- Ocean Science and Technology for Islands, National Institute of Ocean Technology, Ministry of Earth Sciences (Govt. of India), Chennai, India
Introduction
The productivity and sustainability of coastal, marine, and estuarine ecosystems are heavily reliant on the quality of coastal waters (Jha et al., 2013; Jha et al., 2015; Dheenan et al., 2016). These locations are thought to have greater biodiversity than open ocean zones (Gray, 1997). Among the biological components of soft bottom sediments, macrobenthic organisms are essential for ecosystem functioning (Pandey et al., 2022). They play a vital role in secondary production and nutrient exchange between the pelagic and benthic realms and therefore constitute essential elements of estuarine and coastal habitats (Snelgrove, 1998) and are a reliable indicator for assessing the biotic integrity of the coastal ecosystem (Ryu et al., 2011; Pandey et al., 2021). Due to their sedentary lifestyle, long life cycle, and differential response against the perturbation, they also serve as useful bio-indicator (Gesteira-Gomez et al., 2003) for health monitoring and assessment of coastal ecosystems (Ganesh et al., 2014) and evaluating the success of conservation efforts (Winberg et al., 2007).
Identification of factors responsible for spatiotemporal patterns in macrofaunal assemblages is the primary goal amongst many marine benthic ecological studies (Bolam et al., 2008; Dutertre et al., 2013; Pandey and Ganesh, 2019b). However, such pattern in the benthic community is often hard to predict due to the complex interplay between environmental parameters and biological interactions (Ellis et al., 2006). The relationship between natural environmental factors and macrobenthos is reported to help delineate faunal distribution patterns, characterising benthic habitats, establish baseline knowledge, and enabling the detection of spatial and temporal variations (Bolam et al., 2008; Shumchenia and King, 2010; Dutertre et al., 2013). Numerous studies (Van Hoey et al., 2004; Hily et al., 2008; Dutertre et al., 2013; Pandey and Ganesh, 2019b) have identified sediment properties as a significant factor for the distribution of macrofauna. However, hydrodynamic conditions and physicochemical properties are also reported to influence the presence and abundance of benthic species (Ysebaert and Herman, 2002) as these factors control the food supply, larval dispersion, and metabolism (Pearson and Rosenberg, 1987; Shanks et al., 2003). At short temporal scale, such as seasonal, monthly, or fortnightly, the physicochemical properties of the water column can vary significantly while sediment properties remain relatively stable (Dutertre et al., 2013), therefore, in addition to sediment properties, evaluation of physicochemical properties of the water column is equally important for elucidating the distribution of benthic fauna.
Studies relating the environmental parameters and macrobenthos has received global (Bolam et al., 2008; Shumchenia and King, 2010; Cisneros et al., 2011; Dutertre et al., 2013) as well as regional attention in India (Sivadas et al., 2012; Equbal et al., 2018; Pandey and Ganesh, 2019a; Pandey and Ganesh, 2019b; Rehitha et al., 2019). However, the sub-tidal macrobenthic community dynamics concerning environmental drivers are limited, particularly for Diu Island. The island receives the majority of rainfall between June to September due to the southwest monsoon. There are no industries but tourist, domestic discharge, fishing, and port activities are the major source of disturbances to the natural environment. The lack of knowledge about temporal variability makes it difficult to distinguish between natural and man-induced changes (Hewitt and Thrush 2007; Sivadas et al., 2011). The present study was undertaken to understand the temporal variability of macrofaunal community composition and diversity, effects of monsoon-mediated variability in the physicochemical parameters of the water column as well as sediment and their impacts on the macrofauna community.
Materials and methods
Diu (20.71°N and 70.98°E), a union territory of India, is a small island with a geographical area of 40 Km2, situated in the northeastern Arabian Sea. The average annual rainfall is recorded 550 millimeters (https://diu.gov.in/about-diu.php), while the average annual ambient atmospheric temperature ranged between 15°C and 42°C (Jha et al., 2021; Kumar et al., 2022). The benthic sampling was carried out at seven predetermined stations (S-1,2,3,4,5,6, and 7) of Diu island in February, September, and November during the year 2018 covering pre-monsoon (PRM), monsoon (MON), and post-monsoon (POM) periods, respectively (Figure 1A). Each season, triplicate samples were collected from all the stations to estimate the physiochemical properties of seawater and sediments. Seawater samples were collected in polypropylene bottles for nutrient estimation using a Niskin’s water sampler from a boat. Samples for Dissolved Oxygen (DO) and Biochemical Oxygen Demand (BOD) were analyzed by Winkler’s titration method (Grasshoff et al., 1999). For BOD estimation, before titration, samples were stored in a dark chamber for 5 days at 20 ± 1°C (APHA 5210B). Salinity, pH, and temperature were analyzed with a pre-calibrated handheld water quality probe (Hanna HI9829). The nutrient parameters namely nitrite, nitrate, inorganic phosphate (IP), silicate, total nitrogen (TN), ammonia, and total phosphorus (TP) were estimated by following the standard spectrophotometric methods of Grasshoff et al. (1999). At each station, sediment samples were taken for the analysis of organic matter (OM). The sediment samples were dried at 60°C in a hot air oven for the estimation of OM. The total organic carbon (TOC) was estimated by following the modified Walkley Black wet oxidation method (Gaudette et al., 1974) and multiplied with a Van Bemmelen factor of 1.724 (Trask, 1939) and expressed as OM in percentage (%).
