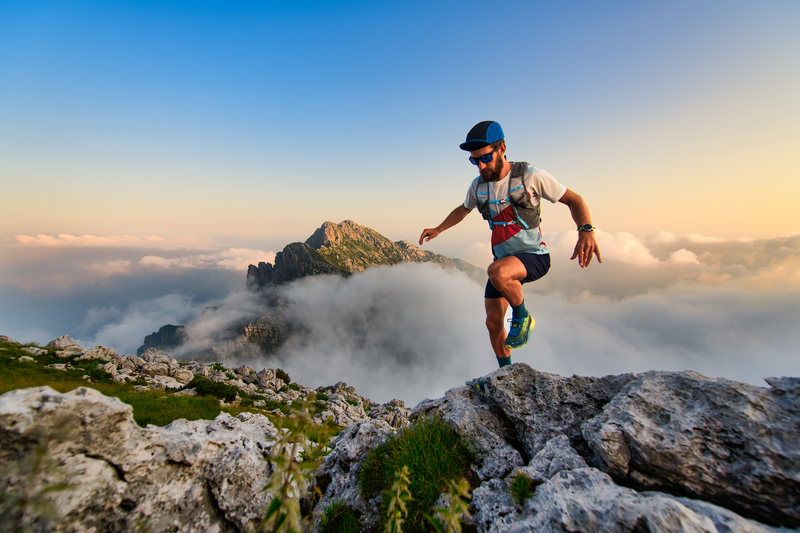
95% of researchers rate our articles as excellent or good
Learn more about the work of our research integrity team to safeguard the quality of each article we publish.
Find out more
ORIGINAL RESEARCH article
Front. Mar. Sci. , 16 January 2023
Sec. Coastal Ocean Processes
Volume 9 - 2022 | https://doi.org/10.3389/fmars.2022.1086775
This article is part of the Research Topic Coastal Ecotones under Anthropogenic Influences: Conservation, Restoration and Realignment View all 14 articles
As a crucial coastal wetland habitat in the transition zone between land and sea, global tidal flats have severely declined by 16% over the last two decades under the dual threats of intense human activities and climate change. The Yangtze River Delta of China, the largest estuary in the western Pacific Ocean, has abundant mudflat resources and a dense human population. It also has some of the most prominent conflicts between economic development and ecological conservation. The current lack of understanding of landscape patterns and influencing factors of the Yangtze River Delta mudflats has severely hampered the region’s ecological conservation and restoration efforts. Based on Landsat time-series images, this study generated a 30-m spatial resolution map of mudflats in the Yangtze River Delta, which shrank by 47% during 1990–2020, with a higher density of mudflat loss in Yancheng and Nantong cities of the Jiangsu province and Hangzhou, Shaoxing, and Ningbo cities of the Zhejiang province. Landscape indices, such as the patch density of tidal flats, have gradually changed since 2000, with most of them showing significant changes in 2010. Mudflats in Lianyungang, northwestern Yancheng, Nanhui, Jiaxing, and Hangzhou showed sharp negative changes in landscape characteristics. Natural and anthropogenic factors had synergistic effects on the above changes in mudflat landscape patterns in the Yangtze River Delta. Mudflat landscape features were mainly influenced by population growth, economic development, reclamation, sediment discharge, and air temperature. Based on the evolving characteristics of mudflat landscape patterns, we recommend improving mudflat landscape management and planning by strengthening mudflat policies, laws, and regulations, developing countermeasures against threats from major stressors, and enhancing the effectiveness of nature reserves for mudflat protection.
Coastal wetlands, including coastal vegetation areas and tidal flats, are transition zones between marine and terrestrial ecosystems (Wang et al., 2020; Song et al., 2021). Tidal flats are an essential part of highly productive coastal zones, which contain abundant mineral, biological, and marine resources (Hill et al., 2021). They provide essential biodiversity maintenance services, serving as energy supply stations for water birds and spawning and nursery grounds for fish and invertebrates (Murray et al., 2014; Ghosh et al., 2016; Jackson et al., 2021). Meanwhile, tidal flats also play an essential role in storm protection, shoreline stabilization, nutrient cycling, as well as carbon storage and sequestration (Barbier et al., 2011; Spalding et al., 2014; Jin et al., 2017; Li et al., 2020b; Lin et al., 2020). However, tidal flats are one of the most vulnerable ecosystems along the coast, under intense pressure from human interference and natural disturbances (Rodriguez et al., 2017; Murray et al., 2022). A worldwide crisis of tidal flat degradation has taken place, with tidal flats falling by 16% globally over the past 20 years (Murray et al., 2019; Lin et al., 2020). Understanding the spatial and temporal changes in tidal landscapes and their drivers is critical for tidal flat conservation (Zahran et al., 2006; Ma et al., 2014; Larson, 2015).
With their dynamic processes, tidal flats are relatively independent ecosystems, but they also depend on surrounding landscapes for the exchange of materials and energy (Mitsch, 1994). Patterns of tidal landscapes refer to the quantity, attributes, geospatial distribution, type conversion, and connectivity of tidal resources, and are related to the resistance to disturbance, resilience, stability, and biodiversity of tidal ecosystems (Zhang et al., 2017; Yang et al., 2021; Zhang et al., 2021). The landscape pattern of tidal flats is constantly changing and evolving. As the expression of dynamic changes in tidal flats, landscape pattern evolution is a comprehensive spatial representation, indicating not only the changing extent of tidal flats and changes in landscape types but also changes in their evolutionary rates and ecological functions (Kahara et al., 2009; Bai et al., 2013). The landscape pattern evolution of tidal flats reflects a combination of different ecological progress and human activities acting upon the tidal flats (Cao, 2008; Huang et al., 2012). Thus, landscape pattern analysis can help understand the rules and mechanisms needed for the conservation of tidal flats.
On the southeastern coast of China, the Yangtze River Delta (YRD) is an ecologically important area with extensive tidal flats (Han and Ma, 2021; Zhang et al., 2022). The YRD is also one of China’s most developed regions, subjected to rapid urbanization and stressed by human activities (Du et al., 2016; Sun et al., 2016). The YRD is, therefore, essential for the sustainable development of regional ecology and economics. Tidal flats in the YRD are crucial for biodiversity conservation, acting as critical habitats for birds migrating between East Asia and Australia (Jackson et al., 2021). However, due to a combination of natural environmental succession and human activities, the tidal flats in the YRD have experienced a noticeable degradation (Wang et al., 2021b). Given the YRD’s strategic position in China, tracking landscape dynamics and identifying the corresponding drivers of tidal flat changes are of great importance for the conservation of tidal flats and the sustainability of coastal development.
Changes in tidal flats and their driving factors have received increasing attention in recent years (Chen et al., 2016; Li et al., 2020b; Wang et al., 2021b). Globally, the distribution and trajectory of tidal flats have been mapped using satellite images and machine-learning technology (Murray et al., 2019). The drivers of tidal flats dynamics mainly include anthropogenic disturbance through coastal development projects (e.g., road construction), which leads to tidal flat degradation and reduction of biodiversity (Reimer et al., 2015). Within China, reclamation of coastal wetlands for urban construction and agriculture in the Yellow River Delta has been shown to have destructive effects on coastal ecosystems (Murray et al., 2014). Over the past three decades, the driving forces of tidal flat evolution have included factors such as river sand transport and afforestation (Wang et al., 2021b). Studies focusing on the YRD examined the trend of tidal flats in the Yangtze estuary and their extent and classification, and also assessed the potential impacts of various estuarine projects such as land reclamation and sedimentation on mudflats (Chen et al., 2016; Zhang et al., 2019; Li et al., 2020b). It concluded that land reclamation and coastal development were the major drivers of tidal flat loss in the YRD (Wang et al., 2020). However, few studies have examined the landscape pattern dynamics of the YRD tidal flats, implying a lack of information on variations of the tidal flats’ landscape patterns and their causal responses to driving factors. By analyzing the landscape patterns of the tidal flats and their drivers over time, we can gain insight into the environment of the YRD and how to develop guidelines for protecting and assessing tidal flats.
