- 1Stazione Zoologica Anton Dohrn, Villa Comunale, Naples, Italy
- 2Department of Natural Resources and the Environment, Marine and Freshwater Research Centre, School of Science and Computing, Galway-Mayo Institute of Technology, Galway, Ireland
- 3Department of Organisms and Ecosystems Research, National Institute of Biology, Ljubljana, Slovenia
- 4Marine Biology Station Piran, National Institute of Biology, Piran, Slovenia
- 5Marine Science Institute, ICM-CSIC, Barcelona, Spain
- 6Institute of Marine Sciences, CNR-ISMAR, Venice, Italy
- 7Institute of Marine Sciences, CNR-ISMAR, La Spezia, Italy
- 8SARTI-MAR Research Group, Universitat Politècnica de Catalunya, Vilanova i la Geltrú, Spain
- 9Ruđer Bošković Institute, Zagreb, Croatia
- 10Department of Fisheries, Apiculture, Wildlife Management and Special Zoology, Faculty of Agriculture, University of Zagreb, Zagreb, Croatia
- 11Ocean Networks Canada (ONC), University of Victoria, Victoria, BC, Canada
- 12Department of Biology, University of Victoria, Victoria, BC, Canada
Deep-sea ecosystems are reservoirs of biodiversity that are largely unexplored, but their exploration and biodiscovery are becoming a reality thanks to biotechnological advances (e.g., omics technologies) and their integration in an expanding network of marine infrastructures for the exploration of the seas, such as cabled observatories. While still in its infancy, the application of environmental DNA (eDNA) metabarcoding approaches is revolutionizing marine biodiversity monitoring capability. Indeed, the analysis of eDNA in conjunction with the collection of multidisciplinary optoacoustic and environmental data, can provide a more comprehensive monitoring of deep-sea biodiversity. Here, we describe the potential for acquiring eDNA as a core component for the expanding ecological monitoring capabilities through cabled observatories and their docked Internet Operated Vehicles (IOVs), such as crawlers. Furthermore, we provide a critical overview of four areas of development: (i) Integrating eDNA with optoacoustic imaging; (ii) Development of eDNA repositories and cross-linking with other biodiversity databases; (iii) Artificial Intelligence for eDNA analyses and integration with imaging data; and (iv) Benefits of eDNA augmented observatories for the conservation and sustainable management of deep-sea biodiversity. Finally, we discuss the technical limitations and recommendations for future eDNA monitoring of the deep-sea. It is hoped that this review will frame the future direction of an exciting journey of biodiscovery in remote and yet vulnerable areas of our planet, with the overall aim to understand deep-sea biodiversity and hence manage and protect vital marine resources.
Introduction
The deep sea (generally below the 200 m limit of the euphotic zone) accounts for the majority of the world’s ocean (>95%; Costello et al., 2010; Wedding et al., 2013). This vast environment hosts a wealth of hydrocarbon and mineral resources and provides a series of ecosystem services associated with its functioning (e.g., nutrients regeneration and global biogeochemical cycles), resulting in a vast repository of complex organic molecules and unexplored biodiversity (Pikitch et al., 2014; Thurber et al., 2014; Kroodsma et al., 2018).
The biodiversity of deep-sea ecosystems is increasingly threatened by anthropogenic impacts resulting from pollutants and other activities such as the extraction of geochemical resources and minerals (Levin and Le Bris, 2015). Marine biodiversity conservation is in growing conflict with resource exploitation, especially when it comes to key deep-sea habitats such as abyssal plains (associated to manganese nodule mining), hydrothermal vents fields (associated to sulfide deposits) or submarine canyons (associated to oil and gas drilling) (Danovaro et al., 2017a). In the context of increasing climatic and human disturbances, deep-sea ecosystems, and biodiversity found in areas beyond national jurisdiction are prime conservation targets (as identified by the United Nations Convention on the Law of the Sea (UNCLOS) and the International Union for Conservation of Nature (IUCN), where the preservation of marine ecosystem functions should be balanced with sustainable use of resources (Danovaro et al., 2008, 2020; McIntyre, 2010; Morato et al., 2010; Pusceddu et al., 2014; Ramírez et al., 2017).
Scientific research must thoroughly investigate all ecosystems’ life components prior to onset of mass industrial activities, among which impending deep-sea mining raises particular concerns (e.g., Koschinsky et al., 2018; Washburn et al., 2019). This demand has resulted in the development of management guidelines for sustainable use of the sea, as reflected in the Aichi Target 11 (Convention on Biological Diversity, CBD) and by the Sustainable Development Goal 14 “Life below water” and the post-2020 Zero draft CBD proposal (UN 2030 Agenda for Sustainable Development; UNEP, 2020; UNESCO, 2020). A more comprehensive and multidimensional understanding of marine biodiversity in all its facets, including how it is shaped by the environment, human impacts, and climate, represents a critical knowledge framework that is needed to inform resource management operators (Howell et al., 2020). To gain this comprehensive knowledge, deep-sea research is merging the information on the number of species (or taxonomic units) with data on their ecological relationships and information on their spatiotemporal distribution (Berry et al., 2019; Costa et al., 2020).
Need for Filling Knowledge Gaps of the Deep-Sea
Biodiversity knowledge relies on access to adequate taxonomic information with emphasis on in situ sample collection, observation, and monitoring strategies (Glover et al., 2018). Nonetheless, the deep sea is still virtually unknown to science as <0.0001% of its surface area has been explored in detail (Ramirez-Llodra et al., 2011). Although it has been argued that richness of marine pelagic species decreases sharply with depth (Colloca et al., 2003; Costello and Chaudhary, 2017), major knowledge gaps still exist with current data likely to be biased by uneven and scattered sampling (Higgs and Attrill, 2015). In particular, less than 1% of the deep pelagic realm has been sampled to date due to its vastness and remoteness (Higgs and Attrill, 2015 and references therein). Overall, it is estimated that about 1.5 million deep-sea species have yet to be discovered (Costello and Chaudhary, 2017; Danovaro et al., 2017b).
Despite growing efforts to collect, store and publicly share biological and ecological data on the deep-sea through international programs such as the Census of Marine Life (CoML), the Ocean Biodiversity Information System (OBIS) and the Deep Ocean Observation System (DOOS), the baseline knowledge in biodiversity is still inadequate, and data on the distribution of deep-sea species over extended spatial and temporal scales are almost entirely lacking (Glover et al., 2010; Wedding et al., 2013). Reports of species occurrence in a given area depend on direct sampling for final taxonomic assignment (Glover et al., 2018; Danovaro et al., 2020). This is typically carried out by vessel-assisted methods and technologies [e.g., remotely operated vehicles (ROVs) and autonomous underwater vehicles (AUVs)], with considerable practical and logistic limitations still affecting sample collection and spatiotemporal replication (Aguzzi et al., 2019). Indeed, the capability of vessel-based research expeditions has advanced significantly in the past decades, however the data gathered provide a snapshot of the local biological complexity but are restricted to the relatively narrow timeframe of the cruise period (Ruth, 2006). This limitation is further emphasized in the deep-sea, where tidal and inertial currents can result in massive benthic and pelagic populations displacements (Gage and Tyler, 1992; Aguzzi and Company, 2010; Aguzzi et al., 2011a,2015). In addition to the many technical constraints of deep-sea surveys, sampling is often targeting specific taxonomic groups, habitats, ecological traits, sizes or behaviors, limiting the taxonomic resolution of species inventories (Hatch et al., 2020; McCowin et al., 2020; Weston et al., 2020). The most notorious example of such limitations is found in deep-sea fishery surveys, where data is collected either by trawl nets of a certain mesh size or using ROVs for habitat characterization, both of which target only benthic megafauna and also have known biases due to selective captures/sampling (e.g., Common Fishery Policy Data Collection Multiannual Program; Aymà et al., 2016; Jac et al., 2021).
Emerging Technological Advances in Deep-Sea Monitoring
Cabled observatories (seabed oceanographic research platforms connected to network systems to provide a continuous monitoring, observing, and recording of various seafloor activities) are constantly transforming ocean research, by establishing networks of interactive, globally distributed sensors for real-time data collection (Danovaro et al., 2017a; Aguzzi et al., 2019; Jahanbakht et al., 2021). These platforms enable the combination of data collection by optoacoustic (HD video and multi-beam rotary or dual-frequency sonar imaging devices), oceanographic and geochemical sensor technologies, in a continuous, high-frequency and long-lasting fashion (e.g., Thomsen et al., 2012, 2017; Howe et al., 2019; Table 1). Coupling the presence of species to the environmental conditions surrounding them makes these platforms the core of emerging in situ marine ecosystem-level laboratories (Rountree et al., 2020). These platforms can provide long-term imagery data sets (e.g., decades), hence enabling the compilation of comprehensive multiannual species richness lists (Juniper et al., 2013; Doya et al., 2017; Chauvet et al., 2018; del Rio et al., 2020). Taxonomic characterization of monitored communities by visual means is also complemented by Passive Acoustic Monitoring (PAM) systems with the use of specific acoustic markers for species identification (e.g., Juanes, 2018).
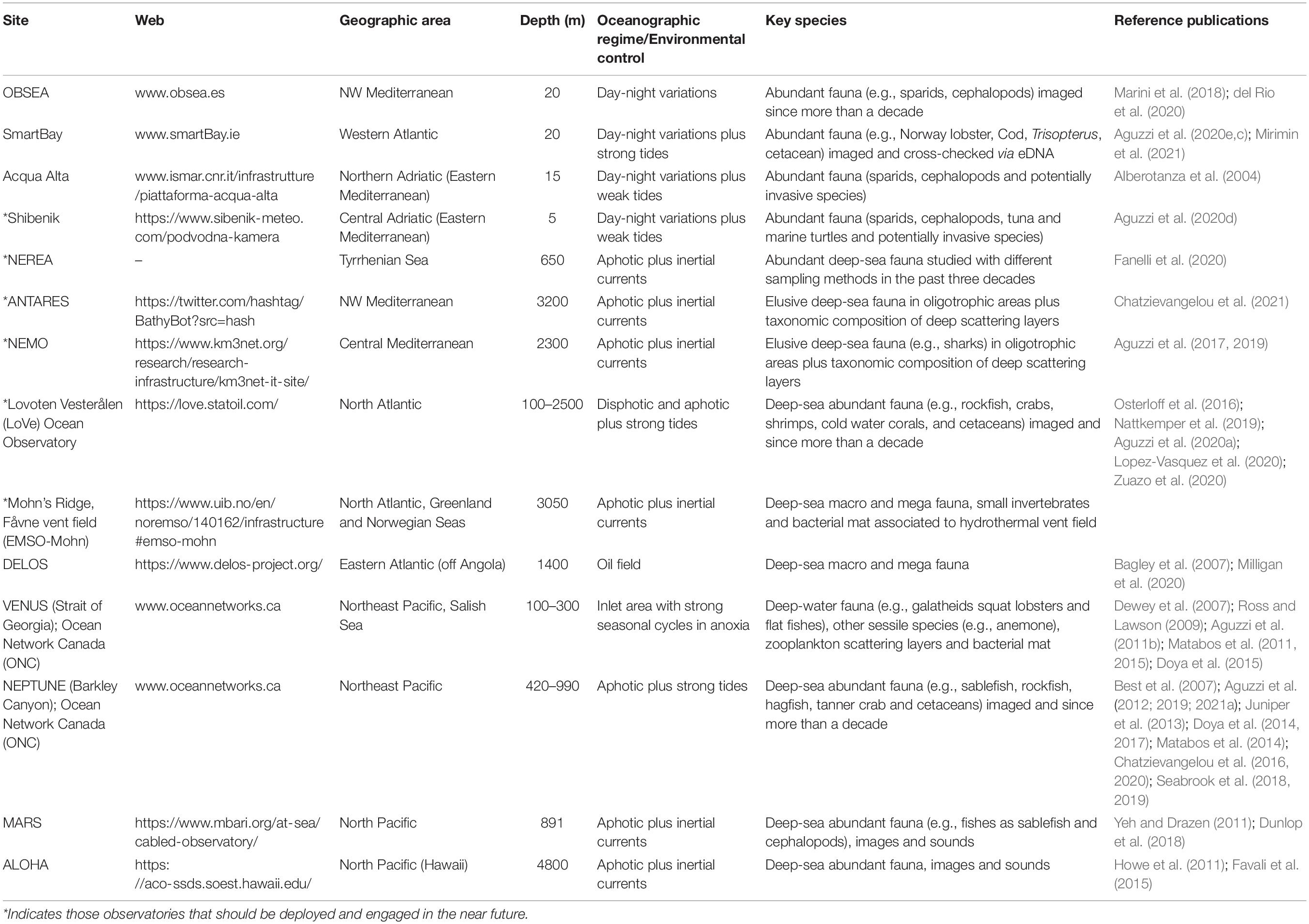
Table 1. List of some of the best known coastal and deep marine cabled observatories that are presently engaged in the recompilation of large image data sets with the implementation of eDNA prospection.
To overcome the spatial constraints imposed by limited fixed-point observation nodes, mobile platforms are being developed to monitor both the seafloor and the water column (Aguzzi et al., 2019). Internet Operated Vehicles (IOVs), such as crawlers and rovers, are benthic mobile platforms that are either tethered to cabled observatories (Purser et al., 2013) or completely free of direct physical connection (Brandt et al., 2016), and can operate with preloaded navigation plans to autonomously return to their docking station (i.e., the cabled observatory) to recharge and offload data (Thomsen et al., 2012, 2017; Aguzzi et al., 2020a). A recent addition to cabled observatories allows the study of subatomic particles such as neutrinos (Agostini et al., 2020). Using a suite of photomultipliers and other light-sensitive sensors, these neutrino telescopes are also capable of continuously monitoring bioluminescence from migrating deep-scattering layers and bacterioplankton (Martini et al., 2013, 2014; Tamburini et al., 2013; Bailly et al., 2021). These cross-disciplinary infrastructures will provide key complementary data for long-term monitoring of bentho-pelagic coupling in a rapidly changing ocean (Chatzievangelou et al., 2021).
Most observatories rely on information acquired by imaging to provide both qualitative and quantitative data on local biodiversity (Bicknell et al., 2016). Thus, the quality of biodiversity information relies upon the ability to classify organisms to the species level, that in turn can be used to compile local inventories (i.e., richness) and relative abundance estimates (Aguzzi et al., 2020a). Unfortunately, imaging does not always allow sufficiently high taxonomical precision, and generally requires the physical collection of samples to validate species identification. Furthermore, organisms’ attraction to or avoidance of submerged infrastructures is likely to cause some degree of bias toward the local communities (Widder et al., 2005; Aguzzi et al., 2019; Rountree et al., 2020; Garcia-Vazquez et al., 2021).
