- 1School of Environmental Science and Engineering, Guangzhou University, Guangzhou, China
- 2Biological and Environmental Sciences and Engineering Division (BESE), King Abdullah University of Science and Technology (KAUST), Thuwal, Saudi Arabia
- 3State Key Laboratory of Marine Environmental Science and College of Ocean and Earth Sciences, Xiamen University, Xiamen, China
Ocean acidification (OA) may interact with anthropogenic pollutants, such as heavy metals (HM), to represent a threat to marine organisms and ecosystems. Here, we perform a quantitative meta-analysis to examine the combined effects of OA and heavy metals on marine organisms. The results reveal predominantly additive interactions (67%), with a considerable proportion of synergistic interactions (25%) and a few antagonistic interactions (8%). The overall adverse effects of heavy metals on marine organisms were alleviated by OA, leading to a neutral impact of heavy metals in combination with OA. However, different taxonomic groups showed large variabilities in their responses, with microalgae being the most sensitive when exposed to heavy metals and OA, and having the highest proportion of antagonistic interactions. Furthermore, the variations in interaction type frequencies are related to climate regions and heavy metal properties, with antagonistic interactions accounting for the highest proportion in temperate regions (28%) and when exposed to Zn (52%). Our study provides a comprehensive insight into the interactive effects of OA and HM on marine organisms, and highlights the importance of further investigating the responses of different marine taxonomic groups from various geographic locations to the combined stress of OA and HM.
Introduction
Marine organisms in coastal waters are exposed to multiple anthropogenic pressures. Among these pressures, heavy metals are major stressors that threaten marine organisms (Ivanina and Sokolova, 2015), and they are conservative in nature and can persist in the environment for extended periods (Reichelt-Brushett, 2012). Heavy metal contamination constitutes a consequence of anthropogenic activities and is recognized as a growing environmental concern (Doney, 2010). They enter the marine environment in various ways, including as agricultural and urban stormwater runoff, industrial effluents, sewage treatment discharge, and through fossil fuel combustion (Davis et al., 2001; Flint and Davis, 2007; Pan and Wang, 2012). Disposal of dredged spoil, ash, and antifouling paints for marine vessels and structures also contribute substantially to the heavy metal contamination of the oceans (Tanaka et al., 2013). Since heavy metals cannot be degraded by chemical or biological processes, they often accumulate up to high levels in sediments, acting as a sink. At the same time, heavy metals can be released from sediments to overlying waters due to natural or anthropogenic disturbances, acting as a source (Pan and Wang, 2012). It is known that high concentrations of heavy metals may cause toxicity in various taxonomic groups of marine organisms, including corals (Biscéré et al., 2015), fish (Cui et al., 2020), macroalgae (Wen and Zou, 2021), microalgae (Zhang et al., 2020), molluscs (Cao et al., 2018), and polychaetes (Nielson et al., 2019), targeting their growth, development, photosynthesis, gametogenesis, and antioxidant systems (Filosto et al., 2008; Pinsino et al., 2010; Matranga et al., 2011). Additionally, toxic heavy metals can be taken up by primary producers, enter the food web and be potentially transferred to higher trophic levels and threaten human beings (Wang, 2002).
Besides the pollution of coastal waters by heavy metals, there is growing concern regarding ocean acidification (OA), driven by increasing atmospheric carbon dioxide concentrations due to anthropogenic activities (Caldeira and Wickett, 2003), which can lead to tremendous impacts on various marine organisms (Kroeker et al., 2013; Hurd et al., 2018; Gao et al., 2019). The increasing amount of CO2 dissolved in the ocean has resulted in a pH decline of sea surface waters by ∼0.1 units since pre-industrial times, and based on RCP 8.5 a further drop in pH of ∼0.4 units by the end of this century (IPCC, 2014; Gattuso et al., 2015). The pH of coastal waters is expected to drop 0.45 units by 2100, which is a 12% faster decrease than in the open ocean (Cai et al., 2011).
In the context of OA, the solubility, adsorption, toxicity, and rates of redox processes of heavy metals in seawater can be affected by the decrease in concentrations of OH– and CO32– ions (Millero et al., 2009; Stockdale et al., 2016). Metals that form strong complexes with carbonate ions are supposed to be most strongly affected by a decrease in pH, resulting in an increase in their free ionic forms in the future as OA progresses (Millero et al., 2009). For example, Cu and Ni both form a strong complex with carbonates (CuCO3, NiCO3), and the decrease in CO32– concentrations driven by OA could result in an increase in free Cu2+ and Ni2+ concentrations (from the present-day ∼8 and 4% to ∼32 and 13% by the year 2250 for Cu2+ and Ni2+, respectively) (Millero et al., 2009). Therefore, over the last decade, there has been a growing interest in the interplay between OA and heavy metals on marine biota (e.g., Moreira et al., 2016; Nardi et al., 2018; Cao et al., 2019; Dong et al., 2020; Zhang et al., 2020).
For instance, studies found that OA increased the accumulation of Mn in the polychaete Nereis diversicolor (Rodríguez-Romero et al., 2014) as well as the concentrations of Zn, Pb, Cu, Ni, Cr, Hg, and As in the carpet clam Ruditapes philippinarum (López et al., 2010). These findings show that OA exacerbated the deleterious effects of heavy metals. A strong synergistic toxicity between OA and heavy metals was also found for larval survival of the polychaete Arenicola marina (Campbell et al., 2014). In contrast, OA enhanced the tolerance of the marine diatom Phaeodactylum tricornutum to the heavy metal Cd, thus benefiting their survival under Cd exposure, suggesting an antagonistic interaction between OA and Cd (Dong et al., 2020). Although the interplay between OA and heavy metals in marine organisms has been examined experimentally (see review by Ivanina and Sokolova, 2015 and references therein), a quantitative meta-analysis investigating the interactions between these two factors on marine biota has not been reported yet. Previous experimental studies showed that the interplay between OA and heavy metals is dependent on the species, the life stage of the organism, and the degree of acidification (e.g., Götze et al., 2014; Rodríguez-Romero et al., 2014; Gao et al., 2017). These facts highlight the need for further empirical investigations of the combined effects of OA and heavy metals and their regulating factors.
Here, we integrated the published literature results and performed a quantitative meta-analysis to improve our knowledge on the interactive effects of OA and heavy metals on marine biota. Specifically, we established a comparative framework and analyzed how various biological responses were impacted by OA, heavy metals, or the combination of both. Secondly, we assessed how expected responses to OA, heavy metals, or both could vary across distinct taxonomical groups (corals, fish, microalgae, macroalgae, molluscs, and polychaetes) and the climate regions where organisms are collected [temperate or (sub-) tropical]. Thirdly, we quantified how the magnitude of the pH change and the concentrations of heavy metals influence the effects across multiple response variables. Finally, we highlight new insights and propose recommendations for future research. The findings presented here can help estimate the combined impacts of OA and heavy metal exposure on marine biota, which can support decision-making processes for human well-being and environmental sustainability.
Materials and Methods
Literature Search
We performed the literature searches using ISI Web of Science (v.5.35) and Google Scholar for studies examining experimental responses of marine organisms to OA and heavy metals in combination using the keywords: ocean acidification, high CO2, elevated CO2, heavy metals. These searches were conducted before 31 January 2020 and updated on 1 April 2021.
Study Selection Criteria
We assessed publications for their suitability and retained only studies that examined the responses of organisms to ocean acidification and heavy metals in a full-factorial experiment, i.e., investigating effects of OA and heavy metals individually and in combination, and comparing responses to an ambient control treatment. This experimental design resulted in four treatments: control (C), ocean acidification (OA), heavy metals (HM), and the combination of ocean acidification and heavy metals (OA+HM). Only studies meeting those criteria were included for further analysis. The stressor manipulation level for OA was based on the representative concentration pathway (RCP) 8.5 emission scenario, the most widely used and well-established projection by IPCC for 2100 (IPCC, 2014). Under this scenario, the atmospheric CO2 level increases from the current ∼400 to 1,000 ppm by the end of this century, leading to a decrease in ocean surface pH of ∼0.4 by 2100. Studies using higher values than those predicted for the RCP 8.5 emission scenario were excluded from the analysis as adopted by Sampaio et al. (2021), although the pH of coastal waters is expected to drop by 0.45 units by the end of this century and is experiencing high diel fluctuations (up to 1 unit) due to high biomass and sufficient or excess nutrients (Cai et al., 2011; Duarte et al., 2013). This approach with this criterion would allow us to provide a comparative study with some of the previous quantitative ones (e.g., Kroeker et al., 2013; Nagelkerken and Connell, 2015; Sampaio et al., 2021). However, we tested the effects of the magnitude of pH changes on the response estimates in a separate dataset. In the context of ocean acidification research, the carbonate system should be appropriately manipulated according to the practice guide proposed by LaRoche et al. (2010). To be included in our study, the allowed maximal drift over the experiment was 10% for dissolved inorganic carbon (DIC) and 0.1 units for pH, as the drawdown of carbonates may decrease the alkalinity, and lead to strong deviation in the seawater carbonate chemistry and pH from the target chemistry according to the RCP 8.5 emission scenario (LaRoche et al., 2010). Studies in which the pH was manipulated using acid addition were excluded from the analysis. For heavy metals, the treatment without heavy metals or with the lowest level tested was considered the control. Each higher concentration of heavy metals was taken individually as an experimental treatment.
Studies that did not report, or where it was impossible to determine, the data variation (Std. dev., SEM, CIs or variance) or sample size (absence or pseudo-replication) were not included, according to the Preferred Reporting Items for Systematic Reviews and Meta-analyses guidelines (PRISMA) (O’Dea et al., 2021).
Data Collection
Datapoints, error estimates (variance or std. dev.), and sample sizes were extracted from published values or relevant figures using GetData Graph Digitizer.1 For the meta-analysis, all extracted error estimates were transformed to standard error. To meet the statistical assumption of independence among observations in the meta-analysis (Hedges et al., 1999), we either collected data at the endpoint (e.g., cell numbers) or derived them using a rate (e.g., growth) reported at multiple time intervals along the study. If an experiment reported the same biological response through different metrics at several time points, only the most inclusive metric for that response variable was considered to avoid pseudo-replication (Kroeker et al., 2013). Although a common strategy in ecological meta-analyses is to avoid non-independence by extracting only a single data point per measurement time series (Curtis et al., 2013), the limited number of studies reporting the responses as a function of time did not allow us to conduct a robust analysis to include the time as a variable.