In this study, we combined the latest remote sensing and ecological datasets, integrating remote sensing (RS), geographic information system (GIS), landscape dynamic analysis, landscape pattern metrics, and mathematical statistics, in an attempt to (1) determine the distribution of tidal flats between 1990 and 2020 by remote sensing cloud computing; (2) investigate the evolutionary processes of landscape patterns of the YRD tidal flats and quantitatively identify the drivers. Our research aims to improve our understanding of the landscape patterns of tidal flats and explore possible pathways for improved conservation measures that will ultimately help achieve sustainable development in the YRD region.
This study determined the geographic location of coastal tidal flats based on existing definitions of tidal flats (Murray et al., 2019; Zhang et al., 2019; Wang et al., 2020). In accordance with the concept of intertidal zones as stated in the Comprehensive Survey of China’s Coastal Zones and Tideland Resources, and considering the topography and coastal type of the YRD, we delineated a remote sensing monitoring area for the coastal tidal flats as the zone extending 10 km from land to sea, from using the Open Street Map (OSM) as a benchmark (29°N–35°N, 119°E–23°E) (Figure 1). Hangzhou Bay is the natural line of distinction between the northern and southern coastal wetlands of China (Sun et al., 2016; Zhang et al., 2019). Therefore, the boundary of this study extended from Hangzhou Bay of Zhejiang Province to Lianyungang in Jiangsu Province, where tidal flats are abundant, and most coast types are silty. This study area is the most rapidly urbanizing region in China, accompanied by rapid population growth and economic development (Haas and Ban, 2014). An estimated 225 million people reside in the YRD region, contributing about one-quarter of China’s GDP (Wei, 2020). Industrialization in the YRD has progressed rapidly over the past two decades, and the development of the maritime industry in particular has made the region the largest and fastest location for port construction in China (Zhao et al., 2021; Lu et al., 2022). Thus, the study area represents a focal area of global change and human activities.
Figure 1 Geographical location map of the study area. YRD: Yangtze River Delta, including Shanghai, Jiangsu, Zhejiang, and Anhui provinces.
This study used remote sensing images generated by the Google Earth Engine (GEE) (https://earthengine.google.com) cloud computing platform to map the YRD tidal flats. For remote sensing mapping of the tidal flats in the target years (1990, 2000, 2010, and 2020), we selected time-series images for each target year and the year before and after it. Landsat TM/ETM+/OLI images were first retrieved and then pre-processed for cloud removal, shadow masking, and mosaicking through the GEE algorithm. The cloud removal was performed using the mask function (FMask), and the recognition results were recorded in the QA band (Foga et al., 2017). Along with spectral information from remote sensing images, NDVI, EVI, LSWI, mNDWI, and ETOPO1 topographic bathymetry data were selected as signature variables in this study, while supervised classification was performed using the Random Forest algorithm on the GEE platform (Pal, 2005).
Based on the historical images from Google Earth Pro, random samples for supervised classification were derived by visual interpretation. We classified the study area following a review of the Guidelines for the Classification of Land Use for Land Use Spatial Survey, Planning, and Use Control as well as the available studies (Fan et al., 2013; Murray et al., 2019; Zhang et al., 2019; Wang et al., 2020). In total, there were five categories identified in this study: tidal flats (TF), seawater (SW), coastal vegetation zones (CV), farmland and forestry land (FL), and construction land (CL). For the final map of coastal tidal flats, the supervised classification results were post-processed according to spatial topological relationships. We first filtered the classification results using plural filtering and then smoothed out the irregular edges by boundary cleaning. Due to spectral overlap, there was a mixed classification of tidal flats and other feature types. Consequently, patches that were incorrectly categorized as not conforming to the spatial distribution of tidal flats were removed (e.g., patches located within the artificial shoreline). We applied overall accuracy (OA) and Kappa coefficient (K) in the confusion matrix for classification accuracy assessment in GEE (Lewis and Brown, 2001). OA refers to the ratio between the number of correctly classified pixels and the total number of pixels. K reflects the confusion matrix balance, which is generally used in consistency tests and contributes to overall classification accuracy (Cohen, 1960).
To investigate the overall spatial pattern of tidal flat landscapes in the YRD, kernel density analysis was used to represent the evolution of tidal flat landscapes over time. Based on kernel density estimation (KDE), the kernel density analysis assumes that geographical events can occur anywhere in space with varying probabilities (Seaman and Powell, 1996). Points with dense clusters have a higher probability of events than those with sparse clusters (Bonnier et al., 2019). Tidal flats have been experiencing constant variation in their spatial pattern in the YRD for many decades. In addition to changing in size, distribution characteristics of tidal flats landscape may also have changed. The formula for Kernel density calculation is as follows:
where fn(x) is the estimated value of kernel density for tidal flat evolution, n is the observation numbers, k is the kernel function, X–Xi is the distance from the estimated point to the sample position, and h is the smoothing parameter. In this study, spatial distribution information on changes of the tidal flat area between 1990 and 2020 was first obtained by overlaying tidal flat maps. Next, kernel density in ArcGIS was used to estimate the nuclear density of the tidal flat change for each point, using patch area as a weighting metric. In addition, the KDE result was graded according to Jenks’ (natural breaks) method to determine the three classes of loss and gain for tidal flats (Jiang et al., 2018; Yuan et al., 2019).
A transfer matrix describes the changes in different landscape types within a certain period, which reveals the rules of landscape pattern evolution (Foody, 2002). In this study, the transfer matrix was applied to clarify the quantity of shifts between tidal flats and other landscape types at each phase. The formula for the transfer matrix is:
Where Pij is the area of each land use type, i and j represent the types of landscapes before and after the transfer, respectively, and n is the total number of landscape types before and after the transformation. We used the transfer matrix to reveal the outflow and inflow of the YRD tidal flat area.
Landscape index changes have been commonly used to analyze the dynamic evolution of landscape patterns (Chen et al., 2022). A landscape index is the result of highly concentrated information about landscape patterns. In addition, a landscape pattern index has important ecological significance since it specifies the characteristics of ecosystem landscape elements. Different sizes, shapes, types, numbers, and spatial configurations of landscape elements reflect the quality of landscape functions and ecological processes throughout the region. Tidal flats in the study area were studied using landscape indices selected from the classes at the YRD to quantify the variation process and characterize the functions of the tidal flat system. The following seven landscape pattern indices were selected for this study: largest patch index (LPI), patch density (PD), mean patch area distribution (MPS), mean shape index (SHAPE_MN), area-weighted mean patch fractal dimension (FRAC_AM), patch cohesion index (COHESION), and splitting index (SPLIT) (Table S1). The LPI values indicate the abundance of tidal flats across the entire landscape. PD values represent the density of tidal flat patches and reflect the heterogeneity of the landscape within a unit area. MPS reflects the average condition of the tidal flats patch. The SHAPE_MN is an indication of the complexity of the tidal flat landscape. Because they express the influence of human activities on a landscape pattern, the FRAC_AM values of natural landscapes with less interference are higher than the values for disturbed landscapes (Li et al., 2020a). COHESION refers to the connectivity between tidal flats within a YRD, while SPLIT represents the degree of separation.
Both anthropogenic and natural factors can drive tidal flat variations. Tidal flats are often considered potential areas for urban development. Therefore, urban development activities like reclamation significantly affect tidal flats (Jackson et al., 2021). There has been a substantial economic benefit to the region from aquaculture, which also occupies tidal flats (Ma et al., 2014). Factors such as population and GDP are also closely associated with the development of tidal flats (Murray et al., 2014; Wang et al., 2021b). Likewise, natural factors play an important role in influencing tidal flat landscapes. Tidal flats are formed and changed primarily by sediment carried by incoming rivers, so sediment plays a crucial role in their evolution (Bi et al., 2014; Chen et al., 2016). There is also evidence that sea-level rise has a significant effect on tidal flats (Zhao et al., 2020). Temperature and precipitation also have an impact on the landscape patterns of tidal flats (Wang et al., 2021b). A total of eight potential factors were selected to analyze the evolution of YRD’s tidal flat landscape: population (POP), gross domestic product (GDP), urban area (UA), aquaculture area (ACA), sediment discharge (Sediment), sea-level rate (SL), annual precipitation (Prcp), and annual average air temperature (Tavg) (Table S2).
