- 1Key Laboratory of Tropical Translational Medicine of Ministry of Education, School of Pharmacy, Hainan Medical University, Haikou, Hainan, China
- 2Shenzhen Key Lab of Marine Genomics, Guangdong Provincial Key Lab of Molecular Breeding in Marine Economic Animals, BGI Academy of Marine Sciences, BGI Marine, Shenzhen, Guangdong, China
- 3Shenzhen BGI-Marine Biomedical Technology Research Institute, Huahong Marine Biomedicine Co. Ltd., BGI Marine, Shenzhen, Guangdong, China
Marine cone snail venoms represent a vast library of bioactive peptides with proven potential as research tools, drug leads, and therapeutics. In this study, a transcriptome library of four different organs, namely radular sheath, venom duct, venom gland, and salivary gland, from piscivorous Conus striatus was constructed and sequenced using both Illumina next-generation sequencing (NGS) and PacBio third-generation sequencing (TGS) technologies. A total of 428 conotoxin precursor peptides were retrieved from these transcriptome data, of which 413 conotoxin sequences assigned to 13 gene superfamilies, and 15 conotoxin sequences were classified as unassigned families. It is worth noting that there were significant differences in the diversity of conotoxins identified from the NGS and TGS data: 82 conotoxins were identified from the NGS datasets while 366 conotoxins from the TGS datasets. Interestingly, we found point mutations in the signal peptide sequences of some conotoxins with the same mature sequence. Therefore, TGS broke the traditional view of the conservation of conotoxin signal peptides and the variability of mature peptides obtained by NGS technology. These results shed light on the integrated NGS and TGS technologies to mine diverse conotoxins in Conus species, which will greatly contribute to the discovery of novel conotoxins and the development of new marine drugs.
Introduction
As slow-moving predatory gastropods, cone snails have evolved sophisticated strategies to subdue quicker or stronger prey (Duda and Kohn, 2005; Imperial et al., 2007). These snails, classified in the Conidae family that belongs to the Conoidea superfamily as a branch of the Neogastropoda clade, form the largest genus (Conus spp.) among marine invertebrates (Imperial et al., 2007; Modica and Holford, 2010; Bouchet et al., 2011). According to variations in their diets, cone snails are divided into three different groups, namely fish hunters (piscivorous), mollusk hunters (molluscivorous), and worm hunters (vermivorous) (Modica and Holford, Puillandre et al., 2012; Dutertre et al., 2014; Yao et al., 2019). Each of the >800 Conus species has been estimated to produce 50–200 conopeptides, and thereby more than 40,000 different conotoxins may exist worldwide (Himaya et al., Gao et al., 2017; Macrander et al., 2018; Gao et al., 2018).
The cone snails venom typically comprises a complex cocktail of mostly different short peptides called conotoxins or conopeptides, which target a wide range of neuromuscular channels or receptors to paralyze prey and interfere with nerve signals to defend against predators (Terlau and Olivera, 2004; Manuel et al., 2013; Robinson and Norton, 2014; Himaya et al., 2015; Peng et al., 2016; Prashanth et al., 2016; Robinson et al., 2017). Therefore, cone snails venoms are an excellent resource of pharmacological probes (Lewis et al., 2012; Casewell et al., 2013). A conotoxin precursor typically comprises 6–50 amino acid residues (aa) with an N-terminal hyper-conserved signal peptide region, a precursor region that may be present or absent, and a C-terminal hyper-variable mature toxin region (Livett et al., 2004; Kaas et al., 2010; Jin et al., 2019b). Conotoxins have high diversity, with a detailed classification of more than 28 different gene superfamilies (namely A, B1, B2, B3, C, D, E, F, G, H, I1, I2, I3, J, K, L, M, N, O1, O2, O3, P, Q, R, S, T, V, Y) and 13 temporary gene superfamilies (Olivera et al., 1999; Kaas et al., 2010; Kaas et al., 2012; Puillandre et al., 2012; Ye et al., 2012; Aguilar et al., 2013; Dutertre et al., 2013; Luo et al., 2013; Li et al., 2020; Zamora-Bustillos et al., 2021). A total of 31 types of cysteine frameworks (I–V, VI/VII, VIII–XXX, XXXII, and XXXIII) and 12 pharmacological families (α, γ, δ, ϵ, ι, κ, µ, ρ, σ, τ, χ, and ωfamilies) have been defined by their molecular targets and pharmacological activities, respectively (Kaas et al., 2010; Kaas et al., 2012; Bernaldez et al., 2013).
Conopeptides secreted by the cone snails constitute a big library of natural drug candidates. However, only approximately 1,400 nucleotide sequences of conotoxin genes over the past decades have been reported because traditional approaches are time-consuming, of low sensitivity, and often limited by sample availability (Kaas et al., 2010; Kaas et al., 2012; Gao et al., 2018). Many research groups have applied next-generation sequencing (NGS) technology to characterize cone snails transcriptomes to discover new superfamilies and novel cysteine patterns (Bernaldez et al., 2013; Himaya et al., 2015; Abalde et al., 2018; Jin et al., 2019a). The NGS technology can achieve high sequencing depth and great coverage of transcriptomes, so even transcripts at low expression levels can be determined (Abalde et al., 2020; Peng et al., 2021). However, the quality and coverage of assembled contigs must be carefully examined to avoid potential artifacts that conotoxin sequence diversity may be overestimated (Jin et al., 2019a; Dutt et al., 2019; Abalde et al., 2020; Peng et al., 2021). The NGS has been employed to determine the diversity of conotoxins in 31 Conus species, such as Conus (Textilia) bullatus (Hu et al., 2011), Conus (Pionoconus) magus (Pardos-Blas et al.), C. striatus (Himaya et al., 2021), Conus (Dendroconus) betulinus (Peng et al., 2016), Conus (Kioconus) tribblei (Barghi et al., 2015) and Conus spurius (Zamora-Bustillos et al., 2021), discovering 100–400 different conotoxins per Conus species. Nevertheless, based on the public ConoServer database, only 8,360 conotoxins from 122 cone snails have been identified, representing < 2% of the total estimated conotoxins (Lu et al., 2014; Peng et al., 2021).
The NGS technology produce many fragments and overlapping groups without splicing, which causes the loss of important information such as variable splicing. Consequently, the innovation of transcriptome sequencing technology is imminent for discovering cone snails diversity. Third-generation sequencing (TGS) technology is a new idea and technical advance for another step forward in sequencing methods. Compared with NGS, the new platform has a powerful ability to sequence single molecules, thus avoiding the biased PCR amplification during library preparation and increasing the read lengths of their outputs (Ozsolak, 2012; Lavezzo et al., 2016). The TGS technology is also called the single-molecule real-time (SMRT) sequencing technology, which is developed by Pacific Biosciences (PacBio) with longer sequencing lengths, full-length transcripts, direct sequencing without fragmentation or post-sequencing assembly, and easy analysis of alternative splicing (AS) (Kuang et al., 2019). The TGS can provide not only nucleotide sequences of the target molecules but also information regarding epigenetic modifications for systematical investigations of gene expression in various Conus species (Levene et al., 2003; Wang et al., 2021).
C. striatus belongs to a well-known clade of hunting fish cone snails, but its differentiation of tissue distribution of venom has not been investigated (Wang et al., 2003; Pi et al., 2006; Walker et al., 2009; Jagonia et al., 2019). In this study, we employed the Illumina NGS and the PacBio TGS platforms to explore additional conotoxins from the transcriptomes of different organs, namely radular sheath, venom duct, venom gland, and salivary gland, of C. striatus. As we know, the TGS technology usually generates a relatively high error rate of raw reads that can be corrected by the sequence reads from the NGS, ensuring the reliability of the sequencing results (Travers et al., 2010; Ozsolak, 2012; Lavezzo et al., 2016). The consistent blast results of several published genes also support the good reliability of the sequencing data in this study. For simplification, the four transcriptome datasets from the different organs were named “RS” (for radular sheath), “VD” (for venom duct), “VG” (for venom gland), and “SG” (for salivary gland). Combined data of the NGS and TGS transcriptomes were used to excavate more potential conotoxins for in-depth development and utilization. This study demonstrates that the transcriptome analysis of the organs of C. striatus by integrating both NGS and TGS data helps to discover novel conotoxins and cysteine patterns, which improves the understanding of the high diversity of conotoxins in various cone snails.
Results
Summary of the sequencing transcriptome assemblies for C. striatus
Statistics of transcriptome sequencing and de novo assembly
NGS transcriptome sequencing generated 11.99, 10.23, 8.70, and 10.362 Gb of raw reads for RS, VD, VG, and SG datasets, respectively. After trimming low-quality reads, 11.82, 10.10, 8.60, and 10.27 Gb of corresponding clean reads were obtained and used for subsequent assembly (Table 1). Before employing the de novo transcriptome assembler Trinity (Grabherr et al., 2011), we removed adaptor sequences, ambiguous nucleotides, and low-quality sequences. Trinity combined reads with a certain length of overlap to form longer fragments, which were called contigs. These contigs were then subjected to further processing of sequence clustering to form longer sequences. These sequences were defined as “unigenes”. The final assembly of each transcriptome contained 95,265 (for RS), 97,832 (for VD), 43,374 (for VD), and 70,725 (for SG) unigenes, with mean lengths of 447.80, 464.99, 482.23, and 649.64 bp, respectively (Table S1).
To remove redundant sequences in the Trinity assembly, the four datasets were combined and clustered by TGICL to obtain the conjoint unigene set. A total of 67,804 unique transcripts and an entire length of 71,031,067 bp were acquired (Table S1). In parallel, the unigene library set used Bowtie2 software to align the transcriptome sequence of different samples. To investigate the transcription levels of different genes in each sample, expression levels were calculated by RSEM (v1.1.12) (Ye et al., 2020): the medium abundance expression levels of RS, VD, VG, and SG were 8.34%, 4.17%, 8.10%, and 10.69%, and the high richness expression levels were 2.69%, 1.58%, 3.50%, and 3.11%, respectively.
A total of 25,165,847,978 post-filter polymerase reads were generated by TGS. After correcting reads and clustering sequences, 41,415,6374 full-length non-chimeric (FLNC) reads with an average length of 1,384 bp were obtained (Table 2). All the 110,574 non-redundant isotype sequences with an average length of 1,424 bp were assembled using CD-hit (version 4.7) (Li and Godzik, 2006). A final list of 30,124 unigenes, with an average length of 1,718 bp, was established via matching gene sequences to the combined databases (Table 2).
Functional annotations and classification
Functional annotations of the assembled transcripts were realized by blasting against several public databases, and a total of 12,446 unigenes (approximately 66.20% of the total unigenes; Table S2) were successfully annotated by the NCBI non-redundant (NR) protein sequences, Swiss-Prot, euKaryotic Ortholog Groups (KOG), Gene Ontology (GO), and Kyoto Encyclopedia of Genes and Genomes (KEGG) databases, as indicated in the Venn diagrams (Figure S1A). All genes were searched against the KOG database (Tatusov et al., 2001) to divide ortholog clusters by phylogenetical relations. A total of 7,593 unigenes (25.21%) were classified into 26 functional clusters (Figure S1B and Table S2). Against NR database, detailed distributions of species were obtained (Figure S1C and Table S2), revealing that 18.90% had high sequence homology to Elysia chlorotical, 17.36% to Aplysia californica, 15.95% to Lottia gigantea, and 8.56% to Crassostrea Gigas.
Finally, a total of 6,482 unigenes (21.52%) were annotated to various biological pathways in the KEGG database (Kanehisa et al., 2004; Kanehisa et al., 2006) (Figure S1D and Table S2). These pathways were subdivided into five categories. “Signal transduction” represented the most abundant group, followed by “Transport and catabolism” and “Translation”. Moreover, the pathways associated with “Endocrine system”, “Circulatory system”, and “Folding, sorting, and degradation” were highly represented. In total, the identified 5,464 unigenes (18.14%) were assigned to 41 GO terms (Ashburner et al., 2000; Ye et al., 2018) in three categories of biological processes, molecular functions, and cellular components (Figure S1E; Table S2).
Summary of conotoxins in the four transcriptomes
The signal and mature regions of putative conotoxins were predicted using ConoPrec (Gao et al., 2017; Yao et al., 2019). After discarding those transcripts with disrupted cysteine frameworks in the mature regions, putative conotoxins with hits in BLASTX Results were translated according to the identified reading frames and were manually inspected. Most of them were assigned to known superfamilies based on their similarity to the highly conserved signal regions from the public ConoServer database. The total number of distinct precursors for C. striatus was 428, of which 405 were assigned to 11 known gene superfamilies (A, B1, M, O1, O2, O3, R, S, T, W, and Z), two cysteine-rich families (Conkunitzin and Con-ikot-ikot), and three hormone families (Conopressin/conophysin, Conoinsulin, and Prohormone-4, see more details in Table S3; Figure 1). Another set of 15 conotoxin precursor sequences could not be assigned to any known conotoxin superfamily (termed “unassigned”), and through alignment analysis, we assigned eight of them to the new superfamily (NFS-1 and NFS-2; Table S3).