Data on different biological responses to stressors assessed in the literature included: growth, abundance, survival, metabolism, photosynthesis, concentrations of biochemical compounds, and enzymatic rates. The wide range of these responses was classified into categories as described by Jin et al. (2019) with minor modifications, including (1) metabolism, the changes in metabolic measurements, (2) growth; (3) photosynthesis (e.g., photosynthetic oxygen evolution rate); (4) cellular/molecular, including the concentrations of biochemical compounds (e.g., soluble proteins), and gene expression; (5) oxidative stress, the activities of antioxidant enzymes [e.g., superoxide dismutase (SOD)] and oxidative stress biomarkers [e.g., levels of malondialdehyde (MDA)]; and (6) pigments, such as carotenoids and chlorophylls a, b, c. In addition to biological responses, data were subdivided into subsets according to (1) taxonomical groups (corals, fish, microalgae, macroalgae, molluscs, and polychaetes); (2) climate region where the organisms reside (temperate and tropical); (3) and the type of heavy metals (Cd, Cu, Co, Zn, Ni).
Effect Size Calculation
We calculated individual, main, and interactive effect sizes for each test using Hedge’s d (Hedges and Olkin, 1985) and followed the methods described by Gurevitch et al. (2000). Individual effects refer to the response to a stressor alone relative to the control, while main effects compare the net effect of a stressor with or without a second stressor. The individual effects of ocean acidification (doa) and heavy metals (dhm) were calculated with respect to the control (dc) using the equations (Gurevitch and Hedges, 1993; Crain et al., 2008):
where Yoa, Yhm, and Yc are means of a variable in the treatment groups of OA, heavy metals, and the control, respectively, s and J(m) are the pooled standard deviation and correction term for small samples, respectively, which were calculated as shown below:
where nc, noa, nhm, and noa+hm are the sample sizes, and sc, soa, shm, and soa+hm are the standard deviations in the control and experimental groups of OA, HM and their combination (OA + HM), respectively, m is the degree of freedom (m = nc + noa + nhm + noa+hm − 4). The main effects of OA (dOA), HM (dHM), and their interaction (dOA+HM) were obtained using the equations:
For individual effects dz (where z is oa or hm), the sampling variance is Gurevitch et al. (2000):
and for a main effect dZ (where Z is OA or HM), the sampling variance is Gurevitch et al. (2000):
The interaction variance vZ (where Z is OA+HM) was calculated as:
If the response traits indicate stress [e.g., activity of superoxide dismutase, contents of malondialdehyde (MDA) and glutathione (GSH)], the effect sizes calculated above were converted using the formula: 0-d, where d is the main or individual effect of OA, heavy metals, or their interaction.
We used individual effect sizes to classify the interactions of OA and HM into additive, synergistic or antagonistic interactions by following the approach proposed by Crain et al. (2008). In brief, if the 95% confidence interval (CI) of the interaction term overlapped with zero, the interactive effect was considered additive. If individual effects were either both negative or one positive and one negative, interaction effects < 0 were classified as synergistic while effects > 0 were antagonistic. Interactions were interpreted the opposite way when both stressors had a positive individual effect, i.e., interaction effects < 0 were antagonistic and > 0 were synergistic (Piggott et al., 2015).
Statistical Analyses
All analyses were performed with the statistical software R, using the function rma.mv (meta-analysis via multivariate/multilevel linear mixed-effects models) available in the metafor package (Viechtbauer, 2010). Firstly, mean interaction effect sizes and variances across studies were estimated from weighted meta-analyses using the function escalc. In every independent analysis, “Assessment ID” was treated as a random-effect to account for the random component of effect size variation among assessments (Supplementary Table 3). In addition to random-effects meta-analyses to assess the global mean interaction effect sizes across all assessments, we performed a series of mixed effects meta-analyses where selected categorical moderators (e.g., taxon, response trait, climate region) were treated as fixed effects to assess mean interactions at each category level afterward as previously described in Jin et al. (2019) (see Supplementary Table 3 for model terms). Categories with sample sizes smaller than four (n < 4) were not included in the analysis to ensure a robust analysis.
We hypothesized that the magnitude of heavy metal concentrations used in experiments may influence the effect size or govern the type of interaction between stressors, so we determined the concentrations of heavy metals for each data point where possible. Subsequently, a continuous random-effects meta-analysis was run to test the effect of heavy metal concentration (as a continuous variable) on the effect size. Since the ranges of concentrations varied greatly between heavy metals (for example, 0–150 μg L–1 for Cu and 0–15,000 μg L–1 for Cd), a separate random-effects meta-analysis was conducted for each heavy metal.
The data of different magnitudes of pH changes (see Supplementary Table 2) was used to perform continuous random-effects meta-analyses to test the effects of the magnitude of pH change on our response estimates. The frequencies of interaction types were calculated for different magnitudes of pH change that were classified into two categories: (a) RCP 8.5 scenario orientated, in which the pH reduction compared to the control was ∼0.4 and (b) beyond RCP 8.5 scenario, where the pH reduction was > 0.4. The frequencies of different interaction types were compared using a chi-square test.
To assess the robustness of observed effects, we tested publication bias using Rosenthal’s method of fail-safe numbers (Rosenthal, 1979) as described in Jin et al. (2019). We estimated a fail-safe number of 77,897, which far exceeds the minimum recommended number based on our sample size [77,897 > 5(n)+10, where n is the number of meta-analysis observations]. In addition, using the trim and fill method (Duval and Tweedie, 2000), we tested how much impact a potential publication bias could have. The trim and fill analysis failed to identify any missing studies needed to restore symmetry (missing studies = 0). Based on the results of those two tests, we concluded there was no evidence of publication bias for the data set compiled.
Results
Database Description
Initially, we found 558 observations of organisms’ responses to OA and heavy metals in 19 articles (data sources can be found in Supplementary Table 1). However, five articles were excluded from the analysis because the pH reduction exceeded 0.4, which did not meet the selection criterion described for this analysis (see section “Materials and Methods”). Hence, 14 articles reporting 298 observations were included in the present study (Supplementary Table 2). The majority of assessments were from macroalgae (32%) and molluscs (39%), with only six assessments recorded for polychaetes (Supplementary Table 2). Based on the selection criteria, our analysis included five different heavy metals, which were Cd (n = 140), Co (n = 6), Cu (n = 120), Zn (n = 23), and Ni (n = 9) (Figure 1). Most of the data points stem from studies with organisms from temperate regions (n = 210), with only 59 observations from tropical regions. In comparison, for the remaining 29 observations, the study location was not reported. Most of the studies were short-term (∼1–2 weeks), with the longest experiment lasting 49 days with the fish species Paralichthys olivaceus (Cui et al., 2020).
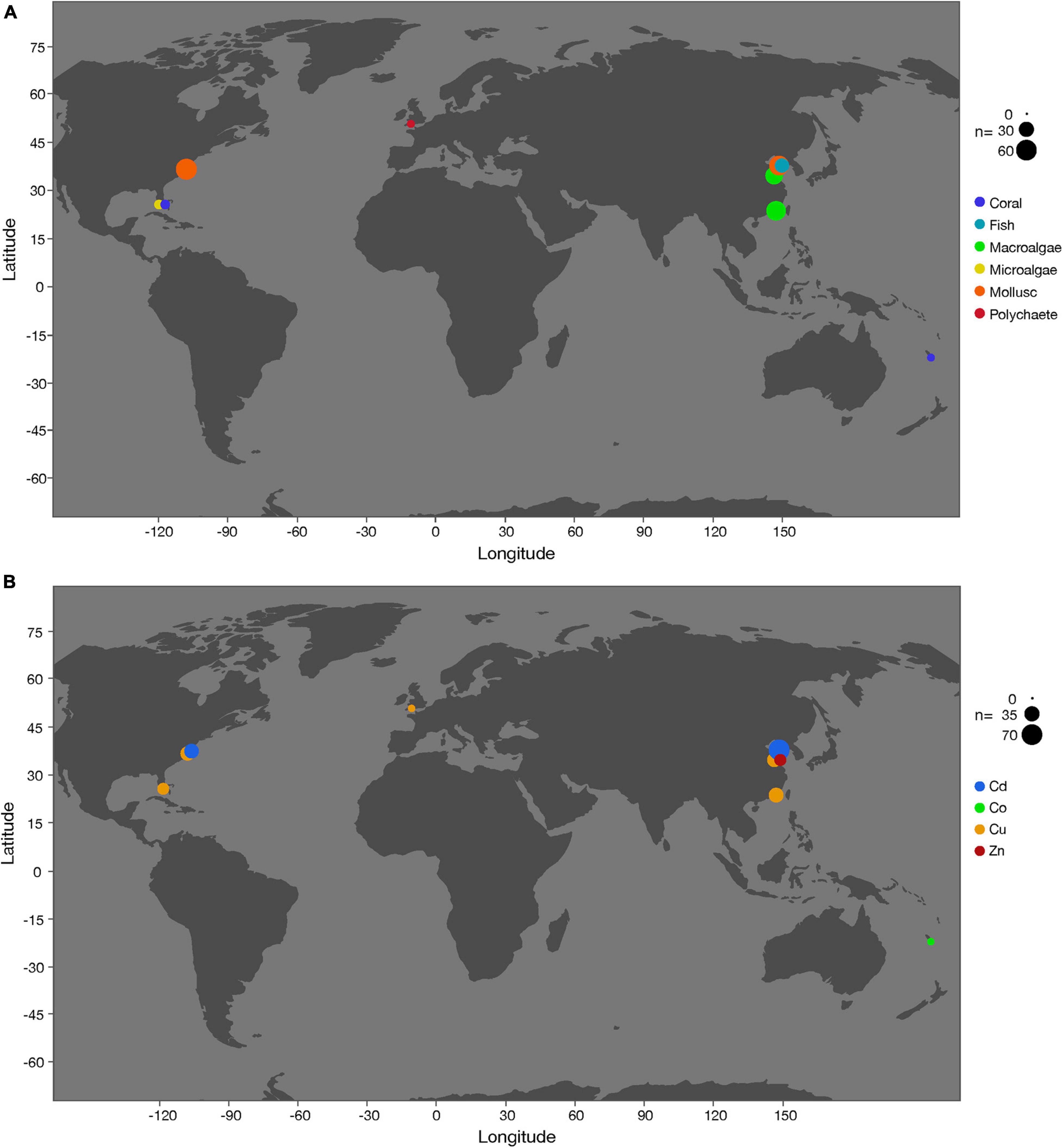
Figure 1. Locations of field studies that investigated the combined effects of ocean acidification and heavy metals (Cd, Co, Cu, Zn) on coral, fish, macroalgae, microalgae, mollusc, and polychaete. Symbol sizes indicate the number of observations. (A) By different taxonomic groups. (B) By different heavy metals.