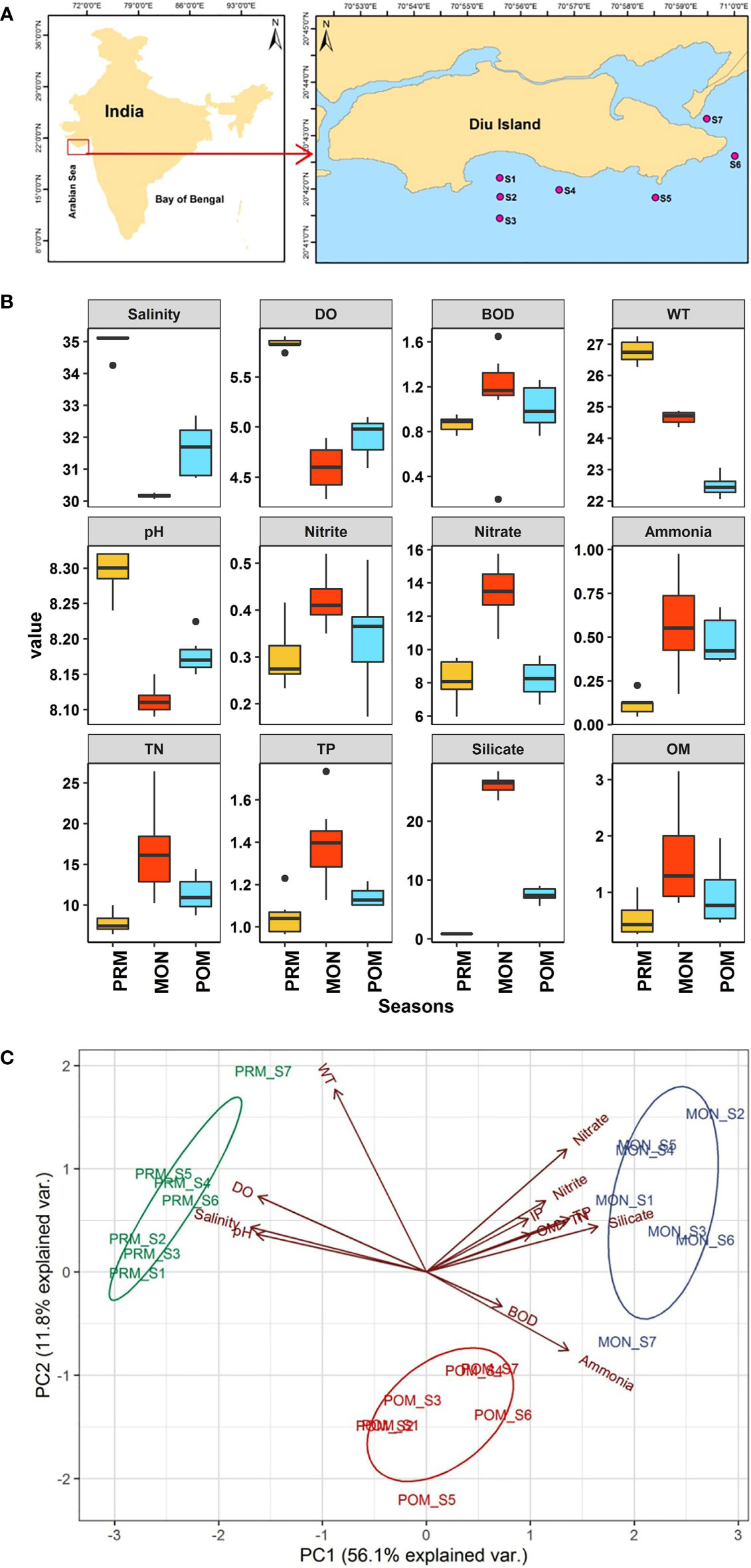
Figure 1 (A) Study area map, red points in enlarged map show the sampling stations along the coastal region of Diu island, (B) Box plots showing the variations in physicochemical parameters during different seasons. The central line of each boxes represents the median, the rectangle gives the interval between 25% and 75% percentiles, whiskers indicate the range and black dots represent outliers. Y- axis represents the value of each parameter namely DO and BOD are in mg/L, Salinity (PSU), WT (°C) and OM in %, whereas Ammonia, IP, Nitrate, Nitrite, Silicate, TN and TP are in µL/L. X-axis represents different seasons, (C) PCA biplot illustrating the variability in the environmental parameters between three seasons.
Macrofaunal samples were collected in triplicates with a van Veen grab (0.02 m2) at each station. To prevent movement and distortion of the macrofauna during sieving, the collected sediment samples were mixed with relaxant (magnesium chloride solution, 73 g of MgCl2 in 1000 ml of filtered seawater) before sieving. A test sieve with a mesh size of 0.5 mm was used to sieve the samples and retained content was transferred to a sample container with 5% buffered formalin mixed with Rose Bengal stain. In the laboratory, the samples were again washed and macrofauna was picked up from the residue, labelled, and stored in 70% ethanol (v/v). All taxa were first segregated into different groups and then identified up to the lowest possible taxonomic level followed by the count of individuals. The species’ taxonomic information was validated and authenticated by the WoRMS Editorial Board (2021) and the density of the macrofauna was represented as individuals per 0.02 square meter area (ind/0.02m2).