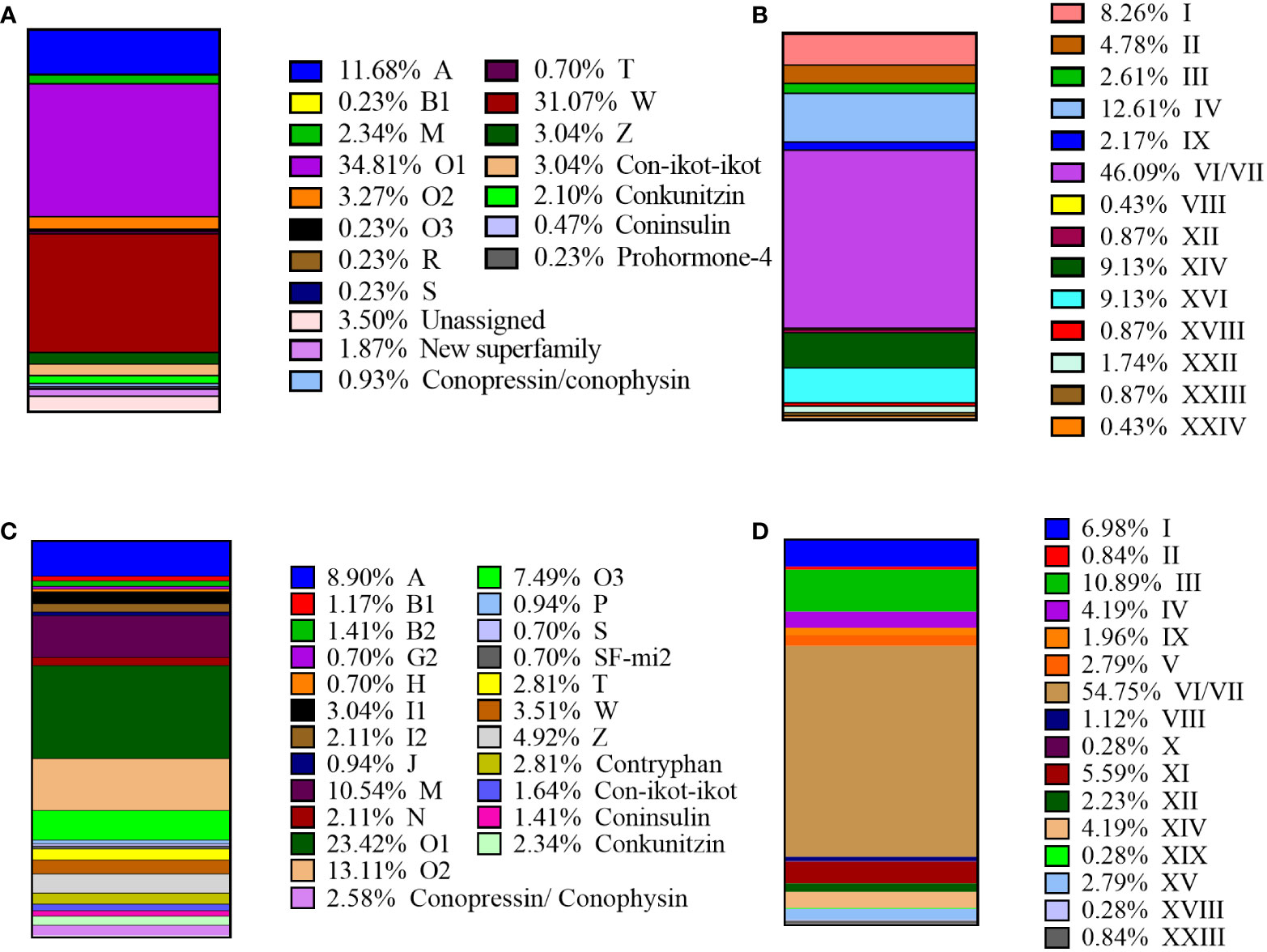
Figure 1 Summary of the superfamilies and cysteine patterns of conotoxins in C striatus: (A) Total superfamilies identified in this study. (B) The cysteine patterns identified in this study. (C) The superfamilies reported previously. (D) The cysteine patterns reported previously.
A total of 14 known cysteine frameworks were also described. The maximum proportion of cysteine framework observed was VI/VII (C-C-CC-C-C), which accounted for 46.09% (Figure 1). We also identified 106 proteins belonging to four different protein families (Table S4), namely protein disulfide isomerase (PDI), conoporin, ferritin, and transcription factors, with potential roles in the processing and application of conotoxins. For example, 33 PDIs may be involved in the formation of disulfide bonds in conotoxins (Wang et al., 2017; Zamora-Bustillos et al., 2021), ten conoporins possibly enhance the hemolytic activity of venom (Hu et al., 2011; Terrat et al., 2012; Barghi et al., 2015), and one venom-related protein elevenin and 32 transcription factors are highly conserved and ubiquitous in other venomous animals (Safavi-Hemami et al., 2014; Jouiaei et al., 2015; Pardos-Blas et al., 2019).
The most diverse conotoxin precursor superfamilies, A, O1, and W, accounted for 77.56% of the total putative conotoxins. Among them, 149 (34.81%) belong to the O1 superfamily, 133 (31.07%) to the peculiar cysteine-poor W superfamily, and 50 (11.68%) to the A superfamily (Figure 2). Interestingly, these data are highly consistent with the dominant position of the previously reported O1 superfamilies and VI/VII cysteine patterns (Pi et al., 2006; Walker et al., 2009; Himaya et al., 2021). Some of the inferred mature regions in the conotoxin precursors didn’t have any cysteine framework, such as the W and Z superfamilies that belonged to superfamilies exclusively formed by members without cysteines. We combined the conotoxin datasets with the total transcript dataset of C. striatus and named the predicted 428 conotoxins as Ts-001 to Ts-428 (Table S3). Through the public ConoServer database, we collected these conotoxins in C. striatus previously identified by traditional methods and NGS technology for classifying into 19 known conotoxin precursor superfamilies, namely A, B1, B2, G2, H, I1, I2, J, M, N, O1, O2, O3, P, S, SF-mi2, T, W, and Z (Figure 1) (Walker et al., 2009; Jagonia et al., 2019; Himaya et al., 2021). Compared with the previously reported conotoxin sequence of C. striatus, the amount of conotoxins found in this study is the sum of the previous amounts. These three superfamilies (R, Conoinsulin, and Prohormone-4) and three cysteine patterns (XVI, XXII, and XXIV) have been reported for the first time in the C. striatus. In addition, 10 of the 428 identified conotoxins have the same mature region sequences as previously reported in C. ermineus, C. striatus, and C. geographus (Table S3), and they are classified into the A, O1, O2, T, and M superfamilies, Con-ikot-ikot, and Conkunitzin. With the technical reform and skilled application of cone snail transcriptome sequencing, more potential conotoxin sequences were excavated to help us establish a systematic conotoxin library.
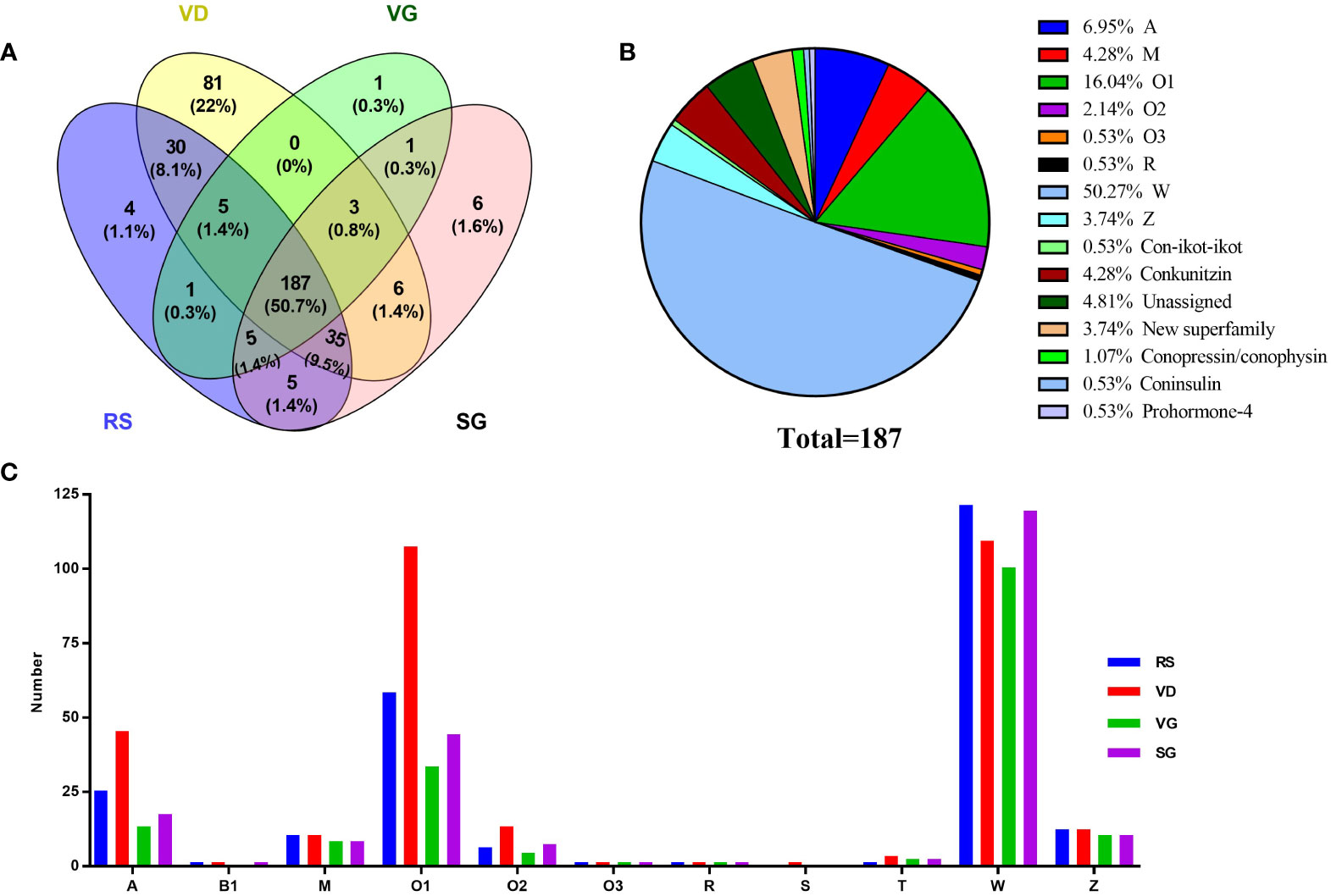
Figure 2 Comparison of conotoxin transcripts from various C striatus datasets. (A) Relationship of the identified conotoxins from the RS, VD, VB, and SG datasets. (B) Total common conotoxins from the four organs. (C) Total superfamilies identified in the RS, VD, VB, and SG, respectively.
Diversity comparison of conotoxins in the four transcriptomes
After removal of those transcripts with duplication or truncated mature region sequences, we identified 272, 346, 203, and 247 conotoxin precursor sequences from the four transcriptome datasets of RS, VD, VG, and SG, respectively (Tables S5-S8). The comparative distribution of putative conotoxin sequences is summarized (Figure 2). Interestingly, each organ had many unique conotoxin precursors, and 50.7% (187) of all inferred precursor peptide sequences were common to all transcriptomes, and 63.8% (235) of transcripts were shared between at least three specimens.
The 187 conotoxin sequences shared by four organs were assigned to 14 superfamilies: A, M, O1, O2, O3, R, W, Z, Con-ikot-ikot, Conkunitzin, New superfamily, Conopressin/conophysin, Conoinsulin, and Prohormone-4 (Figure 2). The O1 and W superfamilies demonstrated abundant diversity in the four organs, while the Z and W superfamilies were evenly distributed (Figure 2). The diversity of members of the different conotoxin precursor superfamilies was uniform across the four organs. In addition, 346 conotoxin precursors were identified from the venom duct transcriptome, accounting for 80.8% of all identified conotoxin sequences, indicating that the venom duct was the most important factory for the organizational production of conotoxins (Table S6).
To investigate the transcription levels of conotoxins in each organ, clean reads were mapped back onto the de novo assembled unigenes, and the values of fragments per kilobase of transcript per million (FPKM) mapped fragments were calculated (Pardos-Blas et al., 2019). The top 20 conotoxins (with the highest FPKM values) were selected from each dataset for comparison to find the expression levels of the most abundant transcripts. We observed that transcription levels in the VD (FPKM values above 10,426.99) were generally higher than those in the RS, VG, and SG (Table S9).
Figure 3 illustrates the differences in the spatial distribution of different conotoxin superfamilies among the RS, VD, VB, and SG datasets of C. striatus. The M, R, Z, W, and new superfamilies were not found in VD (Figure 3). Although there were significant differences in the relative transcription levels of the conotoxin superfamilies, the A and O superfamilies had the highest transcription level in the four organs. Most precursors had widely varying FPKM between specimens, for example, TS-022 was common in the VD transcriptomes (FPKM value of 31,827.03, which decreased to 5,881.78, 154.69, and 2,036.95 in the RS, VG, and SG, respectively, Table S9). The TS-022 was highly similar (only two aa substitutions) to the κ-conotoxins SIVA from C. striatus, suggesting its κ-conotoxin character and potential voltage-gated ion channel activity (Wang et al., 2003; Santos et al., 2004). Ts-018, with a mature region sequence matching the previously identified alpha-conotoxin SI of the A superfamily, had the highest transcription level in the VD. Similarly, Ts-165 was the highest expressing Ca+ channel targeted omega-conotoxin SVIA of superfamily O1 in VD (FPKM value 31,827.03), but it was the lowest in RS, VG, and SG (FPKM values less than 1,591.44). Ts-147 was a highly expressed (Abalde et al., 2018) conotoxin identified in both RS and VD of C. striatus and was assigned to the Contryphans superfamily, and through other studies, it was also found to be dominant in C. ermineus (Abalde et al., 2018; Himaya et al., 2021). The Z and W superfamilies had high sequence diversity despite their relatively low expression levels. These data depicted that most of the identified conotoxins were synthesized at high levels in the venom duct, and some conotoxins were expressed in the venom gland and the salivary gland at low levels. Surprisingly, we also observed that the quantity and expression level of conotoxins in RS were the second highest relative to those in VD (Figure 3).