Overall Analysis
Overall, our results showed a significant (i.e., the 95% CIs did not overlap with zero) negative effect of heavy metals, dHM (Effect size = −0.427, CI = [−0.761, −0.093], Z = −2.503, p = 0.012) (Figure 2 and Supplementary Table 3). However, the 95% CI of the main effect of OA, dOA, and the overall interaction term, dOA+HM, overlapped with zero (dOA: Effect size = 0.137, CI = [−0.063, 0.336], Z = 1.345, p = 0.179; dOA+HM: Effect size = −0.203, CI = [−0.461, 0.055], Z = 1.540, p = 0.124). These results suggest that the negative effects of heavy metals on marine biota were alleviated by OA, leading to a neutral effect of heavy metals in combination with OA (Figure 2). Regarding the interaction types, our results showed that additive effects dominated (67%), with small fractions of antagonistic (25%) and synergistic interactions (8%) (Figure 2). The small proportion of antagonistic interactions outweighed additive and synergistic interactions, which led to limited responses when heavy metals and OA acted together (Figure 2).
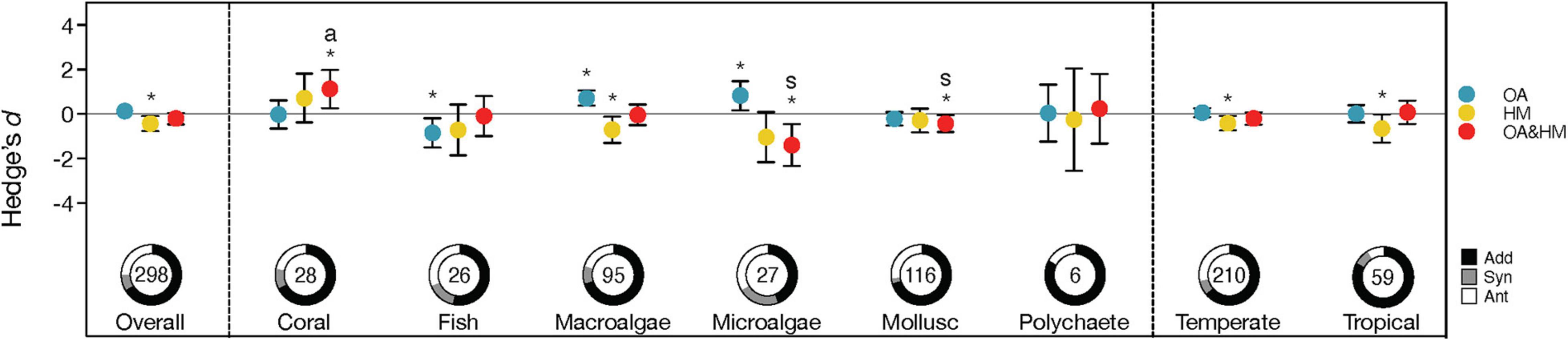
Figure 2. Responses to the effects of ocean acidification (OA) (blue), heavy metals (HM) (yellow) and their interaction (Hedge’s d; red) observed for the overall dataset, different taxa and climatic regions. Error bars indicate 95% confidence intervals. For the main effects of ocean acidification and heavy metals, confidence intervals overlapping zero indicate no effect; effects are positive when confidence interval (CI) > 0 and negative if < 0. Significant effects are denoted by asterisks (antagonistic interactions are highlighted with the letter “a”, synergistic interactions are highlighted with the letter “s”). Pie charts indicate the frequencies (%) of additive (black), synergistic (gray) and antagonistic (white) interaction types. Numbers inside pie charts indicate the number of observations.
Overall, there were significant differences among taxonomic groups when assessing their responses to OA (QM = 29.214, p < 0.001) (Supplementary Table 3). Specifically, OA showed positive effects on microalgae (Effect size = 0.830, CI = [0.175, 1.485], Z = 2.482, p = 0.013) and macroalgae (Effect size = 0.719, CI = [0.373, 1.064], Z = 4.081, p < 0.001), but had significant negative effects on fish (Effect size = −0.845, CI = [−1.503, −0.186], Z = −2.514, p = 0.012). No significant effects of OA were found for corals, molluscs and polychaetes (all p > 0.05) (Figure 2). For heavy metals, we identified that only macroalgae were negatively impacted (Effect size = −0.703, CI = [−1.297, −0.109], Z = −2.392, p = 0.02), while the remaining taxonomic groups were more tolerant with no significant responses to heavy metals (all p > 0.05) (Figure 2). However, when OA and heavy metals acted together, microalgae (Effect size = −1.392, CI = [−2.331, −0.453], Z = −2.905, p = 0.004) and molluscs (Effect size = −0.431, CI = [−0.814, −0.049], Z = −2.208, p = 0.027) showed significant negative responses, while the performance of corals was improved (Effect size = 1.129, CI = [0.272, 1.987], Z = 2.581, p = 0.01) (Figure 2). Other taxonomic groups showed limited responses to the combination of OA and heavy metals (Figure 2). In line with these differences, our results showed significant differences between the frequencies of the interaction types among different taxonomic groups (χ2 = 19.305, p = 0.037, df = 10, n = 298) (Figure 2). Despite these differences, additive interactions between OA and HM dominated in all taxonomic groups, varying from 44 to 83% (Figure 2). Antagonisms were most frequent in microalgae (∼33%), followed by fish (31%) and molluscs (27%). Synergistic interactions were the least frequent interaction type in all taxonomic groups (0–22%) (Figure 2).
Organisms from either temperate or tropical regions showed no significant response to OA (Both 95% CIs overlapped with zero) (QM = 0.056, p = 0.813) (Figure 2 and Supplementary Table 3). Heavy metal exposure exerted significant negative effects on organisms from temperate (Effect size = −0.409, CI = [−0.741, −0.078], Z = −2.420, p = 0.016) and tropical regions (Effect size = -0.649, CI = [−1.277, −0.021], Z = −2.025, p = 0.043) but the responses did not differ between these two regions (QM = 0.436, p = 0.509) (Figure 2 and Supplementary Table 3). Interactive effects of OA and heavy metals were not significant in either climate region (both p > 0.05) (Figure 2). Although the majority of interactions were additive in both climate regions (64% in temperate and 83% in tropical regions), there were significant differences between the frequencies of interaction types (χ2 = 11.600, p = 0.003, df = 2, n = 269) (Figure 2). Antagonisms showed a considerably higher proportion in temperate marine organisms (28%) than in tropical organisms (8%).
Differences Among Response Traits
The magnitude of effect sizes varied among different response traits when exposed to OA (QM = 86.976, p < 0.001) or heavy metals alone (QM = 58.582, p < 0.001) (Figure 3 and Supplementary Table 3). OA had positive effects, with an overall positive Hedge’s d identified for photosynthesis, growth and cellular/molecular of marine organisms (all p < 0.05) (Figure 3). In contrast, heavy metal exposure dramatically decreased their photosynthesis and growth, with growth having the greatest reduction (Effect size = −3.260, CI = [−4.801, −1.718], Z = −4.144, p < 0.001) (Figure 3). Oxidative parameters also showed a significant negative response to OA or heavy metals alone (OA: Effect size = −0.497, CI = [−0.744, −0.251], Z = −3.949, p < 0.001; heavy metals: Effect size = −0.987, CI = [−1.422, −0.552], Z = −4.446, p < 0.001), indicating oxidative damage in marine organisms under OA or heavy metals exposure. No effects were detected in the response traits metabolism and pigmentation in response to OA or heavy metals (both p > 0.05) (Figure 3). Under combined OA and HM exposure, only oxidative parameters showed a significant negative response (Effect size = −0.532, CI = [−0.89, −0.173], Z = −2.907, p = 0.004), indicating that marine organisms exhibited oxidative stress under co-exposure (Figure 3). The other response traits showed neutral responses to combined OA and heavy metal exposure, and the differences among traits were not significant sources of variation in the analysis (QM = 9.035, p = 0.108) (Supplementary Table 3).
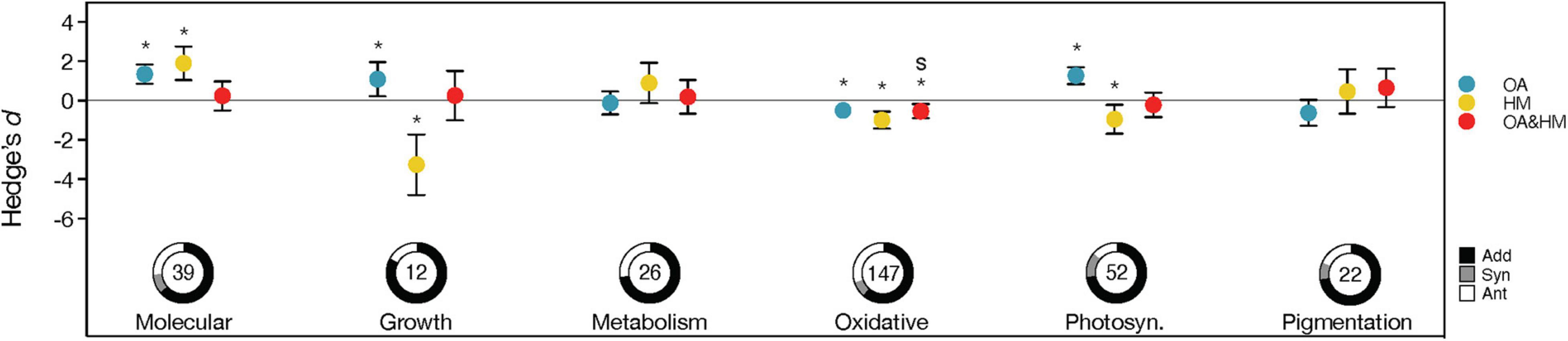
Figure 3. Responses to the effects of ocean acidification (OA) (blue), heavy metals (HM) (yellow) and their interaction (Hedge’s d; red) observed for organismal response levels. For interactions, confidence intervals overlapping 0 indicate additive effects; those > 0 or < 0 indicate a significant interaction (synergistic interactions are highlighted with the letter “s”). Descriptions of the error bars, pie charts, symbols and color coding see Figure 2.
Additive interactions were prevalent in all response traits (62–83%), and the frequencies of interaction types did not differ among various response traits (χ2 = 15.040, p = 0.131, df = 10, n = 298). The negative effects of heavy metals on some response traits, such as growth, were compensated by OA because most interactions were additive. The substantial proportion of antagonistic interactions in some response traits (e.g., cellular/molecular) led to limited responses under combined OA and heavy metals exposure.