Significant advances in molecular methodology and bioinformatics, accompanied by a steady increase in computational power, have made “omics” technologies and data increasingly accessible, with great potential to fill gaps in biodiversity monitoring capabilities of deep-sea cabled observatories (Heidelberg et al., 2010; Garcia-Vazquez et al., 2021). One of the more recent contributions of “omics” to biodiversity monitoring is linked to the collection and analysis of genetic material extracted directly from environmental samples (sediment, water, ice, and air, etc.; Taberlet et al., 2012; Barnes and Tuner, 2016; Cristescu and Hebert, 2018), which can include a mixture of whole organisms and/or environmental DNA (eDNA) (sensu Rodriguez-Ezpeleta et al., 2021). Sequencing of eDNA by means of High Throughput Sequencing (HTS) technology has enabled the development of eDNA metabarcoding. Here, amplicon sequencing with universal primers is used to generate a extremely large (hundreds of thousands to millions) of DNA (mini)barcode reads (Meusnier et al., 2008; Hajibabaei and McKenna, 2012). These are preprocessed and curated using dedicated bioinformatic pipelines. This includes trimming the reads so that only marker sequences remain and quality filtering (e.g., DADA2 – Callahan et al., 2016; Cutadapt – Martin, 2011; Vsearch – Rognes et al., 2016). The quality filtered reads are then clustered into OTUs based on similarity (e.g., 99%, 97%) or taxonomically assigned directly using DNA reference databases (e.g., BOLD – Ratnasingham and Hebert, 2007; GenBank – Benson et al., 2013; PR2 – Guillou et al., 2013; SILVA – Quast et al., 2013; PLANiTS – Banchi et al., 2020; MZG-db – Bucklin et al., 2021). Reads that cannot be assigned to the desired taxonomic level (e.g., species, genus, and family) can still be used to assess alpha and beta diversity evaluation (e.g., Stefanni et al., 2018). eDNA metabarcoding approaches are revolutionizing marine biodiversity assessment and monitoring because they can be used to simultaneously determine entire species communities, even when the exact composition of these assemblages is unknown (e.g., Deiner et al., 2017; Djurhuus et al., 2017; Stefanni et al., 2018; Eble et al., 2020; Kolda et al., 2020; McClenaghan et al., 2020; Seymour et al., 2020; Kawato et al., 2021). eDNA metabarcoding is becoming a particularly valuable tool for deep-sea biodiversity research and monitoring given high species diversity, low animal numbers, difficulties in taxonomic identification due to limited taxonomic expertise, large and remote location, and associated logistical constraints for sample/specimen acquisition (Thomsen et al., 2016; Kersten et al., 2019; Atienza et al., 2020; Canals et al., 2021; Kawato et al., 2021; Merten et al., 2021).
Much of the eDNA work on deep-sea communities has focused on sediment samples to study benthic communities (e.g., Guardiola et al., 2016a; Atienza et al., 2020; Lins et al., 2021) as opposed to fish and pelagic communities. While fish taxa detected by eDNA metabarcoding are generally comparable to those identified by conventional fish survey methods, eDNA captures greater fish diversity than conventional methods when considering a single conventional approach. For example, eDNA metabarcoding in the deep sea generally outperforms trawling because of the presence of species that are typically elusive, small, rare or located on rocky surfaces or steep slopes (Thomsen et al., 2016; Closek et al., 2019; Afzali et al., 2020; Fraija-Fernández et al., 2020; McClenaghan et al., 2020). The advantage of deep ocean water eDNA metabarcoding has also been demonstrated for the study of other communities, including cephalopods (Merten et al., 2021; Visser et al., 2021) and zooplankton (Kersten et al., 2019; Laroche et al., 2020b; Govindarajan et al., 2021). In addition, eDNA extracted from water has also been used to study deep-sea benthic communities (Everett and Park, 2018; Laroche et al., 2020a). However, some studies have shown that samples from the water column are not a viable alternative to sediment samples for benthic diversity inventories (Brandt et al., 2021). Due to the patchiness of benthic fauna (Rosli et al., 2017), eDNA analysis of deep-sea sediment requires sampling of multiple biological replicates and larger samples sizes (Guardiola et al., 2016a, b; Atienza et al., 2020; Brandt et al., 2020). eDNA analysis of sediments may describe past rather than present communities, as sediments contain ancient DNA (aDNA) in addition to contemporary DNA, thus sediment eDNA analysis often targets the very top layer of sediment (Atienza et al., 2020; Brandt et al., 2020) and/or longer amplicons (e.g., COI – Leray et al., 2013).
In the present manuscript, we identify and discuss potential developments in the use of eDNA metabarcoding for deep-sea biodiversity assessment at cabled observatories and associated mobile platforms. Methodological developments are discussed in relation to: (i) Integrating eDNA with optoacoustic imaging; (ii) Development of eDNA repositories and cross-linking with other biodiversity databases; (iii) Artificial Intelligence (AI) for eDNA analyses and integration with imaging data; and (iv) Benefits of eDNA augmented observatories for the conservation and sustainable management of deep-sea biodiversity. We conclude by discussing the technical limitations and recommendations for future eDNA monitoring of the deep-sea.
Integrating Environmental DNA With Optoacoustic Imaging
Among the main benefits of using eDNA as a monitoring tool are the fact that it is an indirect non-invasive technique (i.e., no need to capture the target organism) and it does not require specialist taxonomic expertise to detect taxa across the tree of life (Goricki et al., 2017; Stefanni et al., 2018), though the latter strongly depends on availability of comprehensive reference DNA databases (as further discussed below). Once an environmental sample such as water, biofilm or sediment is acquired (Brandt et al., 2021), the collected eDNA can be queried either by using “universal” markers targeting whole communities by means of HTS (Jerde et al., 2019), or by targeted species-specific assays usually performed by real-time quantitative PCR (qPCR) or digital PCR (dPCR) (Goldberg et al., 2016). The effectiveness of both approaches depends on the availability of reference data, for taxonomy identification of sequenced reads with eDNA metabarcoding and for the development of species-specific assays with the targeted approach. These DNA-based tools offer several advantages over traditional techniques. They improve the ability to unravel the “hidden” biodiversity (e.g., detect rare, cryptic, elusive, and non-native species in the early stage of invasion), which is particularly relevant in the case of remote environments such as the deep-sea, and enable near real-time global census of species (Stat et al., 2017; LeBlanc et al., 2020).
Such features may enable the full integration of eDNA analysis into ecological monitoring procedures when its measurement is coupled with other non-molecular data as optoacoustic imaging (e.g., Stat et al., 2019; Easson et al., 2020; Mirimin et al., 2021). For this purpose, eDNA water sampling should also be provided in real-time by autonomous and independent samplers (e.g., Yamahara et al., 2019; Hansen et al., 2020; Jacobsen, 2021; Moore et al., 2021), with prototypes presently under construction (e.g., the Adjustable Volume eDNA Sampler1, and the Robotic Cartridge Sampling Instrument-RoCSI2) or that can be adjusted for this purpose, as the SALSA system (Kersten et al., 2019; Brandt et al., 2021)3. An alternative to water samplers, would be an opportunistic use of filter feeding organisms such as sponges or bivalves, that act as natural “DNA traps,” concentrating eDNA from water that can be retrieved at different time points (Mariani et al., 2019; Turon et al., 2020; Weber et al., 2021). The advantage of adding eDNA to ecological monitoring protocols is its ability to cross-validate data from other methodologies (e.g., imaging) (e.g., Aguzzi et al., 2019). On the other hand, it is reported that samples from the water column do not provide a good characterization of the underlying benthic taxa suggesting that benthic biodiversity surveys should be also performed (Antich et al., 2021b; Brandt et al., 2021). Seabed sediment acquisition technologies are continuously improved and optimized, so as to obtain more authentic and reliable samples to meet the ever-increasing demands on sampling capabilities (He et al., 2020) and adaptation to cabled observatory infrastructures.
Recent eDNA advancements allow us to study a wide range of taxa (including vertebrates) that are otherwise inaccessible by direct capture or optoacoustic technologies (e.g., Lacoursière-Roussel et al., 2018; Cowart et al., 2020; Laroche et al., 2020b; Canals et al., 2021). Though still limited to the near surface waters, the combined use of video-monitoring and eDNA metabarcoding has also been successfully applied using Baited Remote Underwater Video Systems (BRUVs) to monitor Marine Protected Areas (MPAs) (Stat et al., 2019) or integrated in cabled observatories (Mirimin et al., 2021). In these cases, taxa analyses were represented by visually conspicuous biota (mainly fish) and all post-sample collection steps were carried out off site in dedicated molecular laboratories. The way forward involves the integration and development of sampling methodology and sensing protocols adapted to operate on ROVs, AUVs and even biomimetic platforms (e.g., Aguzzi et al., 2021a), hence further expanding the sampling capability to most remote habitats while minimizing sampling disturbance (e.g., Trenkel et al., 2019).
Development of Environmental DNA Repositories and Cross-Linking With Other Biodiversity Databases
When identifying organisms, scientists can narrow down taxonomic possibilities thanks to the use of a single approach or, preferably, by combining and integrating multiple approaches, although a degree of uncertainty in taxa identification will always remain (Danovaro et al., 2020). In recent years, molecular tools have been integrated into classical morphology-based taxonomic approaches (e.g., Stefanni et al., 2021), which has proven extremely useful in resolving the taxonomic status of cryptic species (e.g., Carreiro-Silva et al., 2017). However, in an ideal integrative taxonomy framework, different lines of evidence obtained at the genetic, physiological, morphological, behavioral, and habitat level should be considered and all combined within Hutchinson’s (1957) multimodal niche (Schlick-Steiner et al., 2010).
Nowadays, most biodiversity data are recompiled into open-access online databases (Gemeinholzer et al., 2020). In the case of marine life, the most comprehensive database – the World Register of Marine Species (WoRMS) – is regularly updated by active communities of marine taxonomists (Costello et al., 2013). Building on this foundation, the World Register of Deep-Sea Species (WoRDSS; Glover et al., 2021), a taxonomic database of deep-sea species, was launched in 2012 by the International Network for Scientific Investigation of Deep-sea Ecosystems (INDEEP). This database also includes the global-scale trait database for the fauna of deep-sea hydrothermal-vents, the sDiv-funded trait database for the Functional Diversity of vents (sFDvent; Chapman et al., 2019). These inventories are exclusively based on records of collected organisms. In parallel, genetic and genomic databases have been implemented, that are either inclusive as in the case of GenBank (Clark et al., 2016) or BOLD (Ratnasingham and Hebert, 2013), or restricted to selected groups of organisms, such as MZGdb (Bucklin et al., 2021), PR2 (Guillou et al., 2013) and PLANiTS (Banchi et al., 2020).
As in conventional DNA barcoding, eDNA sequences are usually compared with a reference database of the expected species community to translate the obtained molecular operational taxonomic units into biological species for the final data interpretation. These matching processes are reliable when based on a comprehensive reference library supported by morphological description of the reference taxon. However, such reference databases are still far from complete, especially for deep-sea communities (Weigand et al., 2019). Additionally, misidentifications of reference sequences have been frequently reported, highlighting the need of refinement and curation of these databases to reduce false negatives, and conflicts in taxonomic assignment (Stefanni et al., 2018; Schroeder et al., 2020; Bucklin et al., 2021).
The performance of eDNA in providing accurate estimates of species’ diversity by matching different genetic repositories, has also been tested. For example, fishes are both a frequent target in eDNA studies and widely represented in genetic repositories by multi-marker sequences. Recently, the performance of eDNA from surface water samples in determining fish diversity, was evaluated by a comparing it to bottom trawl catches (Stoeckle et al., 2021). Fish diversity estimation obtained by eDNA was equal to, or greater than, that obtained from a single 66 million liters trawl. Most (70–87%) species detected by trawl in a given month were also detected by eDNA, and vice versa, including nearly all (92–100%) abundant species (Stoeckle et al., 2021). For a more comprehensive assessment of the local biodiversity including benthic taxa (from metazoans to protist and prokaryotic communities), eDNA from sediment should also be analyzed as only a fraction of total molecular clusters is shared between the eDNA of these two environmental matrices (Atienza et al., 2020; Zhao et al., 2020; Brandt et al., 2021). Furthermore, meiofauna, micro-eukaryotes, and bacteria constitute a large portion of deep-sea abundance and biomass and should not be neglected (Rex et al., 2006; Ingels et al., 2021). Even if these small-size organisms cannot be taxonomically identified due to lack of appropriate reference databases, their contribution to biodiversity can still be evaluated with taxonomy-free approaches (Cordier et al., 2019b).
The improvement of existing marine genetic databases and the development of portals exclusively dedicated to eDNA sequences are considered priorities for global biodiversity assessment and for filling taxonomic and spatial gaps in bio-surveys (Berry et al., 2021). Early initiatives have already been undertaken worldwide to integrate eDNA into biodiversity databases that provide accurate spatial information on aquatic species occurrence based solely on eDNA records collected according to standardized protocols (e.g., United States, New Zealand, and Sweden) (Young et al., 2018; DFO, 2020; Sundberg et al., 2020; Abbott et al., 2021). Integrating dedicated eDNA sequence repositories with high-resolution imaging or other attributes collected in situ (e.g., sound generated by animals; Mooney et al., 2020) can maximize the identification of species together with spatial and temporal resolution (e.g., Bicknell et al., 2016; Howell et al., 2019; Horton et al., 2021; Mirimin et al., 2021). Such integrated open-access online biodiversity databases can further enable a putative taxonomic identification of species detected (as particular OTUs), but not identified by eDNA. If the closest taxonomic match for eDNA sequences is below the percentage that would allow species-level identification, a putative identification of the sequence in question could be made using image or sound identifications taken along, at least until a specimen is collected and properly examined and a reference sequence record deposited for future use. This would provide information on what to expect in future biodiversity inventories in a given remote area.
A further step toward integration of marine biodiversity data repositories has been provided by BOLD, which contains open access records of organisms (including imaging) tagged with one or more standardized short DNA genetic markers (Ratnasingham and Hebert, 2007, 2013). A further step toward integrating marine biodiversity data repositories could be the creation of a single open access platform where data of different origins and typologies (including eDNA markers) are freely searchable (as in the case of the Global Biodiversity Information Facility, GBIF) (Andersson et al., 2020; Heberling et al., 2021). These database platforms have begun to include eDNA records as a new type of biological observation that can be accessed alongside millions of conventional biodiversity records (Berry et al., 2021). The development of AI algorithms (as indicated in the previous section) can facilitate better operational cross-linking between in situ eDNA data and other complementary data (e.g., temperature, pH, current, and etc.). These “Big Data” analyses could be fully embedded into cabled observatories protocols for autonomous data processing to provide reliable spatiotemporal assessment of biodiversity in almost near real time.
Artificial Intelligence for Environmental DNA Analyses and Integration With Imaging Data
The step forward to efficiently augment the in situ deep-sea ecological monitoring capability of cabled observatories and their docked platforms envisions the ability to collect genetic and imaging data in situ and process the information in real time using automated pipelines (e.g., Osterloff et al., 2016, 2019; Lopez-Vasquez et al., 2020; Zuazo et al., 2020). These developments rely on the establishment of AI algorithms for taxonomic assignment as well as dedicated reference DNA sequence databases.
The fully automated integration of eDNA and imaging data represents one of the core development aspects to augment the monitoring capability of deep-sea biodiversity at cabled observatories, enabling the detection of organisms over a wide range of taxa and different body sizes when it comes to fishes. Currently, there are several initiatives to automate in situ eDNA analyses in near real time (Scholin et al., 2017; Ribeiro et al., 2019; Yamahara et al., 2019). Integration of eDNA and imaging data involves the development of appropriate pipelines for: (i) automatic taxonomic identification of eDNA sequences to the highest level (e.g., species); and (ii) cross-check of eDNA taxonomic identification with large image repositories, accounting for a multi-annual status of local richness and biodiversity (i.e., based on species tracking and classification, resulting in time series of data on community structure as well as relative abundance). Both steps can be implemented by applying AI algorithms using Machine Learning (ML) methods.