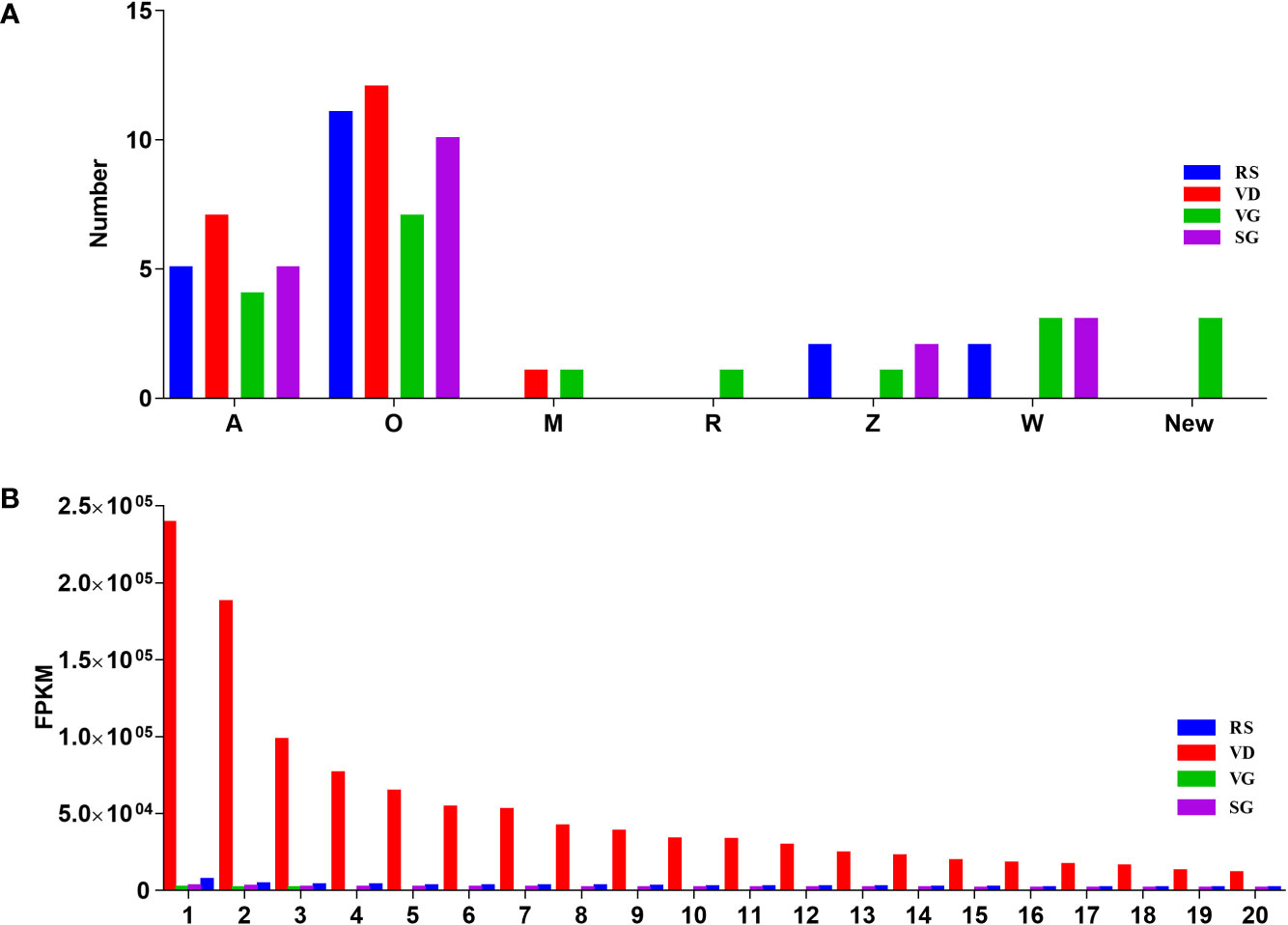
Figure 3 The top 20 conotoxins with the highest transcription values from the four transcriptome datasets. (A) subdivision of conotoxin superfamilies from the VD, VB, and SG datasets. (B) Comparison of the FPKM values for individual conotoxin within each dataset.
Comparison of conotoxin diversity identified by the two sequencing platforms
Using both NGS and TGS to sequence C. striatus specimens, the transcriptome databases were generated after ConoSorter searching and manual editing. To annotate conotoxin coding sequences among the predicted unigenes, we constructed a local reference database of known conotoxins from the public ConoServer database and manually checked them using the ConoPrec (Gao et al., 2017; Yao et al., 2019). By contrast, the NGS and TGS were quite distinct. Only a few conotoxins were easily detectable with the NGS, while the TGS detected a more complex dataset. The TGS yielded 366 conotoxin precursors that were clustered into 11 known gene superfamilies (A, B1, M, O1, O2, O3, R, S, T, W, and Z) and two cysteine-rich families (Conkunitzin and Con-ikot-ikot; Tables 2 and S10), while the NGS identified fewer conotoxin precursors (82) that were classified into nine known gene superfamilies (A, B1, M, O1, O2, O3, R, T, and W) and two cysteine-rich families (Conkunitzin and Con-ikot-ikot; Tables 2 and S11). Most of these conotoxin sequences were reported for the first time, and some possess new cysteine patterns. These predicted conotoxins were summarized and named after the two technologies as Ts-NGS-001 to Ts-NGS-082 and Ts-TGS-001 to Ts-TGS-366 (Tables S10 and S11).
These sequences from the same sample have only 4.7% overlapping between the TGS and NGS platforms, namely the A, B1, O, R, T, and new superfamilies, Conkunitzin and Con-ikot-ikot families, and three hormone families (Conopressin/conophysin, Cononsulin, Prohormone-4; Table S12 and Figure 4). Except for these overlapping conotoxins, 62 and 346 conotoxins were obtained from NGS and TGS, respectively. Among them, the A, O1, and W superfamilies have significant quantitative differences (Figure 4). A total of 151 O1 superfamily conotoxins were identified, and among them, 141 were found by the TGS. Apart from the typical VI/VII cysteine framework, we also discovered I, XIV, XVI, and XVIII (NGS only VI/VII). A total of 133 W superfamily conotoxins were identified without non-cysteine frameworks, of which the TGS-derived conotoxins accounted for 79% (Table 3). Meanwhile, 47 conotoxins belonging to superfamily A were found by TGS. Although their number was much larger than that of NGS, the cysteine patterns were relatively consistent. The S and cysteine-poor Z superfamilies were only discovered by TGS, even though their expression was low. The TGS found many new toxins and cysteine patterns that NGS did not identify, but their overall transcription levels were very low, especially for the A and W superfamilies (Figure 4). The TGS dominates the O superfamilies with a much higher number and transcription of conotoxins than the NGS. The emergence of the TGS technology seems to be reliable in generating a more comprehensive library of conotoxins.
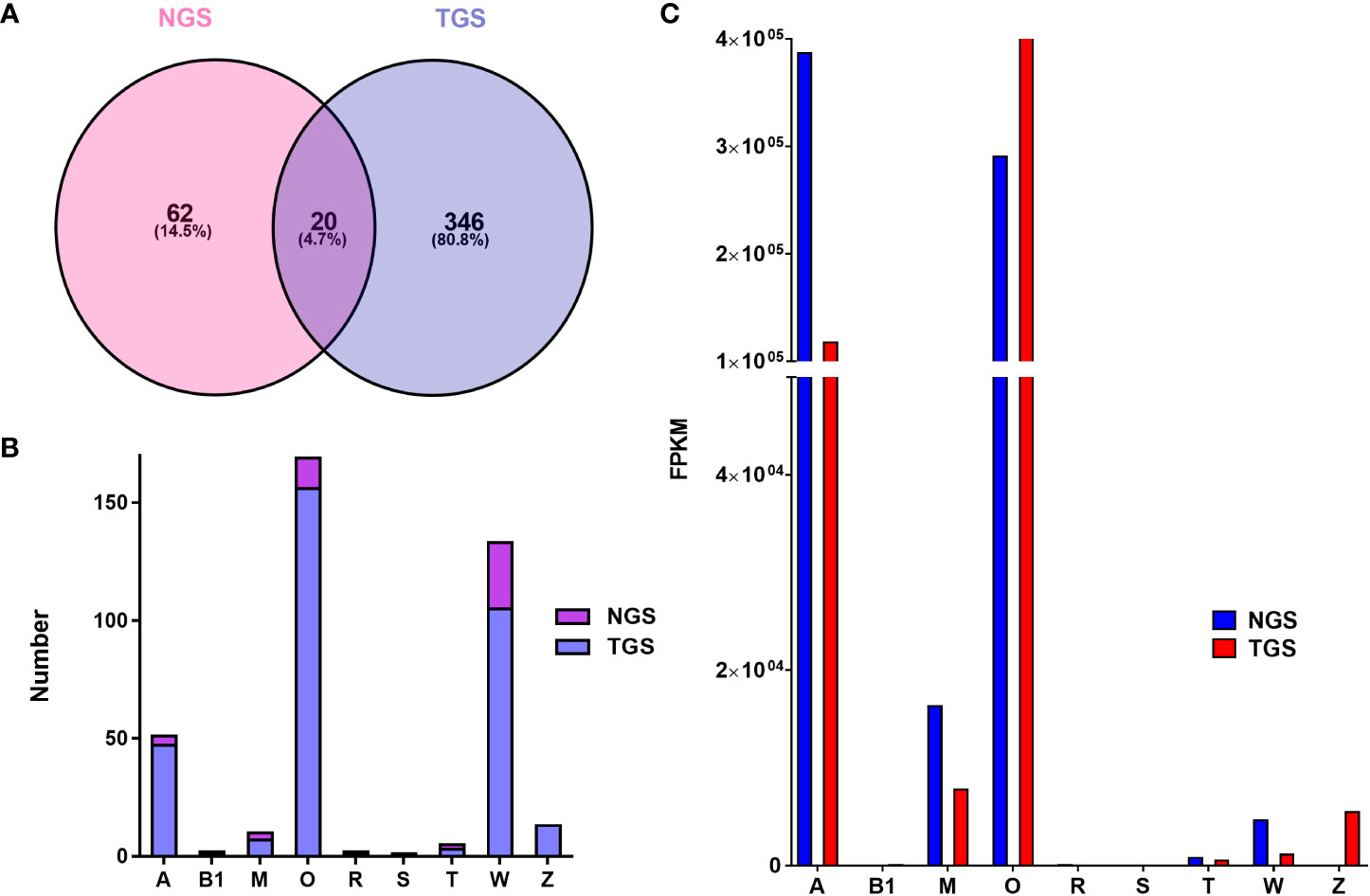
Figure 4 Comparison of conotoxin transcripts from the NGS and TGS datasets. (A) Relationship of the identified conotoxins from the NGS and TGS datasets. (B) Subdivision of conotoxin superfamilies from the NGS and TGS datasets. (C) Transcription of the NGS- and TGS-derived conotoxin superfamilies in C striatus..
Analysis of conotoxins in typical superfamilies
A total of 428 unique conotoxin precursors were classified into 11 known superfamilies, two new superfamilies (NSF-1 and NSF-2), and unassigned superfamilies based on the sequence similarity in C. striatus (Table S3). A total of 216 typical conotoxin sequences from A, O, M, and W superfamilies and new superfamily were selected to construct a phylogenetic tree (Figure 5). The phylogenetic evaluation displayed that the branches of these conotoxin superfamilies from the same Conus species appeared in different lineages. NSF-1 and NSF-2 superfamilies could not be assigned to any known families but were independently distributed among O1, O2, and O3 superfamilies (Figure 5).
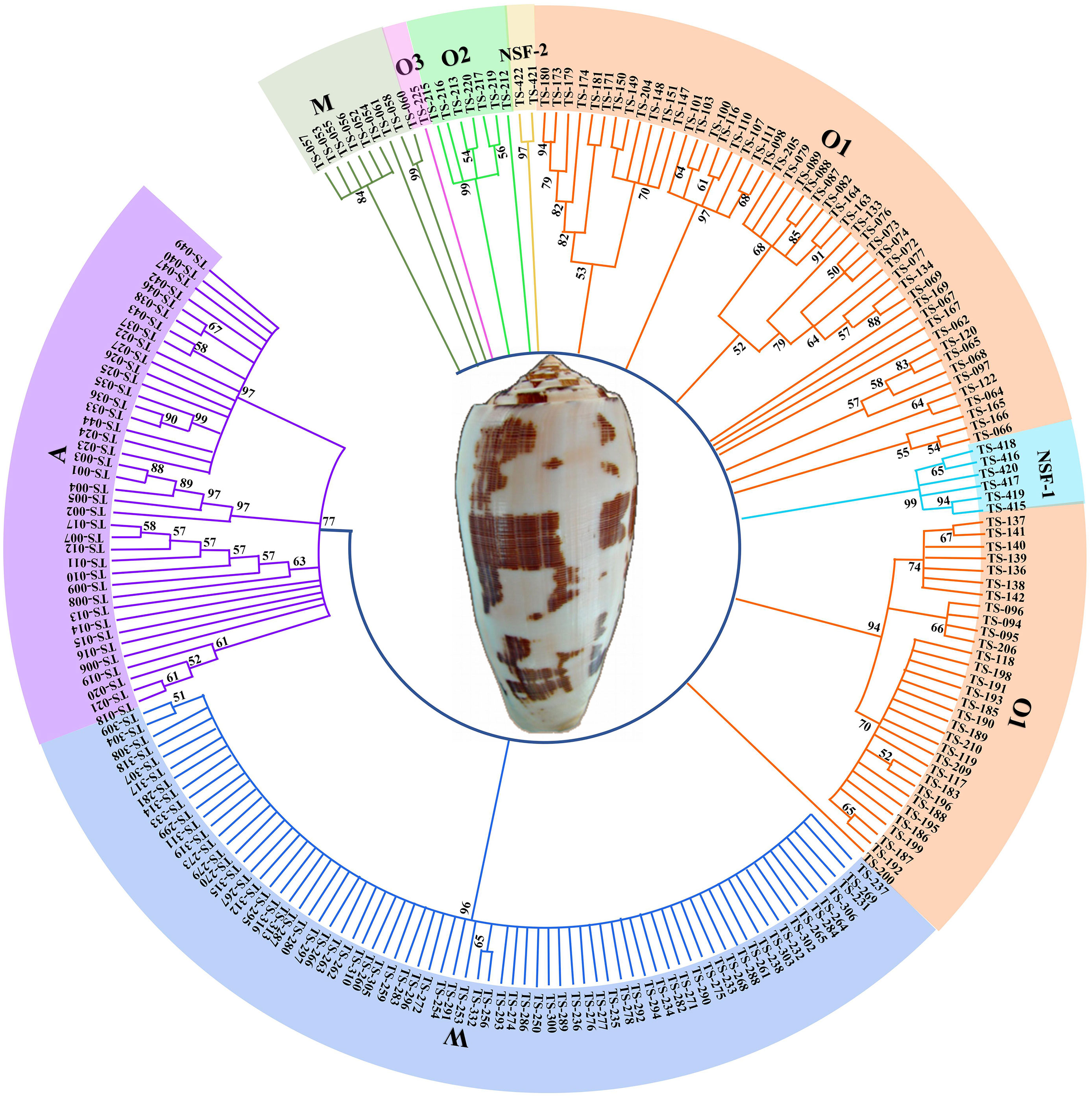
Figure 5 Phylogenetic tree of 216 complete sequences in A, O, M, W and New superfamilies. The amino acid sequences were aligned and a guide tree was constructed using the ClustalW 2.1. Tree building method: Maximum Parsimony.
