- 1Cooperative Institute for Marine and Atmospheric Studies, University of Miami, Miami, FL, United States
- 2Atlantic Oceanographic and Meteorological Laboratory, National Oceanic and Atmospheric Administration, Miami, FL, United States
- 3Fish and Wildlife Research Institute, Florida Fish and Wildlife Conservation Commission, St. Petersburg, FL, United States
- 4Elizabeth Moore International Center for Coral Reef Research & Restoration, Mote Marine Laboratory, Summerland Key, FL, United States
- 5University of South Florida Center for Global Health and Infectious Diseases Research, Tampa, FL, United States
- 6Mote Marine Laboratory and Aquarium, Sarasota, FL, United States
The epizootic disease outbreak known as stony coral tissue loss disease (SCTLD) is arguably the most devastating coral disease in recorded history. SCTLD emerged off the coast of South Florida in 2014 and has since moved into the Caribbean, resulting in coral mortality rates that have changed reef structure and function. Currently, the cause of SCTLD is unknown, but there is evidence from 16S rRNA gene sequencing and bacterial culture studies that the microbial community plays a role in the progression of SCTLD lesions. In this study, we applied shotgun metagenomics to characterize the potential function of bacteria, as well as the composition of the micro-eukaryotic community, associated with SCTLD lesions. We re-examined samples that were previously analyzed using 16S rRNA gene high-throughput sequencing from four coral species: Stephanocoenia intersepta, Diploria labyrinthiformis, Dichocoenia stokesii, and Meandrina meandrites. For each species, tissue from apparently healthy (AH) corals, and unaffected tissue (DU) and lesion tissue (DL) on diseased corals, were collected from sites within the epidemic zone of SCTLD in the Florida Keys. Within the micro-eukaryotic community, the taxa most prominently enriched in DL compared to AH and DU tissue were members of Ciliophora. We also found that DL samples were relatively more abundant in less energy-efficient pathways like the pentose phosphate pathways. While less energy-efficient processes were identified, there were also relatively higher abundances of nucleotide biosynthesis and peptidoglycan maturation pathways in diseased corals compared to AH, which suggests there was more bacteria growth in diseased colonies. In addition, we generated 16 metagenome-assembled genomes (MAGs) belonging to the orders Pseudomonadales, Beggiatoales, Rhodobacterales, Rhizobiales, Rs-D84, Flavobacteriales, and Campylobacterales, and all MAGs were enriched in DL samples compared to AH samples. Across all MAGs there were antibiotic resistance genes that may have implications for the treatment of SCTLD with antibiotics. We also identified genes and pathways linked to virulence, such as nucleotide biosynthesis, succinate dehydrogenase, ureases, nickel/iron transporters, Type-1 secretion system, and metalloproteases. Some of these enzymes/pathways have been previously targeted in the treatment of other bacterial diseases and they may be of interest to mitigate SCTLD lesion progression.
Introduction
The Caribbean is notorious for emerging coral disease outbreaks (Mera and Bourne, 2018). As such, in 2014, a coral disease emerged off the coast of Miami-Dade County, Florida, which has led to an ongoing multi-year epizootic called stony coral tissue loss disease (SCTLD). SCTLD’s unprecedented characteristics, such as high numbers of coral species affected, mortality, transmission rates (Precht et al., 2016; Aeby et al., 2019), and wide geographic distribution and prevalence (Walton et al., 2018; Alvarez-Filip et al., 2019; Muller et al., 2020), has made it the largest coral disease outbreak yet described. Since its first documentation (Miller et al., 2016; Precht et al., 2016), SCTLD has spread through Florida’s Coral Reef (an over 560-km stretch off the coast of Southeast Florida) and is now found in Mexico (Alvarez-Filip et al., 2019), the U.S. Virgin Islands (Meiling et al., 2020), and the Turks and Caicos Islands (Heres et al., 2021), among other regions in the Caribbean (Kramer, 2021).
The distribution and severity of SCTLD have resulted in a significant loss in coral richness, coral tissue cover, and overall coral structure and function (Miller et al., 2016; Precht et al., 2016; Walton et al., 2018; Alvarez-Filip et al., 2019; Gintert et al., 2019; Williams et al., 2021). Given the severity of SCTLD, there is an urgency to identify the causative agent(s) of this disease. However, in the past, it has been difficult to identify pathogenic agents of coral diseases (Vega Thurber et al., 2020); of the ∼18 coral diseases described, Koch’s Postulates have been fulfilled for only six (Bourne et al., 2009). Researchers have mainly focused on identifying bacteria associated with SCTLD using 16S rRNA high-throughput sequencing (Meyer et al., 2019; Iwanowicz et al., 2020; Rosales et al., 2020; Becker et al., 2021; Clark et al., 2021; Thome et al., 2021) and culturing techniques (Ushijima et al., 2020). However, these methodologies have not provided definitive evidence that SCTLD is indeed caused by bacteria.
Bacterial taxa such as Rhodobacterales, Rhizobiales, Clostridiales, Alteromonadales, and Vibrionales are described in multiple SCTLD studies, but these taxa are not consistently found throughout all samples (Meyer et al., 2019; Iwanowicz et al., 2020; Rosales et al., 2020; Becker et al., 2021; Clark et al., 2021; Thome et al., 2021). In addition, these bacterial taxa are not unique to SCTLD, as many have been identified in other coral diseases, such as black band disease (BBD; Miller and Richardson, 2011), white-plague (Sunagawa et al., 2009), and rapid tissue loss (Rosales et al., 2019). There is also no histological evidence that suggests bacteria are primary pathogens for SCTLD (Landsberg et al., 2020). These findings have led to the assumption that these bacteria act as SCTLD opportunists or saprophytic microbes.
While some research suggests there is not a direct link between SCTLD and bacteria (Landsberg et al., 2020), there are significant differences in microbial communities of SCTLD lesions compared to healthy coral tissues and SCTLD lesions can be halted on colonies by antibiotics in both the lab (Aeby et al., 2019) and field (Neely et al., 2021; Shilling et al., 2021; Walker et al., 2021). Consequently, the application of the antibiotic amoxicillin is one of the primary interventions to combat this disease. Thus, there is likely a pivotal role that bacteria play in disease progression, but the relationship between SCTLD and the bacterial community may be more complex than the traditional one-pathogen one-disease dynamic. For example, the presence of the bacterium Vibrio coralliilyticus in corals with SCTLD increases the virulence of the disease, but V. coralliilyticus itself cannot elicit disease (Ushijima et al., 2020). Instead, SCTLD may be polymicrobial, and a destabilized coral holobiont (i.e., the coral animal, micro-eukaryotes, bacteria, archaea, and viruses) may lead to a pathogenic state after a disturbance (Vega Thurber et al., 2020). Therefore, to understand SCTLD pathology, it may be necessary to not just identify members of the diseased holobiont, but also to characterize their genetically encoded functional capabilities.
A powerful tool to comprehend the holobiont dynamics of SCTLD is shotgun metagenomics. This tool can be used to assemble bacterial genomes and genes from other members of the holobiont, as well as to characterize the functional capabilities of the microbial members, which may be imperative to explain how SCTLD manifests. For example, metagenomics has been used in coral biology to associate BBD with bacterial chemotaxis genes, motility genes (Tout et al., 2015), and gene clusters (Gunasekera et al., 2019). The study presented here uses shotgun metagenomics to characterize the taxonomy and potential function of bacteria associated with SCTLD identified from coral species sampled at outbreak reefs. While the microbiome composition of these samples was described previously using amplicon sequencing of the 16S rRNA gene (Rosales et al., 2020), the analysis here furthers our understanding of key bacteria and their potential roles within SCTLD. In addition, we characterize the 18S rRNA gene to identify micro-eukaryotes that also may be associated with SCTLD.
Materials and Methods
Sample Collection
Samples were collected as previously described (Rosales et al., 2020). In brief, samples were collected within the Florida Keys National Marine Sanctuary from Looe Key and East Washerwoman reefs, in April 2018 and June 2018, respectively, when these reefs were in the epidemic zone (i.e., moderate to high levels of disease activity) of SCTLD. At Looe Key, an offshore forereef in the Lower Keys, only colonies of the coral Meandrina meandrites (MMEA) were sampled. At East Washerwoman, a mid-channel patch reef in the Middle Keys, we collected tissue samples from three coral species: Stephanocoenia intersepta (SINT), Diploria labyrinthiformis (DLAB), and Dichocoenia stokesii (DSTO).
Coral tissues were collected by using 10-ml plastic syringes with a blunt end, which was used to scrape a small surface area on the coral. While scraping, the syringe plunger was pulled and the syringe was filled with a coral tissue and mucus slurry. At Looe Key, a total of 13 MMEA samples were collected, three samples from apparently healthy (AH) colonies and 10 samples from five diseased colonies. Each diseased colony consisted of two samples, one sample from unaffected tissue (DU) away from the lesion margin and one sample from the lesion margin (DL). At East Washerwoman, five AH and five diseased colonies were sampled from each of the three coral species (SINT, DLAB, DSTO). Like in the MMEA collections, two samples (DL and DU) were taken from five diseased colonies for each of the three target species, yielding 45 samples from that reef. Between the two reefs, a total of 58 samples were collected. Once on the boat, coral slurry samples were transferred from the syringes to 15-ml falcon tubes, placed on ice in a cooler, and transported to the South Florida Regional Laboratory in Marathon, FL, United States. In the lab, samples were flash-frozen in a liquid nitrogen dewar, taken to Mote Marine Laboratory in Summerland Key, FL, United States, and stored in the –80°C freezer.
Sample Processing
DNA was extracted with the DNeasy PowerSoil Kit (QIAGEN, Germantown, MD, United States). Modifications to the manufacturer’s protocol were conducted and previously reported in detail (Rosales et al., 2020). After extractions, DNA was quantified with the NanoDrop One™ Microvolume UV-Vis Spectrophotometer, which showed a similar range in DNA concentration among samples (17.8 ± 2.72 ng/μl; range = 8.41 [MMEA; DU] – 27.72 [SINT; AH] ng/μl). In total, 58 samples were sent to the Duke Genomic and Computational Biology Sequencing and Genomic Technologies (Durham, NC, United States). The libraries were prepared with the Roche Kapa HyperPrep kit and quantified with qPCR. All samples passed quality control and were sequenced across eight lanes of the HiSeq 4000 to generate 150 bp paired-end reads.
Bioinformatics Analysis
Characterization of Micro-Eukaryotes (18S rRNA Gene) and Bacteria (16S rRNA Gene)
The 18S and 16S small subunit (SSU) rRNA genes were characterized with the program phyloFlash 3.4, which reconstructs SSU rRNAs from metagenomic data by mapping short reads against a modified version of the SILVA SSU 128 99 NR database and assembles the mapped reads with SPAdes (Gruber-Vodicka et al., 2020). This program aligns and assembles longer sequences compared to the 16S rRNA gene sequences that were used previously (Rosales et al., 2020), thus potentially allowing for additional identification of bacterial taxa. The count tables and taxa assignments generated by phyloFlash (Rosales, 2021) were aggregated by the lowest taxonomic assignments and were then tested for significance using the R program Analysis of Compositions of Microbiomes with Bias Correction (ANCOMBC; Lin and Peddada, 2020). The p-values were corrected with a false discovery rate and were considered significant if adjusted p-values (padj) ≤ 0.05 and had a cutoff value of 0.9.
