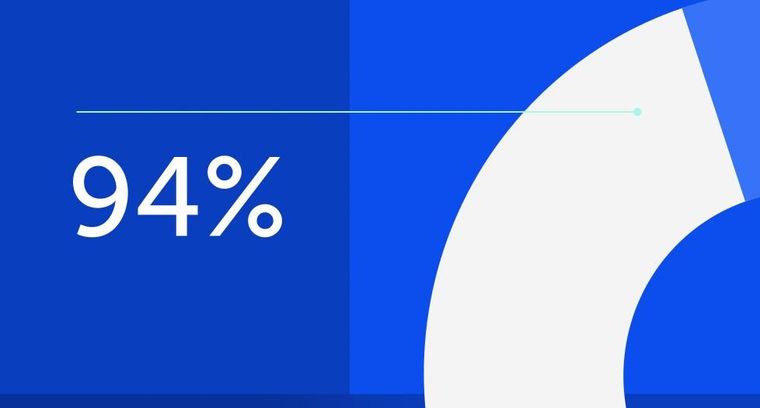
94% of researchers rate our articles as excellent or good
Learn more about the work of our research integrity team to safeguard the quality of each article we publish.
Find out more
PERSPECTIVE article
Front. Mar. Sci., 06 January 2023
Sec. Marine Fisheries, Aquaculture and Living Resources
Volume 9 - 2022 | https://doi.org/10.3389/fmars.2022.1014361
This article is part of the Research TopicProspects and Challenges for the Implementation of HTS Genetic Methods in Fisheries Research Surveys and Stock Assessments.View all 15 articles
The establishment of high-throughput sequencing technologies and subsequent large-scale genomic datasets has flourished across fields of fundamental biological sciences. The introduction of genomic resources in fisheries management has been proposed from multiple angles, ranging from an accurate re-definition of geographical limitations of stocks and connectivity, identification of fine-scale stock structure linked to locally adapted sub-populations, or even the integration with individual-based biophysical models to explore life history strategies. While those clearly enhance our perception of patterns at the light of a spatial scale, temporal depth and consequently forecasting ability might be compromised as an analytical trade-off. Here, we present a framework to reinforce our understanding of stock dynamics by adding also a temporal point of view. We propose to integrate genomic information on temporal projections of species distributions computed by Species Distribution Models (SDMs). SDMs have the potential to project the current and future distribution ranges of a given species from relevant environmental predictors. These projections serve as tools to inform about range expansions and contractions of fish stocks and suggest either suitable locations or local extirpations that may arise in the future. However, SDMs assume that the whole population respond homogenously to the range of environmental conditions. Here, we conceptualize a framework that leverages a conventional Bayesian joint-SDM approach with the incorporation of genomic data. We propose that introducing genomic information at the basis of a joint-SDM will explore the range of suitable habitats where stocks could thrive in the future as a function of their current evolutionary potential.
There is unequivocal evidence that marine biodiversity is declining, with severe impacts on marine ecosystems that reverberate at ecological, social and economic scales (Cardinale et al., 2012). As 40% of the human population lives within 100 km from the coast (Stead, 2018), marine fish constitute one of the most accessible food bases and the main source of protein. Overfishing, habitat destruction and pollution have been pointed as the main responsible causes for marine biodiversity loss both regionally and globally, but the role of climate change in shifting distribution ranges and promoting local extinctions is becoming more and more evident (Brander, 2010; Lam et al., 2020). Climate change is reflected in temperature increments inducing modifications both at biochemical and geological levels, including ocean acidification, hypoxia, sea level rises, and more frequent droughts, storms, or heat waves (Lohbeck et al., 2012; Frölicher and Laufkötter, 2018). Environmental oscillations and anthropogenic pressures such as fisheries have direct effects on the trophodynamic structure and function of marine systems, and consequently a massive impact on worldwide fisheries (Cheung et al., 2009). At the light of such events, fisheries research has become pivotal in understanding stocks response to climatic shifts and attempts to predict their future distribution and abundance. Currently, there are perhaps two major tools at scientists’ disposal to do it so. The first is genomic resources, which became increasingly more reachable for non-model species after the advent of next-generation sequencing a decade ago (Allendorf et al., 2010; Benestan et al., 2016). Genetics has since decades delivered valuable outputs to fisheries management, though restrained to a handful of well-established systems (Bernatchez et al., 2017). Early implementations of genetic information came tackle some of the wider gaps on fisheries management, such as estimates of stock connectivity and delimitation, development of monitoring programs, and design of marine protected areas (Hauser and Carvalho, 2008; Verspoor et al., 2008; Casey et al., 2016; Blasco et al., 2020). Characterizing genetic diversity as respective distribution patterns became more enticing with NGS as it permitted to expand the search beyond the traditional stock structure and connectivity towards signatures of selection and inference of the putative adaptive potential of those same stocks (Therkildsen et al., 2013; Baltazar-Soares et al., 2021b). Inferring adaptive potential on top of stock connectivity is pivotal to estimate the repertoire of genetic-based adaptive responses and build expectations on the spatial reshuffling of adaptive alleles (Eizaguirre and Baltazar-Soares, 2014; Capblancq et al., 2020).
The second one is the development of algorithms to infer species distribution forecasts, which is being performed by using Species Distribution Models (SDM) based on assumptions of niche conservatism (Guisan and Thuiller, 2005). These have been created to provide an understanding of the factors and processes that may cause fluctuations in local populations, and to facilitate climate-ready management of living marine resources under social, economic, and ecological perspectives (Porfirio et al., 2014; Villero et al., 2017). Distribution models can describe essential habitats for early (egg, larval, and pelagic juvenile) and later (juvenile and adult) life history stages of marine fishes (Zurell et al., 2016; Laman et al., 2017). They can also be used to predict potential spawning habitats (Planque et al., 2007), provide a basis to define new fishing areas by evaluating gains and losses in species suitable areas over time, and propose better management options in areas where habitat contractions are predicted in the future (Lima et al., 2022). Different levels of complexity and data integration already exist in SDMs, but efforts are still needed to offer more than correlative outputs (Zurell et al., 2016). Indeed, the integration of complementary methodologies and multidisciplinary approaches, such as genomics and SDM, constitute promising advances on predicting species response to environmental changes by incorporating “genomic vulnerability” or “genomic offset” in the model (Fitzpatrick et al., 2007; Laman et al., 2018; Nielsen et al., 2021; Layton and Bradbury, 2022).
