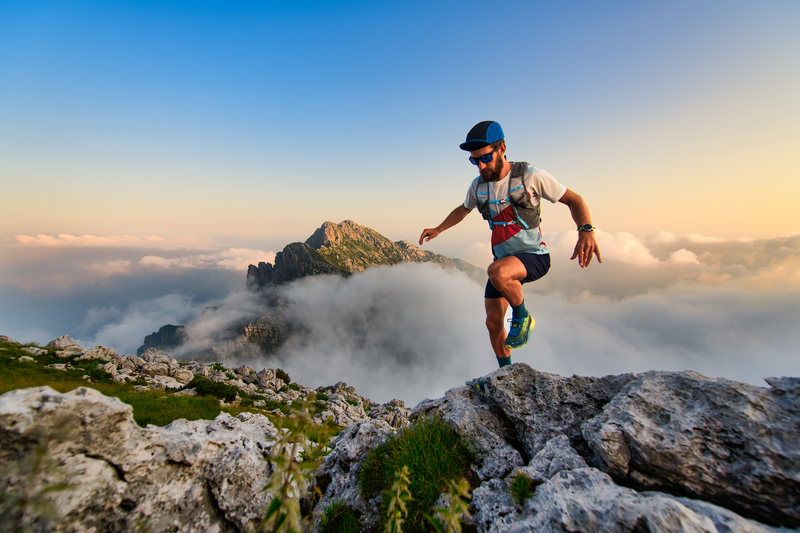
95% of researchers rate our articles as excellent or good
Learn more about the work of our research integrity team to safeguard the quality of each article we publish.
Find out more
ORIGINAL RESEARCH article
Front. Mar. Sci. , 27 September 2022
Sec. Marine Molecular Biology and Ecology
Volume 9 - 2022 | https://doi.org/10.3389/fmars.2022.1027621
This article is part of the Research Topic Better Understanding the Impacts of Global Climate Change on Aquatic Species by Bridging The Gap Between Omics and Marine Science View all 5 articles
Fish-associated microbiota are an integral part to the health of the host fish. The ongoing climate changes including global warming of water may disrupt the composition and diversity of host-microbiota, and subsequently, destabilize the fish homeostasis. Since the knowledge on temperature-sensitive marine fish and environmental bacteria is scarce, we investigated the effects of rearing temperatures on community structure, diversity and assembly process of bacteria on chum salmon (Oncorhynchus keta), which is a temperate salmon species found in the Pacific. Over the course of two weeks, laboratory-raised chum salmon were exposed to three temperatures: high (18°C), low (8°C) and, control (13°C). Their feces, cutaneous mucus, and surrounding water were sampled for community structure analysis based on 16S rRNA gene amplicon sequencing. Temperature changes from the control level triggered significant dysbiosis in the fecal and skin mucus microbiota. In particular, Vibrio and Tenacibaculum sequence variants were highly abundant at high and low temperatures, respectively, and the opportunistic growth of these pathogenic species may impede host immunity. Two temperature-specific taxonomic microbial biomarkers, the class Betaproteobacteria and the genus Flavobacterium were identified at both high and low temperatures. An analysis of bacterial community assembly processes revealed that environmental selection significantly affected the gut microbial community assembly, while the assembly process of the skin microbiota was stochastic. Our study elucidated the potential crisis of fish health when the equilibrium of the cutaneous and intestinal microbiota was disrupted by temperature changes. Our data will be a valuable tool to better understand the effects of climate change, a very pressing and important challenge now and in the future, on the fish microbiota and its homeostasis.
Fish are constantly in contact with surrounding microbes from the environment. These microbes can be commensal, mutualistic, or pathogenic. Symbiotic bacteria have been reported to be abundant in the gastrointestinal tract (GIT) (Bates et al., 2006, Rawls et al., 2006) and cutaneous mucus (Boutin et al., 2014) of fish. The gut microbiota is often regarded as an “extra organ”, as it plays a vital role in maintaining the homeostasis of the host (Butt and Volkoff, 2019), including functions in immunity (Round and Mazmanian, 2009), growth (Li et al., 2013), behavior (Heijtza et al., 2011) and energy utilization (Chevalier et al., 2015). The fish skin microbiota is inhabited by both commensal and opportunistic bacterial populations found in cutaneous mucus (Minniti et al., 2017). The mucus is an external layer covering the skin’s outermost surface and serves as an initial biological, physical, and chemical barrier against infections (Benhamed et al., 2014) as well as the first point of interaction between pathogens and skin cells (Boutin et al., 2013, Trivedi, 2012, Sugita et al., 2002). Mucus has a complex composition that contains several antimicrobial agents secreted by the skin cells of fish, such as lysozymes, lectins, immunoglobulins, lysins, and agglutinins. (Barchi, 2013), and thus acts as a shield to protect the fish from pathogenic infection. Previous studies have revealed that the mucus of some fish contains antimicrobial peptides (Richards et al., 2001). These components can specifically target pathogenic bacteria and protect fish from pathogen infection (Esteban, 2012). Thus, the gut and skin microbiota show strong interactions with their host and play an indispensable role in the health of the fish.
Fluctuations in environmental factors have been reported to disrupt the composition and diversity of the gut and skin mucus microbiota of healthy fish. The robustness and ability of these fish microbiota, likely determined by the diversity and composition of bacterial species, may affect the response of fish to disturbances. Disturbance factors, such as temperature, pH, oxygen, and salinity, can alter the equilibrium of the fish microbiota (Carlson et al., 2015, Boutin et al., 2013). Physiological stresses on the host may favor the overgrowth of opportunistic bacteria by disrupting the balance of commensal species relative abundance, a phenomenon known as dysbiosis in both mammals and fish (Tomasello et al., 2016, Wendling and Wegner, 2013, Stecher et al., 2013, Jones et al., 2014). Previous studies (Soriano et al., 2018, Neuman et al., 2016, Iguchi et al., 2003) examined the effects of temperature, diet, and rearing density as stressors on fish immune systems and reported a surge in disease vulnerability owing to the suppression of immunological parameters, highlighting the close association among stress, pathogenic bacteria, and immune system responses.
Microbial dysbiosis of teleosts can be caused by anoxia (Bello et al., 2017; Ito and Momii, 2015, Boutin et al., 2013), acidification (Koch et al., 2013; Zeebe et al., 2008), salinity changes (Bonte and Zwolsman, 2010; Ojaveer and Kalejs, 2005), exposure to surface-acting disinfectants (Mohammed et al., 2015), inappropriate diets (Green et al., 2013), and stress (Tomasello et al., 2016). Among dysbiosis-causing factors, water temperature is considered one of the most important factors in fish because they are ectothermic animals. Variations in water temperature can also affect the levels of dissolved oxygen, salinity, and pH, among other factors (Bello et al., 2017; Roessig et al., 2004; Xiong et al., 2013). Thus, temperature has individual as well as synergistic effects on fish (Djurichkovic et al., 2019; Pörtner, 2005; Wenger et al., 2016) and may in turn alter the bacterial composition and diversity. Previous reports have suggested that water temperature favors the growth of potentially pathogenic Vibrio species during warm seasons (Neuman et al., 2016). It is hypothesized that, many Vibrio species are barely pathogenic, but are opportunistic whose pathogenic nature is prominent under specific environments, such as, alterations in water quality parameters in controlled aquaculture systems (Thompson et al., 2004). Therefore, temperature changes may drive dysbiosis in the microbial consortia of fish.
Chum salmon, Oncorhynchus keta, is a commercially important species to fisheries, particularly in Japan. They are Pacific salmonid species that migrates from Asia to the coast of the Arctic Ocean (Salo, 1991, Abe et al., 2019). These fish are anadromous but are unique among salmonids because of their very short freshwater life stage, as their fry migrate toward the sea soon after their emergence (Abe et al., 2019). Chum salmon spend a considerable part of their life cycle in the Bering Sea, where the water temperatures rarely exceed 10°C. In Japan, the Sanriku coastal zones of Honshu Island are the southernmost natural breeding grounds of chum salmon, where fry experience dramatically fluctuating temperatures in the bay and at the sea surface (Abe et al., 2019; Tanaka et al., 2000).
To date, very limited documentation of the chum salmon microbiome is available, and the impacts of temperature change on the taxonomic composition of the microbiota of gut and skin are poorly understood, especially under controlled laboratory conditions. Various factors and ecological processes that shape microbial community assembly in chum salmon are also poorly understood. In this context, the present study was undertaken to explore (i) how temperature modulation affects the gut and skin microbiota of chum salmon and (ii) how the chum salmon microbiota is assembled? To address these questions, we randomly distributed 30 seawater-acclimated chum salmon into 3 independent tanks and exposed them to temperature stress for two consecutive weeks. The feces, skin mucus, and surrounding water of the fish were sampled on Day 0, Day 7, and Day 14, and the taxonomic composition of the microbial communities was characterized by targeting the V4-V5 regions of the 16S rRNA gene. We hypothesized that temperature changes trigger dysbiosis of the gut and skin microbiota, which is then replaced by opportunistic pathogens from the surrounding water or unique endogenous groups that are resilient to temperature fluctuations. This dysbiosis may risk the loss of homeostasis in the fish and make them susceptible to infectious diseases. We also hypothesized that the chum salmon gut and skin microbial communities are assembled via environmental selection or random drift, with continuous immigration of microbes from the surrounding water. Therefore, the objectives of the present study were to investigate how temperature modulation affects the gut and skin microbial profile of chum salmon.
