza et al., 2019). In this context, the adoption of the integrated approach with UAV and USV to evaluate any modification due to small-scale habitat variability, registering the position of sessile specimens (e.g., algae, bryozoans, sponges, bivalves) and determining the abundance, the distribution, and the aggregation patterns of vagile fauna (e.g., sea urchins, sea cucumbers, sea stars and epimegabenthos assemblages in general), is unprecedented and fulfils the main aim of fine-scale monitoring programmes.
Although our approach can provide a fast, low-cost, and accurate method for fine-scale mapping of shallow benthic underwater habitats and other above-water coastal features of ecological interest, several drawbacks should be considered when field operations are carried out in sub-optimal conditions. In fact, we performed UAV imagery acquisition with calm sea conditions with wind speed < 1 knot (Beaufort scale = 0) and in very shallow waters where moderate water turbidity have a limited effect on light penetration (mean measured value of Secchi disk transparency = 4.2 ± 0.3 m). However, along the deeper edges of the mapped area, at depths > 3 m, the imagery was more affected by transparency, resulting in a less sharp identification of seagrassmeadows patches which also involved image alignment issues during photogrammetric processing. Sea conditions and weather are also key factors to be carefully evaluated during USV operation. This tool is highly promising in shallow lagoons and back-reef monitoring, although in open coastal areas are necessary low hydrodynamic conditions for optimal imagery acquisition, due to limited stability and manoeuvrability. However, the distance from the seafloor of the camera sensor mounted on USV can be an important limitation in obtaining reliable models in very shallow waters (< 1 m).
Seasonality can also play a crucial role in achieving reliable maps for thematic cartography because macroalgae assemblages can considerably vary over seasons (e.g., summer vegetative phase versus wintry quiescence period of Cystoseira spp. that may lead to the impossibility of thalli detection). Significant accumulation of phanerogamic detritus (dead P. oceanica leaves) can affect the mapping of both above-water features (covered by thick banquettes layers) and below-water substrata (by depositing in sandy patches and holes inside rocky reef) in winter. Regarding aerial and surface platforms configuration most limiting factors for using such tools over large areas are primarily due to the LiPo battery that implies short-medium (< 30 min) flight times. This aspect is less evident for USV platforms which are capable of slow navigation over a longer time (> 2 hours).
Understanding heterogeneity within benthic habitats is a primary step for the environmental monitoring of perturbed and pristine coastal ecosystems. Drones open the possibility to capture data that is useful to finely depict hard bottoms assemblages, seagrass and other above-water features (e.g., banquette, artificial structures), which can play a crucial role in coastal dynamics. Traditional boundary mapping has often been conducted using satellite and airborne imagery. Still, it can be considerably improved with UAV-based imagery, highlighting the need to investigate the fragmentation within meadows, which can provide researchers, stakeholders and ecosystem managers with data on whether a meadow is potentially deteriorating or recovering (Duffy et al., 2018). The combination of aerial and underwater imagery provides high-quality and cost-effective data that can be stored for assessment over time, offering valuable information that can be used in the case of retrospective analyses.
Potential improvements to the methods described here are extensive, including using fixed-wing platforms for increased flight efficiency and spatial coverage, enhanced imaging acquisition (multispectral sensors), and positioning (direct imagery georeferencing with RTK systems) techniques. Finally, applying powerful algorithms for image segmentation and classification capable of processing large datasets will be highly informative in aquatic system management and decision-making, showing great promise in applying UAV and USV high spatial resolution imagery for shallow benthic habitats conservation and monitoring.
The raw data supporting the conclusions of this article will be made available by the authors, without undue reservation.
DV: conceptualization, methodology, field investigation, data analysis, writing original draft. LG, DP: field investigation, writing—review and editing. EC, GM, TV, MS: writing—review and editing. AR: conceptualization, methodology, field investigation, writing—review and editing, project administration. All authors contributed to the article and approved the submitted version.
We thank Antonio d’Argenio, Enrico Iuliano from Strumenti Topografici (GEC Software company) and Aleksandr Levchik from Topodrone team for their support during UAV configuration and PPK processing.
The authors declare that the research was conducted in the absence of any commercial or financial relationships that could be construed as a potential conflict of interest.