Here we proposed an entirely different approach on how SDMs could utilize genomic information. Briefly, the framework we propose consists of 1) utilizing high-throughput sequencing to detect candidate genomic variants whose frequency is associated with environmental conditions at spawning areas, 2) explore links between genotype and phenotype to infer functionality of the relationship and selective value and 3) utilize allelic frequencies as inputs to joint-SDMs, using allele selective values as prior on allele-environmental conditions relationships, taking into account for allele co-occurrence patterns and phylogeny to, 4) predict allele distribution, and de facto species distribution. Our focus on spawning areas is justified by their relevance to define the viability of fish populations as enhancers of reproductive success. It has been demonstrated that broadcast spawning (a reproduction strategy common in the marine environment) have evolved to optimize spawning timing and location to target optimal environmental conditions (Thorrold et al., 2001; Planque et al., 2007; Baltazar-Soares et al., 2018) . For the large majority of marine species, early life stages prior to first feeding check also occurs in the vicinity of spawning areas and thus we hold this framework in the premise that selective pressures at spawning are extremely high and thus require the evolution of adaptive responses. We will first cover how genetics is a tool utilized to understand evolutionary responses within natural populations and revise how high-throughput sequencing revolutionized the statistical power of genomic signatures. We will then approach concepts and methodologies behind SDMs and lastly, we will briefly illustrate ongoing efforts to conjugate both research areas and present our own suggestion to do it so.
Screening genomes has become a routine task in evolutionary genetics in the recent decade, but that has not always been the case. The early 2000s experienced the first steps towards characterizing genome-wide diversity in no-model species with the discovery of short-tandem repeats (STRs) (Ellegren, 2004; Vieira et al., 2016). STRs loci are usually captured across random locations in the genome, each optimally exhibiting high levels of sequence length polymorphism (Jarne and Lagoda, 1996). Therefore, inferences from polymorphic-STRs analyses are based on multiple independent observations of the target genome´s evolution. In general terms, HTS relates to massive parallel production of DNA sequences. Its implementation expanded our capacity to collect multiple molecular markers across the genome (Mardis, 2008a). Nowadays, HTS techniques have evolved to sequence full genomes both in the form of short-reads DNA se, i.e., 250 base-pair sized reads commonly produced with Illumina, or long-reads DNA strings, where average read-lengths are as high as dozens of kilo base-pairs such as those commonly produced with PacBio or Oxford Nanopore technology (Mardis, 2008b; Hu et al., 2021). While the abovementioned strategies aim to sequence the whole of the nuclear DNA molecule, HTS have further expanded to sequence the full spectrum of DNA replication via the characterizing of transcriptomes and proteomes. Sequencing these DNA provides strong evidence of functionality, reinforcing the putative links between genotypic and phenotypic variation that can be observed at individual, population, or species level (Oomen and Hutchings, 2022).
The working-horse on any population genetic analysis are allelic frequencies. Thus, the baseline output after data processing (which involves cleaning of raw sequence data, curation, and variant calling) is a panel of genetic markers with allelic or haplotype frequencies distributed either by loci, individuals, or populations (R. Nielsen and Slatkin, 2013). Population genetics theory holds on principles of mendelian inheritance, evolution, and mathematics to devise how allelic frequencies vary across generations (R. Nielsen and Slatkin, 2013). It is against theoretical expectations of allelic frequency distributions that observed patterns of genetic variation are interpreted at population scales. One of the main goals of applying population genetics theory has been linking evolutionary and demographic processes, where the linear relationship between indices of genetic diversity such as heterozygosity or allelic richness and effective population sizes is well established (Reed and Frankham, 2003). Inferring population structure, effective population size and historical demography with genetic variation that has no impact on individual fitness became staple examples of applied population genetics. However, next generation sequencing effortlessly pushed the limits of population genetic inferences and greatly facilitated the search for molecular signatures of selection (Frankham, 2010).
Genome-wide screens usually target > 20 individuals from as many locations of the species’ distribution as possible. The objective at this stage is to obtain representativity of the overall genetic diversity of the natural population. Here, the most common methodology to identify signatures of selection are environmental associations (EA). These frameworks identify signatures of selection by comparing allelic frequencies against gradients of environmental variables. At the light of evolutionary theory, significant correlations indicate selection for the presence of the candidate allele (in a population) in numbers higher than those expected by chance. Thus, candidate loci (under selection) will be those whose allelic frequencies vary consonant to the hypothesized environmental gradient (Forester et al., 2016). For instances, Benestan et al. (2016) candidate loci putatively involved in the response to thermal adaptation of lobsters by reporting an environmental association of allelic frequencies across a latitudinal gradient (Benestan et al., 2016). Currently, identification of loci under selection is an active and fertile research ground. There exist multiple methodologies, frameworks and even ideologies that are beyond the scope of this manuscript to discuss in detail (Günther and Coop, 2013; Whitlock and Lotterhos, 2015). Still, the major caveat of environmental correlations is arguably the absence of causality. The fact that a functional link cannot be established between genetic variation and a successful response to selective pressures renders environmental associations insufficient to argue with confidence about demographic impacts (Lotterhos and Whitlock, 2015).