The experiment was conducted using 30 seawater-acclimated chum salmon [Oncorhynchus keta (Walbaum, 1792)]. These fish hatched from artificially fertilized eggs from the Unosumai Hatchery, Iwate Prefecture, Northern Japan. They were reared for two years in the aquarium facility of the Atmosphere and Ocean Research Institute, The University of Tokyo, Japan. The fish used in the experiment had never been exposed to seawater before. To eliminate the effects of tanks and other variables, the proximity to human passage to the experimental site was minimal and intensive care was taken throughout the experiment. Prior to start of the experiment, the experimental tanks were thoroughly cleaned with 70% ethanol and air dried to minimize the carry-over of microbes. The water chemistry profile (temperature, dissolved oxygen, pH, salinity) was measured three times every day to ensure the ideal conditions for the experiment. A 14hL:10hD photoperiod was maintained throughout the experiment. Artificial seawater was used in the experiment, which was prepared by dissolving Aqua Salz artificial seawater powder (Nissei Sangyo, Tokyo, Japan) in an aseptic reserve tank. Three independent 250 L cylindrical tanks equipped with recirculating aquaculture system were filled with artificial seawater. Ten chum salmon individuals (~120g) were randomly selected from the stock tank (500 L, 13°C) and introduced to the experimental tanks at 13°C. Each tank is continuously aerated with a sterilized air stone. All the fish were acclimated to the experimental tanks for seven days before the start of the temperature experiment. The dissolved oxygen of the holding water was ~7 mg L-1, and the pH and salinity were ~7.1 and 34-35 PSU, respectively. Chum salmon were fed once a day with commercial pellets designed for salmonids (3.5-4.5 mm pellet size, Protein: >46.0%, Fat: >4.0%, Fiber: >3.0%, Carbohydrate: <15.0%, Calcium: >1.5%, Phosphate: >1.2% from Marubeni Nishin Feed Company, Chita city, Aichi Prefecture, Japan).
As reported by Richter and Kolmes (2005), the optimum temperature for rearing chum salmon is 13.5°C, and extended exposure to 22° to 23°C can be lethal (Hicks, 2000). Therefore, in the present study, we setup the control tank at 13°C, while a lower temperature is set at 8°C and a higher temperature is set at 18°C. A schematic overview of the experimental design, including the sampling plan for different temperatures and time intervals, is provided in Figure 1. Due to the limitation of facilities, only one tank per each temperature was feasible. The temperature of each experimental treatment was maintained at 13°C during the acclimatization period and then gradually increased or decreased at a rate of 1°C per day until the target temperatures were reached. Sampling in the control tank at 13°C was initiated after the seven-day acclimatization period, while sampling in the treatment groups was initiated after seven-day acclimatization period when reached the target temperature. Feces, skin mucus, and water were sampled from each tank on Day 0, Day 7, and Day 14. On a daily basis, feces were removed from the bottom of the tank with a sterile siphon to maintain the water quality. Unconsumed feed was removed daily. Fecal sampling was performed approximately 4-5 hours after feeding. The fish body was cut open to expose the digestive tract, and the intestine was tied off from small intestine to the rectum. The intestine was excised from the body under sterile condition and individual fecal samples were directly collected from the intestine by squeezing out the content into 15 mL tubes. For skin mucus sampling, three individuals from each tank were euthanized with an overdose of (200 mgl-1) tricaine methane sulfonate (MS-222) buffered by sodium bicarbonate during each sampling occasion. Skin mucus sampling was performed by gently rubbing ~50% of the total surface of the right side of each fish with a sterile cotton swab. Three liters of water were collected from each tank during each sampling occasion. Water samples were filtered through 0.22 µm membranes (Sterivex-GP Pressure Filter Unit, Millipore, Merck, Germany) to collect the microbial community in the rearing water. All samples, including feces, skin mucus, and membranes were stored at –80°C until further analyses.
Figure 1 Schematic illustration of the experimental design. A total of 30 fish were used in this experiment, including 10 in each of the control, low-temperature, and high-temperature tanks. Feces, skin mucus, and surrounding water were sampled in triplicates from each tank during each sampling point on Days 0, 7, and 14.
The QIAamp Fast DNA Stool Mini Kit (Qiagen, Germany) was used to extract genomic DNA from fish fecal samples according to the manufacturer’s protocol. The DNeasy Powersoil Pro (Qiagen, Germany) kit was used to extract DNA from cutaneous mucus samples and the 0.2 µm membranes from rearing water samples following the manufacturer’s protocol. The DNA concentration was checked with Quantus Fluorometer® using the Quantifluor® ONE DNA System Kit (Promega Corporation, USA), and DNA quality was assessed using a Nanodrop spectrophotometer following the manufacturer’s protocol. Until amplification, all the extracted DNA samples were kept at –30°C. The sequences of 16S rRNA genes were amplified using the primer set 515FB (5´- GTGYCAGCMGCCGCGGTAA-3´) (Parada et al., 2016) and 926R (5´- CCGYCAATTYMTTTRAGTTT-3´) (Liu et al., 1997), targeting the V4-V5 hypervariable regions. The KAPA HiFi HotStart ReadyMix PCR kit (Roche, USA) was used for PCR amplification following the manufacturer’s protocol. Each reaction tube contained 12.5 µL of KAPA HiFi HotStart ReadyMix and 1 µL of each primer. Volume was completed to 25 µL with PCR grade microbial DNA free water and template DNA. PCR was done in triplicates to reduce PCR bias with the following cycle conditions: initial denaturation at 98°C for 3 min, followed by 30 cycles of 98°C for 20 s, 56°C for 45 s, 72°C for 1 min, and concluded by a final extension at 72°C for 10 min. Electrophoresis on a 1.5% agarose gel was performed to check the quality of the amplicon DNA. After PCR, the 3 reaction replicates performed per sample were pooled in a 0.5 mL PCR tube and kept in a –30°C freezer until further analysis.
To remove primers, dimers, proteins, and phenol, the amplified DNA was purified by using AMPure XP beads (Beckman Coulter Genomics, Brea, CA, United States). The quality of the resulting DNA was checked by electrophoresis on a 1.5% agarose gel. The Nextera ® XT Index Kit v2 (Illumina, San Diego, CA, United States) Set A (96 indexes, 384 samples) and Set B (96 indexes, 384 samples) were used for library preparation. Index PCR was performed using KAPA HiFi HotStart ReadyMix® (Roche, USA) according to the manufacturer’s instructions. Index PCR was performed by combining 12.5 μl of 2X KAPA HiFi HotStart ReadyMix® (Roche, USA) and 2.5 μl of each index 1, index 2 and the purified PCR product per sample. The final reaction volume of 25 μl per sample was made up with nuclease-free PCR grade water. Amplification started with an initial denaturing step for 3 min at 95°C, followed by 8 cycles of denaturation for 30 s at 95°C, annealing for 30 s at 55°C and extension for 30 s at 72°C prior to a final extension for 5 mins at 72°C. The PCR product was then cleaned up using AMPure Beads following Illumina’s 16S Metagenomic Sequencing Library Preparation Protocol (Illumina, Part 15044223, Rev. B). 28 µL of AMPure XP beads was added to each sample. Samples were mixed manually by gently pipetting the entire volume up and down 10 times and incubated at room temperature without shaking. Using a magnetic stand, beads were concentrated on the side of the Eppendorfs and supernatant was removed. Beads were washed twice with freshly prepared 200 µL ethanol 80% and incubated 30 sec at room temperature during each wash. Supernatant was removed and beads were left to air-dry for 10 min. Tubes were removed from the magnetic stand and 13.75 µL of 10mM Tris pH 8.5 was added for elution. All samples were then quantified by Quantus Fluorometer® using the Quantifluor® ONE DNA System Kit (Promega Corporation, USA) following the manufacturer’s instructions. PCR samples were pooled at equimolar concentrations of 4 nM. The quality of the pooled samples was verified on an Agilent 2200 TapeStation® system with High Sensitivity D1000 reagents, followed by heat denaturation of the library, the addition of 10% PhiX, and dilution to 4 pM. The library was finally run on a single MiSeq flow cell using an Illumina ® MiSeq Reagent Kit v3 (Illumina, San Diego, CA, United States) (600 cycles; 2×300) (Catalog Number: MS9922924-600V3). All procedures followed the Illumina guide (16S Metagenomic Sequencing Library Preparation, Part 15044223, Rev. B).