All claims expressed in this article are solely those of the authors and do not necessarily represent those of their affiliated organizations, or those of the publisher, the editors and the reviewers. Any product that may be evaluated in this article, or claim that may be made by its manufacturer, is not guaranteed or endorsed by the publisher.
The Supplementary Material for this article can be found online at: https://www.frontiersin.org/articles/10.3389/fmars.2022.1096594/full#supplementary-material
Airoldi L., Rindi F., Cinelli F. (1995). Structure, seasonal dynamics and reproductive phenology of a filamentous turf assemblage on a sediment influenced, rocky subtidal shore. Botanica Marina 38, 227–237. doi: 10.1515/botm.1995.38.1-6.227
Alkan M. (2018). Information content analysis from very high resolution optical space imagery for updating spatial database. Int. Arch. Photogramm. Remote Sens. Spat. Inf. Sci. 42, 4. doi: 10.5194/isprs-archives-XLII-4-25-2018
Anderson K., Gaston K. J. (2013). Lightweight unmanned aerial vehicles will revolutionize spatial ecology. Front. Ecol. Environ. 11, 138–146. doi: 10.1890/120150
Benedetti-Cecchi L., Cinelli F. (1995). Habitat heterogeneity, sea urchin grazing and the distribution of algae in littoral rock pools on the west coast of Italy (western Mediterranean). Mar. Ecol. Prog. Ser. 126, 203–212. doi: 10.3354/meps126203
Boncagni P., Rakaj A., Fianchini A., Vizzini S. (2019). Preferential assimilation of seagrass detritus by two coexisting Mediterranean sea cucumbers: Holothuria polii and holothuria tubulosa. Estuar. Coast. Shelf Sci. 231, 106464. doi: 10.1016/j.ecss.2019.106464
Borfecchia F., Consalvi N., Micheli C., Carli F. M., Cognetti De Martiis S., Gnisci V., et al. (2019). Landsat 8 OLI satellite data for mapping of the posidonia oceanica and benthic habitats of coastal ecosystems. Int. J. Remote Sens. 40, 1548–1575. doi: 10.1080/01431161.2018.1528020
Boudouresque C. F., Bernard G., Pergent G., Shili A., Verlaque M. (2009). Regression of Mediterranean seagrasses caused by natural processes and anthropogenic disturbances and stress: A critical review. Bot. Mar. 52, 395–418. doi: 10.1515/BOT.2009.057
Bryant D., Rodenburg E., Cox T., Nielsen D. (1995). Coastlines at risk: An index of potential development-related threats to coastal ecosystems. World Resour. Institute. 8.
Burns J. H. R. R., Delparte D., Gates R. D., Takabayashi M. (2015). Integrating structure-from-motion photogrammetry with geospatial software as a novel technique for quantifying 3D ecological characteristics of coral reefs. PeerJ 3, e1077. doi: 10.7717/peerj.1077
Burns J. H. R., Fukunaga A., Pascoe K. H., Runyan A., Craig B. K., Talbot J., et al. (2019). 3D habitat complexity of coral reefs in the northwestern Hawaiian islands is driven by coral assemblage structure. Int. Arch. Photogramm. Remote Sens. Spatial Inf. Sci. XLII-2/W10, 61–67. doi: 10.5194/isprs-archives-XLII-2-W10-61-2019
Castellanos-Galindo G. A., Casella E., Mejía-Rentería J. C., Rovere A. (2019). Habitat mapping of remote coasts: Evaluating the usefulness of lightweight unmanned aerial vehicles for conservation and monitoring. Biol. Conserv. 239, 108282. doi: 10.1016/j.biocon.2019.108282
Chand S., Bollard B. (2021). Detecting the spatial variability of seagrass meadows and their consequences on associated macrofauna benthic activity using novel drone technology. Remote Sens. 14, 160. doi: 10.3390/rs14010160