Differences Among Various Heavy Metals
Due to the various properties of different heavy metals, we hypothesized that marine organisms might respond differently to heavy metals alone than to co-exposure with OA. Our results showed that marine organisms were more sensitive to Cd and Cu than to other heavy metals, showing significant negative responses (Cd: Effect size = −0.592, CI = [−1.075, −0.110], Z = −2.405, p = 0.016; Cu: Effect size = −0.653, CI = [−1.175, −0.131], Z = −2.453, p = 0.014) (Figure 4). Under combined OA and heavy metal exposure, the severity of effects on organisms varied greatly between different heavy metals (QM = 10.764, p = 0.029) (Supplementary Table 3). The performances of marine organisms were negatively impacted when Cd and OA acted in a combination (Effect size = −0.382, CI = [−0.753, −0.011], Z = −2.016, p = 0.044). For the other heavy metals, their combined effects with OA appeared to be neutral (all p > 0.05) (Figure 4). Similarly, we found that the frequencies of interaction types differed significantly among various heavy metals (χ2 = 15.725, p = 0.047, df = 8, n = 298) (Figure 4). Additive interactions accounted for 66–78% of observations and constituted the majority of interactions. The only exception presented the heavy metal Zn, which most frequently interacted antagonistically with OA (52%) (Figure 4). Synergistic interactions were the least frequent (0–17% of observations).
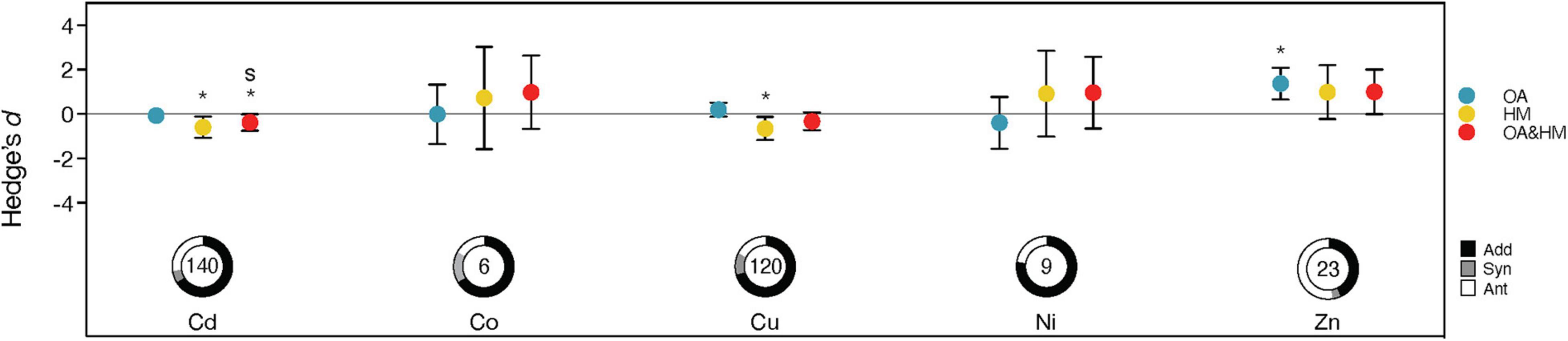
Figure 4. Responses to the effects of ocean acidification (OA) (blue), heavy metals (HM) (yellow) and their interaction (Hedge’s d; red) observed for different metal types. For interactions, confidence intervals overlapping 0 indicate additive effects; those > 0 or < 0 indicate a significant interaction (synergistic interactions are highlighted with the letter “s”). Descriptions of the error bars, pie charts, symbols and color coding see Figure 2.
Effects of the Magnitude of pH Reduction on Effect Size
To assess whether the degree of acidification influences the effect size, we mined data of different magnitudes of pH reduction and tested their relationship using general linear models (Figure 5). The influence of the magnitude of pH reduction was not consistent across taxonomic groups (Figure 5). In macroalgae, we found that either the responses to OA or heavy metals alone, or to their combination were all negatively correlated with pH reductions, suggesting the positive effects of OA alone on macroalgae would turn to be negative under an extreme OA scenario (Supplementary Table 4 and Figure 5). In contrast, these relationships were positive in molluscs, indicating that the individual effects of OA or heavy metals or their combined effects were likely to be buffered or amplified (depending on the response direction, i.e., positive or negative) by further pH reductions (Supplementary Table 4 and Figure 5). We further quantified whether the frequency of the interaction types differs among different magnitudes of pH change. Our results showed that the frequency of the interaction types was similar between the RCP 8.5 scenario and the scenario beyond RCP 8.5 (see definitions in Materials and Methods) (χ2 = 0.026, p = 0.987, df = 2, n = 558) (Figure 6). In summary, our data suggest that various taxonomic groups would respond differentially to different degrees of OA, with some taxa being more negatively impacted under more extreme OA scenarios, although the interaction types are not likely to change.
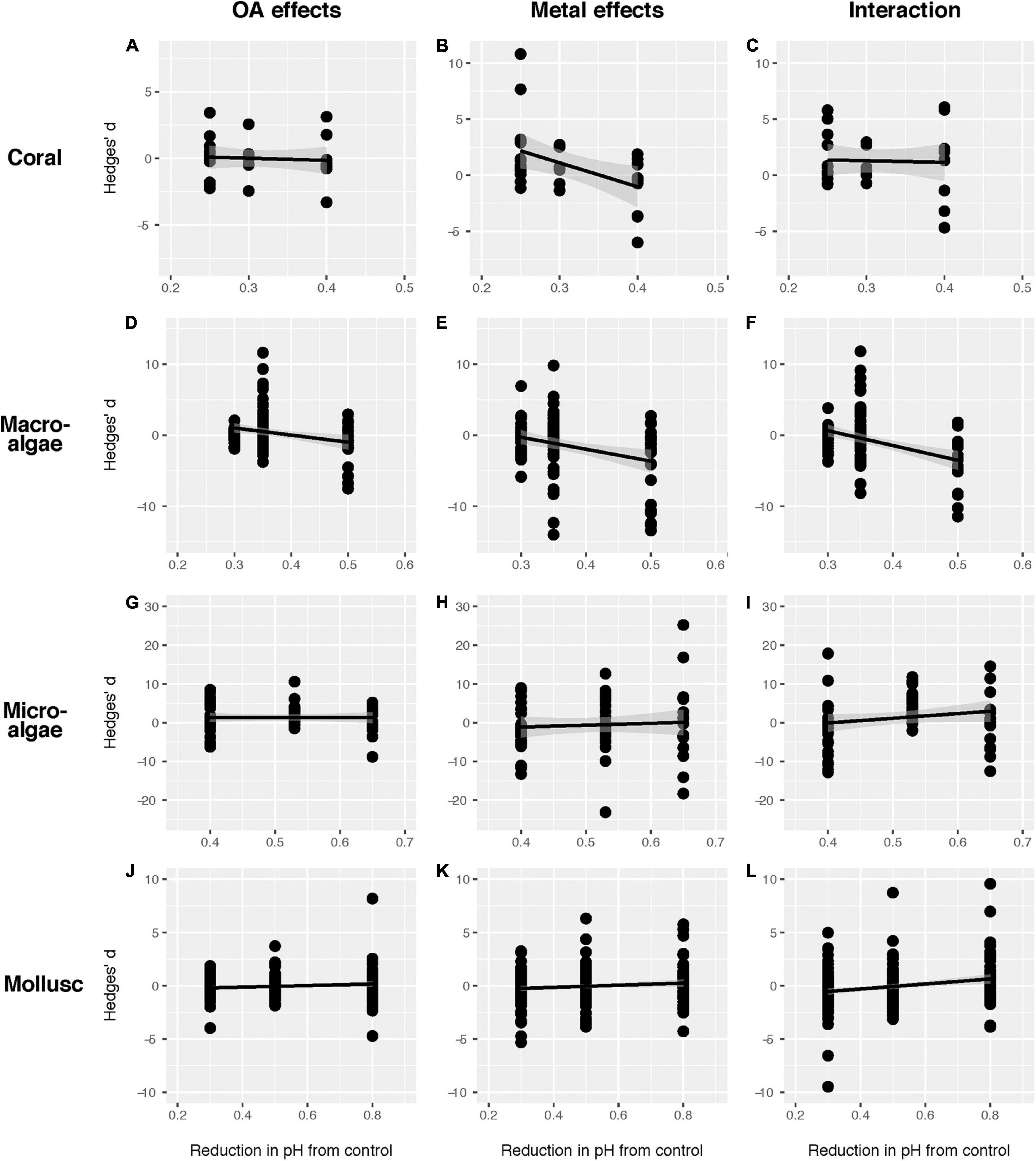
Figure 5. The effect of magnitude of pH reduction on the effect size (Hedge’s d) of ocean acidification (OA) (A,D,G,J), heavy metals (B,E,H,K) and their interaction (C,F,I,L) in various taxonomical groups. (A–C) Coral; (D–F) macroalgae; (G–I) microalgae; (J–L) mollusc. The data were fitted with linear regressions.