To investigate the motives for tidal flat evolution, landscape pattern characteristics of the flats were associated with potential driving factors using correlation analysis. Correlation coefficients were used to investigate the extent and direction of each influencing factor that drove tidal flat dynamics (Humphreys et al., 2019). Further analysis of the driving factors influencing tidal flat landscape patterns was conducted using principal component analysis (PCA). In principal component analysis (PCA), multiple indices are merged into one composite index, or several comprehensive indices are constructed to reduce the dimensionality of a large dataset. To concentrate on potential drivers of tidal flat dynamics, redundant variables (closely related variables) can be eliminated in PCA. For this study, principal components were selected based on the eigenvalue being greater than one and the cumulative contribution rate exceeding 85% (Fang et al., 2017).
Following classification and post-processing, maps of YRD coastal tidal flats were produced at 30 m resolution for four different time periods: 1990, 2000, 2010, and 2020 (Figure 2). We evaluated tidal flat mapping results in the YRD using the OA and K. The study showed that the average OA was 94.40%, and the average K was 0.93. In 1990, 2000, 2010, and 2020, the OA was 96.75%, 96.21%, 92.35% and 92.27%, with K of 0.96, 0.95, 0.90, and 0.90, respectively.
Figure 2 Tidal flat maps for the Yangtze River Delta in the years 1990, 2000, 2010, and 2020. (A) Detection of tidal flats in Jiangsu (32 °15′N~34 °15′N, 120 °15′E~121 v45′E). (B) Detection of tidal flats in Shanghai (29 °55′N~30 °50′N, 120°30′E~122 °00′E). (C) Detection of tidal flats in Zhejiang (30 °40′N~31 °40′N, 121°15′E~122 °15′E).
Area changes of the YRD tidal flats are illustrated in Figure 3. The YRD’s tidal flats have decreased significantly over the past three decades, from 4231 km2 in 1990 to 2236 km2 in 2020, a 47% reduction (Figure 3A). During the period 1990 to 2000, the area of tidal flats decreased by 24%. This was the most dramatic decline during the study period, with an average annual decrease of 100 km2. Since then, the Chinese government has enacted more management measures and nature reserves to protect coastal wetlands. Therefore, tidal flat loss in the YRD slowed down over the next decade, decreasing by 439 km2. In the period 2010 to 2020, the YRD lost 20% of its tidal flats. Based on the KDE results, tidal flats change zones were classified into three levels (class I, class II, and class III). The two classes with high tidal flat loss in the YRD, classes II and III, occurred mainly in Yancheng and Nantong of Jiangsu, but also in Hangzhou, Shaoxing, and Ningbo in Zhejiang, along with sporadic distribution in Shanghai (Figure 3B). The map of the kernel density distribution of tidal flat gains shows that classes II and III with high density occurred mainly in the radial sand ridges of Yancheng in Jiangsu and of Jiuduansha and Nanhui in Shanghai, with scattered occurrence in Zhejiang (Figure 3C). There was a more significant loss of tidal flats in the YRD than a gain. The regions with a high kernel density of tidal flat loss had a greater geographic spread than regions with low kernel density.
Figure 3 Distribution of tidal flat dynamics in the study area. (A) Tidal flat area changes. (B) Kernel density analysis of tidal flat losses from 1990 to 2000. (C) Kernel density analysis of tidal flat gains from 1990 to 2000.
Inflows and turnovers of tidal flats from 1990 to 2020 were examined using the landscape transfer matrix (Figure 4). Tidal flat influx increased and then decreased from 1990 to 2020. Over the first three periods (1990, 2000, and 2010), seawater replenishment of tidal flats accounted for 94.54%, 97.83%, and 95.52%, respectively, of the total inflow to tidal flats. Seawater was the major outflow from tidal flats. As an overall result of conversions between seawater areas and tidal flats, the tidal flat area increased by 91 km2 between 1990 and 2020. Tidal flats were largely outflowing during the study period due to urban construction, which was the main cause of the decrease in tidal flat area. There was a notable conversion of tidal flats to construction land in 1990 and 2000, with 593 km2 and 693 km2 of tidal flats being converted to construction land, respectively. After 2010, comprehensive environmental regulations and laws were enacted, and reclamation projects were tightened (Chen et al., 2016; Zhao et al., 2020; Wang et al., 2021b). The National Marine Function Zoning Plan (2011-2020) published in 2012 strengthened reclamation management and rationalized the reclamation scale (Liu et al., 2018). Therefore, the conversion from tidal flats to construction land moderated slightly after 2010. The conversion between tidal flats and construction land accounted for 66% of the area decrease of tidal flats throughout the study period. The conversion of tidal flats to coastal vegetation zones, farmland, and forests did not show significant changes from 1990 to 2020. The loss of tidal flat area has partly been attributed to the conversion of tidal flats to coastal vegetation zones (16.62%) and the conversion of tidal flats to farmlands and forests (9.51%).
Figure 4 Sankey diagram of tidal flat conversion in the Yangtze River Delta (km2) (SW, seawater; CV, coastal vegetation zones; FL, farmland and forestry land; CL, construction land).
The landscape metrics at the class level for the YRD tidal flats have changed over the last three decades (Figure 5). Tidal flat dominance was indicated by the LPI values for the entire landscape, whereas the LPI values for the YRD tidal flats have decreased over the past thirty years. In particular, the LPI value decreased dramatically (from 0.72 to 0.1) after 2000. The MPS value declined steadily between 1990 and 2020, reflecting the average interannual degradation conditions for the YRD tidal flats. According to the falling PD values of the YRD, tidal flats of the unit area decreased by 0.86 following the year 2000. The decrease in MPS and PD values indicated a fragmentation of the tidal flat patches. There was a slight fluctuation in the SHAPE_MN value between 1990 and 2010, then it decreased during the last decade. The fluctuation in SHAPE_MN suggested that the shape of the tidal flats was constantly changing. As reflected by FRAC_AM indexes that increased and then dramatically decreased, the tidal flats were unstable and susceptible to human activity. The SPLIT value rose 0.89 during the period 2000–2020, denoting that the spatial dispersion of the tidal flats intensified. COHESION decreased by 0.81 simultaneously, showing that the physical connectivity of tidal flat patches in the YRD decreased.
Figure 5 Landscape characteristics (normalized) of tidal flats from 1990 to 2020 (LPI, largest patch index; PD, patch density; MPS, mean patch area distribution; SHAPE_MN, mean shape index; FRAC_AM, area-weighted mean patch fractal dimension; COHESION, patch cohesion index; SPLIT, splitting index).
Based on the landscape index for 2020 (Figure 6), it was clear that the distribution characteristics differed spatially. Tidal flats with high LPI and MPS values can be found mainly in southeast Yancheng and northwest Nantong of Jiangsu, clustered here with high patch densities. The mean patch areas were also high among the tidal flats of Shaoxing and Ningbo in Zhejiang. However, the tidal flats in Lianyungang and northwest Yancheng were even more fragmented, with low LPI, MPS, and PD values. Ningbo’s eastern region has the highest SHANPE_MN value, showing a relatively complex shape for tidal flats. Tidal flats in Lianyungang and northwest Yancheng showed low COHESION and FRAC_AM values and high SPLIT values, indicating poor spatial connectivity and a high degree of disturbance and dispersal. The southeast Yancheng and the northwest Nantong had a few scattered patches of tidal flats with good physical connectivity, which were high in COHESION values and low in SPLIT values. In Zhejiang province, Jiaxing, and Hangzhou cities had low LPI values, low COHESION values, and high SPLIT values. These results indicated low dominance of tidal flats, as well as a scattered distribution and poor connectivity. Shanghai’s tidal flats ranked most frequently in the middle of the landscape index results. Notably, the SPLIT values of the Shanghai tidal flats were high, and LPI and COHESION values were low, particularly in Nanhui, where the tidal flats were widely dispersed and lacked dominance and connectivity.
Figure 6 Distribution of landscape pattern indexes of tidal flats in 2020 (LPI, largest patch index; PD, patch density; MPS, mean patch area distribution; SHAPE_MN, mean shape index; FRAC_AM, area-weighted mean patch fractal dimension; COHESION, patch cohesion index; SPLIT, splitting index).
