- 1Charles Perkins Centre, The University of Sydney, Sydney, NSW, Australia
- 2School of Life and Environmental Sciences, The University of Sydney, Sydney, NSW, Australia
- 3New South Wales Department of Primary Industries, Fisheries, Sydney Institute of Marine Science, Mosman, NSW, Australia
- 4New South Wales Department of Primary Industries, Fisheries, National Marine Science Centre, Coffs Harbour, NSW, Australia
- 5Marine Ecology Research Centre, National Marine Science Centre, Southern Cross University, Coffs Harbour, NSW, Australia
- 6Nutri Lens, East Ryde, NSW, Australia
Multisensor biologging provides a powerful tool for ecological research, enabling fine-scale observation of animals to directly link physiology and movement to behavior across ecological contexts. However, applied research into behavioral disturbance and recovery following human interventions (e.g., capture and translocation) has mostly relied on coarse location-based tracking or unidimensional approaches (e.g., dive profiles and activity/energetic metrics) that may not resolve behaviors and recovery processes. Biologging can improve insights into both disturbed and natural behavior, which is critical for management and conservation initiatives, although challenges remain in objectively identifying distinct behavioral modes from complex multisensor datasets. Using white sharks (Carcharodon carcharias) released from a non-lethal catch-and-release shark bite mitigation program, we explored how combining multisensor biologging (video, depth, accelerometers, gyroscopes, and magnetometers), track reconstruction and behavioral state modeling using hidden Markov models (HMMs) can improve our understanding of behavioral processes and recovery. Biologging tags were deployed on eight white sharks, recording their continuous behaviors, movements, and environmental context (habitat, interactions with other organisms/objects) for periods of 10–87 h post-release. Dive profiles and tailbeat analysis (as a standard, activity-based method for assessing recovery) indicated an immediate “disturbed” period of offshore movement, displaying rapid tailbeats and an average tailbeat-derived recovery period of 9.7 h, with evidence of smaller individuals having longer recoveries. However, further integrating magnetometer-derived headings, track reconstruction and HMM modeling revealed a cryptic shift to diurnal clockwise-counterclockwise circling behavior, which we argue represents compelling new evidence for hypothesized unihemispheric sleep amongst elasmobranchs. By simultaneously providing critical information toward conservation-focused shark management and understudied aspects of shark behavior, our study highlights how integrating multisensor information through HMMs can improve our understanding of both post-release and natural behavior, especially in species that are difficult to observe directly.
Introduction
Establishing how animal movement and behavior shifts across ecological contexts is critical to understanding ecology, yet examining this at relevant spatiotemporal scales in free-ranging individuals presents a long-standing technical challenge (Nathan et al., 2008). Traditionally, animal movements have been recorded using biotelemetry (radio, acoustic, or satellite tracking), but geolocation-based tracking alone precludes detailed behavioral analysis beyond broader-scale movement patterns (Hussey et al., 2015; Kays et al., 2015). However, the recent advent of integrated, multisensor biologging offers new means to directly measure behavioral and physiological states, and link these to movement and the ecological contexts in which they occur (Wilmers et al., 2015). Crucially, biologging enables observation at unprecedently fine scales, providing a vital tool for both pure and applied ecology, especially in cryptic species (Bograd et al., 2010; Wilmers et al., 2015).
For pure and applied objectives, a detailed understanding of both natural behavior and responses to human intervention is paramount. Pure biologging objectives usually focus on examining natural behavior, but this requires accounting for behavioral disturbances resulting from the tagging process, including capture and handling which are often necessitated for deployments on elusive or transient species, or due to tag application requirements (e.g., rigid attachment/careful alignment for accelerometers/magnetometers; Wilson et al., 2008; Shillinger et al., 2012; but see Chapple et al., 2015; Pearson et al., 2017). The magnitude, nature and duration of post-release disturbance can vary between individuals, species and contexts (e.g., capture behavior, environmental conditions; Gallagher et al., 2014; Guida et al., 2016; Whitney et al., 2016), yet these responses are often excluded from detailed analysis as unwanted side-effects to natural behavior (e.g., Coffey et al., 2020). Conversely, understanding animals’ responses to and recovery from human disturbance is a key aim of applied behavioral research, for which comprehensive knowledge of natural behavior is also required as a reference point (Walker, 1998; Wilson et al., 2014). With increasing management and conservation concerns regarding the sublethal effects of capture in wild animals, establishing detailed insights into both natural and disturbed behaviors through biologging should be a priority where possible (Wilson et al., 2014). Careful selection of the most appropriate sensors is critical to this (Williams et al., 2020).
“Daily diary” tags represent the optimal standard for fine-scale recording of combined movement, behavior and environmental context (Wilson et al., 2008). Specifically, these tags integrate a full triaxial inertial measurement unit (IMU, accelerometer, gyroscope and magnetometer) with other sensors (e.g., temperature, pressure, and video cameras) to enable continuous three-dimensional reconstruction of movements via dead reckoning, which can then be linked to specific activities and environments (Wilson et al., 2008). This is particularly advantageous in cryptic aquatic species which are otherwise difficult or impossible to observe at fine scales, although these tags can also reveal important behavioral details beyond that of high-resolution GPS in terrestrial animals (Bidder et al., 2015). So far, daily diary tags have been used to reveal many aspects of aquatic animals’ natural behaviors including foraging activities, cost-efficient movement strategies, and habitat use (Shepard et al., 2011; Benoit-Bird et al., 2013; Andrzejaczek et al., 2019a). By contrast, applied behavioral research on disturbances following capture in marine and terrestrial systems has mostly relied on either traditional biotelemetry, pop-up time-depth archival tags, or accelerometers (Afonso and Hazin, 2014; Rode et al., 2014; Barnes et al., 2016; Becciolini et al., 2019; Bowlby et al., 2021; Shuert et al., 2021). However, biotelemetry-derived movements or depth profiles are often insufficient for resolving cryptic behavioral processes (e.g., foraging and resting) relevant to recovery, especially in aquatic systems where telemetry data are particularly intermittent and coarse (Andrzejaczek et al., 2018, 2019a). Accelerometers provide more detailed activity records useful for quantifying recovery periods (Brivio et al., 2015; Whitney et al., 2016), but complex behavioral disturbances may persist beyond unidimensional recovery thresholds estimated through accelerometry alone (Bullock et al., 2015). Recent technological advancements and increased availability of statistical methods such as hidden Markov models (HMMs) offer new means to probe the multidimensional information streams derived from multisensor biologgers and reveal otherwise hidden behavioral dynamics (Patterson et al., 2017; McClintock and Michelot, 2018; Williams et al., 2020).
Hidden Markov models are uniquely structured for this purpose because they allow time series of observations from biologgers, capturing various biologically informative aspects of behavior (e.g., body orientation, movement, and acceleration), to be combined and related to a most likely sequence of underlying (“hidden”) states which are themselves not directly observable but assumed to be causally related to the observed behaviors (e.g., pre vs. post recovery states; Leos-Barajas et al., 2017; McClintock et al., 2020). HMMs are thus advantageous relative to other more conventional techniques (e.g., linear or non-linear modeling) because they provide an objective, data-driven approach to automatically classify behavioral states using remotely collected sensor data and, importantly, can predict how each state shifts through time in response to intrinsic (e.g., biological) or extrinsic (e.g., environmental) factors (Leos-Barajas et al., 2017). Behavioral applications of HMMs have mostly focused on determining animals’ foraging patterns and movements, revealing how these are shaped by biological (e.g., age, sex; Grecian et al., 2018; Carter et al., 2020), environmental (e.g., oceanographic features; Byrnes et al., 2021; Lee et al., 2021) and anthropogenic factors (e.g., fishing activity, tourism operations; Towner et al., 2016; Mul et al., 2020). Similarly, combining biologging and HMMs offers a promising framework for better characterizing recovery processes following capture, studying natural behavior and, importantly, distinguishing between the two. Yet they are rarely applied for this purpose.
This study combined multisensor biologging and behavioral state modeling to provide new insights into the post-release behavior of a cryptic marine apex predator, the white shark (Carcharodon carcharias), a species that epitomizes both the importance and challenges of fine-scale behavioral analysis in the field. As threatened marine predators that fulfill key ecological roles (Jorgensen et al., 2019; Rigby et al., 2019; Shea et al., 2020), understanding their natural behavior is important for species and ecosystem management. Additionally, white sharks impose risks for human safety (Chapman and McPhee, 2016), and effectively mitigating these risks is complex, but important for societal dynamics as well as shark conservation (McPhee et al., 2021; Simpfendorfer et al., 2021). In New South Wales, Australia, white sharks are caught, relocated ∼1 km offshore, and released through a non-lethal mitigation approach using Shark-Management-Alert-in-Real-Time (SMART) drumlines (Tate et al., 2021a), yet their behavioral responses to capture, and hence the implications of this strategy for both sharks and people, are unknown. Although white sharks’ broad scale movements have been the subject of several studies (Jorgensen et al., 2010; Skomal et al., 2017; Spaet et al., 2020a,b; Lee et al., 2021), knowledge of their fine scale behavior remains restricted to a few specific contexts (e.g., foraging near seal colonies; Jewell et al., 2019; Semmens et al., 2019; Watanabe et al., 2019a,b). A more detailed understanding of both their post-capture responses and natural behavior across ecological contexts is thus critical to their management and conservation. Furthermore, given the challenges of fine-scale behavioral observations in cryptic predators generally, improved insights into the behavior of any one species could provide valuable information for understanding the behavioral ecology of predators more broadly (Machovsky-Capuska et al., 2016).
The primary aim of our study was to use white sharks to explore how combined multisensor biologging, track reconstruction and behavioral state modeling (using HMMs and conventional mixed models) can be applied to enhance our understanding of post-capture and release behavioral responses and distinguish pre- and post-recovery behaviors in free-ranging animals. We modeled shifts in multiple metrics (tailbeats, diving and track tortuosity) in response to capture-associated, intrinsic individual (e.g., size and energy use) and extrinsic (prey presence) factors to infer recovery periods, underlying behavioral processes and their drivers. We combined this information to derive new insights into the broader behavioral ecology of these predators in the wild.
Materials and Methods
Shark Capture and Tagging
White sharks were captured during July and August in 2018 and 2019 on SMART drumlines (Guyomard et al., 2019; Tate et al., 2021a) at Evans Head, New South Wales (NSW), Australia (29.11°S, 153.43°E, Figure 1). SMART drumlines were configured and set as described in Tate et al. (2019). This fishing gear provides real-time alerts when an animal is captured, enabling a rapid response to attend the individual within <30 min. The animal is then tagged and released approximately 1 km offshore as a strategy to reduce the immediate chances of human-shark interactions (McPhee et al., 2021).
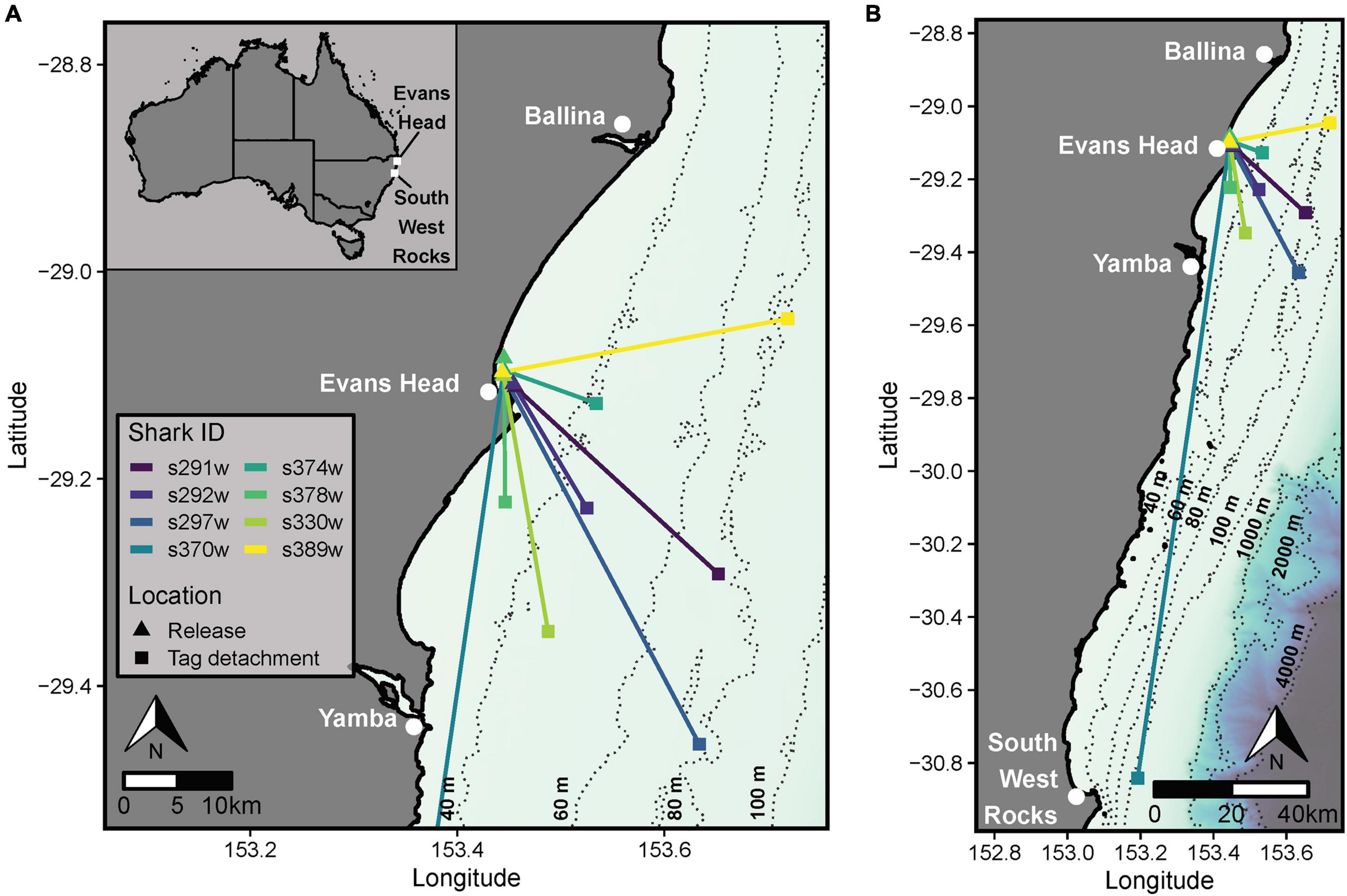
Figure 1. (A) Vectors indicating the net linear displacement bearing and distance moved by sharks between the location of release from SMART drumlines at Evans Head and the detachment of the tag. (B) Long distance movement of shark s370w which had an extended deployment duration (∼136 h) relative to other sharks. The coastline shapefile and bathymetric data were sourced from the GSHHG Database (Wessel and Smith, 1996; available from https://www.ngdc.noaa.gov/mgg/shorelines/) and the GEBCO 2020 15 arc-second bathymetric grid (GEBCO Compilation Group, 2020; available from https://www.gebco.net/data_and_products/gridded_bathymetry_data/gebco_2020/).
Biologging tags (hereafter CATS cams) were designed and manufactured by Customized Animal Tracking Solutions (CATS, Australia1). CATS cams contained a video camera (1080p, 30 frames s–1, up to ∼20 h video), depth sensor and a triaxial IMU including an accelerometer, gyroscope and magnetometer within a custom-built housing [275 (L) × 80 (W) × 45 (H) mm]. Video cameras were configured to record between sunrise and sunset (6 am to 6 pm) and data loggers recorded continuously at 20 Hz. During tagging, captured sharks were secured alongside a research vessel using PVC-covered tail and belly ropes, and inverted to induce tonic immobility. Sharks were sexed, measured to the nearest centimeter (PCL, precaudal length; FL, fork length; TL, total length) and CATS cams were attached to the middle of the underside of the pectoral fin, aligned with the sharks’ body axis, using a clamp and cradle system (Figure 2; Chapple et al., 2015). This position mimics the attachment region of remora fish (Echeneidae) and enabled a view of the mouth for assessing potential foraging behaviors and prey capture (Figure 2 and Supplementary Videos 1–3). CATS cams weighed 650 g [≤1.46% estimated total body mass of tagged sharks using length-weight relationship from Grainger et al. (2020)] and were designed to be slightly positively buoyant, allowing the tag to float to the surface once a galvanic release dissolved (∼17–30 h). For one shark (s370w), the tag failed to release from the clamp cradle until the second galvanic sleeve on the clamp frame corroded (Supplementary Figure 1), resulting in a longer deployment for this individual (∼136 h, Table 1). Floating tags were located and recovered by boat using inbuilt SPOT6 satellite (Wildlife Computers Inc2) and VHF radio tags with a Yagi antenna (Advanced Telemetry Systems3).