Microbial Functional Profiling of Metagenomes
Raw reads were quality-score filtered, trimmed, adapters removed, entropy-filtered (entropy = 0.3), and PhiX reads removed with the program BBDuk 38.84. Quality filtered reads were taxonomy characterized with Kaiju 1.8.2 (Mirdita et al., 2021) and were selected for further analysis. To normalize for the larger number of bacteria reads in DL SINT (see section “Results”) bacterial reads were subsampled to the lowest number of bacteria reads found in a sample (77,550) and were then functionally characterized with HUMAnN3 3.0.0 (Franzosa et al., 2018). The reads were first aligned with the program MetaPhlan2 to the ChocoPhlAn database (Truong et al., 2015). The reads that did not align were then translated and annotated to the UniRef50 protein database and were used to reconstruct MetaCyc pathways (Caspi et al., 2020). The output abundance tables were in Reads Per Kilobase of transcript and were normalized using the relative abundance function.
Metagenome-Assembled Genome Generation
After quality control, the data were normalized with BBNorm 38.84 to down-sample reads found at high depths, to make the sequence coverage more even within each sample, and improve microbial contig assembly. For each sample, the normalized reads were assembled independently with MEGAHIT 1.2.9 (Li et al., 2016). Each assembly was then binned individually using MaxBin 2.0 2.2.6 (Wu et al., 2016). The metagenome-assembled genomes (MAGs) were then de-replicated and representative MAGs were selected with the program dRep 2.4 (Olm et al., 2017). The quality of MAGs was assessed using CheckM 1.0.13 (Parks et al., 2015) and moderate to high-quality genomes (those with at least 69% completion and <10% contamination) were selected for further analysis. The remaining MAGs were compared to the non-redundant NCBI database using BLASTn 2.5.0+ (Altschul et al., 1990) to identify eukaryotic sequences, which were then filtered from the assemblies. RefineM 0.1.2 (Parks et al., 2017) was also used to remove contigs by characterizing contigs that diverged from genomic properties within each MAG, such as G + C content, genome coverage, and tetranucleotide frequencies. Further screening of bins was conducted with CheckM’s SSU Finder to identify 16S rRNA genes. The 16S rRNA genes that did not match the taxonomic identification of the bins (see next section) were removed. CheckM was run again to obtain the quality and completion of the final bins.
Taxonomic Characterization of Metagenome-Assembled Genomes
Taxonomy was assigned to each bin by using the database Genome Taxonomy Database (GTDB) (Parks et al., 2020) with the program Genome Taxonomy Database Toolkit (GTDB-Tk 1.4.1) (Parks et al., 2018; Chaumeil et al., 2019). Using the R program phangorn 2.6.3 (Schliep, 2011), a subset of the tree constructed by GTDB-Tk was extracted by randomly sub-setting one sibling and one first cousin (if present) from each MAG. In addition, if MAGs had an accompanying 16S rRNA gene sequence, these were compared to the non-redundant BLASTn database online.
Functional Annotation of Metagenome-Assembled Genomes
To annotate the potential function of each MAG, the program Distilled and Refined Annotation of Metabolism (DRAM) was used (Shaffer et al., 2020). DRAM uses Prodigal (with an anonymous metagenome setting) to find open reading frames (ORFs) and translates contigs. Each ORF is then searched against five databases (KOfam, UniRef90, Pfam, CAZY, MEROPS) using MMSeq2 (Mirdita et al., 2021). The outputs are tables with genes assigned to specific metabolic functions and the number of reads that were assigned to each function. In addition, pathways of metabolism completion are also provided based on the database structure of Kyoto Encyclopedia of Genes and Genomes (KEGG). The presence or absence of a function is based on the presence of specific key genes present. Given that the KEGG database was not used as one of the DRAM databases, we also used the translated sequences from DRAM and annotated these sequences with the KEGG Automatic Annotation Server using GHOSTx with a single-directional best hit mode (Kanehisa et al., 2016). The KEGG output was then parsed and evaluated for metabolic completeness using the KEGG-Decoder 1.2.1 program. Orthologous genes across all MAGs were also identified with OrthoFinder 2.5.2 (Emms and Kelly, 2019) to identify common and unique orthologous groups from pathways associated with SCTLD. Orthologous genes found across the same orders were then annotated with GhostKOALA (Kanehisa et al., 2016) and KEGG numbers were assigned to each MAG. Shared KEGG pathways were plotted on an alluvial plot, but metabolic pathway categories were not included as they were examined with previous methods. The orthologous genes from Rhodobacterales that were only found in a single MAG and had the largest gene group were compared to the non-redundant BLASTp database using the online interface.
Coverage and Differential Abundance of Metagenome-Assembled Genomes
CoverM was used to generate the coverage of each gene, which was normalized by metagenome library size using the trimmed mean function (settings: –trim-min = 0.1 and –trim-max = 0.9). In addition, to identify significant genes from MAGs, reads from each of the 58-quality controlled (post-BBduk; not normalized reads) samples were aligned using BBmap 38.84 to the genes file generated by DRAM (settings: minid = 0.98, ambiguous = toss, and pairedonly = true). BBmap can internally call the program pileup, which was used to count mapped reads. To test MAG gene differences, only AH SINT and DL SINT were compared, because all MAGs originated from DL SINT (see section “Results”); this also simplified the statistical model. The count values were filtered to remove those at low abundance across samples (sum < 4 across 40% of samples) and then were normalized using the trimmed mean M-values function from edgeR (Robinson et al., 2010). To test for differential abundance, the exactTest function from edgeR 3.32.1 was used and p-values were adjusted with the Benjamini–Hochberg method. The results were considered significant if the padj ≤ 0.05.
Results
We collected samples of apparently healthy (AH) coral tissue, unaffected tissue (DU) from diseased corals, and lesion tissue (DL) from diseased corals from the following coral species: Stephanocoenia intersepta (SINT), Diploria labyrinthiformis (DLAB), Dichocoenia stokesii (DSTO), and Meandrina meandrites (MMEA). Of these species and tissue combinations, prior to quality control, the highest number of reads was found in DL DSTO (51 million [m] reads) and the lowest was in DL MMEA and DL SINT (38 m each). On average, AH libraries had 39 m reads, DU had 41 m reads, and DL had 42 m reads. After quality control, AH libraries had 32 m reads, DU had 34 m reads, and DL had 35 m reads. Once the data were normalized, AH had 24 m reads, and DU and DL had 25 m reads each. The coral species with the highest reads prior to quality control was DSTO (43 m), followed by DLAB (40 m), MMEA (40 m), and finally SINT (39 m). There was, on average, an 8% decrease of reads after quality control: DSTO still had the most reads after quality control (35 m), followed by DLAB (34 m), MMEA (33), and then SINT (33 m). After normalization, DSTO, DLAB, and MMEA all had a similar number of reads (25 m) but SINT had a comparatively lower number of reads (22.9 m); overall, there was an ∼60% decline of sequences from raw reads to normalized reads. The details for each library are found in Supplementary File 1.
Micro-Eukaryotes (18S rRNA) and Bacteria (16S rRNA) Associated With Stony Coral Tissue Loss Disease
In total, 482,981 reads were recruited to the 18S rRNA gene, with 135,518 to AH, 177,355 to DU, and 170,108 to DL. The coral DLAB had the highest number of reads (243,160) and DSTO had the lowest (61,504). The reads from the eukaryotic community were relatively more abundant than reads from the prokaryotic community (Supplementary Figure 1). Across samples, on average, 80.8% of the reads were from the eukaryotic community and accounted for up to 99.1% of reads (found in DU DLAB). The smallest proportion of eukaryotic sequences was from DL SINT samples, which on average had 32.7% eukaryotic reads (Supplementary Figure 1). From the differential abundance analysis, the 18S rRNA gene data resulted in a total of 14 differentially abundant taxa among the three tissue types (Figure 1 and Supplementary File 2).
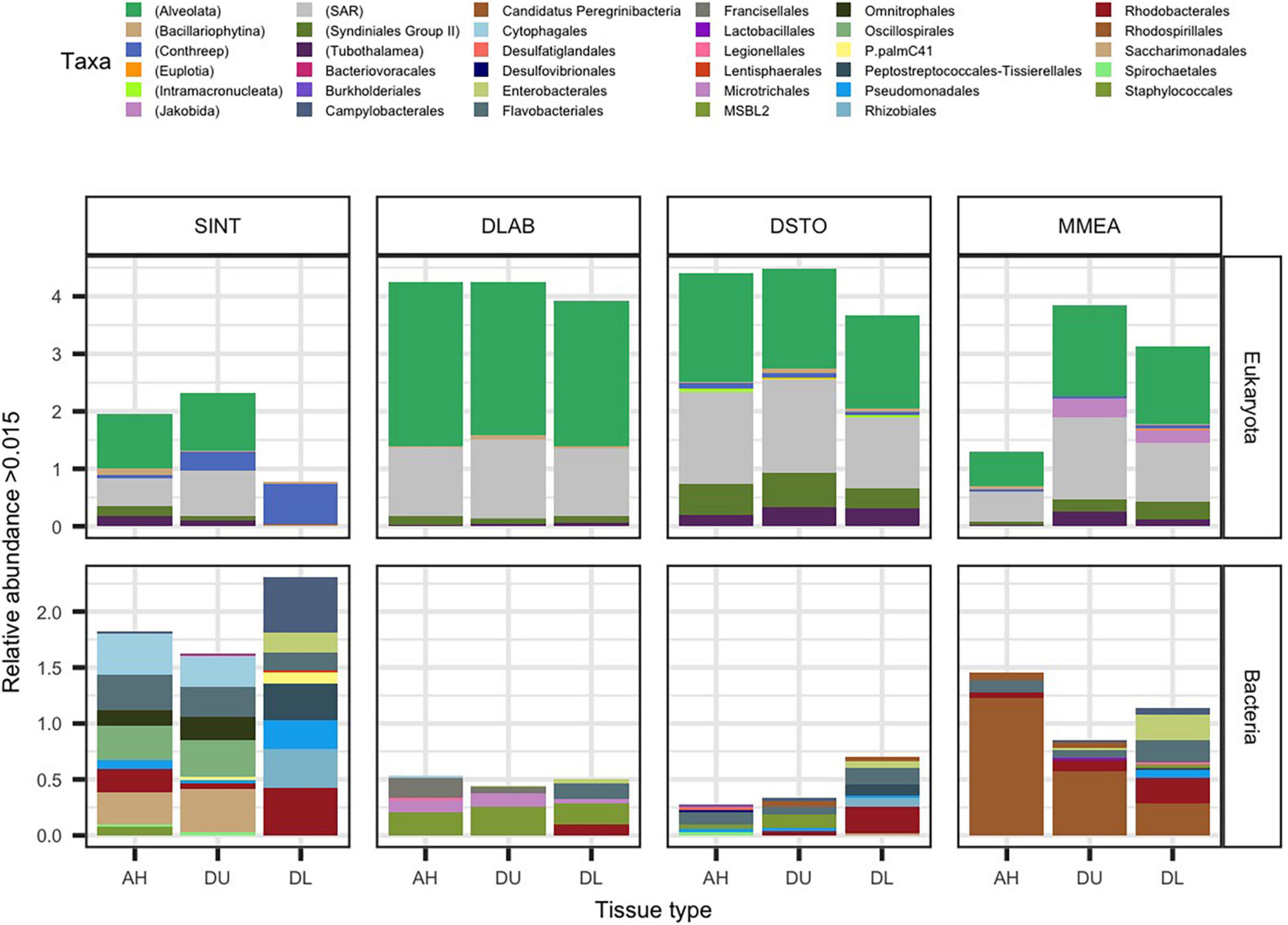
Figure 1. The average relative abundance of significantly differentially abundant rRNA gene counts (padj ≤ 0.05 from ANCOMBC). Samples from apparently healthy colonies (AH), and unaffected (DU) and lesion (DL) tissues on diseased colonies, of the coral species Stephanocoenia intersepta (SINT), Diploria labyrinthiformis (DLAB), Dichocoenia stokesii (DSTO), and Meandrina meandrites (MMEA) were evaluated for micro-eukaryotic (18S rRNA; top panel) and bacterial (16S rRNA; bottom panel) for differential abundance. The most abundant significant taxa are plotted by relative abundance. The stacked bar is colored either by the lowest taxonomic designation for eukaryotes (denoted by parenthesis in the key) or order for bacteria.