Both human and natural factors influenced the YRD’s tidal flat landscape (Figure S1). The population of the study area has grown by 37.6% since 1990. The urban area in 2020 has already exceeded ten times that of 1990. As of 2020, the region’s GDP was over 20 trillion yuan. With high growth in aquaculture, the aquaculture area in 2000 became 2,034 km2. There has been a decrease in the annual sediment discharge recorded at the Datong station. The annual precipitation value fluctuated during the study period, with the lowest value in 2010. An upward trend in mean yearly temperature was observable in the study area. The rate of sea level rise in the Yangtze River Delta was as low as 1.4 mm/year in 1990 and peaked at 3.3 mm/year in 2020, with an upward trend.
Relevant analysis was conducted to investigate the correlation between anthropogenic and natural factors affecting tidal flat landscape changes (Figure 7A). The correlations between features of the tidal flat landscape and four human factors ranged from –0.99 to 0.99, whereas the correlations with natural factors ranged from –0.16 to 1. Significant correlations were found between population and four landscape features and between GDP and one landscape characteristic. The UA was significantly relevant to three landscape indices (p< 0.05). For natural factors, sediment discharge affected three landscape characteristics positively, with annual precipitation related to one feature and average yearly temperature related to five features (p< 0.05). Sediment discharge and mean annual temperature were each significantly related to one tidal flat landscape feature (p< 0.01). Based on the PCA analysis, a contribution of 81.12% was attributed to the first principal component, followed by a contribution of 11.03% (Figure 7B). The driving factors with high load values of the first principal component were, in order, POP, GDP, UA, Tavg, and Sediment.
Figure 7 Relevant analysis results. (A) Correlation coefficient thermodynamic diagram of landscape characteristics of tidal flats and driving factors (* p<=0.05, ** p<=0.01). (B) PCA analysis of driving factors on mudflat landscape patterns.
The time-series distribution maps of the YRD tidal flats were generated by the random forest algorithm on the GEE platform for four decadal periods from 1990 to 2020. Integrating methods such as KDE, land transfer matrix, landscape index, and correlation analysis were used to examine the evolution of landscape patterns and response factors in the study area. In the YRD, tidal flat area decreased during the study period, along with decreased landscape dominance, intensification of fragmentation, decrease in connectivity, and a more dispersed distribution. Both artificial and natural factors influence the landscape features of tidal flats. Quantifying the changes in the landscape and the factors driving those changes in the YRD tidal flats could provide a reference for further tidal flat conservation and management.
We mapped the tidal flats of the YRD using Landsat images and the GEE platform with a spatial resolution of 30 m. For all four decadal periods between 1990 and 2020, the overall accuracy of the tidal flats maps was greater than 90% and the Kappa coefficients were all greater than 0.9, indicating relatively stable classification accuracy throughout the study period. Further validation was provided by comparing our tidal flat map with published maps with the same spatial resolution. There was a strong correlation between our map of the tidal flats and Murray’s research, with R2 of 0.83 and a slope of 1.22 (Figure S2) (Murray et al., 2019). Regarding the spatial distribution of the two tidal flat maps in 2010, our map corresponded well with Murray’s, which was larger in extent and also included parts of supratidal flats. Overall, the tidal flat mapping conducted in this study was accurate and reliable. There are, however, some inaccuracies inherent in the classification of satellite images. Landsat images provide only partial information on the full tidal range, and satellites are usually unable to observe extreme low and high tides (Dhanjal-Adams et al., 2016; Sagar et al., 2017). Remote sensing data with a low spatial resolution may also contribute to lower classification accuracy (Dang et al., 2021). Although the image fusion technique used in our study can improve classification accuracy, the medium resolution (30 m) Landsat images used may exacerbate the mixing of classes with similar spectral characteristics.
The YRD coast is primarily a silty coast with vast tidal flats. The tidal flats in the YRD decreased continuously during the study period from 1990 to 2020 (Wang et al., 2020). The dramatic reduction in tidal flats in the YRD occurred mainly in two time periods, 1990–2000 and 2010–2020. Geographically, the losses of tidal flats in the YRD were concentrated in Yancheng and Nantong of Jiangsu, as well as in Hangzhou, Shaoxing, and Ningbo of Zhejiang. The high-density areas of tidal flat gains occurred primarily at Radiation Sand Ridge in Yancheng of Jiangsu and in Jiuduansha and Nanhui of Shanghai. It is crucial to manage and protect tidal flats that suffer from frequent dynamics with high kernel density. There was a significant interconversion of seawater and tidal flats due to deposition and erosion (Murray et al., 2015). Throughout the study area, the main reason for the reduction in tide flats was the conversion of tidal flats to construction land. There was also a conversion between coastal vegetated zones and tidal flats. Particularly, the invasion of cordgrass (Spartina alterniflora) has had a significant impact on the coastal zone, resulting in changes to tidal flat landscapes (Mao et al., 2019; Jackson et al., 2021). In recent years, Spartina alterniflora has invaded and encroached rapidly on tidal flats in Shanghai and Jiangsu (Huang and Zhang, 2007; Zhu et al., 2022). With the implementation of the Spartina alterniflora management projects, its threat to mudflats has gradually moderated (Liu et al., 2020). With regard to the morphological characteristics of the tidal flats’ landscape, the landscape index changed gradually between 2000 and 2020, with most of the indexes showing significant changes in 2010. According to the results of landscape index analysis, tidal flats in Lianyungang, northwest Yancheng, Nanhui, Jiaxing, and Hangzhou require attention in terms of management and protection.
Characteristics of the tidal flat ecosystem can be directly affected by human activities within a relatively short period. Over the past three decades, the population and economy of the study area have grown rapidly, thereby increasing human–land conflicts. A series of large reclamation projects have been carried out in the YRD since the 1980s, resulting in the loss of vast coastal tidal flats (Ma et al., 2014; Wang et al., 2014; Chen et al., 2016). Through reclamation, the tidal flats were converted into construction land for ports, terminals, transportation, and industry (Zhao et al., 2020). Consequently, the urban area in the YRD expanded significantly between 1990 and 2020. Although offshore aquaculture brings economic benefits to the YRD, it has also resulted in continued encroachment on tidal flats along the coast (Ma et al., 2014). As humans continually transform the land for socioeconomic objectives, substantial ecological impacts occur (Sun et al., 2015). Urban expansion in the YRD may contribute significantly to climate change due to the heat island effect (Yang et al., 2017). Aquaculture may negatively affect regional water quality since it contributes to the eutrophication of water bodies (Li et al., 2021; Wang et al., 2021a). Based on the correlation and PCA analysis, four human factors (i.e., population, GDP, urban area, aquaculture area) were strongly correlated with the characteristics changes of the tidal flat landscape. In response to urbanization, the YRD tidal flats have been shrinking. Urbanization-induced reclamation is the primary cause of the shrinkage. Furthermore, tidal flats have been encroached upon irregularly by human activities, which has adversely affected their original spatial distribution. There has been significant disruption of tidal flats in the YRD due to human activities, resulting in a fragmented, dispersed, and less integrated landscape.
Natural factors have also influenced the development of the YRD’s tidal flats. In terms of climatic factors, temperature and precipitation were important variables affecting the YRD tidal flat ecosystem. A warming temperature without increased precipitation will intensify wetland evaporation, reducing the tidal flat area (Harley et al., 2006). Temperatures in the study area increased gradually from 1990 to 2020, while rainfall fluctuated within a small range. There was a significant correlation between tidal flat area and mean annual temperature in the YRD, but no significant correlation with mean annual rainfall. As a region sensitive to global change, the YRD has also experienced sea-level rise due to global warming (Cazenave and Cozannet, 2014; Moftakhari et al., 2017). The coastal seawall defense capacity of the YRD has been reduced by sea-level rise, and coastal erosion has increased, causing tidal flat loss (Gong et al., 2012; Wang et al., 2012; Kuang et al., 2014). Coastal tidal flat development is driven by sediment transportation and accumulation by rivers and tides. Sediment deposition and the hydrodynamic environment contribute to tidal flat dynamics due to “loss and gain” (Dyer et al., 2000; Xing et al., 2012). With increasing evaporation and water engineering projects, sedimentation in the YRD has weakened, leading to a slowdown in coastal tidal flat development (Zhao et al., 2017; Li et al., 2020b). There was a significant correlation between sediment discharge and characteristics of tidal flats in the YRD from 1990 to 2020, indicating that higher sediment discharges lead to a larger area of tidal flats. Tidal flat morphology and other natural factors, however, had a weak relationship. During the continuous urbanization process, human activities have greatly affected the landscape of the tidal flats (Jiang et al., 2015).