The A superfamily, as a typical conotoxin group in piscivorous Conus species with very distinct structural and functional diversity, can be subdivided into two subgroups: (i) alpha (α) with cysteine frameworks I and II (Azam and McIntosh, 2009), such as those that preferentially target nicotinic acetylcholine receptors (nAChRs) and ultimately inhibit neuromuscular transmission producing paralysis; and (ii) kappa (κ) with cysteine framework IV, which selectively targets voltage-gated potassium channels (K+) (Puillandre et al., 2010; Robinson and Norton, 2014). For the A superfamily, 50 conotoxins were determined in this study, and ten sequences have the common I pattern (CC-C-C framework), 11 sequences share the common II pattern (CCC-C-C-C), and 30 transcripts own the IV cysteine pattern (CC-C-C-C-C). This is consistent with the previously reported abundance and variety of A superfamily conotoxins in other piscivorous Conus species (Hu et al., 2011; Terrat et al., 2012; Safavi-Hemami et al., 2014). However, the typical paralytic Conus peptides found in fish-hunting cone snails are from α-conotoxin families. Among them, the representative α3/5 subfamily has the cysteine framework I. These peptides, such as Ts-018, usually have 3 amino acids between cys2 and cys3 and 5 amino acids between cys3 and cys4 (Figure 6). Ts-014 targeting α1β1γδ nAChRs has the following IV cysteine pattern (Himaya et al., 2021). Ts-023 and Ts-032 have cysteine framework IV, which leads to characteristic spastic paralysis symptoms by inhibiting voltage-gated potassium channels (Craig et al., 1998). In addition, the arrangement of cysteine skeleton residues with the primary structure cysteine framework IV (i.e., CC-C-C-C-C-) belongs to the characteristic signs.
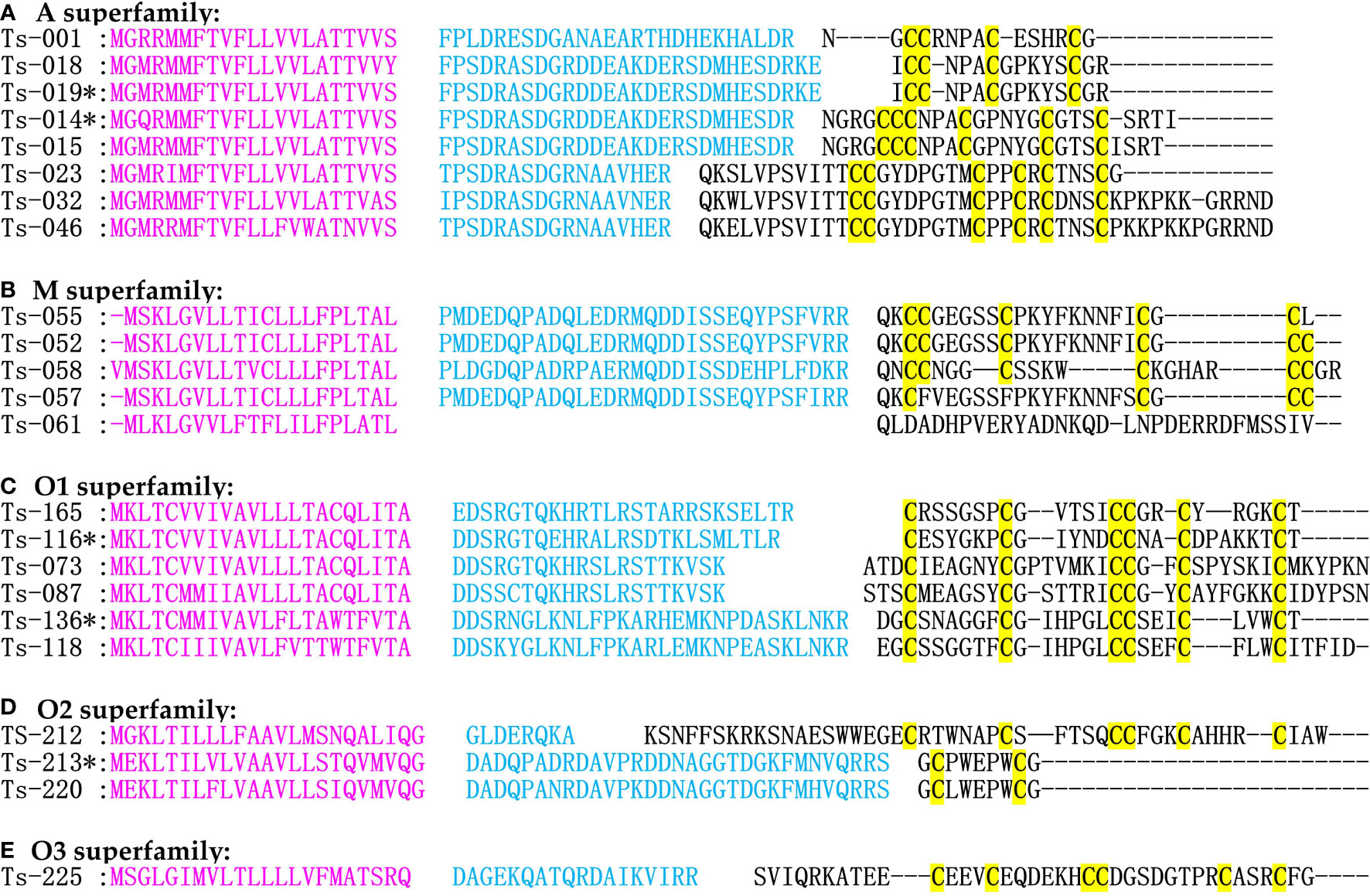
Figure 6 Alignment of the achieved A, M and O superfamilies sequences from our C. striatus transcriptomes. Stars (*) represent those conotoxins reported previously. Signal regions (in pink), pro-peptide regions (blue), mature regions (black), and cysteine residues (in yellow) are marked for comparison.
The M superfamily conotoxins are dominant in terms of transcription abundance and diversity of cysteine frameworks (XXXII, I, II, III, IV, VI/VII, IX, XIV, XVI) (Rajesh, 2015, Jacob and McDougal, 2010, Zhou et al., 2013). According to their pharmacological targets, such as voltage-gated ion channels (Na+, K+, and Ca2+) and ligand-gated ion channels (nAChR, AMPAR, GABAR, and 5-HT3R), these conotoxins are further grouped into different pharmacological families (α, ι, κ, μ) (Jacob and McDougal, 2010; Rajesh, 2015; Franco et al., 2018). A total of nine M superfamily conotoxins presented with distinct cysteine frameworks (Figure 6), including four with III (CC-C-C-CC), two with II (CC-C-C-C), and one with XVI (C-C-CC) cysteine frameworks. μ-Conotoxins Ts-052 and Ts-058 have cysteine framework III, which belongs to S3-G04 and SIIIA conotoxin, respectively, act on Na+ channels, and have analgesic activity (Bulaj et al., 2005; Schroeder et al., 2008; Yao et al., 2008). In addition, the cysteine-free components of Ts-061 have been previously classified as Conomarphins, with only five sequences described in mollusk-hunting Conus species (Akondi et al., 2014).
The O superfamily conotoxins have high transcription levels, and several isoforms have been proved to play important roles in capturing prey and/or defense. In general, the O superfamily has been subdivided into O1, O2, and O3 and is further classified into δ, µ, ω, κ, and γ families with an extensive target repertoire in ion channels (Gilly et al., 2011; Dutertre et al., 2013; Gao et al., 2017). They are the most abundant group in terms of conotoxin number (Table 3), with the extensive I, VI/VII, XIV, XVI, XVIII, and XXIV patterns of cysteine frameworks. Most O1, O2, and O3 superfamily members are unusually hydro-phobic peptides with the same cysteine framework of C-C-CC-C-C and with a similar inhibitory cystine knot motif (Jiang et al., 2017; Bernaldez-Sarabia et al., 2019). A total of 149 O1 superfamily sequences were identified in our present study. Among them, 103 presented the framework VI/VII. This typical isoform was a dominant family in quantity and displayed a high transcription level (such as an FPKM of 37,365.27 for TS-165) in the venom duct. TS-165 is ω-conotoxin SVIA that acts on presynaptic membranes (Figure 6). It binds and blocks Ca2+ channels with the cysteine pattern of VI/VII (Nielsen et al., 1996). Fourteen O2 superfamily conotoxin precursors were identified, which were designated Ts-211 to Ts-224. Two unique O2 superfamily (cysteine framework VI/VII) and 12 Contryphan conotoxins were identified, while only one (Ts-213) had been identified previously (Figure 6). Contryphans are short single disulfide-containing conotoxins (only 8 aa) that display diverse functions, generally targeting Ca2+ channels (Hansson et al., 2004; Sabareesh et al., 2006; Figueroa-Montiel et al., 2018). One O3 superfamily precursor was identified in C. striatus, with the classic signal peptide sequence (Figure 6) and the cysteine-rich framework of VI/VII (Bernaldez-Sarabia et al., 2019; Dutt et al., 2019).
Conoinsulins regulate carbohydrate and fat metabolism and act as neuromodulators of energy homeostasis and cognition. Insulin is usually synthesized as a precursor with three regions (A, B, and C chains), from which proteolytic cleavage of the C peptide in the Golgi releases the insulin heterodimer with A and B chains connected by disulfide bonds (Ward and Lawrence, 2011). Some Conus species weaponize their endogenous Conoinsulins to induce insulin shock (decreases blood glucose levels) to capture prey. Three fish-hunting cone snails, such as C. ermineus (Abalde et al., 2018), C. geographus (Safavi-Hemami et al., 2014), and C. tulipa (Dutt et al., 2019), have evolved specialized insulins, which have much greater similarity to fish insulins than to the molluscan hormones (Safavi-Hemami et al., 2015). Here, we identified two putative venom Conoinsulins in C. striatus, which were highly similar to Con-ins Im1 and Con-ins Im2 from C. imperialis. (Himaya et al., 2021). Multiple sequence alignment of insulin sequences from humans, zebrafish, and cone snails depicted that the primary sequence of insulin family was poorly conserved except for the strictly conserved cysteines (Lu et al., 2020) (Figure 7). By contrast, Conus venom Conoinsulins are greatly variable and differ from vertebrate insulins by having one additional disulfide bond between A and B chains. In brief, venom insulin sequences exhibit pronounced divergence, and the few relatively well-conserved amino acids include Gly5, IIe6, Glu19, and Tyr25 in the A chain, Thr4, Leu7, Pro16, Gly21, and Leu13 in the B chain, and all eight cysteines.
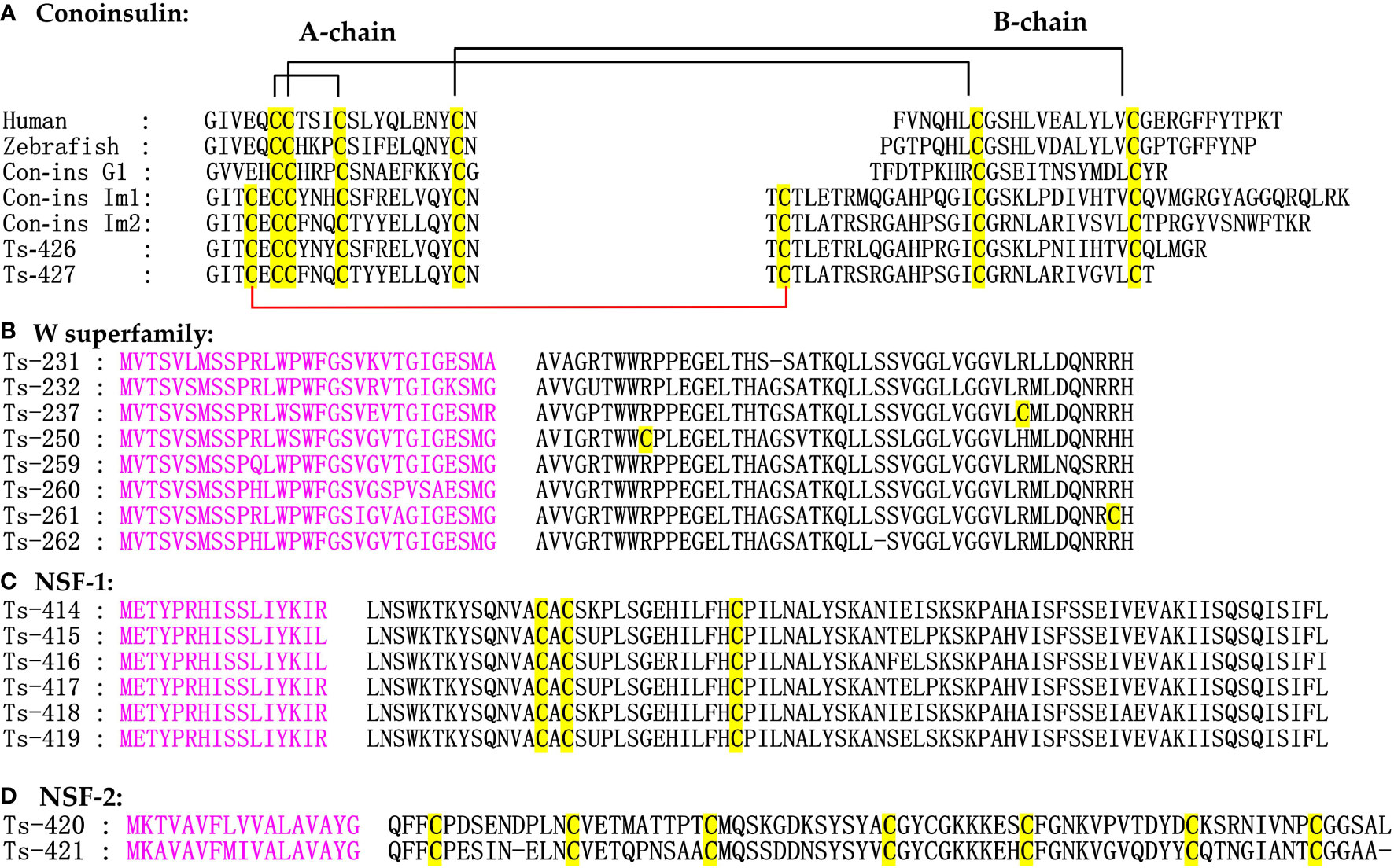
Figure 7 Alignment of the achieved Conoinsulines, W and New superfamilies sequences from our C. striatus transcriptomes. The signal regions (in pink) and cysteine residues (in yellow) are marked for comparison.
The W superfamily conotoxins, with non-cysteine mature peptides and original report in C. marmoreus, were particularly abundant in C. striatus and C. ermineus (Hansson et al., 2004; Dutertre et al., 2013; Pardos-Blas et al., 2019). A total of 133 new W superfamily sequences were identified in our present study, accounting for 31% of conotoxin precursors (Figure 7). This W superfamily lacks cysteine mode, and its biological activity has not been systematically studied. Therefore, further analysis is needed to determine the pharmacological effects of the W superfamily for exploring the evolution of various conotoxin superfamilies.