Chefaoui R. M., Duarte C. M., Serrão E. A. (2018). Dramatic loss of seagrass habitat under projected climate change in the Mediterranean Sea. Glob. Change Biol. 24, 4919–4928. doi: 10.1111/gcb.14401
Cheminée A., Le Direach L., Rouanet E., Astruch P., Goujard A., Blanfuné A., et al. (2021). All shallow coastal habitats matter as nurseries for Mediterranean juvenile fish. Sci. Rep. 11, 14631. https://doi.org/10.1038/s41598-021-93557-2
Cheminée A., Pastor J., Bianchimani O., Thiriet P., Sala E., Cottalorda J. M., et al. (2017). Juvenile fish assemblages in temperate rocky reefs are shaped by the presence of macro-algae canopy and its three-dimensional structure. Sci. Rep. 7, 14638. doi: 10.1038/s41598-017-15291-y
Cheminée A., Sala E., Pastor J., Bodilis P., Thiriet P., Mangialajo L., et al. (2013). Nursery value of cystoseira forests for Mediterranean rocky reef fishes. J. Exp. Mar. Bio. Ecol. 442, 70–79. doi: 10.1016/j.jembe.2013.02.003
Claudet J., Fraschetti S. (2010). Human-driven impacts on marine habitats: A regional meta-analysis in the Mediterranean Sea AN - prod.academic_MSTAR_814234566; 13249761. Biol. Conserv. 143, 2195–2206. doi: 10.1016/j.biocon.2010.06.004
Coll M., Piroddi C., Albouy C., Ben Rais Lasram F., Cheung W. W. L., Christensen V., et al. (2012). The Mediterranean Sea under siege: Spatial overlap between marine biodiversity, cumulative threats and marine reserves. Glob. Ecol. Biogeogr. 21, 465–480. doi: 10.1111/j.1466-8238.2011.00697.x
Courrat A., Lobry J., Nicolas D., Laffargue P., Amara R., Lepage M., et al. (2009). Anthropogenic disturbance on nursery function of estuarine areas for marine species. Estuar. Coast. Shelf Sci. 81, 179–190. doi: 10.1016/j.ecss.2008.10.017
Dauvin J.-C., Alizier S., Rolet C., Bakalem A., Bellan G., Gesteira J. L. G., et al. (2012). Response of different benthic indices to diverse human pressures. Ecol. Indic. 12, 143–153. doi: 10.1016/j.ecolind.2011.03.019
De Reu J., Plets G., Verhoeven G., De Smedt P., Bats M., Cherretté B., et al. (2013). Towards a three-dimensional cost-effective registration of the archaeological heritage. J. Archaeol. Sci. 40, 1108–1121. doi: 10.1016/j.jas.2012.08.040
Diefenbacher E. (2022). Notes on the operation of two types of aquatic remotely operated vehicles used during a mock turtle survey. J. North Am. Herpetol. 2022. 1, 20–23.
Dokulil M. T. (2003). “Algae as ecological bio-indicators,“. Trace metals other contaminants Environ. (Elsevier) 285–327. doi: 10.1016/S0927-5215(03)80139-X
Drăguţ L., Csillik O., Eisank C., Tiede D. (2014). Automated parameterisation for multi-scale image segmentation on multiple layers. ISPRS J. Photogramm. Remote Sens. 88, 119–127. doi: 10.1016/j.isprsjprs.2013.11.018
Droppova V. (2011). The tools of automated generalization and building generalization in an ArcGIS environment. Slovak J. Civ. Eng. 19, 1. doi: 10.2478/v10189-011-0001-4
Duarte C. M. (2002). The future of seagrass meadows. Environ. Conserv. 29, 192–206. doi: 10.1017/S0376892902000127
Duarte B., Martins I., Rosa R., Matos A. R., Roleda M. Y., Reusch T. B. H., et al. (2018). Climate change impacts on seagrass meadows and macroalgal forests: an integrative perspective on acclimation and adaptation potential. Front. Mar. Sci. 5, 190. doi: 10.3389/fmars.2018.00190
Duffy J. P., Pratt L., Anderson K., Land P. E., Shutler J. D. (2018). Spatial assessment of intertidal seagrass meadows using optical imaging systems and a lightweight drone. Estuar. Coast. Shelf Sci. 200, 169–180. doi: 10.1016/j.ecss.2017.11.001