Validating the adaptive potential estimated from molecular signatures can be achieved experimentally. Manipulating environmental settings to test fitness effects of candidate genomic variants in different environmental conditions should provide conclusive evidence of functionality (Lenz et al., 2013; Kaufmann et al., 2014). The challenge is seldom rearing is possible, which is an essential step to a) observe the functionality and b) validate true positives. If rearing is possible, then the method is partially quite established. It consists in exposing specimens to specific selective pressures measuring reproductive success and subsequent fitness of F1 and/or F2 (to mitigate the noise of natural genetic variation and family effects), and genotype individuals either at the end point of the experiment or through biologically established timestamps (Huang et al., 2016; Heckwolf et al., 2020). Genotyping is commonly performed via collecting and sequencing transcripts of individuals exposed to different conditions, where fold-differences or structural variants such as SNPs, copy number and/or splicing variants are indicators of selection and respective adaptive responses (Lenz et al., 2013; Heckwolf et al., 2020).
Species distribution models are valuable statistical tools providing management and conservation supports (Zurell et al., 2022). In addition to describe and explain relationships between species and environmental characteristics based on the niche-biotope duality, they are extensively used to map species’ present-day distributions and to forecast changes over space and time (Zurell et al., 2020). SDMs traditionally follow a bottom-up approach using geo-referenced species records (presence-only, presence-absence [or presence-pseudo-absence], abundance) to estimate species niche in a correlative framework. This framework is based on the extraction of environmental characteristics from a stack of physical and biogeochemical climate model projections (past, present and (or) future) for each sampling point, including potentially the environmental characteristics where the species does not occur (absence or pseudo-absence) (Hollowed et al., 2013; Lima et al., 2022). The chosen algorithm then creates response functions exhibiting the environmental optima and explaining the relationship of the species’ occurrence and the environment (Hollowed et al., 2013). Such relationship is returned as an index of habitat suitability which can be, under several assumptions (e.g., constant detection probability), considered as occurrence probability (Royle et al., 2012). Final SDM outputs are estimated relationships between species occurrence, environmental variables, and habitat suitability maps. SDMs can eventually be used to evaluate whether the spatial variability of a species’ environmental optima will shift under different climate change scenarios over large scale spatial projections (Raybaud et al., 2017; Jghab et al., 2019; Schickele et al., 2020). From these maps it is also possible to define range expansion and contraction in the distribution of the species over time by calculating suitable areas (km2) over the entire distribution range or in specific habitats (Lima et al., 2022). Recent developments have been done to increase SDM’ ecological reliability and tackle many of its caveats (Zurell et al., 2016; Ovaskainen et al., 2017). For example, introducing Bayesian inference allows to cover the simplified vision of niche conservatism where individuals’ occurrences remain fixed throughout space and time in a correlative approach. Bayesian inference has been used to improve the estimation of species-environmental relationships by integrating a priori knowledge on species niche dimensions. In Bayesian theory, the probability of an event to occur is mediated by information on past occurrences of the event. Technically, it translates into the use of priors with a respective distribution density (Gaussian, Poisson, etc. distribution) representing the event’s past occurrence, to build a range of probable future events, or the so-called posterior density distribution (Bolstad and Curran, 2016). Posterior density distributions are constructed with Markov-Chain-Monte-Carlo (MCMC) samplers which, briefly, are a chained repetition of the model utilizing randomly picked priors from the proposed distribution (Van Ravenzwaaij et al., 2018). Bayesian inference coupled with MCMC simulations allow the efficient computation of thousands or millions of scenarios based on prior-posterior conjugations and are regarded as a major improvement in statistical computing frameworks in biology (Huelsenbeck et al., 2001; Yau and Campbell, 2019). Bayesian inferences have been shown to improve the explicative and predictive powers of SDMs (Vermeiren et al., 2020). Another aspect traditionally limiting the ecological reliability of SDMs it their reliance on abiotic variables, when it is factual that biotic variables, such as species interactions, also play a critical role to shape species niche (Zurell et al., 2016). Joint-SDMs have been built to tackle this issue, by considering multi-species co-occurrences inside the same model, using functional traits or phylogenetic relatedness to investigate the dependance pattern between species (Ovaskainen et al., 2017). Estimating multi-species niche, while combining different sources of data improve prediction accuracy, and that is why exploring possibilities to capitalize the amount of information produced by HTS is flourishing research area (Ovaskainen et al., 2017; Peel et al., 2019; Vermeiren et al., 2020; Andrello et al., 2022).
Adaptations accounting from genomic interaction with environmental conditions are usually ignored but might be important to explain and predict species distribution (DeMarche et al., 2019). SDMs usually assume a homogeneity of the genomic composition inside the distribution of a focal species. Relationships between genomic diversity and environmental factors have been used to map the vulnerability of the species at intra-specific level (Ruegg et al., 2018). This can help understanding where individuals of a same species will be more impacted by climate change or will need faster adaptive capacity/facilitation/resilience. To date, most studies have considered that evaluating different SDMs of genetically defined populations of a given species would be a sufficient proxy to integrate adaptive potential (Ikeda et al., 2017; Chardon et al., 2020). These studies used predictions of species’ niche space by considering that genetically distinct populations would respond differently to present-day climate, and thus future climates, to then test for similarity in the climatic niche of the groups (DeMarche et al., 2019). Alternatively, researchers now have been focusing on evolutionary algorithms to combine multiple information in one single learning cycle (Gobeyn et al., 2019). Those frameworks consider that the geographical range of a species is defined by its ability to track favourable environmental conditions depending on its physiology, evolutionary adaptation and the inter and intraspecific biotic interactions (Thuiller et al., 2013). SDMs also span the divide between correlative and mechanistic models using eco-evolutionary forecasting frameworks (Bush et al., 2016; Cotto et al., 2017). The framework combines niche-based projections and individual-based, genetically and spatially explicit stochastic simulations (Cotto et al., 2017). Modelling frameworks such as AdaptR are useful to predict the distribution of species through time steps known as generations (Bush et al., 2016). This hybrid approach allows the incorporation of adaptive capacity as phenotypic plasticity, evolutionary adaptation and adaptive capacity through physiological limits into the same framework (Bush et al., 2016). 2016). In eco-evolutionary dynamic models, local populations on a grid cell are assumed to adapt to local environmental conditions, whilst accounting for stochastic processes of individual life cycle, such as birth, death and migration (i.e. age-structured demographic model). Here, static niche models are used to predict the current distribution of a species based on environmental conditions. Then, the predicted distribution is used to initialize simulated changes in the distribution accounting to adaptation as driven by scenarios of climatic change (Cotto et al., 2017).