Data analysis
The analysis of variance (ANOVA-one way) and Tukey’s Honest Significant Difference (HSD) post hoc test were carried out to find out the significance of spatial and temporal variation for the univariate measures of macrofauna and environmental parameters. The data were examined for normality with Shapiro-Wilk’s W test before being subjected to ANOVA. In case of data normality violation, non-parametric ANOVA (Kruskal–Wallis H test) was used followed by pairwise Wilcoxon tests with multiple testing corrections. The univariate indices such as the number of taxa (S), abundance (N, ind/0.02m2), Margalef’s species richness (d), Shannon-Wiener diversity index (H′, loge), and Pielou’s evenness (J′) were estimated. Principal Component Analysis (PCA) after the normalization of data was performed to identify the dominant environmental factors responsible for sample segregation. To examine how macrofauna and environmental variables were related, BIOENV (Clarke and Ainsworth, 1993) and BVSTEP (Clarke and Warwick, 1998) analyses were used. These analyses helped to find out the best set of environmental variables and taxa by examining all the possible subsets of variables showing the maximum correlation with community dissimilarities. Similarity matrices for taxonomic and environmental data were computed based on Bray-Curtis similarity and Spearman rank correlation coefficients between the two matrices. The Spearman rank ρ values indicate the rank correlation between the matrix; the macrofauna community composition and similarity matrices from the environmental variables. The best subset of taxa was identified by Mantel tests performed by the BVSTEP and subjected to a permutation test to determine significance. The vectors of the best correlated environmental variables and macrofauna were overlaid on the NMDS plot. All the statistical analyses were carried out in open-source software R version 3.6.3 (R Core Team, 2020). Packages used for the analyses were “vegan” (Oksanen et al., 2020), “tidyverse” (Wickham, 2017), and “ggplot2” (Wickham et al., 2019).
Results and discussion
Environmental parameters
The overall seasonal variability of the physicochemical parameters is given in Figure 1B. Salinity ranged between 30.05 and 35.16 PSU with maximum mean salinity of 34.99 ± 0.32 PSU in PRM and a minimum of 30.15 ± 0.06 PSU in MON. The lowering of salinity values during the MON may be attributed to the influx of freshwater due to the high rainfall associated with this season (Kumar et al., 2022). A Kruskal-Wallis H test showed that there was a statistically significant difference in salinity between different seasons, χ2 = 17.853, p = 0.0001. The pairwise comparison revealed that the salinity was significantly different in each season. Similarly, dissolved oxygen, water temperature, and pH also followed the same trend, showing higher values during the PRM and lower values during the MON. The record of high DO (5.83 ± 0.05 mg/L) values during the PRM is associated with the photosynthetic activities of phytoplankton increasing the DO concentration of seawater (Kumar et al., 2022). In contrast, BOD was higher during the MON (1.12 ± 0.45 mg/L) and lower values were observed during the PRM (0.86 ± 0.06 mg/L). Similarly, nutrients (nitrite, nitrate, ammonia, TN, IP, TP, and silicate) in seawater also showed higher values in MON (Figure 1B) whereas organic matter in sediment also showed higher levels in MON (1.58 ± 0.86%) followed by POM (0.95 ± 0.55%) and PRM (0.53 ± 0.3%). The higher level of BOD, nutrients, and OM during the MON appropriately showed a decreasing trend from MON to the PRM. The rainfall is known to impact the physicochemical properties of seawater in tropical regions (Ratnam et al., 2022). The increased OM during the MON was possibly due the land runoff resulting from heavy rainfall that occur during this period (Pandey and Ganesh, 2019b). The one-way parametric and non-parametric ANOVA test showed that, except for BOD and IP (p > 0.05), all the physicochemical parameters were significantly different (p < 0.05) between seasons. The post hoc pairwise comparison revealed that the significant difference in ammonia was due to lower concentration (0.11 ± 0.05 µL/L) during the PRM, whereas the variation in nitrite, nitrate, TN, TP, and OM was due to their significantly high concentration during the MON. In contrast, pH, salinity, silicate, and water temperature were significantly different for each season.
The eigenvalues (>1) showed three significant components and explained 78.7% of the variance in the PCA plot (Figure 1C). However, only the first two components were taken for the result interpretation as they explained 68% of variations. Liu et al. (2003) criteria were followed for factor loadings classification as strong (> 0.75), moderate (0.75-0.50), and weak (0.50-0.30). The first principal component (PC1) explained 56.1% variance and showed strong negative loadings of salinity (-0.95), DO (-0.91), and pH (-0.92) which prevailed during PRM. The second principal component (PC2) explained 11.8% of the variance and showed strong positive loading of water temperature (0.79) and weak negative loadings for BOD (-0.14) and ammonia (-0.34). Overall, the PCA showed a strong temporal variability of physicochemical parameters during the study period. The factor loading matrix, eigenvalues, total & cumulative variance values, and variable loadings (communality) are presented in Table S1 (Supplemental Material).
Macrobenthos structure
Thirty-seven taxa were recorded from the sampling stations covered during the present study at the Diu coast. The majority of taxa belonged to two major faunal groups namely Polychaeta (24 taxa) and Amphipoda (8 taxa), whereas one representative taxa of Tanaidacea, Nemertea, Ophiuroidea, Sipuncula, and Scaphopoda were also observed. Overall, 1083 individuals were recorded during the study, of which 66.94% of the total abundance belonged to Polychaeta and 22.06% to Amphipoda. Numerically, Cirratulidae (12.83%) dominated the community followed by Spionidae (10.24%), Maldanidae (7.66%), Tanaissuidae (7.47%), and Urothoidae (5.63%). The detail on the mean abundance of all the taxa recorded during the study is given in Table S2. The overall abundance of macrofauna ranged between 18 and 100 ind/0.02m2 with maximum mean abundance recorded during the MON (59 ± 27 ind/0.02m2) and the minimum was recorded during the POM (40.71 ± 5.64 ind/0.02m2). However, the difference in the abundance was insignificant between all the seasons (ANOVA; F = 1.663, p = 0.21).