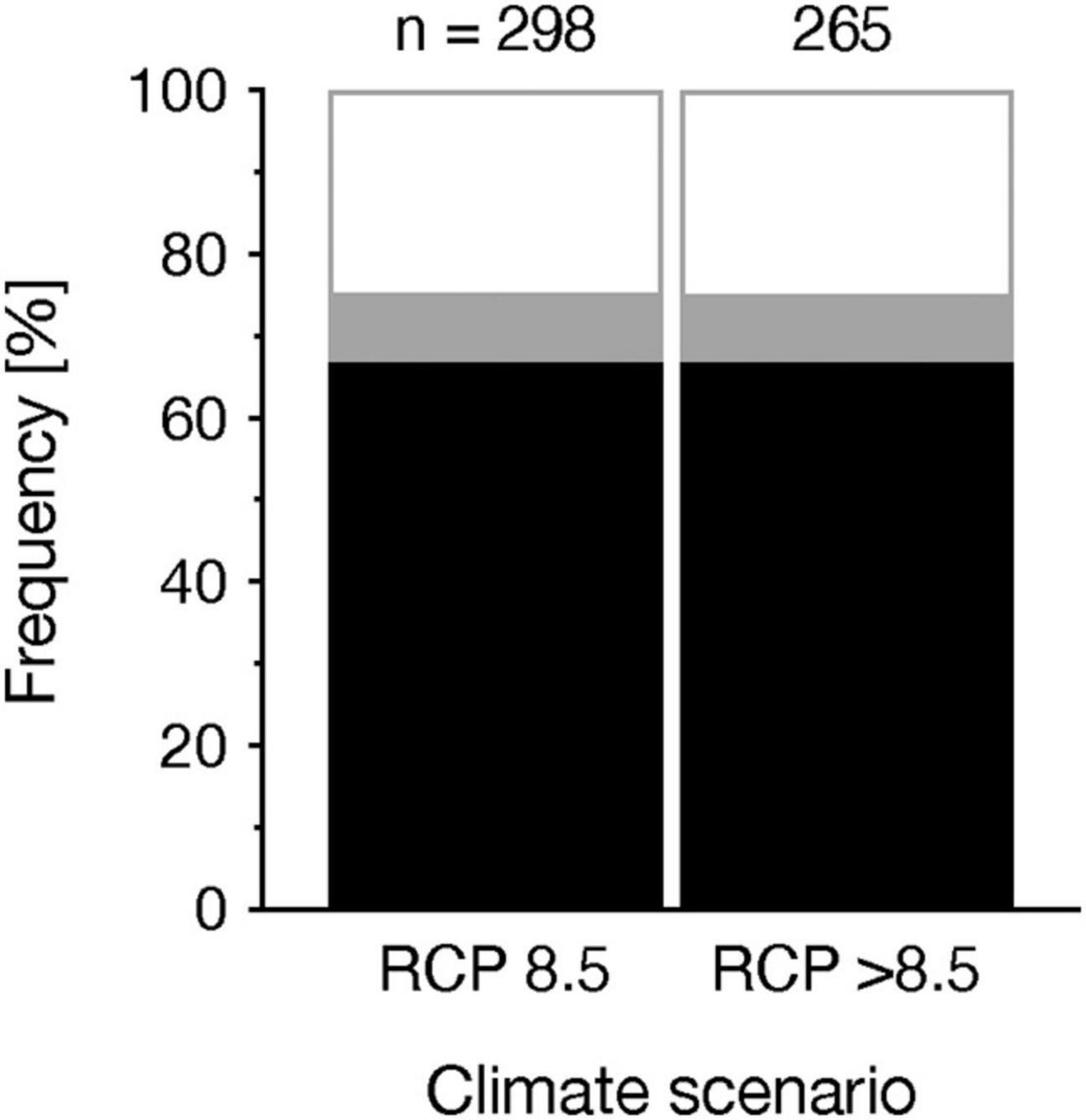
Figure 6. Frequency (in %) of additive (black), synergistic (gray) and antagonistic (white) interactions depending on climate scenario. Values above bars denote the number of observations.
Influence of Heavy Metal Concentration on Effect Size
It was not surprising that the performances of marine organisms were more negatively impacted at higher Cu concentrations (Supplementary Table 5 and Figure 7). The same results could be observed when Cu or Cd acted together with OA. We also found that the sensitivity of marine organisms to Cu concentrations (slope: −0.028) was doubled in response to Cu alone compared to the combination of Cu and OA (−0.014), suggesting that OA buffered the adverse effects of Cu on marine biota with increasing Cu concentrations. However, we did not observe such a trend for other heavy metals included in the present study, such as Ni and Zn, probably due to the limited number of data points at various concentrations (Supplementary Table 5 and Figure 7).
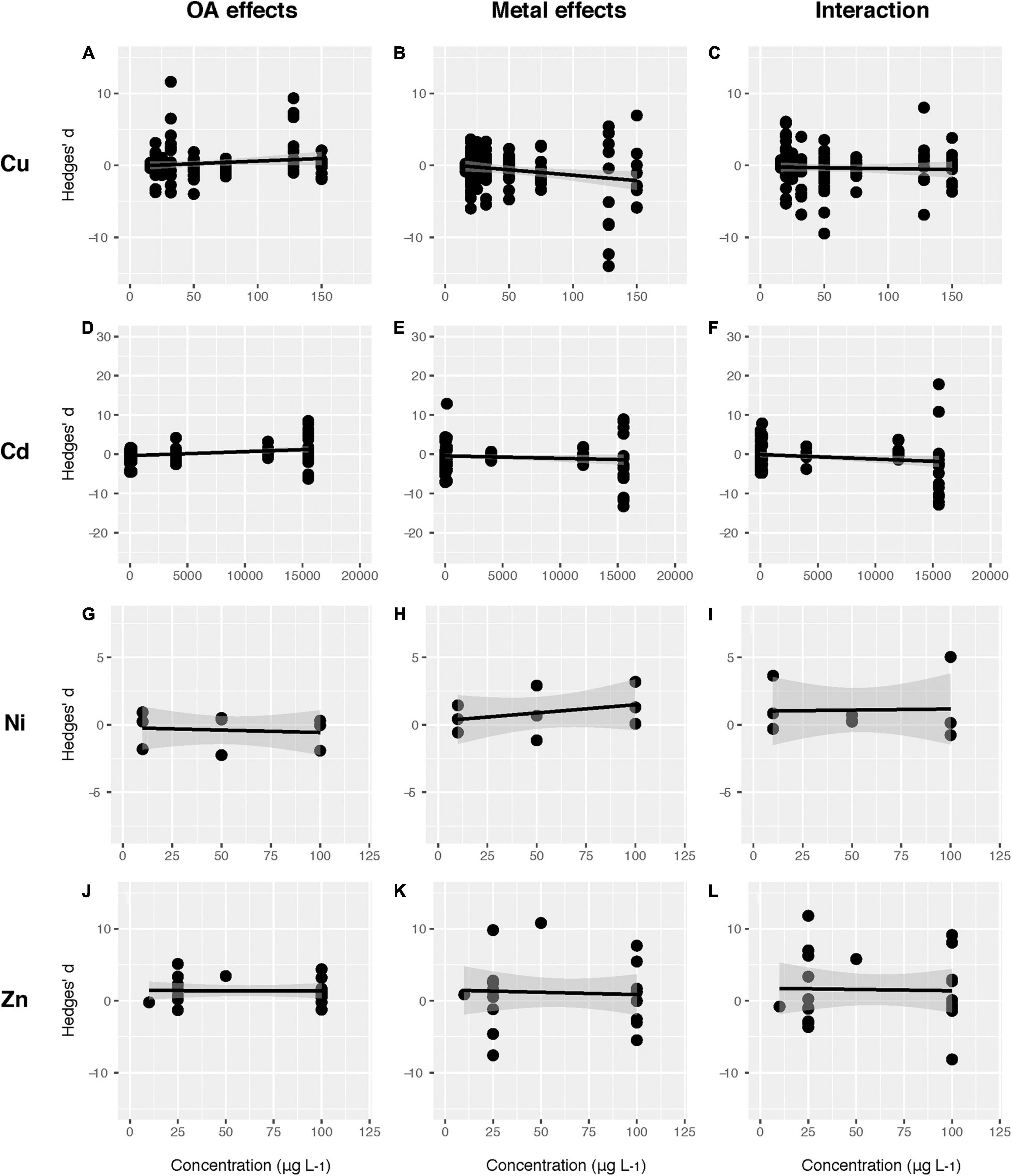
Figure 7. The effect of heavy metal concentrations (μg L– 1) on the effect size (Hedge’s d) of ocean acidification (OA) (A,D,G,J), heavy metals (HM) (B,E,H,K) and their interaction (C,F,I,L). (A–C) Cu; (D–F) Cd; (G–I) Ni; (J–L) Zn. The data were fitted with linear regressions.
Discussion
Our meta-analysis revealed a prevalence of additive interactions between OA and heavy metals, indicating that cumulative effects of OA and heavy metals on marine biota can be reasonably well assessed by summing the single stressor impacts. The prevalence of additive interactions discovered in the present study agrees well with previous meta-analysis studies, in which most stressor interactions were additive across experimental studies (Darling and Côté, 2008; Przeslawski et al., 2015; Jin et al., 2019; Steckbauer et al., 2020), while overall synergisms or antagonisms were uncommon (Burkepile and Hay, 2006; Stephens et al., 2013; Jackson et al., 2016; Yue et al., 2017). Because OA had positive effects on organisms and most frequently interacted additively with heavy metals, our analysis found that OA alleviated the harmful effects of heavy metals, leading to an overall neutral effect co-exposure. Heavy metal exposure can exert deleterious impacts on various physiological processes (such as photosynthesis, metabolism, enzymic activity, immunity), growth, reproduction, development and survival of marine organisms (Ivanina and Sokolova, 2015 and references therein; Gao et al., 2017; Dong et al., 2020; Wen and Zou, 2021). However, these harmful impacts can be mitigated by OA, as documented in our comprehensive analysis and many previous experimental studies. For instance, when oysters were exposed to Cd, the trypsin-like and caspase-like activities of the proteasome were largely inhibited, suggesting a decreased protein synthesis and turnover (Götze et al., 2014), although the energy demand also decreased (Fraser and Rogers, 2007; Deigweiher et al., 2009). However, OA was found to reverse this negative effect of Cd on the oyster proteasome (Götze et al., 2014). Thus, OA and heavy metals acted oppositely on proteasome activities and energy metabolism and hence the energy status was maintained in oysters co-exposed to OA and Cd (Götze et al., 2014).
The mitigating effects of OA on heavy metal toxicity to marine organisms (especially in photosynthetic organisms) can, on one hand, be explained by the acid-base balance. It has been extensively reported that many marine microalgae have evolved CO2 concentrating mechanisms (CCMs) to supply CO2 to Rubisco (Giordano et al., 2005; Raven et al., 2011; Reinfelder, 2011). CCMs require energy for the active uptake of HCO3– and CO2 and maintaining high intracellular Ci levels by counteracting CO2 efflux (Raven, 1991; Hopkinson et al., 2011). CCMs are known to be down-operated (by ∼20%) under OA conditions (Trimborn et al., 2009; Hopkinson et al., 2011; Gao et al., 2012). Consequently, the conserved energy from CCMs’ down-regulations can be used to repair damage induced by heavy metals and then counteract the deleterious impacts of heavy metals. On the other hand, it was suggested that H+ may decrease the toxicity of metals by competitively excluding them from building ligands at the cell surface (Franklin et al., 2000; Gao et al., 2017). The competition between hydrogen ions and some heavy metals such as Cu at the cell surface outcompetes the increased availability of heavy metals caused by decreased pH under OA conditions and hence alleviates the adverse effects of heavy metals (Gao et al., 2017).
However, it is worth noting that marine organisms also need to spend extra energy to maintain the cells’ homeostasis or net H+ efflux under ocean acidification conditions (Suffrian et al., 2011; Raven and Crawfurd, 2012; Jin et al., 2015). Therefore, the mitigation effect of OA on heavy metals also depends on the balance between energy saving and expenditure due to OA. One may hypothesize that the additional energy cost would surpass the energy saving in more extreme OA scenario conditions, thus the net effects of OA would turn to be negative. This hypothesis was evidenced in the present study, in which more pronounced effects were detected in more extreme OA scenarios (i.e., a larger pH reduction) (Figure 5). Consequently, the mitigation effects of OA on the toxicity of heavy metals will be muted under more extreme OA conditions. It has been recognized that the acidification rate of coastal waters is faster than that of the open ocean (Cai et al., 2011), and some coastal waters are experiencing high diel pH fluctuations (>1 pH unit) (Duarte et al., 2013). Therefore, future research should include individual case studies investigating the interactive effects of ocean acidification and heavy metals, and especially studies focusing on coastal water habitats. Moreover, the relationship between the magnitude of pH changes and responses is likely to be nonlinear, and more pronounced changes have been observed in more severe OA conditions (Scheffer and Carpenter, 2003; Ries et al., 2009; Christen et al., 2012). Therefore, it may add to the difficulties in assessing the impacts of multiple stressors (OA + heavy metals) on marine biota.
Besides additive interactions being the most frequent interaction type, our analysis also revealed considerable proportions of non-additive, antagonistic interactions in some marine taxa (e.g., 33% in microalgae and 31% in fish). Thus, this is likely to be a co-adaptation, with marine organisms minimizing the multiple stressors’ net effects (Jackson et al., 2016). Therefore, identifying the primary stressor that drives antagonistic interactions would be essential to predict their overall impacts on marine biota (Piggott et al., 2015). Even though we found that OA can alleviate the adverse effects of heavy metals, the efforts to reduce the exposures of marine organisms to OA and heavy metals should not be lessened as the net effects were still negative in some marine taxa, such as molluscs and microalgae as demonstrated in the present study.
The frequency of interaction types was partially explained by taxonomic groups, climate regions and heavy properties. It is known that populations of the same species from different geographic locations differ in their ability to withstand environmental stress (Bozinovic et al., 2011; Li et al., 2016; Calosi et al., 2017; Vargas et al., 2017). For example, the coastal diatom species Thalassiosira weissflogii and the oceanic diatom Thalassiosira oceanica, which have acclimated to different pH fluctuation histories, responded differentially to OA (Li et al., 2016). Those contrasting responses of marine organisms from different geographic locations to OA may interact with other environmental stressors, leading to large variability in the cumulative effects of multiple stressors. Therefore, we propose that evaluating the cumulative effects of OA and heavy metals on marine biota within various taxonomic groups from different geographic locations is essential in assessing the impacts of multiple stressors in marine ecosystems.
Since most of the marine animals included in the present meta-analysis were at their adult stage, the limited information on other life stages such as larvae and embryos did not allow us to conduct a robust assessment to compare the different responses among various life stages. Since early developmental stages of marine organisms are the most sensitive stages to individual or multiple stressors (Kroeker et al., 2013; Ivanina and Sokolova, 2015; Przeslawski et al., 2015; Sampaio et al., 2021), and the effects of OA on metal accumulation and toxicity are dependent on the life stage of and organism, one may expect that the interaction type may differ across different development stages (Harvey et al., 2013). Thus, we contend that it is further warranted to assess the responses of marine organisms to multiple stressors across life stages, particularly early life stages, which are often difficult or intractable to investigate in field conditions.
Conclusion
In conclusion, our analysis of the research on interactive effects of ocean acidification and heavy metals reveals negative impacts of heavy metals but overall neutral effects when they acted together with OA on marine organisms, despite the variability in their taxonomic groups (Figure 8). Our syntheses suggest that the prevalence of additive interactions and a substantial proportion of antagonistic interactions meant that ocean acidification alleviates these negative effects. However, it should be noted that some marine taxa (such as molluscs and microalgae) were still negatively impacted when co-exposed to ocean acidification and heavy metals (Figure 8). Furthermore, the degree of ocean acidification and concentrations of heavy metals could potentially lessen or exacerbate the effects of heavy metals or ocean acidification, remaining critical areas for future research. In addition, the interaction types differed among organisms from different climatic regions and their variability was also dependent on the type of heavy metals. Future research is needed to understand whether the remaining variations could have been explained by the duration of exposure or life stage. Finally, marine organisms will be subjected to other potential stressors apart from ocean acidification and heavy metals in the future oceans. We recommend that future studies on the concurrent effects of ocean acidification, heavy metals, and other environmental stressors (such as warming and deoxygenation) in marine waters are necessary to predict marine organisms’ responses in future oceans.
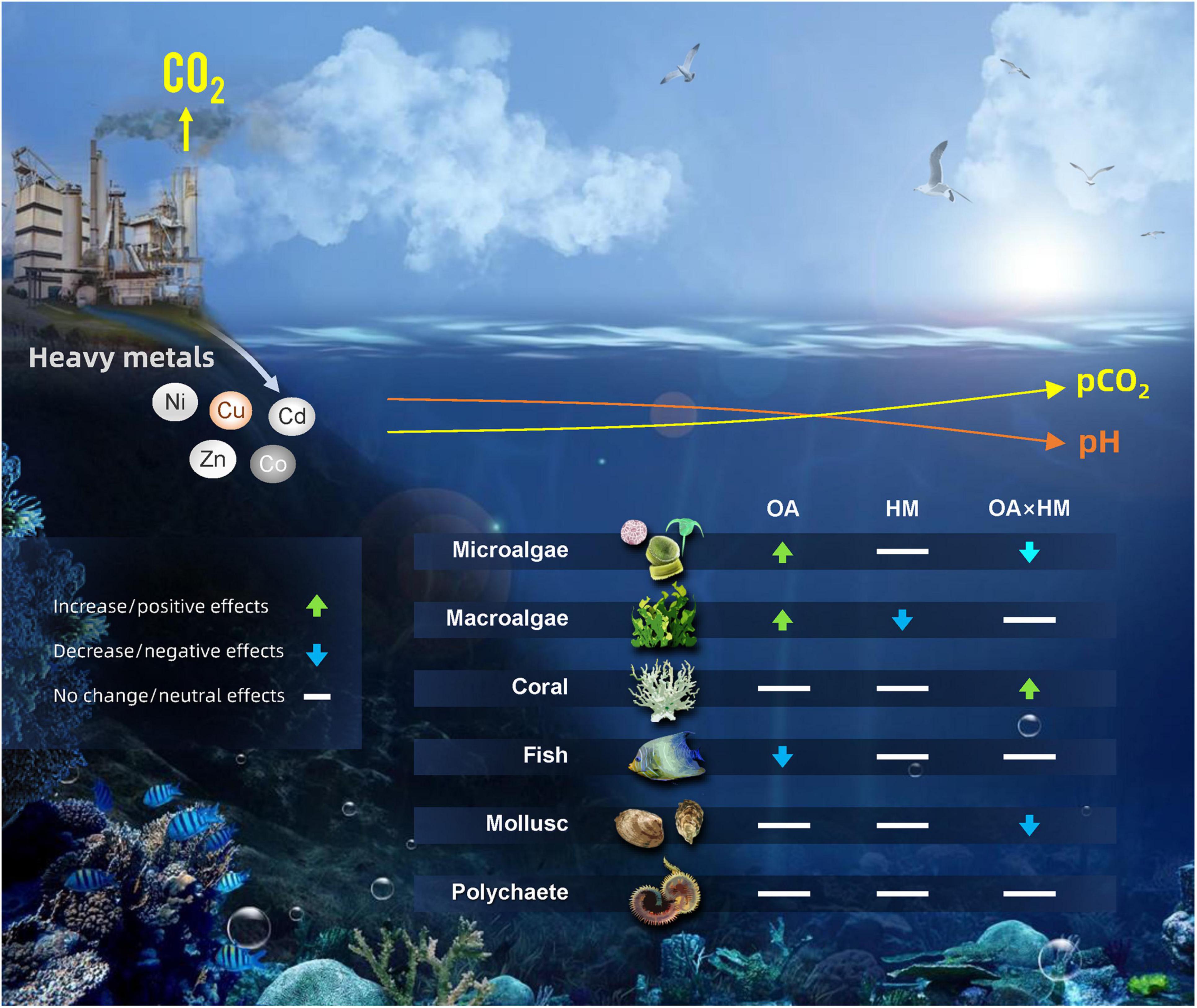
Figure 8. Conceptual diagram illustrating the effects of ocean acidification (OA), heavy metals (HM) and their combination on different taxa (microalgae, macroalgae, coral, fish, mollusc, polychaete) based on the meta-analysis results as shown in the various figures of our study. The arrows indicate the direction of change.
Data Availability Statement
The original contributions presented in the study are included in the article/Supplementary Material, further inquiries can be directed to the corresponding author/s.
Author Contributions
PJ and JX conceptualized the study. JiaZ, JW, PJ, MY, XD, JinZ, and MX acquired the data. PJ, SO, JiaZ, JW, and GG performed the statistical analysis. PJ and SO generated the figures. PJ drafted the manuscript. All authors discussed and edited the manuscript.
Funding
This study was supported by the National Natural Science Foundation of China (Nos. 41806141 and 41890803).
Conflict of Interest
The authors declare that the research was conducted in the absence of any commercial or financial relationships that could be construed as a potential conflict of interest.
Publisher’s Note
All claims expressed in this article are solely those of the authors and do not necessarily represent those of their affiliated organizations, or those of the publisher, the editors and the reviewers. Any product that may be evaluated in this article, or claim that may be made by its manufacturer, is not guaranteed or endorsed by the publisher.