The QIIME2 program (ver. 2020.6; Bolyen et al., 2019) was used to analyze the community profile of the reads. The Cutadapt plug-I (Martin, 2011) was used to eliminate primer sequences. The DADA2 plug-in (Callahan et al., 2016) was used to denoise and cluster the reads based on sequence variants (SVs) at a single-nucleotide resolution. The resulting SVs were searched against the Greengenes database (ver. 13.8; McDonald et al., 2012). The resulting amplicon sequence variant (ASV) table was filtered to exclude any sequences derived from mitochondrial or chloroplast DNA because these sequences are expected to be derived from the mitochondria of the host fish or the fish feed. Rarefaction was performed for adjustment to the minimum number of sequences. Alpha diversity metrics, such as the Chao1 richness and Shannon-Weiner diversity indices, were calculated after performing multiple rarefactions on the data to account for discrepancies in sequencing depth in QIIME2. Microbial community dissimilarity was calculated via nonmetric multidimensional scaling (NMDS) ordination analysis with Bray–Curtis distance and a suitable number of axes to reduce stress. R software v. 1.3.1093 (R core team) and the vegan package (Oksanen et al., 2018) were used for these statistical analyses. The PiCRUSt2 tool was used to observe the possible occurrence of virulence-related genes. The abundance of virulence related KEGG orthologs (KOs) was predicted using the Virulence Factor Database for 16S rRNA gene metagenomics (10.17632/rvxntbtscw.1) against KO abundance estimated using the PICRUSt2 algorithm. The relative abundance of virus-related KOs was calculated by dividing the virulence-related KOs by the total KO abundance predicted by PICRUSt2. Since the data was normally distributed, the two-way ANOVA with a post hoc Tukey’s HSD test was used to test for significant differences between samples collected at different time points under each temperature treatment. Permutational analysis of variance (PERMANOVA) in R software was performed to determine the effects of (i) temperature and (ii) time on the differences in the taxonomic composition of the bacterial communities across samples.
To assess the ecological processes that shape bacterial community assembly in the fish GIT and skin mucus, the nearest taxon index (NTI) was calculated as described by Webb (2000), and ß-NTI was calculated using a null model-based statistical framework as described by Stegen et al. (2015). When the absolute values of ß-NTI are more than 2, it indicates that the communities are assembled by nonrandom mechanisms such as environmental filtering, biotic interactions, and active dispersal. When the values are less than 2, community assembly is thought to be driven by stochastic processes such as homogenizing dispersal, drift, and dispersal limitation (Vestrum et al., 2020).
The water chemistry profile (temperature, dissolved oxygen, pH, salinity) throughout the experimental period is shown in Supplementary Table 1. The temperature in the tanks was regulated by an automatic heating and cooling system. There was negligible deviation of the temperature (0.1-0.2°C) from the adjusted values in all tanks, and the temperature was readjusted to the desired value whenever deviation was observed. Therefore, the temperature was constant at the adjusted value set for each tank throughout the experiment. As dissolved oxygen (DO) is a vital factor for the survival of fish, continuous aeration was provided in each tank to ensure the optimum dissolved oxygen content. DO ranged from 6-7 ppm in all tanks throughout the experimental period, indicating no hypoxia in any tank. The pH values in the control tank ranged from 6.7-7.3, whereas they ranged from 6.7-7.5 and 6.7-7.2 in the low-temperature tank and high-temperature tank, respectively. No natural mortality was recorded during the study.
A total of 2,876,384 reads, assigned to 1710 ASVs (Amplicon sequence variants), were obtained from the 27 fecal samples. After filtering out mitochondrial or chloroplast DNA sequences, 2,727,335 reads remained, which were assigned to 1557 ASVs. Figure 2A displays the relative abundance of the most dominant bacterial classes between each temperature and sampling time. The chum salmon gut microbiota consisted of a complex community, in which Gammaproteobacteria (80%) belonging the phylum Proteobacteria was the most dominant class, followed by Flavobacteriia (19%) of Bacteroidetes at the control temperature on Day 0. At the genus level, the most frequently detected sequences were classified as belonging to Aliivibrio (75%), followed by Flavobacterium (19%) [Supplementary Figure 1 (A); Supplementary Table 2]. However, a clear temporal shift in the gut microbial profile was evident at the control temperature after Days 7 and 14, when Flavobacterium became the most dominant genus (36% and 35%, respectively). Under low temperature (8°C), Flavobacterium maintained a high relative abundance in two consecutive weeks. A notable shift at low temperature after Day 14 was associated with the high relative abundance of Tenacibaculum (13%). On the other hand, the 18°C treatment resulted in apparently greater dispersion among the ASVs than the 13°C and 8°C treatments. On Days 0, 7, and 14, Gammaproteobacteria (72%, 68%, and 53%, respectively) was the most abundant class, followed by Flavobacteriia (19%, 16%, and 10%, respectively). When the ASVs were considered at the genus level, the most abundant sequences came mainly from Vibrio and Aliivibrio. However, the Vibrio population maintained a high relative abundance as time progressed, and exposure to high temperature resulted in a greater dispersion of other bacteria, such as Agarivorans, Colwellia, Pseudoalteromonas, Lewinella, and Arcobacter. [Supplementary Figure 1A; Supplementary Table 2]. In addition, we extracted the sequences assigned to possible virulent genera, such as Tenacibaculum and Vibrio, and manually identified them by using BLAST in the NCBI database to further sort ASVs closely related to virulent strains. The data showed a high relative abundance of ASVs closely related to virulent species of Tenacibaculum and Vibrio at low and high temperatures, respectively (Figure 3). Furthermore, the mean relative abundance of virulence-related genes determined using the PICRUSt2 tool and KEGG ortholog (KO) data revealed that temperature presented a significant correlation (p<0.001) with the increase in virulence-related gene abundance (Supplementary Figure 2). The Shannon and Chao1 indices were higher under high temperature than in the control at all time points. Under low temperature, these indices were higher than those of the control on Day 0 and Day 14 but lower on Day 7 [Figure 4A]. The analysis of fecal microbial community dissimilarity via nonmetric multidimensional scaling (NMDS) ordination analysis with Bray–Curtis distance showed the clustering of bacterial communities according to the temperature and time of exposure [Figure 5A]. PERMANOVA revealed that the differences in the fecal bacterial communities among the 3 different temperature treatments were significant (p<0.0001).
Figure 2 Microbial community composition of chum salmon (A) fecal, (B) skin mucus, and (C) surrounding water samples from different temperatures and time points, shown at the phylum level. Results for two phyla, Proteobacteria and Bacteroidetes, are also shown at the class level. Each letter in brackets indicates membership in Proteobacteria (P) or Bacteroidetes (B). Relative abundance is shown as a mean value calculated from three replicates of each sample. Phyla that contributed less than 2% were combined and referred to as “Others”.
Figure 3 Mean relative abundance of possibly virulent Tenacibaculum and Vibrio species in the fecal microbiota of chum salmon under different temperature treatments on Day 0, Day 7, and Day 14. The bar chart suggests the relative abundance of ASVs matching potentially virulent species among different temperature treatments and time points.
Figure 4 Alpha diversity of the microbial communities in the feces (A), skin mucus (B), and surrounding water (C) of chum salmon at each sampling point. Alpha diversity is indicated by the Shannon (left column) and Chao (right column) indices at control (green), low (blue), and high (red) temperatures.
Figure 5 Nonmetric multidimensional scaling (NMDS) ordination plots based on Bray–Curtis distance indicating bacterial community dissimilarity among (A) fecal, (B) skin mucus, and (C) water samples. Ordination plots show the clustering of bacterial communities according to the temperature treatments and time points. Each sample plot is color-coded according to the time point (green, Day 0; blue, Day 7; and red, Day 14), and the shapes represent the temperature treatments (circle= control, triangle= low, and square= high temperature).
After filtering out mitochondrial or chloroplast DNA sequences, 414,448 16S rRNA gene sequences from 27 skin mucus samples were classified into 455 ASVs. As shown in Figure 2B, the skin-mucosal microbiome of chum salmon consisted mainly of the class Flavobacteriia (83%) of the phylum Bacteroidetes, after which Gammaproteobacteria (7%) and Betaproteobacteria (4%) of Proteobacteria were the most abundant groups. The genus Flavobacterium (81%) was the most abundant genus at the control temperature on Day 0. The class Flavobacteriia and the genus Flavobacterium exhibited the highest relative abundance in most of the samples regardless of the temperature modulation and duration of exposure [Supplementary Figure 1B; Supplementary Table 2]. The most evident taxonomic shift was an increase in the relative abundance of the class Betaproteobacteria under prolonged exposure to high temperature. The skin mucosal microbial community exhibited intraspecies differences even within the same treatment and sampling time point. The Shannon and Chao1 indices varied among the different temperatures and sampling days [Figure 4B]. The highest values were recorded under low-temperature conditions, and the lowest values were recorded under high-temperature conditions. PERMANOVA revealed that the differences in the skin mucosal bacterial communities among the 3 temperature treatments were significant (p<0.0009). The analysis of skin mucus microbial community dissimilarity via (NMDS) ordination analysis with Bray–Curtis distance showed that clustering of bacterial communities was different according to the temperature and time of exposure [Figure 5B].
A total of 4,006,531 reads, assigned to 2724 ASVs, were obtained from the 27 water samples. After removing mitochondrial or chloroplast sequences, 4,004,124 reads remained, which were assigned to 2665 ASVs. As shown in Figure 2C, the class Flavobacteriia (80%) of the phylum Bacteroidetes and the genus Flavobacterium (77%) were the most abundant groups, similar to the skin-mucosal microbial profile of chum salmon. The prolonged exposure to high temperature displayed a dispersion of taxonomic groups. The Shannon and Chao1 indices [Figure 4C] indicated a significant increase in diversity on Days 7 and 14 under high temperature (p<0.0001). In addition, the NMDS plot suggested a distinct clustering pattern of bacteria according to the temperature and time of exposure [Figure 5C]. PERMANOVA revealed that the effect of temperature on the bacterial communities of the rearing water was significant (p<0.0001).
The analysis of fecal, skin mucus, and water microbial community dissimilarity via nonmetric multidimensional scaling (NMDS) ordination analysis with Bray–Curtis distance showed the clustering of bacterial communities according to the temperature and time of exposure (Figure 6). PERMANOVA revealed that the feces, skin mucus and water-derived microbiota were significantly different (p<0.0001) from each other.
Figure 6 Nonmetric multidimensional scaling (NMDS) ordination plots based on Bray–Curtis distance showing bacterial community dissimilarity among fecal, skin mucus, and water samples. Ordination plots show the clustering of bacterial communities according to the sample type and temperature treatment. Each sample is color-coded according to the sample type (green= fecal, red= skin mucus, and blue= water) and plotted according to the temperature treatments (circle= control, triangle= low, and square= high temperature).
In the fecal microbiota, the absolute values of ß-NTI were >2 at low and high temperatures, suggesting closer phylogenetic clustering than expected under stochastic community assembly (Figure 7). On the other hand, the absolute β-NTI values of the skin microbiota were <2, indicating that stochastic processes, such as drift or dispersal limitation, contributed to the community assembly.
Figure 7 ß-NTI values for the comparison of bacterial community assembly processes in the chum salmon gut and skin microbiota under each temperature treatment at Day 0, Day 7 and Day 14. ß-NTI>2 and ß-NTI<-2 indicate heterogeneous and homogeneous selection, respectively. ß-NTI<2 indicates comparisons that are not significantly different from the null model and suggests stochastic community assembly.
The composition of the gut microbiota is likely to change in response to extrinsic factors, such as environmental temperature, in poikilothermic organisms, including fish. The present study investigated the response of the chum salmon gut and skin microbiota when subjected to water temperature stress. The P values obtained from PERMANOVA revealed that temperature had a clearly significant effect on the taxonomic structure of the chum salmon gut (p<0.0001), epithelial mucus (p<0.0009), and rearing water (p<0.0001) microbiota. According to a study on brook charr (Salvelinus fontinalis) (Boutin et al., 2013), the fate of the microbiota following stress exposure is to undergo perturbation of the taxonomic composition of the bacterial community. This perturbation may represent a precursor of dysbiosis, subsequently leading to imbalances in the fish microbiome structure and diversity (Sylvain et al., 2016). This phenomenon is consistent with the results of the present study, as a significant perturbation of the taxonomic structure of the chum salmon bacterial communities was evident in response to temperature changes. Since we tested the effect of temperature using a single tank for each treatment, it should be cautious that such significant differences among treatments could be caused by the tank effect which was different among rearing tanks. Tank-specific variability in fish microbiome structure could not be negligible in some experimental conditions to test the effect of intrinsic and extrinsic factors influencing fish microbiota (Giatsis et al., 2014, Schmidt et al., 2016, Steiner et al., 2021). Biofilters of a recirculating aquaculture system reportedly showed a strong tank effect and influenced water microbiota (Schmidt et al., 2016). We cannot rule out the possibility that the shift of community structure in surrounding water observed in this study were not only caused by the effect of temperature but also a tank or a recirculating system. However, a previous study on the microbiome of Chinook salmon suggested that the effect of temperature was obvious in changing water microbiome structure despite the presence of its tank-specific variability (Zhao et al., 2021). The change of microbiota observed in this study was so drastic as to be reasonably assumed that their shift was caused by the effect of temperature. Even if water microbiota could be affected by the tank effect, microbiome structures of the gut and the epithelial tissue were often distinct from the structure of surrounding water microbiome, which was also the case in this study (Giatsis et al., 2014, Schmidt et al., 2016, Zhao et al., 2021, Steiner et al., 2021). Although some of ASVs of skin and gut microbiota were overlapped with those of water microbiota (Supplementary Fig.3), their structures were distinct from that of water microbiota (Figure 6). These previous and present results suggested that the tank effect on the gut and skin microbiota should be marginal and hardly change the interpretation of our results and suggestions discussed here.
Taxonomic profile data revealed that the chum salmon gut microbiota at the control temperature consisted mainly of members of Proteobacteria and Bacteroidetes, among which the classes Gammaproteobacteria and Flavobacteriia were significantly dominant [Figure 2A]. The findings of the present study support previous studies on Salmo salar in which the phylum-level assignment of OTUs indicated the dominance of Proteobacteria and Bacteroidetes (Llewellyn et al., 2016). As reported by (Rombout et al., 2011), Proteobacteria is a distinctly dominant microbial phylum in the gastrointestinal tract of fish. Proteobacteria, Bacteroidetes and Firmicutes have been shown to account for more than 80% of the fish GIT microbiota in numerous fish species investigated heretofore (Ghanbari et al., 2015); hence, our data imply that the core GIT microbial communities (especially at the phylum level) might be recurrent over a wide range of fish species and play significant roles in different physiological functions of the host (Liu et al., 2016).
Taxonomic analysis results revealed the dominance of Gammaproteobacteria in fecal samples at the beginning of the experiment and under exposure to high temperature [Figure 2A]. A high relative proportion of Gammaproteobacteria in the gastrointestinal tract of fish has been documented in previous reports (Liu et al., 2016, Li et al., 2015; Sullam et al., 2012; Roeselers et al., 2011). These authors reported that Proteobacteria (especially the class Gammaproteobacteria) was the predominant class in many marine as well as freshwater fish species. Conditions within the fish intestine are appropriate for facultative anaerobes (Giatsis et al., 2016), which is ideal for many bacteria belonging to the class Gammaproteobacteria (Llewellyn et al., 2016). Therefore, our data suggest that the high relative abundance of Gammaproteobacteria in fecal samples may be associated with the facultative anaerobic state of the fish intestine (Giatsis et al., 2016) and, thus, with high proportions of facultative anaerobic bacteria such as Vibrio, Aliivibrio, Agarivorans, and Colwellia species [Supplementary Fig. 1 (A), Supplementary Table 2].
Our data suggested that the relative abundance of Vibrio in fecal samples was strongly influenced by water temperature. At low temperature, the relative abundance of this group dramatically declined compared to its abundance at the control temperature. On the other hand, a relatively high proportion of Vibrio was maintained at high temperature. Our results support the theory that the growth of Vibrio is accelerated by warmer temperatures (Neuman et al., 2016). In Salmo salar L. (Neuman et al., 2016), it has been reported that water temperatures favor the growth of potentially pathogenic marine Vibrio species during warm seasons. In the present study, exposure to high temperature resulted in a high relative abundance of potentially virulent Vibrio bacteria, especially Vibrio anguillarum, in the fecal microbiota (Figure 3). Vibrio anguillarum is considered the etiological agent of fatal hemorrhagic septicemia (vibriosis) and possess a major threat to aquaculture and larviculture (Castillo et al., 2017, Frans et al., 2011, Austin et al., 2007). Larval and juvenile individuals are more prone to pathogenic Vibrio infection than other life stages, which ultimately leads to a considerable amount of unanticipated and instantaneous mortality (Egerton et al., 2018). Although the obtained 16S rRNA gene sequences were only partial, they still provide evidence suggesting that the high relative abundance of potentially pathogenic Vibrio may impede chum salmon immunity by favoring the growth of opportunistic pathogens, which may in turn result in the development of disease. Metagenomics studies with bacterial transcriptome and virulence gene profiles should therefore be studied in the future.
Our data also suggested a high relative abundance of the class Flavobacteriia and the genus Flavobacterium in fecal samples under low-temperature treatment. Their dominance in fecal and gut samples at low temperatures has also been documented in previous reports (Zhou et al., 2016, Loch and Faisal, 2015), although some species are able to grow at high temperatures (Bernardet and Bowman, 2006). Flavobacterium, a diverse genus in the Bacteroidetes phylum, is of particular significance in aquaculture, especially because of its pathogenic effects on fish. Flavobacteria infections were first reported in 1922 and have since been considered a potential threat to both wild and aquaculture fish. Flavobacteria are psychrophilic and the notable Flavobacteria pathogens of fish include Flavobacterium psychrophilum, F. columnare, F. branchiophilum, and F. succinicans (Loch and Faisal, 2015). Flavobacteria diseases, such as cold-water disease and bacterial gill diseases cause large mortalities in aquaculture practices, especially in hatcheries (Guérin et al., 2021). Therefore, our results imply that temperature change may cause a risk to chum salmon immunity by favoring the growth of potentially pathogenic Flavobacterium. Moreover, Flavobacterium is a well-known important stress-specific microbial biomarker in fish (Sylvain et al., 2016). Biomarkers (materials produced by living organisms or the living organisms themselves) are efficient and accurate tools for evaluating the biotic condition of an ecosystem (Sorci et al., 1999, Sylvain et al., 2016). As the generation time of bacteria is very short and they are extremely susceptible to alterations in their surrounding environment, bacterial biomarker analysis is a rapid and specific method for detecting the effects of environmental disturbances on a holobiont (Sylvain et al., 2016). Our data strongly imply that Flavobacterium itself presents immense potential for use as a stress-specific microbial taxonomic biomarker. Thus, the available results highlight the importance of a stable environment for microbial and fish homeostasis.
Data from the present study revealed a high relative abundance of possibly virulent Tenacibaculum species in the fecal microbiota in the low-water-temperature treatment (Figure 3). Tenacibaculum is another important genus belonging to the class Flavobacteriia whose members are reported to be important pathogens of marine fish, especially the species Tenacibaculum maritimum (Pérez-Pascual et al., 2017, Avendaño-Herrera et al., 2006), Tenacibaculum dicentrachi (Bridel et al., 2018) and Tenacibaculum soleae (Piñeiro-Vidal et al., 2008). It is the etiological bacterium of the ulcerative disease referred to as tenacibaculosis and has caused severe outbreaks and fish mortality on Norwegian, Spanish, and Chilean marine farms. Although the obtained 16S rRNA gene sequences were only partial, they nevertheless indicated that a high relative abundance of these species may be deleterious to chum salmon immunity.
In this study, the skin microbiota of chum salmon was mainly composed of members of the phyla Bacteroidetes and Protobacteria, especially from the genus Flavobacterium of the class Flavobacteriia and the genus Delftia of the class Betaproteobacteria [Figure 2B, Supplementary Fig. 1 (B), Supplementary Table 2]. The dominance of the phyla Bacteroidetes and Protobacteria in the skin mucus microbiota is consistent with previous reports on a wide variety of teleost fish (Chiarello et al., 2015, Wilson et al., 2008). Flavobacterium was the key group in the chum salmon skin mucus microbiota. In general, these species are widely distributed in marine environments and often accumulate on detrital organic particles such as marine snows and fecal pellets in the ocean (Fontanez et al., 2015). Our data supported the idea that fish skin is another preferred habitat of these bacteria in the ocean. Furthermore, the relative abundance of Delftia in the cutaneous mucus samples increased with increasing exposure to low and high temperatures [Supplementary Fig. 1B], which might not represent a direct temperature response of these bacteria. Interestingly, like Flavobacterium, the class Betaproteobacteria is also reported as an effective stress-specific taxonomic biomarker in fish (Sylvain et al., 2016). The high relative abundance of Betaproteobacteria may thus indicate a stressed condition of fish and demonstrates the importance of a stable environment for maintaining the balance of cutaneous skin microbiota. Temperature change could affect the chum salmon microbiota in two possible ways: by directly affecting the resident microbiota of the host or indirectly modulating the physiological responses of the fish based on thermal variation. To date, the physiological responses of the fish cutaneous mucus microbiota to temperature stress have been poorly investigated. It is a well-established fact that exposure to stress events increases mucus production and ultimately results in the alteration of the immunological conditions of fish mucus (Minniti et al., 2017, Tort et al., 2003). This phenomenon also highlights the protective role of mucus. This change in mucus conditions due to the physiological responses of the fish may have caused an increase in the relative abundance of Delftia, which then overwhelmed Flavobacterium after long exposure to suboptimal temperatures.
An important aspect of the present study was determining the affinity and diversity of the bacterial consortia observed in chum salmon skin mucus and the rearing water. The dominant phylotype analysis revealed that some genera were common in both fish skin mucus and the surrounding water, such as Flavobacterium, Dokdonella, Rubritalea, Polaribacter, Roseivirga, Ralstonia, and Delftia species [Supplementary Fig. 1 (C)]. Thus, our findings are in accord with the assumption that the strains from the rearing water play a significant role in shaping the cutaneous skin microbiome of the fish (Green et al., 2013). It should be noted that it is impractical to avoid rearing water when sampling mucosal swabs from fish. However, regardless of the homogenous distribution of some bacterial classes and genera identified in both microbial niches, the analysis of beta-diversity (NMDS plot, Bray–Curtis distance, Figure 6) revealed a clear clustering pattern between the skin mucus and rearing water-derived microbiomes, indicating that each of the microbial consortia shows unique adaptation toward its native surroundings. Moreover, PERMANOVA revealed that the feces, skin mucus and water-derived microbiota were significantly different (p<0.0001) from each other. A previous report in which the relationship between the bacterial communities of skin mucus and rearing water was assessed via 16S ribosomal RNA sequencing in Atlantic salmon (Salmo salar) indicated a similar outcome (Minniti et al., 2017). These authors reported that although the skin mucosal and water-derived microbial communities mostly contained identical genera, unweighted UniFrac analysis suggested a distinct difference between the microbiomes of the two environments. Therefore, our data imply that even though the skin mucosal microbiome is intimately correlated with the surrounding water microbiota, they are each adapted to their specific environment.
A key aspect of microbial community ecology is the elucidation of the forces that govern the community composition. In the present study, our data suggested that the gut microbial profile was influenced by the community in the surrounding water (Supplementary Fig. 3). According to traditional metacommunity theory, bacteria disperse from water to fish and from fish to the surrounding water via excretion (Miller et al., 2018). Thus, water facilitates microbial dispersal from one fish to another inhabiting the same tank. However, we also demonstrated that the bacterial profile of the fish gut was significantly distinct (PERMANOVA, p<0.0001) from the microbial community of the surrounding water, which indicated the significance of selection in host fish microbial community assembly (Yan et al., 2012, Bakke et al., 2015). This phenomenon was validated by the observed phylogenetic clustering of the ß-NTI values of the fecal samples, which indicated environmental selection by temperature. In the skin-associated bacterial communities, we observed interindividual variation [Figure 2B]. ß-NTI values suggested that the skin microbial community was assembled via stochastic processes, such as drift or dispersal limitation, which might be associated with the selection pressure on the skin mucus microbiota. Epithelial mucus contains an array of antimicrobial compounds, and the potential members of the microbiota are therefore limited. Studies on microbial community assembly in fish carried out previously (Yan et al., 2012, Burns et al., 2016, Heys et al., 2020, Vestrum et al., 2020) have been based on the microbial composition assessed according to either an ecological model (i.e., a neutral model) or a ß-NTI null model. As described by Burns et al. (2016) in zebrafish (Danio rerio), stochastic processes dominate the assembly process and generate considerable interindividual differences. Another study on Atlantic salmon (Salmo salar) revealed that neutral processes dominate the microbial community assembly process (Heys et al., 2020). In addition, deterministic selection imposed by certain environmental factors is sometimes important, but in most cases, stochastic or ecologically neutral processes dominate (Stegen et al., 2012). In this study, we revealed how environmental selection and neutral processes contribute to chum salmon microbiota community assembly. Our findings suggested that the gut microbiota of chum salmon was assembled by deterministic processes, in contrast to their skin microbiota, and these processes might be amplified by strong environmental perturbations such as temperature modulation.
Overall, this study suggested that the exposure of chum salmon to temperature fluctuations triggered dysbiosis in the chum salmon microbiota. We observed an overgrowth of potentially opportunistic pathogens, including Vibrio and Tenacibaculum bacteria, in the temperature treatment groups, which may potentially increase disease risk in chum salmon. The results also revealed two key temperature-specific taxonomic microbial biomarkers: the class Betaproteobacteria, and the genus Flavobacterium. Furthermore, our data suggested that environmental selection exerted a significant effect on gut microbial community assembly, whereas the assembly process was rather stochastic in the skin microbiota. The study implies that temperature is a key factor in maintaining a healthy microbiota balance in fish. Further studies should be focused on revealing how the perturbation of the microbiota affects the homeostasis of host physiological functions.
The 16S rRNA gene sequencing datasets presented in this study can be found in the DDBJ online repositories under the accession number DRA014457.
The animal study was reviewed and approved by SH, Atmosphere and Ocean Research Institute, The University of Tokyo, Japan. E-mail:aHlvZG9AYW9yaS51LXRva3lvLmFjLmpw.
SKG designed the study, conducted the experiment, analyzed data, and wrote the draft. MW and SH designed the experiment, provided technical support, commented on, and revised the manuscript, SG analyzed data, commented on, and revised the manuscript, KH designed, supervised the study, managed the fund, commented on, and revised the manuscript. All authors contributed to the article and approved the submitted version.
The study was supported by The Ocean Research Promotion Technology Development Program from the Ministry of Education, Culture, Sports, Science, and Technology (MEXT), and the Future Society Initiative Fund from the University of Tokyo (Ocean DNA), Japan.
The authors sincerely extend their gratitude to Yoko Makabe-Kobayashi, for her kind technical support during the experiment and sequencing.
The authors declare that the research was conducted in the absence of any commercial or financial relationships that could be construed as a potential conflict of interest.
All claims expressed in this article are solely those of the authors and do not necessarily represent those of their affiliated organizations, or those of the publisher, the editors and the reviewers. Any product that may be evaluated in this article, or claim that may be made by its manufacturer, is not guaranteed or endorsed by the publisher.
The Supplementary Material for this article can be found online at: https://www.frontiersin.org/articles/10.3389/fmars.2022.1027621/full#supplementary-material
Abe T. K., Kitagawa T., Makiguchi Y., Sato K. (2019). Chum salmon migrating upriver adjust to environmental temperatures through metabolic compensation. J. Exp. Biol. 222 (Pt 3). doi: 10.1242/jeb.186189
Austin B., Austin D. A., Munn C. B. (2007). Bacterial fish pathogens: Disease of farmed and wild fish. Vol. 26, p. 552. Chichester: Springer.
Avendaño-Herrera R., Toranzo A. E., Magariños B. (2006). Tenacibaculosis infection in marine fish caused by Tenacibaculum maritimum: a review. Dis. Aquat Organ. 71 (3), 255–266. doi: 10.3354/dao071255
Bakke I., Coward E., Andersen T., Vadstein O. (2015). Selection in the host structures the microbiota associated with developing cod larvae (Gadus morhua). Env. Microbiol. 17 (10), 3914–3924. doi: 10.1111/1462-2920.12888
Bates J. M., Mittge E., Kuhlman. J., Baden K. N., Cheesman S. E., Guillemin K. (2006). Distinct signals from the microbiota promote different aspects of zebrafish gut differentiation. Dev. Biol. 297 (2), 374–386. doi: 10.1016/j.ydbio.2006.05.006
Bello A. A. D., Hashim N. B., Haniffah M. R. M. (2017). Predicting impact of climate change on water temperature and dissolved oxygen in tropical rivers. Climate. 5 (3), 58. doi: 10.3390/cli5030058
Benhamed S., Guardiola F. A., Mars M., Esteban M.Á. (2014). Pathogen bacteria adhesion to skin mucus of fishes. Vet. Microbiol. 171 (1-2), 1–12. doi: 10.1016/j.vetmic.2014.03.008
Bernardet J. F., Bowman J. P. (2006). The genus flavobacterium. prokaryotes. 7, 481–531. doi: 10.1007/0-387-30747-8_17
Bolyen E., Rideout J. R., Dillon M. R., Bokulich N. A., Abnet C. C., Al-Ghalith G. A., et al. (2019). Reproducible, interactive, scalable and extensible microbiome data science using QIIME 2. Nat. Biotechnol. 37 (8), 852–857. doi: 10.1038/s41587-019-0209-9
Bonte M., Zwolsman J. J. (2010). Climate change induced salinisation of artificial lakes in the Netherlands and consequences for drinking water production. Water Res. 44 (15), 4411–4424. doi: 10.1016/j.watres.2010.06.004
Boutin S., Audet C., Derome N. (2013). Probiotic treatment by indigenous bacteria decreases mortality without disturbing the natural microbiota of Salvelinus fontinalis. Can. J. Microbiol. 59 (10), 662–670. doi: 10.1139/cjm-2013-0443
Boutin S., Bernatchez L., Audet C., Derôme N. (2013). Network analysis highlights complex interactions between pathogen, host, and commensal microbiota. PloS One 8 (12), e84772. doi: 10.1371/journal.pone.0084772
Boutin S., Sauvage C., Bernatchez L., Audet C., Derome N. (2014). Inter individual variations of the fish skin microbiota: Host genetics basis of mutualism? PloS One 9 (7), e102649. doi: 10.1371/journal.pone.0102649
Bridel S., Olsen A. B., Nilsen H., Bernardet J. F., Achaz G., Avendaño-Herrera R., et al. (2018). Comparative genomics of Tenacibaculum dicentrarchi and "Tenacibaculum finnmarkense" highlights intricate evolution of fish-pathogenic species. Genome Biol. Evol. 10 (2), 452–457. doi: 10.1093/gbe/evy020
Burns A. R., Stephens W. Z., Stagaman K. (2016). Contribution of neutral processes to the assembly of gut microbial communities in the zebrafish over host development. Isme J. 10, 655. doi: 10.1038/ismej.2015.142
Butt R. L., Volkoff H. (2019). Gut microbiota and energy homeostasis in fish. Front. Endocrinol. (Lausanne). 10. doi: 10.3389/fendo.2019.00009
Callahan B. J., McMurdie P. J., Rosen M. J., Han A. W., Johnson A. J., Holmes S. P. (2016). DADA2: High-resolution sample inference from illumina amplicon data. Nat. Methods 13 (7), 581–583. doi: 10.1038/nmeth.3869
Carlson J. M., Hyde E. R., Petrosino J. F., Manage A. B. W., Primm T. P. (2015). The host effects of gambusia affinis with an antibiotic-disrupted microbiome. Comp. Biochem. Physiol. C Toxicol. Pharmacol. 178, 163–168. doi: 10.1016/j.cbpc.2015.10.004
Castillo D., Alvise P. D., Xu R., Zhang F., Middelboe M., Gram L. (2017). Comparative genome analyses of Vibrio anguillarum strains reveal a link with pathogenicity traits. mSystems. 2 (1), e00001–e00017. doi: 10.1128/mSystems.00001-17
Chevalier C., Stojanović O., Colin D. J., Suarez-Zamorano N., Tarallo V., Veyrat-Durebex C., et al. (2015). Gut microbiota orchestrates energy homeostasis during cold. Cell. 163 (6), 1360–1374. doi: 10.1016/j.cell.2015.11.004
Chiarello M., Villéger S., Bouvier C., Bettarel Y., Bouvier T. (2015). High diversity of skin-associated bacterial communities of marine fishes is promoted by their high variability among body parts, individuals, and species. FEMS Microbiol. Ecol. J. 91 (7), fiv061. doi: 10.1093/femsec/fiv061
Diaz Heijtz R., Wang S., Anuar F., Qian Y., Björkholm B., Samuelsson A., et al. (2011). Normal gut microbiota modulates brain development and behavior. Proc. Natl. Acad. Sci. U S A. 108 (7), 3047–3052. doi: 10.1073/pnas.1010529108
Djurichkovic L. D., Donelson J. M., Fowler A. M., Feary D. A., Booth D. J. (2019). The effects of water temperature on the juvenile performance of two tropical damselfishes expatriating to temperate reefs. Sci. Rep. 9 (1), 13937. doi: 10.1038/s41598-019-50303-z
Egerton S., Culloty S., Whooley J., Stanton C., Ross R. P. (2018). The gut microbiota of marine fish. Front. Microbiol. 9. doi: 10.3389/fmicb.2018.00873
Esteban A. M. (2012). An overview of the immunological defenses in fish skin. ISRN Immunol. 2012, 1–29. doi: 10.5402/2012/853470
Fontanez K. M., Eppley J. M., Samo T. J., Karl D. M., DeLong E. F. (2015). Microbial community structure and function on sinking particles in the north pacific subtropical gyre. Front. Microbiol. 6. doi: 10.3389/fmicb.2015.00469
Frans I., Michiels C. W., Bossier P., Willems K. A., Lievens B., Rediers H. (2011). Vibrio anguillarum as a fish pathogen: Virulence factors, diagnosis, and prevention. J. Fish Dis. 34 (9), 643–661. doi: 10.1111/j.1365-2761.2011.01279.x
Ghanbari M., Kneifel W., Domig K. J. (2015). A new view of the fish gut microbiome: advances from next-generation sequencing. Aquaculture. 448, 464–475. doi: 10.1016/j.aquaculture.2015.06.033
Giatsis C., Sipkema D., Ramiro-Garcia J., Bacanu G. M., Abernathy J., Verreth J., et al. (2016). Probiotic legacy effects on gut microbial assembly in tilapia larvae. Sci. Rep. 6, 33965. doi: 10.1038/srep33965
Giatsis C., Sipkema D., Smidt H., Verreth J., Verdegem M. (2014). The colonization dynamics of the gut microbiota in tilapia larvae. PloS One 9 (7), e103641. doi: 10.1371/journal.pone.0103641
Green T. J., Smullen R., Barnes A. C. (2013). Dietary soybean protein concentrate-induced intestinal disorder in marine farmed Atlantic salmon, Salmo salar is associated with alterations in gut microbiota. Vet. Microbiol. 166 (1-2), 286–292. doi: 10.1016/j.vetmic.2013.05.009
Guérin C., Lee B. H., Fradet B. (2021). Transcriptome architecture and regulation at environmental transitions in flavobacteria: the case of an important fish pathogen. ISME Commun. 1, 33. doi: 10.1038/s43705-021-00029-9
Heys C., Cheaib B., Busetti A., Kazlauskaite R., Maier L., Sloan W. T., et al. (2020). Neutral processes dominate microbial community assembly in Atlantic salmon, Salmo salar. Appl. Environ. Microbiol. 86 (8), e02283–e02219. doi: 10.1128/AEM.02283-19
Hicks M. (2002). “Evaluating standards for protecting aquatic life in washington’s surface water quality standards,” in Draft discussion paper and literature summary, vol. 197 pp. (Olympia, WA: Washington State Department of Ecology).
Hovda M. B., Fontanillas R., McGurk C., Obach A., Rosnes J. T. (2012). Seasonal variations in the intestinal microbiota of farmed Atlantic salmon (Salmo salar l.). Aquaculture Res. 43 (1), 154–159. doi: 10.1111/j.1365-2109.2011.02805.x
Iguchi K. I., Ogawa K., Nagae M., Ito F. (2003). The influence of rearing density on stress response and disease susceptibility of ayu (Plecoglossus altivelis). Aquaculture 220 (1-4), 515–523. doi: 10.1016/S0044-8486(02)00626-9
Ito Y., Momii K. (2015). Impacts of regional warming on long-term hypolimnetic anoxia and dissolved oxygen concentration in a deep lake. Hydrological Processes. 29 (9), 2232–2242. doi: 10.1002/hyp.10362
Jones M. L., Martoni C. J., Ganopolsky J. G., Labbé A., Prakash S. (2014). The human microbiome and bile acid metabolism: Dysbiosis, dysmetabolism, disease and intervention. Expert Opin. Biol. Ther. 4), 467–482. doi: 10.1517/14712598.2014.880420
Koch M., Bowes G., Ross C., Zhang X. H. (2013). Climate change and ocean acidification effects on seagrasses and marine macroalgae. Glob Chang Biol. 19 (1), 103–132. doi: 10.1111/j.1365-2486.2012.02791.x
Li T., Long M., Gatesoupe F. J., Zhang Q., Li A., Gong X. (2015). Comparative analysis of the intestinal bacterial communities in different species of carp by pyrosequencing. Microb. Ecol. 69 (1), 25–36. doi: 10.1007/s00248-014-0480-8
Liu H., Guo X., Gooneratne R., Lai R., Zeng C., Zhan F., et al. (2016). The gut microbiome and degradation enzyme activity of wild freshwater fishes influenced by their trophic levels. Sci. Rep. 6, 24340. doi: 10.1038/srep24340
Liu W. T., Marsh T. L., Cheng H., Forney L. J. (1997). Characterization of microbial diversity by determining terminal restriction fragment length polymorphisms of genes encoding 16S rRNA. Appl. Environ. Microbiol. 63 (11), 4516–4522. doi: 10.1128/AEM.63.11.4516-4522.1997
Li X., Yan. Q., Xie S., Hu W., Yu Y., Hu Z. (2013). Gut microbiota contributes to the growth of fast-growing transgenic common carp (Cyprinus carpio l.). PloS One 8 (5), e64577. doi: 10.1371/journal.pone.0064577
Llewellyn M. S., McGinnity P., Dionne M., Letourneau J., Thonier F., Carvalho G. R., et al. (2016). The biogeography of the Atlantic salmon (Salmo salar) gut microbiome. ISME J. 10 (5), 1280–1284. doi: 10.1038/ismej.2015.189
Loch T. P., Faisal M. (2015). Emerging flavobacterial infections in fish: A review. J. Adv. Res. 6 (3), 283–300. doi: 10.1016/j.jare.2014.10.009
Martin M. (2011). Cutadapt removes adapter sequences from high-throughput sequencing reads. EMBnet. J. 17 (1), 10–12. doi: 10.14806/ej.17.1.200
McDonald D., Price M. N., Goodrich J., Nawrocki E. P., DeSantis T. Z., Probst A., et al. (2012). An improved greengenes taxonomy with explicit ranks for ecological and evolutionary analyses of bacteria and archaea. ISME J. 6 (3), 610–618. doi: 10.1038/ismej.2011.139
Miller E. T., Svanbäck R., Bohannan B. J., et al (2018). Microbiomes as metacommunities: Understanding host-associated microbes through metacommunity ecology. Trends Ecol. Evolution. 33 (12), 926–935. doi: 10.1016/j.tree.2018.09.002
Minniti G., Hagen L. H., Porcellato D., Jørgensen S. M., Pope P. B., Vaaje-Kolstad G. (2017). The skin-mucus microbial community of farmed Atlantic salmon (Salmo salar). Front. Microbiol. 8. doi: 10.3389/fmicb.2017.02043
Mohammed H. H., Arias C. R. (2015). Potassium permanganate elicits a shift of the external fish microbiome and increases host susceptibility to columnaris disease. Vet. Res. 46 (1), 82. doi: 10.1186/s13567-015-0215-y
Neuman C., Hatje E., Zarkasi K. Z., Smullen R., Bowman J. P., Katouli M. (2016). The effect of diet and environmental temperature on the faecal microbiota of farmed Tasmanian Atlantic salmon (Salmo salar l.). Aquaculture Res. 47 (2), 660–672. doi: 10.1111/are.12522
Ojaveer E., Kalejs M. (2005). The impact of climate change on the adaptation of marine fish in the Baltic Sea. ICES J. Mar. Science. 62 (7), 1492–1500. doi: 10.1016/j.icesjms.2005.08.002
Oksanen J., Blanchet F. G., Friendly M., Kindt R., Legendre P., McGlinn D., et al. (2018). Community ecology package (Vienna: R Foundation for Statistical Computing).
Parada A. E., Needham D. M., Fuhrman J. A. (2016). Every base matters: assessing small subunit rRNA primers for marine microbiomes with mock communities, time series and global field samples. Environ. Microbiol. 18 (5), 1403–1414. doi: 10.1111/1462-2920.13023
Pérez-Pascual D., Lunazzi A., Magdelenat G., Rouy Z., Roulet A., Lopez-Roques C., et al. (2017). The complete genome sequence of the fish pathogen Tenacibaculum maritimum provides insights into virulence mechanisms. Front. Microbiol. 8. doi: 10.3389/fmicb.2017.01542
Piñeiro-Vidal M., Carballas C. G., Gómez-Barreiro O., Riaza A., Santos Y. (2008). Tenacibaculum soleae sp Nov., isolated from diseased sole (Solea senegalensis kaup). Int. J. Syst. Evol. Microbiol. 58 (Pt 4), 881–885. doi: 10.1099/ijs.0.65539-0
Pörtner H. O., Berdal B., Blust R., Brix O., Colosimo A., De Wachter B., et al. (2001). Climate induced temperature effects on growth performance, fecundity and recruitment in marine fish: Developing a hypothesis for cause and effect relationships in Atlantic cod (Gadus morhua) and common eelpout (Zoarces viviparus). Continental Shelf Res. 21 (18-19), 1975–1997. doi: 10.1016/S0278-4343(01)00038-3
Rawls J. F., Mahowald M. A., Ley R. E., Gordon J. I. (2006). Reciprocal gut microbiota transplants from zebrafish and mice to germ-free recipients reveal host habitat selection. Cell. 127 (2), 423–433. doi: 10.1016/j.cell.2006.08.043
Rawls J. F., Samuel B. S., Gordon J. I. (2004). Gnotobiotic zebrafish reveal evolutionarily conserved responses to the gut microbiota. Proc. Natl. Acad. Sci. U S A. 101 (13), 4596–4601. doi: 10.1073/pnas.0400706101
Richards R. C., O'Neil D. B., Thibault P., Ewart K. V. (2001). Histone H1: an antimicrobial protein of Atlantic salmon (Salmo salar). Biochem. Biophys. Res. Commun. 284 (3), 549–555. doi: 10.1006/bbrc.2001.5020
Richter A., Kolmes S. A. (2005). Maximum temperature limits for Chinook, coho, and chum salmon, and steelhead trout in the pacific Northwest, reviews in fisheries science 13 1, 23–49. doi: 10.1080/10641260590885861
Roeselers G., Mittge E. K., Stephens W. Z., Parichy D. M., Cavanaugh C. M., Guillemin K., et al. (2011). Evidence for a core gut microbiota in the zebrafish. ISME J. 5 (10), 1595–1608. doi: 10.1038/ismej.2011.38
Roessig J. M., Woodley C. M., Cech J. J., Hansen L. J. (2004). Effects of global climate change on marine and estuarine fishes and fisheries. Rev. fish Biol. fisheries. 14 (2), 251–275. doi: 10.1007/s11160-004-6749-0
Rombout J. H., Abelli L., Picchietti S., Scapigliati G., Kiron V. (2011). Teleost intestinal immunology. Fish Shellfish Immunol. 31 (5), 616–626. doi: 10.1016/j.fsi.2010.09.001
Round J. L., Mazmanian S. K. (2009). The gut microbiota shapes intestinal immune responses during health and disease. Nat. Rev. Immunol. 9 (5), 313–323. doi: 10.1038/nri2515
Salo E. O. (1991). Life history of chum salmon (Oncorhynchus keta). Pacific salmon Life histories, 231–309.