Policies, laws, and regulations provide the basis for the management of tidal flats. Since the 1990s, China has taken a series of measures to manage coastal wetlands, which have played a role in protecting coastal tidal flats (Table S3). The dynamic changes analysis of the tidal flats showed that the reduction of the tidal flats has moderated after 2000. Nonetheless, rapid economic development and the growing reliance of human activities on tidal flats have negatively affected the remaining tidal flats (Jiang et al., 2015; Wei et al., 2015). The YRD tidal flats are still experiencing declines in quantity and landscape quality. Tidal flats are not covered by any national laws or administrative regulations in China. Only local rules are in place. Local government regulations for managing tidal flats are more concerned with resource management than environmental protection. Tidal flats have also been considered a wasteland and the principal source of resource extraction (Zhao et al., 2020). The general public has not yet fully understood the ecological function and value of tidal flats. A strict, science-based, and effective policy, legislative, and regulatory framework will be required for tidal flat protection (Sun et al., 2015). Existing legislation that governs coastal tidal flat management should be improved, and new policies, laws, and regulations should be adopted. Furthermore, local governments should ensure that their policies, laws, and regulations for tidal flat management take into account the ecological environment and need for the protection of tidal flats.
Both natural and human factors have influenced tidal flat landscape evolution. Tidal flat dynamics in the YRD were closely correlated with sediments, temperature, sea level, and human activities. The protection and management of tidal flats can be improved by exploring effective measures to address the main threats. Tidal flats form primarily from sediment carried by rivers into the sea. Due to various evaporation and water engineering projects, sedimentation in the study area decreased, resulting in the loss of tidal flats (Zhao et al., 2017; Li et al., 2020b). Providing artificial silt and sand nourishment can enhance the protection of tidal flats (Deltacommissie, 2008). Sea-level rise has been triggered by rising temperatures, resulting in threats to the integrity and stability of tidal flats (Morris et al., 2002). The YRD should implement a long-term monitoring system and set up an early warning system to prevent damage to tidal flats (Leorri et al., 2013). There are also nature-based solutions for coastal management that can be used to adapt to SLR (Schuerch et al., 2018). Restoration of degraded coastal wetlands is a nature-based solution that can help strengthen coastal protection caused by SLR and associated extreme events (Kim, 2010; Narayan et al., 2016). Besides restoring existing habitats, it is also possible to use hybrid designs, such as natural habitats combined with built infrastructure, providing coastal protection against SLR (Sutton-Grier et al., 2015; Moller, 2019). Human activities such as high intensity development and frequent reclamation have significantly contributed to the loss of tidal flats (Mai and Bartholomä, 2000). The reclamation of tidal flats should be reduced in the future, with a goal of maintaining them within their natural proliferation rate (Hodoki and Murakami, 2006). Moreover, a “red line” could be defined for tidal flats, and reclamation prohibited in the “red line” area to effectively limit the negative impact of human activities on the landscape (Sun et al., 2015).
The establishment of nature reserves may contribute to the conservation of tidal flats (Hill et al., 2021). Nine nature reserves exist in the study area (Figure 8). There were areas within nature reserves with frequent tidal flat dynamics (Zone I) based on KDE results. Landscape index analysis showed a reduction in the tidal flats’ integrity, connectivity, and stability between 1990 and 2020. Geographically, it was evident that the landscape index had changed negatively in some regions (Zone II) not covered by nature reserves. Although natural reserves exist, the degradation of their tidal flats cannot be prevented, which may be because natural factors have caused tidal flats to move outside current reserves, or the reserves were unable to effectively manage them (Carranza et al., 2014; Murray et al., 2019). Reserves in the study area are managed by different departments at a local level, leading to conflicting management goals (Dhanjal-Adams et al., 2016; Zeng et al., 2022). It might be possible to consolidate overlapping reserves and manage them under one department. In Jiangsu and Shanghai, the tidal flats would benefit from combining the jurisdictions of their reserves and coordinating the management. In addition, expanding existing reserves could enhance the connectivity and integrity of the landscape within the various functional zones (Watson et al., 2014). As Yancheng National Reserve extends north-south, it could encompass wetland areas meeting international standards, such as the Ganyu, Dongtai, and Rudong tidal flats (Paulson Institute, 2016). Due to the lack of nature reserves covering tidal flats in Nanhui, Jiaxing, and Hangzhou, new protected areas need to be established to mitigate adverse effects. Finally, nature reserves do not forbid human activities, so their establishment may slow the loss of tidal flats but will not stop it. Consequently, adequate buffer zones should be maintained between human activities and tidal flat protection areas to alleviate anthropogenic impacts.
Figure 8 Distribution of nature reserves within the study area (Department of Nature Reserves at the local level: MOEP, Ministry of Environmental Protection; SOA, State Oceanic Administration; MOA, Ministry of Agriculture; SFA, State Forestry Administration).
It has been observed that tidal flats in the YRD have been degraded from 1990 to 2020, as evidenced by shrinking size, increased fragmentation and disturbance, as well as reduced dominance and connectivity across the tidal flat landscape. Yancheng and Nantong of Jiangsu, Hangzhou, Shaoxing, Ningbo of Zhejiang, and Jiuduansha and Nanhui of Shanghai were found to have high densities of tidal flat dynamics. It is worth mentioning that Lianyungang, northwestern Yancheng, Nanhui, Jiaxing, and Hangzhou have experienced a considerable negative change in their tidal flat landscape. In the YRD tidal flats, variations in landscape characteristics have been driven by various factors such as population, economy, reclamation, climate change, sea-level rise, and sedimentation. Future actions should include three aspects of tidal flat management and protection: strengthening policies, laws, and regulations related to tidal flat protection, preparing measures to counteract threats to tidal flat landscapes, and improving the efficiency of nature reserves to reduce tidal flat degradation.
The original contributions presented in the study are included in the article/Supplementary Material. Further inquiries can be directed to the corresponding author.
We would like to thank the Ministry of Science and Technology of China (2022YFC3102400), National Natural Science Foundation of China (42142018, 42206082), Shanghai Pilot Program for Basic Research-Shanghai Jiao Tong University (21TQ1400220), the Key Laboratory of Marine Ecological Monitoring and Restoration Technologies (MEMRT202112), the Oceanic Interdisciplinary Program of Shanghai Jiao Tong University (SL2021PT101), and Shanghai Frontiers Science Center of Polar Science (SCOPS) for financial support. Any opinions, findings, and conclusions or recommendations expressed in this material are those of the authors and do not necessarily reflect the views of the funders. We thank the editor and reviewers, whose comments would help to improve this manuscript.
The authors declare that the research was conducted in the absence of any commercial or financial relationships that could be construed as a potential conflict of interest.
All claims expressed in this article are solely those of the authors and do not necessarily represent those of their affiliated organizations, or those of the publisher, the editors and the reviewers. Any product that may be evaluated in this article, or claim that may be made by its manufacturer, is not guaranteed or endorsed by the publisher.
Any opinions, findings, conclusions or recommendations expressed in this material are those of the authors and do not necessarily reflect the views of the funders.