In AH tissue, there was no significant log-fold change of any of the micro-eukaryotes when compared to DU or DL tissue (Figure 1). In DU tissue, the highest log-fold change when compared to AH samples were the free-living eukaryotes Jakobida (0.29) driven by MMEA samples, the protists superclade CONthreeP (0.11; phylum Ciliophora) driven by SINT samples, and the ciliates class Phyllopharyngea (0.09; phylum Ciliophora) driven by DLAB samples. In DL tissue, the top four highest log-fold changes compared to AH samples were all part of CONthreeP: Prostomatea (1.4) and Phyllopharyngea (1.4), which were enriched in all coral species, Oligohymenophorea (1.3) driven by SINT, DSTO, and MMEA, and CONthreeP (1.3) driven by SINT alone. The taxa Euplotia (order Ciliophora) and a CONthreeP were the only differentially abundant taxa that were absent in AH (Figure 1 and Supplementary File 2).
The bacterial community, on average, had a smaller proportion of reads (19.2%) compared to eukaryotes within the sampled coral tissue. The highest proportion of bacteria sequences was from DL SINT (67.3%) and the lowest was from DU DLAB (0.9%). In total 77,406 sequences aligned to the 16S rRNA gene, with 4,023 to AH, 3,973 to DU, and 69,410 to DL. The highest number of bacterial reads was found in SINT (69,969) and the lowest was in DSTO (1,830; Supplementary Figure 1). When the four coral species were pooled together, 135 microbial taxa were differentially abundant among the three tissue types (Figure 1 and Supplementary File 2). The 16S rRNA counts used in the present study identified 41 more differently abundant taxa than previously described for these same samples (Rosales et al., 2020).
When comparing AH coral tissue to DU tissue, the bacteria taxon within the family Terasakiellaceae (order Rhodospirillales) had the highest log-fold change (–0.90) in AH; this taxon was more abundant in AH tissues than in DU tissues, but this was driven by AH samples from MMEA. When comparing AH to DL tissue, the highest log-fold change (–0.50) in AH was for Cyclobacteriaceae (order Cytophagales), driven by AH samples from SINT (Figure 1 and Supplementary File 2).
In DU tissue compared to AH tissue, the highest log-fold changes of bacteria taxa in DU were found in the phylum Lentisphaeria (0.11; order Lentisphaeraceae), Sulfurimonas (order Campylobacterales; 0.10), and Thalassobius mediterraneus (order Rhodobacterales; 0.10). In DL tissue compared to AH tissue, the bacteria with the largest fold changes in DL were Rhodobacteraceae (order Rhodobacterales; 1.5) enriched in lesions of all coral species, Cohaesibacter (order Rhizobiales; 1.3) driven by SINT, DLAB, and DSTO, Fusibacter (order Peptostreptococcales-Tissierellales; 1.3) driven by SINT and DSTO, and Arcobacteraceae (order Campylobacterales; 1.1) driven by SINT (Figure 1 and Supplementary File 2).
There Was a Relatively Higher Number of Microbial Functional Genes in Diseased Corals
From the full metagenomes (unassembled reads) there was a total of 159 functional pathways identified by HUMAnN 3, which were then agglomerated by pathway name (n = 81; Figure 2). The total number of pathways was higher in DL samples (n = 75), followed by DU (n = 20), and lowest in AH (n = 11). The PWY-3781: aerobic respiration I (cytochrome c) had the highest mean relative abundance across the data and was highest in DU (2.3e–03) samples followed by DL (2.1e–03) and AH (6.0e–04). The second most abundant pathways, PWY-7220: Adenosine deoxyribonucleotides de novo biosynthesis II, and PWY-7222: Guanosine deoxyribonucleotides de novo biosynthesis II, were also at higher relative mean abundances in DU (1.0e–03) and DL (5.0e–04) compared to AH (4.4e–04).
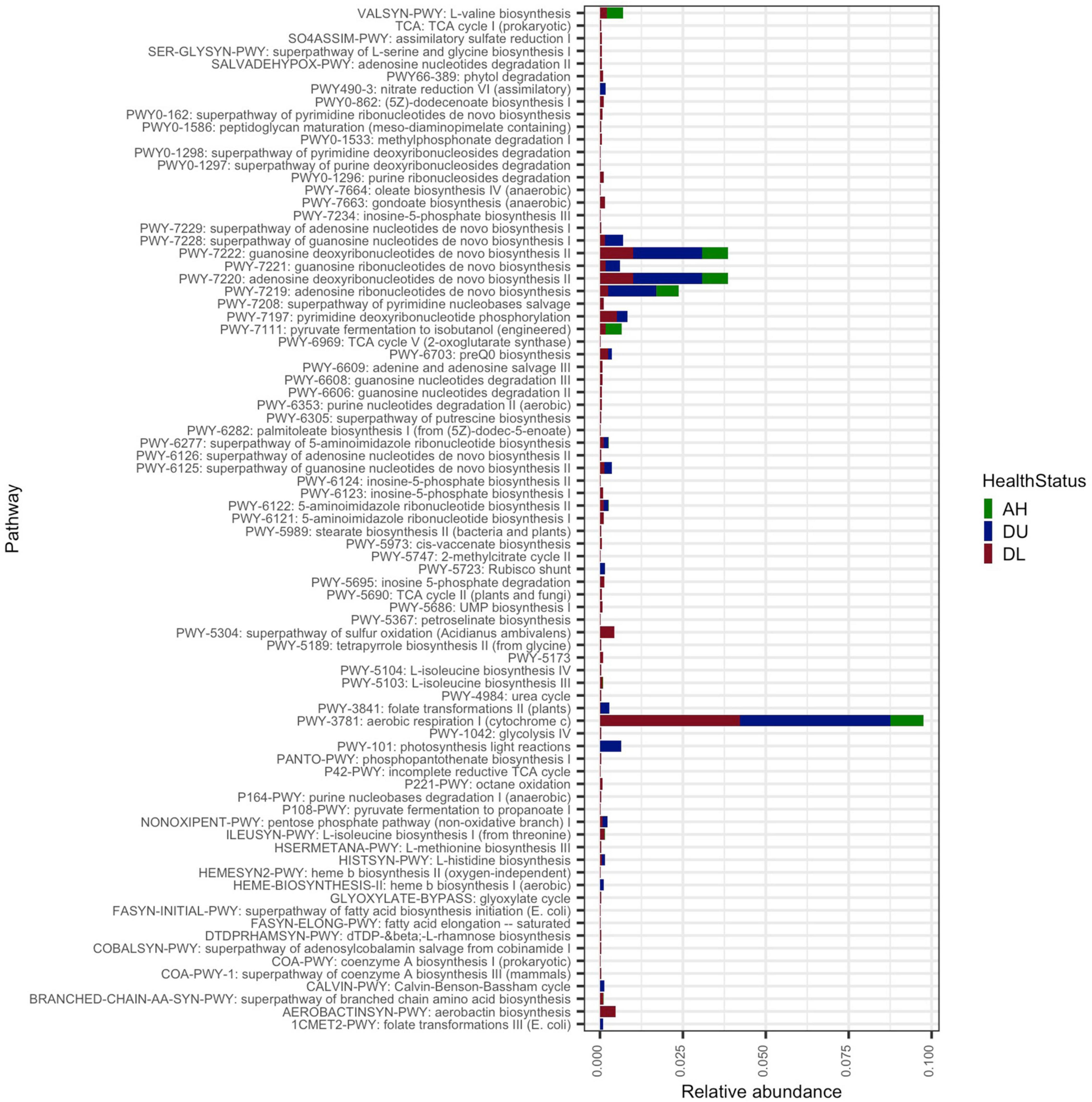
Figure 2. The average relative abundance of functional pathways. All coral samples from apparently healthy colonies (AH), and unaffected (DU) and lesion (DL) tissues on diseased colonies.
Phylogenetic Characterization of Metagenome-Assembled Genomes
Contigs were constructed from each sample and, on average, the highest number of contigs were in AH (555,142; N50 = 91,460) and the lowest in DU (479,713; N50 = 77,890; Supplementary File 1). Of the coral species, SINT had the highest average number of contigs (767,960; N50 = 164,939) and DLAB had the lowest (382,729; N50 = 48,285). The contigs were binned individually for each sample and 312 bins were generated across the 58 samples. There were only two bins associated with 51 of the samples, but for the five DL SINT samples, there were between 15 and 78 bins per sample (Supplementary File 2). After dereplication, 17 metagenome-assembled genomes (MAGs) were selected for further analysis based on >69% genome completion and <10% contamination. After further quality assessment of each MAG with refineM, CheckM, and BLASTn, 16 bins passed quality control and were selected for the remainder of the analysis with MAGs between 51.72 and 99.87% (median = 93.4%) genome completion and <7% (median 3.3%) contamination. Of note, all MAGs were unexpectedly generated from only the five DL SINT samples. Details of each MAG (bin) are presented in Supplementary File 3.
Phylogenetic analysis showed that the 16 MAGs spanned three phyla (Proteobacteria, Bacteroidota, and Campylobacterota) and seven orders (Pseudomonadales, Beggiatoales, Rhodobacterales, Rhizobiales, Rs-D84, Flavobacteriales, and Campylobacterales; Figure 3). With a minimum alignment fraction of 0.65, MAGs were not assigned an average nucleotide identity (ANI) value to any species in the Genome Taxonomy Database (GTDB). While one MAG could only be characterized to order (6.25%), others were further characterized to the family (25%; n = 4) and genus (69%; n = 11) levels. The three MAGs from the order Rhizobiales were characterized within the genus Cohaesibacter and two Pseudomonadales were classified as the same genus: UBA_1124; all other MAGs had unique taxonomic designations. Only five MAGs (31%; bins 12, 18, 9, 8, and 43.014) had 16S rRNA genes assembled with MEGAHIT. The gene sequences with the highest similarities to sequences in the database were for bins 12 (Beggiatoales; Beggiabaceae), which was similar to sequences from a study on black band disease (BBD; EU019373.1; max score = 2,181; Sekar et al., 2009); for bin 18 (Flavobacteriales; Flavobacteriaceae), which was similar to a sequence from a white syndrome study (EU780277.1; max score = 1,223); for bin 8 (Rs-D84), which was similar to a sequence from a crude oil study (CU915025.1; max score = 1,373; Zrafi-Nouira et al., 2009); and bins 9 (Rhodobacterales; Mangrovicoccus) and 43.014 (Campylobacterales; SZUA.545), which were similar to sequences from white plague disease studies (FJ203197.1; max score = 1,657, and FJ202415; max score = 784; Sunagawa et al., 2009).