Schmidt V., Amaral-Zettler L., Davidson J., Summerfelt S., Good C. (2016). Influence of fishmeal-free diets on microbial communities in Atlantic salmon (Salmo salar) recirculation aquaculture systems. Appl. Environ. Microbiol. 82 (15), pp.4470–4481. doi: 10.1128/AEM.00902-16
Schram E., Bierman S., Teal L. R., Haenen O., van de Vis H., Rijnsdorp A. D. (2013). Thermal preference of juvenile Dover sole (Solea solea) in relation to thermal acclimation and optimal growth temperature. PloS One 8 (4), e61357. doi: 10.1371/journal.pone.0061357
Sorci J. J., Paulauskis J. D., Ford T. E. (1999). 16S rRNA restriction fragment length polymorphism analysis of bacterial diversity as a biomarker of ecological health in polluted sediments from new Bedford harbor, Massachusetts, USA. Mar. pollut. Bulletin. 38 (8), 663–675. doi: 10.1016/S0025-326X(98)90199-0
Soriano E. L., Ramírez D. T., Araujo D. R., Gómez-Gil B., Castro L. I., Sánchez C. G. (2018). Effect of temperature and dietary lipid proportion on gut microbiota in yellowtail kingfish Seriola lalandi juveniles. Aquaculture. 1 (497), 269–277. doi: 10.1016/j.aquaculture.2018.07.065
Stecher B., Maier L., Hardt W. D. (2013). 'Blooming' in the gut: how dysbiosis might contribute to pathogen evolution. Nat. Rev. Microbiol. 11 (4), 277–284. doi: 10.1038/nrmicro2989
Stegen J. C., Lin X., Fredrickson J. K., Konopka A. E. (2015). Estimating and mapping ecological processes influencing microbial community assembly. Front. Microbiol. 6. doi: 10.3389/fmicb.2015.00370
Stegen J. C., Lin X., Konopka A. E., Fredrickson J. K. (2012). Stochastic and deterministic assembly processes in subsurface microbial communities. The ISME journal 6 (9), 1653–1664. doi: 10.1038/ismej.2012.22
Steiner K., Heasman K., Laroche O., Pochon X., Preece M., Bowman J. P., et al. (2021). The microbiome of Chinook salmon (Oncorhynchus tshawytscha) in a recirculation aquaculture system. Aquaculture 534, p.736227. doi: 10.1016/j.aquaculture.2020.736227
Sugita H., Ito Y. (2006). Identification of intestinal bacteria from Japanese flounder (Paralichthys olivaceus) and their ability to digest chitin. Lett. Appl. Microbiol. 43 (3), 336–342. doi: 10.1111/j.1472-765X.2006.01943.x
Sullam K. E., Essinger S. D., Lozupone C. A., O'Connor M. P., Rosen G. L., Knight R., et al. (2012). Environmental and ecological factors that shape the gut bacterial communities of fish: a meta-analysis. Mol. Ecol. 21 (13), 3363–3378. doi: 10.1111/j.1365-294X.2012.05552.x
Sylvain F.É., Cheaib B., Llewellyn M., Gabriel Correia T., Barros Fagundes D., Luis Val A., et al. (2016). pH drop impacts differentially skin and gut microbiota of the Amazonian fish tambaqui (Colossoma macropomum). Sci. Rep. 6, 32032. doi: 10.1038/srep32032
Tanaka H. I., Takagi Y. A., Naito Y. A. (2000). Behavioral thermoregulation of chum salmon during homing migration in coastal waters. J. Exp. Biol. 203 (12), 1825–1833. doi: 10.1242/jeb.203.12.1825
Team R. C. (2018). R: A language and environment for statistical computing (Vienna, Austria: R Foundation for Statistical Computing).
Thompson F. L., Iida T., Swings J. (2004). Biodiversity of vibrios. Microbiol. Mol. Biol. Rev. 68 (3), 403–431. doi: 10.1128/MMBR.68.3.403-431.2004
Tomasello G., Mazzola M., Leone A., Sinagra E., Zummo G., Farina F., et al. (2016). Nutrition, oxidative stress and intestinal dysbiosis: Influence of diet on gut microbiota in inflammatory bowel diseases. BioMed. Pap Med. Fac Univ Palacky Olomouc Czech Repub. 160 (4), 461–466. doi: 10.5507/bp.2016.052
Tort L., Balasch J. C., Mackenzie S. (2003). Fish immune system A crossroads between innate and adaptive responses. Inmunología. 22 (3), 277–286.
Trivedi B. (2012). Microbiome: The surface brigade. Nature. 492 (7429), S60–S61. doi: 10.1038/492S60a
Vestrum R. I., Attramadal K. J. K., Vadstein O., Gundersen M. S., Bakke I. (2020). Bacterial community assembly in Atlantic cod larvae (Gadus morhua): contributions of ecological processes and metacommunity structure. FEMS Microbiol. Ecol. 96 (9), fiaa163. doi: 10.1093/femsec/fiaa163
Webb C. O. (2000). Exploring the phylogenetic structure of ecological communities: an example for rain forest trees. Am. Naturalist. 156 (2), 145–155. doi: 10.1086/303378
Wendling C. C., Wegner K. M. (2013). Relative contribution of reproductive investment, thermal stress, and vibrio infection to summer mortality phenomena in pacific oysters. Aquaculture. 412, 88–96. doi: 10.1016/j.aquaculture.2013.07.009
Wilson B., Danilowicz B. S., Meijer W. G. (2008). The diversity of bacterial communities associated with Atlantic cod Gadus morhua. Microb. Ecol. 55 (3), 425–434. doi: 10.1007/s00248-007-9288-0
Xiong Y. J., Chen Z. H., Tan S. L. (2013). “Relationship between salinity and sea surface temperature in pearl river estuary, China,” in In2013 IEEE international geoscience and remote sensing symposium-IGARSS (Melbourne, Australia: IEEE), 1575–1578.
Yan Q., van der Gast C. J., Yu Y. (2012). Bacterial community assembly and turnover within the intestines of developing zebrafish. PloS One 7 (1), e30603. doi: 10.1371/journal.pone.0030603
Zeebe R. E., Zachos J. C., Caldeira K., Tyrrell T. (2008). Oceans Carbon emissions and acidification. Science. 321 (5885), 51–52. doi: 10.1126/science.1159124
Zhao R., Symonds J. E., Walker S. P., Steiner K., Carter C. G., Bowman J. P., et al. (2021). Effects of feed ration and temperature on Chinook salmon (Oncorhynchus tshawytscha) microbiota in freshwater recirculating aquaculture systems. Aquaculture 543, p.736965. doi: 10.1016/j.aquaculture.2021.736965
Keywords: temperature, chum salmon, bacteria, skin mucus, gut, community assembly, fish
Citation: Ghosh SK, Wong MK-S, Hyodo S, Goto S and Hamasaki K (2022) Temperature modulation alters the gut and skin microbial profiles of chum salmon (Oncorhynchus keta). Front. Mar. Sci. 9:1027621. doi: 10.3389/fmars.2022.1027621
Received: 25 August 2022; Accepted: 12 September 2022;
Published: 27 September 2022.
Edited by:
Li Lian Wong, Universiti Malaysia Terengganu, MalaysiaReviewed by:
Fangrui Lou, Yantai University, ChinaCopyright © 2022 Ghosh, Wong, Hyodo, Goto and Hamasaki. This is an open-access article distributed under the terms of the Creative Commons Attribution License (CC BY). The use, distribution or reproduction in other forums is permitted, provided the original author(s) and the copyright owner(s) are credited and that the original publication in this journal is cited, in accordance with accepted academic practice. No use, distribution or reproduction is permitted which does not comply with these terms.
*Correspondence: Koji Hamasaki, aGFtYXNha2lAZy5lY2MudS10b2t5by5hYy5qcA==
Disclaimer: All claims expressed in this article are solely those of the authors and do not necessarily represent those of their affiliated organizations, or those of the publisher, the editors and the reviewers. Any product that may be evaluated in this article or claim that may be made by its manufacturer is not guaranteed or endorsed by the publisher.
Research integrity at Frontiers
Learn more about the work of our research integrity team to safeguard the quality of each article we publish.