The Supplementary Material for this article can be found online at: https://www.frontiersin.org/articles/10.3389/fmars.2022.1086775/full#supplementary-material
Bai J. H., Lu Q. Q., Wang J. J., Zhao Q. Q., Ouyang H., Deng W., et al. (2013). Landscape pattern evolution processes of alpine wetlands and their driving factors in the zoige plateau of China. J. Mountain Sci. 10 (1), 54–67. doi: 10.1007/s11629-013-2572-1
Barbier E. B., Hacker S. D., Kennedy C., Koch E. W., Stier A. C., Silliman B. R. (2011). The value of estuarine and coastal ecosystem services. Ecol. Monogr. 81 (2), 169–193. doi: 10.1890/10-1510.1
Bi N. S., Wang H. J., Yang Z. S. (2014). Recent changes in the erosion-accretion patterns of the active huanghe (Yellow river) delta lobe caused by human activities. Continental Shelf Res. 90, 70–78. doi: 10.1016/j.csr.2014.02.014
Bonnier A., Finne M., Weiberg E. (2019). Examining land-use through GIS-based kernel density estimation: A re-evaluation of legacy data from the berbati-limnes survey. J. Field Archaeology 44 (2), 70–83. doi: 10.1080/00934690.2019.1570481
Cao X. X. (2008). Dynamics of wetland landscape pattern in Kaifeng City from 1987 to 2002. Chin. Geographical Sci. 18 (2), 146–154. doi: 10.1007/s11769-008-0146-x
Carranza T., Balmford A., Kapos V., Manica A. (2014). Protected area effectiveness in reducing conversion in a rapidly vanishing ecosystem: The Brazilian cerrado. Conserv. Lett. 7 (3), 216–223. doi: 10.1111/conl.12049
Cazenave A., Cozannet G. L. (2014). Sea Level rise and its coastal impacts. Earths Future 2 (2), 15–34. doi: 10.1002/2013ef000188
Chen K. X., Cong P. F., Qu L. M., Liang S. X., Sun Z. C. (2022). Annual variation of the landscape pattern in the liao river delta wetland from 1976 to 2020. Ocean Coast. Manage. 224. doi: 10.1016/j.ocecoaman.2022.106175
Chen Y., Dong J. W., Xiao X. M., Zhang M., Tian B., Zhou Y. X., et al. (2016). Land claim and loss of tidal flats in the Yangtze estuary. Sci. Rep. 6. doi: 10.1038/srep24018
Cohen J. (1960). A coefficient of agreement for nominal scales. Educ. Psychol. Measurement 20 (1), 37–46. doi: 10.1177/001316446002000104
Dang A. T. N., Kumar L., Reid M., Nguyen H. (2021). Remote sensing approach for monitoring coastal wetland in the Mekong delta, Vietnam: Change trends and their driving forces. Remote Sens. 13 (17), 3359. doi: 10.3390/rs13173359
Deltacommissie (2008) Working together with water: A living land builds for its future. Available at: http://resolver.tudelft.nl/uuid:af79991f-31e7-47a4-a6ef-bfd54ca59c57 (Accessed October 29, 2021).
Dhanjal-Adams K. L., Hanson J. O., Murray N. J., Phinn S. R., Wingate V. R., Mustin K., et al. (2016). The distribution and protection of intertidal habitats in Australia. Emu-Austral Ornithology 116 (2), 208–214. doi: 10.1071/mu15046
Du H. Y., Wang D. D., Wang Y. Y., Zhao X. L., Qin F., Jiang H., et al. (2016). Influences of land cover types, meteorological conditions, anthropogenic heat and urban area on surface urban heat island in the Yangtze river delta urban agglomeration. Sci. Total Environ. 571, 461–470. doi: 10.1016/j.scitotenv.2016.07.012
Dyer K. R., Christie M. C., Feates N., Fennessy M. J., Pejrup M., van der Lee W. (2000). An investigation into processes influencing the morphodynamics of an intertidal mudflat, the dollard estuary, the Netherlands: I. hydrodynamics and suspended sediment. Estuar. Coast. Shelf Sci. 50 (5), 607–625. doi: 10.1006/ecss.1999.0596
Fang S. B., Jia R. F., Tu W. R., Sun Z. L. (2017). ). assessing factors driving the change of irrigation water-use efficiency in China based on geographical features. Water 9 (10), 759. doi: 10.3390/w9100759
Fan D. D., Wang Y., Liu M. (2013). Classifications, sedimentary features and facies associations of tidal flats. J. Palaeogeogr. 2 (1), 66–80. doi: 10.3724/SP.J.1261.2013.00018
Foga S., Scaramuzza P. L., Guo S., Zhu Z., Dilley R. D., Beckmann T., et al. (2017). Cloud detection algorithm comparison and validation for operational landsat data products. Remote Sens. Environ. 194, 379–390. doi: 10.1016/j.rse.2017.03.026
Foody G. M. (2002). Status of land cover classification accuracy assessment. Remote Sens. Environ. 80 (1), 185–201. doi: 10.1016/S0034-4257(01)00295-4
Ghosh S., Mishra D. R., Gitelson A. A. (2016). Long-term monitoring of biophysical characteristics of tidal wetlands in the northern gulf of Mexico - a methodological approach using MODIS. Remote Sens. Environ. 173, 39–58. doi: 10.1016/j.rse.2015.11.015
Gong Z., Zhang C. K., Wan L. M., Zuo J. C. (2012). Tidal level response to Sea-level rise in the Yangtze estuary. China Ocean Eng. 26 (1), 109–122. doi: 10.1007/s13344-012-0008-2
Haas J., Ban Y. F. (2014). Urban growth and environmental impacts in jing-Jin-Ji, the Yangtze, river delta and the pearl river delta. Int. J. Appl. Earth Observation Geoinformation 30, 42–55. doi: 10.1016/j.jag.2013.12.012
Han Z. C., Ma H. L. (2021). Adaptability assessment and analysis of temporal and spatial differences of water-Energy-Food system in Yangtze river delta in China. Sustainability 13 (24). doi: 10.3390/su132413543
Harley C. D., Randall Hughes A., Hultgren K. M., Miner B. G., Sorte C. J., Thornber C. S., et al. (2006). The impacts of climate change in coastal marine systems. Ecol. Lett. 9 (2), 228–241. doi: 10.1111/j.1461-0248.2005.00871.x
Hill N. K., Woodworth B. K., Phinn S. R., Murray N. J., Fuller R. A. (2021). Global protected-area coverage and human pressure on tidal flats. Conserv. Biol. 35 (3), 933–943. doi: 10.1111/cobi.13638
Hodoki Y., Murakami T. (2006). Effects of tidal flat reclamation on sediment quality and hypoxia in Isahaya bay. Aquat. Conservation-Marine Freshw. Ecosyst. 16 (6), 555–567. doi: 10.1002/aqc.723
Huang L. B., Bai J. H., Yan D. H., Chen B., Xiao R., Gao H. F. (2012). Changes of wetland landscape patterns in dadu river catchment from 1985 to 2000, China. Front. Earth Sci. 6 (3), 237–249. doi: 10.1007/s11707-012-0312-4
Huang H., Zhang L. (2007). ). a study of the population dynamics of spartina alterniflora at jiuduansha shoals, Shanghai, China. Ecol. Eng. 29 (2), 164–172. doi: 10.1016/j.ecoleng.2006.06.005
Humphreys R. K., Puth M. T., Neuhauser M., Ruxton G. D. (2019). Underestimation of pearson's product moment correlation statistic. Oecologia 189 (1), 1–7. doi: 10.1007/s00442-018-4233-0