The number of macrofaunal taxa (S) ranged between 13 and 29, species richness (Margalef’s d) (3.03 to 7.53), and evenness (J’) (0.84 to 0.97), Shannon-Wiener diversity index (H′ loge) (2.36 to 3.26). All the indices showed higher values during the PRM (Figure 2A). Except for the evenness (χ2 = 2.278, p = 0.32), all the indices showed a significant difference (p < 0.05) between the seasons. The Tukey pairwise HSD post hoc test revealed that the significant difference was due to lower values during the MON, whereas, there was no significant difference between PRM and POM (Table S3). The results of ANOVA and pairwise seasonal comparison of environmental as well as univariate measures of macrofauna is summarised in Table S3. Taken together, the results indicate that the PRM and POM period harbored a rather diverse and stable macrobenthic faunal community compared to that of MON. Generally, in tropical conditions, the abundance of macrofauna tends to decrease during the monsoon due to the variation in the environmental parameters (Alongi, 1990; Sivadas et al., 2011; Sivadas et al., 2012). However, in the island ecosystem, this general pattern varies as our results did not show significant variation in the abundance between seasons which is in agreement with the macrofaunal community pattern in the Andaman Islands (Pandey and Ganesh, 2019b). Since, all macrofauna are not equally adapted to withstand the salinity fluctuation and organic matter influx that happens during the monsoon (Gray et al., 2002), therefore, as the monsoon progresses, sensitive species that are susceptible to such disturbances start to diminish in abundance and are replaced by tolerant species in greater numbers. As a result, overall abundance increases while diversity begins to decrease. Further, heavy metals are also known to impact the benthic fauna and may limit the species composition or defaunate the sediment (Pandey et al., 2021). A comparatively higher concentration of cadmium metal was reported from the same region in the MON (Jha et al., 2021). Under such a scenario, sensitive taxa get eliminated which paves the way for tolerant and opportunistic fauna to proliferate. However, in present study, the abundance of macrofauna was not different from a random pattern but the relative abundance of tolerance and opportunistic taxa such as Cirriformia filigera, C. tentaculata, Dodecaceria sp., Paraprionospio cordifolia, P. patiens, Cossura coasta, Maldanella capensis, and Notomastus aberans was higher during the MON (Table S2). These opportunistic faunas have remarkable tolerance to organic enrichment and other pollutants (Levin et al., 1996; Chandler et al., 1997; Holmer et al., 1997) and proliferate in a reduced environment which was evident from their higher numbers during the MON. As the monsoon passes and the environment progresses towards stability, the abundance of tolerant species declines due to competition for food and space leading to increased evenness, higher diversity, and a stable benthic environment during the post-monsoon condition (Parulekar et al., 1980; Harkantra and Rodrigues, 2003). The post-monsoon period in the present study also showed significant improvement in terms of richness and diversity indicating the faster recovery of the macrobenthic faunal community during the stable POM and PRM.
The NMDS ordination revealed temporal variability in the macrobenthic assemblage pattern. However, the major differences in the assemblage were observed between the MON and the non-monsoon periods including PRM and POM. A distinct assemblage composition was not observed between PRM and POM. BIOENV analysis showed a relatively weak correlation between the environmental parameters and macrofauna. However, the best subset of environmental parameters with the highest rank correlation was obtained with a combination of salinity, temperature, nitrite, total nitrogen, total phosphate, silicate, and OM (ρ = 0.42). Environmental parameters namely nitrite, total nitrogen, total phosphate, silicate, and OM were important variables associated with differences in macrofaunal community structure between monsoon (MON) and non-monsoon (PRM and POM) seasons (Figure 2B). The best subset of taxa identified by BVSTEP routine were Cossura coasta, Sigambra robusta, Goniada emerita, Notomastus aberans, Armandia intermedia, Ophelina acuminata, Paraprinospio patiens, Sabella fusca, Urothoe marina, and Aoroides sp., with a rank correlation of 0.89. Among the identified fauna, except S. robusta, O. acuminata, and Aoroides sp., all were significantly correlated with community dissimilarity (Table S4). Macrobenthic community is structured by a combination of different parameters rather than being controlled by any single parameter alone (Snelgrove and Butman, 1994). This was also reflected in the marofaunal community pattern of the Diu coast which was supported by the integrated NMDS and BIOENV results which revealed a combination of parameters plays different roles in different seasons. The different assemblage pattern during the MON was governed by the increased concentration of nitrite, total nitrogen, total phosphate, silicate, and OM. In contrast, increased salinity and temperature were responsible for the assemblage pattern during PRM and POM. Likewise, taxa that showed significant association with the corresponding seasons were identified by BVSTEP. The analysis revealed three species, namely Cossura coasta, Notomastus aberans, and Paraprinospio patiens showing significant association with the MON (Figure 2B). These species may be indicators given their prevalence in the MON with increased organic concentration. The significant association of these taxa with the MON justified the prevailing notion of disturbance caused by monsoon turbulence along the west coast of the Indian Peninsula. The current assessment is a preliminary investigation that will be useful to assess the health of the local benthic and associated aquatic fauna, which in turn will help in coastal conservation and fisheries management (Murugan et al., 2005; Kumar et al., 2009; Jha et al., 2017).