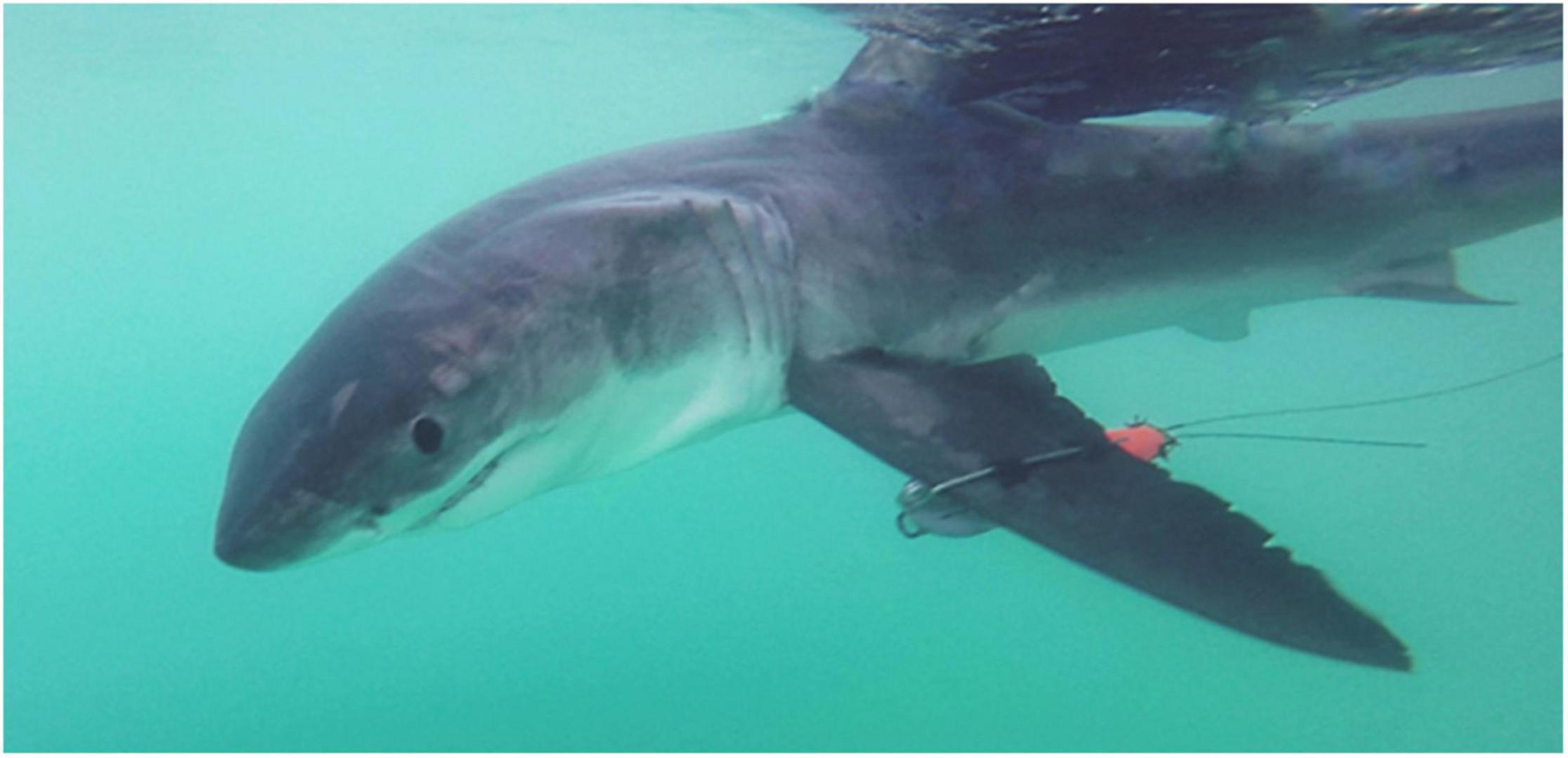
Figure 2. Deployment of a CATS cam tag on the pectoral fin of a juvenile white shark (2.15 m fork length), mimicking the attachment of a remora fish (Echeneidae).
Data Processing
All data processing and statistical analyses were performed in R v4.0.2 (R Core Team, 2020). To quantify general movements after release, net linear displacement (NLD) distance, movement rate and bearing between the initial (tagging) and final (tag detachment) locations for each shark were computed using the “TrackReconstruction” package (Battaile, 2019). Detachment locations were adjusted for consistent, linear drift in prevailing currents during the period between detachment from the shark and receipt of the first satellite location (range 0–7 h) using linear models of drift tracks (i.e., the predicted latitude/longitude at the detachment time).
Depth Data and Vertical Velocity
Zero offsets (mean depth over ∼15 s while at the surface during tagging) were applied to correct depth profiles for each shark (ensure surface depth = 0), and depth data were then smoothed using a 10 s rolling mean (Whitney et al., 2016). Vertical velocity (VV, m s–1), an instantaneous measure of diving rate, was then computed as the central difference of smoothed depths over a rolling 1 s interval (Whitney et al., 2016).
Derivation of Static and Dynamic Acceleration, Pitch, and Roll
Static (gravitational) acceleration (Astat), which is indicative of body posture (pitch/roll), was derived from a 3 s rolling average of raw (total) acceleration (Atotal) for each axis (Shepard et al., 2008). Astat was then subtracted from Atotal to determine dynamic acceleration (Adyn), a measure of the magnitude of dynamic body movement (Shepard et al., 2008). Overall dynamic body acceleration (ODBA, in g-force units) was then computed as a proxy for energy expenditure at each time point using the sum of absolute Adyn across all axes (Wilson et al., 2006). Astat was calibrated (“calibrate.axis” function; Farrell and Fuiman, 2013) using the minimum and maximum Astat values from a series of calibration rotations (each axis aligned with the gravity vector), and pitch and roll angles were then computed from Astat using the “animalTrack” package (Farrell and Fuiman, 2013).
Tailbeat Cycle
Tailbeat cycle length (TBC, in seconds) was determined through continuous wavelet transformation (CWT, “WaveletComp” package; Roesch and Schmidbauer, 2018) of lateral angular velocity (gyroscope yaw) data, which produced the clearest tailbeat signal. TBC was extracted using WaveletComp’s “ridge” function, which identifies peaks across the time-frequency wavelet power spectrum returned from the CWT (Figure 3). The “ridge” function was modified to identify ridges whenever power exceeded 0.2 because the default relative threshold (>10% of maximum power) failed to identify normal tailbeats when they were < 10% of the maximum power observed (e.g., when high amplitude movements also occurred in the time series). This fixed threshold correctly identified active swimming and gliding evident in the raw gyroscope data across individuals (Figure 3). The period of the ridge with the highest wavelet power (dominant oscillation signal) was used as the TBC, with gliding (no tailbeat) inferred when no ridge was identified.
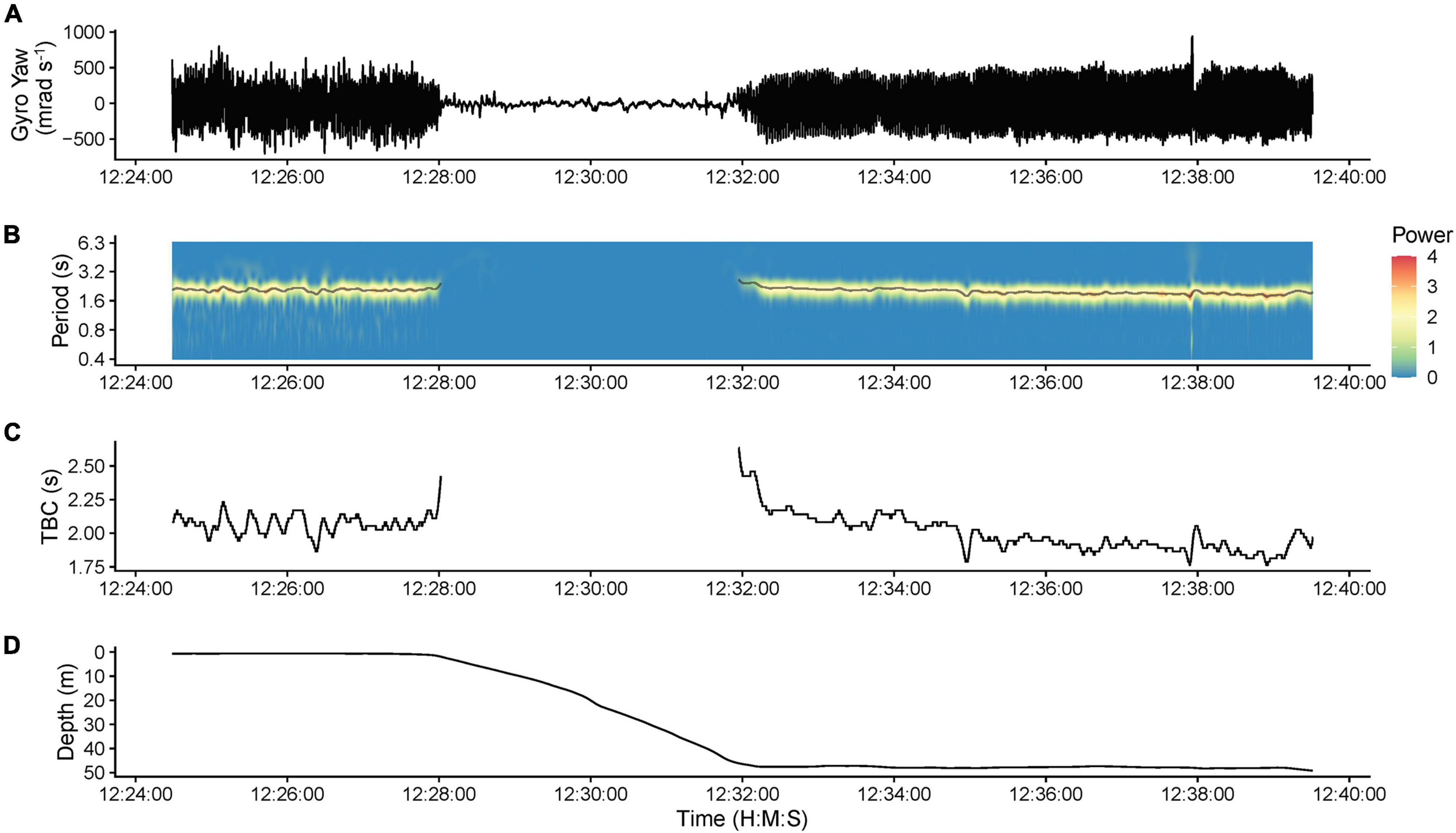
Figure 3. Example data from shark s292w (193 cm fork length, female) illustrating the determination of tailbeat cycle length and gliding behavior (no tailbeats) using continuous wavelet transformation of gyroscope data. (A) Raw gyroscope yaw (lateral angular velocity) data. (B) The corresponding time-frequency wavelet power spectrum, determined through continuous wavelet transformation, showing the different oscillation periods present in the gyroscope yaw data. The gray line overlayed on the spectrum is the output of the ridge determination procedure and indicates the period of the ridge with the highest power value (dominant oscillation signal) at each time point. (C) The tailbeat cycle (TBC) extracted from the ridge determination procedure. (D) The depth profile of the shark. Gliding behavior (no tailbeat) was determined when no ridge was identified. Gliding is evident with a lack of periodicity in the gyroscope data during a descent from the surface to ∼50 m depth, after which tailbeats resumed.
Heading and Dead Reckoned Pseudo-Track Computation
To visualize the fine-scale movements of sharks, pseudo-tracks (fine-scale approximations of horizontal movements; Andrzejaczek et al., 2018) were computed from magnetometer-derived headings using the “animalTrack” package (Farrell and Fuiman, 2013). Raw magnetometer data were calibrated for magnetic distortions by subtracting a hard iron offset (to center data on the origin), and multiplying by a soft iron rotation matrix (to correct elliptical distortion), with these correction factors determined using MIP Iron Calibration software (Parker-LORD MicroStrain® Sensing4) from a series of 360° calibration rotations (also see Bidder et al., 2015). Pitch- and roll-corrected headings (computed with the “tilt_compensate” function) were smoothed with a 3 s running average to filter out tailbeat yaw and then used to compute pseudo-tracks with the “dead_reckoning” function using a fixed horizontal speed of 0.82 m s–1 (Andrzejaczek et al., 2018; Colefax et al., 2020; tags did not include a speed sensor) adjusted for depth changes over successive steps.
Video Analysis
Behavioral events were recorded from video footage using BORIS software (Friard and Gamba, 2016). These included habitat use/position in the water column (Supplementary Figure 2 for classifications), the occurrence of fish and other organisms/objects, and interactions with these (see Supplementary Videos 2, 4 for highlights involving notable interactions). Only observations between 7 am and 4 pm were retained in subsequent analyses to avoid ambiguities in video analysis during twilight hours.
Statistical Analysis
Prior to statistical analyses, data were thinned to a 1 s interval, matching the temporal resolution at which relevant response variables (e.g., TBC and VV) were calculated.
Tailbeat Cycle Recovery Period
Shifts in TBC after release were used as a proxy to quantify behavioral recovery periods following Whitney et al. (2016) and Andrzejaczek et al. (2019a). For each shark, mean TBC was calculated over 15 min windows, and then related to time since release (TSR) as the predictor. An exploratory generalized additive mixed model (GAMM, “mgcv” package; Wood, 2011) suggested an asymptotic relationship between these variables (p < 0.001). Thus the effect of TSR on mean TBC was quantified using a non-linear self-starting asymptotic mixed model (“nlme” package; Pinheiro et al., 2020) with an individual random effect on the asymptote (Asym), initial (R0) and natural log of rate constant (lrc) coefficients to allow for individual variability in recovery patterns (Whitney et al., 2016), and a first-order autoregressive [AR(1)] correlation structure to accommodate within-individual temporal autocorrelation. Model fit was assessed via diagnostic plots (fitted vs. normalized residuals plots, Q-Q plots and normalized residual autocorrelation plots). The TBC recovery period was then calculated at the population and individual level (using random effect coefficients) as the time taken for the TBC to increase 80% of the difference between its initial (R0) and predicted asymptotic (Asym) value (Whitney et al., 2016).
To explore factors influencing TBC-derived recovery periods, several candidate generalized linear models (GLM) were then fit (“stats” package; R Core Team, 2020) with individual sharks’ recovery periods as the response variable, a gamma error (recovery periods were non-negative, continuous, and positively skewed) and inverse link, and varying combinations of sex, FL and capture duration (total time from hooking until release of the shark) as predictors. Models were compared to each other and a null model (intercept only) using small sample corrected Akaike information criterion (AICc, “MuMIn” package; Barton, 2020) to determine the favored model (lowest AICc).
Shifts in Horizontal and Vertical Movement: Hidden Markov Model
Hidden Markov models were used to further explore post-release behavioral shifts and processes based on vertical (diving) and horizontal (swimming tortuosity) movement patterns. HMMs are time series models explicitly suited to high resolution, serially dependent, biologging data (Leos-Barajas et al., 2017). Briefly, they allow partitioning of multidimensional time series comprising m data streams (measured variables) into n distinct states, each of which is described by its own state-dependent probability distribution, from which inferences on likely behaviors associated with each state can be made (Zucchini et al., 2016; McClintock et al., 2020). Subsequently, HMMs can be applied for behavioral classification (via global decoding) based on complex, multifaceted time series, and to explore effects of covariates on otherwise cryptic behavioral dynamics (probabilities of occupying or switching between states; McClintock and Michelot, 2018). Thus, we applied HMMs as an intuitive and effective method for more holistically examining shifts in fine-scale movements and behavioral processes after release, and how these relate to biological and capture-associated covariates.
Hidden Markov models were fit with the “momentuHMM” package (McClintock and Michelot, 2018) using two data streams; (1) absolute of mean VV (VVAM; m s–1), which distinguished diving (VVAM ≫ 0) from level (VVAM ≈ 0) swimming, and (2) mean resultant length () of headings (“circular” package; Agostinelli and Lund, 2017), which separated linear (as ) and more tortuous (as ) behaviors. VVAM and were computed over non-overlapping 1 min windows. This temporal resolution was chosen to maximize the range of observed , helping to distinguish tortuosity states, whilst being sufficiently short enough to capture fine-scale, rapid behavioral shifts evident in pseudo-tracks. Gamma and beta probability distributions were assumed for VVAM (continuous, non-negative, positively skewed), and [continuous on interval (0,1)], respectively (McClintock and Michelot, 2018).
A combination of model selection criteria (AIC), pseudo-residual plots and visual validation from decoded behavioral states overlaid onto pseudo-tracks (i.e., biological interpretability; Conners et al., 2021) were used to determine the best-fit model (number of states, inclusion of covariates) from a suite of candidate models. Several baseline models (no covariates) were first fit to determine the number of behavioral states included (up to a maximum of 5). Models with 4 or 5 states were then extended to incorporate covariates on state transition probabilities, including all combinations of sex, FL, time of day (TOD, 24 h cyclicity accommodated using the “cosinor” special function), and TSR. A TOD*TSR interaction was also included because a diurnal diving pattern became apparent in some individuals after release (see section “Results”). Capture duration and time on the line (time from capture alert to securement at the research vessel) both varied minimally among most individuals and all but two sharks had capture durations within 8 min of each other (49–57 min, Table 1). Given the small sample size (n = 8), it was therefore unlikely reliable inference could be drawn based mostly on the two sharks with shorter (s370w, 27 min) and longer (s291w, 72 min) capture durations. Additionally, proportional state use by these two individuals fell mostly within observed values of other sharks (Supplementary Figure 5). Given these considerations, capture duration (and time on the line) were not included as covariates.
Use of discrete random effects has been suggested to account for generic individual heterogeneity in transition probabilities where longitudinal time series from multiple individuals (such as in our dataset) are modeled with HMMs (Towner et al., 2016). However, generic discrete random effects groupings are sensitive to small sample sizes and difficult to interpret in such circumstances (e.g., can produce false convergence or erroneous inferences on covariates), and do not appreciably affect state assignment (McClintock, 2021). Given our relatively small sample (n = 8 time series), we did not include discrete random effects in addition to existing informative individual-specific covariates (e.g., FL; McClintock, 2021). Nonetheless, we note that HMM results were corroborated by complementary GAMM analyses that included an individual random effect (see section “Results” below), providing additional support to our findings.
Fitted HMMs were re-optimized through 50 random perturbations to initial parameter values to evaluate for the global maximum of the likelihood (McClintock and Michelot, 2018). Global decoding (Viterbi algorithm) based on the best-fit model was used to classify behavioral states corresponding to each 1 min time window, and these were plotted over pseudo-tracks to visually validate inferred behaviors. Marginal stationary state probabilities were also computed for each covariate individually (“plotStationary” function, holding other covariates at their mean) to visualize their effects on state use (McClintock and Michelot, 2018). Given only one individual exceeded a deployment duration of 46.3 h, HMMs were also re-fit using datasets clipped to ≤46.3 h post-release (ensuring observations from ≥2 individuals at all modeled time points) to evaluate any impacts on model outputs (e.g., Carter et al., 2020). Model outputs were largely consistent between clipped and full analyses for the period ≤ 46.3 h post-release (Supplementary Figure 3A and section “Results”). We therefore retained the full analysis with the caveat that modeled behaviors beyond 46.3 h represented those of only a single individual.
Shifts in Horizontal and Vertical Movement: Generalized Additive Mixed Models
Generalized additive mixed models were used complementarily to HMMs to further investigate the relationship between TOD, TSR and their interaction on path tortuosity, and to examine whether diving and path tortuosity were related. A diving ratio (DR, proportion of time assigned as diving states from global decoding; modified from Andrzejaczek et al., 2019a) and of headings were used to quantify diving and path tortuosity, respectively, over 15 min windows. The effects of smooths of TOD (cyclic cubic regression spline), TSR (thin-plate regression spline) and their tensor interaction on was assessed using a GAMM (beta error, logit link; “mgcv” package; Wood, 2011) with an individual random effect and within-individual AR(1) temporal correlation structure. The GAMM was also re-fit using the clipped dataset (≤46.3 h post-release), as for HMMs above. Modeled patterns from clipped and full analyses were again mostly consistent (Supplementary Figure 3B and section “Results”), so the full analysis was retained. The relationship between DR (predictor) and (response) was assessed using a beta GAMM with individual random effects and correlation structures as above. Model fits were assessed using diagnostic plots (as for TBC analysis above) and smoother significance was set at p < 0.001 given that GAMM p values are approximate (Zuur et al., 2014).
Exploring Drivers of Movement Shifts: Energy Use Proxies (Tailbeat Cycle and Overall Dynamic Body Acceleration)
To assess, relationships between diving (DR), path tortuosity () and energy use, two energetic proxies were computed over 15 min windows, as above; (1) mean TBC and (2) mean ODBA (which incorporates other dynamic body movements not detected by TBC analysis alone; Leos-Barajas et al., 2017). Candidate linear mixed models (LMMs, “nlme” package; Pinheiro et al., 2020) were then fit with either mean TBC or mean ODBA (natural log transformed for normalization) as the response, , DR or DR + as predictors, an individual random intercept, or random intercept + slope (to accommodate any between-individual variation in relationships; Schielzeth and Forstmeier, 2009) and a within-individual AR(1) correlation structure. AICc was used to compare models to each other and a null (intercept only) to determine the favored model (fixed and random effects specifications). Predictor collinearity was assessed using variance inflation factors (VIF; “performance” package; Lüdecke et al., 2020) where necessary, which was negligible (VIF = 1.3). Model diagnostic plots were checked as above.
Exploring Drivers of Movement Shifts: Fish Presence
To investigate the relationship between diving (DR), tortuosity () and foraging opportunity, the proportion of time fish were present in video (pfish, all species pooled due to rarity of individual species, which included known or potential prey based on stomach contents; Grainger et al., 2020; see Supplementary Video 2) was quantified as a proxy for prey availability over 15 min windows. Several other non-prey/miscellaneous objects were also observed (e.g., jellyfish, starfish, and detached kelp), but excluded from analysis due to their rarity (absent in >90% of summary windows for most individuals).
Relationships between , DR and pfish were investigated by fitting several candidate zero-inflated beta mixed models (ZIBMM, accommodating zeros where fish were absent; Douma and Weedon, 2019) using the “glmmTMB” package, with , DR or DR + as predictors, an individual random intercept or intercept + slope (as for LMMs above), and an Ornstein-Uhlenbeck (OU) temporal correlation structure (“ou” function) for irregularly spaced time series (night-time hours between 4 pm and 7 am excluded from analysis; Brooks et al., 2017). Fixed, random effects and correlation structures were specified for both the conditional and zero-inflated components of each model. The favored model was determined using AICc comparisons as above. Convergence failed in several models unless the OU correlation was removed from the conditional and/or zero-inflated component, however this produced correlated residuals and did not improve the AICc. Thus, model selection only considered converged models including the OU structure.
Results
Overall Movements and Displacement After Release
CATS cams were deployed on eight white sharks (4 males and 4 females, 168–258 cm FL) for 9.7–136.2 h, producing 258.8 h of logger data (mean ± SD = 32.4 ± 25.1 h, range = 9.7–87.4 h) and 81.6 h of video (mean ± SD = 10.2 ± 4.2 h, range = 5.0–15.3 h, between 7 am and 4 pm daily; Table 1). All sharks moved offshore after release (∼10–30 km from coastline) with a mean ± SD NLD distance from the tagging location of 45.2 ± 61.5 km and NLD rate of 1.1 ± 0.4 km h–1 (Table 1 and Figure 1). Most sharks moved south-east of the tagging location (mean ± SD NLD bearing = 146.6 ± 36.7°, Table 1 and Figure 1). This immediate post-release movement was corroborated by video and the oscillatory diving of sharks between the surface and seabed, with the maximum depth of consecutive dives (approximating seabed depth) increasing until the ∼40–60 m depth contour (mean ± SD time to reach 40 m contour = 5.6 ± 3.4 h, range 2.3–11.3 h, Figure 4). One shark with a longer deployment duration (s370w) travelled 195.2 km southward in 5.7 days, with a similar NLD rate to other individuals (Table 1 and Figure 1B). Tailbeat analyses indicated instances of gliding (no tailbeat), mostly during diving descents (Figures 3, 4 and Supplementary Video 1). Sharks reached a mean ± SD maximum depth of 69.6 ± 25.2 m (range 41.5–118.4 m), and generally remained in waters > 40 m depth after their initial offshore movement (Figure 4). Following an initial diving phase, some individuals tended to swim level during the day and dive more at night (Figure 4). However, shark s378w exhibited a different pattern, remaining closer to shore (despite a similar deployment length to other individuals, Figure 1 and Table 1) and diving continuously (Figure 4). Video analysis showed that sharks swam mostly at the seabed (mean ± SD, 39.8 ± 25.2% of time) or surface (37.1 ± 24.8%) and spent less time in the middle of the water column (23.1 ± 15.6%). Sand was the most common substratum (38.9 ± 26.0% of total analyzed video), with low use of other habitats (Supplementary Figures 2, 4).
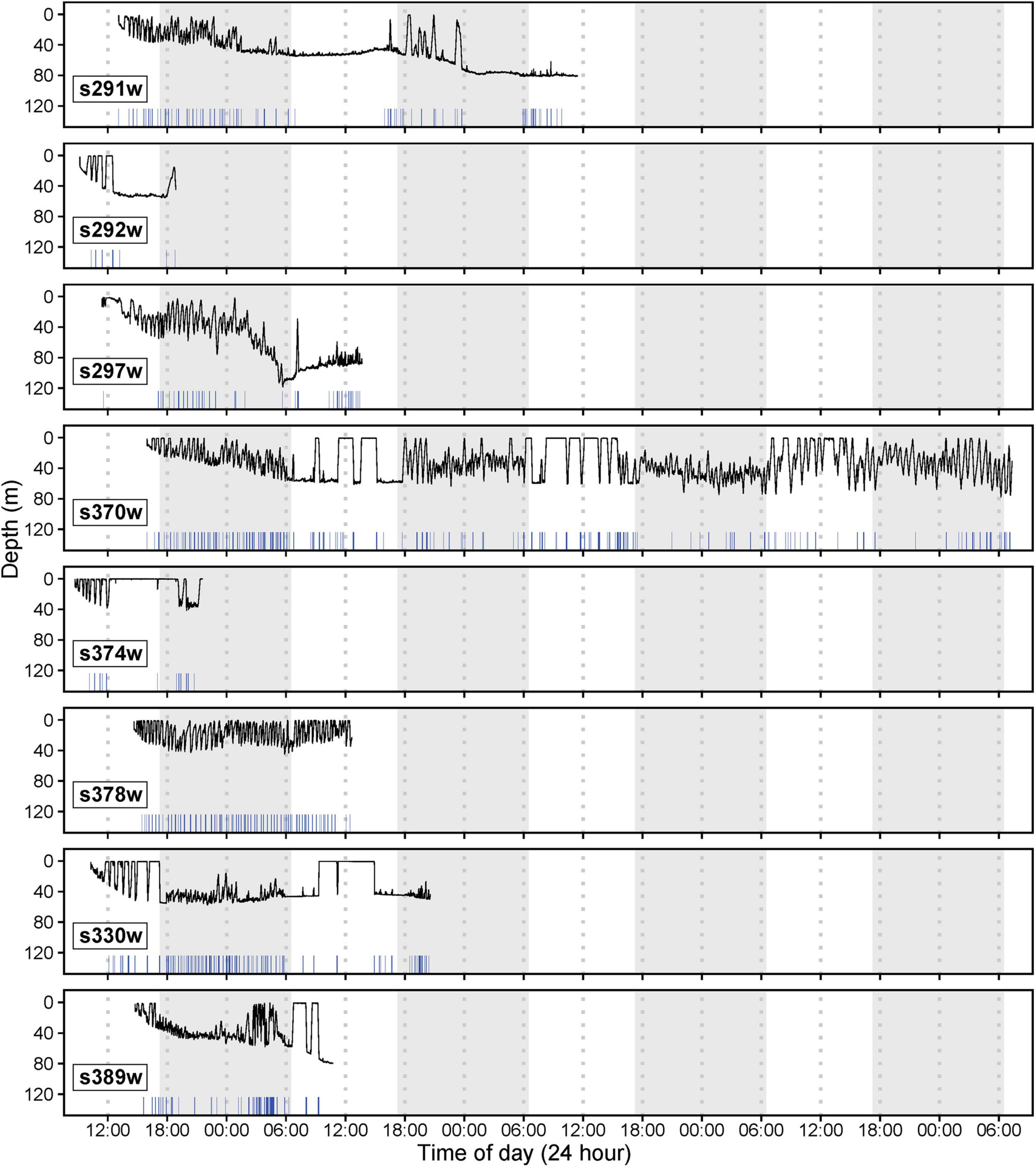
Figure 4. Diving profiles for eight deployments of CATS cams on white sharks released from SMART drumlines (shark ID numbers indicated in bottom left of panels). White and gray shading indicates day and night (sunset to sunrise), respectively. X-axis rug plots indicate instances of gliding behavior (no tailbeat) determined through tailbeat analysis.
Post-release Recovery Period and Behavioral Shifts
Tailbeat Cycle Recovery Period
Tailbeats were significantly faster (lower mean TBC) after release and gradually subsided to a more constant, slower rate (higher mean TBC, predicted increase = 0.6 s), indicating an average recovery time of 9.7 h (range 3.3–30.4 h, Table 2 and Figure 5A). Variation in individuals’ recovery periods was best explained by their length (Supplementary Table 1), with larger sharks exhibiting significantly shorter recoveries (estFL ± SE = 0.0014 ± 0.0005, t = 2.67, p = 0.037, null deviance explained = 50.8%, Figure 5B).
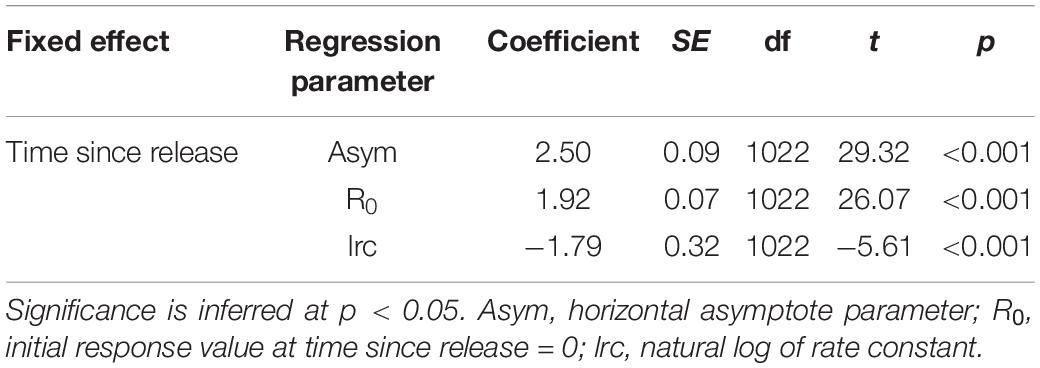
Table 2. Coefficients and significance for parameters from an asymptotic mixed effects model predicting mean tailbeat cycle (TBC) by time since release.
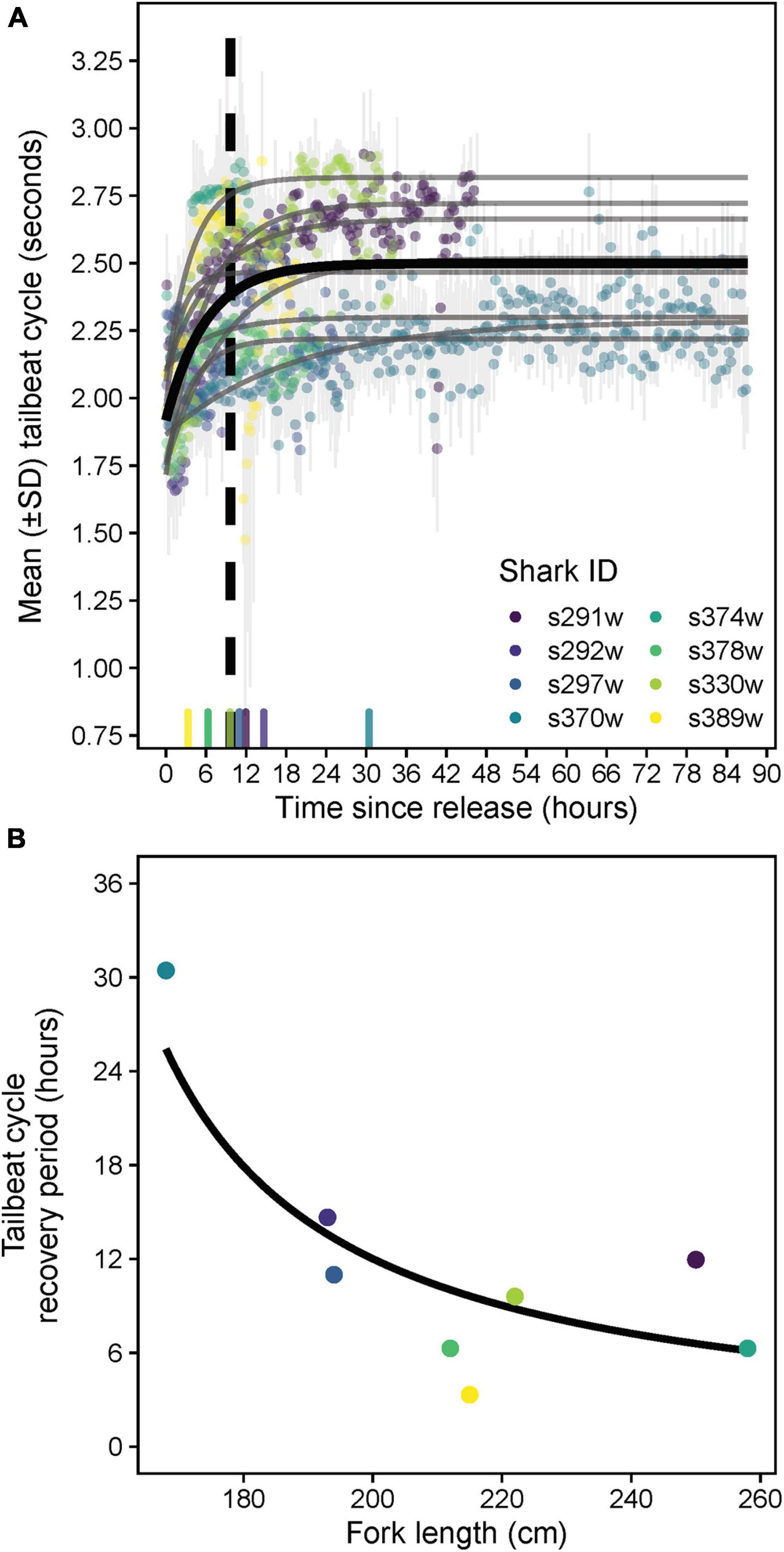
Figure 5. (A) Predicted population- (black) and individual-level (gray) asymptotic relationships between mean tailbeat cycle length and time since release for white sharks released from SMART drumlines. The X-axis rug plot shows recovery periods (time at 80% of the difference between initial and asymptotic tailbeat cycle values) for individual sharks, with the dashed vertical line indicating the population-level recovery period. Note recovery times for sharks s374w and s378w were similar and thus overlap on the rug plot. (B) Predicted relationship between fork length and the tailbeat cycle-derived recovery periods of sharks from a gamma generalized linear model.
Shifts in Horizontal and Vertical Movement: Hidden Markov Model
A five-state model was favored by AIC (Supplementary Table 2). This included two diving states (state 1 = “diving slow/linear,” state 2 = “diving rapid/tortuous,” mean VVAM ≥ 0.046 m s–1), and three level swimming states (mean VVAM ≤ 0.012 m s–1) with sequentially increasing tortuosity (smaller , state 3 = “level linear,” state 4 = “level meandering,” and state 5 = “level highly tortuous/circular”; Figures 6A,B and Supplementary Table 3). Inspection of pseudo-tracks supported a five-state model which better separated linear, meandering and highly tortuous behaviors (i.e., states 3–5) compared to modeling with fewer states, and allowed visual validation of classifications and shifts between states (Figures 6C–F). For instance, representative pseudo-track and dive profile data overlayed with decoded states indicated transitions from level, highly linear swimming (state 3) at the surface, followed a rapid descent to the sea floor (state 2), a short, mostly linear (states 3, 4) bottom phase (∼2 min), then a relatively slow ascent (state 1) and resumption of linear, level swimming (state 3, Figures 6C,D). Furthermore, visualization with pseudo-tracks allowed horizontal movement modes to be readily distinguished, including shifts from meandering to circling tortuous behavior (state 4 and 5, respectively, Figure 6E), neither of which were discernable from depth data alone (Figure 6F).
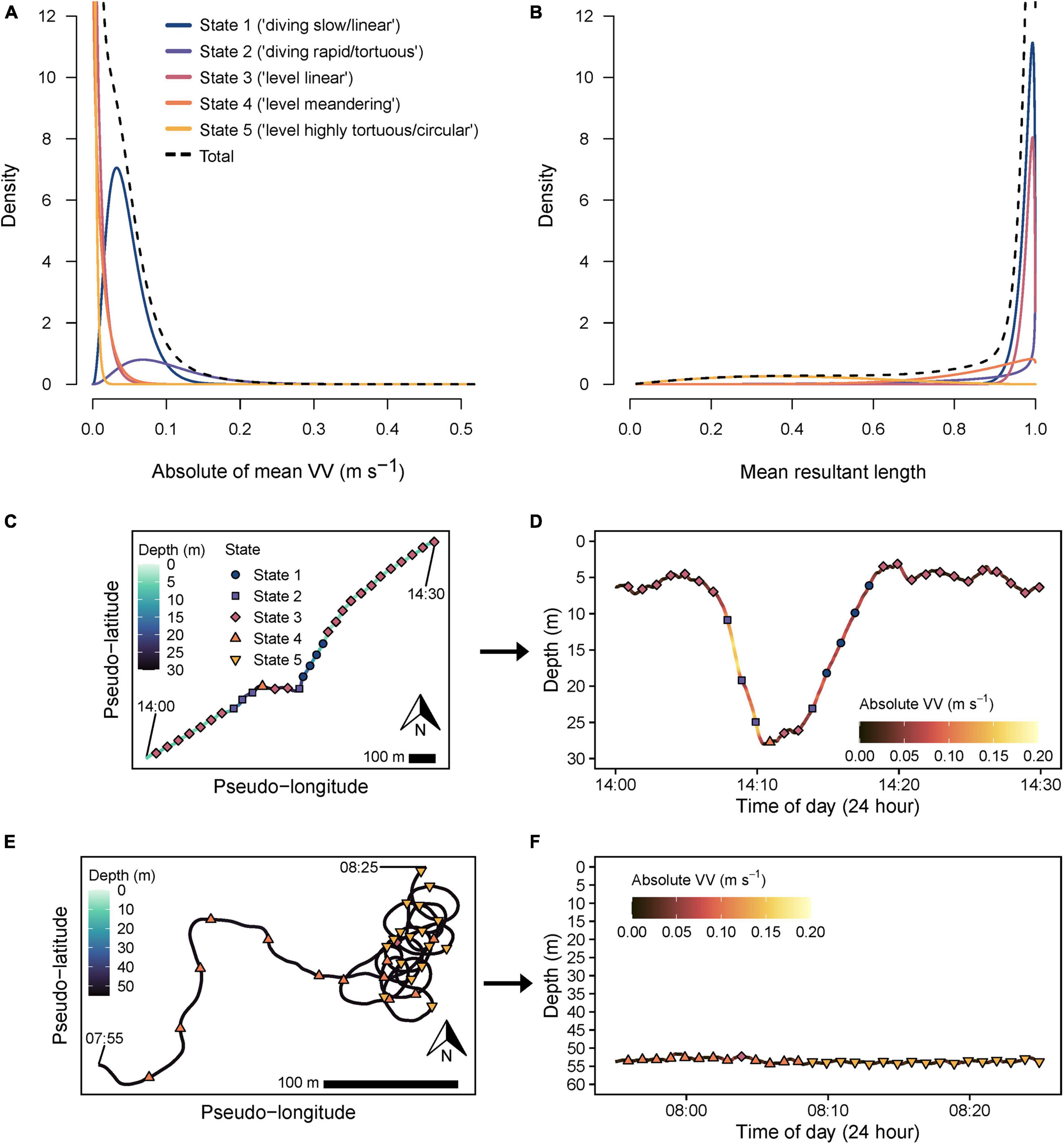
Figure 6. State-dependent probability distributions for (A) absolute of mean vertical velocity (VV) and (B) mean resultant length data streams from the final selected five-state hidden Markov model. Total (black dashed line) indicates the overall pooled distribution for each data stream. Interpreted definitions corresponding to modeled states are indicated in the key in panel (A). Representative 30 min pseudo-tracks and their corresponding depth profiles (indicated by arrows) showing track sections assigned through global decoding (Viterbi algorithm) as either (C,D) state 1 (navy circles), 2 (purple squares) or 3 (crimson diamonds), or (E,F) state 4 (orange triangle) and 5 (gold inverted triangle). Start and finish times (24 h time) are labeled to indicate track directionality. X- and Y-axes are scaled equally in each pseudo-track plot. A 100 m scale bar is provided for comparison of the approximate relative spatial scale of movements across each plot, assuming a fixed swimming speed (0.82 m s–1) for all sharks.
The final HMM also included covariates FL, TSR, TOD, and a TSR*TOD interaction on state transition probabilities (Supplementary Table 2). Although sex was also suggested as an explanatory factor (Supplementary Table 2), this was excluded due to the combination of small within-sex sample sizes (n = 4 per sex), and the differences being primarily driven by only two females (high probabilities of state 5 behavior), with remaining individuals being more similar (Supplementary Figure 5). Longer sharks showed increased use (stationary state probability) of level linear (state 3) and reduced level tortuous/circular (state 5) behavior (Figure 7A). Level tortuous/circular behavior also peaked during the day (∼12 pm), whilst linear diving (state 1) predominated at night (Figure 7B). Level tortuous/circular swimming (state 5) also increased and peaked at ∼24 h post-release, which was preceded by a reduction in level linear swimming (state 3) and followed by an increase in other states (Figure 7C). Results from HMMs based on the full (Figure 7) and clipped datasets (Supplementary Figure 3A) were generally consistent for the period up to 46.3 h post-release, supporting the observed patterns, although we note that model outputs beyond this time are representative of the responses of the single shark with a longer time-series.
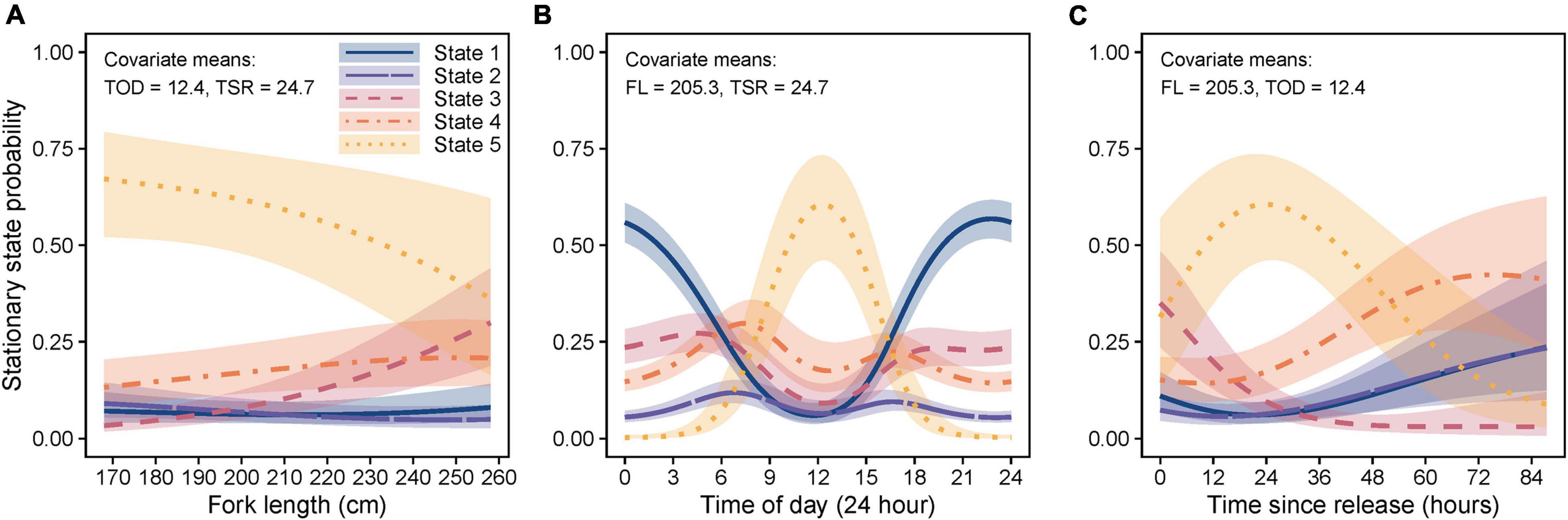
Figure 7. Marginal stationary state probabilities (shading = 95% confidence intervals) for covariates (A) fork length (FL), (B) time of day (TOD), and (C) time since release (TSR), included in the best-fit five-state hidden Markov model. Marginal stationary state probabilities were computed across the range of observed values for each covariate individually while holding all other covariates at their mean values (indicated at the top of each plot). State 1 (navy/solid line) = diving slow/linear, state 2 (purple/long dash line) = diving rapid/tortuous, state 3 (crimson/dashed line) = level linear, state 4 (orange/dot-dash line) = level meandering, and state 5 (gold/dotted line) = level highly tortuous/circular.
Shifts in Horizontal and Vertical Movement: Generalized Additive Mixed Models
A TSR*TOD tensor interaction significantly influenced path tortuosity (, Table 3), with linear swimming (≈ 0.7–0.8) following release (Figure 8A), consistent with an offshore transit (Figures 8B,C), preceding a transition (∼12–24 h post-release) to daytime tortuous behavior (≈ 0.3–0.4, Figure 8A). GAMM modeling based on the clipped dataset supported this pattern (Supplementary Figure 3B). A subsequent reduction in tortuosity beyond ∼45 h post-release was exhibited by the shark with the longer deployment (Figure 8A). These results corroborated findings from HMMs. Pseudo-tracks indicated highly tortuous periods were characterized by extended durations (lasting from 20 min to 4.7 h continuously) where sharks swam in circles, alternating between clockwise and counterclockwise rotations (individual circles completed in ∼1–2 min, Figures 8D,E and Supplementary Figure 6). Nonetheless, we note that because conventional GAMM analysis predicts a smooth continuum of responses (Figure 8A), this was less objective for identifying patterns in circling behavior specifically, compared to HMMs that explicitly separated dynamics in circling (state 5) from those of other movement states (e.g., meandering, state 4; Figure 7). was positively related to DR (estimated df = 4.24, F = 86.19, p < 0.001, Figure 9A), with tortuous swimming/circling occurring during level swimming at the seabed or surface, and linear swimming occurring more with diving (Figures 9B,C). We also noted instances where sharks remained approximately stationary whilst circling northward into a southward-flowing current in offshore waters (Supplementary Video 3).
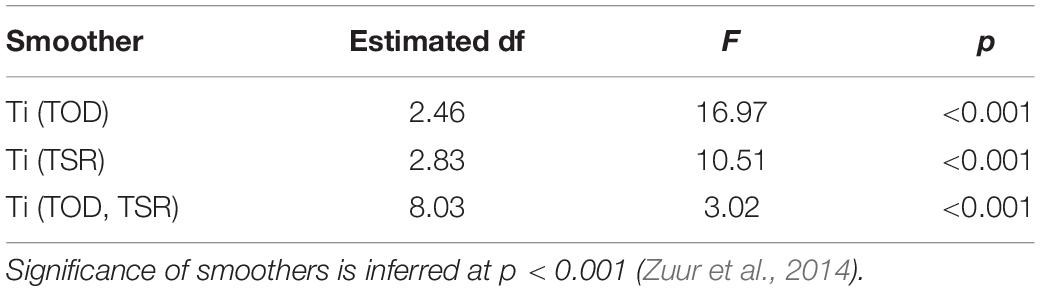
Table 3. Coefficients and significance of smooth terms for time of day (TOD), time since release (TSR), and their tensor interaction from a beta generalized additive mixed model predicting mean resultant length.
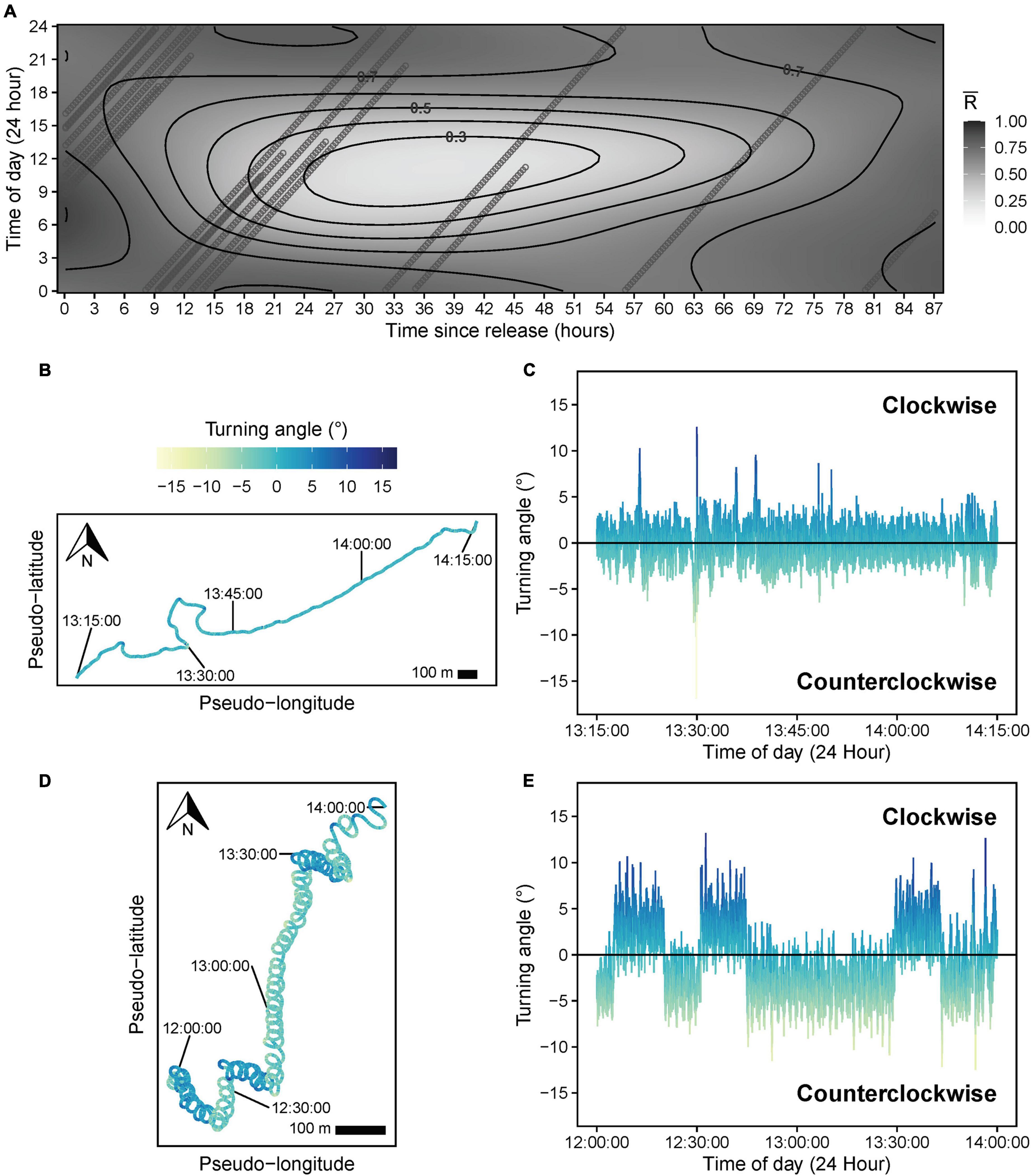
Figure 8. (A) Response surface showing predicted values for mean resultant length () based on a significant tensor smooth interaction of time of day (TOD) and time since release (TSR) from a beta generalized additive mixed model. Heading variance (path tortuosity) decreases as increases from 0 (tortuous swimming) to 1 (linear swimming). Points show the distribution of predictor values (TOD and TSR) for all individual sharks across the response surface. (B) One hour pseudo-track and (C) corresponding turning angle data (computed at 1 s intervals), illustrating linear swimming during the middle of the day shortly (∼1 h) after release. (D) Two hour pseudo-track and (E) corresponding turning angle data illustrating highly tortuous, clockwise, and counterclockwise circular swimming behavior during the middle of the day, ∼24 h after release. The turning angle color legend is consistent across all turning angle and pseudo-track plots. X- and Y-axes are scaled equally for pseudo-tracks. A 100 m scale bar is provided to indicate the approximate relative scale of movement, assuming a fixed swimming speed (0.82 m s–1) for each shark.
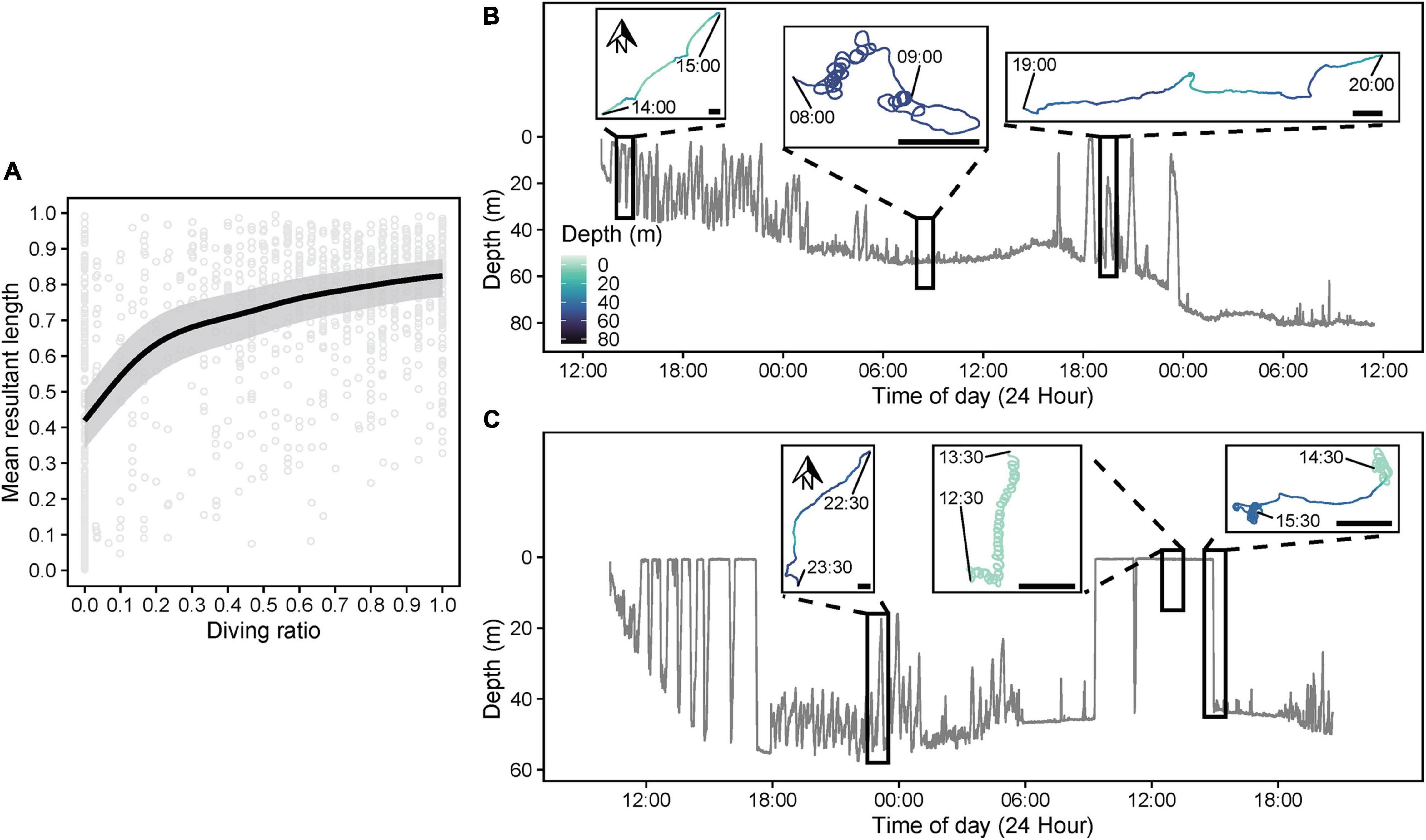
Figure 9. (A) Predicted relationship (gray shading = 95% confidence intervals) between diving ratio (DR, proportion of time spent diving/in states 1 or 2) and mean resultant length (, 1 = low heading variance, 0 = high heading variance) from a beta generalized additive mixed model. DR and were computed over 15 min windows for each shark. Dive profiles and corresponding pseudo-track sections over relevant windowed periods (black rectangles) for sharks (B) s291w and (C) s330w indicating representative examples of linear swimming during intensive diving periods and tortuous activity, including circling behavior, during level phases at the seabed and the surface. The depth color scale is consistent among all plots. For pseudo-tracks, x- (pseudo-longitude) and Y-axes (pseudo-latitude) are scaled equally, and north is toward the top of each plot. A 200 m scale bar is also indicated on each pseudo-track to indicate the approximate relative spatial scale of movement, assuming a fixed swimming speed (0.82 m s–1) for all sharks.
Exploring Drivers of Movement Shifts: Energy Use Proxies (Tailbeat Cycle and Overall Dynamic Body Acceleration)
Mean TBC increased (slower tailbeat) subtly albeit significantly with increased tortuosity (lower , predicted mean difference = 0.2 s), but there was no significant relationship with DR (Supplementary Table 4, Table 4, and Figures 10A,B). Only DR was favored as a predictor of log mean ODBA (Supplementary Table 4), indicating little effect of (Figure 10C). Log mean ODBA decreased significantly as diving increased (Table 4 and Figure 10D).
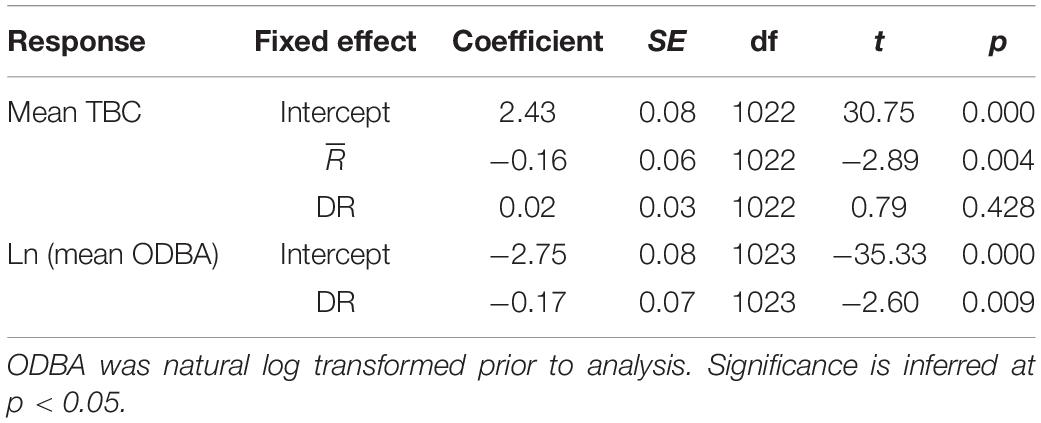
Table 4. Coefficients and significance for parameters from linear mixed models evaluating relationships between mean resultant length (), diving ratio (DR), mean tailbeat cycle (TBC), and mean overall dynamic body acceleration (ODBA).
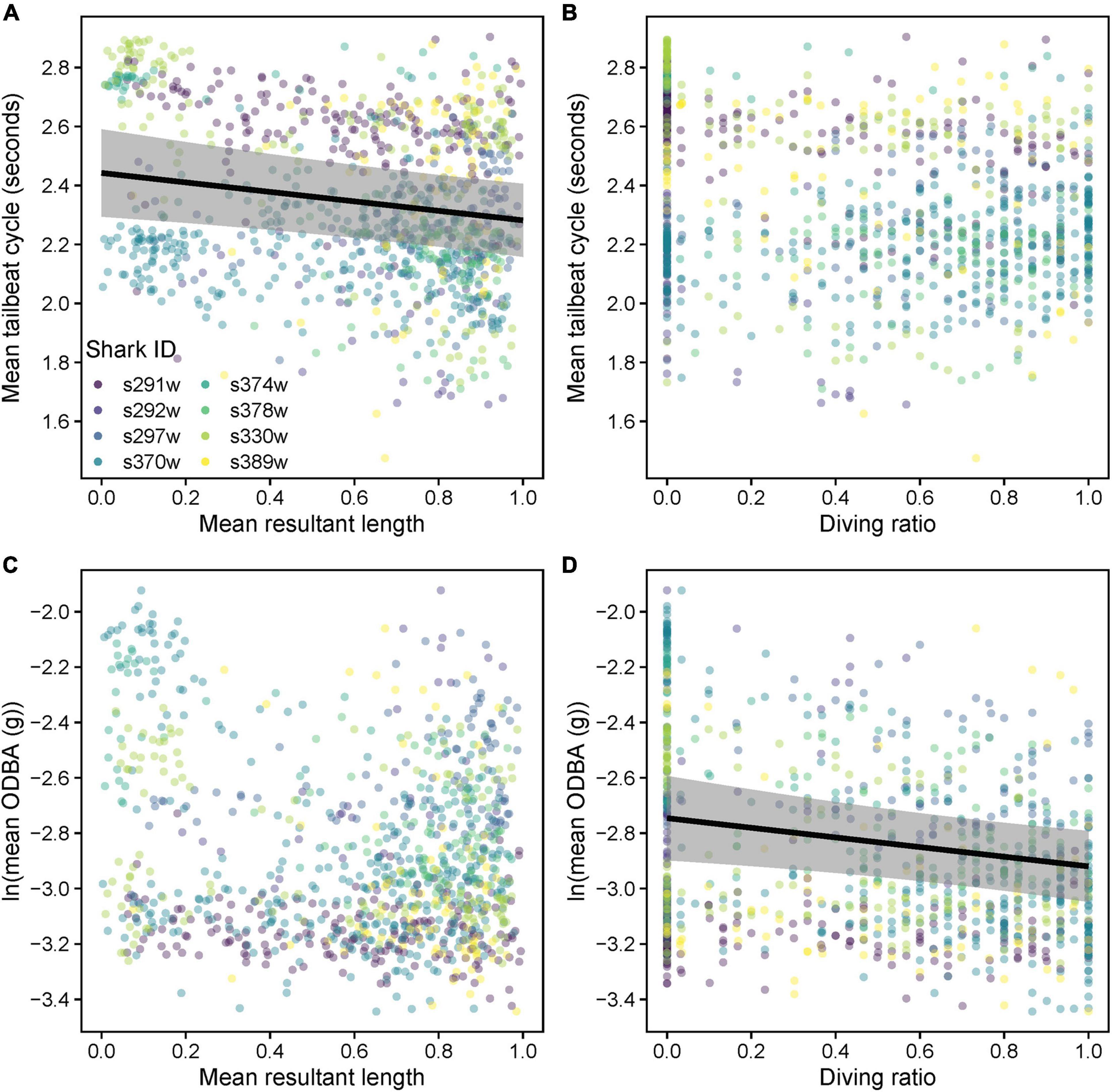
Figure 10. Relationships between mean tailbeat cycle length (A) mean resultant length () and (B) diving ratio (DR), and between mean overall dynamic body acceleration (ODBA, natural log transformed) (C) and (D) DR. Variables were computed over 15 min windows for each shark. Predicted relationships (gray shading = 95% confidence intervals) from a linear mixed model are shown where there was a significant effect (p < 0.05).
Exploring Drivers of Movement Shifts: Fish Presence
Overall, encounters with other fish were rare (mean ± SD, 1.2 ± 1.5% of analyzed video footage, range = 0.1–4.7%). When other fish were present in video footage, the most commonly observed species (by time percentage) were silver trevally (Pseudocaranx sp., 62.9%), unidentified teleosts (19.0%) and scad (Trachurus sp., 16.3%), with mado sweep (Atypichthys strigatus), leatherjackets (Monacanthidae), snapper (Chrysophrys auratus), carcharhind sharks (Carcharhinidae), scombrids (Scombridae), and flatheads (Platycephalidae) observed rarely (<1.0%; Supplementary Video 2). Fish were mostly encountered on the seabed (mean ± SD, 71.6 ± 31.0% by time) or in the water column (25.4 ± 26.0%), but rarely at the surface (3.0 ± 8.4%). Although there were no confirmed feeding events, two active prey pursuits were observed on a leatherjacket and small carcharhinid shark (Supplementary Video 2). Sharks also investigated several other “non-prey” objects including detached kelp, jellyfish, and seabirds (Supplementary Video 2).