Jackson M. V., Fuller R. A., Gan X., Li J., Mao D., Melville D. S., et al. (2021). Dual threat of tidal flat loss and invasive spartina alterniflora endanger important shorebird habitat in coastal mainland China. J. Environ. Manage. 278, 111549. doi: 10.1016/j.jenvman.2020.111549
Jiang Y. L., Liang Z. Z., Gao H., Guo Y., Zhong Z. M., Yang C., et al. (2018). An improved constraint-based Bayesian network learning method using Gaussian kernel probability density estimator. Expert Syst. Appl. 113, 544–554. doi: 10.1016/j.eswa.2018.06.058
Jiang T. T., Pan J. F., Pu X. M., Wang B., Pan J. J. (2015). Current status of coastal wetlands in China: Degradation, restoration, and future management. Estuar. Coast. Shelf Sci. 164, 265–275. doi: 10.1016/j.ecss.2015.07.046
Jin H. R., Huang C. Q., Lang M. W., Yeo I. Y., Stehman S. V. (2017). Monitoring of wetland inundation dynamics in the Delmarva peninsula using landsat time-series imagery from 1985 to 2011. Remote Sens. Environ. 190, 26–41. doi: 10.1016/j.rse.2016.12.001
Kahara S. N., Mockler R. M., Higgins K. F., Chipps S. R., Johnson R. R. (2009). Spatiotemporal patterns of wetland occurrence in the prairie pothole region of Eastern south Dakota. Wetlands 29 (2), 678–689. doi: 10.1672/07-09.1
Kim S. G. (2010). The evolution of coastal wetland policy in developed countries and Korea. Ocean Coast. Manage. 53 (9), 562–569. doi: 10.1016/j.ocecoaman.2010.06.017
Kuang C. P., Chen W., Gu J., Zhu D. Z., He L. L., Huang H. C. (2014). Numerical assessment of the impacts of potential future Sea-level rise on hydrodynamics of the Yangtze river estuary, China. J. Coast. Res. 30 (3), 586–597. doi: 10.2112/Jcoastres-D-13-00149.1
Leorri E., Cearreta A., García-Artola A., Irabien M. J., Blake W. H. (2013). Relative sea-level rise in the Basque coast (N spain): Different environmental consequences on the coastal area. Ocean Coast. Manage. 77, 3–13. doi: 10.1016/j.ocecoaman.2012.02.007
Lewis H. G., Brown M. (2001). A generalized confusion matrix for assessing area estimates from remotely sensed data. Int. J. Remote Sens. 22 (16), 3223–3235. doi: 10.1080/01431160152558332
Li H. K., Chen S. H., Liao K., Lu Q., Zhou W. G. (2021). Microalgae biotechnology as a promising pathway to ecofriendly aquaculture: A state-of-the-art review. J. Chem. Technol. Biotechnol. 96 (4), 837–852. doi: 10.1002/jctb.6624
Lin W. J., Wu J., Lin H. J. (2020). Contribution of unvegetated tidal flats to coastal carbon flux. Global Change Biol. 26 (6), 3443–3454. doi: 10.1111/gcb.15107
Liu Y. F., Ma J., Wang X. X., Zhong Q. Y., Zong J. M., Wu W. B., et al. (2020). Joint effect of spartina alterniflora invasion and reclamation on the spatial and temporal dynamics of tidal flats in Yangtze river estuary. Remote Sens. 12 (11). doi: 10.3390/rs12111725
Liu L., Xu W., Yue Q., Teng X., Hu H. (2018). Problems and countermeasures of coastline protection and utilization in China. Ocean Coast. Manage. 153, 124–130. doi: 10.1016/j.ocecoaman.2017.12.016
Li X., Zhang X., Qiu C. Y., Duan Y. Q., Liu S. A., Chen D., et al. (2020b). Rapid loss of tidal flats in the Yangtze river delta since 1974. Int. J. Environ. Res. Public Health 17 (5). doi: 10.3390/ijerph17051636
Li J. H., Zhou K. C., Dong H. M., Xie B. G. (2020a). Cultivated land change, driving forces and its impact on landscape pattern changes in the dongting lake basin. Int. J. Environ. Res. Public Health 17 (21). doi: 10.3390/ijerph17217988
Lu T., Lin C., Wang Y. P., Wu H., Zhou M. X., Chen Y., et al. (2022). Mapping the most heavily reclaimed shorelines of the Yangtze river delta urban agglomerations. Front. Earth Sci. 10. doi: 10.3389/feart.2022.981606
Mai S., Bartholomä A. (2000). The missing mud flats of the wadden Sea: a reconstruction of sediments and accommodation space lost in the wake of land reclamation. Proc. Mar. Sci. 2, 257–272. doi: 10.1016/S1568-2692(00)80021-2
Ma Z., Melville D. S., Liu J., Chen Y., Yang H., Ren W., et al. (2014). Rethinking china's new great wall. Science 346 (6212), 912–914. doi: 10.1126/science.1257258
Mao D. H., Liu M. Y., Wang Z. M., Li L., Man W. D., Jia M. M., et al. (2019). Rapid invasion of spartina alterniflora in the coastal zone of mainland China: Spatiotemporal patterns and human prevention. Sensors 19 (10). doi: 10.3390/s19102308
Moftakhari H. R., Salvadori G., AghaKouchak A., Sanders B. F., Matthew R. A. (2017). Compounding effects of sea level rise and fluvial flooding. Proc. Natl. Acad. Sci. U. S. A. 114 (37), 9785–9790. doi: 10.1073/pnas.1620325114
Moller I. (2019). Applying uncertain science to nature-based coastal protection: Lessons from shallow wetland-dominated shores. Front. Environ. Sci. 7. doi: 10.3389/fenvs.2019.00049
Morris J. T., Sundareshwar P. V., Nietch C. T., Kjerfve B., Cahoon D. R. (2002). Responses of coastal wetlands to rising sea level. Ecology 83 (10), 2869–2877. doi: 10.2307/3072022
Murray N. J., Clemens R. S., Phinn S. R., Possingham H. P., Fuller R. A. (2014). Tracking the rapid loss of tidal wetlands in the yellow Sea. Front. Ecol. Environ. 12 (5), 267–272. doi: 10.1890/130260
Murray N. J., Ma Z. J., Fuller R. A. (2015). Tidal flats of the yellow Sea: A review of ecosystem status and anthropogenic threats. Austral Ecol. 40 (4), 472–481. doi: 10.1111/aec.12211
Murray N. J., Phinn S. R., DeWitt M., Ferrari R., Johnston R., Lyons M. B., et al. (2019). The global distribution and trajectory of tidal flats. Nature 565 (7738), 222–225. doi: 10.1038/s41586-018-0805-8
Murray N. J., Worthington T. A., Bunting P., Duce S., Hagger V., Lovelock C. E., et al. (2022). High-resolution mapping of losses and gains of earth's tidal wetlands. Science 376 (6594), 744–749. doi: 10.1126/science.abm9583
Narayan S., Beck M. W., Reguero B. G., Losada I. J., van Wesenbeeck B., Pontee N., et al. (2016). The effectiveness, costs and coastal protection benefits of natural and nature-based defences. PloS One 11 (5). doi: 10.1371/journal.pone.0154735
Pal M. (2005). Random forest classifier for remote sensing classification. Int. J. Remote Sens. 26 (1), 217–222. doi: 10.1080/01431160412331269698
Paulson Institute (2016) Blueprint of coastal wetland conservation and management in China. Available at: https://paulsoninstitute.org.cn/ (Accessed April 09, 2020).