Supplementary Material
The Supplementary Material for this article can be found online at: https://www.frontiersin.org/articles/10.3389/fmars.2021.801889/full#supplementary-material
Footnotes
References
Biscéré, T., Rodolfo-Metalpa, R., Lorrain, A., Chauvaud, L., Thébault, J., Clavier, J., et al. (2015). Responses of two scleractinian corals to cobalt pollution and ocean acidification. PLoS One 10:e0122898. doi: 10.1371/journal.pone.0122898
Bozinovic, F., Calosi, P., and Spicer, J. (2011). Physiological correlates of geographic range in animals. Annu. Rev. Ecol. Evol. Syst. 42, 155–179. doi: 10.1146/annurev-ecolsys-102710-145055
Burkepile, D. E., and Hay, M. E. (2006). Herbivore vs. nutrient control of marine primary producers: context-dependent effects. Ecology 87, 3128–3139. doi: 10.1890/0012-9658(2006)87[3128:hvncom]2.0.co;2
Cai, W., Hu, X., Huang, W., Murrell, M. C., Lehrter, J. C., Lohrenz, S. E., et al. (2011). Acidification of subsurface coastal waters enhanced by eutrophication. Nat. Geosci. 4, 766–770. doi: 10.1038/ngeo1297
Caldeira, K., and Wickett, M. E. (2003). Anthropogenic carbon and ocean pH. Nature 425:365. doi: 10.1038/425365a
Calosi, P., Melatunan, S., Turner, L., Artioli, Y., Davidson, R. L., Byrne, J. J., et al. (2017). Regional adaptation defines sensitivity to future ocean acidification. Nat. Commun. 8:13994. doi: 10.1038/ncomms13994
Campbell, A. L., Mangan, S., Ellis, R. P., and Lewis, C. (2014). Ocean acidification increases copper toxicity to the early life-history stages of the polychaete Arenicola marina in artificial seawater. Environ. Sci. Technol. 48, 9745–9753. doi: 10.1021/es502739m
Cao, R., Liu, Y., Wang, Q., Dong, Z., Yang, D., Liu, H., et al. (2018). Seawater acidification aggravated cadmium toxicity in the oyster Crassostrea gigas: metal bioaccumulation, subcellular distribution and multiple physiological responses. Sci. Total Environ. 642, 809–823. doi: 10.1016/j.scitotenv.2018.06.126
Cao, R. W., Zhang, T. Y., Li, X., Zhao, Y., Wang, Q., Yang, D., et al. (2019). Seawater acidification increases copper toxicity: a multi-biomarker approach with a key marine invertebrate, the Pacific Oyster Crassostrea gigas. Aquat. Toxicol. 210, 167–178. doi: 10.1016/j.aquatox.2019.03.002
Christen, N., Calosi, P., McNeill, C. L., and Widdicombe, S. (2012). Structural and functional vulnerability to elevated pCO2 in marine benthic communities. Mar. Biol. 160, 2113–2128. doi: 10.1007/s00227-012-2097-0
Crain, C. M., Kroeker, K., and Halpern, B. S. (2008). Interactive and cumulative effects of multiple human stressors in marine systems. Ecol. Lett. 11, 1304–1315. doi: 10.1111/j.1461-0248.2008.01253.x
Cui, W., Cao, L., Liu, J., Ren, Z., Zhao, B., and Dou, S. (2020). Effects of seawater acidification and cadmiumon the antioxidant defense of flounder Paralichthys olivaceus larvae. Sci. Total Environ. 718:137234. doi: 10.1016/j.scitotenv.2020.137234
Curtis, P. S., Mengersen, K., and Lajeunesse, M. J. (2013). “Extraction and critical appraisal of data,” in Handbook of Meta-Analysis in Ecology and Evolution, eds J. Koricheva, J. Gurevitch, and K. Mengersen (New Jersey, NJ: Princeton), 52–60. doi: 10.1371/journal.pmed.1001744
Darling, E. S., and Côté, I. M. (2008). Quantifying the evidence for ecological synergies. Ecol. Lett. 11, 1278–1286. doi: 10.1111/j.1461-0248.2008.01243.x
Davis, A. P., Shokouhian, M., and Ni, S. B. (2001). Loading estimates of lead, copper, cadmium and zinc in urban runoff from specific sources. Chemosphere 44, 997–1009. doi: 10.1016/s0045-6535(00)00561-0
Deigweiher, K., Hirse, T., Bock, C., Lucassen, M., and Pörtner, H. O. (2009). Hypercapnia induced shifts in gill energy budgets of Antarctic notothenioids. J. Comp. Physiol. B 180, 347–359. doi: 10.1007/s00360-009-0413-x
Doney, S. C. (2010). The growing human footprint on coastal and open-ocean biogeochemistry. Science 328, 1512–1516. doi: 10.1126/science.1185198
Dong, F., Wang, P., Qian, W., Tang, X., Zhu, X., Wang, Z., et al. (2020). Mitigation effects of CO2-driven ocean acidification on Cd toxicity to the marine diatom Skeletonema costatum. Environ. Pollut. 259:113850. doi: 10.1016/j.envpol.2019.113850
Duarte, C. M., Hendriks, I. E., Moore, T. S., Olsen, Y. S., Steckbauer, A., Ramajo, L., et al. (2013). Is ocean acidification an open-ocean syndrome? Understanding anthropogenic impacts on seawater pH. Estuar. Coasts 36, 221–236. doi: 10.1007/s12237-013-9594-3
Duval, S., and Tweedie, R. (2000). Trim and fill: a simple funnelplot-based method of testing and adjusting for publication bias in meta-analysis. Biometrics 56, 455–463. doi: 10.1111/j.0006-341X.2000.00455.x
Filosto, S., Roccheri, M. C., Bonaventura, R., and Matranga, V. (2008). Environmentally relevant cadmium concentrations affect development and induce apoptosis of Paracentrotus lividus larvae cultured in vitro. Cell Biol. Toxicol. 24, 603–610. doi: 10.1007/s10565-008-9066-x
Flint, K. R., and Davis, A. P. (2007). Pollutant mass flushing characterization of highway stormwater run off from an ultra-urban area. J. Environ. Eng. 133, 616–626. doi: 10.1061/(ASCE)0733-93722007133:6(616)
Franklin, N. M., Stauber, J. L., Markich, S. J., and Lim, R. P. (2000). pH-dependent toxicity of copper and uranium to a tropical freshwater alga (Chlorella sp.). Aquat. Toxicol. 48, 275–289. doi: 10.1016/s0166-445x(99)00042-9
Fraser, K. P. P., and Rogers, A. D. (2007). Protein metabolism in marine animals: the underlying mechanism of growth. Adv. Mar. Biol. 51, 267–363. doi: 10.1016/S0065-2881(06)52003-6
Gao, G., Liu, Y., Li, X., Feng, Z., Xu, Z., Wu, H., et al. (2017). Expected CO2-induced ocean acidification modulates copper toxicity in the green tide alga Ulva prolifera. Environ. Exp. Bot. 135, 63–72. doi: 10.1016/j.envexpbot.2016.12.007
Gao, K., Beardall, J., Häder, D.-P., Hall-Spencer, J. M., Gao, G., and Hutchins, D. A. (2019). Effects of ocean acidification on marine photosynthetic organisms under the concurrent influences of warming, uv radiation, and deoxygenation. Front. Mar. Sci. 6:322. doi: 10.3389/fmars.2019.00322
Gao, K., Xu, J., Gao, G., Li, Y., Hutchins, D. A., Huang, B., et al. (2012). Rising CO2 and increased light exposure synergistically reduce marine primary productivity. Nat. Clim. Chang. 2, 519–523. doi: 10.1038/nclimate1507
Gattuso, J. P., Magnan, A., Billé, R., Cheung, W. W. L., Howes, E. L., Joos, F., et al. (2015). Contrasting futures for ocean and society from different anthropogenic CO2 emissions scenarios. Science 349:aac4722. doi: 10.1126/science.aac4722
Giordano, M., Beardall, J., and Raven, J. A. (2005). CO2 concentrating mechanisms in algae: mechanisms, environmental modulation, and evolution. Annu. Rev. Plant Biol. 56, 99–131. doi: 10.1109/68.769733
Götze, S., Matoo, O. B., Beniash, E., Saborowski, R., and Sokolova, I. M. (2014). Interactive effects of CO2 and trace metals on the proteasome activity and cellular stress response of marine bivalves Crassostrea virginica and Mercenaria mercenaria. Aquat. Toxicol. 149, 65–82. doi: 10.1016/j.aquatox.2014.01.027
Gurevitch, J., and Hedges, L. V. (1993). “Meta-analysis: combining the results of independent experiments,” in Design and Analysis of Ecological Experiments, eds S. Scheiner and J. Gurevitch (New York, NY: Chapman and Hall), 347–370.
Gurevitch, J., Morrison, J. A., and Hedges, L. V. (2000). The interaction between competition and predation: a meta-analysis of field experiments. Am. Natural. 155, 435–453. doi: 10.1086/303337
Harvey, B. P., Gwynn-Jones, D., and Moore, P. J. (2013). ) Meta-analysis reveals complex marine biological responses to the interactive effects of ocean acidification and warming. Ecol. Evol. 4, 1016–1030. doi: 10.1002/ece3.516
Hedges, L. V., Gurevitch, J., and Curtis, P. S. (1999). The meta-analysis of response ratios in experimental ecology. Ecology 80, 1150–1156. doi: 10.2307/177062
Hedges, L. V., and Olkin, I. (1985). Statistical methods for meta-analysis. New Direct. Program Eval. 1984, 25–42. doi: 10.1002/ev.1376
Hopkinson, B. M., Dupont, C. L., Allen, A. E., and Morel, F. M. M. (2011). Efficiency of the CO2-concentrating mechanism of diatoms. Proc. Natl. Acad. Sci. U.S.A. 108, 3830–3837. doi: 10.1073/pnas.1018062108
Hurd, C. L., Lenton, A., Tilbrook, B., and Boyd, P. W. (2018). Current understanding and challenges for oceans in a higher-CO2 world. Nat. Clim. Chang. 8, 686–694. doi: 10.1038/s41558-018-0211-0
IPCC (2014). Synthesis Report. Contribution of Working Groups I. II and III to the Fifth Assessment Report of the Intergovernmental Panel on Climate Change. Geneva: IPCC, 151.
Ivanina, A. V., and Sokolova, I. M. (2015). Interactive effects of metal pollution and ocean acidification physiology of marine organisms. Curr. Zool. 61, 653–668. doi: 10.1093/czoolo/61.4.653
Jackson, M. C., Loewen, C. J. G., Vinebrooke, R. D., and Chimimba, C. T. (2016). Net effects of multiple stressors in freshwater ecosystems: a meta-analysis. Glob. Chang. Biol. 22, 180–189. doi: 10.1111/gcb.13028
Jin, P., Overmans, S., Duarte, C. M., and Agustí, S. (2019). Increasing temperature within thermal limits compensates negative ultraviolet-B radiation effects in terrestrial and aquatic organisms. Glob. Ecol. Biogeogr. 28, 1695–1711. doi: 10.1111/geb.12973
Jin, P., Wang, T., Liu, N., Dupont, S., Beardall, J., Boyd, P. W., et al. (2015). Ocean acidification increases the accumulation of toxic phenolic compounds across trophic levels. Nat. Commun. 6:8714. doi: 10.1038/ncomms9714
Kroeker, K. J., Kordas, R. L., Crim, R., Hendriks, I. E., Ramajo, L., Singh, G. S., et al. (2013). Impacts of ocean acidification on marine organisms: quantifying sensitivities and interaction with warming. Glob. Chang. Biol. 19, 1884–1896. doi: 10.1111/gcb.12179
LaRoche, J., Rost, B., and Engel, A. (2010). “Bioassay, batch culture and chemostat experimentation,” in Guide for Best Practices for Ocean Acidification Research and Data Reporting, eds U. Riebesell, V. Fabry, L. Hansson, and J. P. Gattuso (Luxembourg: Publications Office of the European Union), 81–94. doi: 10.1111/jpy.12047
Li, F., Wu, Y., Hutchins, D. A., Fu, F., and Gao, K. (2016). Physiological responses of coastal and oceanic diatoms to diurnal fluctuations in seawater carbonate chemistry under two CO2 concentrations. Biogeosciences 13, 6247–6259. doi: 10.5194/bg-2016-281
López, I. R., Kalman, J., Vale, C., and Blasco, J. (2010). Influence of sediment acidification on the bioaccumulation of metals in Ruditapes philippinarum. Environ. Sci. Pollut. Res. Int. 17, 1519–1528. doi: 10.1007/s11356-010-0338-7
Matranga, V., Bonaventura, R., Costa, C., Karakostis, K., Pinsino, A., Russo, R., et al. (2011). “Chapter 8: echinoderms as blueprints for biocalcification: regulation of skeletogenic genes and matrices,” in Molecular Biomineralization. Progress in Molecular and Subcellular Biology, ed. W. E. G. Müller (Berlin: Springer-Verlag), 225–248. doi: 10.1007/978-3-642-21230-7_8
Millero, F. J., Woosley, R., DiTrolio, B., and Waters, J. (2009). Effect of ocean acidification on the speciation of metals in seawater. Oceanography 22, 72–85. doi: 10.5670/oceanog.2009.98
Moreira, A., Figueira, E., Soares, A. M. V. M., and Freitas, R. (2016). The effects of arsenic and seawater acidification on antioxidant and biomineralization responses in two closely related Crassostrea species. Sci. Total Environ. 54, 569–581. doi: 10.1016/j.scitotenv.2015.12.029
Nagelkerken, I., and Connell, S. D. (2015). Global alteration of ocean ecosystem functioning due to increasing human CO2 emissions. Proc. Natl. Acad. Sci. U.S.A. 112, 13272–13277. doi: 10.1073/pnas.1510856112
Nardi, A., Benedetti, M., d’Errico, G., Fattorini, D., and Regoli, F. (2018). Effects of ocean warming and acidification on accumulation and cellular responsiveness to cadmium in mussels Mytilus galloprovincialis: importance of the seasonal status. Aquat. Toxicol. 204, 171–179. doi: 10.1016/j.aquatox.2018.09.009
Nielson, C., Hird, C., and Lewis, C. (2019). Ocean acidification buffers the physiological responses of the king ragworm Alitta virens to the common pollutant copper. Aquat. Toxicol. 212, 120–127. doi: 10.1016/j.aquatox.2019.05.003
O’Dea, R. E., Lagisz, M., Jennions, M. D., Koricheva, J., Noble, D. W. A., Parker, T. H., et al. (2021). Preferred reporting items for systematic reviews and meta-analyses in ecology and evolutionary biology: a PRISMA extension. Biol. Rev. 96, 1695–1722. doi: 10.1111/brv.12721
Pan, K., and Wang, W. X. (2012). Trace metal contamination in estuarine and coastal environments in China. Sci. Total Environ. 421-422, 3–16. doi: 10.1016/j.scitotenv.2011.03.013
Piggott, J. J., Townsend, C. R., and Matthaei, C. D. (2015). Reconceptualizing synergism and antagonism among multiple stressors. Ecol. Evol. 5, 1538–1547. doi: 10.1002/ece3.1465
Pinsino, A., Matranga, V., Trinchella, F., and Roccheri, M. C. (2010). Sea urchin embryos as an in vivo model for the assessment of manganese toxicity: developmental and stress response effects. Ecotoxicology 19, 555–562. doi: 10.1007/s10646-009-0432-0
Przeslawski, R., Byrne, M., and Mellin, C. (2015). A review and meta-analysis of the effects of multiple abiotic stressors on marine embryos and larvae. Glob. Chan. Biol. 21, 2122–2140. doi: 10.1111/gcb.12833
Raven, J. A. (1991). Physiology of inorganic C acquisition and implications for resource use efficiency by marine phytoplankton: relation to increased CO2 and temperature. Plant Cell Environ. 14, 779–794. doi: 10.1111/j.1365-3040.1991.tb01442.x
Raven, J. A., and Crawfurd, K. (2012). Environmental controls on coccolithophore calcification. Mar. Ecol. Prog. 470, 137–166. doi: 10.3354/meps09993
Raven, J. A., Giordano, M., Beardall, J., and Maberly, S. C. (2011). Algal and aquatic plant carbon concentrating mechanisms in relation to environmental change. Photosynth. Res. 109, 281–296. doi: 10.1007/s11120-011-9632-6
Reichelt-Brushett, A. (2012). Risk assessment and ecotoxicology: limitations and recommendations for ocean disposal of mine waste in the coral triangle. Oceanography 25, 40–51. doi: 10.5670/oceanog.2012.64
Reinfelder, J. R. (2011). Carbon concentrating mechanisms in eukaryotic marine phytoplankton. Annu. Rev. Mar. Sci. 3, 291–315. doi: 10.1146/annurev-marine-120709-142720
Ries, J. B., Cohen, A. L., and McCorkle, D. C. (2009). Marine calcifiers exhibit mixed responses to CO2-induced ocean acidification. Geology 37, 1131–1134. doi: 10.1130/G30210A.1
Rodríguez-Romero, A., Jiménez-Tenorio, N., Basallote, M. D., Orte, M. R. D., Blasco, J., and Riba, I. (2014). Predicting the impact of CO2 leakage from subseabed storage: effect of metal accumulation and toxicity on the model benthic organism Ruditapes phillipinarum. Environ. Sci. Technol. 48, 12292–12301. doi: 10.1021/es501939c
Rosenthal, R. (1979). The file drawer problem and tolerance for null results. Psychol. Bull. 86, 638–641. doi: 10.1037/0033-2909.86.3.638
Sampaio, E., Santos, C., Rosa, I. C., Ferreira, V., Pörtner, H.-O., Duarte, C. M., et al. (2021). Impacts of hypoxic events surpass those of future ocean warming and acidification. Nat. Ecol. Evol. 5, 311–321. doi: 10.1038/s41559-020-01370-3
Scheffer, M., and Carpenter, S. R. (2003). Catastrophic regime shifts in ecosystems: linking theory to observation. Trends Ecol. Evol. 18, 648–656. doi: 10.1016/j.tree.2003.09.002
Steckbauer, A., Klein, S. G., and Duarte, C. M. (2020). Additive impacts of deoxygenation and acidification threaten marine biota. Glob. Chang. Biol. 26, 5602–5612. doi: 10.1111/GCB.15252
Stephens, A. E. A., Srivastava, D. S., and Myers, J. H. (2013). Strength in numbers? Effects of multiple natural enemy species on plant performance. Proc. R. Soc. B Biol. Sci. 280, 1–9. doi: 10.1098/rspb.2012.2756
Stockdale, A., Tipping, E., Lofts, S., and Mortimer, R. J. G. (2016). The effect of Ocean Acidification on Organic and Inorganic Speciation of Trace Metals. Environ. Sci. Technol. 50, 1906–1913. doi: 10.1021/acs.est.5b05624
Suffrian, K., Schulz, K. G., Gutowska, M. A., Riebesell, U., and Bleich, M. (2011). Cellular pH measurements in Emiliania huxleyi reveal pronounced membrane proton permeability. New Phytol. 190, 595–608. doi: 10.1111/j.1469-8137.2010.03633.x
Tanaka, K., Ohde, S., Cohen, M. D., Snidvongs, A., Ganmanee, M., and McLeod, C. W. (2013). Metal contents of Porites corals from Khang Khao Island, Gulf of Thailand: anthropogenic input of river runoff into a coral reef from urbanized areas, Bangkok. Appl. Geochem. 37, 79–86. doi: 10.1016/j.apgeochem.2013.07.005
Trimborn, S., Wolf-Gladrow, D., Richter, K. U., and Rost, B. (2009). The effect of pCO2 on carbon acquisition and intracellular assimilation in four marine diatoms. J. Exp. Mar. Biol. Ecol. 376, 26–36. doi: 10.1016/j.jembe.2009.05.017
Vargas, C., Lagos, N., Lardies, M., Duarte, C., Manríquez, P. H., Aguilera, V. M., et al. (2017). Species-specific responses to ocean acidification should account for local adaptation and adaptive plasticity. Nat. Ecol. Evol. 1, 1–7. doi: 10.1038/s41559-017-0084
Viechtbauer, W. (2010). Conducting Meta-analyses in r with the metafor package. J. Stat. Softw. 36, 1–48. doi: 10.18637/jss.v036.i03
Wang, W. X. (2002). Interactions of trace metals and different marine food chains. Mar. Ecol. Prog. Ser. 243, 295–309. doi: 10.3354/meps243295
Wen, J. Y., and Zou, D. H. (2021). Interactive effects of increasing atmospheric CO2 and copper exposure on the growth and photosynthesis in the young sporophytes of Sargassum fusiforme (Phaeophyta). Chemosphere 269:129397. doi: 10.1016/j.chemosphere.2020.129397
Yue, K., Fornara, D. A., Yang, W. Q., Peng, Y., Peng, C., Liu, Z., et al. (2017). Influence of multiple global change drivers on terrestrial carbon storage: additive effects are common. Ecol. Lett. 20, 663–672. doi: 10.1111/ele.12767
Keywords: ocean acidification, heavy metals, marine organisms, interactive effects, additive
Citation: Jin P, Zhang J, Wan J, Overmans S, Gao G, Ye M, Dai X, Zhao J, Xiao M and Xia J (2021) The Combined Effects of Ocean Acidification and Heavy Metals on Marine Organisms: A Meta-Analysis. Front. Mar. Sci. 8:801889. doi: 10.3389/fmars.2021.801889
Received: 26 October 2021; Accepted: 23 November 2021;
Published: 23 December 2021.
Edited by:
Gang Li, South China Sea Institute of Oceanology, Chinese Academy of Sciences (CAS), ChinaCopyright © 2021 Jin, Zhang, Wan, Overmans, Gao, Ye, Dai, Zhao, Xiao and Xia. This is an open-access article distributed under the terms of the Creative Commons Attribution License (CC BY). The use, distribution or reproduction in other forums is permitted, provided the original author(s) and the copyright owner(s) are credited and that the original publication in this journal is cited, in accordance with accepted academic practice. No use, distribution or reproduction is permitted which does not comply with these terms.
*Correspondence: Jianrong Xia, anJ4aWFAZ3podS5lZHUuY24=