There was no strong relationship between tortuosity () and pfish, with only DR favored as a predictor (Supplementary Table 5 and Figure 11A). Indeed, highly tortuous swimming/circling was mostly initiated and persisted despite the absence of other fish/immediate foraging opportunity (Supplementary Figure 6). Pfish increased marginally, yet significantly during level swimming (low DR, conditional model estDR ± SE = −1.17 ± 0.59, z = 1.98, p = 0.047, Figure 11B).
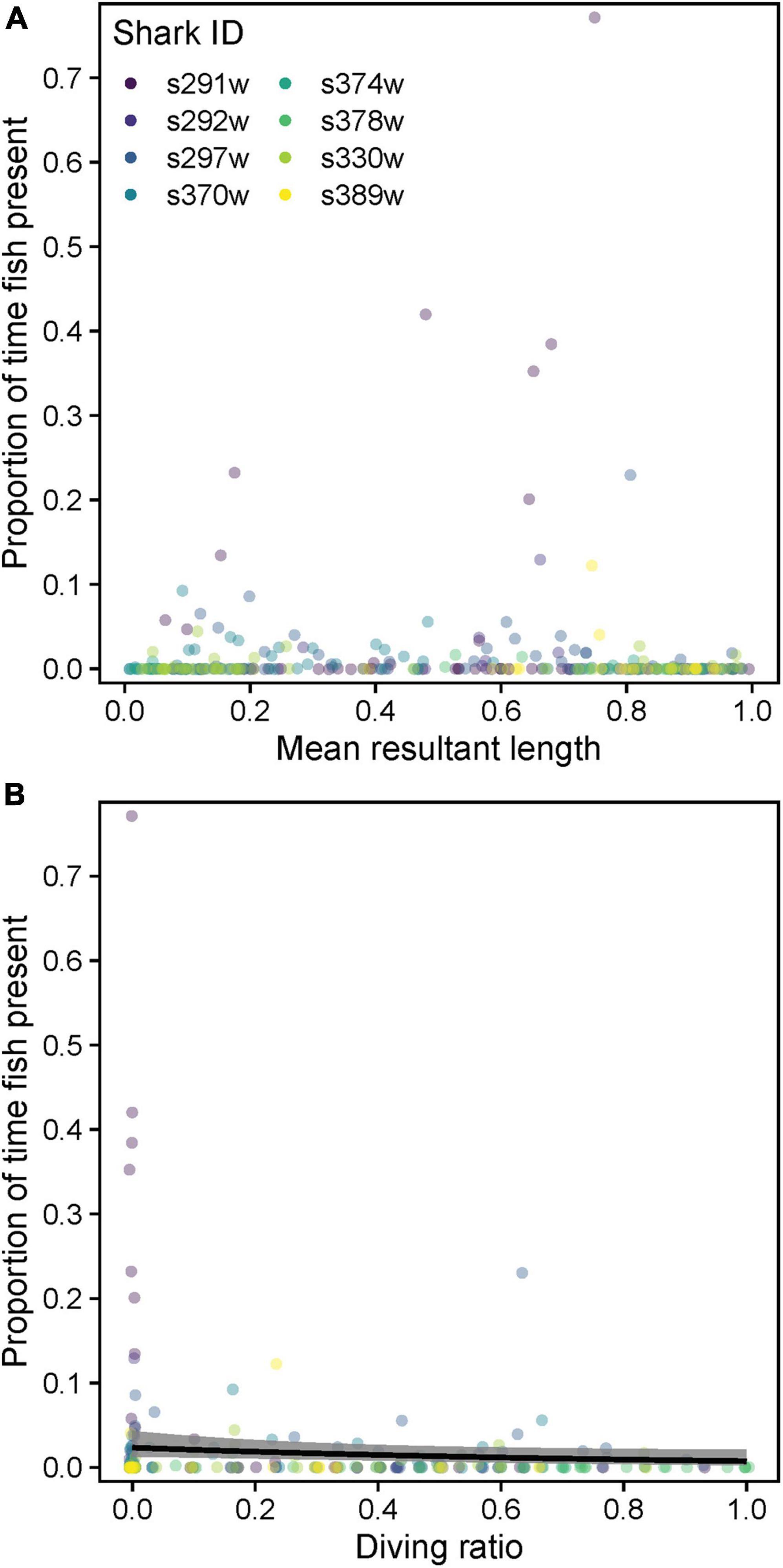
Figure 11. Relationships between the proportion of time with fish visible in video footage (pfish) and (A) mean resultant length and (B) diving ratio. Variables were computed over 15 min windowed intervals for each shark. Predicted relationships (gray shading = 95% confidence intervals) from a zero-inflated beta mixed model are shown where a significant effect was detected (p < 0.05).
Discussion
We combined video analysis, inertial measurement data, three-dimensional track reconstruction and behavioral state modeling through HMMs to perform an integrated analysis of post-release recovery processes in white sharks, revealing new insights into the nature and timing of cryptic post-release behavioral shifts, and factors influencing these. Overall, post-release responses included a period of offshore movement combined with more rapid tailbeats, followed by a transition to a diel pattern of daytime circling behavior with increased diving at night. Our findings provide critical information for the management and conservation of a threatened marine apex predator, but further offer important new insights into underlying functional bases of hidden aspects of animal movement and behavior. More broadly, we show how multisensor biologging in combination with HMMs and track reconstruction can improve our knowledge of post-release recovery and natural behavior, alike, which is critical for applied management (Wilson et al., 2014), and to address methodological and ethical considerations in pure biologging research (Williams et al., 2020).
Our results indicate a period of consistent, immediate offshore dispersal in white sharks after release from capture, with most individuals relocating into continental shelf waters (≥40–60 m depth, ∼10–30 km offshore) within ∼6 h and remaining there for the duration of their deployments (up to 136.2 h). Although some animals can exhibit disorientation and reduced movement rates immediately following capture (e.g., Luschi et al., 2020), rapid post-release offshore movements, similar to our observations in white sharks, have also been described across a range of marine taxa (Gunn et al., 2003; Mangel et al., 2011; Afonso and Hazin, 2014; Barnes et al., 2016), and have been interpreted to be a “flight” response associated with capture stress (Lear and Whitney, 2016). Supporting this explanation, offshore dispersal of white sharks coincided with a period of faster tailbeats (i.e., rapid movement), which gradually slowed to a more constant average rate, indicating a population-level recovery period (return to “baseline” tailbeat signature) of 9.7 h. This pattern and rate of TBC recovery is similar to that identified for common blacktip sharks (Carcharhinus limbatus, 9 h; Whitney et al., 2016), but longer than for tiger sharks (Galeocerdo cuvier, 4 h; Andrzejaczek et al., 2019a), potentially suggesting lower sensitivity to capture in tiger sharks (consistent with Gallagher et al., 2014). Activity level metrics such as TBC are clearly useful for enabling standardized comparisons of recovery rates both between and within species (Brivio et al., 2015; Whitney et al., 2016; Shuert et al., 2021), yet such applications amongst elasmobranchs remain rare or are limited by short post-release monitoring periods (Bullock et al., 2015; Whitney et al., 2018; Raoult et al., 2019). Despite a low sample size, we also revealed that recovery times increased as shark length decreased. Although physiological stress responses to capture (e.g., lactate accumulation) are known to be magnified in smaller individuals for some shark species, which can influence post-release outcomes (Gallagher et al., 2014; Talwar et al., 2017; Bowlby et al., 2021), white sharks across a range of sizes are quite physiologically resilient to short captures (<75 min, as per our study; Gallagher et al., 2019; Tate et al., 2019). Therefore, other factors (e.g., size-specific personalities) may underlie the 10-fold variance in behavioral recovery (∼3–30 h) we observed across sizes. Nevertheless, the relatively rapid tailbeat recoveries overall (<16 h, excluding the smallest individual) combined with offshore dispersal of released individuals holds important applied management implications for white sharks, supporting SMART drumlines as a promising non-lethal alternative approach to shark bite mitigation via temporary offshore relocation of potentially dangerous sharks (Tate et al., 2021a,b).
While activity level-based methods such as tailbeat analysis or accelerometry can provide more accurate indications of recovery compared to telemetry or dive profiles (Whitney et al., 2016), these unidimensional approaches can still miss or only partly resolve important behavioral dynamics (e.g., fine scale shifts in movement patterns or habitat use; Bullock et al., 2015). Our findings provide a prime example of this, highlighting the importance of a multifaceted approach to assessing recovery. By also integrating video analysis, magnetometer, accelerometer and depth data, track reconstruction and behavioral state modeling with HMMs, we revealed a further behavioral shift, occurring subsequent to the tailbeat-derived recovery period, involving highly tortuous, alternating clockwise-counterclockwise circling behaviors occurring over spatially restricted scales (10s of meters, with rotations completed in ∼1–2 min). This finding represents the first record of this behavior in white sharks, although it has recently been identified in a few other species of sharks and marine megafauna (Andrzejaczek et al., 2018; Narazaki et al., 2021). These previous studies suggested that circling may represent a prey search strategy, or assist in navigation via geomagnetic alignment, although direct observations (e.g., video) linking to either of these explanations remain limited (Andrzejaczek et al., 2018; Narazaki et al., 2021). However, video analysis in our study indicated that this activity was initiated and persisted during the day mostly in the absence of prey. Concomitantly, fish presence was not significantly related to white shark swimming tortuosity over the time scales at which circling occurred (min to h), and most direct foraging opportunities (e.g., presence of large snapper) passed without interaction (Supplementary Video 2). These results indicate that foraging is an unlikely explanation for the circling activity documented in our study. We also report longer circling durations (often for extended continuous periods, up to a maximum 4.7 h) than previously identified (maximum ∼30–55 min; Narazaki et al., 2021), and it is unlikely that such extensive circling would be required purely for obtaining navigational information.
Rather, as a new explanation for consistent circling in sharks, we suggest this activity may represent a behavioral manifestation of unihemispheric sleep. Some seabirds, cetaceans and pinnipeds have been documented to lateralize brain activity to sleep unihemispherically whilst on the move, and this has also been hypothesized in obligate ram ventilating elasmobranchs, although it has not yet been physiologically proven or previously linked to circling activity in sharks (Kelly et al., 2019). However, seabirds circle in flight toward the side of the brain exhibiting unihemispheric slow wave sleep (Rattenborg et al., 2016, 2019) and we suggest a similar functional basis for the alternating clockwise-counterclockwise circling we observed in white sharks. The potential use of currents to maintain oxygenated water flow over gills whilst minimizing swimming activity during sleep has also been hypothesized for ram ventilators (Kukulya et al., 2016; Kelly et al., 2019). Our observations of white sharks maintaining an almost stationary position with slow tail beats whilst facing into the current during these circular swimming patterns supports the hypothesis that they are maximizing oxygen uptake during apparent rest behavior (see Supplementary Video 3). The observed diurnal peak in circling (similarly apparent in tiger sharks; Narazaki et al., 2021) also implies that sleep and coinciding reductions in energy consumption (e.g., slower tailbeats) occur primarily during the day. Diurnally reduced activity levels, responsiveness and so-called “milling” behavior suggest similar patterns in several other buccal pumping and ram ventilating species (Kelly et al., 2019, 2020; Byrnes et al., 2021).
Although surface swimming has previously been linked to traveling in white sharks (Jorgensen et al., 2012), we found that level swimming periods (with very low VVAM; Supplementary Table 3) at the surface and seabed were also strongly associated with circling events. Conversely, white shark diving and swimming path linearity were positively related and increased at night. These are critical insights for the interpretation of dive profiles, which are widely analyzed among marine taxa, although typically without complementary behavioral data (e.g., horizontal movements and energetic metrics) at appropriate spatiotemporal scales necessary to link diving patterns to specific behaviors (Andrzejaczek et al., 2019a,b). For instance, alternating periods with nocturnal “rapid oscillatory diving” and diurnal level swimming, punctuated by irregular, deep U-shaped dives, similar to what we observed, have previously been recorded for white sharks and other epipelagic fish, although a lack of complementary fine scale behavioral data in many of these studies limited inferences on the underlying behavioral motivations for these diving patterns (Dewar et al., 2004; Weng et al., 2007; Bruce and Bradford, 2012; Thums et al., 2013; Andrzejaczek et al., 2019b). The increase in track linearity and reduction in ODBA we detected during intensive diving offers empirical support to suggestions that rapid oscillations represent a cost-efficient transiting behavior, although this could also assist in targeting vertically transient prey at night (Jorgensen et al., 2012; Andrzejaczek et al., 2019b), or satisfy both of these objectives simultaneously (e.g., Gleiss et al., 2011). Overall, our study highlights the clear benefit of incorporating a combination of magnetometers and accelerometers, which remain underutilized in ecology, for unravelling functional correlations between animal movement patterns and specific behavioral modes (Williams et al., 2017; Gunner et al., 2020).
Hidden Markov models also presented an effective method for integrating multisensor information to enable behavioral inferences, and our study provides a first application combining these approaches to explicitly evaluate post-release behaviors. As a joint modeling method (sensu Leos-Barajas et al., 2017), HMMs were advantageous in allowing us to: (1) probabilistically and objectively relate our observations to distinct behavioral states; and then (2) evaluate how capture- and individual-specific covariates influenced complex behavioral dynamics. Conventional analyses (i.e., GAMMs) of tortuosity and diving patterns supported our HMM results and revealed similar overall patterns. However, the classification approach of HMMs was advantageous for teasing apart dynamics and shifts between specific movement states, which were difficult to discern objectively from conventional modeling approaches. The need to more effectively quantify and predict sublethal impacts of capture in animals is widely recognized, both for the ethical considerations of ecological research, and to improve the success of applied management objectives (e.g., threatened species conservation, species rehabilitation, and relocation strategies; Guy et al., 2013; Wilson et al., 2014; Williams et al., 2020). Whilst metrics and sensors of interest may vary between applications and study systems, generalized HMMs can flexibly incorporate a broad array of data types (probability distributions) and unlimited data streams (McClintock and Michelot, 2018). We see this as a widely applicable and intuitive approach for obtaining detailed information on post-capture and release responses. Importantly, this can also yield insights into the broader ecology of animals, as evidenced by the dynamics in circling activity we uncovered and hypothesized to represent unihemispheric sleep in white sharks. Extending movement-based HMMs (such as those we used) to also include physiological data streams (e.g., from heart rate or “neurologging” electroencephalogram (EEG) sensors) would likely improve links between physiological, behavioral and movement state variability and capture-associated or environmental covariates. Although there are ethical and technical hurdles for such technologies (Whitney et al., 2018; Williams et al., 2020; but see Yu et al., 2021), they provide dynamic indicators of internal state which could assist in validating behavioral inferences. In our case, concurrent EEG monitoring could confirm that circling activity in sharks is a behavioral marker of unihemispheric sleep (as demonstrated in seabirds; Rattenborg et al., 2016), although physiological monitoring generally is likely to help clarify the motivations (e.g., stress, fear, and physiological requirements) behind animals’ responses to external factors (Ditmer et al., 2015; Coffey et al., 2020). Future improvements for understanding post-capture and release behaviors could also be achieved through georeferenced mapping of HMM-modeled behaviors to specific environmental contexts, following implementation of variable speed estimates and GPS/ARGOS-anchored dead reckoned reconstruction of animals’ tracks (Battaile et al., 2015).
Our study illustrates the potential of multisensor biologging, combined with HMMs for distinguishing post-capture recovery phases and identifying cryptic behaviors in wild animals. In the case of white sharks, we revealed two post-release phases; (1) an offshore transit combined with rapid tailbeats and, (2) a transition to diurnal circling behavior, which we suggest could be an additional period linked to sleep and rest. Consequently, our findings provide both critical information for the management of human-wildlife conflict involving white sharks, and novel insights into understudied aspects of shark behavior. With increasing anthropogenic impacts on wild populations into the future, such approaches, especially if combined with novel physiological monitoring, will be important to help better inform management and conservation strategies (Wilson et al., 2014) and improve our understanding of the behavioral ecology of animals across marine and terrestrial realms (Battaile et al., 2015; Bidder et al., 2015).
Data Availability Statement
The raw data supporting the conclusions of this article will be made available by the authors, without undue reservation.
Ethics Statement
All methods for capture, handling, sampling, and tagging of white sharks were approved under New South Wales Department of Primary Industries (NSW DPI) Animal Care and Ethics permits ACEC 17/04 and ACEC 07/08 and NSW DPI scientific collection permit #P01/0059(A)-4.0.
Author Contributions
RG, DR, VP, and GM-C conceived the study. RG collected and analyzed the data and led the writing of the manuscript. All authors contributed critically to the intellectual content, writing of the manuscript, and gave final approval for publication.
Funding
This work was supported by the New South Wales Department of Primary Industries through the Shark Management Strategy and University of Sydney. RG was supported by an Australian Government Research Training Program Stipend and supplementary scholarship from the NSW DPI/University of Sydney.
Conflict of Interest
The authors declare that the research was conducted in the absence of any commercial or financial relationships that could be construed as a potential conflict of interest.
Publisher’s Note
All claims expressed in this article are solely those of the authors and do not necessarily represent those of their affiliated organizations, or those of the publisher, the editors and the reviewers. Any product that may be evaluated in this article, or claim that may be made by its manufacturer, is not guaranteed or endorsed by the publisher.
Acknowledgments
We thank New South Wales Department of Primary Industries staff, including Ian Stockton and Craig Brand, and SMART drumline contractors, for their assistance in catching and tagging sharks, and helping to recover our tags at sea. Special thanks also to Nikolai Liebsch for his technical assistance and advice in configuring and operating the CATS cam tags, and beachcomber for finding and returning one of our tags that washed ashore a year after it was deployed.
Supplementary Material
The Supplementary Material for this article can be found online at: https://www.frontiersin.org/articles/10.3389/fmars.2021.791185/full#supplementary-material
Footnotes
- ^ http://www.cats.is/
- ^ https://wildlifecomputers.com/
- ^ https://atstrack.com/
- ^ https://www.microstrain.com/software
References
Afonso, A. S., and Hazin, F. H. V. (2014). Post-release survival and behavior and exposure to fisheries in juvenile tiger sharks, Galeocerdo cuvier, from the South Atlantic. J. Exp. Mar. Biol. Ecol. 454, 55–62. doi: 10.1016/j.jembe.2014.02.008
Agostinelli, C., and Lund, U. (2017). ‘circular’: Circular Statistics. R package version 0.4.93. Available online at: https://r-forge.r-project.org/projects/circular/ (accessed February 15, 2020).
Andrzejaczek, S., Gleiss, A. C., Lear, K. O., Pattiaratchi, C. B., Chapple, T. K., and Meekan, M. G. (2019a). Biologging tags reveal links between fine-scale horizontal and vertical movement behaviors in Tiger Sharks (Galeocerdo cuvier). Front. Mar. Sci. 6:229. doi: 10.3389/fmars.2019.00229
Andrzejaczek, S., Gleiss, A. C., Pattiaratchi, C. B., and Meekan, M. G. (2018). First insights into the fine-scale movements of the sandbar shark, Carcharhinus plumbeus. Front Mar Sci. 5, 483. doi: 10.3389/fmars.2018.00483
Andrzejaczek, S., Gleiss, A. C., Pattiaratchi, C. B., and Meekan, M. G. (2019b). Patterns and drivers of vertical movements of the large fishes of the epipelagic. Rev. Fish. Biol. Fisher. 29:2. doi: 10.1007/s11160-019-09555-1
Barnes, C. J., Butcher, P. A., Macbeth, W. G., Mandelman, J. W., Smith, S. D. A., and Peddemors, V. M. (2016). Movements and mortality of two commercially exploited carcharhinid sharks following longline capture and release off eastern Australia. Endanger. Species Res. 30, 193–208. doi: 10.3354/esr00730
Barton, K. (2020). MuMIn: Multi-Model Inference. R package version 1.43.17. Available online at: https://CRAN.R-project.org/package=MuMIn (accessed April 15, 2020).
Battaile, B. (2019). TrackReconstruction: Reconstruct Animal Tracks from Magnetometer, Accelerometer, Depth and Optional Speed Data. R package version 1.2. Available online at: https://CRAN.R-project.org/package=TrackReconstruction (accessed July 15, 2019).
Battaile, B. C., Nordstrom, C. A., Liebsch, N., and Trites, A. W. (2015). Foraging a new trail with northern fur seals (Callorhinus ursinus): lactating seals from islands with contrasting population dynamics have different foraging strategies, and forage at scales previously unrecognized by GPS interpolated dive data. Mar. Mammal. Sci. 31:4. doi: 10.1111/mms.12240
Becciolini, V., Lanini, F., and Ponzetta, M. P. (2019). Impact of capture and chemical immobilization on the spatial behaviour of red deer Cervus elaphus hinds. Wildlife Biol. 1, 1–8. doi: 10.1111/2981/w1b.00499
Benoit-Bird, K. J., Battaile, B. C., Nordstrom, C. A., and Trites, A. W. (2013). Foraging behavior of northern fur seals closely matches the hierarchical patch scales of prey. Mar. Ecol. Prog. Ser. 479, 283–302. doi: 10.3354/meps10209
Bidder, O. R., Walker, J. S., Jones, M. W., Holton, M. D., Urge, P., Scantlebury, D. M., et al. (2015). Step by step: reconstruction of terrestrial animal movement paths by dead-reckoning. Mov. Ecol. 3:23. doi: 10.1186/s40462-015-0055-4
Bograd, S. J., Block, B. A., Costa, D. P., and Godley, B. J. (2010). Biologging technologies: new tools for conservation. Introduction. Endanger. Species Res. 10, 1–7. doi: 10.3354/esr00269
Bowlby, H. D., Benoît, H. P., Joyce, W., Sulikowski, J., Coelho, R., Domingo, A., et al. (2021). Beyond post-release mortality: inferences on recovery periods and natural mortality from electronic tagging data for discarded Lamnid Sharks. Front. Mar. Sci. 8:619190. doi: 10.3389/fmars.2021.619190
Brivio, F., Grignolio, S., Sica, N., Cerise, S., and Bassano, B. (2015). Assessing the impact of capture on wild animals: the case study of chemical immobilisation on alpine ibex. PLoS One 10:e0130957. doi: 10.1371/journal.pone.0130957
Brooks, M. E., Kristensen, K., Van Benthem, K. J., Magnusson, A., Berg, C. W., Nielsen, A., et al. (2017). glmmTMB balances speed and flexibility among packages for zero-inflated generalized linear mixed modeling. R J. 9, 378–400. doi: 10.32614/RJ-2017-066
Bruce, B. D., and Bradford, R. W. (2012). “Habitat use and spatial dynamics of juvenile white sharks, Carcharodon carcharias,” in Eastern Australia,” in Global Perspectives on the Biology and Life History of the White Shark, ed. M. L. Domeier (Boca Raton, FL: CRC Press), 225–254.
Bullock, R. W., Guttridge, T. L., Cowx, I. G., Elliott, M., and Gruber, S. H. (2015). The behaviour and recovery of juvenile lemon sharks Negaprion brevirostris in response to external accelerometer tag attachment. J. Fish. Biol. 87, 1342–1354. doi: 10.1111/jfb.12808
Byrnes, E. E., Daly, R., Leos-Barajas, V., Langrock, R., and Gleiss, A. C. (2021). Evaluating the constraints governing activity patterns of a coastal marine top predator. Mar. Biol. 168, 11. doi: 10.1007/s00227-020-03803-w
Carter, M. I. D., McClintock, B. T., Embling, C. B., Bennett, K. A., Thompson, D., and Russell, D. J. F. (2020). From pup to predator: generalized hidden Markov models reveal rapid development of movement strategies in a naïve long-lived vertebrate. Oikos 129, 630–642. doi: 10.1111/oik.06853
Chapman, B. K., and McPhee, D. (2016). Global shark attack hotspots: Identifying underlying factors behind increased unprovoked shark bite incidence. Ocean Coast. Manage. 133, 72–84. doi: 10.1016/j.ocecoaman.2016.09.010
Chapple, T. K., Gleiss, A. C., Jewell, O. J., Wikelski, M., and Block, B. A. (2015). Tracking sharks without teeth: a non-invasive rigid tag attachment for large predatory sharks. Anim. Biotelemetry 3:14. doi: 10.1186/s40317-015-0044-9
Coffey, D. M., Royer, M. A., Meyer, C. G., and Holland, K. N. (2020). Diel patterns in swimming behavior of a vertically migrating deepwater shark, the bluntnose sixgill (Hexanchus griseus). PLoS One 15:e0228253. doi: 10.1371/journal.pone.0228253
Colefax, A. P., Kelaher, B. P., Pagendam, D. E., and Butcher, P. A. (2020). Assessing White Shark (Carcharodon carcharias) behavior along coastal beaches for conservation-focused shark mitigation. Front. Mar. Sci. 7:268. doi: 10.3389/fmars.2020.00268
Conners, M. G., Michelot, T., Heywood, E. I., Orben, R. A., Phillips, R. A., Vyssotski, A. L., et al. (2021). Hidden Markov models identify major movement modes in accelerometer and magnetometer data from four albatross species. Mov. Ecol. 9:7. doi: 10.1186/s40462-021-00243-z
Dewar, H., Domeier, M., and Nasby-Lucas, N. (2004). Insights into young of the year white shark, Carcharodon carcharias, behavior in the Southern California Bight. Environ. Biol. Fishes 70, 133–143. doi: 10.1023/B:EBFI.0000029343.54027.6a
Ditmer, M. A., Garshelis, D. L., Noyce, K. V., Laske, T. G., Iaizzo, P. A., Burk, T. E., et al. (2015). Behavioral and physiological responses of American black bears to landscape features within an agricultural region. Ecosphere 6, 1–21. doi: 10.1890/es14-00199.1
Douma, J. C., and Weedon, J. T. (2019). Analysing continuous proportions in ecology and evolution: a practical introduction to beta and Dirichlet regression. Methods Ecol. Evol. 10, 1412–1430. doi: 10.1111/2041-210x.13234
Farrell, E., and Fuiman, L. (2013). animalTrack: Animal Track Reconstruction for High Frequency 2-Dimensional (2D) or 3-Dimensional (3D) Movement Data. R package version 1.0.0. Available onlline at: https://CRAN.R-project.org/package=animalTrack (accessed September 23, 2013).
Friard, O., and Gamba, M. (2016). BORIS: a free, versatile open-source event-logging software for video/audio coding and live observations. Methods Ecol Evol. 7, 1325–1330. doi: 10.1111/2041-210x.12584
Gallagher, A. J., Meyer, L., Pethybridge, H. R., Huveneers, C., and Butcher, P. A. (2019). Effects of short-term capture on the physiology of white sharks Carcharodon carcharias: amino acids and fatty acids. Endanger. Species Res. 40, 297–308. doi: 10.3354/esr00997
Gallagher, A. J., Serafy, J. E., Cooke, S. J., and Hammerschlag, N. (2014). Physiological stress response, reflex impairment, and survival of five sympatric shark species following experimental capture and release. Mar. Ecol. Prog. Ser. 496, 207–218. doi: 10.3354/meps10490
Gleiss, A. C., Norman, B., and Wilson, R. P. (2011). Moved by that sinking feeling: variable diving geometry underlies movement strategies in whale sharks. Funct. Ecol. 25, 595–607. doi: 10.1111/j.1365-2435.2010.01801.x
Grainger, R., Peddemors, V. M., Raubenheimer, D., and Machovsky-Capuska, G. E. (2020). Diet composition and nutritional niche breadth variability in juvenile white sharks (Carcharodon carcharias). Front. Mar. Sci. 7:422. doi: 10.3389/fmars.2020.00422
Grecian, W. J., Lane, J. V., Michelot, T., Wade, H. M., and Hamer, K. C. (2018). Understanding the ontogeny of foraging behaviour: insights from combining marine predator bio-logging with satellite-derived oceanography in hidden Markov models. J. R. Soc. Interface 15:20180084. doi: 10.1098/rsif.2018.0084
Guida, L., Walker, T. I., and Reina, R. D. (2016). Temperature Insensitivity and Behavioural Reduction of the Physiological Stress Response to Longline Capture by the Gummy Shark, Mustelus antarcticus. PLoS One 11:e0148829. doi: 10.1371/journal.pone.0148829
Gunn, J. S., Patterson, T. A., and Pepperell, J. G. (2003). Short-term movement and behaviour of black marlin Makaira indica in the Coral Sea as determined through a pop-up satellite archival tagging experiment. Mar. Freshw. Res. 54, 515–525. doi: 10.1071/mf03022
Gunner, R. M., Wilson, R. P., Holton, M. D., Scott, R., Hopkins, P., and Duarte, C. M. (2020). A new direction for differentiating animal activity based on measuring angular velocity about the yaw axis. Ecol. Evol. 10, 7872–7886. doi: 10.1002/ece3.6515
Guy, A. J., Curnoe, D., and Banks, P. B. (2013). A survey of current mammal rehabilitation and release practices. Biodivers. Conserv. 22, 825–837. doi: 10.1007/s10531-013-0452-1
Guyomard, D., Perry, C., Tournoux, P. U., Cliff, G., Peddemors, V., and Jaquemet, S. (2019). An innovative fishing gear to enhance the release of non-target species in coastal shark-control programs: the SMART (shark management alert in real-time) drumline. Fish. Res. 216, 6–17. doi: 10.1016/j.fishres.2019.03.011
Hussey, N. E., Kessel, S. T., Aarestrup, K., Cooke, S. J., Cowley, P. D., Fisk, A. T., et al. (2015). Aquatic animal telemetry: a panoramic window into the underwater world. Science 348:1255642. doi: 10.1126/science.1255642
Jewell, O. J. D., Gleiss, A. C., Jorgensen, S. J., Andrzejaczek, S., Moxley, J. H., Beatty, S. J., et al. (2019). Cryptic habitat use of white sharks in kelp forest revealed by animal-borne video. Biol. Lett. 15:20190085. doi: 10.1098/rsbl.2019.0085
Jorgensen, S. J., Anderson, S., Ferretti, F., Tietz, J. R., Chapple, T., Kanive, P., et al. (2019). Killer whales redistribute white shark foraging pressure on seals. Sci. Rep. 9:6153. doi: 10.1038/s41598-019-39356-2
Jorgensen, S. J., Arnoldi, N. S., Estess, E. E., Chapple, T. K., Ruckert, M., Anderson, S. D., et al. (2012). Eating or Meeting? Cluster analysis reveals intricacies of white shark (Carcharodon carcharias) migration and offshore behavior. PLoS One 7:e0047819. doi: 10.1371/journal.pone.0047819
Jorgensen, S. J., Reeb, C. A., Chapple, T. K., Anderson, S., Perle, C., Van Sommeran, S. R., et al. (2010). Philopatry and migration of Pacific white sharks. Proc R Soc B-Biol Sci. 277, 1682. doi: 10.1098/rspb.2009.1155
Kays, R., Crofoot, M. C., Jetz, W., and Wikelski, M. (2015). Terrestrial animal tracking as an eye on life and planet. Science 348, aaa2478. doi: 10.1126/science.aaa2478
Kelly, M. L., Collin, S. P., Hemmi, J. M., and Lesku, J. A. (2019). Evidence for sleep in sharks and rays: behavioural, physiological, and evolutionary considerations. Brain Behav. Evol. 94, 37–50. doi: 10.1159/000504123
Kelly, M. L., Spreitzenbarth, S., Kerr, C. C., Hemmi, J. M., Lesku, J. A., Radford, C. A., et al. (2020). Behavioural sleep in two species of buccal pumping sharks (Heterodontus portusjacksoni and Cephaloscyllium isabellum). J. Sleep Res. 30:e13139. doi: 10.1111/jsr.13139
Kukulya, A. L., Stokey, R., Fiester, C., Padilla, E. M. H., and Skomal, G. (2016). “Multi-Vehicle Autonomous Tracking and Filming of White Sharks Carcharodon carcharias,” in Proccedings of the Autonomous Underwater Vehicles (AUV), 2016 IEEE/OES. (Tokyo), 423–430. doi: 10.1109/AUV.2016.7778707
Lear, K. O., and Whitney, N. M. (2016). Bringing data to the surface: recovering data loggers for large sample sizes from marine vertebrates. Anim. Biotelemetry 4:12. doi: 10.1186/s40317-016-0105-8
Lee, K. A., Butcher, P. A., Harcourt, R. G., Patterson, T. A., Peddemors, V. M., Roughan, M., et al. (2021). Oceanographic conditions associated with white shark (Carcharodon carcharias) habitat use along eastern Australia. Mar. Ecol. Prog. Ser. 659, 143–159. doi: 10.3354/meps13572
Leos-Barajas, V., Photopoulou, T., Langrock, R., Patterson, T. A., Watanabe, Y. Y., Murgatroyd, M., et al. (2017). Analysis of animal accelerometer data using hidden Markov models. Methods Ecol. Evol. 8, 161–173. doi: 10.1111/2041-210x.12657
Lüdecke, D., Makowski, D., Waggoner, P., and Patil, I. (2020). Assessment of Regression Models Performance. CRAN. Available online at: https://easystats.github.io/performance/ (accessed January 10, 2021).
Luschi, P., Sozbilen, D., Cerritelli, G., Ruffier, F., Baskale, E., and Casale, P. (2020). A biphasic navigational strategy in loggerhead sea turtles. Sci. Rep. 10:18130. doi: 10.1038/s41598-020-75183-6
Machovsky-Capuska, G. E., Coogan, S. C. P., Simpson, S. J., and Raubenheimer, D. (2016). Motive for killing: what drives prey choice in wild predators? Ethology 122, 703–711. doi: 10.1111/eth.12523
Mangel, J. C., Alfaro-Shigueto, J., Witt, M. J., Dutton, P. H., Seminoff, J. A., and Godley, B. J. (2011). Post-capture movements of loggerhead turtles in the southeastern Pacific Ocean assessed by satellite tracking. Mar. Ecol. Prog. Ser. 433, 261–272. doi: 10.3354/meps09152
McClintock, B. T. (2021). Worth the effort? A practical examination of random effects in hidden Markov models for animal telemetry data. Methods Ecol. Evol. 12, 1475–1497. doi: 10.1111/2041-210x.13619
McClintock, B. T., Langrock, R., Gimenez, O., Cam, E., Borchers, D. L., Glennie, R., et al. (2020). Uncovering ecological state dynamics with hidden Markov models. Ecol. Lett. 23, 1878–1903. doi: 10.1111/ele.13610
McClintock, B. T., and Michelot, T. (2018). momentuHMM: R package for generalized hidden Markov models of animal movement. Methods Ecol. Evol. 9, 1518–1530. doi: 10.1111/2041-210x.12995
McPhee, D. P., Blount, C., Smith, M. P. L., and Peddemors, V. M. (2021). A comparison of alternative systems to catch and kill for mitigating unprovoked shark bite on bathers or surfers at ocean beaches. Ocean Coast. Manage. 201, 105492. doi: 10.1016/j.ocecoaman.2020.105492
Mul, E., Blanchet, M. A., McClintock, B. T., Grecian, W. J., Biuw, M., and Rikardsen, A. (2020). Killer whales are attracted to herring fishing vessels. Mar. Ecol. Prog. Ser. 652, 1–13. doi: 10.3354/meps13481
Narazaki, T., Nakamura, I., Aoki, K., Iwata, T., Shiomi, K., Luschi, P., et al. (2021). Similar circling movements observed across marine megafauna taxa. iScience 24:102221. doi: 10.1016/j.isci.2021.102221
Nathan, R., Getz, W. M., Revilla, E., Holyoak, M., Kadmon, R., Saltz, D., et al. (2008). A movement ecology paradigm for unifying organismal movement research. Proc. Natl. Acad. Sci. U.S.A. 105, 19052–19059. doi: 10.1073/pnas.0800375105
Patterson, T. A., Parton, A., Langrock, R., Blackwell, P. G., Thomas, L., and King, R. (2017). Statistical modelling of individual animal movement: an overview of key methods and a discussion of practical challenges. AStA Adv. Stat. Anal. 101, 399–438. doi: 10.1007/s10182-017-0302-7
Pearson, H. C., Jones, P. W., Srinivasan, M., Lundquist, D., Pearson, C. J., Stockin, K. A., et al. (2017). Testing and deployment of C-VISS (cetacean-borne video camera and integrated sensor system) on wild dolphins. Mar. Biol. 164:42. doi: 10.1007/s00227-017-3079-z
Pinheiro, J., Bates, D., DebRoy, S., Sarkar, D., and R Core Team (2020). nlme: Linear and Nonlinear Mixed Effects Models. R package version 3.1-148. Available online at: https://CRAN.R-project.org/package=nlme (accessed 09 07, 2021).
R Core Team (2020). R: A Language and Environment for Statistical Computing. Vienna: R Foundation for Statistical Computing.
Raoult, V., Williamson, J. E., Smith, T. M., and Gaston, T. F. (2019). Effects of on-deck holding conditions and air exposure on post-release behaviours of sharks revealed by a remote operated vehicle. J. Exp. Mar. Biol. Ecol. 511, 10–18. doi: 10.1016/j.jembe.2018.11.003
Rattenborg, N. C., van der Meij, J., Beckers, G. J. L., and Lesku, J. A. (2019). Local Aspects of Avian Non-REM and REM Sleep. Front. Neurosci. 13:567. doi: 10.3389/fnins.2019.00567
Rattenborg, N. C., Voirin, B., Cruz, S. M., Tisdale, R., Dell’Omo, G., Lipp, H. P., et al. (2016). Evidence that birds sleep in mid-flight. Nat. Commun. 7, 12468. doi: 10.1038/ncomms12468
Rigby, C. L., Barreto, R., Carlson, J., Fernando, D., Fordham, S., Francis, M. P., et al. (2019). Carcharodon carcharias. The IUCN Red List of Threatened Species 2019. e.T3855A2878674. Available online at: https://dx.doi.org/10.2305/IUCN.UK.2019-3.RLTS.T3855A2878674.en. (accessed October 6, 2021).
Rode, K. D., Pagano, A. M., Bromaghin, J. F., Atwood, T. C., Durner, G. M., Simac, K. S., et al. (2014). Effects of capturing and collaring on polar bears: findings from long-term research on the southern Beaufort Sea population. Wildlife Res. 41, 311–322. doi: 10.1071/wr13225
Roesch, A., and Schmidbauer, H. (2018). WaveletComp: Computational Wavelet Analysis. R package version 1.1. Available online at: https://CRAN.R-project.org/package=WaveletComp (accessed March 18, 2021).
Schielzeth, H., and Forstmeier, W. (2009). Conclusions beyond support: overconfident estimates in mixed models. Behav. Ecol. 20, 416–420. doi: 10.1093/beheco/arn145
Semmens, J. M., Kock, A. A., Watanabe, Y. Y., Shepard, C. M., Berkenpas, E., Stehfest, K. M., et al. (2019). Preparing to launch: biologging reveals the dynamics of white shark breaching behaviour. Mar. Biol. 166:95. doi: 10.1007/s00227-019-3542-0
Shea, B. D., Benson, C. W., de Silva, C., Donovan, D., Romeiro, J., Bond, M. E., et al. (2020). Effects of exposure to large sharks on the abundance and behavior of mobile prey fishes along a temperate coastal gradient. PLoS One 15:e0230308. doi: 10.1371/journal.pone.0230308
Shepard, E. L. C., Lambertucci, S. A., Vallmitjana, D., and Wilson, R. P. (2011). Energy beyond food: foraging theory informs time spent in thermals by a large soaring bird. PLoS One 6:e0027375. doi: 10.1371/journal.pone.0027375
Shepard, E. L. C., Wilson, R. P., Halsey, L. G., Quintana, F., Laich, A. G., Gleiss, A. C., et al. (2008). Derivation of body motion via appropriate smoothing of acceleration data. Aquat. Biol. 4, 235–241. doi: 10.3354/ab00104
Shillinger, G. L., Bailey, H., Bograd, S. J., Hazen, E. L., Hamann, M., Gaspar, P., et al. (2012). Tagging through the stages: technical and ecological challenges in observing life histories through biologging. Mar. Ecol. Prog. Ser. 457, 165–170. doi: 10.3354/meps09816
Shuert, C. R., Marcoux, M., Hussey, N. E., Watt, C. A., and Auger-Methe, M. (2021). Assessing the post-release effects of capture, handling and placement of satellite telemetry devices on narwhal (Monodon monoceros) movement behaviour. Conserv. Physiol. 9:coaa128. doi: 10.1093/conphys/coaa128
Simpfendorfer, C. A., Heupel, M. R., and Kendal, D. (2021). Complex human-shark conflicts confound conservation action. Front. Conserv. Sci. 2:692767. doi: 10.3389/fcosc.2021.692767
Skomal, G. B., Braun, C. D., Chisholm, J. H., and Thorrold, S. R. (2017). Movements of the white shark Carcharodon carcharias in the North Atlantic Ocean. Mar. Ecol. Prog. Ser. 580, 1–16. doi: 10.3354/meps12306
Spaet, J. L. Y., Manica, A., Brand, C. P., Gallen, C., and Butcher, P. A. (2020a). Environmental conditions are poor predictors of immature white shark Carcharodon carcharias occurrences on coastal beaches of eastern Australia. Mar. Ecol. Prog. Ser. 653, 167–179. doi: 10.3354/meps13488
Spaet, J. L. Y., Patterson, T. A., Bradford, R. W., and Butcher, P. A. (2020b). Spatiotemporal distribution patterns of immature Australasian white sharks (Carcharodon carcharias). Sci. Rep. 10, 10169. doi: 10.1038/s41598-020-66876-z
Talwar, B., Brooks, E. J., Mandelman, J. W., and Grubbs, R. D. (2017). Stress, post-release mortality, and recovery of commonly discarded deep-sea sharks caught on longlines. Mar. Ecol. Prog. Ser. 582, 147–161. doi: 10.3354/meps12334
Tate, R. D., Cullis, B. R., Smith, S. D. A., Kelaher, B. P., Brand, C. P., Gallen, C. R., et al. (2019). The acute physiological status of white sharks (Carcharodon carcharias) exhibits minimal variation after capture on SMART drumlines. Conserv Physiol. 7:1. doi: 10.1093/conphys/coz042
Tate, R. D., Kelaher, B. P., Brand, C. P., Cullis, B. R., Gallen, C. R., Smith, S. D. A., et al. (2021a). The effectiveness of Shark-Management-Alert-in-Real-Time (SMART) drumlines as a tool for catching white sharks, Carcharodon carcharias, off coastal New South Wales, Australia. Fish. Manag. Ecol. 28, 496–506. doi: 10.1111/fme.12489
Tate, R. D., Kelaher, B. P., Brand, C. P., Gallen, C. R., Smith, S. D. A., and Butcher, P. A. (2021b). Shark behaviour and marine faunal assemblage beneath SMART drumlines. Fish Res. 243, 106102. doi: 10.1016/j.fishres.2021.106102
Thums, M., Meekan, M., Stevens, J., Wilson, S., and Polovina, J. (2013). Evidence for behavioural thermoregulation by the world’s largest fish. J. R. Soc. Interface 10:20120477. doi: 10.1098/rsif.2012.0477
Towner, A. V., Leos-Barajas, V., Langrock, R., Schick, R. S., Smale, M. J., Kaschke, T., et al. (2016). Sex-specific and individual preferences for hunting strategies in white sharks. Funct. Ecol. 30, 1397–1407. doi: 10.1111/1365-2435.12613
Walker, B. (1998). The art and science of wildlife management. Wildlife Res. 25, 1–9. doi: 10.1071/wr97030
Watanabe, Y. Y., Payne, N. L., Semmens, J. M., Fox, A., and Huveneers, C. (2019a). Hunting behaviour of white sharks recorded by animal-borne accelerometers and cameras. Mar. Ecol. Prog. Ser. 621, 221–227. doi: 10.3354/meps12981
Watanabe, Y. Y., Payne, N. L., Semmens, J. M., Fox, A., and Huveneers, C. (2019b). Swimming strategies and energetics of endothermic white sharks during foraging. J. Exp. Biol. 222(Pt 4), jeb185603. doi: 10.1242/jeb.185603
Weng, K. C., Boustany, A. M., Pyle, P., Anderson, S. D., Brown, A., and Block, B. A. (2007). Migration and habitat of white sharks (Carcharodon carcharias) in the eastern Pacific Ocean. Mar. Biol. 152, 877–894. doi: 10.1007/s00227-007-0739-4
Wessel, P., and Smith, W. H. F. (1996). A global, self-consistent, hierarchical, high-resolution shoreline database. J. Geophys. Res. 101, 8741–8743. doi: 10.1029/96JB00104
Whitney, N. M., Lear, O., Gleiss, A. C., Payne, N., and White, C. F. (2018). “Advances in the application of high-resolution biologgers to elasmobranch fishes,” in Shark Research: Emerging Technologies and Applications for the Field and Laboratory, eds J. C. Carrier, M. R. Heithaus, and C. A. Simpfendorfer (Boca Raton, FL: CRC Press), 45–70.
Whitney, N. M., White, C. F., Gleiss, A. C., Schwieterman, G. D., Anderson, P., Hueter, R. E., et al. (2016). A novel method for determining post-release mortality, behavior, and recovery period using acceleration data loggers. Fish. Res. 183, 210–221. doi: 10.1016/j.fishres.2016.06.003
Williams, H. J., Holton, M. D., Shepard, E. L. C., Largey, N., Norman, B., Ryan, P. G., et al. (2017). Identification of animal movement patterns using tri-axial magnetometry. Mov. Ecol. 5, 6. doi: 10.1186/s40462-017-0097-x
Williams, H. J., Taylor, L. A., Benhamou, S., Bijleveld, A. I., Clay, T. A., de Grissac, S., et al. (2020). Optimizing the use of biologgers for movement ecology research. J. Anim. Ecol. 89, 186–206. doi: 10.1111/1365-2656.13094
Wilmers, C. C., Nickel, B., Bryce, C. M., Smith, J. A., Wheat, R. E., and Yovovich, V. (2015). The golden age of bio-logging: how animal-borne sensors are advancing the frontiers of ecology. Ecology 96, 1741–1753. doi: 10.1890/14-1401.1
Wilson, R. P., Shepard, E., and Liebsch, N. (2008). Prying into the intimate details of animal lives: use of a daily diary on animals. Endanger. Species Res. 4, 123–137. doi: 10.3354/esr00064
Wilson, R. P., White, C. R., Quintana, F., Halsey, L. G., Liebsch, N., Martin, G. R., et al. (2006). Moving towards acceleration for estimates of activity-specific metabolic rate in free-living animals: the case of the cormorant. J. Anim. Ecol. 75, 1081–1090. doi: 10.1111/j.1365-2656.2006.01127.x
Wilson, S. M., Raby, G. D., Burnett, N. J., Hinch, S. G., and Cooke, S. J. (2014). Looking beyond the mortality of bycatch: sublethal effects of incidental capture on marine animals. Biol. Conserv. 171, 61–72. doi: 10.1016/j.biocon.2014.01.020
Wood, S. N. (2011). Fast stable restricted maximum likelihood and marginal likelihood estimation of semiparametric generalized linear models. J. R. Stat. Soc. Ser. B Stat. Methodol. 73, 3–36. doi: 10.1111/j.1467-9868.2010.00749.x
Yu, Y. C., Li, N., Li, Y., and Liu, W. T. (2021). A portable waterproof EEG acquisition device for dolphins. Sensors 21:3336. doi: 10.3390/s21103336
Zucchini, W., MacDonald, I. L., and Langrock, R. (2016). Hidden Markov Models for Time Series: An Introduction Using R. Boca Raton, FL: Chapman and Hall/CRC Press.
Keywords: Carcharodon carcharias, dead reckoning, hidden Markov model, multisensor biologging, post-release behavior, shark behavior, SMART drumline, unihemispheric sleep
Citation: Grainger R, Raubenheimer D, Peddemors VM, Butcher PA and Machovsky-Capuska GE (2022) Integrating Biologging and Behavioral State Modeling to Identify Cryptic Behaviors and Post-capture Recovery Processes: New Insights From a Threatened Marine Apex Predator. Front. Mar. Sci. 8:791185. doi: 10.3389/fmars.2021.791185
Received: 08 October 2021; Accepted: 29 November 2021;
Published: 04 January 2022.
Edited by:
Rob Harcourt, Macquarie University, AustraliaCopyright © 2022 Grainger, Raubenheimer, Peddemors, Butcher and Machovsky-Capuska. This is an open-access article distributed under the terms of the Creative Commons Attribution License (CC BY). The use, distribution or reproduction in other forums is permitted, provided the original author(s) and the copyright owner(s) are credited and that the original publication in this journal is cited, in accordance with accepted academic practice. No use, distribution or reproduction is permitted which does not comply with these terms.
*Correspondence: Richard Grainger, rgra1274@uni.sydney.edu.au; David Raubenheimer, david.raubenheimer@sydney.edu.au
†ORCID: Richard Grainger, orcid.org/0000-0001-9737-281X; David Raubenheimer, orcid.org/0000-0001-9050-1447; Victor M. Peddemors, orcid.org/0000-0002-8743-9782; Paul A. Butcher, orcid.org/0000-0001-7338-6037; Gabriel E. Machovsky-Capuska, orcid.org/0000-0001-8698-8424