Conosorter compared the conserved amino acid sequences in conotoxin sequences and found that the eight conotoxin precursors had sufficient differences from the sequences of any known superfamily. Therefore, the eight conotoxins were divided into NSF-1 and NSF-2 signal superfamilies through software analysis and phylogenetic analysis (Figure 7C). In the two new superfamily conotoxin sequences, there were only a signal region and a mature region, and the precursor region was lacking. We unexpectedly found that the number of cysteines in these sequences was singular, which may form dimers. The NSF-1 superfamily sequence contained a total of 90 aa and had a C-C-C cysteine pattern. The NSF-2 conotoxin precursors had a signal region sequence (MKAVAVFMIVALAVAYG) and an XXII disulfide framework, while the physiological and pharmacological properties of their mature peptides should be characterized with more functional studies (Pardos-Blas et al., 2019).
In addition to the above-mentioned major superfamilies, several less representative B1, R, S, T, and Z superfamilies and the Con-ikot-ikot and Conkunitzin families were discovered in the four transcriptomes. This study identified R, S, T, and Z superfamiles sequences with the cysteine-free pattern. Nine Conkunitzin family sequences presented with IX (C-C-C-C-C-C-), XXII (C-C-C-C-C-C-C-C-C), or VIII (C-C-C-C-C-C-C-C-C-C) pattern (Table S3). Meanwhile, 12 Con-ikot-ikot peptides with the novel (C-CC-C-C-C-CC-C-C-C) framework were identified, which were reported to specifically target post-synaptic AMPA receptors (Walker et al., 2009). Interestingly, no transcriptomic evidence of I superfamily conotoxins was found, although they are often widespread among other piscivorous cone snails.
Diversity of conotoxin signal sequences
Previous studies have revealed that each complete conotoxin precursor has a hypervariable mature region, a fairly conserved precursor region, and a hyperconserved signal region (Wu et al., 2013; Yang and Zhou, 2020). However, we found that the diversity relationship among the mature, precursor and signal regions is more complex than reported. In the present study, some conotoxin precursors had the same hypervariable mature regions, but the putative hyperconserved signal regions were different due to point mutation [including insertions and deletions (indels) and/or substitutions].
All 172 identical mature regions of different conotoxins were aligned to detect point mutations and indels in the signal regions, among the A, M, O, and W superfamilies, these variances account for 25%, 1%, 58%, and 16%, respectively (Table S13, Figure S2). As listed in Table S13, the conotoxin sequences have the same mature region; 74 and 71 conotoxins were deleted and replaced in the signal region, and 11 and 31 conotoxins were deleted and replaced in the precursor region, respectively. A total of 146 point mutations were present in the signal regions, suggesting that point mutations are frequent in the signal regions but infrequent in the precursor regions (Figure 8). In addition, 158-point mutation sequences were identified by the TGS technology, and only 14 sequences were found by the NGS technology, indicating that the TGS broke the traditional view of the conservation of conotoxin signal peptides and the variability of mature peptides obtained by the traditional NGS technology.
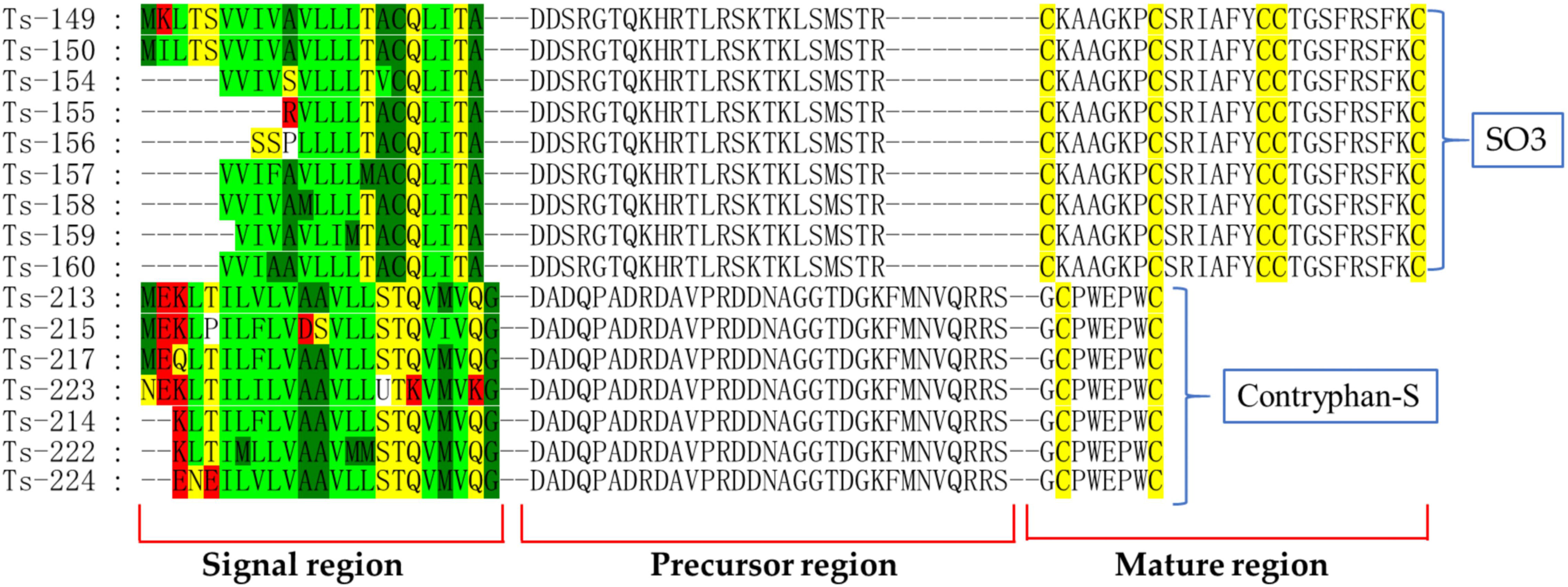
Figure 8 Alignment of same mature regions but different signal regions in the O superfamily sequences from our C. striatus transcriptomes.
Discussion
With the rapid development of high-throughput transcriptomics and the continuous reduction of costs, transcriptome sequencing technology has been used to explore conotoxins in many Conus species (Dutertre et al., 2014; Rajaian Pushpabai et al., 2021). According to the ConoServer database, the current list of 8,360 conotoxins from 122 cone snails has been identified through traditional methods and NGS, representing < 2% of all potential conotoxins (Kaas et al., 2008; Kaas et al., 2010; Kaas et al., 2012; Zhang et al., 2019). Although transcriptome-based NGS technology is powerful on a large scale and has been widely used to uncover Conus genes, the NGS technology may produce fragments and overlapping groups that are not spliced and may lose important information resulting in the omission of certain conotoxins (Zeng et al., 2018; Yuan et al., 2021; Zhan et al., 2021; Zhang et al., 2021). In addition, the NGS with a short read length may restrict right sequence assembly and annotation, resulting in the prediction of fewer conotoxins (Zeng et al., 2018; Yang et al., 2018b; Yuan et al., 2021; Zhan et al., 2021). The TGS technology with SMRT sequencing can overcome these limitations. This strategy can produce assembly-free, highly accurate, full-length and near full-length unigenes, significantly improving gene annotation and transcriptional quantification (Lluisma et al., 2012; Ozsolak, 2012; Cacho et al., 2014; Lavezzo et al., 2016; He et al., 2017; Liu et al., 2017; Kuang et al., 2019). As per our knowledge, no previous studies have employed the TGS technology to study C. striatus.
To date, 423 conotoxins have been found from C. striatus using traditional methods and NGS technology, and assigned to 25 superfamilies (Himaya et al., 2021). A total of 428 unique conotoxins in RS, VD, VG, and SG from C. striatus were discovered using the combination of NGS and TGS technologies for the first time. The TGS identified almost 85.5% of the conotoxins in C. striatus, but only 82 conotoxin transcripts were identified by the NGS, confirming that the TGS technology is more efficient than the NGS in the identification of diverse conotoxins (Xue et al., 2013; Xu et al., 2015; Yang et al., 2018a). The sequences generated from the same sample had only 4.7% overlapping between the TGS and NGS technologies, which proves that the combination of the two technologies can identify more conotoxins. For the NGS, only one assembler cannot fully recover the most comprehensive toxin profile (Xie et al., 2022). However, applying multiple assemblers to improve details will become time-consuming and challenging, which requires a thorough knowledge of those assemblers. Emergence of TGS can overcome this deficiency. Although the TGS technology has more advantages, 62 toxin sequences remain undiscovered in C. striatus compared with the NGS technology. Therefore, the comprehensive strategy combines NGS and TGS technology to obtain a complete transcriptome of C. striatus, which contributes to discovering more conotoxins.
A total of 428 conotoxins and 106 related proteins (processed conotoxin peptides) were found in C. striatus by integrated sequencing technology. These conotoxins were assigned to 13 gene superfamilies, of which 11 gene superfamilies (A, B1, M, O1, O2, O3, R, T, and W) were found by the NGS, and 13 gene superfamilies were found by the TGS (A, B1, M, O1, O2, O3, R, S, T, W, and Z). The TGS technology found two gene superfamilies (S and Z) that the NGS technology could not find.
Similarly, we found 14 typical cysteine frameworks (I, II, III, IV, IX, VI/VII, VIII, XII, XIV, XVI, XVIII, XXII, XXIII, and XXIV) in C. striatus, of which the NGS technology found ten cysteine frameworks and the TGS technology found 13 cysteine frameworks. This shows that the TGS technology found four cysteine frameworks (XVI, XVIII, XXIII, and XXIV) that were not found by the NGS technology (Figure 2 and Table S3). The conotoxin transcripts in the low-level superfamilies were more diverse, such as the W superfamily had a high sequence diversity despite relatively low transcription in four organs (Figure 3). This superfamily has no cysteine pattern. Thereby, its toxicity is possibly weak (as non-predatory toxins) (Abalde et al., 2018). The discovery of W and Z superfamilies from TGS dataset reveals that the long-read sequencing technology was more suitable for identifying these toxin sequences (Table S10). In-depth investigations should be carried out to determine the pharmacological activities of these W and Z superfamilies due to the lack of cysteine mode, and more detailed studies on the effects of mutations in signal regions are required to explore the evolution of various cone snails.
A total of 272, 346, 203, and 247 putative conotoxin transcripts were identified from the four transcriptome datasets of RS, VD, VG, and SG, respectively. By comparing each of the four transcriptomes, we determined that 187 (50.7%) identical putative conotoxins were collectively identified in RS, VD, SG, and VB. Table S9 lists the same highly expressed conotoxin sequences in all four organ specimens, indicating good homology of conotoxin in different organs. These conotoxin precursors have both diversity and consistency in four organs of C. striatus, and the highest diversity and expression levels of conotoxins were revealed in the venom duct. Four C. striatus transcriptomes were dominated by transcripts from A and O superfamilies, accounting for > 97% of the total identified conotoxin transcripts in each specimen, which is consistent with several previous reports (Wang et al., 2003; Pi et al., 2006; Jagonia et al., 2019; Himaya et al., 2021). The A superfamily conotoxins are those with the highest FPKM values in the C. striatus that selectively acts on the nAChRs and K+ channels. κA-conotoxins are conserved and dominant in the Pionoconus clade, and they can be used as representative toxins in the branch of fish hunting. A large number of O superfamily (O1, O2 and O3 superfamily) conotoxins have been found in C. striatus and they have rich diversity and widely act on various targets. The representative O superfamily conotoxin MVIIA (SNX-111, ziconotide) is the only N-type Ca2+ channel (Cav2.2) inhibitor approved by the US FDA for the management of severe chronic pains in patients who were unresponsive to opioid therapy (Vetter and Lewis, 2012). In our present study, 149 O superfamily conotoxins were identified from the piscivorous C. striatus, and some sequences are highly similar to MVIIA (may have the same target), laying a foundation for establishing an analgesic conopeptide library. In addition, the Contryphans peptides containing 7-12 amino acid residues were found to be a branch of the O2 superfamily and the dominant conotoxins in C. striatus. Some previous studies have shown that the “stiff tail” syndrome will occur when the conotoxin of Contryphans is injected into the brain of mice, which might act on Ca2+ or K+ channels (Massilia et al., 2001; Lebbe and Tytgat, 2016; Han et al., 2017). Therefore, identifying diverse and divergent conotoxins with new cysteine patterns in C. striatus using both NGS and TGS techniques provides a tremendous genetic resource for discovering numerous conotoxins that may become a valuable peptide bank for drug development and biomedical studies.
Interestingly, we found point mutations in the signal peptide sequences of some conotoxins with the same mature peptides from C. striatus. Yang and other scholars analyzed the M superfamily conotoxin precursors and found that different conotoxin precursors have the same mature region (Yang and Zhou, 2020). Point mutations in the signal peptide sequences were identified in both the NGS and TGS datasets, 91% of which occurred in the TGS data, implying that TGS broke through the conservative nature of conotoxin signal peptides and the traditional concept of the variability of mature peptides obtained by NGS technology. However, the perception that the signal region is hyperconserved, the proregion is relatively conserved, and the mature region is hypervariable remains valid. These findings in this study complement the traditional perception, and they would not change putative classification of gene superfamilies, cysteine frameworks, or pharmacological families based on this dogma. Therefore, point mutations of amino acids are crucial for the physiological functions of mature conotoxins and play an essential role in conotoxins’ sequence and functional diversity.
Materials and methods
Sampling and RNA extraction
Three C. striatus specimens, about 9 cm in length, were collected from the offshore areas of the West Island near Sanya, South China Sea, China. These cone snails were shipped to the indoor laboratory at Hainan Medical University (Haikou, Hainan, China), and then were kept in an ecological breeding system for about 3 days. Four organs of the cone snails, including radular sheath, venom duct, venom gland, and salivary gland, were dissected and then immediately stored at –80°C before use.
Total RNA was extracted from these samples using QIAzol reagent (Qiagen, Fredrick, MD, USA). A NanoDrop nucleic acid quantifier was used to detect the purity of RNA (A260: A230 ratio of 2.0–2.2; A260:A280 ratio of 1.8–2.1), a Qubit RNA assay (Invitrogen, Carlsbad, CA, USA) was used to accurately quantify the RNA concentrations, and an Agilent 2100 Bioanalyzer (Palo Alto, CA, USA) was used to detect the integrity of RNAs.