Fabbrizzi E., Scardi M., Ballesteros E., Benedetti-Cecchi L., Cebrian E., Ceccherelli G., et al. (2020). Modeling macroalgal forest distribution at Mediterranean scale: Present status, drivers of changes and insights for conservation and management. Front. Mar. Sci. 7, 20. doi: 10.3389/fmars.2020.00020
Fallati L., Saponari L., Savini A., Marchese F., Corselli C., Galli P. (2020). Multi-temporal UAV data and object-based image analysis (OBIA) for estimation of substrate changes in a post-bleaching scenario on a maldivian reef. Remote Sens. 12, 2093. doi: 10.3390/rs12132093
Fu G., Zhao H., Li C., Shi L. (2013). Segmentation for high-resolution optical remote sensing imagery using improved quadtree and region adjacency graph technique. Remote Sens. 5, 3259–3279. doi: 10.3390/rs5073259
Gaw L. Y.-F., Yee A. T. K., Richards D. R. (2019). A high-resolution map of singapore’s terrestrial ecosystems. Data 4, 116. https://doi.org/10.3390/data4030116
Gravina M. F. M. F., Bonifazi A., Del Pasqua M., Giampaoletti J., Lezzi M., Ventura D., et al. (2020). Perception of changes in marine benthic habitats: The relevance of taxonomic and ecological memory. Diversity 12, 480. doi: 10.3390/d12120480
Grosso L., Rakaj A., Fianchini A., Tancioni L., Vizzini S., Boudouresque C., et al. (2022). Trophic requirements of the Sea urchin paracentrotus lividus varies at different life stages: Comprehension of species ecology and implications for effective feeding formulations. Front. Mar. Sci. 9, 865450. doi: 10.3389/fmars.2022.865450
Hardin P. J., Hardin T. J. (2010). Small-scale remotely piloted vehicles in environmental research. Geogr. Compass 4, 1297–1311. doi: 10.1111/j.1749-8198.2010.00381.x
Harmelin-Vivien M. L., Harmelin J. G., Leboulleux V. (1995). Microhabitat requirements for settlement of juvenile sparid fishes on Mediterranean rocky shores. Hydrobiologia, 300, 309–320. doi: 10.1007/BF00024471
Heck K. L. Jr., Hays G., Orth R. J., Heck K. L., Hays G., Orth R. J. (2003). Critical evaluation of the nursery role hypothesis for seagrass meadows. Mar. Ecol. Prog. Ser. 253, 123–136. doi: 10.3354/meps253123
Heumann B. W. (2011). An object-based classification of mangroves using a hybrid decision tree–support vector machine approach. Remote Sens. 3, 2440–2460. doi: 10.3390/rs3112440
Kabiri K. (2020). Mapping coastal ecosystems and features using a low-cost standard drone: case study, nayband bay, Persian gulf, Iran. J. Coast. Conserv. 24, 1–8. doi: 10.1007/s11852-020-00780-6
Kislik C., Genzoli L., Lyons A., Kelly M. (2020). Application of UAV imagery to detect and quantify submerged filamentous algae and rooted macrophytes in a non-wadeable river. Remote Sens. 12, 1–24. doi: 10.3390/rs12203332
Lakshmi A., Rajagopalan R. (2000). Socio-economic implications of coastal zone degradation and their mitigation: a case study from coastal villages in India. Ocean Coast. Manage. 43, 749–762. doi: 10.1016/S0964-5691(00)00057-0
Lechner A. M., Fletcher A., Johansen K., Erskine P. (2012). Characterising upland swamps using object-based classification methods and hyper-spatial resolution imagery derived from an unmanned aerial vehicle. ISPRS Ann. Photogramm. Remote Sens. Spat. Inf. Sci., I–4, 101–106. doi: 10.5194/isprsannals-I-4-101-2012
Lee I.-C., Wu B., Li R. (2009). Shoreline extraction from the integration of lidar point cloud data and aerial orthophotos using mean-shift segmentation. in. Proc. ASPRS Annu. Conf, 2, 3033–3040.