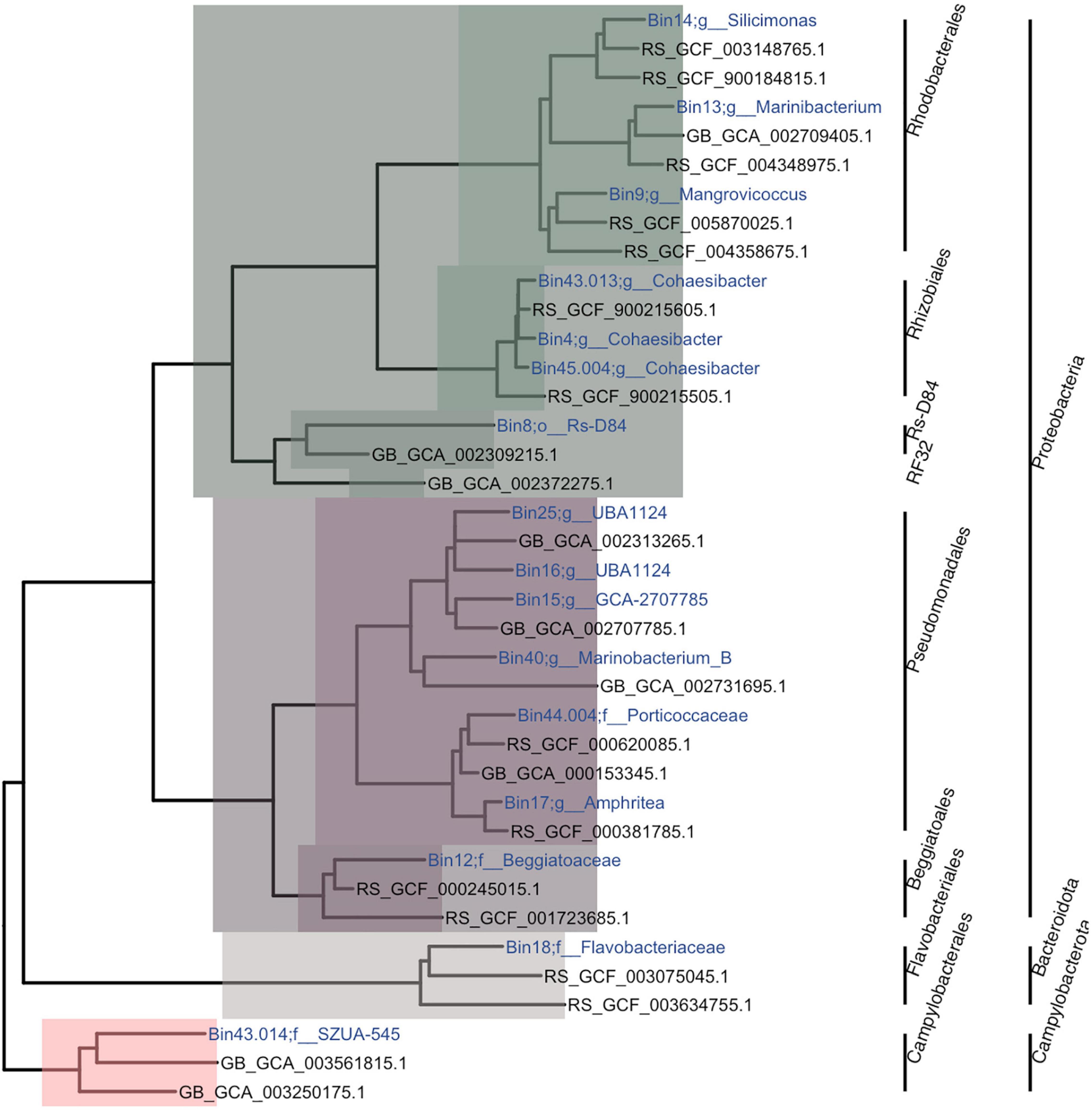
Figure 3. Phylogenetic tree of metagenome-assembled genomes (MAGs). The tree is a subtree from the Genome Taxonomy Database Toolkit (GTDB-Tk). For each MAG, one sibling and one first cousin, if they existed, were randomly chosen to be plotted. The colors represent the classes Alphaproteobacteria (green), Gammaproteobacteria (purple), Flavobacteria (gray), and Campylobacterales (salmon). Within each class, the different color tones represent different clades and bacterial orders. The name of each MAG was assigned based on bin number and the lowest taxonomic assignment designated.
Potential Metabolism of Metagenome-Assembled Genomes
Metagenome-assembled genomes showed a diversity of methods for glycolysis (Figure 4; top bracket of heatmap) and other metabolic pathways (Supplementary Figure 2). The two most prevalent pathways that were also complete across MAGs were the glyoxylate cycle (in bins 8 [Rhodobacterales; Rs-D84], 12 [Beggiatoales; Beggiabaceae], 14 [Rhodobacterales; Silicimonas], and 004 [Pseudomonadales; Porticoccaceae]), and the citrate cycle (in bins 16 [Pseudomonadales; UBA1124], 18 [Flavobacteriales; Flavobacteriaceae], 13 [Rhodobacterales; Marinibacterium], and 004). All other MAGs had a partially completed glyoxylate cycle (40–80%) and citrate cycle (37.5–87.5%) except bins 40 (Pseudomonadales; Marinobacterium) and 4 (Rhizobiales; Cohaesibacter), where they were absent. Another completed pathway was the pentose phosphate pathway, but it was only fully present in bin 18 and ranged in completeness from 42.9 to 71.4% in other MAGs. Also, the Entner–Doudoroff pathway was completed in bin 16 but was found at lower completeness rates in all other MAGs (0–75%). Another glycolysis pathway, the Embden–Meyerhof Pathway, was found in all MAGs at a range between 56 and 78% completeness.
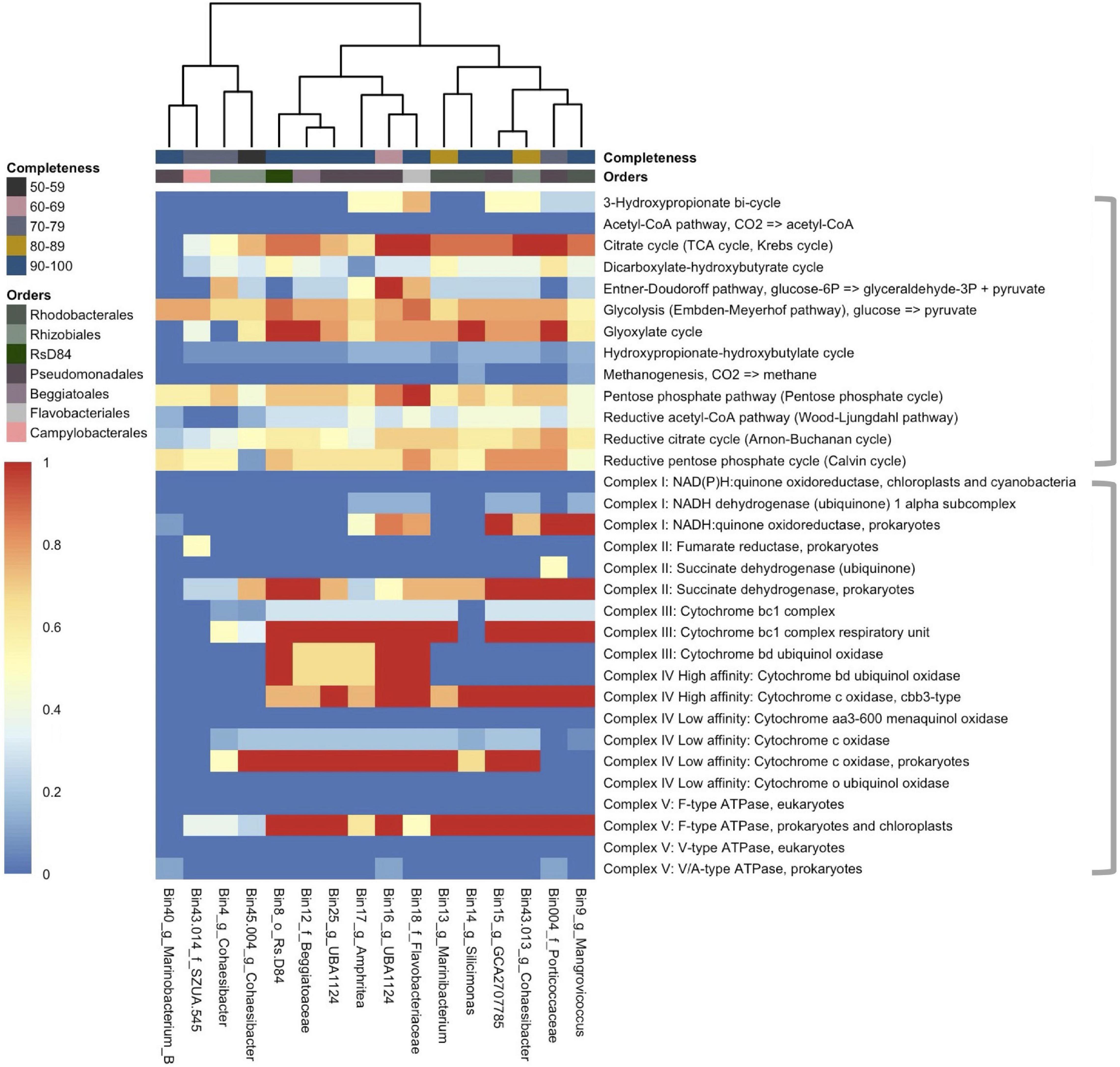
Figure 4. The coverage of common metabolic pathways and electron transport chain complexes across 16 metagenome-assembled genomes (MAGs). The heatmap represents the completeness of each module with “1” (red) indicating a complete module and “0” (deep blue) indicating that the module is not present. The heatmap is split by gray brackets. The top bracket encompasses major metabolic functions and the bottom brackets encompass electron transport chain complexes. The MAGs (x-axis) are labeled by bin number and the lowest taxonomic assignment designated. The x-axis is hierarchically clustered by Euclidean distance among the pathways present in each MAG (bottom) and colored (as in Figure 3) by the respective bacterial order and by genome completeness of the MAGs (top).