NGS library construction
The high-quality RNA samples (OD260/280 = 1.8–2.2, OD260/230 ≥ 2.0, RIN ≥ 8, > 1 μg) were used to construct individual sequencing library. PolyA mRNA was purified from total RNA using oligo-dT-attached magnetic beads and then fragmented by a fragmentation buffer. Taking these short fragments as templates, first-stranded cDNA was synthesized using reverse transcriptase and random primers, followed by second-stranded cDNA synthesis. Then, the synthesized cDNA was subjected to end-repair, phosphorylation, and “A” base addition according to the library construction protocol. Afterward, sequencing adapters were added to both sides of the cDNA fragments. After PCR amplification of cDNA fragments, 150–250 bp targets were obtained. Finally, we performed the paired-end sequencing on an Illumina HiSeq X Ten platform (Illumina Inc., San Diego, CA, USA).
TGS library construction
Total RNA was extracted by grinding tissues in TRIzol reagent (Cat# DP424, TIANGEN Biotech Co. Ltd, Beijing, China) on dry ice and was processed following the manufacturer’s instructions. The integrity of the RNA was determined with the Agilent 2100 Bioanalyzer and agarose gel (Lonza) electrophoresis. The purity and concentration of the RNA were determined with the Nanodrop micro-spectrophotometer (Thermo Fisher Scientific, USA). The mRNA was enriched by Oligo (dT) magnetic beads. Then, the enriched mRNA was reverse transcribed into cDNA using a Clontech SMARTer PCR cDNA Synthesis Kit (TAKARA). PCR cycle optimization was used to determine the optimal amplification cycle number for the downstream large-scale PCR reactions (PrimeSTAR GXL DNA polymerase). Then, the optimized cycle number was used to generate double-stranded cDNA. The large-scale PCR was performed for the next SMRTbell library construction. cDNAs were damage-repaired and end-repaired, and the sequencing adapters were ligated using a SMRTbell Template Prep Kit (PacBio). Each SMRT bell template was annealed to a sequencing primer, bound to polymerase, and sequenced on a PacBio sequel platform by Nextomics Biosciences Co. Ltd. (Wuhan, Hubei, China). Circular consensus sequences were obtained using the SMRTlink 5.1 software (PacBio). A total of 35.6 Gb subread sequences were obtained by correction between subreads. According to the existence of 5’-end primer, 3’-end primer, or polyA tail, these sequences were divided into FLNC reads and non-full-length sequences. The former was clustered by iterative clustering in Iterative Clustering for Error Correction (Wang et al., 2021) algorithm software to generate the cluster consensus sequences. Subsequently, we corrected the polished consensus sequences of the TGS data through LoRDEC software (PacBio) with default parameters, and any redundancy in corrected consensus reads was removed by CD-hit (version 4.7) (Li and Godzik, 2006) to obtain the final transcripts for subsequent analysis.
Gene functional annotation and CDS prediction
Illumina NGS data from the same samples were assembled with Trinity to produce unigenes (Shimizu et al., 2006). Transcripts were annotated by searching against five public databases, namely, clusters of orthologous/prokaryotic groups of proteins (Tatusov et al., 2001), NCBI NR (Li et al., 2002), GO (Ye et al., 2006), KEGG Ortholog (Kanehisa et al., 2017), and SwissProt (Soudy et al., 2020; UniProt, 2021). Transcripts >500 bp were selected for SSR analysis using the MIcroSAtellite identification tool (MISA; http://pgrc.ipk-gatersleben.de/misa, http://pgrc.ipk-gatersleben.de/misa/) (Thiel et al., 2003). MISA can identify seven SSR types, mononucleotide, dinucleotide, trinucleotide, tetranucleotide, pentanucleotide, hexanucleotide, and compound SSR, by analyzing transcript sequences. We used ANGEL software (Shimizu et al., 2006) (https://github.com/PacificBiosciences/ANGEL ) to perform CDS and protein predictions for all isoforms. Using a custom Python script (Wang et al., 2018) (alternative_splice.py, https://github.com/Nextomics/pipeline-for-isoseq ), AS events were identified from alignments and classified into exon skipping, intron retention, alternative donor site, alternative acceptor site, and alternative position. We used the ncRNA pipeline process (Li et al., 2014) (https://bitbucket.org/arrigonialberto/lncrnas-pipeline ) to obtain the LncRNA redundant transcript sequence.
Prediction and identification of conotoxins
Homologous searches and ab initio prediction methods (Kaas et al., 2012) were used to predict conotoxins from transcriptome datasets. All previously known conotoxins were downloaded from the ConoServer database for homologous prediction to build a local reference dataset. We subsequently used BLASTX (with an E-value of 1e-5) to run our assembled sequences against the local dataset. Those unigenes with the best hits in the BLASTX data were translated into conotoxins sequences. In addition, an ab initio prediction method using Hidden Markov Model (HMM) (Laht et al., 2012)was adopted to discover new conotoxins. The four conotoxin datasets were grouped into different classes according to the superfamily and family classification in the ConoServer database. Sequences of each familes were aligned with ClustalW, and the ambiguous results were checked by manual correction. Finally, a profile HMM was built for the conserved domain of each class using hmmbuild from the HMMER 3.0 package (http://hmmer.janelia.org ), and the hmmsearch tool was then applied to scan every assembled unigene/EST for identification of conotoxins (Wheeler and Eddy, 2013).
Classification of gene superfamilies
Conserver is a machine program for large-scale recognition and classification of conotoxins (Kaas et al., 2012). The Conoprec tool implemented in the Conserver database was used to identify the three domains (signal, propeptide, and mature) of the predicted conotoxin precursors and the cysteine frameworks of the mature functional peptides. Precursors were assigned to different protein superfamilies based on the percentage of sequence identity (> 70%) to the highly conserved signal region. If the conservation of the signal region was lower than the threshold of any reported conotoxin superfamily, members of the new superfamily were named “NSF-1 and NSF-2” according to the conservation of the conotoxin precursor peptide (Robinson and Norton, 2014). Those conotoxins without signal regions or incomplete signal regions but still showing similarity in either the pro- or mature region were classified as the “unassigned” group.
Alignment and phylogenetic analysis
Multiple sequence alignments for all conotoxins were performed using MUSCLE (v. 3.8.31) (Edgar, 2004), followed by manual adjustment using the GeneDoc software. A phylogenetic analysis was carried out using the maximum likelihood method with RAxML8.1, and statistical supports were assessed using 1,000 bootstrap pseudo-replicates. We used the complete conotoxin to perform a phylogenetic analysis of the highly diverse A, M, O, W, and new conotoxin precursor superfamilies. The amino acid sequences were aligned using ClustalW 2.1 software (Thompson et al., 1994). We chose maximum parsimony as a tree-building method in MEGA 7.0.14 software (Kumar et al., 2016).
Transcript abundance and differential expression analyses
Clean reads were mapped back onto the assembled precursors, and transcript abundance was calculated in FPKM. The FPKM value of 0.1 was used as a threshold for judging whether the gene was expressed. RSEM software (v1.1.12) was used to calculate the expression levels, and the unigene set clustered by TGICL software was used to build a library (Pertea et al., 2003; Li and Dewey, 2011). Bowtie2 software was used to align the transcriptome sequence of each sample to the unigene library, and the transcription levels of all unigenes in different samples were counted (Langdon, 2015).
Data visualization
The presented multi-omics data were visualized using Prism software v7.0.0, and Venn maps were generated using Venny v2.1.0 software.
Conclusions
This study is the first report to explore the diverse conotoxins in C. striatus using the integrated NGS and TGS techniques. A total of 428 putative conotoxin sequences were identified, and 18 new superfamilies were classified. As expected, most identified conotoxins in four organs were novel, with divergent signal regions and mature regions from known conotoxins. TGS data included almost 85.5% of conotoxin sequences in C. striatus. Among them, S and Z superfamilies were identified only by the TGS technology. The discovery of these new conotoxins in C. striatus enhances our understanding of the high conotoxin diversity in various cone snails. Meanwhile, the application of the Pacbio TGS technology not only enriches the conotoxin information in the representative C. striatus but also paves the way for extensive applications of TGS technology in this largest genus among marine invertebrates for the exploration of numerous novel conotoxin sequences.
Data availability statement
Raw data of the C. striatus for the newly sequenced transcriptomes in this study were deposited at China National GeneBank (https://db.cngb.org/) repository, accession number “CNP0002891”.
Author contributions
BG and QS conceived and designed the project; YL, CP, and YZ analyzed the data; YL and BG wrote the paper; BG, QS, YL, CP, JF, and ZR revised the manuscript. All authors contributed to the article and approved the submitted version.
Funding
This research was financially supported by Hainan Provincial Keypoint Research and Invention Program (no. ZDYF2022SHFZ309), National Natural Science Foundation of China (no. 82060686), Hainan Provincial Natural Science Foundation (no. 820RC636), Special Scientific Research Project of Hainan Academician Innovation Platform (no. YSPTZX202132), and Hainan Medical University graduate innovation and entrepreneurship training program (no. HYYS2021A29).
Conflict of interest
Authors CP and QA were employed by Huahong Marine Biomedicine Co. Ltd.
The remaining authors declare that the research was conducted in the absence of any commercial or financial relationships that could be construed as a potential conflict of interest.
Publisher’s note
All claims expressed in this article are solely those of the authors and do not necessarily represent those of their affiliated organizations, or those of the publisher, the editors and the reviewers. Any product that may be evaluated in this article, or claim that may be made by its manufacturer, is not guaranteed or endorsed by the publisher.
Supplementary material
The Supplementary Material for this article can be found online at: https://www.frontiersin.org/articles/10.3389/fmars.2022.1060432/full#supplementary-material
References
Abalde S., Tenorio M. J., Afonso C. M. L., Zardoya R. (2018). Conotoxin diversity in chelyconus ermineus (Born 1778) and the convergent origin of piscivory in the Atlantic and indo-pacific cones. Genome Biol. Evol. 10, 2643–2662. doi: 10.1093/gbe/evy150
Abalde S., Tenorio M. J., Afonso C. M. L., Zardoya R. (2020). Comparative transcriptomics of the venoms of continental and insular radiations of West African cones. Proc. Biol. Sci. 287, 20200794. doi: 10.1098/rspb.2020.0794
Aguilar M. B., Ortiz E., Kaas Q., Lopez-Vera E., Becerril B., Possani L. D., et al. (2013). Precursor De13.1 from conus delessertii defines the novel G gene superfamily. Peptides 41, 17–20. doi: 10.1016/j.peptides.2013.01.009
Akondi K. B., Muttenthaler M., Dutertre S., Kaas Q., Craik D. J., Lewis R. J., et al. (2014). Discovery, synthesis, and structure-activity relationships of conotoxins. Chem. Rev. 114, 5815–5847. doi: 10.1021/cr400401e
Ashburner M., Ball C. A., Blake J. A., Botstein D., Butler H., Cherry J. M., et al. (2000). Gene ontology: tool for the unification of biology. the gene ontology consortium. Nat. Genet. 25, 25–29. doi: 10.1038/75556
Azam L., Mcintosh J. M. (2009). Alpha-conotoxins as pharmacological probes of nicotinic acetylcholine receptors. Acta Pharmacol. Sin. 30, 771–783. doi: 10.1038/aps.2009.47
Barghi N., Concepcion G. P., Olivera B. M., Lluisma A. O. (2015). High conopeptide diversity in conus tribblei revealed through analysis of venom duct transcriptome using two high-throughput sequencing platforms. Mar. Biotechnol. (NY) 17, 81–98. doi: 10.1007/s10126-014-9595-7
Bernaldez J., Roman-Gonzalez S. A., Martinez O., Jimenez S., Vivas O., Arenas I., et al. (2013). A conus regularis conotoxin with a novel eight-cysteine framework inhibits CaV2.2 channels and displays an anti-nociceptive activity. Mar. Drugs 11, 1188–1202. doi: 10.3390/md11041188
Bernaldez-Sarabia J., Figueroa-Montiel A., Duenas S., Cervantes-Luevano K., Beltran J. A., Ortiz E., et al. (2019). The diversified O-superfamily in californiconus californicus presents a conotoxin with antimycobacterial activity. Toxins (Basel) 11, 128. doi: 10.3390/toxins11020128
Bouchet P., Kantor Y. I., Sysoev A., Puillandre N. (2011). A new operational classification of the conoidea (Gastropoda). J. Molluscan Stud. 77, 273–308. doi: 10.1093/mollus/eyr017
Bulaj G., West P. J., Garrett J. E., Watkins M., Zhang M. M., Norton R. S., et al. (2005). Novel conotoxins from conus striatus and conus kinoshitai selectively block TTX-resistant sodium channels. Biochemistry 44, 7259–7265. doi: 10.1021/bi0473408
Cacho R. A., Tang Y., Chooi Y. H. (2014). Next-generation sequencing approach for connecting secondary metabolites to biosynthetic gene clusters in fungi. Front. Microbiol. 5, 774. doi: 10.3389/fmicb.2014.00774
Casewell N. R., Wuster W., Vonk F. J., Harrison R. A., Fry B. G. (2013). Complex cocktails: the evolutionary novelty of venoms. Trends Ecol. Evol. 28, 219–229. doi: 10.1016/j.tree.2012.10.020
Craig A. G., Zafaralla G., Cruz L. J., Santos A. D., Hillyard D. R., Dykert J., et al. (1998). An O-glycosylated neuroexcitatory conus peptide. Biochemistry 37, 16019–16025. doi: 10.1021/bi981690a
Duda T. F., JR., Kohn A. J. (2005). Species-level phylogeography and evolutionary history of the hyperdiverse marine gastropod genus conus. Mol. Phylogenet Evol. 34, 257–272. doi: 10.1016/j.ympev.2004.09.012
Dutertre S., Jin A. H., Kaas Q., Jones A., Alewood P. F., Lewis R. J. (2013). Deep venomics reveals the mechanism for expanded peptide diversity in cone snail venom. Mol. Cell Proteomics 12, 312–329. doi: 10.1074/mcp.M112.021469
Dutertre S., Jin A. H., Vetter I., Hamilton B., Sunagar K., Lavergne V., et al. (2014). Evolution of separate predation- and defence-evoked venoms in carnivorous cone snails. Nat. Commun. 5, 3521. doi: 10.1038/ncomms4521
Dutt M., Dutertre S., Jin A. H., Lavergne V., Alewood P. F., Lewis R. J. (2019). Venomics reveals venom complexity of the piscivorous cone snail, conus tulipa. Mar. Drugs 17, 71. doi: 10.3390/md17010071
Edgar R. C. (2004). MUSCLE: multiple sequence alignment with high accuracy and high throughput. Nucleic Acids Res. 32, 1792–1797. doi: 10.1093/nar/gkh340
Figueroa-Montiel A., Bernaldez J., Jimenez S., Ueberhide B., Gonzalez L. J., Licea-Navarro A. (2018). Antimycobacterial activity: A new pharmacological target for conotoxins found in the first reported conotoxin from conasprella ximenes. Toxins (Basel) 10, 51. doi: 10.3390/toxins10020051
Franco A., Dovell S., Moller C., Grandal M., Clark E., Mari F. (2018). Structural plasticity of mini-m conotoxins - expression of all mini-m subtypes by conus regius. FEBS J. 285, 887–902. doi: 10.1111/febs.14372
Gao B., Peng C., Yang J., Yi Y., Zhang J., Shi Q. (2017). Cone snails: A big store of conotoxins for novel drug discovery. Toxins (Basel) 9, 397. doi: 10.3390/toxins9120397
Gao B., Peng C., Zhu Y., Sun Y., Zhao T., Huang Y., et al. (2018). High throughput identification of novel conotoxins from the vermivorous oak cone snail (Conus quercinus) by transcriptome sequencing. Int. J. Mol. Sci. 19, 3901. doi: 10.3390/ijms19123901
Gilly W. F., Richmond T. A., Duda T. F. Jr., Elliger C., Lebaric Z., Schulz Bingham J.J. P., et al. (2011). A diverse family of novel peptide toxins from an unusual cone snail, conus californicus. J. Exp. Biol. 214, 147–161. doi: 10.1242/jeb.046086
Grabherr M. G., Haas B. J., Yassour M., Levin J. Z., Thompson D. A., Amit I., et al. (2011). Full-length transcriptome assembly from RNA-seq data without a reference genome. Nat. Biotechnol. 29, 644–652. doi: 10.1038/nbt.1883
Han P., Cao Y., Liu S., Dai X., Yao G., Fan C., et al. (2017). Contryphan-bt: A pyroglutamic acid containing conopeptide isolated from the venom of conus betulinus. Toxicon 135, 17–23. doi: 10.1016/j.toxicon.2017.05.022
Hansson K., Ma X., Eliasson L., Czerwiec E., Furie B., Furie B. C., et al. (2004). The first gamma-carboxyglutamic acid-containing contryphan. a selective l-type calcium ion channel blocker isolated from the venom of conus marmoreus. J. Biol. Chem. 279, 32453–32463. doi: 10.1074/jbc.M313825200
He L., Fu S., Xu Z., Yan J., Xu J., Zhou H., et al. (2017). Hybrid sequencing of full-length cDNA transcripts of stems and leaves in dendrobium officinale. Genes (Basel) 8, 257. doi: 10.3390/genes8100257
Himaya S. W., Jin A. H., Dutertre S., Giacomotto J., Mohialdeen H., Vetter I., et al. (2015). Comparative venomics reveals the complex prey capture strategy of the piscivorous cone snail conus catus. J. Proteome Res. 14, 4372–4381. doi: 10.1021/acs.jproteome.5b00630
Himaya S. W. A., Jin A. H., Hamilton B., Rai S. K., Alewood P., Lewis R. J. (2021). Venom duct origins of prey capture and defensive conotoxins in piscivorous conus striatus. Sci. Rep. 11, 13282. doi: 10.1038/s41598-021-91919-4
Hu H., Bandyopadhyay P. K., Olivera B. M., Yandell M. (2011). Characterization of the conus bullatus genome and its venom-duct transcriptome. BMC Genomics 12, 60. doi: 10.1186/1471-2164-12-60
Imperial J. S., Kantor Y., Watkins M., Heralde F. M. 3rd, Stevenson B., Chen P., et al. (2007). Venomous auger snail hastula (Impages) hectica (Linnaeu): molecular phylogeny, foregut anatomy and comparative toxinology. J. Exp. Zool B Mol. Dev. Evol. 308, 744–756. doi: 10.1002/jez.b.21195
Jacob R. B., Mcdougal O. M. (2010). The m-superfamily of conotoxins: a review. Cell Mol. Life Sci. 67, 17–27. doi: 10.1007/s00018-009-0125-0
Jagonia R. V. S., Dela Victoria R. G., Bajo L. M., Tan R. S. (2019). Conus striatus venom exhibits non-hepatotoxic and non-nephrotoxic potent analgesic activity in mice. Mol. Biol. Rep. 46, 5479–5486. doi: 10.1007/s11033-019-04875-8
Jiang S., Tae H. S., Xu S., Shao X., Adams D. J., Wang C. (2017). Identification of a novel O-conotoxin reveals an unusual and potent inhibitor of the human alpha9alpha10 nicotinic acetylcholine receptor. Mar. Drugs 15, 170. doi: 10.3390/md15060170
Jin A. H., Dutertre S., Dutt M., Lavergne V., Jones A., Lewis R. J., et al. (2019a). Transcriptomic-proteomic correlation in the predation-evoked venom of the cone snail, conus imperialis. Mar. Drugs 17, 177. doi: 10.3390/md17030177
Jin A. H., Muttenthaler M., Dutertre S., Himaya S. W. A., Kaas Q., Craik D. J., et al. (2019b). Conotoxins: Chemistry and biology. Chem. Rev. 119, 11510–11549. doi: 10.1021/acs.chemrev.9b00207
Jouiaei M., Yanagihara A. A., Madio B., Nevalainen T. J., Alewood P. F., Fry B. G. (2015). Ancient venom systems: A review on cnidaria toxins. Toxins (Basel) 7, 2251–2271. doi: 10.3390/toxins7062251
Kaas Q., Westermann J. C., Craik D. J. (2010). Conopeptide characterization and classifications: an analysis using ConoServer. Toxicon 55, 1491–1509. doi: 10.1016/j.toxicon.2010.03.002
Kaas Q., Westermann J. C., Halai R., Wang C. K., Craik D. J. (2008). ConoServer, a database for conopeptide sequences and structures. Bioinformatics 24, 445–446. doi: 10.1093/bioinformatics/btm596
Kaas Q., Yu R., Jin A. H., Dutertre S., Craik D. J. (2012). ConoServer: updated content, knowledge, and discovery tools in the conopeptide database. Nucleic Acids Res. 40, D325–D330. doi: 10.1093/nar/gkr886
Kanehisa M., Furumichi M., Tanabe M., Sato Y., Morishima K. (2017). KEGG: new perspectives on genomes, pathways, diseases and drugs. Nucleic Acids Res. 45, D353–D361. doi: 10.1093/nar/gkw1092
Kanehisa M., Goto S., Hattori M., Aoki-Kinoshita K. F., Itoh M., Kawashima S., et al. (2006). From genomics to chemical genomics: new developments in KEGG. Nucleic Acids Res. 34, D354–D357. doi: 10.1093/nar/gkj102
Kanehisa M., Goto S., Kawashima S., Okuno Y., Hattori M. (2004). The KEGG resource for deciphering the genome. Nucleic Acids Res. 32, D277–D280. doi: 10.1093/nar/gkh063
Kuang X., Sun S., Wei J., Li Y., Sun C. (2019). Iso-seq analysis of the taxus cuspidata transcriptome reveals the complexity of taxol biosynthesis. BMC Plant Biol. 19, 210. doi: 10.1186/s12870-019-1809-8
Kumar S., Stecher G., Tamura K. (2016). MEGA7: Molecular evolutionary genetics analysis version 7.0 for bigger datasets. Mol. Biol. Evol. 33, 1870–1874. doi: 10.1093/molbev/msw054
Laht S., Koua D., Kaplinski L., Lisacek F., Stocklin R., Remm M. (2012). Identification and classification of conopeptides using profile hidden Markov models. Biochim. Biophys. Acta 1824, 488–492. doi: 10.1016/j.bbapap.2011.12.004
Langdon W. B. (2015). Performance of genetic programming optimised Bowtie2 on genome comparison and analytic testing (GCAT) benchmarks. BioData Min 8, 1. doi: 10.1186/s13040-014-0034-0
Lavezzo E., Barzon L., Toppo S., Palu G. (2016). Third generation sequencing technologies applied to diagnostic microbiology: benefits and challenges in applications and data analysis. Expert Rev. Mol. Diagn. 16, 1011–1023. doi: 10.1080/14737159.2016.1217158
Lebbe E. K., Tytgat J. (2016). In the picture: disulfide-poor conopeptides, a class of pharmacologically interesting compounds. J. Venom Anim. Toxins Incl Trop. Dis. 22, 30. doi: 10.1186/s40409-016-0083-6
Levene M. J., Korlach J., Turner S. W., Foquet M., Craighead H. G., Webb W. W. (2003). Zero-mode waveguides for single-molecule analysis at high concentrations. Science 299, 682–686. doi: 10.1126/science.1079700
Lewis R. J., Dutertre S., Vetter I., Christie M. J. (2012). Conus venom peptide pharmacology. Pharmacol. Rev. 64, 259–298. doi: 10.1124/pr.111.005322
Li X., Chen W., Zhangsun D., Luo S. (2020). Diversity of conopeptides and their precursor genes of conus litteratus. Mar. Drugs 18, 464. doi: 10.3390/md18090464
Li B., Dewey C. N. (2011). RSEM: accurate transcript quantification from RNA-seq data with or without a reference genome. BMC Bioinf. 12, 323. doi: 10.1186/1471-2105-12-323
Li W., Godzik A. (2006). Cd-hit: a fast program for clustering and comparing large sets of protein or nucleotide sequences. Bioinformatics 22, 1658–1659. doi: 10.1093/bioinformatics/btl158
Li W., Jaroszewski L., Godzik A. (2002). Tolerating some redundancy significantly speeds up clustering of large protein databases. Bioinformatics 18, 77–82. doi: 10.1093/bioinformatics/18.1.77
Liu S., Chen G., Xu H., Zou W., Yan W., Wang Q., et al. (2017). Transcriptome analysis of mud crab (Scylla paramamosain) gills in response to mud crab reovirus (MCRV). Fish Shellfish Immunol. 60, 545–553. doi: 10.1016/j.fsi.2016.07.033
Livett B. G., Gayler K. R., Khalil Z. (2004). Drugs from the sea: conopeptides as potential therapeutics. Curr. Med. Chem. 11, 1715–1723. doi: 10.2174/0929867043364928
Li A., Zhang J., Zhou Z. (2014). PLEK: a tool for predicting long non-coding RNAs and messenger RNAs based on an improved k-mer scheme. BMC Bioinf. 15, 311. doi: 10.1186/1471-2105-15-311
Lluisma A. O., Milash B. A., Moore B., Olivera B. M., Bandyopadhyay P. K. (2012). Novel venom peptides from the cone snail conus pulicarius discovered through next-generation sequencing of its venom duct transcriptome. Mar. Genomics 5, 43–51. doi: 10.1016/j.margen.2011.09.002
Luo S., Christensen S., Zhangsun D., Wu Y., Hu Y., Zhu X., et al. (2013). A novel inhibitor of alpha9alpha10 nicotinic acetylcholine receptors from conus vexillum delineates a new conotoxin superfamily. PloS One 8, e54648. doi: 10.1371/journal.pone.0054648
Lu A., Watkins M., Li Q., Robinson S. D., Concepcion G. P., Yandell M., et al. (2020). Transcriptomic profiling reveals extraordinary diversity of venom peptides in unexplored predatory gastropods of the genus clavus. Genome Biol. Evol. 12, 684–700. doi: 10.1093/gbe/evaa083
Lu A., Yang L., Xu S., Wang C. (2014). Various conotoxin diversifications revealed by a venomic study of conus flavidus. Mol. Cell Proteomics 13, 105–118. doi: 10.1074/mcp.M113.028647
Macrander J., Panda J., Janies D., Daly M., Reitzel A. M. (2018). Venomix: a simple bioinformatic pipeline for identifying and characterizing toxin gene candidates from transcriptomic data. PeerJ 6, e5361. doi: 10.7717/peerj.5361
Manuel J., Tenorio T., Eichhorst, Tucker J. K. (2013). Illustrated catalog of the living cone shells. Am. Conchologist 41, 16–19.
Massilia G. R., Schinina M. E., Ascenzi P., Polticelli F. (2001). Contryphan-vn: a novel peptide from the venom of the Mediterranean snail conus ventricosus. Biochem. Biophys. Res. Commun. 288, 908–913. doi: 10.1006/bbrc.2001.5833
Modica M. V., Holford M. (2010). The neogastropoda: Evolutionary innovations of predatory marine snails with remarkable pharmacological potential. Evolutionary Biol. – Concepts Mol. Morphological Evol: 249–270. doi: 10.1007/978-3-642-12340-5_15
Nielsen K. J., Thomas L., Lewis R. J., Alewood P. F., Craik D. J. (1996). A consensus structure for omega-conotoxins with different selectivities for voltage-sensitive calcium channel subtypes: comparison of MVIIA, SVIB and SNX-202. J. Mol. Biol. 263, 297–310. doi: 10.1006/jmbi.1996.0576
Olivera B. M., Walker C., Cartier G. E., Hooper D., Santos A. D., Schoenfeld R., et al. (1999). Speciation of cone snails and interspecific hyperdivergence of their venom peptides. potential evolutionary significance of introns. Ann. N Y Acad. Sci. 870, 223–237. doi: 10.1111/j.1749-6632.1999.tb08883.x
Ozsolak F. (2012). Third-generation sequencing techniques and applications to drug discovery. Expert Opin. Drug Discovery 7, 231–243. doi: 10.1517/17460441.2012.660145
Pardos-Blas J. R., Irisarri I., Abalde S., Tenorio M. J., Zardoya R. (2019). Conotoxin diversity in the venom gland transcriptome of the magician's cone, pionoconus magus. Mar. Drugs 17, 553. doi: 10.3390/md17100553
Peng C., Huang Y., Bian C., Li J., Liu J., Zhang K., et al. (2021). The first conus genome assembly reveals a primary genetic central dogma of conopeptides in c. betulinus. Cell Discovery 7, 11. doi: 10.1038/s41421-021-00244-7
Peng C., Yao G., Gao B. M., Fan C. X., Bian C., Wang J., et al. (2016). High-throughput identification of novel conotoxins from the Chinese tubular cone snail (Conus betulinus) by multi-transcriptome sequencing. Gigascience 5, 17. doi: 10.1186/s13742-016-0122-9
Pertea G., Huang X., Liang F., Antonescu V., Sultana R., Karamycheva S., et al. (2003). TIGR gene indices clustering tools (TGICL): a software system for fast clustering of large EST datasets. Bioinformatics 19, 651–652. doi: 10.1093/bioinformatics/btg034
Pi C., Liu Y., Peng C., Jiang X., Liu J., Xu B., et al. (2006). Analysis of expressed sequence tags from the venom ducts of conus striatus: focusing on the expression profile of conotoxins. Biochimie 88, 131–140. doi: 10.1016/j.biochi.2005.08.001
Prashanth J. R., Dutertre S., Jin A. H., Lavergne V., Hamilton B., Cardoso F. C., et al. (2016). The role of defensive ecological interactions in the evolution of conotoxins. Mol. Ecol. 25, 598–615. doi: 10.1111/mec.13504
Puillandre N., Koua D., Favreau P., Olivera B. M., Stocklin R. (2012). Molecular phylogeny, classification and evolution of conopeptides. J. Mol. Evol. 74, 297–309. doi: 10.1007/s00239-012-9507-2
Puillandre N., Watkins M., Olivera B. M. (2010). Evolution of conus peptide genes: duplication and positive selection in the a-superfamily. J. Mol. Evol. 70, 190–202. doi: 10.1007/s00239-010-9321-7
Rajaian Pushpabai R., Wilson Alphonse C. R., Mani R., Arun A. D., Franklin J. B. (2021). Diversity of conopeptides and conoenzymes from the venom duct of the marine cone snail conus bayani as determined from transcriptomic and proteomic analyses. Mar. Drugs 19, 202. doi: 10.3390/md19040202
Rajesh R. P. (2015). Novel m-superfamily and T-superfamily conotoxins and contryphans from the vermivorous snail conus figulinus. J. Pept. Sci. 21, 29–39. doi: 10.1002/psc.2715
Robinson S. D., Li Q., Bandyopadhyay P. K., Gajewiak J., Yandell M., Papenfuss A. T., et al. (2017). Hormone-like peptides in the venoms of marine cone snails. Gen. Comp. Endocrinol. 244, 11–18. doi: 10.1016/j.ygcen.2015.07.012
Robinson S. D., Norton R. S. (2014). Conotoxin gene superfamilies. Mar. Drugs 12, 6058–6101. doi: 10.3390/md12126058
Sabareesh V., Gowd K. H., Ramasamy P., Sudarslal S., Krishnan K. S., Sikdar S. K., et al. (2006). Characterization of contryphans from conus loroisii and conus amadis that target calcium channels. Peptides 27, 2647–2654. doi: 10.1016/j.peptides.2006.07.009
Safavi-Hemami H., Gajewiak J., Karanth S., Robinson S. D., Ueberheide B., Douglass A. D., et al. (2015). Specialized insulin is used for chemical warfare by fish-hunting cone snails. Proc. Natl. Acad. Sci. U.S.A. 112, 1743–1748. doi: 10.1073/pnas.1423857112
Safavi-Hemami H., Hu H., Gorasia D. G., Bandyopadhyay P. K., Veith P. D., Young N. D., et al. (2014). Combined proteomic and transcriptomic interrogation of the venom gland of conus geographus uncovers novel components and functional compartmentalization. Mol. Cell Proteomics 13, 938–953. doi: 10.1074/mcp.M113.031351
Santos A. D., Mcintosh J. M., Hillyard D. R., Cruz L. J., Olivera B. M. (2004). The a-superfamily of conotoxins: structural and functional divergence. J. Biol. Chem. 279, 17596–17606. doi: 10.1074/jbc.M309654200
Schroeder C. I., Ekberg J., Nielsen K. J., Adams D., Loughnan M. L., Thomas L., et al. (2008). Neuronally micro-conotoxins from conus striatus utilize an alpha-helical motif to target mammalian sodium channels. J. Biol. Chem. 283, 21621–21628. doi: 10.1074/jbc.M802852200
Shimizu K., Adachi J., Muraoka Y. (2006). ANGLE: a sequencing errors resistant program for predicting protein coding regions in unfinished cDNA. J. Bioinform. Comput. Biol. 4, 649–664. doi: 10.1142/S0219720006002260
Soudy M., Anwar A. M., Ahmed E. A., Osama A., Ezzeldin S., Mahgoub S., et al. (2020). UniprotR: Retrieving and visualizing protein sequence and functional information from universal protein resource (UniProt knowledgebase). J. Proteomics 213, 103613. doi: 10.1016/j.jprot.2019.103613
Tatusov R. L., Natale D. A., Garkavtsev I. V., Tatusova T. A., Shankavaram U. T., Rao B. S., et al. (2001). The COG database: new developments in phylogenetic classification of proteins from complete genomes. Nucleic Acids Res. 29, 22–28. doi: 10.1093/nar/29.1.22
Terlau H., Olivera B. M. (2004). Conus venoms: a rich source of novel ion channel-targeted peptides. Physiol. Rev. 84, 41–68. doi: 10.1152/physrev.00020.2003
Terrat Y., Biass D., Dutertre S., Favreau P., Remm M., Stocklin R., et al. (2012). High-resolution picture of a venom gland transcriptome: case study with the marine snail conus consors. Toxicon 59, 34–46. doi: 10.1016/j.toxicon.2011.10.001
Thiel T., Michalek W., Varshney R. K., Graner A. (2003). Exploiting EST databases for the development and characterization of gene-derived SSR-markers in barley (Hordeum vulgare l.). Theor. Appl. Genet. 106, 411–422. doi: 10.1007/s00122-002-1031-0
Thompson J. D., Higgins D. G., Gibson T. J. (1994). CLUSTAL W: improving the sensitivity of progressive multiple sequence alignment through sequence weighting, position-specific gap penalties and weight matrix choice. Nucleic Acids Res. 22, 4673–4680. doi: 10.1093/nar/22.22.4673
Travers K. J., Chin C. S., Rank D. R., Eid J. S., Turner S. W. (2010). A flexible and efficient template format for circular consensus sequencing and SNP detection. Nucleic Acids Res. 38, e159. doi: 10.1093/nar/gkq543
Uniprot C. (2021). UniProt: the universal protein knowledgebase in 2021. Nucleic Acids Res. 49, D480–D489. doi: 10.1093/nar/gkaa1100
Vetter I., Lewis R. J. (2012). Therapeutic potential of cone snail venom peptides (conopeptides). Curr. Top. Med. Chem. 12, 1546–1552. doi: 10.2174/156802612802652457
Walker C. S., Jensen S., Ellison M., Matta J. A., Lee W. Y., Imperial J. S., et al. (2009). A novel conus snail polypeptide causes excitotoxicity by blocking desensitization of AMPA receptors. Curr. Biol. 19, 900–908. doi: 10.1016/j.cub.2009.05.017
Wang Y., Alangari M., Hihath J., Das A. K., Anantram M. P. (2021). A machine learning approach for accurate and real-time DNA sequence identification. BMC Genomics 22, 525. doi: 10.1186/s12864-021-07841-6
Wang C. Z., Jiang H., Ou Z. L., Chen J. S., Chi C. W. (2003). cDNA cloning of two a-superfamily conotoxins from conus striatus. Toxicon 42, 613–619. doi: 10.1016/j.toxicon.2003.08.005
Wang M., Wang P., Liang F., Ye Z., Li J., Shen C., et al. (2018). A global survey of alternative splicing in allopolyploid cotton: landscape, complexity and regulation. New Phytol. 217, 163–178. doi: 10.1111/nph.14762
Wang L., Wang X., Ren Z., Tang W., Zou Q., Wang J., et al. (2017). Oxidative folding of conopeptides modified by conus protein disulfide isomerase. Protein J. 36, 407–416. doi: 10.1007/s10930-017-9738-6
Ward C. W., Lawrence M. C. (2011). Landmarks in insulin research. Front. Endocrinol. (Lausanne) 2, 76. doi: 10.3389/fendo.2011.00076
Wheeler T. J., Eddy S. R. (2013). Nhmmer: DNA homology search with profile HMMs. Bioinformatics 29, 2487–2489. doi: 10.1093/bioinformatics/btt403
Wu Y., Wang L., Zhou M., You Y., Zhu X., Qiang Y., et al. (2013). Molecular evolution and diversity of conus peptide toxins, as revealed by gene structure and intron sequence analyses. PloS One 8, e82495. doi: 10.1371/journal.pone.0082495
Xie B., Dashevsky D., Rokyta D., Ghezellou P., Fathinia B., Shi Q., et al. (2022). Dynamic genetic differentiation drives the widespread structural and functional convergent evolution of snake venom proteinaceous toxins. BMC Biol. 20, 4. doi: 10.1186/s12915-021-01208-9
Xue W., Li J. T., Zhu Y. P., Hou G. Y., Kong X. F., Kuang Y. Y., et al. (2013). L_RNA_scaffolder: scaffolding genomes with transcripts. BMC Genomics 14, 604. doi: 10.1186/1471-2164-14-604
Xu Z., Peters R. J., Weirather J., Luo H., Liao B., Zhang X., et al. (2015). Full-length transcriptome sequences and splice variants obtained by a combination of sequencing platforms applied to different root tissues of salvia miltiorrhiza and tanshinone biosynthesis. Plant J. 82, 951–961. doi: 10.1111/tpj.12865
Yang X., Ikhwanuddin M., Li X., Lin F., Wu Q., Zhang Y., et al. (2018b). Comparative transcriptome analysis provides insights into differentially expressed genes and long non-coding RNAs between ovary and testis of the mud crab (Scylla paramamosain). Mar. Biotechnol. (NY) 20, 20–34. doi: 10.1007/s10126-017-9784-2
Yang L., Jin Y., Huang W., Sun Q., Liu F., Huang X. (2018a). Full-length transcriptome sequences of ephemeral plant arabidopsis pumila provides insight into gene expression dynamics during continuous salt stress. BMC Genomics 19, 717. doi: 10.1186/s12864-018-5106-y
Yang M., Zhou M. (2020). Insertions and deletions play an important role in the diversity of conotoxins. Protein J. 39, 190–195. doi: 10.1007/s10930-020-09892-2
Yao G., Peng C., Zhu Y., Fan C., Jiang H., Chen J., et al. (2019). High-throughput identification and analysis of novel conotoxins from three vermivorous cone snails by transcriptome sequencing. Mar. Drugs 17, 193. doi: 10.3390/md17030193
Yao S., Zhang M. M., Yoshikami D., Azam L., Olivera B. M., Bulaj G., et al. (2008). Structure, dynamics, and selectivity of the sodium channel blocker mu-conotoxin SIIIA. Biochemistry 47, 10940–10949. doi: 10.1021/bi801010u
Ye J., Fang L., Zheng H., Zhang Y., Chen J., Zhang Z., et al. (2006). WEGO: a web tool for plotting GO annotations. Nucleic Acids Res. 34, W293–W297. doi: 10.1093/nar/gkl031
Ye M., Khoo K. K., Xu S., Zhou M., Boonyalai N., Perugini M. A., et al. (2012). A helical conotoxin from conus imperialis has a novel cysteine framework and defines a new superfamily. J. Biol. Chem. 287, 14973–14983. doi: 10.1074/jbc.M111.334615
Ye Z., Wu Y., Ul Haq Muhammad Z., Yan W., Yu J., Zhang J., et al. (2020). Complementary transcriptome and proteome profiling in the mature seeds of camellia oleifera from hainan island. PloS One 15, e0226888. doi: 10.1371/journal.pone.0226888
Ye J., Zhang Y., Cui H., Liu J., Wu Y., Cheng Y., et al. (2018). WEGO 2.0: a web tool for analyzing and plotting GO annotation Update. Nucleic Acids Res. 46, W71–W75. doi: 10.1093/nar/gky400
Yuan Z., Ge L., Sun J., Zhang W., Wang S., Cao X., et al. (2021). Integrative analysis of iso-seq and RNA-seq data reveals transcriptome complexity and differentially expressed transcripts in sheep tail fat. PeerJ 9, e12454. doi: 10.7717/peerj.12454
Zamora-Bustillos R., Martinez-Nunez M. A., Aguilar M. B., Colli-Dula R. C., Brito-Dominguez D. A. (2021). Identification of novel conotoxin precursors from the cone snail conus spurius by high-throughput RNA sequencing. Mar. Drugs 19:547. doi: 10.3390/md19100547
Zeng D., Chen X., Peng J., Yang C., Peng M., Zhu W., et al. (2018). Single-molecule long-read sequencing facilitates shrimp transcriptome research. Sci. Rep. 8, 16920. doi: 10.1038/s41598-018-35066-3
Zhang C., Chen J., Huang W., Song X., Niu J. (2021). Transcriptomics and metabolomics reveal purine and phenylpropanoid metabolism response to drought stress in dendrobium sinense, an endemic orchid species in hainan island. Front. Genet. 12, 692702. doi: 10.3389/fgene.2021.692702
Zhang H., Fu Y., Wang L., Liang A., Chen S., Xu A. (2019). Identifying novel conopepetides from the venom ducts of conus litteratus through integrating transcriptomics and proteomics. J. Proteomics 192, 346–357. doi: 10.1016/j.jprot.2018.09.015
Zhan Y., Wu T., Zhao X., Wang Z., Chen Y. (2021). Comparative physiological and full-length transcriptome analyses reveal the molecular mechanism of melatonin-mediated salt tolerance in okra (Abelmoschus esculentus l.). BMC Plant Biol. 21, 180. doi: 10.1186/s12870-021-02957-z
Keywords: cone snail, Conus striatus, conotoxin, transcriptome sequencing, next-generation sequencing technology, third-generation sequencing technology
Citation: Liao Y, Peng C, Zhu Y, Fu J, Ruan Z, Shi Q and Gao B (2022) High conopeptide diversity in Conus striatus: Revealed by integration of two transcriptome sequencing platforms. Front. Mar. Sci. 9:1060432. doi: 10.3389/fmars.2022.1060432
Received: 03 October 2022; Accepted: 26 October 2022;
Published: 15 November 2022.
Edited by:
Zhongshan Zhang, Huzhou University, ChinaReviewed by:
Bing Xie, European Bioinformatics Institute (EMBL-EBI), United KingdomTianming Wang, Zhejiang Ocean University, China
Ying Fu, Hainan University, China
Copyright © 2022 Liao, Peng, Zhu, Fu, Ruan, Shi and Gao. This is an open-access article distributed under the terms of the Creative Commons Attribution License (CC BY). The use, distribution or reproduction in other forums is permitted, provided the original author(s) and the copyright owner(s) are credited and that the original publication in this journal is cited, in accordance with accepted academic practice. No use, distribution or reproduction is permitted which does not comply with these terms.
*Correspondence: Qiong Shi, shiqiong@genomics.cn; Bingmiao Gao, gaobingmiao@hainmc.edu.cn
†These authors have contributed equally to this work and share first authorship