Analysis of eDNA metabarcoding data using ML methods is a new and developing field. There are two main approaches in the use of ML methods for biodiversity monitoring, and while one operates on taxonomically assigned OTUs, the other is taxonomy-free, where there is no longer the need of a reference database, thus overcoming the limits of taxonomy-based eDNA bioassessment (Cordier et al., 2018). Such taxonomy-free approach still requires “training” data sets in order to feed into predictive models that can be used to make inference on previously unexplored taxa (Cordier et al., 2018).
Cordier et al. (2017) focused on the problem of lacking inventories for eDNA data from benthic foraminifera and showed that supervised ML approaches (i.e., random-forests and self-organizing-maps) can classify unknown sequences and infer biotic indices of macro-invertebrates reasonably well. They argued that ML makes good predictions and outperforms analyses based only on known sequences (Cordier et al., 2018).
Machine learning tools are currently part of many pipelines for eDNA data analysis. Dully et al. (2021a; 2021b) showed that ML-based pipelines are sufficiently robust even for rarefied samples. Other authors reached similar conclusions (Cordier et al., 2019a; Apothéloz-Perret-Gentil et al., 2021; Frühe et al., 2021; He et al., 2021) and Mathon et al. (2021) reviewed literature on “eDNA and Machine Learning.” In ML analysis, data is first pre-processed with common bioinformatic pipelines as for general metabarcoding analysis (Mathon et al., 2021) and subsequently processed through an automated DNA-Barcode Classifier (taxonomy assignment). ML supports this classification task with a consolidated pipeline. Sequences contained in DNA-barcode repositories (e.g., GenBank, GB; Barcoding of Life Database, BOLD) are first used to train a ML-based classifier (e.g., Cordier et al., 2017, 2019a; Frühe et al., 2021). The trained classifier is then ready to identify the taxa contained in the sample. We prospect, that in the framework of cabled observatories further assessment of the identification results could be obtained by cross-checking the eDNA taxonomy classification with organism identified through video/image data analysis. In this case, images have to be acquired contextually to eDNA sampling and a content-based image classification have to be performed in order to classify the framed organisms (e.g., fishes).
Applied underwater image classification based on ML demonstrated to provide high quality results (Langenkämper et al., 2020; Lopez-Vasquez et al., 2020; Malde et al., 2020; Mathur et al., 2020). The ML-based image classifier needs to be trained from an image ground-truth dataset. Then, the taxa of the classified specimens can be compared with those returned by the eDNA classifier. The diagram in Figure 1 shows a conceptual pipeline for handling the eDNA data and image cross-check.
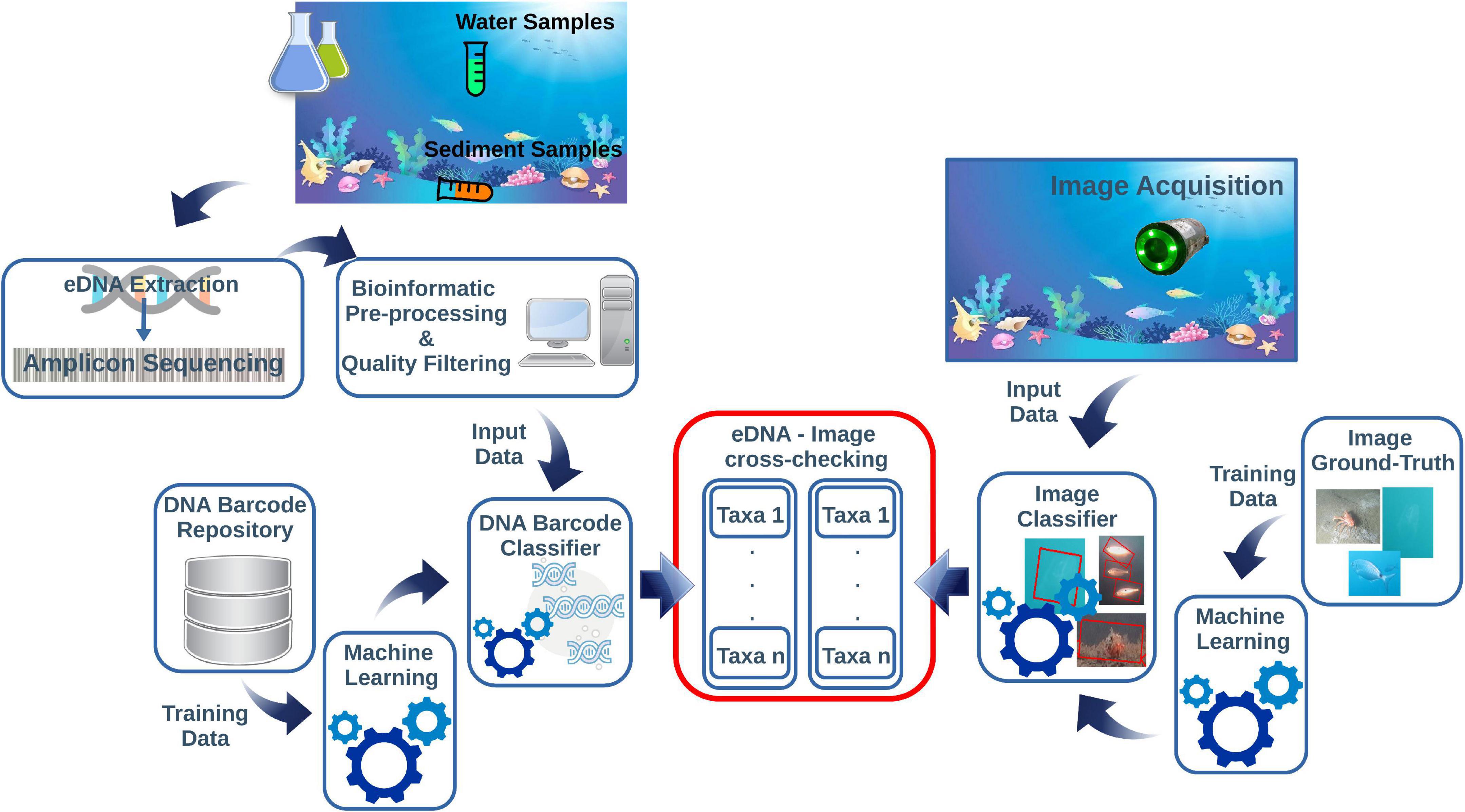
Figure 1. This diagram is summarizing the main steps of the pipeline for eDNA and imaging data integration. The eDNA is collected from the water or sediment and processed through metabarcoding protocols. This step includes several bioinformatics pre-processing actions before going through an automated DNA-barcode classifier pipeline. The images acquired on cameras contextually to eDNA sampling are post-processed through an image classifier routine. Both protocols need independent reference repositories to train the ML classifiers before the cross-checking of the taxonomic assignments derived from eDNA and images.
Benefits of Using Environmental DNA-Augmented Observatories for the Conservation and Sustainable Management of Deep-Sea Biodiversity
Achieving conservation and sustainability goals through ecosystem-based management is challenging, particularly for deep-sea ecosystems, as lack of knowledge hinders science-based prioritization of appropriate management and conservation strategies (Glover et al., 2018; Howell et al., 2020; Manea et al., 2020). Cabled observatories have already been recognized as key tools capable of filling knowledge gaps through systematic monitoring (Danovaro et al., 2017a; Aguzzi et al., 2020a,b, 2021b). Integration eDNA surveillance within the monitoring capabilities offered by cabled observatories makes them even more promising (Mirimin et al., 2021). Indeed, eDNA has been highlighted as a key approach that will enable conservation managers and marine spatial planners to detect target species for conservation, provide biotic indexes for impact assessment, increase the spatio-temporal capability of biodiversity surveys, and map vulnerable deep-sea species or ecosystems (Aylagas et al., 2014; Pawlowski et al., 2018; Bani et al., 2020; Kutti et al., 2020). Another value of eDNA augmented cable observatories is their potential contribution to two synergistic global initiatives addressing monitoring of the marine environment: The Essential Ocean Variables (EOVs), supported by the Global Ocean Observing System (GOOS); the Essential Biodiversity Variables (EBVs), developed by the Group on Earth Observations Biodiversity Observation Network (GEOBON) (Pereira et al., 2013; Bax et al., 2018). These two frameworks are being developed to inform global policies and sustainability strategies, and produce comparable and integrated data through harmonization of monitoring (Canonico et al., 2019; Jetz et al., 2019).
Addressing conservation priorities in the deep sea, and monitoring the level of effectiveness of conservation measures are critical steps. The use of eDNA analyses has been recently extended to biodiversity assessment in the context of deep-seabed mining of polymetallic nodules to guide management of this deep-sea resource exploitation that is foreseen to have one of the highest environmental impacts in the near future (Wedding et al., 2015; Laroche et al., 2020a; Leray and Machida, 2020), being also suggested as a cost-effective method (Le et al., 2021). The performance of this high throughput approach has also been tested in impact assessment of offshore oil and gas drilling and extraction (Laroche et al., 2018), and in fish stock assessment to inform fishery management (Salter et al., 2019).
DNA-based tools coupled with cabled observatories and supported by visual and acoustic census can enhance monitoring capability within MPAs, as it has been tested in recent biodiversity assessments (Stat et al., 2019; Gold et al., 2021). Such an approach would be greatly beneficial to the monitoring of Large Scale Marine Protected Areas (LSMPAs). LSMPAs are greater than 150,000 km2 and may encompass critical habitats for migratory species (Lewis et al., 2017), but monitoring such areas is challenging if not impractical (O’Leary et al., 2018).
Furthermore, boosting knowledge of deep-sea biodiversity would help in the prioritization of deep-sea areas of conservation. The Ecologically and Biologically Significant Marine Areas (EBSAs) have been previously proposed to focus attention on where and what type of conservation measures could be established in offshore and deep-sea areas, including the designation of new MPAs (Ardron et al., 2009; Portman et al., 2013; Johnson et al., 2018). However, many potential EBSAs have been removed from the original list due to insufficient knowledge needed to inform the selection criteria, and a call has been made to strengthen scientific research in these areas (Johnson et al., 2019). Both LSMPAs and EBSAs initiatives are hindered by the absence of concrete knowledge of connectivity within and between regions (Cannizzo et al., 2021), as well as by the challenge of describing the links between ocean depths and the fundamental bentho-pelagic coupling (Johnson et al., 2018; O’Leary and Roberts, 2018) – these issues might be resolved by eDNA augmented observatories applying metaphylogeography tools for the analysis within OTUs connectives (Turon et al., 2019; Antich et al., 2021a).
Finally, in response to the urgency to increase knowledge of deep-sea ecosystems and manage deep-sea resources in a scientifically sound manner, the Deep Ocean Observing Strategy (DOOS) has been established to coordinate monitoring and observing efforts. As part of this strategy, genetic studies have been identified as key knowledge sources for biodiversity and connectivity assessment (Baco et al., 2016; Levin et al., 2019), as well as prioritizing the need of defining deep-sea ecological variables to feed global monitoring frameworks (Danovaro et al., 2020).
Technical Limitations and Steps Forward for Environmental DNA Monitoring in the Deep-Sea
While underwater imaging in deep-sea cabled observatories is usually used to detect and identify big to medium sized animals, advantages in image processing and pattern recognition have also made it possible to automatically or semi-automatically identify zooplankton (Gorsky et al., 2010). Zooplankton imaging instruments have gone beyond just laboratory bench-top application (e.g., ZooScan) and now allow in-flow onboard counting and classification (e.g., ZooCAM; Colas et al., 2018) or are mounted on AUVs (Ohman et al., 2018) or even integrated into shallow water cabled observatories (the COSYNA-AWIPEV observatory in the Kongsfjorden Arctic fjord system and the COSYNA-Helgoland observstory; Fischer et al., 2020). It is prospected that similar imaging systems could be integrated into deep-sea cabled observatories for imaging of mero-planktonik larvae. Future modification of such systems could be used to analyse meiobenthos (e.g., FlowCAM; Kitahashia et al., 2018) or benthos could also be studied with the assistance of Sediment Profiling Imaging (SPI) systems.
Despite the rapid and widespread adoption of eDNA metabarcoding analysis for species identification, limitations still exist, and are the subject of much active research. Sequence length constrains imposed by HTS technology may contribute to the detection of false positives, when the target species is absent but its DNA, or rather the DNA of a close match, is recovered. Moreover, primer biases may generate false negatives, i.e., species that are not detected even though they are present. These limitations have been carefully evaluated but only partially overcome (Taberlet et al., 2012; Cristescu and Hebert, 2018 and references therein). Strategies to address such limitations can be intrinsic to the eDNA approach, e.g., the use of multiple markers, (Stefanni et al., 2018; Liu and Zhang, 2021), capture by hybridization approach (Günther et al., 2021), or long reads sequencing (Davidov et al., 2020) but it is also expected that this will improve with the integration of multidisciplinary survey approaches (e.g., combining imaging with eDNA).
Although ML methods (see previous section) could provide valuable tools to reduce errors, their application presents some difficulties. ML methods require ad hoc training sets of sequences and images, that are used as benchmark data repositories to reduce problems with taxonomy assignments of sequences, such as in the cases of: (i) false positives, when incorrect species are assigned to certain sequences based on sequence similarity with a close match; and (ii) rarity or endemism, when eDNA sequences match species that are not detected by video or in the historical records of the area. The above-mentioned data gaps and erroneous entries in genetic repositories are another source of uncertainty for classifier algorithms. ML methods are capable to solve these issues by using existing datasets and generating multiple species trees based on a percentage of similarities. Moreover, by applying the Lowest Common Ancestor (LCA) algorithm, ML can still identify unassigned sequences whose taxonomy is deficient due to the lack of reference sequences deposited in publicly accessible repositories. A different ML based methodology involves the taxonomy-free approach, where bio-monitoring information is obtained through the treatment of data obtained from DNA sequencing without taxonomic assignment (Apothéloz-Perret-Gentil et al., 2017; Feio et al., 2020). The main limits of this approach are the possibility to the under-sampling the input data and the need to calibrate the used bio-index (Apothéloz-Perret-Gentil et al., 2017).
The discovery and implementation of new barcoding markers will be necessary to address low resolution power of existing markers in taxa characterized by exceptionally low rates of mitochondrial evolution (e.g., anthozoans; Hebert et al., 2003) or recently diverged species (e.g., cypraeid marine gastropods; Meyer and Paulay, 2005). A solution may be found in complementing short read amplicon sequencing with sequencing technologies (ONT, PacBio) capable of longer read lengths (e.g., full genes or even mitogenomes). The development of such approaches could indeed be facilitated by integration with video/image data analysis and reference sequence repositories to further enhance species level identification capabilities.
Further technological limitations for eDNA methodology derive from knowledge gaps regarding the persistence and transport of eDNA (Collins et al., 2018; Murakami et al., 2019), which are largely unexplored in deep-sea environments. Persistence of eDNA in the marine environment can be assessed according to specific seascape properties of the sampled water mass, which can be easily measured by cabled observatories multiparametric habitat sensors assets (Aguzzi et al., 2010). eDNA decay involves multiple processes, including cellular, microbial degradation, and also spontaneous degradation of DNA caused and/or accelerated by UV, temperature, and pH (Collins et al., 2018; Harrison et al., 2019; Hunter et al., 2019). Furthermore, the spatial coverage of species detection by eDNA depends on local hydrodynamics (i.e., strength and direction of currents), which affects the dispersal and transport of molecules from neighboring areas (Harrison et al., 2019). The combined action of oceanographic and biogeochemical variables should be carefully considered when inferring the temporal and spatial coverage of the information provided by eDNA markers (Harrison et al., 2019), and taken into account in sampling design and collection.