The potential of electron transport usage of each MAG was also evaluated (Figure 4; bottom bracket of heatmap). The two most prevalent pathways were Complex II: Succinate dehydrogenase for prokaryotes (25–100% completeness), and Complex V: F-type ATPase for prokaryotes and chloroplasts (25–100%), which were both found in all bins except bin 40. The key genes necessary (1–2 genes) to carry other forms of metabolism were also evaluated (Supplementary Figure 2). Nitrogen metabolism was found in all but three MAGs (bins 40, 43.014 [Campylobacterales; SZUA.545], and 45.004 [Rhizobiales; Cohaesibacter]) with nitrate to nitric oxide being the more prevalent pathway across MAGs (11/16 bins). Thiosulfate oxidation by SOX (SRY box) complex was the most prevalent sulfur metabolic pathway and it was found in 7/16 bins.
Potential Transporters of Metagenome-Assembled Genomes
Transporter genes from 5/7 Alphaproteobacteria formed a cluster that showed a higher abundance of transporter genes compared to all other MAG clusters (Figure 5). In this cluster, which consisted of the bacterial orders Rhodobacterales and Rhizobiales, the most abundant transporter was for the peptides/nickel system (read count = 350). This was followed by the branched-chain amino acid transport system (read count = 137) and the iron complex transport system (read count = 129), which all had the highest frequency in the Alphaproteobacteria cluster. A putative ATP binding cassette (ABC) transport system was the fourth most abundant transporter (read count = 118), and genes for this transporter were found across all MAGs.
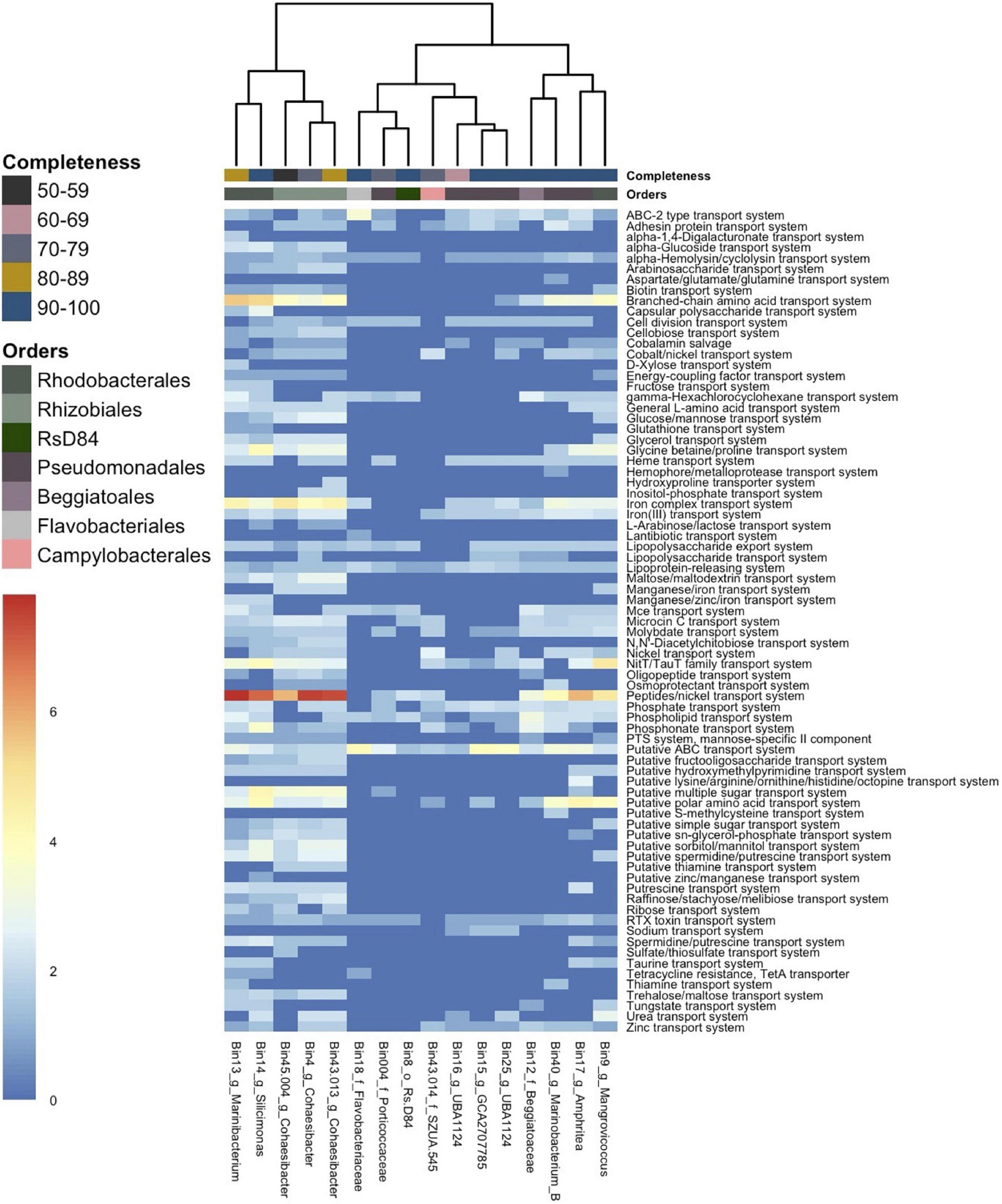
Figure 5. Gene counts of transporter genes across 16 metagenome-assembled genomes (MAGs). The heatmap shows the number of gene counts (square root transformed) found in each MAG from Distilled and Refined Annotation of Metabolism (DRAM) analysis. The MAGs (x-axis) are labeled by bin number and the lowest taxonomic assignment designated. The x-axis is hierarchically clustered by Euclidean distance among genes present in each MAG (bottom) and colored (as in Figure 3) by the respective bacteria order (top) and by genome completeness of the MAGs (top).
Orthologous Genes, Genes, and Pathways in Metagenome-Assembled Genomes Associated With Pathogenesis
To look for orthologous genes commonly associated with pathogens, the Kyoto Encyclopedia of Genes and Genomes (KEGG) analysis was focused on pathways such as chemotaxis, secretion systems, antibiotic resistance, biofilm formation, and flagellar assembly (Supplementary Figure 3). Although orthologous genes associated with pathogenesis were found (Supplementary Figure 3), the completion (Supplementary Figure 4) and the numbers of sequences that aligned (Supplementary Figure 5) to these pathways varied for each MAG. For chemotaxis pathways, there were no orthologous genes shared across all MAGs but genes for chemotaxis were present in the orders Campylobacterales (n = 3), Rhizobiales (n = 2), and Rhodobacterales (n = 1; Supplementary Figure 3). However, chemotaxis pathways (non-orthologous genes) were present (12–88% complete) in all but three MAGs (bin 004, Pseudomonadales; bin 13, Rhodobacterales, and bin 8, Rs -D84) and were almost complete (88%) in three Pseudomonadales (bins 15, 17, and 40) and Campylobacterales (bin 43.014). Bacterial secretion system orthologous genes were shared across all MAGs and Rhizobiales was the only order with unique orthologous genes (Supplementary Figure 3). The type I secretion system was the most commonly found secretion system and was 99% complete in bin 17 (Pseudomonadales; Amphritea), but in all other MAGs was only 33% complete (Supplementary Figure 4). Orthologous genes for beta-lactam resistance pathways were present in all MAGs and unique orthologous genes were present in Rhizobiales (n = 2) and Rhodobacterales (n = 1; Supplementary Figure 3), but in general there was a low abundance of reads that aligned to antibiotic resistance genes (Supplementary Figure 5). Biofilm formation orthologous genes were found in Pseudomonadales (n = 2), Campylobacterales (n = 1), Rhizobiales (n = 1), Rhodobacterales (n = 1), and were shared across all MAGs (n = 8; Supplementary Figure 3), but key biofilm pathways selected by KEGG-Decoder such as biofilm regulator BssS (only 25% in bin 14, Rhodobacterales), biofilm PGA Synthesis protein, and colanic acid and biofilm transcriptional regulator were absent (Supplementary Figure 4). However, all MAGs had orthologous genes for flagellar assembly and quorum sensing, which are part of the biofilm formation pathway. Specifically, Pseudomonadales (bins 15, and 17) had the highest pathway completion for flagellum (87%; Supplementary Figure 4).
The order Rhizobiales shared the most orthologous genes (n = 263) compared to all other groups. This is despite bin 45.004 having the lowest genome completion (51.72%; Supplementary File 3), but this aligns with the phylogenetic tree result, which shows a shared clade and short branch lengths among Rhizobiales (Figure 3). The order Rhodobacterales shared the second-highest number of orthologs (n = 163) and was followed by the intersection of all MAGs, which shared 45 orthologous genes, possibly representing common genes important for SCTLD progression. The three Rhizobiales did not show any unique orthologous genes within each MAG, but the three Rhodobacterales MAGs each had unique orthologous genes (Supplementary Figure 3). For Rhodobacterales, the largest unique gene group belonged to an FG-GAP (phenyl-alanyl-glycyl [FG] and glycyl-alanyl-prolyl [GAP]) repeat protein in bin 9, a Hint domain-containing protein in bin 13, and a VPLPA-CTERM sorting domain-containing protein in bin 14.
Coverage and Differentially Abundant Genes in Metagenome-Assembled Genomes
All MAGs had a higher coverage in DL compared to DU and AH samples (Supplementary File 4), which was driven by SINT samples. There were three non-DL SINT samples that had coverage for one MAG each: one DL MMEA had coverage in bin 17 (Pseudomonadales; Amphritea), one DL DSTO had coverage in bin 45.004 (genus Cohaesibacter), and one DL DLAB had coverage in bin 13 (genus Marinibacterium). Bin 45.004 (genus Cohaesibacter) was present in all SINT samples (AH, DU, and DL), but at higher coverage rates in DL. All other bins were absent in all AH and DU samples. Since bins were most abundant in DL SINT, we focused on evaluating the prevalence of bins across the five SINT samples (Figure 6A). Bin 004 (family Porticoccaceae) was the least prevalent, found only in one sample, and bin 17 was prevalent in all five samples and had the highest coverage across the five samples. Out of the 16 bins, 11 (69%) were present in all five DL SINT samples.