Leriche A., Pasqualini V., Boudouresque C.-F., Bernard G., Bonhomme P., Clabaut P., et al. (2006). Spatial, temporal and structural variations of a posidonia oceanica seagrass meadow facing human activities. Aquat. Bot. 84, 287–293. doi: 10.1016/j.aquabot.2005.10.001
Letourneur Y., Ruitton S., Sartoretto S. (2003). Environmental and benthic habitat factors structuring the spatial distribution of a summer infralittoral fish assemblage in the north-western Mediterranean Sea. J. Mar. Biol. Assoc. United Kingdom 83, 193–204. doi: 10.1017/S0025315403006970h
Levin P. S., Kelble C. R., Shuford R. L., Ainsworth C., deReynier Y., Dunsmore R., et al. (2014). Guidance for implementation of integrated ecosystem assessments: a US perspective. ICES J. Mar. Sci. 71, 1198–1204. doi: 10.1093/icesjms/fst112
Marre G., Deter J., Holon F., Boissery P., Luque S. (2020). Fine-scale automatic mapping of living posidonia oceanica seagrass beds with underwater photogrammetry. Mar. Ecol. Prog. Ser. 643, 63–74. doi: 10.3354/meps13338
Marre G., Holon F., Luque S., Boissery P., Deter J. (2019). Monitoring marine habitats with photogrammetry: A cost-effective, accurate, precise and high-resolution reconstruction method. Front. Mar. Sci. 6. doi: 10.3389/fmars.2019.00276
Martin C. S., Giannoulaki M., De Leo F., Scardi M., Salomidi M., Knitweiss L., et al. (2014). Coralligenous and maërl habitats: predictive modelling to identify their spatial distributions across the Mediterranean Sea. Sci. Rep. 4, 1–8. doi: 10.1038/srep05073
Morris R. L., Graham T. D. J., Kelvin J., Ghisalberti M., Swearer S. E. (2020). Kelp beds as coastal protection: wave attenuation of ecklonia radiata in a shallow coastal bay. Ann. Bot. 125, 235–246. https://doi.org/10.1093/aob/mcz127
Morroni L., Rakaj A., Grosso L., Fianchini A., Pellegrini D., Regoli F. (2020). Sea Cucumber holothuria polii (Delle chiaj) as new model for embryo bioassays in ecotoxicological studies. Chemosphere 240, 124819. doi: 10.1016/j.chemosphere.2019.124819
Mumby P. J., Edwards A. J. (2002). Mapping marine environments with IKONOS imagery: enhanced spatial resolution can deliver greater thematic accuracy. Remote Sens. Environ. 82, 248–257. doi: 10.1016/S0034-4257(02)00041-X
Nagelkerken I., Sheaves M., Baker R., Connolly R. M. (2015). The seascape nursery: a novel spatial approach to identify and manage nurseries for coastal marine fauna. Fish Fish. 16, 362–371. doi: 10.1111/faf.12057
Nieuwenhuis B. O., Marchese F., Casartelli M., Sabino A., van der Meij S. E. T., Benzoni F. (2022). Integrating a UAV-derived DEM in object-based image analysis increases habitat classification accuracy on coral reefs. Remote Sens. 14, 5017. doi: 10.3390/rs14195017
Nikolakopoulos K. G., Kozarski D., Kogkas S. (2017). Coastal areas mapping using UAV photogrammetry. Earth Resour. Environ. Remote Sensing/GIS Appl. VIII (SPIE) 10428, 104280O. doi: 10.1117/12.2278121
O’Connor N. E. (2013). Impacts of sewage outfalls on rocky shores: Incorporating scale, biotic assemblage structure and variability into monitoring tools. Ecol. Indic. 29, 501–509. doi: 10.1016/j.ecolind.2013.01.020
Parravicini V., Rovere A., Vassallo P., Micheli F., Montefalcone M., Morri C., et al. (2012). Understanding relationships between conflicting human uses and coastal ecosystems status: a geospatial modeling approach. Ecol. Indic. 19, 253–263. doi: 10.1016/j.ecolind.2011.07.027
Pensa D., Fianchini A., Grosso L., Ventura D., Scardi M., Rakaj A. (2022). Tracking population status and structure of Mediterranean pen shell pinna nobilis after a mass mortality outbreak. npj biodivers 1, 3. doi: 10.21203/rs.3.rs-1425249/v1
Pergent-Martini C., Monnier B., Lehmann L., Barralon E., Pergent G. (2022). Major regression of posidonia oceanica meadows in relation with recreational boat anchoring: A case study from Sant’Amanza bay. J. Sea Res. 188, 102258. doi: 10.1016/j.seares.2022.102258
Piazza P., Cummings V., Guzzi A., Hawes I., Lohrer A., Marini S., et al. (2019). Underwater photogrammetry in Antarctica: Long-term observations in benthic ecosystems and legacy data rescue. Polar Biol. 42, 1061–1079. doi: 10.1007/s00300-019-02480-w
Pipaud I., Lehmkuhl F. (2017). Object-based delineation and classification of alluvial fans by application of mean-shift segmentation and support vector machines. Geomorphology 293, 178–200. doi: 10.1016/j.geomorph.2017.05.013
Prado E., Rodríguez-Basalo A., Cobo A., Ríos P., Sánchez F. (2020). 3D fine-scale terrain variables from underwater photogrammetry: A new approach to benthic microhabitat modeling in a circalittoral rocky shelf. Remote Sens. 12, 2466. doi: 10.3390/rs12152466
Price D. M., Robert K., Callaway A., Lo lacono C., Hall R. A., Huvenne V. A. I. (2019). Using 3D photogrammetry from ROV video to quantify cold-water coral reef structural complexity and investigate its influence on biodiversity and community assemblage. Coral Reefs 38, 1007–1021. doi: 10.1007/s00338-019-01827-3
Rakaj A., Morroni L., Grosso L., Fianchini A., Pensa D., Pellegrini D., et al. (2021). Towards sea cucumbers as a new model in embryo-larval bioassays: Holothuria tubulosa as test species for the assessment of marine pollution. Sci. Total Environ. 787, 147593. doi: 10.1016/j.scitotenv.2021.147593
Raoult V., Gaston T. F. (2018). Rapid biomass and size-frequency estimates of edible jellyfish populations using drones. Fish. Res. 207, 160–164. doi: 10.1016/j.fishres.2018.06.010
Raoult V., Reid-Anderson S., Ferri A., Williamson J. (2017). How reliable is structure from motion (SfM) over time and between observers? a case study using coral reef bommies. Remote Sens. 9, 740. doi: 10.3390/rs9070740
Raoult V., Tosetto L., Williamson J. E. (2018). Drone-based high-resolution tracking of aquatic vertebrates. Drones 2, 37. doi: 10.3390/drones2040037
Rende S. F., Bosman A., Di Mento R., Bruno F., Lagudi A., Irving A. D., et al. (2020). Ultra-High-Resolution mapping of posidonia oceanica (L.) delile meadows through acoustic, optical data and object-based image classification. J. Mar. Sci. Eng. 8, 647. https://doi.org/10.3390/jmse8090647
Roelfsema C. M., Phinn S. R. (2010). Integrating field data with high spatial resolution multispectral satellite imagery for calibration and validation of coral reef benthic community maps. J. Appl. Remote Sens. 4, 43527. doi: 10.1117/1.3430107
Sales M., Ballesteros E., Anderson M. J., Iveša L., Cardona E. (2012). Biogeographical patterns of algal communities in the Mediterranean Sea: Cystoseira crinita-dominated assemblages as a case study. J. Biogeogr. 39, 140–152. doi: 10.1111/j.1365-2699.2011.02564.x
Seytre C., Francour P. (2014). A long-term survey of posidonia oceanica fish assemblages in a Mediterranean marine protected area: emphasis on stability and no-take area effectiveness. Mar. Freshw. Res. 65, 244–254. doi: 10.1071/MF13080
Shintani C., Fonstad M. A. (2017). Comparing remote-sensing techniques collecting bathymetric data from a gravel-bed river. Int. J. Remote Sens. 38, 2883–2902. doi: 10.1080/01431161.2017.1280636
Taddia Y., Stecchi F., Pellegrinelli A. (2019). Using DJI phantom 4 RTK drone for topographic mapping of coastal areas. Int. Arch. Photogramm. Remote Sens. Spat. Inf. Sci. 42, 625–630. https://doi.org/10.5194/isprs-archives-XLII-2-W13-625-2019
Tait L. W., Orchard S., Schiel D. R. (2021). Missing the forest and the trees: Utility, limits and caveats for drone imaging of coastal marine ecosystems. Remote Sens. 13, 3136. doi: 10.3390/rs13163136
Takasu T., Yasuda A. (2009). “Development of the low-cost RTK-GPS receiver with an open source program package RTKLIB,” in International symposium on GPS/GNSS (Jeju Korea: International Convention Center).
Telesca L., Belluscio A., Criscoli A., Ardizzone G., Apostolaki E. T., Fraschetti S., et al. (2015). Seagrass meadows (Posidonia oceanica) distribution and trajectories of change. Sci. Rep. 5, 1–14. doi: 10.1038/srep12505
Ventura D., Bonifazi A., Gravina M. F., Belluscio A., Ardizzone G. (2018). Mapping and classification of ecologically sensitive marine habitats using unmanned aerial vehicle (UAV) imagery and object-based image analysis (OBIA). Remote Sens. 10, 1331. doi: 10.3390/rs10091331
Ventura D., Dubois S. F. S. F., Bonifazi A., Jona Lasinio G., Seminara M., Gravina M. F. M. F. M. F., et al. (2020). Integration of close-range underwater photogrammetry with inspection and mesh processing software: a novel approach for quantifying ecological dynamics of temperate biogenic reefs. Remote Sens. Ecol. Conserv. 7, 169–186. doi: 10.1002/rse2.178
Ventura D., Jona Lasinio G., Ardizzone G. (2015). Temporal partitioning of microhabitat use among four juvenile fish species of the genus diplodus (Pisces: Perciformes, sparidae). Mar. Ecol. 36, 1013–1032. doi: 10.1111/maec.12198
Ventura D., Mancini G., Casoli E., Pace D. S. D. S., Lasinio G. J. G. J., Belluscio A., et al. (2022). Seagrass restoration monitoring and shallow-water benthic habitat mapping through a photogrammetry-based protocol. J. Environ. Manage. 304, 114262. doi: 10.1016/j.jenvman.2021.114262
Williamson J. E., Duce S., Joyce K. E., Raoult V. (2021). Putting sea cucumbers on the map: projected holothurian bioturbation rates on a coral reef scale. Coral Reefs 40, 559–569. doi: 10.1007/s00338-021-02057-2
Yang B., Hawthorne T. L., Hessing-Lewis M., Duffy E. J., Reshitnyk L. Y., Feinman M., et al. (2020). Developing an introductory UAV/drone mapping training program for seagrass monitoring and research. Drones 4, 70. doi: 10.3390/drones4040070
Young G. C., Dey S., Rogers A. D., Exton D. (2017). Cost and time-effective method for multiscale measures of rugosity, fractal dimension, and vector dispersion from coral reef 3D models. PloS One 12, 1–18. doi: 10.1371/journal.pone.0175341
Zapata-Ramírez P., Scaradozzi D., Sorbi L., Palma M., Pantaleo U., Ponti M., et al. (2013). Innovative study methods for the Mediterranean coralligenous habitats. Adv. Oceanogr. Limnol. 4, 102–119. doi: 10.1080/19475721.2013.849758
Keywords: SfM photogrammetry, seagrass, algal assemblages, cartography, GIS, OBIA, unmanned aerial vehicles (UAV), unmanned surface vehicles (USV)
Citation: Ventura D, Grosso L, Pensa D, Casoli E, Mancini G, Valente T, Scardi M and Rakaj A (2023) Coastal benthic habitat mapping and monitoring by integrating aerial and water surface low-cost drones. Front. Mar. Sci. 9:1096594. doi: 10.3389/fmars.2022.1096594
Received: 12 November 2022; Accepted: 30 December 2022;
Published: 13 January 2023.
Edited by:
Monica Montefalcone, University of Genoa, ItalyReviewed by:
Luca Fallati, University of Milano-Bicocca, ItalyCopyright © 2023 Ventura, Grosso, Pensa, Casoli, Mancini, Valente, Scardi and Rakaj. This is an open-access article distributed under the terms of the Creative Commons Attribution License (CC BY). The use, distribution or reproduction in other forums is permitted, provided the original author(s) and the copyright owner(s) are credited and that the original publication in this journal is cited, in accordance with accepted academic practice. No use, distribution or reproduction is permitted which does not comply with these terms.
*Correspondence: Daniele Ventura, ZGFuaWVsZS52ZW50dXJhQHVuaXJvbWExLml0
Disclaimer: All claims expressed in this article are solely those of the authors and do not necessarily represent those of their affiliated organizations, or those of the publisher, the editors and the reviewers. Any product that may be evaluated in this article or claim that may be made by its manufacturer is not guaranteed or endorsed by the publisher.
Research integrity at Frontiers
Learn more about the work of our research integrity team to safeguard the quality of each article we publish.