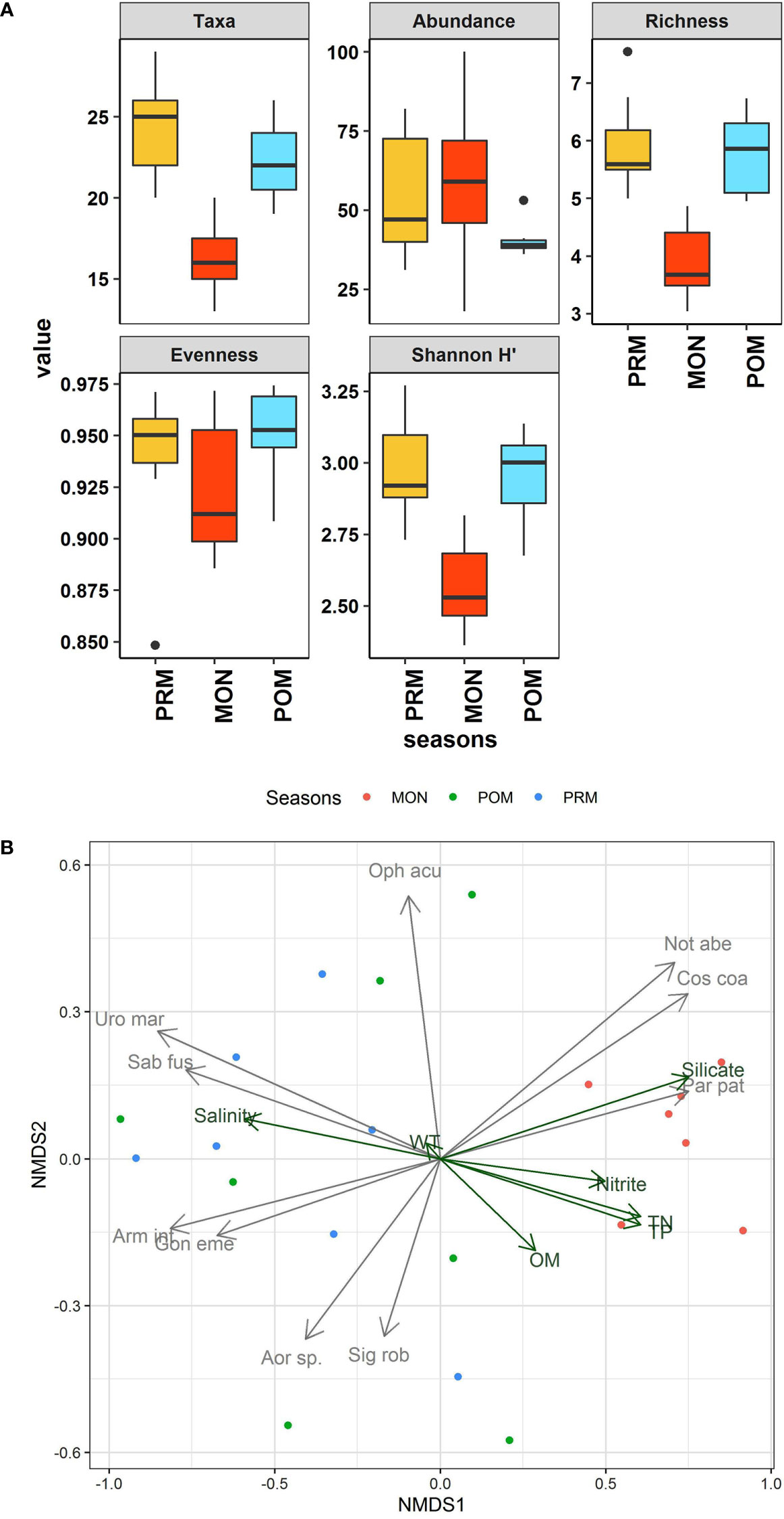
Figure 2 (A) Box plots showing the variations in community characteristics of macrobenthic fauna during different season. In each boxplot, the central line represents the median, the rectangle gives the interval between 25% and 75% percentiles, whiskers indicate the range and black dots represent outliers. Abundance is represented as ind/0.02m2. (B) NMDS ordination of the correlation between the macrofaunal community composition and the best subset of environmental variables and taxa with maximum correlation with community dissimilarities plotted as vectors. The vectors represented in green correspond to environmental variable whereas grey vectors represent taxa (abbreviation as per Table S4).
Conclusion
The environmental perturbation during the monsoon season in Diu Island brings significant changes in physicochemical and biological characteristics. The study revealed that nutrient and organic enrichment during the monsoon season were the main drivers of the community pattern observed along the Diu coast. These community drivers were stable and did not show significant variation during the post and pre-monsoon periods thereby justifying the healthy benthic environment. The influence of these variables was evidenced on the macrofaunal community during the monsoon which resulted in lower diversity and higher relative abundance of tolerant and opportunistic taxa which is consistent with the community pattern of other tropical islands. This could also be attributed to organic enrichment and nutrient influx resulting from land runoff and natural upwelling incidents on the west coast of India. The result will be significant for comparing benthic diversity and its impact on the ecosystem to other tropical coastal environments.