Reimer J. D., Yang S. Y., White K. N., Asami R., Fujita K., Hongo C., et al. (2015). Effects of causeway construction on environment and biota of subtropical tidal flats in Okinawa, Japan. Mar. Pollut. Bull. 94 (1-2), 153–167. doi: 10.1016/j.marpolbul.2015.02.037
Rodriguez J. F., Saco P. M., Sandi S., Saintilan N., Riccardi G. (2017). Potential increase in coastal wetland vulnerability to sea-level rise suggested by considering hydrodynamic attenuation effects. Nat. Commun. 8 (1), 1–12. doi: 10.1038/ncomms16094
Sagar S., Roberts D., Bala B., Lymburner L. (2017). Extracting the intertidal extent and topography of the Australian coastline from a 28 year time series of landsat observations. Remote Sens. Environ. 195, 153–169. doi: 10.1016/j.rse.2017.04.009
Schuerch M., Spencer T., Temmerman S., Kirwan M. L., Wolff C., Lincke D., et al. (2018). Future response of global coastal wetlands to sea-level rise. Nature 561 (7722), 231. doi: 10.1038/s41586-018-0476-5
Seaman D. E., Powell R. A. (1996). An evaluation of the accuracy of kernel density estimators for home range analysis. Ecology 77 (7), 2075–2085. doi: 10.2307/2265701
Song K., Choi Y. E., Han H. J., Chon J. (2021). Adaptation and transformation planning for resilient social-ecological system in coastal wetland using spatial-temporal simulation. Sci. Total Environ. 789. doi: 10.1016/j.scitotenv.2021.148007
Spalding M. D., McIvor A. L., Beck M. W., Koch E. W., Moller I., Reed D. J., et al. (2014). Coastal ecosystems: A critical element of risk reduction. Conserv. Lett. 7 (3), 293–301. doi: 10.1111/conl.12074
Sun T. T., Lin W. P., Chen G. S., Guo P. P., Zeng Y. (2016). Wetland ecosystem health assessment through integrating remote sensing and inventory data with an assessment model for the hangzhou bay, China. Sci. Total Environ. (566), 627–640. doi: 10.1016/j.scitotenv.2016.05.028
Sun Z., Sun W., Tong C., Zeng C., Yu X., Mou X. (2015). China's coastal wetlands: conservation history, implementation efforts, existing issues and strategies for future improvement. Environ. Int. 79, 25–41. doi: 10.1016/j.envint.2015.02.017
Sutton-Grier A. E., Wowk K., Bamford H. (2015). Future of our coasts: The potential for natural and hybrid infrastructure to enhance the resilience of our coastal communities, economies and ecosystems. Environ. Sci. Policy 51, 137–148. doi: 10.1016/j.envsci.2015.04.006
Wang J., Gao W., Xu S. Y., Yu L. Z. (2012). Evaluation of the combined risk of sea level rise, land subsidence, and storm surges on the coastal areas of Shanghai, China. Climatic Change 115 (3), 537–558. doi: 10.1007/s10584-012-0468-7
Wang W., Liu H. I., Li Y. Q., Su J. L. (2014). Development and management of land reclamation in China. Ocean Coast. Manage. 102, 415–425. doi: 10.1016/j.ocecoaman.2014.03.009
Wang R. J., Wang Q. B., Dong L. S., Zhang J. F. (2021a). Cleaner agricultural production in drinking-water source areas for the control of non-point source pollution in China. J. Environ. Manage. 285. doi: 10.1016/j.jenvman.2021.112096
Wang X. X., Xiao X. M., Xu X., Zou Z. H., Chen B. Q., Qin Y. W., et al. (2021b). Rebound in China's coastal wetlands following conservation and restoration. Nat. Sustainability 4 (12), 1076–1083. doi: 10.1038/s41893-021-00793-5
Wang X. X., Xiao X. M., Zou Z. H., Chen B. Q., Ma J., Dong J. W., et al. (2020). Tracking annual changes of coastal tidal flats in China during 1986-2016 through analyses of landsat images with Google earth engine. Remote Sens. Environ. 238. doi: 10.1016/j.rse.2018.11.030
Watson J. E. M., Dudley N., Segan D. B., Hockings M. (2014). The performance and potential of protected areas. Nature 515, 67–73. doi: 10.1038/nature13947
Wei L. (2020) The total GDP accounts for one-fourth of the country the Yangtze river delta depends on what. Available at: https://baijiahao.baidu.com/s?id=1673940305900228629&wfr=spider&for=pc (Accessed December 27, 2021).
Wei W., Tang Z. H., Dai Z. J., Lin Y. F., Ge Z. P., Gao J. J. (2015). Variations in tidal flats of the changjiang (Yangtze) estuary during 1950s-2010s: Future crisis and policy implication. Ocean Coast. Manage. 108, 89–96. doi: 10.1016/j.ocecoaman.2014.05.018
Xing F., Wang Y. P., Wang H. V. (2012). Tidal hydrodynamics and fine-grained sediment transport on the radial sand ridge system in the southern yellow Sea. Mar. Geology 291, 192–210. doi: 10.1016/j.margeo.2011.06.006
Yang M., Gong J. G., Zhao Y., Wang H., Zhao C. P., Yang Q., et al. (2021). Landscape pattern evolution processes of wetlands and their driving factors in the xiong'an new area of China. Int. J. Environ. Res. Public Health 18 (9). doi: 10.3390/ijerph18094403
Yang X. C., Leung L. R., Zhao N. Z., Zhao C., Qian Y., Hu K. J., et al. (2017). Contribution of urbanization to the increase of extreme heat events in an urban agglomeration in east China. Geophysical Res. Lett. 44 (13), 6940–6950. doi: 10.1002/2017gl074084
Yuan K. X. J., Cheng X. Q., Gui Z. P., Li F., Wu H. Y. (2019). A quad-tree-based fast and adaptive kernel density estimation algorithm for heat-map generation. Int. J. Geographical Inf. Sci. 33 (12), 2455–2476. doi: 10.1080/13658816.2018.1555831
Zahran S., Brody S. D., Grover H., Vedlitz A. (2006). Climate change vulnerability and policy support. Soc. Natural Resour. 19 (9), 771–789. doi: 10.1080/08941920600835528
Zeng X., Chen M. Y., Zeng C., Cheng S., Wang Z. H., Liu S. R., et al. (2022). Assessing the management effectiveness of china's marine protected areas: Challenges and recommendations. Ocean Coast. Manage. 224. doi: 10.1016/j.ocecoaman.2022.106172
Zhang W. D., Bussmann R. W., Li J., Liu B., Xue T. T., Yang X. D., et al. (2022). Biodiversity hotspots and conservation efficiency of a large drainage basin: Distribution patterns of species richness and conservation gaps analysis in the Yangtze river basin, China. Conserv. Sci. Pract. 4 (4). doi: 10.1111/csp2.12653
Zhang K. Y., Dong X. Y., Liu Z. G., Gao W. X., Hu Z. W., Wu G. F. (2019). Mapping tidal flats with landsat 8 images and Google earth engine: A case study of the china's Eastern coastal zone circa 2015. Remote Sens. 11 (8). doi: 10.3390/rs11080924
Zhang F., Kung H. T., Johnson V. C. (2017). Assessment of land-Cover/Land-Use change and landscape patterns in the two national nature reserves of ebinur lake watershed, xinjiang, China. Sustainability 9, (5) 724. doi: 10.3390/su9050724
Zhang X. J., Wang G. Q., Xue B. L., Zhang M. X., Tan Z. X. (2021). Dynamic landscapes and the driving forces in the yellow river delta wetland region in the past four decades. Sci. Total Environ. 787. doi: 10.1016/j.scitotenv.2021.147644
Zhao Y. F., Liu Q., Huang R. Q., Pan H. C., Xu M. (2020). Recent evolution of coastal tidal flats and the impacts of intensified human activities in the modern radial sand ridges, East China. Int. J. Environ. Res. Public Health 17 (9). doi: 10.3390/ijerph17093191
Zhao X., Zhang Q., He G. Z., Zhang L., Lu Y. L. (2021). Delineating pollution threat intensity from onshore industries to coastal wetlands in the bohai rim, the Yangtze river delta, and the pearl river delta, China. J. Cleaner Production 320. doi: 10.1016/j.jclepro.2021.128880
Zhao Y., Zou X., Liu Q., Yao Y., Li Y., Wu X., et al. (2017). Assessing natural and anthropogenic influences on water discharge and sediment load in the Yangtze river, China. Sci. Total Environ. 607-608, 920–932. doi: 10.1016/j.scitotenv.2017.07.002
Keywords: intertidal zone, mudflats, Yangtze River Delta, landscape dynamics, protected areas
Citation: Cheng S, Zeng X, Wang Z, Zeng C and Cao L (2023) Spatiotemporal variations of tidal flat landscape patterns and driving forces in the Yangtze River Delta, China. Front. Mar. Sci. 9:1086775. doi: 10.3389/fmars.2022.1086775
Received: 01 November 2022; Accepted: 20 December 2022;
Published: 16 January 2023.
Edited by:
Qiang He, Fudan University, ChinaReviewed by:
Junlin Ren, Fudan University, ChinaCopyright © 2023 Cheng, Zeng, Wang, Zeng and Cao. This is an open-access article distributed under the terms of the Creative Commons Attribution License (CC BY). The use, distribution or reproduction in other forums is permitted, provided the original author(s) and the copyright owner(s) are credited and that the original publication in this journal is cited, in accordance with accepted academic practice. No use, distribution or reproduction is permitted which does not comply with these terms.
*Correspondence: Ling Cao, Y2FvbGluZ0BzanR1LmVkdS5jbg==
Disclaimer: All claims expressed in this article are solely those of the authors and do not necessarily represent those of their affiliated organizations, or those of the publisher, the editors and the reviewers. Any product that may be evaluated in this article or claim that may be made by its manufacturer is not guaranteed or endorsed by the publisher.
Research integrity at Frontiers
Learn more about the work of our research integrity team to safeguard the quality of each article we publish.