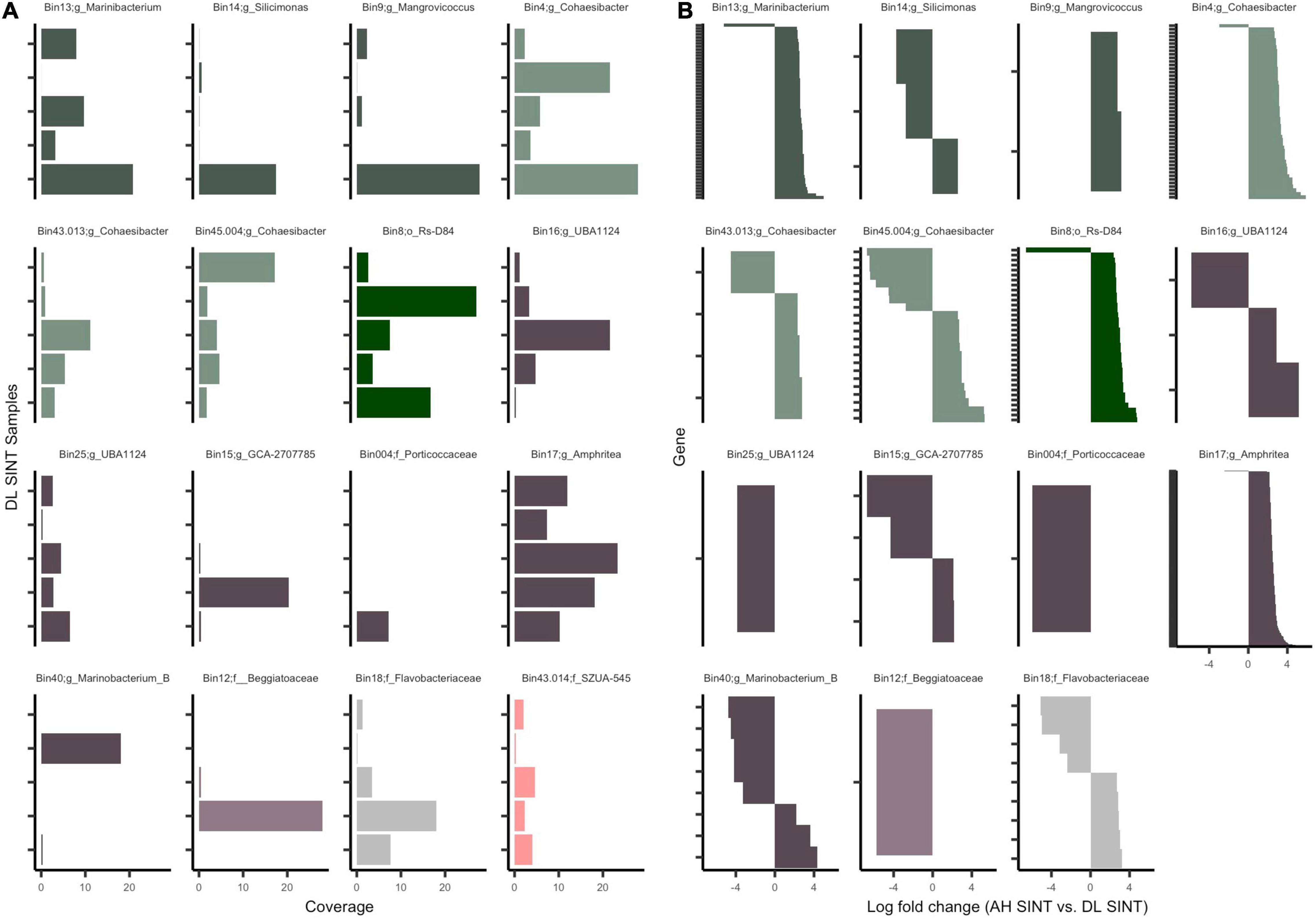
Figure 6. Mapped reads to the open reading frames (ORFs) of metagenome-assembled genomes (MAGs). (A) Coverage of ORFs in the five-disease lesion (DL) samples from Stephanocoenia intersepta (SINT). (B) Significantly differentially abundant genes (padj ≤ 0.05 from edgeR analysis) among apparently healthy (AH) SINT and DL SINT coral samples. Gene sets (y-axis) are parsed by MAGs and show the log-fold change between AH SINT and DL SINT. Each bin color represents bacterial classes: Gammaproteobacteria (purple), Alphaproteobacteria (green), and Flavobacteria (gray). Within each class, the different color tones represent different clades and bacterial orders.
Across the 58 samples, on average a total of 219,842 reads mapped per sample to the 16 MAGs. The highest number of reads mapped (1,186,815–3,393,946) to DL SINT. The lowest number of reads mapped (1,812–8,462) to three AH samples and one DU sample from the coral DLAB. Given the higher recruitment of sequences to SINT, only AH and DL SINT corals were further evaluated. Among the MAGs there were 48,283 genes; after filtration, only 8,278 were tested for differential abundance, and of those, 444 were found to be significantly differentially abundant between SINT AH and DL samples (Figure 6B and Supplementary File 5). In total, DL SINT had 414 enriched genes and AH SINT had 30 enriched genes. Bin 43.013 had the most genes enriched in AH (n = 9) and was followed by bin 40 (n = 5). Bin 17 had the most gene enrichment in DL (n = 244) and was followed by bin 13 (n = 58).
Discussion
In this study, we evaluated 58 metagenomes to understand the microbial community and function of SCTLD by looking at three coral tissue sample types (apparently healthy [AH], unaffected tissue on diseased corals [DU], and lesion tissue [DL] on diseased corals) collected from four coral species: Stephanocoenia intersepta (SINT), Diploria labyrinthiformis (DLAB), Dichocoenia stokesii (DSTO), and Meandrina meandrites (MMEA). We found that only the five DL tissue samples originating from SINT had a large proportion of bacterial sequences and most of our results were driven by these five samples. In spite of this, we were able to characterize the micro-eukaryote community and the metabolic functions of the metagenomic libraries, and assemble and characterize 16 MAGs. Here, we found that ciliates are a part of SCTLD dynamics, but further research is needed to understand their etiological role. There also was a higher number of metabolic functional genes within samples taken from corals showing signs of SCTLD, which may be tied to bacteria proliferation and antibiotic resistance. Finally, we documented the presence of virulent factor genes that may be associated with SCTLD lesion progression and may be targeted to treat SCTLD.
Ciliophora Is Associated With Stony Coral Tissue Loss Disease
In our micro-eukaryotes analysis, we found a diversity of Ciliophora across all tissue types, but some ciliates were specifically associated with diseased corals, which is consistent with SCTLD histological findings (Landsberg et al., 2020; Eaton et al., 2021). Unlike histological findings, we were able to genetically characterize the taxa enriched in DL compared to AH corals and found Euplotia and a CONthreeP (the protists superclade) were present in SCTLD lesions and absent in AH (Figure 1; top panel). Euplotia is a diverse and ubiquitous ciliate in aquatic ecosystems (Syberg-Olsen et al., 2016) and has also been associated with white syndrome and brown band disease (Sweet and Séré, 2016). CONthreeP, on the other hand, has not, to our knowledge, been previously associated with coral disease, but its sequences have been found in the guts of reef fish (Shraim et al., 2017). Thus, while there have been histological reports of ciliates in SCTLD, this is the first study to document their genetics, which helps to understand the players involved in SCTLD dynamics. While the role of Ciliophora in SCTLD is unknown, they may function to feed on bacteria, necrotic tissue, other protists, and potentially symbiotic algae (Sweet and Séré, 2016).
The Bacteria Taxa Found in Metagenomes Are Largely Members Previously Associated With Stony Coral Tissue Loss Disease
The 16S rRNA results presented here using metagenomics (Figure 1) are comparable to previous work on the same samples that were used in 16S rRNA amplicon sequencing (Rosales et al., 2020). In the present study, the taxa that were significantly more enriched in DL and DU samples compared to AH were from the orders Rhodobacterales, Rhizobiales, Campylobacterales, and Peptostreptococcales-Tissierellales. Similar to our previous results for DL tissue (Rosales et al., 2020), Campylobacterales was only present in SINT corals, and Rhodobacterales was present at high abundances in all DL samples (Figure 1; bottom panel). Rhizobiales were previously found in the DL tissue of all four species (Rosales et al., 2020), and here we identified it in all species except MMEA (Supplementary File 2). However, the genus Cohaesibacter, which was significantly more prevalent in DL in the current study (Figure 1), was mostly absent in MMEA in our previous work (only one MMEA sample had Cohaesibacter; Rosales et al., 2020). Thus, it appears that although Rhizobiales may be an important signature of SCTLD lesion tissues overall, the DL tissues of MMEA were characterized by Rhizobiales of a different genus than the DL tissues of the other three coral species - characterized mostly by Cohaesibacter. Among this sample set, this result may have been influenced by the different timing (April) and habitat (forereef) of the MMEA samples compared to the others (sampled in June from patch reefs). Peptostreptococcales-Tissierellales (Fusibacter) was not detected in our previous analysis but was detected in another SCTLD study (Meyer et al., 2019). While the order Rhodospirillales was the most abundant taxon in AH samples, it was driven by the microbiome of MMEA, for which Rhodospirillales was identified as the dominant community member (Figure 1; Rosales et al., 2020).
From the 16 MAGs identified (Figure 3), 50% matched bacterial orders that were significantly differentially abundant between tissue types in our previous work (Rosales et al., 2020) and in the 16S rRNA analysis from this study (Figure 1). These include higher abundances of the orders Campylobacterales, Rhizobiales, and Rhodobacterales within diseased colony tissue compared with healthy coral tissue. In addition, Flavobacteriales and Pseudomonadales were found at higher relative abundances within diseased samples compared with AH samples in the 16S rRNA analysis in this study (Figure 1). Three of the orders identified (Pseudomonadales, Beggiatoales, and Rs-D84) have not been associated with SCTLD. The additional bacterial orders identified may be due to all of our MAGs originating from DL SINT tissues; to our knowledge, this is the only sample set for SCTLD microbial analysis to date that includes this coral species.
All of the MAGs identified in this study originated from DL SINT colonies and it is likely that SINT has a higher bacterial load when showing signs of SCTLD. This result was not due to a difference in sample DNA concentrations, as these values were similar among the 58 samples, or sequencing depth, which, while also similar among the sample set, was lowest within the DL SINT samples (Supplementary File 1). Overall, only 8.6% of our samples generated at least medium-quality MAGs, highlighting the difficulties with assembling bacterial genomes from coral samples, likely because the majority of sequences (>90%) therein are from the coral host and symbiotic algae. As the SINT samples showed no differences in processing and sequencing, we hypothesize that SINT samples just had more bacteria relative to coral tissue and symbiotic algae than the other coral species we sampled; accordingly, SINT had a higher proportion of bacteria sequence reads in the five libraries. Of our study coral species, all are categorized as highly susceptible to SCTLD except SINT. SINT is considered moderately susceptible, and the prevalence of lesions and mortality in SINT colonies are usually lower than the other three study species (Case Definition: Stony Coral Tissue Loss Disease (SCTLD), 2018; Sharp et al., 2020; Combs et al., 2021). We know from our previous study (Rosales et al., 2020) that there were more bacterial taxa enriched in DL tissue of SINT compared to the other corals. We also found bacterial taxa affiliated with diseased corals in AH SINT colonies – and not in AH colonies of the other coral species – in both the epidemic (active SCTLD outbreak) and endemic (post-SCTLD outbreak) zones of SCTLD (but not the vulnerable zone [pre-SCTLD invasion]). We hypothesize that the SINT coral species can tolerate some level of the virulent/potentially pathogenic bacteria associated with SCTLD without succumbing to tissue loss, but large concentrations of these bacteria will initiate lesions, resulting in the bacteria being more enriched in the DL tissues of SINT than they are in the DL tissues of other coral species.
Furthermore, the three Rhodobacterales from this study are currently uncharacterized species and are from three distinct genera (Figure 3 and Supplementary File 3). Although the genomes assembled may be novel, the 16S rRNA gene assembled by MEGAHIT in bin 9 (Rhodobacterales) was similar to a white plague disease study in the Caribbean (Sunagawa et al., 2009), and this bacterium may be a common secondary pathogen or opportunist in coral diseases. Although, the 16S rRNA gene from bin 9 may not provide the specificity necessary to fully support this assumption, since the 16S rRNA gene may not distinguish species or strains; therefore, the Rhodobacterales from bin 9 could be a novel bacteria associated with SCTLD (Escobar-Zepeda et al., 2018). Whole-genome bacteria comparisons across different coral diseases, along with commensal/mutualistic bacteria, are needed to understand the diversity of bacteria in coral pathology. This is especially important in bacteria taxa like Rhodobacterales, which have been implicated in multiple coral diseases (Sunagawa et al., 2009; Pratte and Richardson, 2016).
Also, as previously noted (Rosales et al., 2020), the increased presence of Rhodobacterales in SCTLD seems to be represented by a consortium of this taxonomic group, and the identification here of three phylogenetic groups supports the idea that a diversity of Rhodobacterales may play a role in SCTLD. While there are shared orthologous among this order, each MAG also has a unique set of ortholog genes that may play a role in niche specificity (Supplementary Figure 3). For instance, bin 9 had gene similarities to an FG-GAP repeat protein, which is thought to play a role in cell adhesion and biofilm formation (Fong and Yildiz, 2015) indicating that bin 9 could play a specialized role in microbe-microbe interactions to form biofilms. Furthermore, unlike Rhodobacterales, which were from different bacteria genera, the three Rhizobiales MAGs identified were from the same genus, Cohaesibacter. Our previous study also found multiple members of this genus in these samples (Rosales et al., 2020), suggesting this specific genus could be important in SCTLD. This is further supported by the identification of Cohaesibacter within SCTLD-affected corals in the U.S. Virgin Islands (Becker et al., 2021), indicating some consistency across space and time.
Microbial Metabolism May Switch to Less Energy-Efficient Pathways During Stony Coral Tissue Loss Disease
Overall, there were more metabolic functions characterized in DL samples (Figure 2). Since central metabolism is a global regulator, which can control virulence genes (Ken-ichi et al., 2001), understanding the metabolic potential of SCTLD-associated bacteria can help to understand pathways of virulence and potential virulence mitigation. The Embden–Meyerhof–Parnas (EMP) pathway is a relatively energy-efficient form of carbohydrate degradation (Romano and Conway, 1996) and has a significantly higher gene expression rate in coral-dominated reefs, but unhealthy reefs show higher abundances of Entner–Doudoroff (ED) and pentose phosphate (PP) pathways - less efficient forms of carbohydrate degradation (Haas et al., 2016). In accordance, in DL samples there was a higher relative abundance of the less energy efficient PP pathway (Figure 2). Since a switch in carbon sources can trigger commensal bacteria to become pathogenic ones (Cárdenas et al., 2018), it is probable that this is occurring during SCTLD. Increased carbon availability can cause bacteria to shift to ED and PP pathways and is correlated with increased coral mortality (Kline et al., 2006). The MAGs in this study all have genes involved in the PP pathways and four bins, namely Rhizobiales (bin 4), Pseudomonadales (bins 17 and 16), and Flavobacteriales (bin 18), showed at least 50% completion for the ED pathway (Figure 4). Our metagenome data suggest that MAGs from this study can potentially switch from EMP to PP/ED pathways during SCTLD, which may ultimately lead to an increase in virulence factors.
Diseased Samples Had Higher Relative Abundances of Peptidoglycan and Nucleotide Biosynthesis Pathways
There was also a relatively higher abundance of peptidoglycan maturation and nucleotide biosynthesis pathways in diseased samples (Figure 2). It is probable that more production of nucleic acids and cell wall synthesis is occurring in diseased coral and is leading to more bacteria growth compared to AH. Interestingly, penicillin-binding proteins are part of the peptidoglycan biosynthesis process, which targets beta-lactam antibiotics (Typas et al., 2012). The present study found antibiotic resistance genes in all MAGs (Supplementary Figure 5), which suggests that there is potential for the development of antibiotic resistance in these MAGs. To treat SCTLD, practitioners typically use amoxicillin from the beta-lactam family of antibiotics (Neely et al., 2020, 2021; Walker et al., 2021). We found that all MAGs had orthologous genes specific to beta-lactam resistance, but it is important to note that there were no complete antibiotic resistance pathways found in any MAGs (Supplementary Figure 3). It is possible that some MAGs utilize different beta-lactam resistance pathways. For example, in the MAG gene enrichment analysis (Figure 6B and Supplementary File 5), there was a significant abundance of a metallo-beta-lactamases gene in DL SINT. These enzymes are a growing concern in human medicine because they can be shared via horizontal gene transfer and lack inhibitors (Bebrone, 2007), making the use of amoxicillin a potential concern in coral treatments. A possible alternative to amoxicillin is to instead inhibit nucleotide biosynthesis enzymes. As noted, nucleotide biosynthesis was more abundant in diseased coral (Figure 2), and inhibiting this process may reduce bacterial growth (Samant et al., 2008).
Succinate Dehydrogenase Was Enriched in Stephanocoenia intersepta Lesions
Another potential metabolic enzyme to target for SCTLD mitigation is succinate dehydrogenase/fumarate reductase, which was enriched in DL SINT (Figure 6B and Supplementary File 5). This enzyme is part of the electron transport chain (ETC): Complex II pathway and is sometimes needed for bacterial virulence (Cook et al., 2014). Only Rhodobacterales (bin 9) had a significantly higher gene abundance of this enzyme in DL SINT tissue (Figure 6B and Supplementary File 5), but Complex II: succinate dehydrogenase was found across MAGs except bin 40 (Figure 4). Succinate dehydrogenase oxidizes succinate to fumarate, which results in an electron donation to the ETC, and inhibition of this enzyme can mitigate bacterial diseases in fish (Altinok et al., 2015) and humans (Hartman et al., 2014; Keohane et al., 2018). Thus, the inhibition of this enzyme could also be explored in the field of coral biology for SCTLD mitigation.
Peptides/Nickel Transporters Are Abundant in Rhodobacterales, Rhizobiales, and Pseudomonadales
Within MAGs, we detected a high abundance of the ATP binding cassette (ABC) transporter superfamily (Supplementary Figure 3B), which are ubiquitous in eukaryotes, bacteria, and archaea and are one of the largest families of transporters (Davidson et al., 2008). Of the ABC superfamily, the peptides/nickel transport system had a higher relative abundance in Rhodobacterales and Rhizobiales (Figure 5) and was significantly enriched in Rhodobacterales (bin 13) and Pseudomonadales (bin 17; Figure 6B and Supplementary File 5). This suggests that the peptides/nickel transporter is a potentially important pathway of virulence for these taxa during SCTLD. This transporter mediates the uptake of nickel (Hiron et al., 2010), which is an important cofactor for virulence factors (Maier and Benoit, 2019) and for neutralizing acidic environments. This may also explain the enrichment of urease, found in bin 13 (Rhodobacterales; Figure 6B and Supplementary File 5), which is nickel-dependent. The high abundance of nickel transporters and urease may also indicate that nickel itself is an important trace element for SCTLD bacteria, and given the difficulties of culturing SCTLD bacteria, it may be advantageous to use high nickel concentrations in culture mediums to select for SCTLD bacteria (Lusi et al., 2017). Ureases are also associated with various diseases and release ammonia as a byproduct, which may then further compromise the health of the coral host (Huang et al., 2011). In addition, much attention has been given to urease inhibitors due to their frequency in diseases. The use of drugs that can inhibit urease, like colloidal bismuth subcitrate, may be suitable to test on corals for SCTLD mitigation (Yang et al., 2018).
Iron Transporter Diversity in Metagenome-Assembled Genomes
Another ABC transporter that was found at high abundances within MAGs is the iron complex transport system (Figure 5). Like nickel, iron is a transition metal and it is an essential cofactor of enzymatic functions – including virulence factors (Passalacqua et al., 2016). These iron transporters are specifically known to be important factors of virulence in Streptococcus suis (order Lactobacillales) (Aranda and Cortés, 2009) and Campylobacter jejuni (order Campylobacterales) (Naikare et al., 2006). While the iron complex transport system was found across multiple MAGs (but was absent in bins 40, 18, and 14), it was most abundant in all Rhizobiales and in one Rhodobacterales (bin 13). In addition to these iron transporters, the Ferrous iron transporter (FeoB) pathway, a known virulence factor, was present in Campylobacterales, Flavobacteriales, and Beggiatoales (Supplementary Figure 4). This variance of iron transportation across the MAGs suggests niche specificity that may be needed to acquire iron during SCTLD and different methods of virulence.
Cytotoxins Found in Pseudomonadales Could Be Secreted Through the Type-1 Secretion System
There was a significant abundance of genes associated with cytotoxic serralysins and putative peptide zinc metalloprotease proteins in SINT DL compared to AH (Figure 6B and Supplementary File 5). Serralysins are metalloprotease and virulence factors (Baumann, 2006) and are best described in the coral pathogen Serratia marcescens (Sutherland et al., 2011). To cause cytotoxicity, metalloproteases are secreted through the Type-1 secretion (T1SS) system, which is known to play a role in the secretion of virulence factors such as toxins (Linhartová et al., 2010; Fuche et al., 2015), bacteriocins, lipases, and proteases (Linhartová et al., 2010). Bin 17 (Pseudomonadales; Amphritea) was the only MAG that had a complete T1SS pathway (Supplementary Figure 4) and was enriched in metalloprotease genes (Figure 6B and Supplementary File 5). While not previously associated with SCTLD, the genus Amphritea has been found in black band disease (Séré et al., 2016) and also could be an important player in SCTLD, as bin 17 was found across the five DL SINT samples and had the highest coverage (Figure 6A). Other Pseudomonadales bacteria (Pseudomonas aeruginosa) use the TISS to secrete kinases that cause cytotoxicity to eukaryotic cells (Zaborina et al., 1999). Thus, this pathway may be used to secrete cytotoxins to coral or algal symbiont cells. Although histopathological examinations have revealed few bacteria associated with the lytic necrosis of coral tissue characteristic of SCTLD (Landsberg et al., 2020), bacteria with T1SS may contribute to at least some of the cell lysis through cytotoxins like metalloproteases.
Metagenome-Assembled Genomes as Putative Pathogens
It is difficult to determine whether any of the MAGs identified in this study correspond to pathogens. While our results show that MAGs have the potential to be pathogenic bacteria because of their encoded virulence factors, we do not know from our analysis whether any of these pathways were actually being expressed or just being used for survival. Future studies should pair metagenomics with functional analysis to better understand SCTLD microbial function. We also recognize that our study is limited by the completeness of each individual MAG. If the MAGs had almost or complete genomes, we may have seen different patterns in pathway completion or virulence genes. Although our MAGs did have a range in genome completeness, we did not see any clustering by this factor. However, given that these MAGs were assembled from DL tissue and none were derived from either DU tissue or AH colonies, there is support to consider these bacteria as involved in SCTLD lesion progression and as potential pathogenic agents, regardless of whether they are primary or secondary pathogens. Of course, commensal and mutualistic bacteria can also be identified in diseased coral, but our coverage data showed that MAGs were only enriched in DL tissue compared to AH and DU corals (Supplementary File 4). However, it is important to reiterate the limitation of our study that all the MAGs were assembled from DL SINT samples, so it is not surprising that MAG coverage was higher in DL tissue. Enrichment of the bacterial community prior to extractions may have yielded a more enriched bacteria community across samples during analysis. Nevertheless, we do think the high abundance of bacteria within DL SINT tissue is biological and not an artifact of sample processing or sequencing. This is supported by the near-ubiquity of the MAGs across the DL SINT replicate samples (Figure 6A) and that three MAGs were found in DL samples of either DLAB, DSTO, or MMEA.
Conclusion
In the present study, we detected ciliates at higher abundances in SCTLD samples and additional micro-eukaryotes which may play a role in SCTLD dynamics, in addition to bacteria. Here, for the first time, we also provide the genomic capabilities of bacteria associated with SCTLD. We found an increase in relative abundance in metabolic gene functions in SCTLD diseased colonies compared to apparently healthy colonies. The higher relative abundance of the pentose phosphate pathway over the Embden–Meyerhof–Parnas pathway may indicate there was an induction of virulent factors in these samples. We also characterized 16 MAGs associated with SCTLD and provided a list of potential genes that may serve as targets for bactericidal development to ultimately reduce the use of broad-spectrum antibiotics in the treatment of SCTLD. Indeed, the development of novel treatments appears essential given the presence of antibiotic resistance genes found across the MAGs. We hypothesize that nucleotide biosynthesis, ureases, succinate dehydrogenase, and nickel and iron acquisition pathways may be targeted to halt SCTLD lesion progression on coral colonies. We also found enrichment of cytotoxin genes in Pseudomonadales that may be secreted by its Type-1 secretion system and could be a pathway through which bacteria are responsible for coral and algal symbiont cell lysis. Moving forward, more shotgun metagenomic studies should attempt to enrich the concentration of bacteria prior to sequencing, and future studies should also pair metagenomics with functional omics data to better understand which genes, proteins, and metabolites bacteria utilize during SCTLD.
Data Availability Statement
The datasets presented in this study can be found in online repositories. The names of the repository/repositories and accession number(s) can be found below: NCBI (accession: PRJNA576217). The feature tables, metadata, MAGs, orthologous gene sequences, 16S rRNA sequences, phylogenetic tree, and code used for this analysis are publicly available (Rosales, 2021).
Author Contributions
EM, LH, and RR conceived of the collection design and provided funding. SR provided funding for sequencing and analyzed the data. LH, AC, and RR collected the samples. AC processed the samples. SR and RM made the tables and figures. SR wrote the manuscript with assistance from LH. All authors edited the manuscript and assisted with the interpretation of the results.
Funding
This study was funded by the Environmental Protection Agency (EPA) 2017 South Florida Initiative grant (X7-00D66417-0), the National Oceanic and Atmospheric Administration (NOAA) Coral Reef Conservation Program (CRCP) Project/Task#S8KMCRPP00, ID 31194, and the Oceanic and Atmospheric Research (OAR) division of the NOAA omics initiative.
Conflict of Interest
The authors declare that the research was conducted in the absence of any commercial or financial relationships that could be construed as a potential conflict of interest.
Publisher’s Note
All claims expressed in this article are solely those of the authors and do not necessarily represent those of their affiliated organizations, or those of the publisher, the editors and the reviewers. Any product that may be evaluated in this article, or claim that may be made by its manufacturer, is not guaranteed or endorsed by the publisher.
Acknowledgments
We like to thank Ray Banister, Tiffany Boisvert, Katy Cummings, Kari Imhof, Lauri Maclaughlin, Stephanie Schopmeyer, and Jenni Stein for assistance with sample collection and processing in the field. The samples were collected under permits #FKNMS-2018-057 and #FKNMS-2017-100. We like to thank Nastassia Patin and Jean Lim for their feedback and for sharing their expert knowledge on metagenomics analysis.
Supplementary Material
The Supplementary Material for this article can be found online at: https://www.frontiersin.org/articles/10.3389/fmars.2021.776859/full#supplementary-material
Supplementary Figure 1 | The cumulative relative abundance of small subunit rRNA sequence reads across the kingdoms Archaea, Bacteria, Eukaryota found within coral tissue. Samples from apparently healthy coral colonies (AH), and unaffected (DU) and lesion (DL) tissues on diseased colonies, of the coral species Stephanocoenia intersepta (SINT), Diploria labyrinthiformis (DLAB), Dichocoenia stokesii (DSTO), and Meandrina meandrites (MMEA) were evaluated.
Supplementary Figure 2 | A presence/absence heatmap of other metabolic functions in 16 metagenome-assembled genomes (MAGs). The teal boxes denote the presence of a function, which is considered present if a characteristic gene of the metabolic function is found in the MAG; otherwise, the pathway is marked absent and denoted by gray cells. For some functions, there are multiple columns (x-axis) because multiple genes represent that function. The MAGs (y-axis) are labeled by bin number and the lowest taxonomic assignment designated.
Supplementary Figure 3 | Orthologous gene clusters among metagenome-assembled genomes (MAGs). (A) Upset plot showing the intersections of shared orthologous gene clusters. Each bar represents the number of orthologous gene clusters shared and the dot plot represents the MAGs in which the groups intersect. Highlighted are the shared orthologs within each order: Pseudomonadales (dark purple), Beggiatoales (light purple), Rhodobacterales (dark green), Rhizobiales (light green), Flavobacteriales (gray), and Campylobacterales (salmon). The orthologous gene clusters found in all MAGs are highlighted by the gold dots. (B) Alluvial plot of KEGG pathway counts of orthologous gene clusters that are intersected by bacterial order and among all MAGs (labeled “All”).
Supplementary Figure 4 | The coverage of KEGG pathways across 16 metagenome-assembled genomes (MAGs) based on KEGG Decoder. The heatmap represents the completeness of each module with “1” (red) indicating a complete module and “0” (deep blue) indicating that the module is not present. The MAGs (x-axis) are labeled by bin number and the lowest taxonomic assignment designated. The x-axis is hierarchically clustered by Euclidean distance among the pathways present in each MAG (bottom) and colored (as in Figure 3) by the respective bacterial order and by genome completeness of the MAGs (top).
Supplementary Figure 5 | Gene counts of miscellaneous genes across 16 metagenome-assembled genomes (MAGs). The heatmap represents the number of gene counts (square root transformed) found in each MAG from DRAM’s miscellaneous assignments. The MAGs (x-axis) are labeled by bin number and the lowest taxonomic assignment designated. The x-axis is hierarchically clustered by Euclidean distance among the genes present in each MAG genetic similarity (bottom) and colored (as in Figure 3) by the respective bacterial order and by genome completeness of the MAGs (top).
Supplementary File 1 | Details on the metagenome libraries and the metagenome assembly statistics from all coral samples from apparently healthy colonies (AH), and unaffected (DU) and lesion (DL) tissues on diseased colonies, for the coral species Stephanocoenia intersepta (SINT), Diploria labyrinthiformis (DLAB), Dichocoenia stokesii (DSTO), and Meandrina meandrites (MMEA).
Supplementary File 2 | A list of significantly differentially abundant small subunit (SSU) rRNA (padj ≤ 0.05) from bacteria and eukaryotes from all coral samples from apparently healthy colonies (AH), and unaffected (DU) and lesion (DL) tissues on diseased colonies, for the coral species Stephanocoenia intersepta (SINT), Diploria labyrinthiformis (DLAB), Dichocoenia stokesii (DSTO), and Meandrina meandrites (MMEA).
Supplementary File 3 | Summary of statistics for each metagenome-assembled genome (MAG) phylogenetic classification. Statistical outputs were generated by the programs Genome Taxonomy Database Toolkit (GTDB-Tk) and dRep.
Supplementary File 4 | The coverage results from CoverM.
Supplementary File 5 | A list of significant differentially abundant genes (padj ≤ 0.05) between apparently healthy (AH) and disease lesions (DL) in Stephanocoenia intersepta (SINT) coral using edgeR.
References
Aeby, G. S., Ushijima, B., Campbell, J. E., Jones, S., Williams, G. J., Meyer, J. L., et al. (2019). Pathogenesis of a tissue loss disease affecting multiple species of corals along the Florida reef tract. Front. Mar. Sci. 6:678. doi: 10.3389/fmars.2019.00678
Altinok, I., Capkin, E., and Karsi, A. (2015). Succinate dehydrogenase mutant of Listonella anguillarum protects rainbow trout against vibriosis. Vaccine 33, 5572–5577. doi: 10.1016/j.vaccine.2015.09.003
Altschul, S. F., Gish, W., Miller, W., Myers, E. W., and Lipman, D. J. (1990). Basic local alignment search tool. J. Mol. Biol. 215, 403–410. doi: 10.1016/S0022-2836(05)80360-2
Alvarez-Filip, L., Estrada-Saldívar, N., Pérez-Cervantes, E., Molina-Hernández, A., and González-Barrios, F. J. (2019). A rapid spread of the stony coral tissue loss disease outbreak in the Mexican Caribbean. PeerJ 7:e8069. doi: 10.7717/peerj.8069
Aranda, J., and Cortés, P. (2009). Contribution of the FeoB transporter to Streptococcus suis virulence. Int. Microbiol. 12, 137–143. doi: 10.2436/20.1501.01.91
Baumann, U. (2006). “Serralysin,” in Handbook of Metalloproteins, eds A. Messerschmidt, R. Huber, T. Poulas, K. Wieghardt, M. Cygler, and W. Bode (Chichester: John Wiley & Sons, Ltd), met030. doi: 10.1002/0470028637.met030
Bebrone, C. (2007). Metallo-β-lactamases (classification, activity, genetic organization, structure, zinc coordination) and their superfamily. Biochem. Pharmacol. 74, 1686–1701. doi: 10.1016/j.bcp.2007.05.021
Becker, C. C., Brandt, M., Miller, C. A., and Apprill, A. (2021). Microbial bioindicators of Stony Coral Tissue Loss Disease identified in corals and overlying waters using a rapid field-based sequencing approach. Environ. Microbiol. doi: 10.1111/1462-2920.15718.
Bourne, D. G., Garren, M., Work, T. M., Rosenberg, E., Smith, G. W., and Harvell, C. D. (2009). Microbial disease and the coral holobiont. Trends Microbiol. 17, 554–562. doi: 10.1016/j.tim.2009.09.004
Cárdenas, A., Neave, M. J., Haroon, M. F., Pogoreutz, C., Rädecker, N., Wild, C., et al. (2018). Excess labile carbon promotes the expression of virulence factors in coral reef bacterioplankton. ISME J. 12, 59–76. doi: 10.1038/ismej.2017.142
Case Definition: Stony Coral Tissue Loss Disease (SCTLD) (2018). Case Definition: Stony Coral Tissue Loss Disease (SCTLD). Available online at: https://floridadep.gov/sites/default/files/Copy%20of%20StonyCoralTissueLossDisease_CaseDefinition%20final%2010022018.pdf (accessed August 14, 2021).
Caspi, R., Billington, R., Keseler, I. M., Kothari, A., Krummenacker, M., Midford, P. E., et al. (2020). The MetaCyc database of metabolic pathways and enzymes – a 2019 update. Nucleic Acids Res. 48, D445–D453. doi: 10.1093/nar/gkz862
Chaumeil, P.-A., Mussig, A. J., Hugenholtz, P., and Parks, D. H. (2019). GTDB-Tk: a toolkit to classify genomes with the Genome Taxonomy Database. Bioinformatics 36, 1925–1927. doi: 10.1093/bioinformatics/btz848
Clark, A. S., Williams, S. D., Maxwell, K., Rosales, S. M., Huebner, L. K., