- 1Department of Ecology and Coastal Management, Institute of Marine Sciences of Andalusia (ICMAN), Spanish National Research Council (CSIC), Cadiz, Spain
- 2Institute of Marine Sciences (ISMAR), Italian National Research Council (CNR), Rome, Italy
- 3Institut des Substances et Organismes de la Mer (ISOMer), Nantes University, Nantes, France
Rugulopteryx okamurae is a species of brown macroalgae belonging to the Dictyotaceae family and native to the north-western Pacific. As an Invasive Alien Species (IAS), it was first detected in the Strait of Gibraltar in 2015. Since then, R. okamurae has been spreading rapidly through the submerged euphotic zone, colonizing from 0 to 50 m depth and generating substantial economic and environmental impacts on the Andalusian coasts (southern Spain). More than 40% of marine IAS in the European Union (EU) are macroalgae, representing one of the main threats to biodiversity and ecosystem functioning in coastal habitats. This study presents a monitoring pilot of beached R. okamurae and fresh R. okamurae down to 5 m depth in Tarifa (Cadiz, Spain), combining multispectral remote sensing data collected by sensors on-board Unmanned Aerial Vehicles (UAVs) and satellites, and how this information can be used to support decision-making and policy. We used an UAV flight carried out at Bolonia beach (Tarifa, Spain) on 1st July 2021 and Sentinel-2 (S2) and Landsat-8 (L8) image acquisitions close to the drone flight date. In situ data were also measured on the same date of the flight, and they were used to train the supervised classification Super Vector Machine (SVM) method based on the spectral information obtained for each substrate cover. The results obtained show how multispectral images allow the detection of beached R. okamurae, and the classification accuracy for water, land vegetation, sand and R. okamurae depending on the image resolution (8.3 cm/pixel for UAV flight, 10 m/pixel for S2 and 30 m/pixel for L8). While the UAV imagery precisely delimited the area occupied by this macroalgae, satellite data were capable of detecting its presence, and able to generate early warnings. This study demonstrates the usefulness of multispectral remote sensing techniques to be incorporated in continuous monitoring programmes of the marine IAS R. okamurae in coastal areas. This information is also key to supporting regional, national and European policies in order to adapt strategic management of invasive marine macrophytes.
1 Introduction
Macroalgae play a key role in the ecology of rocky bottoms as primary producers and habitat providers for a myriad of species (Thornber et al., 2017). However, seaweed invasions are considered among the major threats to biodiversity and good ecosystem functioning in coastal habitats, since they represent more than 40% of marine Invasive Alien Species (IAS) in the EU (Schaffelke et al., 2006; Williams and Smith, 2007). These marine IAS are frequently introduced through international maritime shipping (Katsanevakis et al., 2014; Ojaveer et al., 2014; Gallardo et al., 2019), and display invasive behaviour resulting in degradation of local marine biodiversity and negative impacts on environmental health and coastal ecosystem services, i.e. habitat fragmentation (Faria et al., 2022). The Strait of Gibraltar supports the highest density of maritime traffic in the Western Mediterranean (Abdulla and Linden, 2008; Endrina et al., 2018), where both ballast waters and boat hull fouling are recognized as potential transport vectors for IAS (Ribera, 2003).
Rugulopteryx okamurae (E.Y. Dawson) I.K. Hwang, W.J. Lee & H. S. Kim is currently threatening the rocky bottoms of the Andalusian coast in the Strait of Gibraltar. It is a brown seaweed belonging to the Dictyotaceae family and native to the temperate-subtropical northwestern Pacific (Figure 1). This species was first recorded as an IAS in the Mediterranean open sea in the Strait of Gibraltar (Ceuta, Spanish peninsula and Morocco) in 2015 as unusually abundant littoral wrack deposits (Altamirano-Jeschke et al., 2016; Ocaña et al., 2016), where it quickly became invasive. R. okamurae is also very similar to other native species of the genus Dictyota; therefore it is likely to have been present in southern Spanish waters previously without being detected (Altamirano-Jeschke et al., 2016). The species was first recorded in 2002, not in the open sea but in the Thau coastal lagoon (Occitania, France), having been introduced there through the oyster aquaculture activity (Verlaque et al., 2009). Since then, the species has continued spreading rapidly throughout the coastal areas of the Strait of Gibraltar, also reaching the Azores Islands (Faria et al., 2022), and colonizing a wide range of habitats from 0 to 50 m depth (Altamirano-Jeschke et al., 2017; El Aamri et al., 2018; García-Gómez et al., 2018; García-Gómez et al., 2020). Its rapid spread since its first record in 2015 ranks R. okamurae among the most invasive IAS in the Mediterranean Sea (García-Gómez et al., 2020; García-Gómez et al., 2021; Sempere-Valverde et al., 2021).
According to Altamirano et al. (2019) and Muñoz et al. (2019), the environmental favorability model for R. okamurae points towards the Mediterranean Sea and the Atlantic coasts as potentially threatened, being areas with higher settlement success of invaders (De Villèle and Verlaque, 1995), whose effects have already been demonstrated. R. okamurae is causing important ecological impacts such as loss of biodiversity, alteration of marine habitat and displacement of native species, even in Natura 2000 network sites, with over 85% coverage from 10 to 30 m depth on rocky seafloors (García-Gómez et al., 2021). Previous studies have observed how this invasive seaweed competes with most shallow native communities and grows by epibiosis on coralligenuos species of ecological importance in the Mediterranean Sea, already in decline due to other anthropogenic impacts and the effects of climate change (Linares et al., 2007; Garrabou et al., 2009; Lejeusne et al., 2010). Moreover, beyond the ecological consequences, R. okamurae is causing an important economic impact with losses in the aquaculture and fishery sector, clogging and deteriorating fishing gear, but also in the tourism sector, where the municipalities have to manage large amounts of dead R. okamurae biomass on their coasts throughout the summer season (Figure 2). The high costs derived from the management of the biomass that reaches the beaches are carried by each municipality.
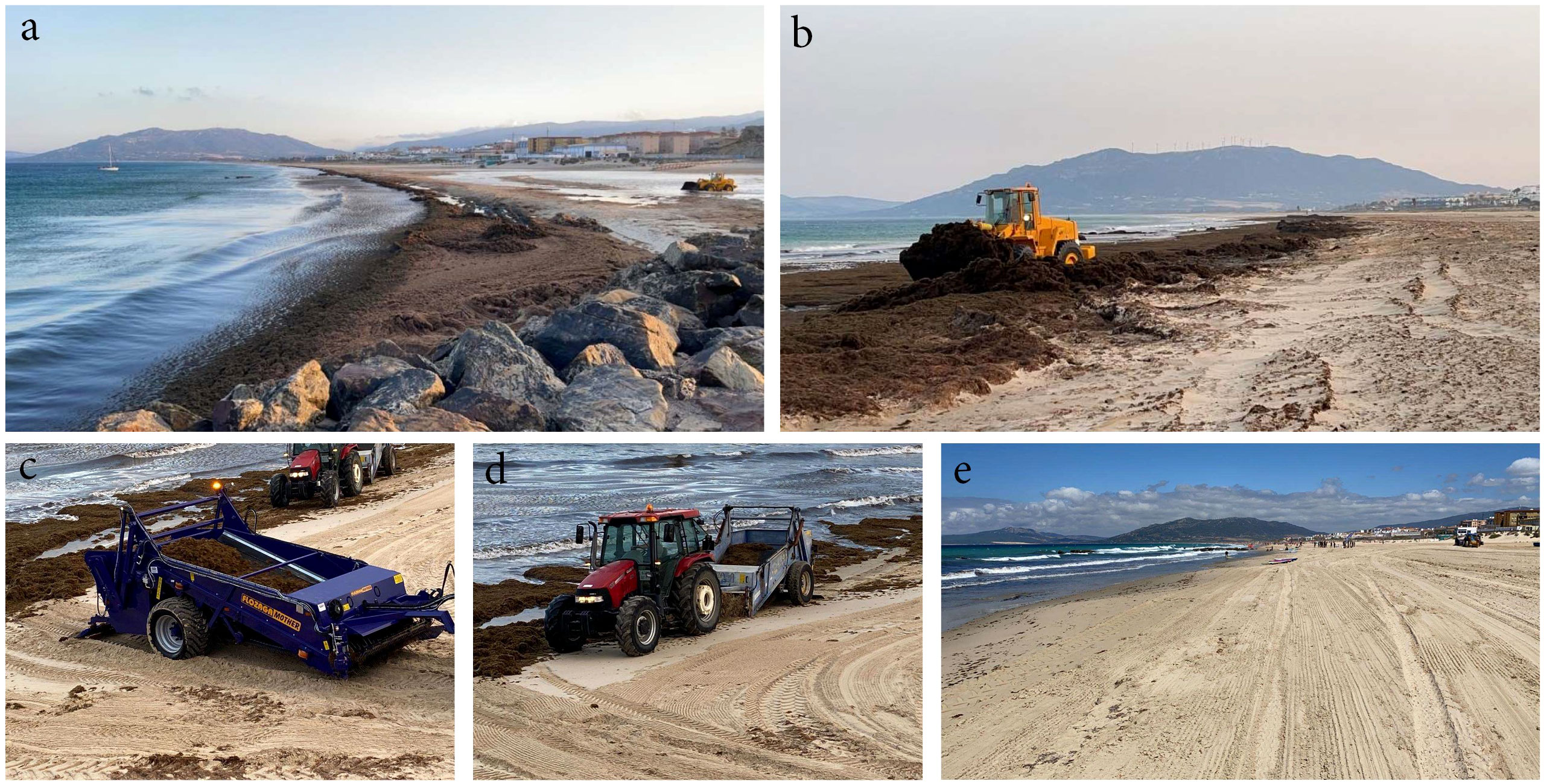
Figure 2 Mechanical removal of R. okamurae beachings on Los Lances and Atlanterra beaches (Tarifa, Spain) during the 2021 summer (A-D) R. okamurae beaching and its removal with heavy machinery (6.213 tons in only two months); (E) the same beach after seaweed removal. Photos and data provided by the City Council of Tarifa.
To date, according to Giakoumi et al. (2019), management strategies have been largely established for terrestrial invasive taxa and less for marine invasions, where monitoring information is key. In 2015, the European Union (EU) launched the Regulation 1143/2014 for IAS (Union, E.P.a.C.o.t.E, 2014), with a set of measures to be taken for regulating IAS at EU Member State level, including the “List of Invasive Alien Species of Union concern”. Only the species included in the EU list are subject to restrictions and measures set out in the IAS Regulation. In this regard, both the European Commission and individual Member States can propose additional species to be included in this list, according to Article 4(4) of the Regulation, by providing a risk assessment of the proposed species. In 2007, the Spanish Law 42/2007 (España, 2007) on Natural Heritage and Biodiversity came into force on 14th December (BOE 299 de 14/12/2007), which includes, similar to the EU list, the Spanish Catalog of Invasive Alien Species at national level, where new IAS can be proposed for their inclusion. R. okamurae is already included in the Spanish IAS list and has been proposed to be included in the EU list. However, there is no established monitoring protocol, and long-term monitoring is essential in the characterization and evaluation of IAS derived changes (Lodge et al., 2006; García Gómez, 2015).
In this regard, new remote sensing techniques can be very useful in the continuous monitoring of IAS thanks to the high and very high resolution imagery from satellites such as Sentinel-2 (S2) and Landsat-8 (L8), and Unmanned Aerial Vehicles (UAVs), respectively. However, marine species monitoring is always challenging, in particular at depths where the downwelling irradiance is largely attenuated by seawater colored constituents, with water molecules being the main absorbing component beyond 700 nm (Oppelt et al., 2012; Tait et al., 2019). Because R. okamurae is distributed down to 50 meters depth, the main monitoring technique is scuba diving (García-Gómez et al., 2021), which is costly to perform and lacks information on species spatial distribution (Beca-Carretero et al., 2020; Veettil et al., 2020). Remote sensing techniques can serve as an added-value to in situ sampling on a broader scale, generating synoptic, cost-effective and continuous distribution information of R. okamurae at depths where it is still visible from the surface (Beca-Carretero et al., 2020; Kaufman and Bell, 2020). These are cost-effective techniques that can generate key transferable information in the optically shallow waters and the seaweed beached on dry beaches with a higher revisit time.
Multispectral imaging sensors onboard satellites or UAVs can provide very useful information to monitor macrophytes (Román et al., 2021; Diruit et al., 2022). On the one hand, S2 is a constellation of two twin satellites (Sentinel-2A and Sentinel-2B) of the European Union's Copernicus programme in the European Space Agency (ESA). The MultiSpectral Instruments (MSI) onboard both S2 satellites (S2/MSI) are operational with a global 5-day revisit frequency at the equator. The MSI samples 13 spectral bands: four bands at 10 m, six bands at 20 m and three bands at 60 m spatial resolution. On the other hand, the Operational Land Imager (OLI) onboard the L8 satellite (L8/OLI) of the USGS-NASA acquires images of the Earth’s surface in the visible, Near Infra-Red (NIR), short wave, and thermal infrared regions with a 16-day revisit frequency and 30-meter spatial resolution (Knight and Kvaran, 2014).
Although Red-Green-Blue (RGB) composite satellite imagery has been used for monitoring specific marine vegetation (Hedley et al., 2017), there are some limitations to assessing patchy habitats of macroalgae due to the spectral resolution (Knudby and Nordlund, 2011). The use of multispectral sensors provides a notably improved ability in spectral signature distinction compared to RGB sensors, offering higher spectral resolution to detect specific organisms through the isolation of key parts of the electromagnetic spectrum from non-overlapping bands (Tait et al., 2019). Moreover, the infrared band is commonly used for exposed marine vegetation detection through remote sensing, with many studies using the Normalized Difference Vegetation Index (NDVI) for monitoring seagrass meadows, macroalgae and microphytobenthos, among others (Barillé et al., 2010; Brito et al., 2013; Kohlus et al., 2020; Méléder et al., 2020; Zoffoli et al., 2020; Román et al., 2021; Zoffoli et al., 2021).
Therefore, the overall aim of this study was to determine potential applications and limitations of multispectral remote sensing onboard UAV and satellites in order to detect and monitor R. okamurae on the Andalusian coasts in the optically shallow waters and beachings. Here, we propose a synoptic tool for monitoring R. okamurae, resulting in useful information for decision-makers and relevant administrations in order to manage this invasive species.
2 Materials and methods
2.1 In situ measurements
The present study is focused on the Atlantic coast of the Strait of Gibraltar. Concretely, on Bolonia beach, located in the municipality of Tarifa (Spain). The Area of Interest (AOI) was delimited by the drone flight, between the beginning of the rocky beach to the south (36° 4’ 15.43’’N, 5° 44’ 55.82’’W) and the Churriana stream to the north (36° 4’ 37.15’’N, 5° 45’ 27.7’’W) (Figure 3). In this area, beached R. okamurae was monitored on 1st of July 2021 for a 1.1 km strip of coastline, including in situ sampling, hyperspectral field radiometry and a UAV flight with multispectral sensor.
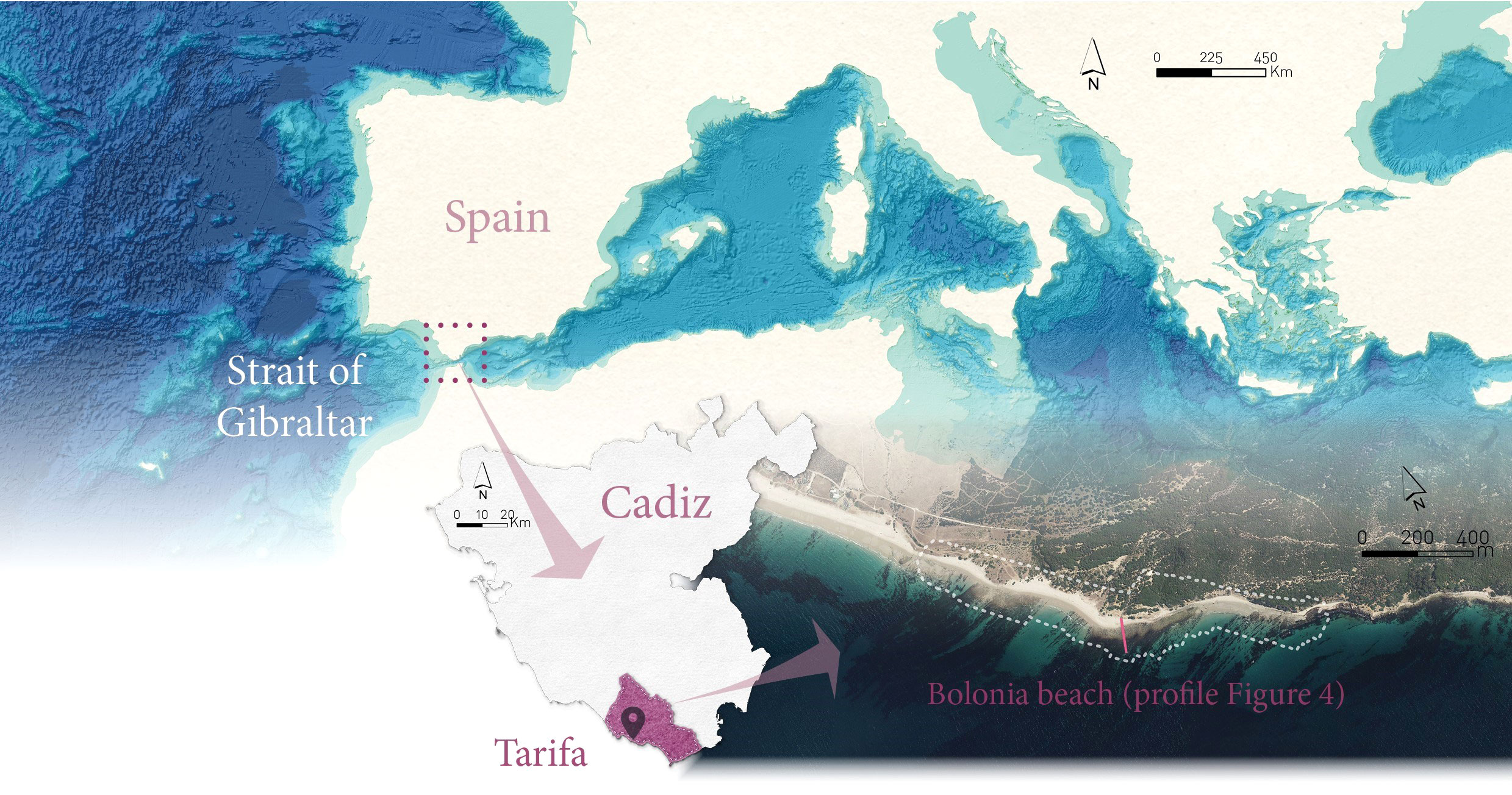
Figure 3 Study area on Bolonia beach (Tarifa, Spain), located on the Atlantic side of the Strait of Gibraltar.
On the day of the flight, field reflectance (Rinsitu) data were acquired with a hyperspectral radiometer (ASD HandHeld2) over eight different stages of R. okamurae across the beach, from nearshore subtidal waters (depth < 6 m) to wrack deposits along the dry beach. The reflectance of sand samples was also acquired for subsequent image classification and UAV calibration (Figure 4). The R. okamurae samples are described in Table 1 for the different zones (S01: 1 m depth; S02: 2 m depth; S03: in-water floating algae; S04: intertidal area; S05: 5 m depth; S06: dry macroalgae on the dry beach; and S07: sand), taking 10-20 measures for each sample, and the result being the mean of all values in each sample. Underwater samples of R. okamurae (i.e., S01 – 03, and S05) were collected and brought to land for immediate reflectance measurements onshore. Prior to the measurement, other co-occurring macroalgae species were carefully removed from the samples. R. okamurae samples from S01, S02 and S05 were highly colonized by ephiphytes which were not removed. For the other samples, the reflectance was directly measured in situ.
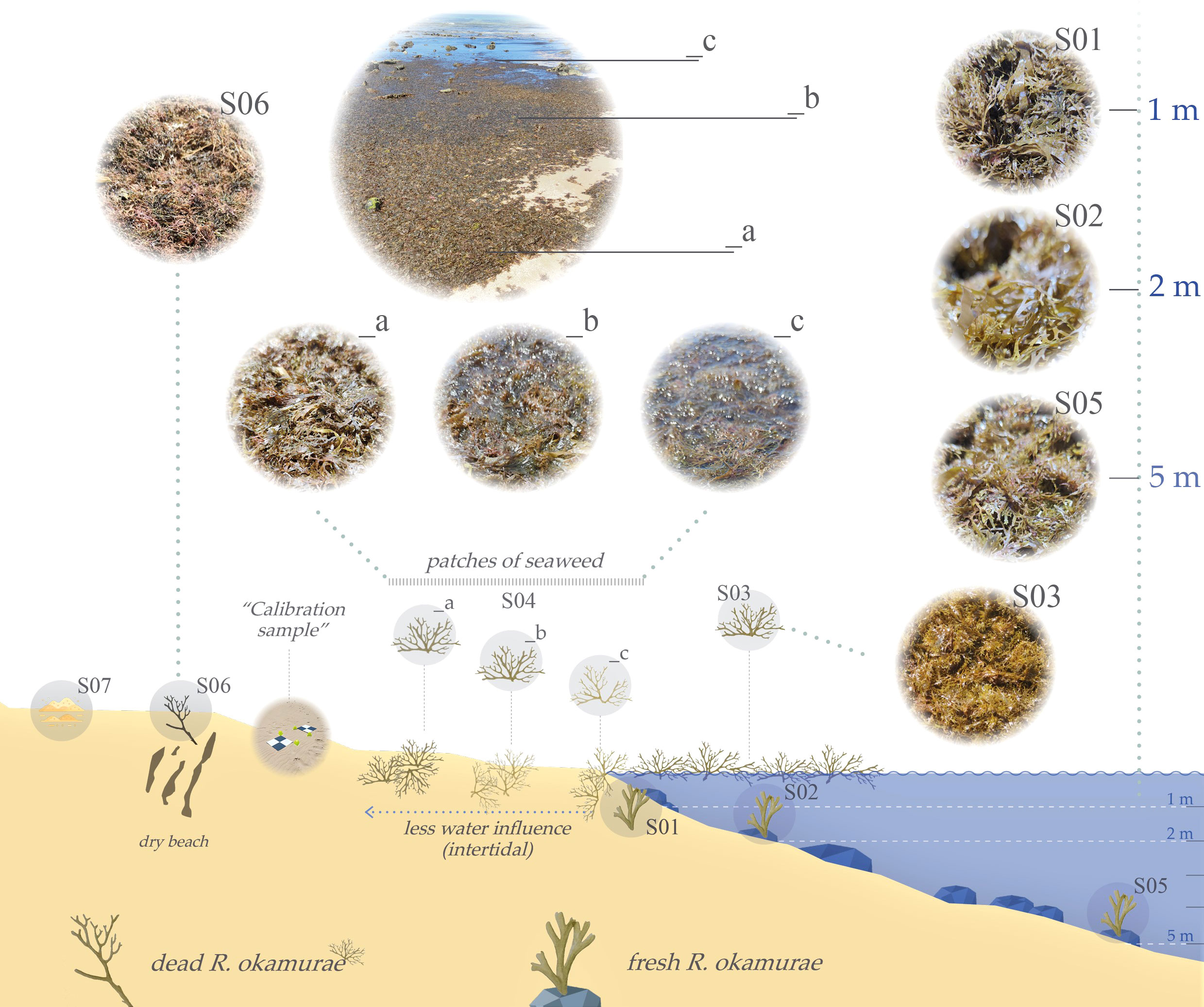
Figure 4 Diagramme of collected samples measured with the hyperspectral field radiometer on Bolonia beach (Tarifa, Spain) on 1st of July 2021. Fresh R. okamurae was collected between 1 and 5 m depth; dead R. okamurae was collected in different stages across the beach profile. Codes are described in Table 1.
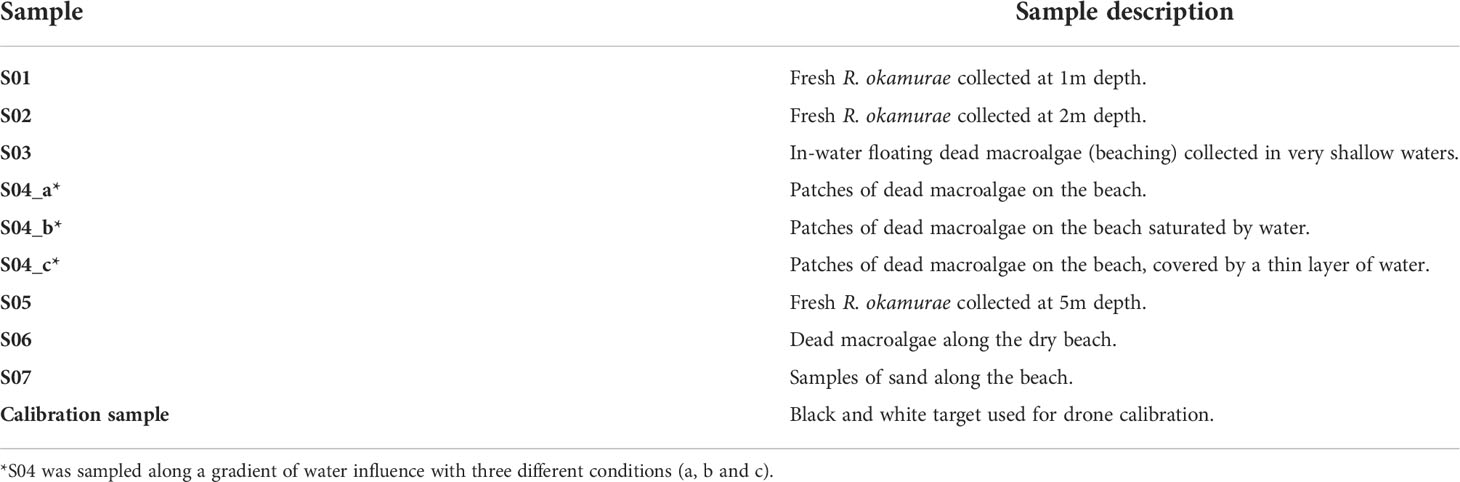
Table 1 Types of samples for Rugulopteryx okamurae collected in Tarifa on 1st July 2021 for in situ spectrum.
2.2 Remote sensing data and processing
2.2.1 Unmanned aerial vehicle
The UAV used in this study was a hexacopter with three-bladed propellers (Condor, Dronetools ©), which has a DJI6010 electric motor powered by 4 Li-ion batteries (7000 mA each). The empty weight of the equipment including the four batteries is 11.8 kg (with a maximum takeoff weight (MTOW) of 14.9 kg), and a maximum flight autonomy of up to 60 minutes (without payload). The Micasense RedEdge-MX multispectral dual sensor, onboard the UAV in this study, is capable of capturing spectral information in 10 spectral bands: blue (444 nm and 475 nm), green (531 nm and 560 nm), red (650nm and 668nm), red edge (705nm, 717nm and 740nm) and near infrared (840nm) wavelengths. The sensor resolution is 1.2 megapixels for each of the multispectral bands. In addition, it allows a broad surface coverage since it has a horizontal field of view of 47.2°, and a ground sampling distance (GSD) of 8 cm/pixel from 120 m above ground level (AGL). Standard format metadata tags are embedded for each image since the sensor has its own positioning system. It also has a Downwelling Light Sensor (DLS) with built-in GPS and Inertial Measurement Unit (IMU), which provides more accurate and reliable measurements of irradiance and solar angle during in-flight image acquisition. A reference panel (RP04-1924106-0B) was used for radiometric calibration. By using both the DLS and the calibration panel during the data collection, it is possible to obtain a high-quality reflectance map after image processing.
The UAV flight was performed on 1st July 2021 on Bolonia beach (Cadiz) during sunny and windy meteorological conditions, at a constant flight height of 120 m, acquiring an image with approximately 8.3 cm/pixel. Flight planning was carried out with the DJI Ground Station Pro (GSP) software and subsequently uploaded to the UAV computer system. The flight plan considered front and side overlap of 80%. For the radiometric correction of the images, individual photos of the calibration panel were captured for each waveband before the flight in the absence of shadow covering the panel.
The software Pix4D mapper (Pix4D SA, Lausanne, Switzerland) was used to process the multispectral images for the generation of the orthomosaics following a Structure-from-Motion (SfM) photogrammetric process. The software creates reflectance data orthomosaic (RUAV) values by following three recognized steps: i) initial processing (image alignment); ii) the creation of a 3D point cloud; and iii) the generation of the digital surface model (DSM), orthomosaic and indices. The resulted orthomosaics were then projected to WGS 84/ETRS89 UTM zone 29N (EPSG: 25829). Light sensor data calibration was not necessary in this case, due to sunny and clear weather conditions. In the final processing step, the radiometric processing and calibration were performed using the method “Camera and Sun Irradiance”. As a result, the generated Rrs orthomosaic (8.3 cm/pixel spatial resolution) for each waveband achieved a horizontally and vertically absolute accuracy of 16 cm/pixel and 24 cm/pixel, respectively, for georeferencing.
2.2.2 Sentinel-2
S2 Level-2A surface reflectance (RS2, adimensional) corresponding to 30th June 2021 (tile 29SQA) was downloaded from the Copernicus Open Access Hub (https://scihub.copernicus.eu/). The Level-2A processing includes an atmospheric correction (Sen2Cor) using the Sen2Cor processor algorithm (Main-Knorn et al., 2017). All of the S2 bands were resampled to 10 m spatial resolution using the SNAP software.
2.2.3 Landsat-8
A L8 Level-2 image acquired on the 28th June 2021 (path 202 and row 035) was downloaded from Earth Explorer (https://earthexplorer.usgs.gov/), which was already orthorectified and atmospherically corrected using the Land Surface Reflectance Code (LaSRC) algorithm (Version 1.5.0). Data were acquired as surface reflectance (RL8, adimensional), the fraction of incoming solar radiation that is reflected from the Earth’s surface to the Landsat sensor. The LaSRC surface reflectance algorithm corrects for the spatially and spectrally varying scattering and absorbing effects of atmospheric gases, aerosols, and water vapor, which is necessary to reliably characterize the Earth’s land surface.
2.3 Image classification
Image classification was performed using the “Support Vector Machine” (SVM) supervised classification technique. The SVM algorithm was chosen because it has been proved that non-parametric algorithms for thematic classification offered the best performance when using a small number of training samples, as suggested by (Foody and Mathur, 2004; Mountrakis et al., 2011; Rupasinghe et al., 2019; Román et al., 2022). The SVM is a machine learning algorithm able to handle data with unknown statistical distribution, using a variety of training sets created from spectral data and validated with in situ information (Vapnik, 1999). This approach applies Kernel functions that map the training data into a larger-dimensional space in which classes can be linearly separated by a hyperplane using Geographic Information System (GIS). The SVM was computed in SAGA GIS software (Conrad et al., 2015), classifying each remote sensing image into four clusters using the spectral information of each pixel: sand, water, R. okamurae, and terrestrial vegetation. In this study, the radial base function was used as the transformation nucleus (Miranda et al., 2020), applying the classification parameters described in Table 2. Using SAGA GIS, manually trained AOIs were drawn for each spectral class, up to a total of 25 AOIs with an area of 1 m2 maximum. The supervised classification and performance assessment (see below) were carried out in QGIS [QGIS Development Team (2011)]. Using this sieving process made it possible to clean the classification of all individual pixels from misclassification error, as well as to improve the graphical representation.
The performance and accuracy of the classification algorithm was assessed using standard metrics in QGIS. Error matrices were drawn up, and statistical parameters such as the Cohen’s Kappa and Overall Accuracy coefficients were computed in QGIS using the Supervised Classification Plugin (SCP) postprocessing tool, following the method proposed by Olofsson et al. (2014). This method generates a stratified random sampling designed by comparing the thematic classification, considering 1416 pixels for UAV and 1280 pixels for each S2 and L8 image for the supervised classification and accuracy test. The classification was considered accurate when the Overall Accuracy was >80%. The Cohen’s Kappa coefficient returns results between -1 and +1, with +1 corresponding to highest agreement. For the present study, a Kappa coefficient >0.75 was considered as an accurate deviation of all pixels. Each spectral class was computed for each remote sensing sensor, and inter-compared. Finally, the surface area of each class was computed, considering R. okamurae in two classes: beached and in-water.
2.4 Proxy of R. okamurae biomass
For pixels classified as exposed R. okamurae, the Normalized Difference Vegetation Index (NDVI) was computed using QGIS as a proxy of macrophyte biomass. The NDVI was computed as:
were λNIR and λred correspond to the NIR and red spectral bands of each sensor, with respectively 840 and 668 nm for the Micasense sensor onboard the UAV, 842 and 665 nm for MSI at 10 m spatial resolution, and 865 and 655 nm for OLI.
The NDVI was used to check the consistency of the SVM classification, as vegetated pixels have a typically higher NDVI than pixels corresponding to water or to soil areas.
3 Results
3.1 Spectral signatures for R. okamurae
All Rinsitu samples of R. okamurae showed an overall similar spectral shape, with a red-edge feature typical of macrophytes. Most reflectance spectra were characterized by low values in the visible, a rapid increase between the red and NIR spectral regions, and a NIR maximum. In the visible, two local peaks around 600 and 650 nm were noticeable (Figure 5). The Rinsitu of the fresh, in-water R. okamurae samples collected at 2 and 5 m was very similar. The fresh sample collected at 1 m showed a similar shape but with a lower magnitude in the NIR region. Regarding the dead samples of R. okamurae, their reflectance was similar in the visible, but large differences in magnitude were observed due to the variation of water content. Due to an increase in water content, the influence of water absorption gradually increased from sample S04_a to S04_c, resulting in a progressive decrease of Rinsitu in the NIR. The reflectance of the dry sample of R. okamurae (S06) was different from all other samples: it showed a higher reflectance in the visible, the peaks around 600 and 650 nm were hardly noticeable, and it displayed a linear increase after 700 nm instead of a NIR plateau. The differences between the spectral signature of the submerged vs. dry R. okamurae have to be taken into account within the classification of different macroalgae in the intertidal zone.
In situ reflectance was compared with reflectance measured by the other sensors (Micasense, MSI and OLI) in order to evaluate their limitation for the detection of R. okamurae. Rinsitu was closer to RUAV than to RL8 and RS2, both for dry and submerged samples (Figure 6). Overall, satellite data showed an overestimation in reflectance values in the visible compared with in situ and UAV measurements, in both types of targets. In the latter type of analysis, the differences with the satellite data are greater than when analyzing the spectral response of beached R. okamurae.
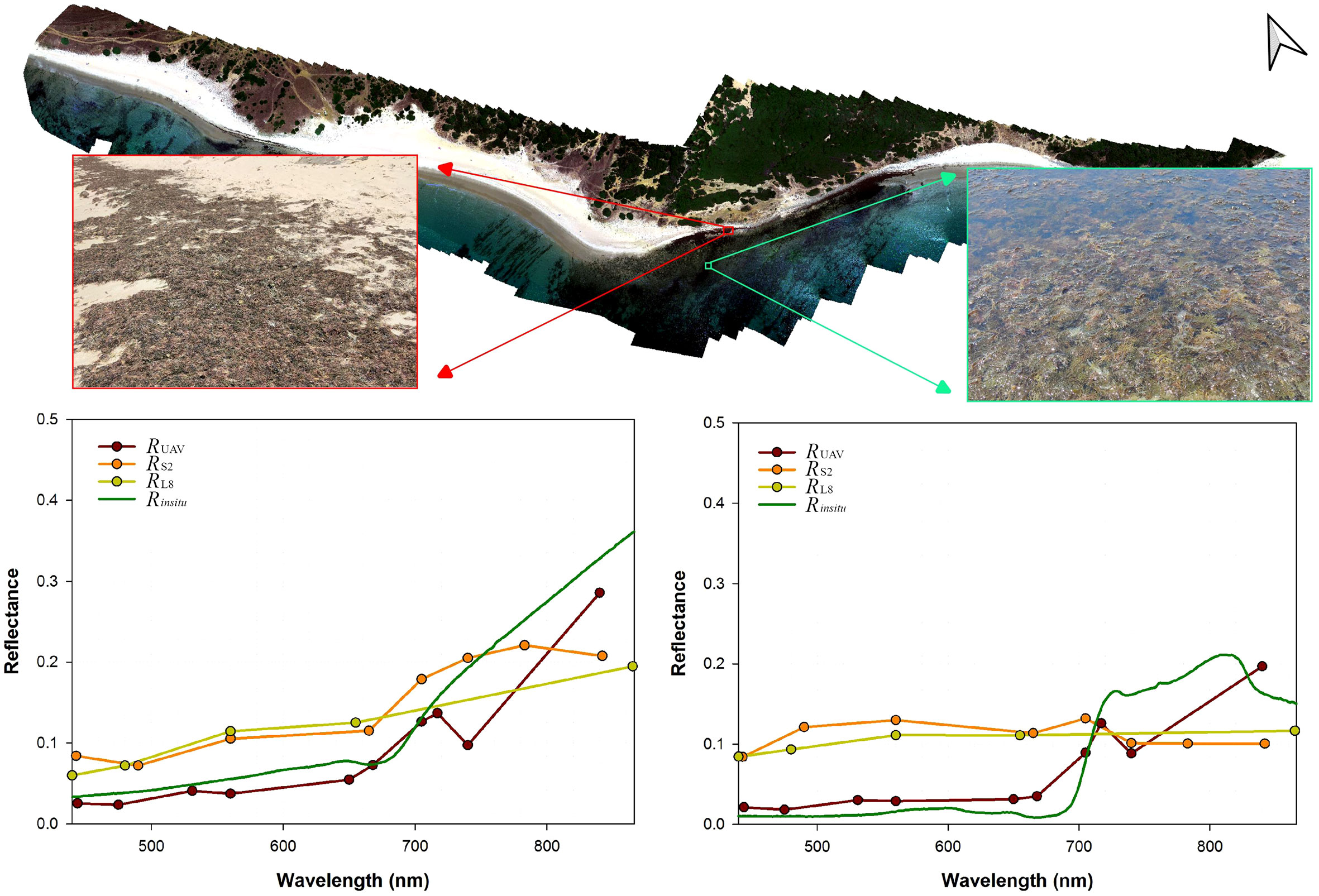
Figure 6 Spectral signatures for R. okamurae in the beaching zone (left) and submerged zone (right) for the different sensors (in situ hyperspectral, UAV, S2 and L8).
3.2 UAVs and satellites for monitoring R. okamurae
Figure 7 shows the orthomosaic of RUAV and RS2 for different spectral bands, showing three parallel stripes of color corresponding to the beach, coastal deposits of R. okamurae and coastal water. As expected, the lower spatial resolution (e.g. Figure 7, band at 443nm with native resolution of 60 m), results in lower accuracy in identifying and delimiting different types of cover.
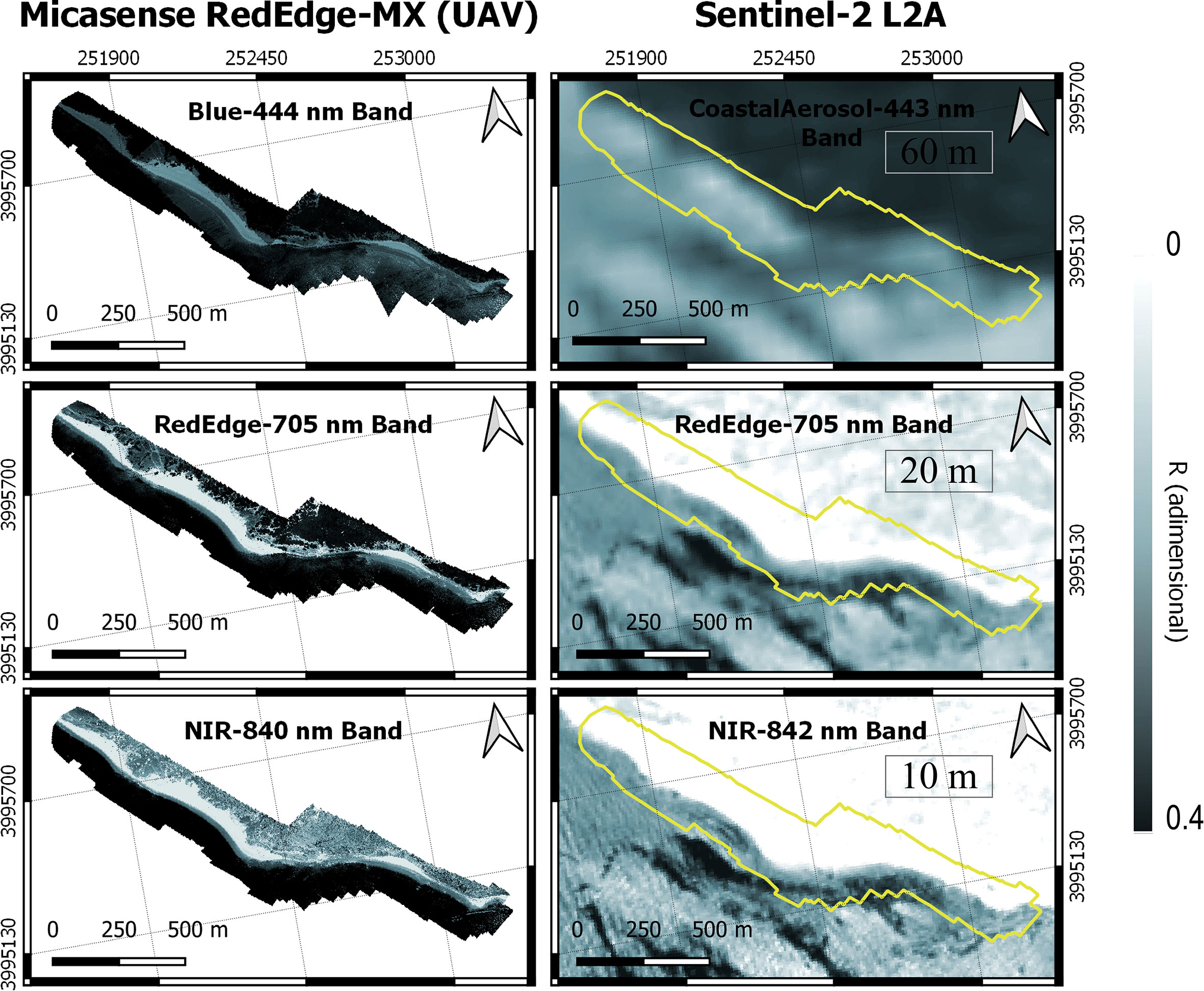
Figure 7 Orthomosaic of reflectances at different channels for the UAV flight (left) and for Sentinel-2 L2A (right) in the study area. Band spatial resolution for S2 channels from 10 to 60 m detailed.
Firstly, Figure 8 shows RGB images for the three sensors, where the difference in spatial resolution is noticeable for UAV, S2 and L8 at 8 cm, 10 m and 30 m, respectively. Secondly, the calculated NDVI allowed the visual identification of vegetation on the shore, beach, floating at the water surface and terrestrial portions of the image. Finally, the SVM classification algorithm allowed concise differentiating of each of the soil cover types, with UAV, S2 and L8 displaying a Cohen’s Kappa of 0.8763, 0.8634 and 0.8983 an overall accuracy (%) of 91.3188, 91.5130 and 93.7407, respectively. Although both statistics for L8 shows better apparent supervised classification, UAV imagery show the best performance due to its spatial resolution even in the submerged zone. Table 3 shows how S2 more accurately quantifies the area of beached R. okamurae than L8 (1,33 and 2,05 ha, respectively), S2 overestimating only 12,5% when compared with the UAV estimation of beached R. okamurae (1,52 ha). However, when using satellite imagery, neither S2 nor L8 were capable of detecting submerged R. okamurae because of the water layer that dominated the reflectance signal, with the estimation done only for the in-water intertidal zone. Although the overall area detected by L8 (6,9 ha) is more similar to the area calculated by the UAV (9,37 ha), the L8 image was capable of detecting the presence of this seaweed but its 30 m spatial resolution was not sufficient to demarcate its extent, assuming a larger area for beached R. okamurae.
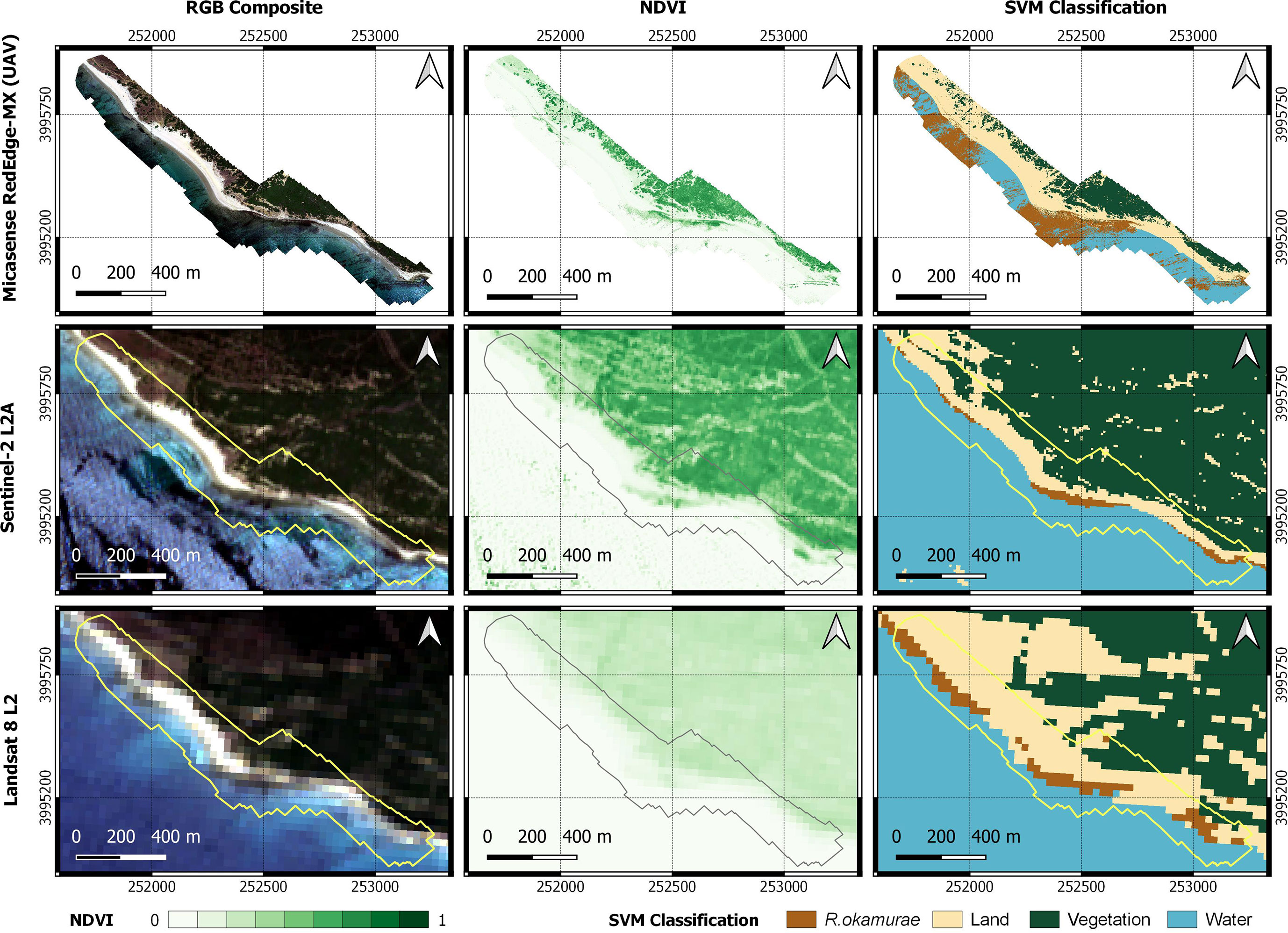
Figure 8 Supervised classification resulting from the application of SVM to reflectance data obtained with the multispectral sensor onboard the UAV, S2 and L8, with spatial resolutions of 8 cm, 10 m and 30 m, respectively.
4 Discussion
Here, we presented the first attempt at monitoring R. okamurae using remote sensing since its colonization along the African and Spanish coasts of the Strait of Gibraltar and rapid change of status from alien to invasive (Altamirano-Jeschke et al., 2016; García-Gómez et al., 2020). Different remote sensing observations including hyperspectral in situ radiometry and multispectral UAV and satellite sensors were evaluated showing the usefulness, potential and limitations of these technologies as a complementary tool to monitor the distribution of this marine IAS.
4.1 Potential of field radiometry and remote sensing to monitor R. okamurae
This study shows that different states of R. okamurae (in-water samples of living algae, fresh samples of dead algae, and dry samples) along the beach profile can be discriminated by their hyperspectral reflectance signature. For example, the spectral shape of dry R. okamurae indicated a loss of photosynthetic pigments compared with fresh samples, evidenced by the absence of spectral features around 600 and 650 nm, as well as a higher reflectance around 675 nm where Chl-a presents an absorption peak.
Over the investigated stations, the UAV reflectance was correlated with in situ hyperspectral measurements. The UAV sensor, although not hyperspectral, was sufficiently spectrally resolved to capture radiometric differences between the two main status of R. okamurae, in-water/submerged and beached. Therefore, the UAV mapping and classification can be used as a reference to validate derived satellite classification. Due to the intrapixel contribution of other types of targets and uncertainties related to atmospheric correction, differences in reflectance were higher as the pixel size increased. While intertidal marine macroalgae can be identified using UAV and satellite observation, detection of underwater vegetation remains a challenge and more sophisticated techniques are required to minimize the water column effect (Pegau et al., 1997; Oppelt et al., 2012; Zoffoli et al., 2014; Traganos and Reinartz, 2018; Tait et al., 2019; Vahtmäe et al., 2021). Hence, multispectral sensors offer a higher spectral resolution than RGB sensors, which can be perfectly correlated with hyperspectral in situ data, but NIR wavelengths are largely absorbed by water, increasing difficulties at greater depths (Oppelt et al., 2012; Tait et al., 2019; Casal-Porras et al., 2021).
Regarding satellite measurements, there are two main issues to be overcome. Firstly, atmospheric correction uncertainties, and secondly, low spatial resolution generating spectral mixing within a single pixel. Spatial and spectral resolution are key in terms of generating a reliable and robust monitoring tool for IAS distribution, especially for highly variable marine assemblages or similar spectral signatures, where pixel confusion can be an issue (Knudby and Nordlund, 2011). In this study, taking the UAV classification as an accurate classification reference (Figure 8), S2 showed a better performance than L8 in terms of R. okamurae classification, especially for the wrack deposits, with UAV and S2 being capable of accurately mapping the R. okamurae extension, as L8 is much less accurate due to a larger pixel size. Therefore, S2 images were suitable for delimiting the extent of beached R. okamurae, being a potential tool for monitoring this species. The combination of all these resources can provide an effective response for monitoring R. okamurae in shallow waters, since the accuracy of the satellite mapping is also better for the beached macroalgae than for those in the water.
UAV, S2 and L8 provide 8 cm, 10 m and 30 m spatial resolution, respectively. Although using an UAV is more accurate, it is time-consuming, it covers a very low area to be mapped, and the method described is challenging to apply to other sites as new vegetation classes have to be considered (Manfreda et al., 2018). However, satellite imagery obtained from S2 or L8 has a lower accuracy, but high revisit-time allowing time-series of observations, which are useful for continuous monitoring, allowing the upscaling of this approach worldwide. While UAVs are being used more frequently for environmental monitoring (Murfitt et al., 2017; Konar and Iken, 2018; Ventura et al., 2018; Brunier et al., 2022; Diruit et al., 2022), S2 seems to be a promising tool for detecting the presence of R. okamurae along the dry and intertidal beach, demonstrated in this study down to 5 m depth, as spectral profile separation becomes more complex with increasing water depth. Bridging the gap between the weaknesses of both satellites and UAVs can provide reliable information to validate long-term satellite monitoring. The added value of the S2 products in terms of frequency and synoptic observations is of utmost importance for ecological and management purposes at regional and national scales (Caballero et al., 2020).
4.2 Policy support and operational management of IAS
Marine invasive species, and R. okamurae in particular, are spreading rapidly throughout the western Mediterranean Sea, and beyond, thus requiring monitoring information for proper management. Since these species do not respect borders, and maritime traffic (ballast water and fouling of boats/ships) is an important vector for their spread, transboundary coordination in management actions across the EU are most likely to be more effective than individual activities at Member State level. At the moment there is a lack of common monitoring and management approaches and data flow to the European Alien Species Information Network (EASIN), for example, which is based on voluntary contributions (personal communication). Binding data provision procedures could be beneficial for policy makers at national and EU level for the definition of policies to reduce or even prevent further spread of this species and concomitant loss of biodiversity.
In 2019, R. okamurae was included in the Spanish national catalogue of invasive species, which is freely available on the website of the Spanish Ministry for ecological transition and demographic challenge (in Spanish: https://www.miteco.gob.es/es/biodiversidad/temas/conservacion-de-especies/especies-exoticas-invasoras/ce-eei-catalogo.aspx). In the report about R. okamurae, included in the catalogue, numerous measures were proposed, including several that may be facilitated by a zoomed-out approach as described in this paper, and which could be further considered in succeeding studies. For example, monitoring and surveillance of the distribution and abundance of R. okamurae populations, especially in marine protected areas, habitats of interest and within the limits of its distribution. An established monitoring procedure would support the creation of early warning systems in areas where R. okamurae predictive models have shown highly favorable environmental conditions, especially in Protected Areas of the Natura 2000 Network, for example. Furthermore, these types of protected habitats may especially benefit from a less-invasive monitoring approach, as that described in this study, compared to in situ approaches, such as diving. In the Spanish national context, in addition to transboundary coordination, inter-administrative coordination among local, regional and state administrations with competences in environment, tourism, fishing, transport, among others, could be further facilitated by such an approach.
The next step towards a more coordinated management approach would be to include R. okamurae in the official “List of Invasive Alien Species of Union concern”, i.e. at EU level. In this regard, in April 2021, the Spanish Ministry for ecological transition and demographic challenge (MITECO) submitted a risk assessment together with a request for inclusion of R. okamurae in the EU list. The last update available of the list is from 2019 and, so far, the species has neither been included, nor officially excluded.
This study highlights the potential of remote sensing approaches to be established by coastal managers for the monitoring of R. okamurae in order to generate information for the management of the environmental crisis generated by the occurrence of this macroalgae. In order to address the current situation, the implementation of a tool for long-term monitoring together with scuba diving campaigns to reach deeper areas is critical. This strategy can also work as an early-warning system for the detection of new R. okamurae settlements along the coast, useful for short-term and emergency response, providing up-to-date information for the shallow distribution, advance and damage critical for optimizing its management. However, further research is required in order to test the taxonomical differentiation using UAV and satellite imagery classification between R. okamurae and native species.
5 Conclusions
The hyperspectral data set obtained by in situ radiometry allowed us to analyze the reflectance of Rugulopteryx okamurae in its different states (submerged 0-5 m and beached in a range of water conditions). These data are in agreement with the spectral signatures obtained from the multispectral sensor mounted on the UAV, whereas S2 and L8 satellites revealed overestimated reflectance in the visible spectrum. This study demonstrates the usefulness of these techniques, combining field-based and Earth Observation for monitoring the marine IAS R. okamurae. This information, if continuously generated, can be key for proper management of this species, with positive implications of this approach in terms of supporting regional, national and European policies, including the EU Biodiversity Strategy 2030, as well as the EU Objectives to restore marine ecosystems. The Spanish Mediterranean coasts and those of the Strait of Gibraltar are a highly favorable environment for the spreading of R. okamurae, which may favor its expansion and concomitant impacts. In this regard, the inherent bureaucratic sluggishness, most certainly influenced by the health crisis during the past couple years, appear not to be able to keep up with this rapidly spreading invasive alien species. In fact, satellite and drone monitoring could be a key tool to be scaled-up and implemented in current management programmes, providing a holistic view to supporting national and European policy in a continuous and cost-effective way (Papathanasopoulou et al., 2019; El Mahrad et al., 2020).
Data availability statement
The raw data supporting the conclusions of this article will be made available by the authors, without undue reservation.
Author contributions
GN, IC, and MD developed the design of the study, MR and MD carried out the initial literature review and writing of the first drafts. MR and AR designed the figures and geoprocessed data. MR designed the graphical abstract and developed the scientific illustration. IC processed atmospheric corrections for satellite imagery and MZ processed hyperspectral data. GN, AR, IC, MZ, and PG participated in the field campaign on 1st July 2021, both for UAV flights and hyperspectral in situ sampling. All authors contributed to the reviewing, editing and supervision and agreed to the final version of the manuscript.
Funding
This study has been funded by the Andalusian Regional Government (project PY20-00244), OAPN-2715/2021 and projects RTI2018-098784-J-I00, IJC2019-039382-I, EQC2018-004275 and EQC2019-00572 funded by MCIN/AEI/10.13039/501100011033 and by ”ERDF A way of making Europe“, and grants FPU20/01294 and FPU19/04557 funded by the Ministry of Universities of the Spanish Government. This research was also supported by the project ”Biodiversity of the Coastal Ocean: Monitoring with Earth Observation“ (BiCOME) funded by the European Space Agency (contract No. 4000135756/21/I-EF) in the frame of the FutureEO-1 BIODIVERSITY+ PRECURSORS call. This work represents a contribution to CSIC Interdisciplinary Thematic Platform (PTI) Teledetección (PTI-TELEDETECT).
Acknowledgments
We would like to thank the OPECAM and SEADRON teams from the Institute of Marine Sciences of Andalusia, Spanish National Research Council (ICMAN-CSIC) for the UAV technical support and sample collection. Also thank ESA and USGS for the provision of S2 and L8 data, and the City Council of Tarifa for the provision of photos and data of Los Lances beach (Tarifa).
Conflict of interest
The authors declare that the research was conducted in the absence of any commercial or financial relationships that could be construed as a potential conflict of interest.
Publisher’s note
All claims expressed in this article are solely those of the authors and do not necessarily represent those of their affiliated organizations, or those of the publisher, the editors and the reviewers. Any product that may be evaluated in this article, or claim that may be made by its manufacturer, is not guaranteed or endorsed by the publisher.
References
Abdulla A., Linden O. (2008). Maritime traffic effects on biodiversity in the Mediterranean Sea: Review of impacts, priority areas and mitigation measures IUCN, 1.
Altamirano M., de la Rosa J., Carmona R., Zanolla M., Muñoz A. (2019). Macroalgas invasoras en las costas andaluzas. Algas 55e, 10–13.
Altamirano-Jeschke M., de la Rosa Álamos J., Martínez Medina F. J. (2016). Arribazones de la especia exótica Rugulopteryx okamurae (EY Dawson) en el estrecho de Gibraltar. Available at: https://riuma.uma.es/xmlui/handle/10630/12433
Altamirano-Jeschke M., de la Rosa J., Martínez Gil F. J., Román Muñoz Gallego A. (2017). Prolifera en el estrecho un alga nunca citada en nuestro litoral: de origen asiático,” Rugulopteryx okamurae” ocupa ya una gran extensión. Quercus 374), 32–33.
Barillé L., Robin M., Harin N., Bargain A., Launeau P. (2010). Increase in seagrass distribution at bourgneuf bay (France) detected by spatial remote sensing. Aquat. Bot. 92 (3), 185–194. doi: 10.1016/j.aquabot.2009.11.006
Beca-Carretero P., Varela S., Stengel D. B. (2020). A novel method combining species distribution models, remote sensing, and field surveys for detecting and mapping subtidal seagrass meadows. Aquat. Conservat.: Mar. Freshw. Ecosyst. 30 (6), 1098–1110. doi: 10.1002/aqc.3312
Brito A. C., Benyoucef I., Jesus B., Brotas V., Gernez P., Mendes C. R., et al. (2013). Seasonality of microphytobenthos revealed by remote-sensing in a south European estuary. Continental Shelf Res. 66, 83–91. doi: 10.1016/j.csr.2013.07.004
Brunier G., Oiry S., Gruet Y., Dubois S. F., Barillé L. (2022). Topographic analysis of intertidal polychaete reefs (Sabellaria alveolata) at a very high spatial resolution. Remote Sens. 14 (2), 307. doi: 10.3390/rs14020307
Caballero I., Fernández R., Escalante O. M., Mamán L., Navarro G. (2020). New capabilities of sentinel-2A/B satellites combined with in situ data for monitoring small harmful algal blooms in complex coastal waters. Sci. Rep. 10 (1), 8743. doi: 10.1038/s41598-020-65600-1
Casal-Porras I., Zubía E., Brun F. G. (2021). Dilkamural: A novel chemical weapon involved in the invasive capacity of the alga Rugulopteryx okamurae in the strait of Gibraltar. Estuar. Coast. Shelf Sci. 257, 107398. doi: 10.1016/j.ecss.2021.107398
Conrad O., Bechtel B., Bock M., Dietrich H., Fischer E., Gerlitz L., et al. (2015). System for automated geoscientific analyses (SAGA) v. 2.1. 4. Geosci. Model. Dev. 8 (7), 1991–2007. doi: 10.5194/gmd-8-1991-2015
De Villèle X., Verlaque M. (1995). Changes and degradation in a posidonia oceanica bed invaded by the introduced tropical alga caulerpa taxifolia in the north Western Mediterranean. Botanica Mar. 38 (1-6), 79–88. doi: 10.1515/botm.1995.38.1-6.79
Diruit W., Le Bris A., Bajjouk T., Richier S., Helias M., Burel T., et al. (2022). Seaweed habitats on the shore: Characterization through hyperspectral UAV imagery and field sampling. Remote Sens. 14 (13), 3124. doi: 10.3390/rs14133124
El Aamri F., Idhalla M., Tamsouri M. N. (2018). Occurrence of the invasive brown seaweed Rugulopteryx okamurae (EY Dawson) IK hwang, WJ Lee & HS Kim (Dictyotales, phaeophyta) in Morocco (Mediterranean Sea). Mediterr. Fish. Aquacul. Res. 1 (2), 92–96.
El Mahrad B., Newton A., Icely J. D., Kacimi I., Abalansa S., Snoussi M. (2020). Contribution of remote sensing technologies to a holistic coastal and marine environmental management framework: A review. Remote Sens. 12 (14), 2313. doi: 10.3390/rs12142313
Endrina N., Rasero J.C., Konovessis D. (2018). Risk analysis for RoPax vessels: A case of study for the Strait of Gibraltar. Ocean Engineering 151, 141–151. doi: 10.1016/j.oceaneng.2018.01.038
España G.d. (2007). Ley 42/2007, de 13 de diciembre, del patrimonio natural y de la biodiversidad Agencia Estatal Boletín Oficial del Estad, Spain. Available at: https://www.boe.es/buscar/act.php?id=BOE-A-2007-21490
Faria J., Prestes A. C. L., Moreu I., Cacabelos E., Martins G. M. (2022). Dramatic changes in the structure of shallow-water marine benthic communities following the invasion by Rugulopteryx okamurae (Dictyotales, ochrophyta) in Azores (NE Atlantic). Mar. pollut. Bull. 175, 113358. doi: 10.1016/j.marpolbul.2022.113358
Foody G. M., Mathur A. (2004). Toward intelligent training of supervised image classifications: directing training data acquisition for SVM classification. Remote Sens. Environ. 93 (1), 107–117. doi: 10.1016/j.rse.2004.06.017
Gallardo B., Bacher S., Bradley B., Comín F. A., Gallien L., Jeschke J. M., et al. (2019). InvasiBES: Understanding and managing the impacts of invasive alien species on biodiversity and ecosystem services. NeoBiota 50, 109. doi: 10.3897/neobiota.50.35466
García Gómez J. C. (2015). “A guide on environmental monitoring of rocky seabeds in mediterranean marine protected areas and surrounding zones,” in A guide on the identification and monitoring of disturbance-sensitive, benthic (rocky seabeds), sessile target species for scientific, professional and recreational divers, academics working in the area, specialized businesses and public authorities connected to the environment and all involved actors in the management, impact assessment, monitoring and conservation of coastal environment (Sevilla: RAC/SPA: Consejería de Medio Ambiente y Ordenación del Territorio (CMAOT) de la Junta de Andalucía).
García-Gómez J. C., Florido M., Olaya-Ponzone L., Rey Díaz de Rada J., Donázar-Aramendía I., Chacón M., et al. (2021). Monitoring extreme impacts of Rugulopteryx okamurae (Dictyotales, ochrophyta) in El estrecho natural park (Biosphere reserve). showing radical changes in the underwater seascape. Front. Ecol. Evol. 9. doi: 10.3389/fevo.2021.639161
García-Gómez J. C., Sempere-Valverde J., González A. R., Martínez-Chacón M., Olaya-Ponzone L., Sánchez-Moyano E., et al. (2020). From exotic to invasive in record time: The extreme impact of Rugulopteryx okamurae (Dictyotales, ochrophyta) in the strait of Gibraltar. Sci. Tot. Environ. 704, 135408. doi: 10.1016/j.scitotenv.2019.135408
García-Gómez J. C., Sempere-Valverde J., Ostalé-Valriberas E., Martínez M., Olaya-Ponzone L., González A. R., et al. (2018). Rugulopteryx okamurae (EY Dawson) IK hwang, WJ Lee & HS Kim (Dictyotales, ochrophyta), alga exótica “explosiva” en el estrecho de gibraltar. observaciones preliminares de su distribución e impacto. Almoraima 48, 97–113.
Garrabou J., Coma R., Bensoussan N., Bally M., Chevaldonné P., Cigliano M., et al. (2009). Mass mortality in northwestern Mediterranean rocky benthic communities: effects of the 2003 heat wave. Global Change Biol. 15 (5), 1090–1103. doi: 10.1111/j.1365-2486.2008.01823.x
Giakoumi S., Katsanevakis S., Albano P. G., Azzurro E., Cardoso A. C., Cebrian E., et al. (2019). Management priorities for marine invasive species. Sci. Tot. Environ. 688, 976–982. doi: 10.1016/j.scitotenv.2019.06.282
Hedley J. D., Russell B. J., Randolph K., Pérez-Castro M.Á., Vásquez-Elizondo R. M., Enríquez S., et al. (2017). Remote sensing of seagrass leaf area index and species: The capability of a model inversion method assessed by sensitivity analysis and hyperspectral data of Florida bay. Front. Mar. Sci. 4. doi: 10.3389/fmars.2017.00362
Katsanevakis S., Wallentinus I., Zenetos A., Leppäkoski E., Çinar M. E., Oztürk B., et al. (2014). Impacts of invasive alien marine species on ecosystem services and biodiversity: a pan-European review. Aquat. Invasions 9 (4), 391–423. doi: 10.3391/ai.2014.9.4.01
Kaufman K. A., Bell S. S. (2020). The use of imagery and GIS techniques to evaluate and compare seagrass dynamics across multiple spatial and temporal scales. Estuar. Coasts. 45, 1028–1044. doi: 10.1007/s12237-020-00773-6
Knight E. J., Kvaran G. (2014). Landsat-8 operational land imager design, characterization and performance. Remote Sens. 6 (11), 10286–10305. doi: 10.3390/rs61110286
Knudby A., Nordlund L. (2011). Remote sensing of seagrasses in a patchy multi-species environment. Int. J. Remote Sens. 32 (8), 2227–2244. doi: 10.1080/01431161003692057
Kohlus J., Stelzer K., Müller G., Smollich S. (2020). Mapping seagrass (Zostera) by remote sensing in the schleswig-Holstein wadden Sea. Estuar. Coast. Shelf Sci. 238, 106699. doi: 10.1016/j.ecss.2020.106699
Konar B., Iken K. (2018). The use of unmanned aerial vehicle imagery in intertidal monitoring. Deep Sea Res. Part II: Top. Stud. Oceanog. 147, 79–86. doi: 10.1016/j.dsr2.2017.04.010
Lejeusne C., Chevaldonné P., Pergent-Martini C., Boudouresque C. F., Pérez T. (2010). Climate change effects on a miniature ocean: the highly diverse, highly impacted Mediterranean Sea. Trends Ecol. Evol. 25 (4), 250–260. doi: 10.1016/j.tree.2009.10.009
Linares C., Doak D. F., Coma R., Díaz D., Zabala M. (2007). Life history and viability of a long-lived marine invertebrate: The octocoral paramuricea clavata. Ecology 88 (4), 918–928. doi: 10.1890/05-1931
Lodge D. M., Williams S., MacIsaac H. J., Hayes K. R., Leung B., Reichard S., et al. (2006). BIOLOGICAL INVASIONS: RECOMMENDATIONS FOR U.S. POLICY AND MANAGEMENT. Ecol. Appl. 16 (6), 2035–2054. doi: 10.1890/1051-0761(2006)016[2035:BIRFUP]2.0.CO;2
Main-Knorn M., Pflug B., Louis J., Debaecker V., Müller-Wilm U., Gascon F. (2017). “Sen2Cor for sentinel-2,” in Image and signal processing for remote sensing XXIII (Warsaw, Poland: International Society for Optics and Photonics), 1042704.
Manfreda S., McCabe M. F., Miller P. E., Lucas R., Pajuelo Madrigal V., Mallinis G., et al. (2018). On the use of unmanned aerial systems for environmental monitoring. Remote Sens. 10 (4), 641. doi: 10.3390/rs10040641
Méléder V., Savelli R., Barnett A., Polsenaere P., Gernez P., Cugier P., et al. (2020). Mapping the intertidal microphytobenthos gross primary production part I: Coupling multispectral remote sensing and physical modeling. Front. Mar. Sci. 7. doi: 10.3389/fmars.2020.00520
Miranda V., Pina P., Heleno S., Vieira G., Mora C. (2020). Monitoring recent changes of vegetation in fildes peninsula (King George island, Antarctica) through satellite imagery guided by UAV surveys. Sci. Tot. Environ. 704, 135295. doi: 10.1016/j.scitotenv.2019.135295
Mountrakis G., Im J., Ogole C. (2011). Support vector machines in remote sensing: A review. ISPRS J. Photogrammet. Remote Sens. 66 (3), 247–259. doi: 10.1016/j.isprsjprs.2010.11.001
Muñoz A.-R., Martín-Taboada A., de la Rosa J., Carmona R., Zanolla M., Altamirano M. (2019). La modelación de la distribución de especies como herramienta en la gestión de invasiones biológicas en el medio marino: el caso de Rugulopteryx okamurae (Dictyotaceae, ochrophyta) en el mediterráneo. Algas 55e, 37.
Murfitt S. L., Allan B. M., Bellgrove A., Rattray A., Young M. A., Ierodiaconou D. (2017). Applications of unmanned aerial vehicles in intertidal reef monitoring. Sci. Rep. 7 (1), 10259. doi: 10.1038/s41598-017-10818-9
Ocaña V. O., Afonso-Carrillo J. M., Ballesteros E. (2016). Massive proliferation of a dictyotalean species (Phacophyccae, ochriohyta) througn the strait of Gibraltar. Rev. la Academia Canaria Ciencias:= Folia Canariensis Academiae Scientiarum 28 (1), 165–169.
Ojaveer H., Galil B. S., Minchin D., Olenin S., Amorim A., Canning-Clode J., et al. (2014). Ten recommendations for advancing the assessment and management of non-indigenous species in marine ecosystems. Mar. Policy 44, 160–165. doi: 10.1016/j.marpol.2013.08.019
Olofsson P., Foody G. M., Herold M., Stehman S. V., Woodcock C. E., Wulder M. A. (2014). Good practices for estimating area and assessing accuracy of land change. Remote Sens. Environ. 148, 42–57. doi: 10.1016/j.rse.2014.02.015
Oppelt M. N., Florian S., Katja D., Inga E., Inka B. (2012). Hyperspectral classification approaches for intertidal macroalgae habitat mapping: a case study in heligoland. Optical Eng. 51 (11), 1–12. doi: 10.1117/1.OE.51.11.111703
Papathanasopoulou E., Simis S., Alikas K., Ansper A., Anttila J., Barillé A., et al. (2019). Satellite-assisted monitoring of water quality to support the implementation of the water framework directive. EOMORES white Pap. 1–27. doi: 10.5281/zenodo.3556478
Pegau W. S., Gray D., Zaneveld J. R. V. (1997). Absorption and attenuation of visible and near-infrared light in water: dependence on temperature and salinity. Appl. Optics 36 (24), 6035–6046. doi: 10.1364/AO.36.006035
Ribera S. (2003). Pathways of biological invasions of marine plants. Invasive species: Vectors Manage. strategies, 183–226.
Román A., Navarro G., Caballero I., Tovar-Sánchez A. (2022). High-spatial resolution UAV multispectral data complementing satellite imagery to characterize a chinstrap penguin colony ecosystem on deception island (Antarctica). GISci. Remote Sens. 59 (1), 1159–1176. doi: 10.1080/15481603.2022.2101702
Román A., Tovar-Sánchez A., Olivé I., Navarro G. (2021). Using a UAV-mounted multispectral camera for the monitoring of marine macrophytes. Front. Mar. Sci. 8. doi: 10.3389/fmars.2021.722698
Rupasinghe P. A., Simic Milas A., Arend K., Simonson M. A., Mayer C., Mackey S. (2019). Classification of shoreline vegetation in the Western basin of lake Erie using airborne hyperspectral imager HSI2, Pleiades and UAV data. Int. J. Remote Sens. 40 (8), 3008–3028. doi: 10.1080/01431161.2018.1539267
Schaffelke B., Smith J. E., Hewitt C. L. (2006). Introduced macroalgae – a growing concern. J. Appl. Phycol. 18 (3), 529–541. doi: 10.1007/s10811-006-9074-2
Sempere-Valverde J., Ostalé-Valriberas E., Maestre M., González Aranda R., Bazairi H., Espinosa F. (2021). Impacts of the non-indigenous seaweed Rugulopteryx okamurae on a Mediterranean coralligenous community (Strait of gibraltar): The role of long-term monitoring. Ecol. Indic. 121, 107135. doi: 10.1016/j.ecolind.2020.107135
Tait L., Bind J., Charan-Dixon H., Hawes I., Pirker J., Schiel D. (2019). Unmanned aerial vehicles (UAVs) for monitoring macroalgal biodiversity: Comparison of RGB and multispectral imaging sensors for biodiversity assessments. Remote Sens. 11 (19), 2332. doi: 10.3390/rs11192332
Thornber C. S., Guidone M., Deacutis C., Green L., Ramsay C. N., Palmisciano M. (2017). Spatial and temporal variability in macroalgal blooms in a eutrophied coastal estuary. Harmful Algae 68, 82–96. doi: 10.1016/j.hal.2017.07.011
Traganos D., Reinartz P. (2018). Mapping Mediterranean seagrasses with sentinel-2 imagery. Mar. pollut. Bull. 134, 197–209. doi: 10.1016/j.marpolbul.2017.06.075
Union, E.P.a.C.o.t.E (2014). “REGULATION (EU) no 1143/2014 OF THE EUROPEAN PARLIAMENT AND OF THE COUNCIL of 22 October 2014 on the prevention and management of the introduction and spread of invasive alien species,” in Official journal of the European union Official journal of the European union: Brussels.
Vahtmäe E., Kotta J., Lõugas L., Kutser T. (2021). Mapping spatial distribution, percent cover and biomass of benthic vegetation in optically complex coastal waters using hyperspectral CASI and multispectral sentinel-2 sensors. Int. J. Appl. Earth Observat. Geoinformat. 102, 102444. doi: 10.1016/j.jag.2021.102444
Vapnik V. (1999). The nature of statistical learning theory (New York, USA: Springer science & business media). doi: 10.1007/978-1-4757-3264-1
Veettil B. K., Ward R. D., Lima M. D. A. C., Stankovic M., Hoai P. N., Quang N. X. (2020). Opportunities for seagrass research derived from remote sensing: A review of current methods. Ecol. Indic. 117, 106560. doi: 10.1016/j.ecolind.2020.106560
Ventura D., Bonifazi A., Gravina M. F., Belluscio A., Ardizzone G. (2018). Mapping and classification of ecologically sensitive marine habitats using unmanned aerial vehicle (UAV) imagery and object-based image analysis (OBIA). Remote Sens. 10 (9), 1331. doi: 10.3390/rs10091331
Verlaque M., Steen F., De Clerck O. (2009). Rugulopteryx (Dictyotales, phaeophyceae), a genus recently introduced to the Mediterranean. Phycologia 48 (6), 536–542. doi: 10.2216/08-103.1
Williams S. L., Smith J. E. (2007). A global review of the distribution, taxonomy, and impacts of introduced seaweeds. Annu. Rev. Ecol. Evol. Systemat. 38 (1), 327–359. doi: 10.1146/annurev.ecolsys.38.091206.095543
Zoffoli M. L., Frouin R., Kampel M. (2014). Water column correction for coral reef studies by remote sensing. Sensors 14 (9), 16881–16931. doi: 10.3390/s140916881
Zoffoli M. L., Gernez P., Godet L., Peters S., Oiry S., Barillé L. (2021). Decadal increase in the ecological status of a north-Atlantic intertidal seagrass meadow observed with multi-mission satellite time-series. Ecol. Indic. 130, 108033. doi: 10.1016/j.ecolind.2021.108033
Keywords: invasive macroalgae, algae monitoring, multispectral sensor, hyperspectral sensor, UAV, Sentinel-2, Landsat-8, machine learning
Citation: Roca M, Dunbar MB, Román A, Caballero I, Zoffoli ML, Gernez P and Navarro G (2022) Monitoring the marine invasive alien species Rugulopteryx okamurae using unmanned aerial vehicles and satellites. Front. Mar. Sci. 9:1004012. doi: 10.3389/fmars.2022.1004012
Received: 26 July 2022; Accepted: 28 September 2022;
Published: 13 October 2022.
Edited by:
Ricardo Bermejo, University of Malaga, SpainReviewed by:
Hanzhi Lin, Science Systems and Applications, Inc., United StatesSita Karki, Irish Centre for High-End Computing (ICHEC), Ireland
Copyright © 2022 Roca, Dunbar, Román, Caballero, Zoffoli, Gernez and Navarro. This is an open-access article distributed under the terms of the Creative Commons Attribution License (CC BY). The use, distribution or reproduction in other forums is permitted, provided the original author(s) and the copyright owner(s) are credited and that the original publication in this journal is cited, in accordance with accepted academic practice. No use, distribution or reproduction is permitted which does not comply with these terms.
*Correspondence: Isabel Caballero, isabel.caballero@icman.csic.es