Data availability statement
The original contributions presented in the study are included in the article/Supplementary Material, further inquiries can be directed to the corresponding author/s.
Author contributions
VP: Conceptualization, sample collection, laboratory analysis, data processing, and writing-original draft. DJ: Sampling design, data validation, and manuscript review. KR: Sample collection and manuscript review. SV: Field investigation. PSK: Field investigation. PV: Data validation and review. GD: Reviewing the manuscript, suggestion, and project administration. All authors contributed to the article and approved the submitted version.
Acknowledgments
The authors are grateful for the support provided by the Earth System Sciences Organization (ESSO), Ministry of Earth Sciences (MoES), Govt. of India, in carrying out the present research study. We are thankful to Dr. G.A. Ramadass, Director, NIOT, Chennai, for his constant encouragement, guidance, and support in completing this research work. We are also thankful to all the supporting staff for their help during the sampling.
Conflict of interest
The authors declare that the research was conducted in the absence of any commercial or financial relationships that could be construed as a potential conflict of interest.
Publisher’s note
All claims expressed in this article are solely those of the authors and do not necessarily represent those of their affiliated organizations, or those of the publisher, the editors and the reviewers. Any product that may be evaluated in this article, or claim that may be made by its manufacturer, is not guaranteed or endorsed by the publisher.
Supplementary material
The Supplementary Material for this article can be found online at: https://www.frontiersin.org/articles/10.3389/fmars.2022.1006689/full#supplementary-material
References
Alongi D. (1990). The ecology of tropical soft-bottom benthic ecosystems. Oceanogr. Mar. Biol. an Annu. Rev. 28, 381–496.
Bolam S. G., Eggleton J., Smith R., Mason C., Vanstaen K., Rees H. L. (2008). Spatial distribution of macrofaunal assemblages along the English channel. J. Mar. Biol. Assoc. U. K. 88, 675–687. doi: 10.1017/S0025315408001276
Chandler G. T., Shipp M. R., Donelan T. L. (1997). Bioaccumulation, growth and larval settlement effects of sediment-associated polynuclear aromatic hydrocarbons on the estuarine polychaete, Streblospio benedicti (Webster). J. Exp. Mar. Biol. Ecol. 213, 95–110. doi: 10.1016/S0022-0981(97)00011-7
Cisneros K. O., Smit A. J., Laudie J., Schoeman D. S. (2011). Complex dynamic combination of physical, chemical and nutritional variables controls spatio-temporal variation of sandy beach community structure. PLosOne 6 (8), e23724. doi: 10.1371/journal.pone.0023724
Clarke K. R., Ainsworth M. (1993). A method of linking multivariate community structure to environmental variables. Mar. Ecol. Prog. Ser. 92, 205–219. doi: 10.3354/meps092205
Clarke K. R., Warwick R. M. (1998). Quantifying structural redundancy in ecological communities. Oecologia 113, 278–289. doi: 10.1007/s004420050379
Dheenan P. S., Jha D. K., Das A. K., Vinithkumar N. V., Prashanthi Devi M., Kirubagaran R. (2016). Geographic information systems and multivariate analysis to evaluate fecal bacterial pollution in coastal waters of Andaman, India. Environmental Pollution 214, 45–53. doi: 10.1016/j.envpol.2016.03.065
Dutertre M., Hamon D., Chevalier C., Ehrhold A. (2013). The use of the relationships between environmental factors and benthic macrofaunal distribution in the establishment of a baseline for coastal management. ICES (Int. Counc. Explor. Sea) J. Mar. Sci. 70 (2), 294–308. doi: 10.1093/icesjms/fss170
Ellis J. T., Ysebaert T., Hume A., Norkko T., Bult P., Herman S., et al. (2006). Predicting macrofaunal species distributions in estuarine gradients using logistic regression and classification systems. Mar. Ecol. Prog. Ser. 316, 69–83. doi: 10.3354/meps316069
Equbal J., Lakra R., Savurirajan M., Satyam K., Ganesh T. (2018). Testing performances of marine benthic biotic indices under the strong seasonality in the tropical intertidal habitats, south Andaman, India. Mar. pollut. Bull. 135, 266–282. doi: 10.1016/j.marpolbul.2018.07.034
Ganesh T., Rakhesh M., Raman A. V., Sateesh N., Moore S., Rajanna B. (2014). Macrobenthos response to sewage pollution in a tropical inshore area. Environ. Monit. Assess. 186, 3553–3566. doi: 10.1007/s10661-014-3638-4
Gaudette H. E., Wilson R. F., Toner L., Folger D. W. (1974). An inexpensive titration method for determination of organic carbon in recent sediments. J. Sediment. Petrol. 44, 249–253. doi: 10.1306/74D729D7-2B21-11D7-8648000102C1865D
Gesteira-Gomez J. L., Dauvin J. C., Salvande Fraga M. (2003). Taxonomic level for assessing oil spill effects on soft-bottom sublittoral benthic communities. Mar. Poll. Bull. 46, 562–572. doi: 10.1016/S0025-326X(03)00034-1
Grasshoff K., Kremling K., Ehrhardt M. (1999). Methods of Seawater Analysis, Third Ed (Weinheim, Germany: Verlag Chemie), 159–228.