Spatiotemporal coverage of environmental data collected by networks of observatories provides a unique opportunity to define optimized eDNA sampling strategies, in terms of the best timing for seawater collection based on eDNA persistence and passive dispersion in the local marine environment (i.e., as a “molecular connectivity”). This may be even further aided by continuous multiparametric data collection and increasing knowledge of environmental conditions favoring eDNA detection. Data integration, performed for example with Lagrangian models of eDNA dispersal (Andruszkiewicz et al., 2019), can form the basis for innovative sampling scenarios (e.g., timing and repetition of sampling depending un current status). In this way, the spatial and temporal distribution of a species across a given area could be predicted even before planning the eDNA sampling, based on the status of a combined set of local environmental factors (e.g., currents, temperature, and nutrients), complemented by video-counts of target species (see previous section).
Some examples of time-strategies for eDNA sampling that would benefit from this approach include: (i) pre-programming continuous or time-lapse sampling based on modeled, forecasted environmental conditions; (ii) pre-programming surveillance approaches based on real-time remote sampling that is activated only when a predefined set of conditions is met (e.g., within a range of current intensity, pH, temperature, or while the camera is activated); and (iii) synchronous sampling over large areas (by multiple samplers) thanks to the network of IOVs operating away from the cabled observatories.
Final Remarks
There is an impending need for the laying out of a roadmap for the effective collection and synthesis of high-quality deep-sea biodiversity data to fill knowledge gaps required for policy decisions and environmental management (Levin et al., 2020). This requires the identification of (i) consensus biodiversity variables to be monitored, and (ii) adequate and harmonized methods for their monitoring and assessment.
While optoacoustics can help generate baseline data on some taxa and their size and relative abundance, integration of DNA-based approaches (Scholin, 2010) can provide precise taxonomic information on species richness, including their response to shifts in local environmental conditions. In particular, eDNA metabarcoding allows augmented monitoring of biodiversity because it has the potential to detect organisms across the tree of life. It can be used for a variety of studies, from detecting invasive species to measuring the impact of human activities on ecosystems.
Integration of datasets obtained from eDNA, images, and other sources such as sound, can now be almost completely automated thanks to ML algorithms. Several existing coastal and deep-sea cabled observatories can host pilot studies. For those that have been in operation for many years, long-term time series of biological, and environmental data in different ecological contexts are already available hence providing solid baseline datasets (Table 1). Some of these observatories have already started to experiment with inclusion of long-term images acquisition and eDNA analyses, while others are planning to include eDNA surveys in the future. Cabled observatories and the network of IOVs operating from these platforms augmented by eDNA sensors could not only provide a framework to evaluate the effectiveness of eDNA protocols in situ, but could more importantly improve our knowledge on deep-sea biodiversity at an unprecedented spatial and temporal dimension. Under this vision, eDNA augmented observatories provide unprecedented opportunities to fill knowledge gaps on deep-sea biodiversity and ecosystem functioning, thus supporting monitoring and conservation strategies and contributing to the decade of deep-sea exploration that is now upon us.
Author Contributions
SS, LM, JA, and SM conceptualized and proposed the idea. SS and LM provided background knowledge to molecular technology and sequencing. LB, EM, and AG provided background knowledge to international conservation and management initiatives. DS and MM provided background knowledge to genetic databanks and bioinformatics. SM and FB provided background knowledge to imaging data analysis and machine learning methods. JA, FD, NC, JR, DC, and SS provided background knowledge to technology development. All authors contributed to the writing and finalization of the manuscript.
Funding
This research has been funded within the framework of the following project activities: ARIM (Autonomous Robotic Sea-Floor Infrastructure for Benthopelagic Monitoring; MarTERA ERA-Net Cofound); RESBIO (TEC2017-87861-R; Ministerio de Ciencia, Innovación y Universidades); JERICO-S3: (Horizon 2020; Grant Agreement no. 871153); ENDURUNS (Research Grant Agreement H2020-MG-2018-2019-2020 n.824348); Slovenian Research Agency (Research Core Funding Nos. P1-0237 and P1-0255 and project ARRS-RPROJ-JR-J1-3015). We also profited of the funding from the Spanish Government through the “Severo Ochoa Centre of Excellence” accreditation (CEX2019-000928-S) and Italian Ministry of Education (MIUR) under the “Bando premiale FOE 2015” (nota prot. N. 850, dd. 27 ottobre 2017) with the project EarthCruisers “EARTH’s CRUst Imagery for Investigating Seismicity, Volcanism, and Marine Natural Resources in the Sicilian Offshore”. Ocean Networks Canada was funded through Canada Foundation for Innovation-Major Science Initiative (CFI-MSI) fund 30199.
Conflict of Interest
The authors declare that the research was conducted in the absence of any commercial or financial relationships that could be construed as a potential conflict of interest.
The reviewer XT declared a shared affiliation, though no other collaboration, with several of the authors DC and JA to the handling editor.
Publisher’s Note
All claims expressed in this article are solely those of the authors and do not necessarily represent those of their affiliated organizations, or those of the publisher, the editors and the reviewers. Any product that may be evaluated in this article, or claim that may be made by its manufacturer, is not guaranteed or endorsed by the publisher.
Acknowledgments
JA, JR, and DC are members of the Research Unit Tecnoterra (ICM-CSIC/UPC), providing the conceptual framework for technological development applied to ecosystem remote, autonomous, and long-lasting multidisciplinary monitoring. The authors wish to thank the two reviewers whose work has greatly improved the manuscript.
Footnotes
- ^ https://twilightzone.whoi.edu/work-impact/technology/high-volume-edna-sampler/
- ^ https://www.iatlantic.eu/imirabilis2-expedition/science/edna_sampling/
- ^ https://wwz.ifremer.fr/gm_eng/Cruises-and-data/Years/Cruises-sheets/Welcome-to-the-blog-of-the-HERMINE-Cruise/Exploration-tools/SALSA
References
Abbott, C., Coulson, M., Gagné, N., Lacoursière-Roussel, A., Parent, G. J., Bajno, R., et al. (2021). Guidance on the Use of Targeted Environmental DNA (eDNA) Analysis for the Management of Aquatic Invasive Species and Species at Risk. Available online at: https://www.dfo-mpo.gc.ca/csas-sccs/Publications/ResDocs-DocRech/2021/2021_019-eng.html
Afzali, S.F., Bourdages, H., Laporte, M., Mérot, C., Normandeau, E., Audet, C., and Bernatchez, L. (2020). Comparing environmental metabarcoding and trawling survey of demersal fish communities in the Gulf of St. Lawrence, Canada. Environmental DNA 3, 22-42.
Agostini, M., Böemer, M., Bosma, J., Clark, K., and Danninger, M. (2020). The pacific neutrino experiment. Nat. Astron. 4, 913–915. doi: 10.1038/s41550-020-1182-4
Aguzzi, J., Albiez, J., Flögel, S., Rune, Godø, O., Grimsbø, E., et al. (2020a). A flexible autonomous robotic observatory infrastructure for bentho-pelagic monitoring. Sensors 20:1614. doi: 10.3390/s20061614
Aguzzi, J., Chatzievangelou, D., Francescangeli, M., Marini, S., Bonofiglio, F., del Rio, J., et al. (2020b). The hierarchic treatment of marine ecological information from spatial networks of benthic platforms. Sensors 20:1751. doi: 10.3390/s20061751
Aguzzi, J., Chatzievangelou, D., Company, J. B., Thomsen, L., Marini, S., Bonofiglio, F., et al. (2020c). Fish-stock assessment using video imagery from worldwide cabled observatory networks. ICES J. Mar. Sci. 77, 2396–2410. doi: 10.1093/icesjms/fsaa169
Aguzzi, J., López-Romero, D., Marini, S., Costa, C., Berry, A., Chumbinho, R., et al. (2020e). Multiparametric monitoring of fish activity rhythms in an Atlantic coastal cabled observatory. J. Mar. Syst. 212:103424. doi: 10.1016/j.jmarsys.2020.103424
Aguzzi, J., Iveša, N., Gelli, M., Costa, C., Gavrilovic, A., Cukrov, N., et al. (2020d). Ecological video monitoring of marine protected areas by underwater cabled surveillance cameras. Mar. Policy 119:104052. doi: 10.1016/j.marpol.2020.104052
Aguzzi, J., and Company, J. B. (2010). Chronobiology of deep-water decapod crustaceans on continental margins. Adv. Mar. Biol. 58, 155–225. doi: 10.1016/B978-0-12-381015-1.00003-4
Aguzzi, J., Chatzievangelou, D., Marini, S., Fanelli, E., Danovaro, R., Flögel, S., et al. (2019). New high-tech interactive and flexible networks for the future monitoring of deep-sea ecosystems. Environ. Sci. Technol. 53, 6616–6631. doi: 10.1021/acs.est.9b00409
Aguzzi, J., Company, J. B., Costa, C., Matabos, M., Azzurro, E., Mànuel, A., et al. (2012). Challenges to assessment of benthic populations and biodiversity as a result of rhythmic behaviour: video solutions from cabled observatories. Oceanogr. Mar. Biol. Annu. Rev. 50, 235–286.
Aguzzi, J., Company, J. B., Costa, C., Menesatti, P., Garcia, J. A., Bahamon, N., et al. (2011a). Activity rhythms in the deep-sea: a chronobiological approach. Front. Biosci. 16, 131–150. doi: 10.2741/3680
Aguzzi, J., Costa, C., Robert, K., Matabos, M., Antonucci, F., Juniper, K., et al. (2011b). Automated image analysis for the detection of benthic crustaceans and bacterial mat coverage using the VENUS undersea cabled network. Sensors 11, 10534–10556. doi: 10.3390/s111110534
Aguzzi, J., Costa, C., Furushima, Y., Chiesa, J. J., Company, J. B., Menesatti, P., et al. (2010). Behavioral rhythms of hydrocarbon seep fauna in relation to internal tides. Mar. Ecol. Prog. Ser. 418, 47–56. doi: 10.3354/meps08835
Aguzzi, J., Fanelli, E., Ciuffardi, T., Schirone, A., Craig, G., The, N. E. M. O., et al. (2017). Inertial bioluminescence rhythms at the central mediterranean KM3NeT deep-sea neutrino telescope. Sci. Rep. 7:44938. doi: 10.1038/srep44938
Aguzzi, J., Flögel, S., Marini, S., Thomsen, L., Lbiez, J., Weiss, P., et al. (2021a). Developing technological synergies between deep-sea and space research. Elementa. (in press).
Aguzzi, J., Nixon, B., O’Malley, C., Berry, A., Gaughan, P., Doyle, J., et al. (2021b). Burrow emergence rhythms of Nephrops norvegicus: UWTV, surveying biases and novel technological scenarios. ICES Sci. Rep. 3, 15–17. doi: 10.1038/s41598-021-85240-3
Aguzzi, J., Sbragaglia, V., Tecchio, S., Navarro, J., and Company, J. B. (2015). Rhythmic behaviour of marine benthopelagic species and the synchronous dynamics of benthic communities. Deep Sea Res. I 95, 1–11. doi: 10.1016/j.dsr.2014.10.003
Alberotanza, L., Chiggiato, J., and Profeti, G. (2004). Analysis of the seasonal stratification at the Acqua Alta Oceanographic Tower, northern Adriatic Sea. Int. J. Remote Sens. 25, 1473–1480. doi: 10.1080/01431160310001592472
Andersson, A. F., Bissett, A., Finstad, A., Fossøy, F., Grosjean, M., Hope, M., et al. (2020). Publishing DNA-Derived Data Through Biodiversity data Platforms [Community Review Draft]. Copenhagen: GBIF Secretariat.
Andruszkiewicz, E. A., Koseff, J. R., Fringer, O. B., Ouellette, N. T., Lowe, A. B., Edwards, C. A., et al. (2019). Modeling environmental DNA transport in the coastal ocean using Lagrangian particle tracking. Front. Mar. Sci. 6:477. doi: 10.3389/fmars.2019.00477
Antich, A., Palacín, C., Cebrian, E., Golo, R., Wangensteen, O. S., and Turon, X. (2021a). Marine biomonitoring with eDNA: can metabarcoding of water samples cut it as a tool for surveying benthic communities? Mol. Ecol. 30, 175–3188. doi: 10.1111/mec.15641
Antich, A., Palacin, C., Wangensteen, O. S., and Turon, X. (2021b). To denoise or to cluster, that is not the question: optimizing pipelines for COI metabarcoding and metaphylogeography. BMC Bioinformatics 22:177. doi: 10.1186/s12859-021-04115-6
Apothéloz-Perret-Gentil, L., Bouchez, A., Cordier, T., Cordonier, A., Guéguen, J., Rimet, F., et al. (2021). Monitoring the ecological status of rivers with diatom eDNA metabarcoding: a comparison of taxonomic markers and analytical approaches for the inference of a molecular diatom index. Mol. Ecol. 30, 2959–2968. doi: 10.1111/mec.15646
Apothéloz-Perret-Gentil, L., Cordonier, A., Straub, F., Iseli, J., Esling, P., and Pawlowski, J. (2017). Taxonomy-free molecular diatom index for high-throughput eDNA biomonitoring. Mol. Ecol. Resour. 17, 1231–1242. doi: 10.1111/1755-0998.12668
Ardron, G., Dunn, D., Corrigan, C., Gjerde, K., Halpin, P., Rice, J., et al. (2009). “Defining ecologically or biologically significant areas in the open oceans and deep seas: Analysis, tools, resources and illustrations,” in Proceedings of the Convention on Biological Diversity Expert Workshop on Scientific and Technical Guidance on the Use of Biogeographic Classification Systems and Identification of Marine Areas Beyond National Jurisdiction in Need of Protection, Ottowa, CA.
Atienza, S., Guardiola, M., Præbel, K., Antich, A., Turon, X., and Wangensteen, O. S. (2020). DNA metabarcoding of deep-sea sediment communities using COI: community assessment, spatio-temporal patterns and comparison with 18S rDNA. Diversity 12:123. doi: 10.3390/d12040123
Aylagas, E., and Borja, Á, and Rodríguez-Ezpeleta, N. (2014). Environmental status assessment using DNA metabarcoding: towards a genetics based marine biotic index (gAMBI). PLoS One 9:e90529. doi: 10.1371/journal.pone.0090529
Aymà, A., Aguzzi, J., Canals, M., Lastras, G., Bahamòn, N., Mecho, A., et al. (2016). Behavioural observations of deep-water fauna in submarine canyons of the Northwestern Mediterranean Sea. Deep Sea Res. I 114, 149–159. doi: 10.1016/j.dsr.2016.05.013
Baco, A. R., Etter, R. J., Ribeiro, P. A., von, der Heyden, S., Beerli, P., et al. (2016). A synthesis of genetic connectivity in deep-sea fauna and implications for marine reserve design. Mol. Ecol. 25, 3276–3298. doi: 10.1111/mec.13689
Bagley, P. M., Smith, K., Bett, B., Priede, I. G., Rowe, G., Clarke, J., et al. (2007). “Deep-ocean Environmental Long-term Observatory System (DELOS): long-term (25 year) monitoring of the deep-ocean animal community in the vicinity of offshore hydrocarbon operations,” in Proceedings of the OCEANS 2007 - Europe, VOLS 1-3, (Piscataway, NJ: IEEE Press). doi: 10.1109/OCEANSE.2007.4302250
Bailly, N., Beddard, J., Bömer, M., Bosma, J., and Brussow, D. (2021). Two-year optical site characterization for the Pacific Ocean Neutrino Experiment (P-ONE) in the Cascadia Basin. Eur. Phys. J. C. 81:1071. doi: 10.1140/epjc/s10052-021-09872-5
Banchi, E., Ametrano, C. G., Greco, S., Stanković, D., Muggia, L., and Pallavicini, A. (2020). PLANiTS: a curated sequence reference dataset for plant ITS DNA metabarcoding. Database 2020:baz155. doi: 10.1093/database/baz155
Bani, A., De Brauwer, M., Creer, S., Dumbrell, A. J., Limmon, G., Jompa, J., et al. (2020). Informing marine spatial planning decisions with environmental DNA. Adv. Ecol. Res. 62, 375–407. doi: 10.1016/bs.aecr.2020.01.011
Barnes, M. A., and Tuner, C. R. (2016). The ecology of environmental DNA and implications for conservation genetics. Conserv. Genet. 17, 1–17. doi: 10.1007/s10592-015-0775-4
Bax, N. J., Appeltans, W., Brainard, R., Duffy, J. E., Dunstan, P., Hanich, Q., et al. (2018). Linking capacity development to GOOS monitoring networks to achieve sustained ocean observation. Front. Mar. Sci. 5:346. doi: 10.3389/fmars.2018.00346
Benson, D. A., Cavanaugh, M., Clark, K., Karsch-Mizrachi, I., Lipman, D. J., Ostell, J., et al. (2013). GenBank. Nucleic Acids Res. 41, D36–D42. doi: 10.1093/nar/gks1195
Berry, O., Jarman, S., Bissett, A., Hope, M., Paeper, C., Bessey, C., et al. (2021). Making environmental DNA (eDNA) biodiversity records globally accessible. Environ. DNA 3, 699–705. doi: 10.1002/edn3.173
Berry, T. E., Saunders, B. J., Coghlan, M. L., Stat, M., Jarman, S., and Richardson, A. J. (2019). Marine environmental DNA biomonitoring reveals seasonal patterns in biodiversity and identifies ecosystem responses to anomalous climatic events. PLoS Genet. 2019:e1007943. doi: 10.1371/journal.pgen.1007943
Best, M. M., Bornhold, B. D., Juniper, S. K., and Barnes, C. R. (2007). NEPTUNE Canada regional cabled observatory: science plan. Sea Technol. 49, 10–14. doi: 10.1109/OCEANS.2007.4449316
Bicknell, A. W., Godley, B. J., Sheehan, E. V., Votier, S. C., and Witt, M. J. (2016). Camera technology for monitoring marine biodiversity and human impact. Front. Ecol. Environ. 14:424–432. doi: 10.1002/fee.1322
Brandt, A., Gutt, J., Hildebrandt, M., Pawlowski, J., Schwendner, J., Soltwedel, T., et al. (2016). Cutting the umbilical: new technological perspectives in benthic deep-sea research. J. Mar. Sci. Eng. 4:36. doi: 10.3390/jmse4020036
Brandt, M. I., Pradillon, F., Trouche, B., Henry, N., Liautard-Haag, C., Cambon-Bonavita, M.-A., et al. (2021). Evaluating sediment and water sampling methods for the estimation of deep-sea biodiversity using environmental DNA. Sci. Rep. 11:7856. doi: 10.1038/s41598-021-86396-8
Brandt, M. I., Trouche, B., Henry, N., Liautard-Haag, C., Maignien, L., de Vargas, C., et al. (2020). An assessment of environmental metabarcoding protocols aiming at favoring contemporary biodiversity in inventories of deep-sea communities. Front. Mar. Sci. 7:234. doi: 10.3389/fmars.2020.00234
Bucklin, A., Peijnenburg, K. T. C. A., Kosobokova, K. N., O’Brien, T. D., Blanco-Bercial, L., Cornils, A., et al. (2021). Toward a global reference database of COI barcodes for marine zooplankton. Mar. Biol. 168:78. doi: 10.1007/s00227-021-03887-y
Callahan, B. J., McMurdie, P. J., Rosen, M. J., Han, A. W., Johnson, A. J. A., and Holmes, S. P. (2016). DADA2: high-resolution sample inference from Illumina amplicon data. Nat. Methods 13, 581–583. doi: 10.1038/nmeth.3869
Canals, O., Mendibil, I., Santos, M., Irigoien, X., and Rodríguez-Ezpeleta, N. (2021). Vertical stratification of environmental DNA in the open ocean captures ecological patterns and behavior of deep-sea fishes. Limnol. Oceangr. 6, 339–347. doi: 10.1002/lol2.10213
Cannizzo, Z. J., Lausche, B., and Wenzel, L. (2021). Advancing Marine Conservation Through Ecological Connectivity: Building Better Connections for Better Protection. Berkeley: Parks Stewardship Forum. doi: 10.5070/P537354731
Canonico, G., Buttigieg, P. L., Montes, E., Muller-Karger, F. E., Stepien, C., Wright, D., et al. (2019). Global observational needs and resources for marine biodiversity. Front. Mar. Sci. 6:367. doi: 10.3389/fmars.2019.00367
Carreiro-Silva, M., Ocaña, O., Braga Henriques, A., Matos, V., Sampaio, I., Porteiro, F. M., et al. (2017). Zoantharians (Hexacorallia: Zoantharia) associated with cold-water corals in the azores region: new species and associations in the deep sea. Front. Mar. Sci. 4:88. doi: 10.3389/fmars.2017.00088
Chapman, A. S. A., Beaulieu, S. E., Colaço, A., Gebruk, A. V., Hilario, A., Kihara, T. C., et al. (2019). sFDvent: a global trait database for deep-sea hydrothermal-vent fauna. Glob. Ecol. Biogeogr. 28, 1538–1551. doi: 10.1111/geb.12975
Chatzievangelou, D., Aguzzi, J., Ogston, A., Suárez, A., and Thomsen, L. (2020). Spatio-temporal monitoring of key deep-sea megafauna with Internet Operated crawlers as a tool for ecological status assessment. Prog. Oceanogr. 184:102321. doi: 10.1016/j.pocean.2020.102321
Chatzievangelou, D., Bahamon, N., Martini, S., del Rio, J., Riccobene, G., Tangherlini, M., et al. (2021). Integrating diel vertical migrations of bioluminescent deep scattering layers into monitoring programs. Front. Mar. Sci. 8:661809.
Chatzievangelou, D., Doya, C., Mihály, S., Sastri, A. R., Thomsen, L., and Aguzzi, J. (2016). High-frequency patterns in the abundance of benthic species near a cold-seep: an internet operated vehicle application. PLoS One 11:e0163808. doi: 10.1371/journal.pone.0163808
Chauvet, P., Metaxas, A., Hay, A. E., and Matabos, M. (2018). Annual and seasonal dynamics of deep-sea megafaunal epibenthic communities in Barkley Canyon (British Columbia, Canada): a response to climatology, surface productivity and benthic boundary layer variation. Prog. Oceanogr. 169, 89–105. doi: 10.1016/j.pocean.2018.04.002
Clark, K., Karsch-Mizrachi, I., Lipman, D. J., Ostell, J., and Sayers, E. W. (2016). GenBank. Nucleic Acids Res. 44, D67–D72. doi: 10.1093/nar/gkv1276
Closek, C. J., Santora, J. A., Starks, H. A., Schroeder, I. D., Andruszkiewicz, E. A., Sakuma, K. M., et al. (2019). Marine vertebrate biodiversity and distribution within the central california current using environmental DNA (eDNA) metabarcoding and ecosystem surveys. Front. Mar. Sci. 6:732. doi: 10.3389/fmars.2019.00732
Colas, F., Tardivel, M., Perchoc, M., Lunven, M., Forest, B., and Guyader, G. (2018). The ZooCAM, a new in-flow imaging system for fast onboard counting, sizing and classification of fish eggs and metazooplankton. Prog. Oceanogr. 166, 54–65. doi: 10.1016/j.pocean.2017.10.014
Collins, R. A., Wangensteen, O. S., O’Gorman, E. J., Mariani, S., Sims, D. W., and Genner, M. J. (2018). Persistence of environmental DNA in marine systems. Commun. Biol. 1:185. doi: 10.1038/s42003-018-0192-6
Colloca, F., Cardinale, M., Belluscio, A., and Ardizzone, G. (2003). Pattern of distribution and diversity of demersal assemblages in the central Mediterranean Sea. Estuarine Coast. Shelf. Sci. 56, 469–480. doi: 10.1016/S0272-7714(02)00196-8
Cordier, T., Esling, P., Lejzerowicz, F., Visco, J., Ouadahi, A., Martins, C., et al. (2017). Predicting the ecological quality status of marine environments from eDNA metabarcoding data using supervised machine learning. Environ. Sci. Technol. 51, 9118–9126. doi: 10.1021/acs.est.7b01518
Cordier, T., Forster, D., Dufresne, Y., Martins, C. I. M., Stoeck, T., and Pawlowski, J. (2018). Supervised machine learning outperforms taxonomy-based environmental DNA metabarcoding applied to biomonitoring. Mol. Ecol. Resour. 18, 1381–1391. doi: 10.1111/1755-0998.12926
Cordier, T., Frontalini, F., Cermakova, K., Apothéloz-Perret-Gentil, L., Treglia, M., Scantaburlo, E., et al. (2019a). Multi-marker eDNA metabarcoding survey to assess the environmental impact of three offshore gas platforms in the North Adriatic Sea (Italy). Mar. Environ. Res. 146, 24–34. doi: 10.1016/j.marenvres.2018.12.009
Cordier, T., Lanzén, A., Apothéloz-Perret-Gentil, L., Stoeck, T., and Pawlowski, J. (2019b). Embracing environmental genomics and machine learning for routine biomonitoring. Trends Microbiol. 27, 387–397. doi: 10.1016/j.tim.2018.10.012
Costa, C., Fanelli, E., Marini, S., Danovaro, R., and Aguzzi, J. (2020). Global deep-sea biodiversity research trends highlighted by science mapping approach. Front. Mar. Sci. 7:384. doi: 10.3389/fmars.2020.00384
Costello, M. J., and Chaudhary, C. (2017). Marine biodiversity, biogeography, deep-sea gradients, and conservation. Curr. Biol. 27, R511–R527. doi: 10.1016/j.cub.2017.06.015
Costello, M. J., Bouchet, P., Boxshall, G., Fauchald, K., Gordon, D., Hoeksema, B. W., et al. (2013). Global coordination and standardisation in marine biodiversity through the world register of marine species (WoRMS) and related databases. PLoS One 8:e51629. doi: 10.1371/journal.pone.0051629
Costello, M. J., Cheung, A., and De Hauwere, N. (2010). Surface area and the seabed area, volume, depth, slope, and topographic variation for the world’s seas, oceans, and countries. Environ. Sci. Technol. 44, 8821–8828. doi: 10.1021/es1012752
Cowart, D. A., Matabos, M., Brandt, M. I., Marticorena, J., and Sarrazin, J. (2020). Exploring environmental DNA (eDNA) to assess biodiversity of hard substratum faunal communities on the lucky strike vent field (Mid-Atlantic Ridge) and investigate recolonization dynamics after an induced disturbance. Front. Mar. Sci. 6:783.
Cristescu, M. E., and Hebert, P. D. N. (2018). Uses and misuses of environmental DNA in biodiversity science and conservation. Ann. Rev. Ecol. Evol. Syst. 49, 209–230. doi: 10.1146/annurev-ecolsys-110617-062306
Danovaro, R., Aguzzi, J., Fanelli, E., Billet, D., Gjerde, K., Jamieson, A., et al. (2017a). A new international ecosystem-based strategy for the global deep ocean. Science 355, 452–454. doi: 10.1126/science.aah7178
Danovaro, R., Corinaldesi, C., Dell’Anno, A., and Snelgrove, P. V. R. (2017b). The deep-sea under global change. Curr. Biol. 27, R461–R465. doi: 10.1016/j.cub.2017.02.046
Danovaro, R., Fanelli, E., Aguzzi, J., Billett, D., Carugati, L., Corinaldesi, C., et al. (2020). Ecological variables for developing a global deep-ocean monitoring and conservation strategy. Nat. Ecol. Evol. 4, 181–192. doi: 10.1038/s41559-019-1091-z
Danovaro, R., Gambi, C., Dell’Anno, A., Corinaldesi, C., Fraschetti, S., Vanreusel, A., et al. (2008). Exponential decline of deep-sea ecosystem functioning linked to benthic biodiversity loss. Curr. Biol. 18, 1–8. doi: 10.1016/j.cub.2007.11.056
Davidov, K., Iankelevich-Kounio, E., Yakovenko, I., Koucherov, Y., Rubin-Blum, M., and Oren, M. (2020). Identification of plastic-associated species in the Mediterranean Sea using DNA metabarcoding with Nanopore MinION. Sci. Rep. 10:17533. doi: 10.1038/s41598-020-74180-z
Deiner, K., Bik, H. M., Mächler, E., Seymour, M., Lacoursière-Roussel, A., Altermatt, F., et al. (2017). Environmental DNA metabarcoding: transforming how we survey animal and plant communities. Mol. Ecol. 26, 5872–5895. doi: 10.1111/mec.14350
del Rio, J., Nogueras, M., Aguzzi, J., Toma, D., Masmitja, I., Carandell, M., et al. (2020). A decadal balance for a cabled observatory deployment. IEEE Access 8, 33163–33177. doi: 10.1109/access.2020.2973771
Dewey, R., Round, A., Macoun, P., Vervynck, J., and Tunnicliffe, V. (2007). “The VENUS cabled observatory: engineering meets science on the seafloor,” in Proceedings of the OCEANS 2007, (Vancouver, BC).
DFO (2020). Advice on the Use of Targeted Environmental DNA (eDNA) Analysis for the Management of Aquatic Invasive Species and Species at Risk. Available Online at: https://publications.gc.ca/collections/collection_2021/mpo-dfo/fs70-6/Fs70-6-2020-058-eng.pdf
Djurhuus, A., Port, J., Closek, C. J., Yamahara, K. M., Romero-Maraccini, O., Walz, K. R., et al. (2017). Evaluation of filtration and DNA extraction methods for environmental DNA biodiversity assessments across multiple trophic levels. Front. Mar. Sci. 4:314.
Doya, C., Aguzzi, J., Chatzievangelou, D., Costa, C., Company, J. B., and Tunnicliffe, V. (2015). The seasonal use of small-scale space by benthic species in a transiently hypoxic area. J. Mar. Syst. 154, 280–290.
Doya, C., Aguzzi, J., Pardo, M., Matabos, M., Company, J. B., Costa, C., et al. (2014). Diel behavioral rhythms in the sablefish (Anoplopoma fimbria) and other benthic species, as recorded by deep-sea cabled observatories in Barkley canyon (NEPTUNE-Canada). J. Mar. Syst. 130, 69–78. doi: 10.1016/j.jmarsys.2013.04.003
Doya, C., Chatzievangelou, D., Bahamon, N., Purser, A., De Leo, F., Juniper, K., et al. (2017). Seasonal monitoring of deep-sea cold-seep benthic communities using an Internet Operated Vehicle (IOV). PLoS One 12:e0176917. doi: 10.1371/journal.pone.0176917
Dully, V., Balliet, H., Frühe, L., Däumer, M., Thielen, A., Gallie, S., et al. (2021a). Robustness, sensitivity and reproducibility of eDNA metabarcoding as an environmental biomonitoring tool in coastal salmon aquaculture–an inter-laboratory study. Ecol. Indic. 121:107049. doi: 10.1016/j.ecolind.2020.107049
Dully, V., Wilding, T. A., Mühlhaus, T., and Stoeck, T. (2021b). Identifying the minimum amplicon sequence depth to adequately predict classes in eDNA-based marine biomonitoring using supervised machine learning. Comput. Struct. Biotechnol. J. 19, 2256–2268. doi: 10.1016/j.csbj.2021.04.005
Dunlop, K. M., Jarvis, T., Benoit-Bird, K. J., Waluk, C. M., Caress, D. W., Thomas, H., et al. (2018). Detection and characterisation of deep-sea benthopelagic animals from an autonomous underwater vehicle with a multibeam echosounder: a proof of concept and description of data-processing methods. Deep Sea Res. Part I: Oceanogr. Res. Papers 134, 64–79. doi: 10.1016/j.dsr.2018.01.006
Easson, C. G., Boswell, K. M., Tucker, N., Warren, J. D., and Lopez, J. V. (2020). Combined eDNA and acoustic analysis diel vertical migration of mixed consortia in the gulf of Mexico. Front. Mar. Sci. 7:552.
Eble, J. A., Daly-Engel, T. S., DiBattista, J. D., Koziol, A., and Gaither, M. R. (2020). “Chapter two - marine environmental DNA: approaches, applications, and opportunities,” in Advances in Marine Biology, ed. C. Sheppard (Cambridge, MA: Academic Press). doi: 10.1016/bs.amb.2020.01.001
Everett, M. V., and Park, L. K. (2018). Exploring deep-water coral communities using environmental DNA. Deep Sea Res. Part II Top. Stud. Oceanogr. 150, 229–241. doi: 10.11646/zootaxa.4878.3.2
Fanelli, E., Aguzzi, J., Marini, S., del Rio, J., Nogueras, M., Canese, S., et al. (2020). Towards Naples Ecological REsearch for Augmented observatories (NEREA): the NEREA-fix module, a stand-alone platform for long-term deep-sea ecosystem monitoring. Sensors 20:2911. doi: 10.3390/s20102911
Favali, P., Laura, B., and Angelo, D. S. (2015). Seafloor Observatories: a New Vision of the Earth From the Abyss. Berlin: Springer.
Feio, M. J., Serra, S. R. Q., Mortágua, A., Bouchez, A., Rimet, F., Vasselon, V., et al. (2020). A taxonomy-free approach based on machine learning to assess the quality of rivers with diatoms. Sci. Total Environ. 722:137900. doi: 10.1016/j.scitotenv.2020.137900
Fischer, P., Brix, H., Baschek, B., Kraberg, A., Brand, M., Cisewski, B., et al. (2020). Operating cabled underwater observatories in rough shelf-sea environments: a technological challenge. Front. Mar. Sci. 7:551.
Fraija-Fernández, N., Bouquieaux, M.-C., Rey, A., Mendibil, I., Cotano, U., and Irigoien, I. (2020). Marine water environmental DNA metabarcoding provides a comprehensive fish diversity assessment and reveals spatial patterns in a large oceanic area. Ecol. Evol. 10, 7560–7584. doi: 10.1002/ece3.6482
Frühe, L., Cordier, T., Dully, V., Breiner, H.-W., Lentendu, G., Pawloski, J., et al. (2021). Supervised machine learning is superior to indicator value inference in monitoring the environmental impacts of salmon aquaculture using eDNA metabarcodes. Mol. Ecol. 30, 2988–3006. doi: 10.1111/mec.15434
Gage, J. D., and Tyler, P. A. (1992). Deep-Sea Biology. a Natural History of Organisms at the Deep-Sea Floor. Cambridge, UK: Cambridge University Press.
Garcia-Vazquez, E., Georges, O., Fernandez, S., and Ardura, A. (2021). eDNA metabarcoding of small plankton samples to detect fish larvae and their preys from Atlantic and Pacific waters. Sci. Rep. 11:7224. doi: 10.1038/s41598-021-86731-z
Gemeinholzer, B., Vences, M., Beszteri, B., Bruy, T., Felden, J., Kostadinov, I., et al. (2020). Data storage and data re-use in taxonomy—the need for improved storage and accessibility of heterogeneous data. Org. Divers. Evol. 20, 1–8. doi: 10.1007/s13127-019-00428-w
Glover, A. G., Gooday, A. J., Bailey, D. M., Billett, D. S. M., Chevaldonné, P., Colaço, A., et al. (2010). Temporal change in deep-sea benthic ecosystems: a review of the evidence from recent time-series studies. Adv. Mar. Biol. 58, 1–95. doi: 10.1016/B978-0-12-381015-1.00001-0
Glover, A. G., Higgs, N., and Horton, T. (2021). World Register of Deep-Sea species (WoRDSS). Available Online at http://www.marinespecies.org/deepsea (accessed July 15, 2021).
Glover, A. G., Wiklund, H., Chen, C., and Dahlgren, T. G. (2018). Managing a sustainable deep-sea ‘blue economy’ requires knowledge of what actually lives there. eLife 7:e41319. doi: 10.7554/eLife.41319
Gold, Z., Sprague, J., Kushner, D. J., Zerecero Marin, E., and Barber, P. H. (2021). eDNA metabarcoding as a biomonitoring tool for marine protected areas. PLoS One 16:e0238557. doi: 10.1371/journal.pone.0238557
Goldberg, C. S., Turner, C. R., Deiner, K., Klymus, K. E., Thomsen, P. F., Murphy, M. A., et al. (2016). Critical considerations for the application ofenvironmental DNA methods to detect aquatic species. Methods Ecol. Evol. 7, 1299–1307. doi: 10.1111/2041-210x.12595
Goricki, Š, Stanković, D., Snoj, A., Kuntner, M., Jeffery, W. R., Trontelj, P., et al. (2017). Environmental DNA in subterranean biology: range extension and taxonomic implications for Proteus. Sci. Rep. 7:45054. doi: 10.1038/srep45054
Gorsky, G., Ohman, M. D., Picheral, M., Gasparini, S., Stemmann, L., Romagnan, J.-B., et al. (2010). Digital zooplankton image analysis using the ZooScan integrated system. J. Plankton Res. 32, 285–303. doi: 10.1093/plankt/fbp124
Govindarajan, A. F., Francolini, R. D., Jech, J. M., Lavery, A. C., Llopiz, J. K., Wiebe, P. H., et al. (2021). Exploring the use of environmental DNA (eDNA) to detect animal taxa in the mesopelagic zone. Front. Ecol. Evol. 9:574877.
Guardiola, M., Uriz, M. J., Taberlet, P., Coissac, E., Wangensteen, O. S., and Turon, X. (2016a). Deep-sea, deep-sequencing: metabarcoding extracellular DNA from sediments of marine canyons. PLOS one 11:e0153836.
Guardiola, M., Wangensteen, O. S., Taberlet, P., Coissac, E., Uriz, M. J., and Turon, X. (2016b). Spatiotemporal monitoring of deep-sea communities using metabarcoding of sediment DNA and RNA. PeerJ 4:e2807.
Guillou, L., Bachar, D., Audic, S., Bass, D., Berney, C., Bittner, L., et al. (2013). The Protist Ribosomal Reference database (PR2): a catalog of unicellular eukaryote small sub-unit rRNA sequences with curated taxonomy. Nucleic Acids Res. 41, D597–D604. doi: 10.1093/nar/gks1160
Günther, B., Marre, S., Defois, C., Merzi, T., Blanc, P., Peyret, P., et al. (2021). Capture by hybridization for full-length barcode-based eukaryotic and prokaryotic biodiversity inventories of deep sea ecosystems. Mol. Ecol. Resour. Online ahead of print. doi: 10.1111/1755-0998.13500
Hajibabaei, M., and McKenna, C. (2012). DNA mini-barcodes. Methods Mol. Biol. 858, 339–353. doi: 10.1007/978-1-61779-591-6_15
Hansen, B. K., Jacobsen, M. W., Middelboe, A. L., Preston, C. L., Marin, R., Bekkevold, D., et al. (2020). Remote, autonomous real-time monitoring of environmental DNA from commercial fish. Sci. Rep. 10:13272. doi: 10.1038/s41598-020-70206-8
Harrison, J. B., Sunday, J. M., and Rogers, S. M. (2019). Predicting the fate of eDNA in the environment and implications for studying biodiversity. Proc. R. Soc. B 286:20191409. doi: 10.1098/rspb.2019.1409
Hatch, A., Liew, H., Hourdez, S., and Rouse, G. (2020). Hungry scale worms: phylogenics of peinaleopolynoe (Polynoidae, Annelida), with four new species. Zookeys 932, 27–74. doi: 10.3897/zookeys.932.48532
Heidelberg, K. B., Gilbert, J., and Joint, I. (2010). Marine genomics: at the interface of marine microbial ecology and biodiscovery. Microb. Biotechnol. 3, 531–543.
He, S., Peng, Y., Jin, Y., Wan, B., and Liu, G. (2020). Review and analysis of key techniques in marine sediment sampling. Chin. J. Mech. Eng. 33:66.
He, X., Gilmore, S. R., Sutherland, T. F., Hajibabaei, M., Miller, K. M., Westfall, K. M., et al. (2021). Biotic signals associated with benthic impacts of salmon farms from eDNA metabarcoding of sediments. Mol. Ecol. 30, 3158–3174. doi: 10.1111/mec.15814
Heberling, J. M., Miller, J. T., Noesgaard, D., Weingart, S. B., and Schigel, D. (2021). Data integration enables global biodiversity synthesis. Proc. Natl. Acad. Sci. USA 118:e2018093118. doi: 10.1073/pnas.2018093118
Hebert, P. D. N., Ratnasingham, S., and deWaard, J. R. (2003). Barcoding animal life: cytochrome oxidase subunit 1 divergences among closely related species. Proc. R. Soc. London B 270(Suppl.), S96–S99.
Higgs, N. D., and Attrill, M. J. (2015). Biases in biodiversity: wide-ranging species are discovered first in the deep sea. Front. Mar. Sci. 2:61.
Horton, T., Marsh, L., Bett, B. J., Gates, A. R., Jones, D. O. B., Benoist, N. M. A., et al. (2021). Recommendations for the standardisation of open taxonomic nomenclature for image-based identifications. Front. Mar. Sci. 8:620702.
Howe, B. M., Arbic, B. K., Aucan, J., Barnes, C. R., Bayliff, N., Becker, N., et al. (2019). SMART cables for observing the global ocean: science and implementation. Front. Mar. Sci. 6:424.
Howe, B. M., Roger, L., Fred, D., and David, K. (2011). “ALOHA cabled observatory installation,” in Proceedings of the OCEANS’11 MTS/IEEE KONA, (Waikoloa, HI).
Howell, K. L., Davies, J. S., Allcock, A. L., Braga-Henriques, A., Buhl-Mortensen, P., Carreiro-Silva, M., et al. (2019). A framework for the development of a global standardised marine taxon reference image database (SMarTaR-ID) to support image-based analyses. PLoS One 14:e0218904. doi: 10.1371/journal.pone.0218904
Howell, K. L., Hilário, A., Allcock, A. L., Bailey, D. M., Baker, M., Clark, M. R., et al. (2020). A blueprint for an inclusive, global deep-sea ocean decade field program. Front. Mar. Sci. 7:999.
Hunter, M. E., Ferrante, J. A., Meigs-Friend, G., and Ulmer, A. (2019). Improving eDNA yield and inhibitor reduction through increased water volumes and multi-filter isolation techniques. Sci. Rep. 9:5259. doi: 10.1038/s41598-019-40977-w
Ingels, J., Vanreusel, A., Pape, E., Pasotti, F., Macheriotou, L., Arbizu, P. M., et al. (2021). Ecological variables for deep-ocean monitoring must include microbiota and meiofauna for effective conservation. Nat. Ecol. Evol. 5, 27–29.
Jac, C., Desroy, N., Duchêne, J.-C., Foveau, A., Labrune, C., Lescure, L., et al. (2021). Assessing the impact of trawling on benthic megafauna: comparative study of video surveys vs. Scientific Trawling. ICES J. Mar. Sci. 78, 1636–1649. doi: 10.1093/icesjms/fsab033
Jacobsen, M. W. (2021). Autonomous in situ sampling and analysis of eDNA using an Environmental Sample Processor (ESP). ARPHA Conference Abstracts 4:e65171.
Jahanbakht, M., Xiang, W., Hanzo, L., and Azghadi, M. R. (2021). Internet of underwater things and big marine data analytics—a comprehensive survey. IEEE Commun. Surveys Tutorials 23, 904–956. doi: 10.1109/comst.2021.3053118
Jerde, C. L., Wilson, E. A., and Dressler, T. L. (2019). Measuring global fish species richness with eDNA metabarcoding. Mol. Ecol. Resour. 19, 19–22. doi: 10.1111/1755-0998.12929
Jetz, W., McGeoch, M. A., Guralnick, R., Ferrier, S., Beck, J., Costello, M. J., et al. (2019). Essential biodiversity variables for mapping and monitoring species populations. Nat. Ecol. Evol. 3, 539–551. doi: 10.1038/s41559-019-0826-1
Johnson, D. E., Barrio Froján, C., Bax, N., Dunstan, P., Woolley, S., Halpin, P., et al. (2019). The global ocean biodiversity initiative: promoting scientific support for global ocean governance. Aquatic Conserv. Mar. Freshw. Ecosyst. 29, 162–169. doi: 10.1007/s00267-017-0993-2
Johnson, D. E., Froján, C. B., Turner, P. J., Weaver, P., Gunn, V., Dunn, D. C., et al. (2018). Reviewing the EBSA process: improving on success. Mar. Policy 88, 75–85.
Juanes, F. (2018). Visual and acoustic sensor for early detection of biological invasions: current uses and future potential. J. Nat. Conserv. 42, 7–11. doi: 10.1016/j.jnc.2018.01.003
Juniper, S. K., Matabos, M., Mihaìly, S., Ajayamohan, R. S., Gervais, F., and Bui, A. O. V. (2013). A year in barkley canyon: a time-series observatory study of mid-slope benthos and habitat dynamics using the NEPTUNE Canada network. Deep Sea Res. II 92, 114–123.
Kawato, M., Yoshida, T., Miya, M., Tsuchida, S., Nagano, Y., Nomura, M., et al. (2021). Optimization of environmental DNA extraction and ampli?cation methods for metabarcoding of deep-sea ?sh. MethodsX 8:101238. doi: 10.1016/j.mex.2021.101238
Kersten, O., Vetter, E. W., Jungbluth, M. J., Smith, C. R., and Goetze, E. (2019). Larval assemblages over the abyssal plain in the Pacific are highly diverse and spatially patchy. PeerJ 7:e7691. doi: 10.7717/peerj.7691
Kitahashia, T., Watanabea, H. K., Tsuchiyaa, M., Yamamotob, H., and Yamamoto, H. (2018). A new method for acquiring images of meiobenthic images using the FlowCAM. MethodsX 5, 1330–1335. doi: 10.1016/j.mex.2018.10.012
Kolda, A., Gavrilović, A., Jug-Dujaković, J., Ljubešić, Z., El-Matboulie, M., Lillehaugf, A., et al. (2020). Profiling of bacterial assemblages in the marine cage farm environment, with implications on fish, human and ecosystem health. Ecol. Indic. 118:106785. doi: 10.1016/j.ecolind.2020.106785
Koschinsky, A., Heinrich, L., Boehnke, K., Cohrs, J. C., Markus, T., Shani, M., et al. (2018). Deep-sea mining: interdisciplinary research on potential environmental, legal, economic, and societal implications. Integr. Environ. Assess. Manag. 14, 672–691. doi: 10.1002/ieam.4071
Kroodsma, D. A., Mayorga, J., Hochberg, T., Miller, N. A., Boerder, K., Ferretti, F., et al. (2018). Tracking the global footprint of fisheries. Science 359, 904–908. doi: 10.1126/science.aao5646
Kutti, T., Johnsen, I. A., Skaar, K. S., Ray, J. L., Husa, V., and Dahlgren, T. G. (2020). Quantification of eDNA to map the distribution of cold-water coral reefs. Front. Mar. Sci. 7:446.