Our proposal places adaptive potential – from genomics – at the basis of an SDM approach (Figure 1). Conceptually, we propose to adjust the Bayesian J-SDM framework presented in the previous section to utilize information at genotype level, or specifically, allelic frequencies. In essence, we are literally transfiguring the concept of “species” into “alleles”. This means that instead of a pool of species (or individuals of a given species), we would have a pool of allelic frequencies from the candidate loci obtained either via environmental associations or transcriptomes of experimentally exposed individuals. The biological input variable would thus be allele presence-absence or frequency between populations of either specimens collected across the species distribution range or experimental groups. Naturally, the concept can be extended to a pool of candidate loci (and respective alleles), as the identification of several or dozens of candidate loci either through environmental associations or experimental work is nevertheless common (Hoban et al., 2016). The main goal is to consider adaptive genomic information as valid predictors of shifts in species distribution, implying that to be a canonical response to change of environmental conditions. Here we can make use of the more informative power of experimental approaches by utilizing reaction norms - or the range of phenotypic expression of a specific genotype - as priors of the Bayesian-JSDM framework we are proposing. Because reactions norms relate to the phenotypic response of the measured trait of which the genotype would be responsible for, these transcriptome-derived priors are key to model posterior distribution of adaptive genetic variation. When candidate loci are identified with environmental associations, then the priors for allele association with environmental variable can be the correlation statistics reported by currently utilized environmental association software. To name some, Bayenv2 (Günther and Coop, 2013) reports Bayes factors and Spearman’s rho statistic for each allele-environment variable association, and the latent factor mixed models (lfmm) incorporated in R package LEA (Frichot and François, 2015) identifies candidate loci considering a z-score distribution of correlation values also for each allele-environment variable association. This candidate-detection software also employs Bayesian statistics and thus correlation statistics outputs of several runs consecutively identifying the same candidate loci can be utilized as prior distribution for allelic response. Certainly, these priors are far less indicative of an allelic response than reaction norms obtained from experimental set-ups. Nevertheless, having a prior on allele-abiotic predictor relationships can reduce the risk for spurious correlation in the SDM framework and become useful in case of sampling bias.
Figure 1 Conceptual eco-evolutionary framework adapted from a Bayesian joint-species distribution model (J-SDM) to estimate species distribution from allele occurrence patterns and fitness. The framework is divided in four steps, (A) fish sampling at spawning locations representative of the species distribution, (B) identification of the candidate genes and if possible, empirical assessment of allele reaction norm for a focal environmental variable, (C) model conception based on allele occurrence and environmental conditions at sampling sites, using optimum and variance of the reaction norm to inform prior distributions and phylogenetic trees to identify similarities between sampled locations genetic composition to characterize allele cooccurrence patterns, and (D) explain allele relationships with environmental variables, predict allele distributions and eventually forecast species spawning distribution as the stacked allele distributions changes in response to environmental variable changes.
Lastly, the abiotic input variables would be those relevant to explain species niche, such as temperature, salinity, oxygen concentrations, etc. Priors for these variables are key - because environmental conditions are highly dynamic in the marine environment - and can be obtained from several databases such as BioOracle (Assis et al., 2018). At the end, the output variable is the occurrence probability, or frequency, of candidate loci’s alleles in response to abiotic predictors. Species distribution is eventually obtained by stacking together genotype distributions, returning the potential full habitat suitability map at species level as a function of the adaptive potential if its populations (Figure 1). Adding phylogenetic trees to the J-SDM like framework can help identifying similarities between sampled locations genetic composition and characterize allele co-occurrence patterns.
The combined framework we propose here explores the adaptive potential of fish stocks while projecting it into future species distributions. As such, the framework not only has the potential to inform about the suitability of areas beyond the currently known viable distribution but also that species might adapt to environmental shifts within current distribution boundaries. In this context, mobility (to follow environmental optima) and adaptation (to remain in the same environment) are not mutually exclusive. For fisheries management, the realization of a dynamic fish stock both in terms of mobility and adaptive potential would necessarily translate in the following considerations:
1 – Local or regional extinction of stocks – often assumed when only mobility is considered – might not necessarily occur as a function of environmental shifts. This means that management bodies should not “give up” on the protection of specimens that are already moving to outside their respective areas of governance. If anything, it becomes even more important to protect mobile stocks because selective pressure posed by environmental shifts acts synergistically with anthropogenic pressure of fishing (Baltazar-Soares et al., 2021a; Hočevar and Kuparinen, 2021). In practice, identifying adaptive potential of stocks potentiates the development of measures to maintain local stocks levels despite species’ migratory capacity. Notably, mechanisms to implement measures stemming from the above premise already exist and are commonly applied upon stock crashes: fishing restrictions and imposition of quotas. The context however would differ, because in our particular example fish stocks are not necessarily in a vulnerable state in present conditions.
2 - New areas might be deemed as suitable on top of those that are currently being proposed by traditional SDMs approaches. The implications for management here are multiple and range from an increase of monitoring activities to detect the species presence at those sites, to the design of measures to preserve/protect of future spawning areas, and to a deeper investigation of biotic interactions that might result from those expansions. Perhaps in a first stage, biomonitoring activities appears to be immediately applicable measure in scientific fisheries surveys. Identifying this type of climate migrants and where/when they start to occur would be an indicator of change and evidence of shifting climatic conditions. Within a management framework, it would also likely lead to access the impact of these newcomers to nonnative to the sustainability of local fisheries.