Gray J. S., Wu R. S. S., Ying Y. O. (2002). Effects of hypoxia and organic enrichment on the coastal marine environment. Mar. Ecol. Prog. Ser. 238, 249–279. doi: 10.3354/meps238249
Gray J. S. (1997). Marine biodiversity: Patterns, threats and conservation needs. Biodiversity and Conservation 6, 153–175. doi: 10.1023/A:1018335901847
Harkantra S. N., Rodrigues N. R. (2003). Pattern of species succession of soft-bottom macrofauna in the estuaries of goa, west coast of India. Curr. Sci. 85 (10), 1458–1474.
Hewitt J.E., Thrush S.F. (2007). Effective Long-term Ecological Monitoring Using Spatially and Temporally Nested Sampling. Environ Monit Assess 133, 295–307. doi: 10.1007/s10661-006-9584-z
Hily C., Le Loc’h F., Grall J., Glemarec M. (2008). Soft bottom macrobenthic communities of north Biscay revisited: Long-term evolution under fisheries-climate forcing. Estuar. Coast. Shelf Sci. 78, 413–425. doi: 10.1016/j.ecss.2008.01.004
Holmer M., Forbes V. E., Forbes T. L. (1997). Impact of the polychaete capitella sp. on microbial activity in organic-rich marine sediment contaminated with the polycyclic aromatic hydrocarbon fluoranthene. Mar. Biol. 128, 679–688. doi: 10.1007/s002270050135
Jha D. K., Dharani G., Verma P., Ratnam K., Sendhil Kumar R., Rajaguru S. (2021). Evaluation of factors influencing the trace metals in puducherry and diu coasts of India through multivariate techniques. Mar. pollut. Bull. 167, 112342. doi: 10.1016/j.marpolbul.2021.112342
Jha D. K., Rajaprabhu G., Kirubagaran R., Sendhil Kumar R., Dharani G., Das A. K., et al. (2017). Estimation of potential zones for offshore mariculture in the Indian Sea using geographical information system as a management tool. J. Coast. Conserv. 21, 893–902. doi: 10.1007/s11852-017-0556-y
Jha D. K., Vinithkumar N. V., Sahu B. K., Dheenan P. S., Das A. K., Mehmuna B., et al. (2015). Multivariate and geo-spatial approach for seawater quality of chidiyatappu bay, south Andaman islands, India. Marine Pollution Bulletin 96, 1–2, 463–470. doi: 10.1016/j.marpolbul.2015.05.004
Jha D. K., PrashanthiDevi M., Vinithkumar N.V., Das A.K., Kumar A., Dheenan P. S., et al. (2013). Comparative investigation of water quality parameters of aerial & rangat bay, Andaman islands using In-situ measurements and spatial modelling techniques. Water Qual. Expo. Health 5, 57–67. doi: 10.1007/s12403-013-0086-2
Kumar P. S., Venkatnarayanan S., Pandey V., Ratnam K., Jha D. K., Rajaguru S., et al. (2022). Multivariate approach to evaluate the factors controlling the phytoplankton abundance and diversity along the coastal waters of diu, northeastern Arabian Sea. Oceanologia 64, 2, 267–275. doi: 10.1016/j.oceano.2021.11.005
Kumar T. S., Vijayakumaran M., Murugan T. S., Jha D. K., Sreeraj G., S. M. (2009). Captive breeding and larval development of the scyllarine lobster petrarctusrugosus. New Z. J. Mar. Freshw. Res. 43:1, 101–112. doi: 10.1080/00288330909509985
Levin L., Caswell H., Bridges T., Di Bacco C., Cabrera D., Plaia G. (1996). Demographic responses of estuarine polychaetes to pollutants: Life table response experiments. Ecol. Appl. 6, 1295–1313. doi: 10.2307/2269608
Liu C.W., Lin K.H0., Kuo Y.M.. (2003). Application of factor analysis in the assessment of groundwater quality in a blackfoot disease area in Taiwan. Sci. Total Environ. 313, 77–89. doi: 10.1016/S0048-9697(02)00683-6
Murugan T. S., Remany M. C., Mary Leema T., Jha D. K., Santhanakumar J., Vijayakumaran M., et al. (2005). Growth, repetitive breeding, and aquaculture potential of the spiny lobster, panulirus ornatus. New Z. J. Mar. Freshw. 39:2, 311–315. doi: 10.1080/00288330.2005.9517311
Oksanen J., Blanchet F. G., Friendly M., Kindt R., Legendre P., McGlinn D., et al. (2020). Vegan: Community ecology package. R Package version 2, 5–7.
Pandey V., Ganesh T. (2019a). Spatial and temporal variability in the vertical distribution of gastropods on the rocky shores along the east coast of south Andaman island, India. Mar. Biodivers. 49, 633–645. doi: 10.1007/s12526-017-0838-5
Pandey V., Ganesh T. (2019b). Spatial and temporal variability of sandy intertidal macrobenthic communities and their relationship with environmental factors in a tropical island. Estuar. Coast. Shelf Sci. 224, 73–83. doi: 10.1016/j.ecss.2019.04.045
Pandey V., Jha D. K., Sathish Kumar P., Santhanakumar J., Venkatnaraynan S., Prince Prakash Jebakumar J., et al. (2022). Effect of multiple stressors on the functional traits of sub-tidal macrobenthic fauna: a case study of the southeast coast of India. Mar. pollut. Bull. 175, 113355. doi: 10.1016/j.marpolbul.2022.113355
Pandey V., Venkatnarayanan S., Kumar P. S., Ratnam K., Jha D. K., Rajaguru S., et al. (2021). Assessment of ecological health of swarnamukhi river estuary, southeast coast of India, through AMBI indices and multivariate tools. Mar. pollut. Bull. 164, 112031 doi: 10.1016/j.marpolbul.2021.112031
Parulekar A. H., Dhargalkar V. K., Singbal S. Y. S. (1980). Benthic studies in goa estuaries: Part III- annual cycle of macrofaunal distribution, production and trophic relations. Indian J. Mar. Sci. 9, 189–200.
Pearson T. H., Rosenberg R. (1987). “Feast and famine: Structuring factors in marine benthic communities,” in Organization of communities past and present. Eds. Gee J. H. R., Wiley-Blackwell P.S.G. (Oxford), 373–395.
Ratnam K., Jha D. K., Prashanthi Devi M., Dharani G. (2022). Evaluation of physicochemical characteristics of coastal waters of nellore, southeast coast of India, by a multivariate statistical approach. Front. Mar. Sci. 9. doi: 10.3389/fmars.2022.857957
R Core Team (2020). R: A language and environment for statistical computing. RFoundation for Statistical Computing, Vienna, Austria. Available at: https://www.R-project.org/.
Rehitha T. V., Madhu N. V., Vineetha G., Vipindas P. V., Resmi P., Revichandran C. (2019). Spatio-temporal variability in macrobenthic communities and trophic structure of a tropical estuary and its adjacent coastal waters. Environ. Monit. Assess. 191, 341. doi: 10.1007/s10661-019-7460-x
Ryu J., Khim J.S., Kang S.G., Kang D., Lee C., Koh C. (2011). The impact of heavy metal pollution gradients in sediments on benthic macrofauna at population and community levels. Environ. Pollut. 159 (10), 2622–2629. doi: 10.1016/j.envpol.2011.05.034
Shanks A. L., Grantham B. A., Carr M. H. (2003). Propagule dispersal distance and the size and spacing of marine reserves. Ecol. Appl. 13, S159–S169. doi: 10.1890/1051-0761(2003)013[0159:PDDATS]2.0.CO;2
Shumchenia E. J., King J. W. (2010). Comparison of methods for integrating biological and physical data for marine habitat mapping and classification. Cont. Shelf Res. 30, 1717–1729. doi: 10.1016/j.csr.2010.07.007
Sivadas S. K., Ingole B., Ganesan P., Sautya S., Nanajkar M. (2012). Role of environmental heterogeneity in structuring the macrobenthic community in a tropical sandy beach, west coast of India. J. Oceanogr 68, 295–305. doi: 10.1007/s10872-011-0099-z
Sivadas S., Ingole B., Nanajkar M. (2011). Temporal variability of macrofauna from a disturbed habitat of zuari estuary, west coast of India. Environ. Monit. Assess. 173, 65–78. doi: 10.1007/s10661-010-1371-1
Snelgrove P. V. R. (1998). The biodiversity of macrofaunal organisms in marine sediments. Biodiver. Conserv. 7, 1123–1132. doi: 10.1023/A:1008867313340
Snelgrove P. V. R., Butman C. A. (1994). Animal-sediment relationships revisited: cause versus effect. Oceanogr. Mar. Biol. Annu. Rev. 32, 111–117.
Van Hoey G., Degraer S., Vinx M. (2004). Macrobenthic community structure of soft-bottom sediments at the Belgian continental shelf. estuarine. Coast. Shelf Sci. 59, 599–613. doi: 10.1016/j.ecss.2003.11.005
Wickham H., Averick M., Bryan J., Chang W., McGowan L.D., François R., Grolemund G., Hayes A., et al (2019). Welcome to the tidyverse. J. Open Source Softw. 4 (43), 1686. doi: 10.21105/joss.01686.
Winberg P. C., Lynch T. P., Murray A., Jones A. R., Davis A. R. (2007). The importance of spatial scale for the conservation of tidal flat macrobenthos: An example from new south Wales, Australia. Biol. Conserv. 134, 310–320. doi: 10.1016/j.biocon.2006.07.019
WoRMS Editorial Board (2021) World register of marine species. Available at: https://www.marinespecies.org.
Keywords: macrofauna, temporal variation, Diu Island, nutrients, environmental relationship
Citation: Pandey V, Jha DK, Ratnam K, Venkatnarayanan S, Sathish Kumar P, Verma P and Dharani G (2022) Seasonal variability and its impact on the sub-tidal macrobenthic fauna of Diu Island, north-eastern Arabian Sea: A multivariate approach. Front. Mar. Sci. 9:1006689. doi: 10.3389/fmars.2022.1006689
Received: 29 July 2022; Accepted: 17 October 2022;
Published: 15 November 2022.
Edited by:
Pablo Muniz, Universidad de la República, UruguayReviewed by:
Rajamanickam Krishnamurthy, Arignar Anna Government Arts and Science College Chennai, IndiaThilagam Harikrishnan, Pachaiyappa’s College for Men, India
Helio Checon, State University of Campinas, Brazil
Copyright © 2022 Pandey, Jha, Ratnam, Venkatnarayanan, Sathish Kumar, Verma and Dharani. This is an open-access article distributed under the terms of the Creative Commons Attribution License (CC BY). The use, distribution or reproduction in other forums is permitted, provided the original author(s) and the copyright owner(s) are credited and that the original publication in this journal is cited, in accordance with accepted academic practice. No use, distribution or reproduction is permitted which does not comply with these terms.
*Correspondence: Vikas Pandey, vicky2555@gmail.com; Gopal Dharani, dhara@niot.res.in