Lacoursière-Roussel, A., Howland, K., Normandeau, E., Grey, E. K., Archambault, P., Deiner, K., et al. (2018). eDNA metabarcoding as a new surveillance approach for coastal Arctic biodiversity. Ecol. Evol. 8, 7763–7777. doi: 10.1002/ece3.4213
Langenkämper, D., van Kevelaer, R., Purser, A., and Nattkemper, T. W. (2020). Gear-induced concept drift in marine images and its effect on deep learning classification. Front. Mar. Sci. 7:506.
Laroche, O., Kersten, O., Smith, C. R., and Goetze, E. (2020a). Environmental DNA surveys detect distinct metazoan communities across abyssal plains and seamounts in the western clarion clipperton zone. Mol. Ecol. 29, 4588–4604. doi: 10.1111/mec.15484
Laroche, O., Kersten, O., Smith, C. R., and Goetze, E. (2020b). From sea surface to seafloor: a benthic allochthonous eDNA survey for the abyssal ocean. Front. Mar. Sci. 7:682.
Laroche, O., Wood, S. A., Tremblay, L. A., Ellis, J. I., Lear, G., and Pochon, X. (2018). A cross-taxa study using environmental DNA/RNA metabarcoding to measure biological impacts of offshore oil and gas drilling and production operations. Mar. Pollut. Bull. 127, 97–107. doi: 10.1016/j.marpolbul.2017.11.042
Le, J. T., Levin, L. A., Lejzerowicz, F., Cordier, T., Gooday, A. J., and Pawlowski, J. (2021). Scientific and budgetary tradeoffs between morphological and molecular methods for deep-sea biodiversity assessment. Integr. Environ. Assess. Manag. Online ahead of print. doi: 10.1002/ieam.4466
LeBlanc, F., Belliveau, V., Watson, E., Coomber, C., Simard, N., DiBacco, C., et al. (2020). Environmental DNA (eDNA) detection of marine aquatic invasive species (AIS) in Eastern Canada using a targeted species-specific qPCR approach. Manag. Biol. Invasions 11, 201–217. doi: 10.3391/mbi.2020.11.2.03
Leray, M., and Machida, R. J. (2020). Seabed mining could come at a high price for a unique fauna. Mol. Ecol. 29, 506–4509. doi: 10.1111/mec.15678
Leray, M., Yang, J. Y., Meyer, C. P., Mills, S. C., Agudelo, N., and Ranwez, V. (2013). A new versatile primer set targeting a short fragment of the mitochondrial COI Region for metabarcoding metazoan diversity: application for characterizing coral reef fish gut contents. Front. Zool. 10:34. doi: 10.1186/1742-9994-10-34
Levin, L. A., Amon, D. J., and Lily, H. (2020). Nature challenges to the sustainability of deep-seabed mining. Nat. Sustain. 3, 784–794. doi: 10.1038/s41893-020-0558-x
Levin, L. A., and Le Bris, N. (2015). The deep ocean under climate change. Science 350, 766–768. doi: 10.1126/science.aad0126
Levin, L. A., Bett, B. J., Gates, A. R., Heimbach, P., Howe, B. M., Janssen, F., et al. (2019). Global observing needs in the deep ocean. Front. Mar. Sci. 6:241.
Lewis, N., Day, J. C., Wilhelm, A., Wagner, D., Gaymer, C., Parks, J., et al. (2017). Large-Scale Marine Protected Areas: Guidelines for design and Management. Best Practice Protected Area Guidelines Series, No. 26. Switzerland: IUCN.
Lins, L., Zeppilli, D., Menot, L., Michel, L. N., Bonifácio, P., Brandt, M., et al. (2021). Toward a reliable assessment of potential ecological impacts of deep- sea polymetallic nodule mining on abyssal infauna. Limnol. Oceanogr. Methods 19, 626–650.
Liu, J., and Zhang, H. (2021). Combining multiple markers in environmental DNA metabarcoding to assess deep-sea benthic biodiversity. Front. Mar. Sci. 8:684955.
Lopez-Vasquez, V., Lopez-Guede, J.-M., Marini, S., Fanelli, E., Johnsen, E., and Aguzzi, J. (2020). Video-imaging enhancement and machine learning pipeline for animal tracking and classification at cabled observatories. Sensors 20:726. doi: 10.3390/s20030726
Malde, K., Handegard, N. O., Eikvil, L., and Salberg, A.-B. (2020). Machine intelligence and the data-driven future of marine science. ICES J. Mar. Sci. 77, 1274–1285. doi: 10.1093/icesjms/fsz057
Manea, E., Bianchelli, S., Fanelli, E., Danovaro, R., and Gissi, E. (2020). Towards an ecosystem-based marine spatial planning in the deep Mediterranean Sea. Sci. Total Environ. 715:136884. doi: 10.1016/j.scitotenv.2020.136884
Mariani, S., Baillie, C., Colosimo, G., and Riesgo, A. (2019). Sponges as natural environmental DNA samplers. Curr. Biol. 29, R401–R402. doi: 10.1016/j.cub.2019.04.031
Marini, S., Fanelli, E., Sbragaglia, V., Azzurro, E., del Rio, J., and Aguzzi, J. (2018). Tracking fish abundance by underwater image recognition. Sci. Rep. 8:13748. doi: 10.1038/s41598-018-32089-8
Martin, M. (2011). Cutadapt removes adapter sequences from high-throughput sequencing reads. EMBnet J. 17, 10–12. doi: 10.1089/cmb.2017.0096
Martini, S., Guasco, S., Michotey, V., Casalot, L., Garel, M., Lefevre, D., et al. (2013). Temporal survey, presence and activity of bioluminescent bacteria at the deep ANTARES station (Mediterranean Sea, 2200 m depth). Rapp. Comm. Int. Mer Médit. 40:422.
Martini, S., Nerini, D., and Tamburini, C. (2014). Relation between deep bioluminescence and oceanographic variables: a statistical analysis using time–frequency decompositions. Prog. Oceanogr. 127, 117–128. doi: 10.1016/j.pocean.2014.07.003
Matabos, M., Aguzzi, J., Robert, K., Costa, C., Menesatti, P., Company, J. B., et al. (2011). Multi-parametric study of behavioural modulation in demersal decapods at the VENUS cabled observatory in Saanich Inlet, British Columbia, Canada. J. Exp. Mar. Biol. Ecol. 401, 89–96.
Matabos, M., Bui, A. O., Mihály, S., Aguzzi, J., Juniper, D. S. K., and Ajayamohan, R. S. (2014). High-frequency study of benthic megafaunal community dynamics in Barkley canyon: a multidisciplinary approach using the NEPTUNE Canada network. J. Mar. Syst. 130, 56–68. doi: 10.1016/j.jmarsys.2013.05.002
Matabos, M., Piechaud, N., De Montigny, F., Sarradin, P. M., and Sarrazin, J. (2015). The VENUS cabled observatory as a method to observe fish behaviour and species assemblages in a hypoxic fjord, Saanich Inlet (British Columbia, Canada). Can. J. Fish. Aquat. Sci. 72, 24–36. doi: 10.1139/cjfas-2013-0611
Mathon, L., Valentini, A., Guérin, P. E., Normandeau, E., Noel, C., Lionnet, C., et al. (2021). Benchmarking bioinformatic tools for fast and accurate eDNA metabarcoding species identification. Mol. Ecol. Resour. 21, 2565–2579. doi: 10.1111/1755-0998.13430
Mathur, M., Vasudev, D., Sahoo, S., Jain, D., and Goel, N. (2020). Crosspooled FishNet: transfer learning based fish species classification model. Multimed. Tools Appl. 79, 31625–31643. doi: 10.1007/s11042-020-09371-x
McClenaghan, B., Fahner, N., Cote, D., Chawarski, J., McCarthy, A., Rajabi, H., et al. (2020). Harnessing the power of eDNA metabarcoding for the detection of deep-sea fishes. PLoS One 15:e0236540. doi: 10.1371/journal.pone.0236540
McCowin, M., Feehery, C., and Rouse, G. (2020). Spanning the depths or depth-restricted: three new species of Bathymodiolus (Bivalvia, Mytilidae) and a new record for the hydrothermal vent Bathymodiolus thermophilus at methane seeps along the Costa Rica margin. Deep Sea Res. Part I: Oceanogr. Res. Papers 164:103322. doi: 10.1016/j.dsr.2020.103322
McIntyre, A. (2010). Life in the World’s Oceans: Diversity, Distribution, and Abundance. Chichester: Wiley-Blackwell.
Merten, V., Bayer, T., Reusch, T. B. H., Puebla, O., Fuss, J., Stefanschitz, J., et al. (2021). An integrative assessment combining deep-sea net sampling, in situ observations and eDNA analysis identifies Cabo Verde as a cephalopod biodiversity hotspot in the Atlantic Ocean. Front. Mar. Sci. 8:760108.
Meusnier, I., Singer, G. A., Landry, J. F., Hickey, J.-F., Hebert, P. D. N., and Hajibabaei, M. (2008). A universal DNA mini-barcode for biodiversity analysis. BMC Genomics 9:214. doi: 10.1186/1471-2164-9-214
Meyer, C. P., and Paulay, G. (2005). DNA barcoding: error rates based on comprehensive sampling. PLoS Biol. 3:e422. doi: 10.1371/journal.pbio.0030422
Milligan, R. J., Scott, E. M., Jones, D. O. B., Bett, J. B., Jemieson, A. J., and O’Brien, R. (2020). Evidence for seasonal cycles in deep-sea fish abundances: a great migration in the deep SE Atlantic? J. Anim. Ecol. 89, 1593–1603. doi: 10.1111/1365-2656.13215
Mirimin, L., Desmet, S., López Romero, D., Fernandez Fernandez, S., Miller, D., Mynott, S., et al. (2021). Don’t catch me if you can – Using cabled observatories as multidisciplinary platforms for marine fish community monitoring: a case study combining underwater video and environmental DNA (eDNA) data. Sci. Total Environ. 773:145351. doi: 10.1016/j.scitotenv.2021.145351
Mooney, T. A., Iorio, L. D., Lammers, M., Lin, T.-H., Nedelec, S. L., Parsons, M., et al. (2020). Listening forward: approaching marine biodiversity assessments using acoustic methods. R. Soc. Open Sci. 7:201287. doi: 10.1098/rsos.201287
Moore, S. K., Mickett, J. B., Doucette, G. J., Adams, N. G., Mikulski, C. M., Birch, J. M., et al. (2021). An autonomous platform for near real-time surveillance of harmful algae and their toxins in dynamic coastal shelf environments. J. Mar. Sci. Eng. 9:336. doi: 10.3390/jmse9030336
Morato, T., Hoyle, S. D., Allain, V., and Nicol, S. J. (2010). Seamounts are hotspots of pelagic biodiversity in the open ocean. Proc. Nat. Acad. Sci. 107, 9707–9711. doi: 10.1073/pnas.0910290107
Murakami, H., Yoon, S., Kasai, A., Minamoto, T., Yamamoto, S., Sakata, M. K., et al. (2019). Dispersion and degradation of environmental DNA from caged fish in a marine environment. Fish Sci. 85, 327–337. doi: 10.1007/s12562-018-1282-6
Nattkemper, T., Osterloff, J., Nilssen, I., Van Engeland, T., Buhl-Mortensen, P., and Järnegren, J. (2019). Computer vision enables short- and long-term analysis of Lophelia pertusa polyp behaviour and colour from an underwater observatory. Sci. Rep. 9:6578. doi: 10.1038/s41598-019-41275-1
O’Leary, B. C., Ban, N. C., Fernandez, M., Friedlander, A. M., García-Borboroglu, P., Golbuu, Y., et al. (2018). Addressing criticisms of large-scale marine protected areas. Bioscience 68, 359–370. doi: 10.1093/biosci/biy021
Ohman, M. D., Davis, R. E., Sherman, J. T., Grindley, K. R., Whitmore, B. M., Nickels, C. F., et al. (2018). Zooglider: an autonomous vehicle for optical and acoustic sensing of zooplankton. Limnol. Oceanogr. Methods 17, 69–86. doi: 10.1002/lom3.10301
O’Leary, B. C., and Roberts, C. M. (2018). Ecological connectivity across ocean depths: implications for protected area design. Glob. Ecol. Conserv. 15:e00431.
Osterloff, J., Nilssen, I., and Nattkemper, T. W. (2016). A computer vision approach for monitoring the spatial and temporal shrimp distribution at the LoVe observatory. Methods Oceanog. 15, 114–128.
Osterloff, J., Nilssen, I., Järnegren, J., Van Engeland, T., Buhl-Mortensen, P., and Nattkemper, T. W. (2019). Computer vision enables short-and long-term analysis of Lophelia pertusa polyp behaviour and colour from an underwater observatory. Sci. Rep. 9:6578.
Pawlowski, J., Levin, L., Recio-Blanco, X., Le, J., Lejzerowicz, F., Cordier, T., et al. (2018). Enabling the use of Environmental DNA for Regulatory Policymaking in the Marine Industrial Revolution. SNIS Project Working Paper. Available online at: https://snis.ch/wp-content/uploads/2020/01/2015_Pawlowski_Working-Paper.pdf
Pereira, H. M., Ferrier, S., Walters, M., Geller, G. N., Jongman, R. H. G., Scholes, R. J., et al. (2013). Essential biodiversity variables. Science 339, 277–278.
Pikitch, E. K., Rountos, K. J., Essington, T. E., Santora, C., Pauly, D., Watson, R., et al. (2014). The global contribution of forage fish to marine fisheries and ecosystems. Fish Fish. 15, 43–64. doi: 10.1111/faf.12004
Portman, M. E., Notarbartolo-di-Sciara, G., Agardy, T., Katsanevakis, S., Possingham, H. P., and Di-Carlo, G. (2013). He who hesitates is lost: why conservation in the Mediterranean Sea is necessary and possible now. Mar. Policy 42, 270–279.
Purser, A., Thomsen, L., Hofbauer, M., Menzel, M., Wagner, H., Chapman, R., et al. (2013). Temporal and spatial benthic data collection via internet operated deep sea crawler. Methods Oceanogr. 5, 1–18. doi: 10.1016/j.mio.2013.07.001
Pusceddu, A., Bianchelli, S., Martín, J., Puig, P., Palanques, A., Masqué, P., et al. (2014). Chronic and intensive bottom trawling impairs deep-sea biodiversity and ecosystem functioning. Proc. Nat. Acad. Sci. 111, 8861–8866. doi: 10.1073/pnas.1405454111
Quast, C., Pruesse, E., Yilmaz, P., Gerken, J., Schweer, T., Yarzaet, P., et al. (2013). The SILVA ribosomal RNA gene database project: improved data processing and web-based tools. Nucleic Acids Res. 41, D590–D596. doi: 10.1093/nar/gks1219
Ramírez, F., Afán, I., Davis, L. S., and Chiaradia, A. (2017). Biodiversity losses and conservation responses in the Anthropocene. Sci. Adv. 3:e1601198. doi: 10.1126/science.aam9317
Ramirez-Llodra, E., Tyler, P. A., Baker, M. C., Bergstad, O. A., Clark, M. R., Escobar, E., et al. (2011). Man and the last great wilderness: human impact on the deep sea. PLoS One 6:e22588. doi: 10.1371/journal.pone.0022588
Ratnasingham, S., and Hebert, P. D. (2013). A DNA-based registry for all animal species: the barcode index number (BIN) system. PLoS One 8:e66213. doi: 10.1371/journal.pone.0066213
Ratnasingham, S., and Hebert, P. D. N. (2007). BOLD: the barcode of life data system (http://www.barcodinglife.org). Mol. Ecol. Notes 7, 355–364. doi: 10.1111/j.1471-8286.2007.01678.x
Rex, M., Etter, R., Morris, J., Crouse, J., McClain, C., Johnson, N., et al. (2006). Global bathymetric patterns of standing stock and body size in the deep-sea benthos. Mar. Ecol. Progr. Ser. 317, 1–8. doi: 10.3354/meps317001
Ribeiro, H., Martins, A., Gonçalves, M., Guedes, M., Tomasino, M. P., Dias, N., et al. (2019). Development of an autonomous biosampler to capture in situ aquatic microbiomes. PLoS One 14:e0216882. doi: 10.1371/journal.pone.0216882
Rodriguez-Ezpeleta, N., Morissette, O., Bean, C. W., Manu, S., Banerjee, P., Lacoursière-Roussel, A., et al. (2021). Trade-offs between reducing complex terminology and producing accurate interpretations from environmental DNA: comment on “environmental DNA: what’s behind the term?” by Pawlowski et al., (2020). Mol. Ecol. 30, 4601–4605. doi: 10.1111/mec.15942
Rognes, T., Flouri, T., Nichols, B., Quince, C., and Mahé, F. (2016). VSEARCH: a versatile open source tool for metagenomics. PeerJ 4:e2584. doi: 10.7717/peerj.2584
Rosli, N., Leduc, D., Rowden, A. A., and Probert, K. (2017). Review of recent trends in ecological studies of deep-sea meiofauna, with focus on patterns and processes at small to regional spatial scales. Mar. Biodivers. 48, 13–34. doi: 10.1007/s12526-017-0801-5
Ross, T., and Lawson, G. (2009). Long-term broadband acoustic observations of zooplankton scattering layers in Saanich Inlet, British Columbia. J. Acoust. Soc. Am. 125, 2551–2551. doi: 10.1121/1.4783649
Rountree, R., Aguzzi, J., Marini, S., Fanelli, E., De Leo, F., Del Rio, J., et al. (2020). Towards an optimal design for ecosystem-level ocean observatories. Oceanogr. Mar. Biol. Annu. Rev. 58, 79–106. doi: 10.1201/9780429351495-2
Salter, I., Joensen, M., Kristiansen, R., Steingrund, P., and Vestergaard, P. (2019). Environmental DNA concentrations are correlated with regional biomass of Atlantic cod in oceanic waters. Commun. Biol. 2:461. doi: 10.1038/s42003-019-0696-8
Schlick-Steiner, B. C., Steiner, F. M., Seifert, B., Stauffer, C., Christian, E., and Crozier, R. H. (2010). Integrative taxonomy: a multisource approach to exploring biodiversity. Annu. Rev. Entomol. 55, 421–438. doi: 10.1146/annurev-ento-112408-085432
Scholin, C. A. (2010). What are “ecogenomic sensors?” a review and thoughts for the future. Ocean Sci. 6, 51–60. doi: 10.5194/os-6-51-2010
Scholin, C. A., Birch, J., Jensen, S., Marin, R., Massion, E., and Pargett, D. (2017). The quest to develop ecogenomic sensors: a 25-Year history of the Environmental Sample Processor (ESP) as a case study. Oceanography 30, 100–113. doi: 10.5670/oceanog.2017.427
Schroeder, A., Stanković, D., Pallavicini, A., Gionechetti, F., Pansera, M., and Camatti, E. (2020). DNA metabarcoding and morphological analysis - assessment of zooplankton biodiversity in transitional waters. Mar. Environ. Res. 160:104946. doi: 10.1016/j.marenvres.2020.104946
Seabrook, S., De Leo, F. C., and Thurber, A. R. (2019). Flipping for food: the use of a methane seep by tanner Crabs (Chionoecetes tanneri). Front. Mar. Sci. 6:43.
Seabrook, S., De Leo, F. C., Baumberger, T., Raineault, N., and Thurber, A. R. (2018). Heterogeneity of methane seep biomes in the Northeast Pacific. Deep Sea Res. Part II Top. Stud. Oceanogr. 150, 195–209. doi: 10.1016/j.dsr2.2017.10.016
Seymour, M., Edwards, F. K., Cosby, B. J., Kelly, M. G., Gary, de Bruyn, M., et al. (2020). Executing multi-taxa eDNA ecological assessment via traditional metrics and interactive networks. Sci. Total Environ. 729:138801. doi: 10.1016/j.scitotenv.2020.138801
Stat, M., Huggett, M. J., Bernasconi, R., DiBattista, J. B., Berry, T. E., Newman, S. J., et al. (2017). Ecosystem biomonitoring with eDNA: metabarcoding across the tree of life in a tropical marine environment. Sci. Rep. 7:12240. doi: 10.1038/s41598-017-12501-5
Stat, M., John, J., DiBattista, J. D., Newman, S. J., Bunce, M., and Harvey, E. S. (2019). Combined use of eDNA metabarcoding and video surveillance for the assessment of fish biodiversity. Conserv Biol. 33, 196–205. doi: 10.1111/cobi.13183
Stefanni, S., Catarino, D., Ribeiro, P., Freitas, M., Menezes, G., Neat, F., et al. (2021). Molecular systematics of the long-snouted deepwater dogfish (Centrophoridae, Deania) with implication to taxonomy and conservation. Front. Mar. Sci. 7:588192. doi: 10.3389/fmars.2020.588192
Stefanni, S., Stanković, D., Borme, D., de Olazabal, A., Juretić, T., Pallavicini, A., et al. (2018). Multi-marker metabarcoding approach to study mesozooplankton at basin scale. Sci. Rep. 8:12085. doi: 10.1038/s41598-018-30157-7
Stoeckle, M. Y., Adolf, J., Charlop-Powers, Z., Dunton, K. J., Hinks, G., and VanMorter, S. M. (2021). Trawl and eDNA assessment of marine fish diversity, seasonality, and relative abundance in coastal New Jersey, USA. ICES J. Mar. Sci. 78, 293–304. doi: 10.1093/icesjms/fsaa225
Sundberg, P., Obst, M., Dahlgren, T., Panova, M., and Axberg, A. (2020). Swedish eDNA. Gotherburg: University of Gotherburg.
Taberlet, P., Coissac, E., Hajibabaei, M., and Rieseberg, L. H. (2012). Environmental DNA. Mol. Ecol. 21, 1789–1793. doi: 10.1111/j.1365-294X.2012.05542.x
Tamburini, C., Canals, M., Durrieu, de Madron, X., Houpert, L., Lefèvre, D., et al. (2013). Deep-sea bioluminescence blooms after dense water formation at the ocean surface. PLoS One 8:e67523. doi: 10.1371/journal.pone.0067523
Thomsen, L., Aguzzi, J., Costa, F. C., De Leo, F. C., Ogston, A., and Purser, A. (2017). The oceanic biological pump: rapid carbon transfer to depth at continental margins during winter. Sci. Rep. 7:10763. doi: 10.1038/s41598-017-11075-6
Thomsen, L., Barnes, C., Best, M., Chapman, R., Pirenne, B., Thomson, R., et al. (2012). Ocean circulation promotes methane release from gas hydrate outcrops at the NEPTUNE Canada Barkley Canyon node. Geophys. Res. Lett. 39, n/a–n/a. doi: 10.1029/2012GL052462
Thomsen, P. F., Møller, P. R., Sigsgaard, E. E., Knudsen, S. W., Jørgensen, O. A., and Willerslev, E. (2016). Environmental DNA from seawater samples correlate with trawl catches of subarctic, deepwater fishes. PLoS One 11:e0165252. doi: 10.1371/journal.pone.0165252
Thurber, A. R., Sweetman, A. K., Narayanaswamy, B. E., Jone, D. O. B., Ingels, J., and Hansman, R. L. (2014). Ecosystem function and services provided by the deep sea. Biogeosciences 11, 3941–3963. doi: 10.5194/bg-11-3941-2014
Trenkel, V. M., Vaz, S., Albouy, C., Brind’Amour, A., Laffargue, P., Romagnan, J. B., et al. (2019). We can reduce the impact of monitoring on marine living resources. Mar. Ecol. Prog. Ser. 609, 277–282. doi: 10.3354/meps12834
Turon, M., Angulo-Preckler, C., Antich, A., Præbel, K., and Wangensteen, O. S. (2020). More than expected from old sponge samples: a natural sampler dna metabarcoding assessment of marine fish diversity in Nha Trang Bay (Vietnam). Front. Mar. Sci. 7:605148. doi: 10.3389/fmars.2020.605148
Turon, X., Antich, A., Palacin, C., Præbel, K., and Wangensteen, O. S. (2019). From metabarcoding to metaphylogeography: separating the wheat from the chaff. Ecol. Appl. 30:e02036. doi: 10.1002/eap.2036
UNEP (2020). Zero Draft of the Post-2020 Global Biodiversity Framework. Kunming: Convention of Biological Diversity.
UNESCO (2020). Progress Towards the Sustainable Development Goals Report of the Secretary-General, High-level Political Forum on Sustainable Development, Convened Under the Auspices of the Economic and Social Council (E/2020/57). Paris: UNESCO.
Visser, F., Merten, V. J., Bayer, T., Oudejans, M. G., de Jonge, D. S. W., Puebla, O., et al. (2021). Deep-sea predator niche segregation revealed by combined cetacean biologging and eDNA analysis of cephalopod prey. Sci. Adv. 7:eabf5908. doi: 10.1126/sciadv.abf5908
Washburn, T. W., Turner, P. J., Durden, J. M., Jones, D. O. B., Weaver, P., and Van Dover, C. L. (2019). Ecological risk assessment for deep-sea mining. Ocean Coast. Manag. 176, 24–39. doi: 10.1016/j.ocecoaman.2019.04.014
Weber, S., Brink, L., Wörner, M., Künzel, S., Veith, M., Teubner, D., et al. (2021). Molecular diet analysis in zebra and quagga mussels (Dreissena spp.) and an assessment of the utility of aquatic filter feeders as biological eDNA filters. BioRxiv doi: 10.1101/2021.03.01.432951
Wedding, L. M., Friedlander, A. M., Kittinger, J. N., Watling, L., Gaines, S. D., Bennett, M., et al. (2013). From principles to practice: a spatial approach to systematic conservation planning in the deep sea. Proc. R. Soc. Lond. B 280:20131684. doi: 10.1098/rspb.2013.1684
Wedding, L. M., Reiter, S. M., Smith, C. R., Gjerde, K. M., Kittinger, J., Friedlander, A. M., et al. (2015). Managing exploitation of the deep seabed. Science 349, 144–145. doi: 10.1126/science.aac6647
Weigand, H., Beermann, A. I., Čiampor, F., Costa, F. O., Csabai, Z., et al. (2019). DNA barcode reference libraries for the monitoring of aquatic biota in Europe: gap-analysis and recommendations for future work. Sci. Total Environ. 678, 499–524. doi: 10.1016/j.scitotenv.2019.04.247
Weston, J., Carrillo-Barragan, P., Linley, T., Reid, W., and Jamieson, A. (2020). New species of Eurythenes from hadal depths of the mariana Trench, Pacific Ocean (Crustacea: Amphipoda). ZooTaxa 4748:zootaxa. doi: 10.11646/zootaxa.4748.1.9
Widder, E. A., Robison, B. H., Reisenbichler, K. R., and Haddock, S. H. D. (2005). Using red light for in situ observations of deep-sea fishes. Deep Sea Res. Part I 52, 2077–2085. doi: 10.1016/j.dsr.2005.06.007
Yamahara, K. M., Preston, C. M., Birch, J., Walz, K., Marin, R., Jensen, S., et al. (2019). In situ autonomous acquisition and preservation of marine environmental DNA using an autonomous underwater vehicle. Front. Mar. Sci. 6:373. doi: 10.3389/fmars.2019.00373
Yeh, J., and Drazen, J. C. (2011). Baited-camera observations of deep-sea megafaunal scavenger ecology on the California slope. Mar. Ecol. Prog. Ser. 424, 145–156.
Young, M. K., Isaak, D. J., Schwartz, M. K., McKelvey, K. S., Nagel, D. E., Franklin, T. W., et al. (2018). Species Occurrence data from the Aquatic eDNAtlas Database: Forest Service Research Data Archive. Madison: US Department of Agriculture.
Zhao, F., Filker, S., Xu, K., Huang, P., and Zheng, S. (2020). Microeukaryote communities exhibit phyla-specific distance-decay patterns and an intimate link between seawater and sediment habitats in the Western Pacific Ocean. Deep Res. Part I Oceanogr. Res. Pap. 160:103279. doi: 10.1016/j.dsr.2020.103279
Keywords: omics sensors, eDNA metabarcoding, genetic markers, imaging, artificial intelligence, data repositories, deep sea conservation
Citation: Stefanni S, Mirimin L, Stanković D, Chatzievangelou D, Bongiorni L, Marini S, Modica MV, Manea E, Bonofiglio F, del Rio Fernandez J, Cukrov N, Gavrilović A, De Leo FC and Aguzzi J (2022) Framing Cutting-Edge Integrative Deep-Sea Biodiversity Monitoring via Environmental DNA and Optoacoustic Augmented Infrastructures. Front. Mar. Sci. 8:797140. doi: 10.3389/fmars.2021.797140
Received: 18 October 2021; Accepted: 29 November 2021;
Published: 12 January 2022.
Edited by:
Kevin M. Kocot, University of Alabama, United StatesReviewed by:
Miriam Isabelle Brandt, Norwegian Research Institute (NORCE), NorwayXavier Turon, Spanish National Research Council (CSIC), Spain
Copyright © 2022 Stefanni, Mirimin, Stanković, Chatzievangelou, Bongiorni, Marini, Modica, Manea, Bonofiglio, del Rio Fernandez, Cukrov, Gavrilović, De Leo and Aguzzi. This is an open-access article distributed under the terms of the Creative Commons Attribution License (CC BY). The use, distribution or reproduction in other forums is permitted, provided the original author(s) and the copyright owner(s) are credited and that the original publication in this journal is cited, in accordance with accepted academic practice. No use, distribution or reproduction is permitted which does not comply with these terms.
*Correspondence: Sergio Stefanni, c2VyZ2lvLnN0ZWZhbm5pQHN6bi5pdA==; Jacopo Aguzzi, amFndXp6aUBpY20uY3NpYy5lcw==