We acknowledge that it might be challenging to accept the above considerations in the absence of the hindsight the future inevitably offers. Still, tackling climate-related issues requires enhancing of our predictive capacity and arguably no other framework would do it better besides one that incorporates ecological and evolutionary components. Still, it is important to remember that a framework like ours holds on assumptions that are in themselves speculations in the respective area of research. The most notable are those associated with the assignment of genetic variation to selective advantage and with habitat suitability disregarding biotic interactions in new habitats. It is obvious that our framework will certainly benefit from knowledge increments on genotype-phenotype-environment interactions in providing conclusive evidence for genomic signatures of selection, and in SDMs considering biotic interactions (HilleRisLambers et al., 2013; Pigot and Tobias, 2013). For example, expanding the spatial scope to other areas critical to marine organism’s life cycle such as nurseries or feeding grounds will certainly increment the resolution of analytical strategies focused on evolutionary responses. Likewise, considering genetic other architectures more complex than the simplistic genotype x phenotype view we illustrated here would also offer a more realistic picture of evolutionary responses. Until complexity arises, frameworks such as the one we propose here remain highly exploratory but nevertheless a stepping stone to the flourishing field of advanced fisheries research.
The original contributions presented in the study are included in the article/supplementary material. Further inquiries can be directed to the corresponding author.
All authors contributed to the article and approved the submitted version.
The authors acknowledge the Portuguese Foundation for Science and Technology (FCT) through the strategic project MARE-UIDB/04292/2020 granted to MARE (Marine and Environmental Sciences Centre) and through the project LA/P/0069/2020 granted to the Associate Laboratory ARNET. AL acknowledges the European Union’s (EU’s) Horizon 2020 (H2020) research and innovation programme under the Marie Skłodowska-Curie grant agreement No 101038057. EG acknowledges the Turku Collegium for Science, Medicine and Technology. MBS acknowledges the Academy of Finland funding decision 321417.
We also thank members of the ICES-PICES Working Group on Small Pelagic Fish (WGSPF) for helpful discussions.
The authors declare that the research was conducted in the absence of any commercial or financial relationships that could be construed as a potential conflict of interest.
All claims expressed in this article are solely those of the authors and do not necessarily represent those of their affiliated organizations, or those of the publisher, the editors and the reviewers. Any product that may be evaluated in this article, or claim that may be made by its manufacturer, is not guaranteed or endorsed by the publisher.
Allendorf F. W., Hohenlohe P. A., Luikart G. (2010). Genomics and the future of conservation genetics. Nat. Rev. Genet. 11 (10), 697–709. doi: 10.1038/nrg2844
Andrello M., D’Aloia C., Dalongeville A., Escalante M. A., Guerrero J., Perrier C., et al. (2022). Evolving spatial conservation prioritization with intraspecific genetic data. Trends Ecol. Evol 37 (6), 5537–564. doi: 10.1016/j.tree.2022.03.003
Assis J., Tyberghein L., Bosch S., Verbruggen H., Serrão E. A., De Clerck O. (2018). Bio-ORACLE v2. 0: Extending marine data layers for bioclimatic modelling. Global Ecol. biogeogr. 27 (3), 277–284. doi: 10.1111/geb.12693
Baltazar-Soares M., Hans-Harald H., Eizaguirre C. (2018). Integrating population genomics and biophysical models towards evolutionary-based fisheries management, ICES J. Mar. Sci. (2018) (Wiley Online Library), 75.4 1245–1257.
Baltazar-Soares M., Brans K. I., Eizaguirre C. (2021a). Human-induced evolution: Signatures, processes and mechanisms underneath anthropogenic footprints on natural systems. (Wiley Online Library) 14, 2335–2341.
Baltazar-Soares M., de Araújo Lima A. R., Silva G. (2021b). Targeted sequencing of mitochondrial genes reveals signatures of molecular adaptation in a nearly panmictic small pelagic fish species. Genes 12 (1), 91. doi: 10.3390/genes12010091
Benestan L. M., Ferchaud A. L., Hohenlohe P. A., Garner B. A., Naylor G. J., Baums I. B., et al. (2016). Conservation genomics of natural and managed populations: Building a conceptual and practical framework. Mol. Ecol. 25 (13), 2967–2977. doi: 10.1111/mec.13647
Bernatchez L., Wellenreuther M., Araneda C., Ashton D. T., Barth J. M. I., Beacham T. D., et al. (2017). Harnessing the power of genomics to secure the future of seafood. Trends Ecol. Evol. 32 (9), 665–680. doi: 10.1016/j.tree.2017.06.010
Blasco G. D., Ferraro D. M., Cottrell R. S., Halpern B. S., Froehlich H. E. (2020). Substantial gaps in the current fisheries data landscape. Front. Mar. Sci. 7, 612831. doi: 10.3389/fmars.2020.612831
Bolstad W. M., Curran J. M. (2016). Introduction to Bayesian statistics ( Hoboken, New Jersey: John Wiley & Sons, Inc.,).
Brander K. (2010). Impacts of climate change on fisheries. J. Mar. Syst. 79 (3-4), 389–402. doi: 10.1016/j.jmarsys.2008.12.015
Bush A., Mokany K., Catullo R., Hoffmann A., Kellermann V., Sgrò C., et al. (2016). Incorporating evolutionary adaptation in species distribution modelling reduces projected vulnerability to climate change. Ecol. Lett. 19 (12), 1468–1478. doi: 10.1111/ele.12696
Capblancq T., Fitzpatrick M. C., Bay R. A., Exposito-Alonso M., Keller S. R. (2020). Genomic prediction of (mal) adaptation across current and future climatic landscapes. Annu. Rev. Ecol. Evol System. 51 (1), 245–269. doi: 10.1146/annurev-ecolsys-020720-042553
Cardinale B. J., Duffy J. E., Gonzalez A., Hooper D. U., Perrings C., Venail P., et al. (2012). Biodiversity loss and its impact on humanity. Nature 486 (7401), 59–67. doi: 10.1038/nature11148
Casey J., Jardim E., Martinsohn J. T. (2016). The role of genetics in fisheries management under the EU common fisheries policy. J. Fish. Biol. 89 (6), 2755–2767. doi: 10.1111/jfb.13151
Chardon N. I., Pironon S., Peterson M. L., Doak D. F. (2020). Incorporating intraspecific variation into species distribution models improves distribution predictions, but cannot predict species traits for a wide-spread plant species. Ecography 43 (1), 60–74. doi: 10.1111/ecog.04630
Cheung W. W. L., Lam V. W. Y., Sarmiento J. L., Kearney K., Watson R., Pauly D. (2009). Projecting global marine biodiversity impacts under climate change scenarios. Fish Fish. 10 (3), 235–251. doi: 10.1111/j.1467-2979.2008.00315.x
Cotto O., Wessely J., Georges D., Klonner G., Schmid M., Dullinger S., et al. (2017). A dynamic eco-evolutionary model predicts slow response of alpine plants to climate warming. Nat. Commun. 8 (1), 1–9. doi: 10.1038/ncomms15399
DeMarche M. L., Doak D. F., Morris W. F. (2019). Incorporating local adaptation into forecasts of species’ distribution and abundance under climate change. Global Change Biol. 25 (3), 775–793. doi: 10.1111/gcb.14562
Eizaguirre C., Baltazar-Soares M. (2014). Evolutionary conservation–evaluating the adaptive potential of species. Evol. Appl. 7 (9), 963–967. doi: 10.1111/eva.12227
Ellegren H. (2004). Microsatellites: simple sequences with complex evolution. Nat. Rev. Genet. 5 (6), 435–445. doi: 10.1038/nrg1348
Fitzpatrick M. C., Weltzin J. F., Sanders N. J., Dunn R. R. (2007). The biogeography of prediction error: why does the introduced range of the fire ant over-predict its native range? Global Ecol. biogeogr. 16 (1), 24–33. doi: 10.1111/j.1466-8238.2006.00258.x
Forester B. R., Jones M. R., Joost S., Landguth E. L., Lasky J. R. (2016). Detecting spatial genetic signatures of local adaptation in heterogeneous landscapes. Mol. Ecol. 25 (1), 104–120. doi: 10.1111/mec.13476
Frankham R. (2010). Where are we in conservation genetics and where do we need to go? Conserv. Genet. 11 (2), 661–663. doi: 10.1007/s10592-009-0010-2
Frichot E., François O. (2015). LEA: An r package for landscape and ecological association studies. Methods Ecol. Evol. 6 (8), 925–929. doi: 10.1111/2041-210X.12382
Frölicher T. L., Laufkötter C. (2018). Emerging risks from marine heat waves. Nat. Commun. 9 (1), 1–4. doi: 10.1038/s41467-018-03163-6
Gobeyn S., Mouton A. M., Cord A. F., Kaim A., Volk M., Goethals P. L. (2019). Evolutionary algorithms for species distribution modelling: A review in the context of machine learning. Ecol. Model. 392, 179–195. doi: 10.1016/j.ecolmodel.2018.11.013
Guisan A., Thuiller W. (2005). Predicting species distribution: offering more than simple habitat models. Ecol. Lett. 8 (9), 993–1009. doi: 10.1111/j.1461-0248.2005.00792.x
Günther T., Coop G. (2013). Robust identification of local adaptation from allele frequencies. Genetics 195 (1), 205–220. doi: 10.1534/genetics.113.152462
Hauser L., Carvalho G. R. (2008). Paradigm shifts in marine fisheries genetics: ugly hypotheses slain by beautiful facts. Fish. Fish. 9 (4), 333–362. doi: 10.1111/j.1467-2979.2008.00299.x
Heckwolf M. J., Meyer B. S., Häsler R., Höppner M. P., Eizaguirre C., Reusch T. B. (2020). Two different epigenetic information channels in wild three-spined sticklebacks are involved in salinity adaptation. Sci. Adv. 6 (12), eaaz1138. doi: 10.1126/sciadv.aaz1138
HilleRisLambers J., Harsch M. A., Ettinger A. K., Ford K. R., Theobald E. J. (2013). How will biotic interactions influence climate change–induced range shifts? Ann. New York Acad. Sci. 1297 (1), 112–125. doi: 10.1111/nyas.12182
Hoban S., Kelley J. L., Lotterhos K. E., Antolin M. F., Bradburd G., Lowry D. B., et al. (2016). Finding the genomic basis of local adaptation: Pitfalls, practical solutions, and future directions. Am. Nat. 188 (4), 379–397. doi: 10.1086/688018
Hočevar S., Kuparinen A. (2021). Marine food web perspective to fisheries-induced evolution. Evol. Appl. 14 (10), 2378–2391. doi: 10.1111/eva.13259
Hollowed A. B., Barange M., Beamish R. J., Brander K., Cochrane K., Drinkwater K., et al. (2013). Projected impacts of climate change on marine fish and fisheries. ICES J. Mar. Sci. 70 (5), 1023–1037. doi: 10.1093/icesjms/fst081
Huang Y., Chain F. J. J., Panchal M., Eizaguirre C., Kalbe M., Lenz T. L., et al. (2016). Transcriptome profiling of immune tissues reveals habitat-specific gene expression between lake and river sticklebacks. Mol. Ecol. 25 (4), 943–958. doi: 10.1111/mec.13520
Hu T., Chitnis N., Monos D., Dinh A. (2021). Next-generation sequencing technologies: An overview. Hum. Immunol. 82 (11), 801–811. doi: 10.1016/j.humimm.2021.02.012
Huelsenbeck J. P., Ronquist F., Nielsen R., Bollback J. P. (2001). Bayesian Inference of phylogeny and its impact on evolutionary biology. Science 294 (5550), 2310–2314 doi: 10.1126/science.1065889.
Ikeda D. H., Max T. L., Allan G. J., Lau M. K., Shuster S. M., Whitham T. G. (2017). Genetically informed ecological niche models improve climate change predictions. Global Change Biol. 23 (1), 164–176. doi: 10.1111/gcb.13470
Jarne P., Lagoda P. J. (1996). Microsatellites, from molecules to populations and back. Trends Ecol. Evol. 11 (10), 424–429. doi: 10.1016/0169-5347(96)10049-5
Jghab A., Vargas-Yañez M., Reul A., Garcia-Martínez M., Hidalgo M., Moya F., et al. (2019). The influence of environmental factors and hydrodynamics on sardine (Sardina pilchardus, walbaum 1792) abundance in the southern alboran Sea. J. Mar. Syst. 191, 51–63. doi: 10.1016/j.jmarsys.2018.12.002
Kaufmann J., Lenz T. L., Milinski M., Eizaguirre C. (2014). Experimental parasite infection reveals costs and benefits of paternal effects. Ecol. Lett. 17 (11), 1409–1417. doi: 10.1111/ele.12344
Lam V. W., Allison E. H., Bell J. D., Blythe J., Cheung W. W., Frölicher T. L., et al. (2020). Climate change, tropical fisheries and prospects for sustainable development. Nat. Rev. Earth Environ. 1 (9), 440–454. doi: 10.1038/s43017-020-0071-9
Laman E. A., Rooper C. N., Rooney S. C., Turner K. A., Cooper D. W., Zimmermann M. (2017). Model-based essential fish habitat definitions for Bering Sea groundfish species.
Laman E. A., Rooper C. N., Turner K., Rooney S., Cooper D. W., Zimmermann M. (2018). Using species distribution models to describe essential fish habitat in Alaska. Can. J. Fish. Aquat. Sci. 75 (8), 1230–1255. doi: 10.1139/cjfas-2017-0181
Layton K. K., Bradbury I. R. (2022). Harnessing the power of multi-omics data for predicting climate change response. J. Anim. Ecol. 91 (6), 1064–1072. doi: 10.1111/1365-2656.13619
Lenz T. L., Eizaguirre C., Rotter B., Kalbe M., Milinski M. (2013). Exploring local immunological adaptation of two stickleback ecotypes by experimental infection and transcriptome-wide digital gene expression analysis. Mol. Ecol. 22 (3), 774–786. doi: 10.1111/j.1365-294X.2012.05756.x
Lima A. R., Baltazar-Soares M., Garrido S., Riveiro I., Carrera P., Piecho-Santos A. M., et al. (2022). Forecasting shifts in habitat suitability across the distribution range of a temperate small pelagic fish under different scenarios of climate change. Sci. Total Environ. 804, 150167. doi: 10.1016/j.scitotenv.2021.150167
Lima A. R., Garrido S., Riveiro I., Rodrigues D., Borges R., Angélico M. M., et al. (2022). Seasonal approach to forecast the suitability of spawning habitats of a temperate small pelagic fish under a high-emission climate change scenario. Front. Mar. Sci., 1523. doi: 10.3389/fmars.2022.956654
Lohbeck K. T., Riebesell U., Reusch T. B. H. (2012). Adaptive evolution of a key phytoplankton species to ocean acidification. Nat. Geosci. 5 (5), 346–351. doi: 10.1038/ngeo1441
Lotterhos K. E., Whitlock M. C. (2015). The relative power of genome scans to detect local adaptation depends on sampling design and statistical method. Mol. Ecol. 24 (5), 1031–1046. doi: 10.1111/mec.13100
Mardis E. R. (2008a). The impact of next-generation sequencing technology on genetics. Trends Genet. 24 (3), 133–141. doi: 10.1016/j.tig.2007.12.007
Mardis E. R. (2008b). Next-generation DNA sequencing methods. Annu. Rev. Genomics Hum. Genet. 9 (1), 387–402. doi: 10.1146/annurev.genom.9.081307.164359
Nielsen E. S., Henriques R., Beger M., von der Heyden S. (2021). Distinct interspecific and intraspecific vulnerability of coastal species to global change. Global Change Biol. 27 (15), 3415–3431. doi: 10.1111/gcb.15651
Nielsen R., Slatkin M. (2013). An introduction to population genetics (Sunderland, MA: Sinauer Associates).
Oomen R. A., Hutchings J. A. (2022). Genomic reaction norms inform predictions of plastic and adaptive responses to climate change. J. Anim. Ecol. 91 (6), 1073–1087. doi: 10.1111/1365-2656.13707
Ovaskainen O., Tikhonov G., Norberg A., Guillaume Blanchet F., Duan L., Dunson D., et al. (2017). How to make more out of community data? a conceptual framework and its implementation as models and software. Ecol. Lett. 20 (5), 561–576. doi: 10.1111/ele.12757
Peel S. L., Hill N. A., Foster S. D., Wotherspoon S. J., Ghiglione C., Schiaparelli S. (2019). Reliable species distributions are obtainable with sparse, patchy and biased data by leveraging over species and data types. Methods Ecol. Evol. 10 (7), 1002–1014. doi: 10.1111/2041-210X.13196
Pigot A. L., Tobias J. A. (2013). Species interactions constrain geographic range expansion over evolutionary time. Ecol. Lett. 16 (3), 330–338. doi: 10.1111/ele.12043
Planque B., Bellier E., Lazure P. (2007). Modelling potential spawning habitat of sardine (Sardina pilchardus) and anchovy (Engraulis encrasicolus) in the bay of Biscay. Fish. Oceanogr. 16 (1), 16–30. doi: 10.1111/j.1365-2419.2006.00411.x
Porfirio L. L., Harris R. M., Lefroy E. C., Hugh S., Gould S. F., Lee G., et al. (2014). Improving the use of species distribution models in conservation planning and management under climate change. PloS One 9 (11), e113749. doi: 10.1371/journal.pone.0113749
Raybaud V., Bacha M., Amara R., Beaugrand G. (2017). Forecasting climate-driven changes in the geographical range of the European anchovy (Engraulis encrasicolus). ICES J. Mar. Sci. 74 (5), 1288–1299. doi: 10.1093/icesjms/fsx003
Reed D. H., Frankham R. (2003). Correlation between fitness and genetic diversity correlación entre adaptabilidad y diversidad genética. Conserv. Biol. 17 (1), 230–237. doi: 10.1046/j.1523-1739.2003.01236.x
Royle J. A., Chandler R. B., Yackulic C., Nichols J. D. (2012). Likelihood analysis of species occurrence probability from presence-only data for modelling species distributions. Methods Ecol. Evol. 3 (3), 545–554. doi: 10.1111/j.2041-210X.2011.00182.x
Ruegg K., Bay R. A., Anderson E. C., Saracco J. F., Harrigan R. J., Whitfield M., et al. (2018). Ecological genomics predicts climate vulnerability in an endangered southwestern songbird. Ecol. Lett. 21 (7), 1085–1096. doi: 10.1111/ele.12977
Schickele A., Goberville E., Leroy B., Beaugrand G., Hattab T., Francour P., et al (2020). European Small pelagic fish distribution under global change scenarios. Fish. Fish. 22 (1), 212–225. doi: 10.1111/faf.12515
Stead S. M. (2018). Rethinking marine resource governance for the united nations sustainable development goals. Curr. Opin. Environ. Sustainability 34, 54–61. doi: 10.1016/j.cosust.2018.12.001
Therkildsen N. O., Hemmer-Hansen J., Hedeholm R. B., Wisz M. S., Pampoulie C., Meldrup D., et al. (2013). Spatiotemporal SNP analysis reveals pronounced biocomplexity at the northern range margin of Atlantic cod gadus morhua. Evol. Appl. 6 (4), 690–705. doi: 10.1111/eva.12055
Thorrold Simon R., et al. (2001). Natal homing in a marine fish metapopulation. Science 291.5502, 297–299.
Thuiller W., Münkemüller T., Lavergne S., Mouillot D., Mouquet N., Schiffers K., et al. (2013). A road map for integrating eco-evolutionary processes into biodiversity models. Ecol. Lett. 16, 94–105. doi: 10.1111/ele.12104
Van Ravenzwaaij D., Cassey P., Brown S. D. (2018). A simple introduction to Markov chain Monte–Carlo sampling. Psychonomic. Bull. Rev. 25 (1), 143–154. doi: 10.3758/s13423-016-1015-8
Vermeiren P., Reichert P., Schuwirth N. (2020). Integrating uncertain prior knowledge regarding ecological preferences into multi-species distribution models: Effects of model complexity on predictive performance. Ecol. Model. 420, 108956. doi: 10.1016/j.ecolmodel.2020.108956
Verspoor E., Stradmeyer L., Nielsen J. L. (2008). The Atlantic salmon: genetics, conservation and management (Oxford: : Blackwell Pub).
Vieira M. L. C., Santini L., Diniz A. L., Munhoz C. (2016). Microsatellite markers: what they mean and why they are so useful. Genet. Mol. Biol. 39, 312–328. doi: 10.1590/1678-4685-GMB-2016-0027
Villero D., Pla M., Camps D., Ruiz-Olmo J., Brotons L. (2017). Integrating species distribution modelling into decision-making to inform conservation actions. Biodiver. Conserv. 26 (2), 251–271. doi: 10.1007/s10531-016-1243-2
Whitlock M. C., Lotterhos K. E. (2015). Reliable detection of loci responsible for local adaptation: inference of a null model through trimming the distribution of f ST. Am. Nat. 186 (S1), S24–S36. doi: 10.1086/682949
Yau C., Campbell K. (2019). Bayesian Statistical learning for big data biology. Biophys. Rev. 11 (1), 95–102. doi: 10.1007/s12551-019-00499-1
Zurell D., Franklin J., König C., Bouchet P. J., Dormann C. F., Elith J., et al. (2020). A standard protocol for reporting species distribution models. Ecography 43 (9), 1261–1277. doi: 10.1111/ecog.04960
Zurell D., König C., Malchow A. K., Kapitza S., Bocedi G., Travis J., et al. (2022). Spatially explicit models for decision-making in animal conservation and restoration. Ecography 2022 (4). doi: 10.1111/ecog.05787
Keywords: high-throughput sequencing, genomics, species distribution model (SDMs), fisheries applications, evolutionary ecology
Citation: Baltazar-Soares M, Lima ARA, Silva G and Gaget E (2023) Towards a unified eco-evolutionary framework for fisheries management: Coupling advances in next-generation sequencing with species distribution modelling. Front. Mar. Sci. 9:1014361. doi: 10.3389/fmars.2022.1014361
Received: 08 August 2022; Accepted: 06 December 2022;
Published: 06 January 2023.
Edited by:
Fran Saborido-Rey, Spanish National Research Council (CSIC), SpainReviewed by:
Jitendra Kumar Sundaray, Central Institute of Freshwater Aquaculture (ICAR), IndiaCopyright © 2023 Baltazar-Soares, Lima, Silva and Gaget. This is an open-access article distributed under the terms of the Creative Commons Attribution License (CC BY). The use, distribution or reproduction in other forums is permitted, provided the original author(s) and the copyright owner(s) are credited and that the original publication in this journal is cited, in accordance with accepted academic practice. No use, distribution or reproduction is permitted which does not comply with these terms.
*Correspondence: Miguel Baltazar-Soares, bWlndWVsYWxleHNvYXJlc0BnbWFpbC5jb20=
Disclaimer: All claims expressed in this article are solely those of the authors and do not necessarily represent those of their affiliated organizations, or those of the publisher, the editors and the reviewers. Any product that may be evaluated in this article or claim that may be made by its manufacturer is not guaranteed or endorsed by the publisher.
Research integrity at Frontiers
Learn more about the work of our research integrity team to safeguard the quality of each article we publish.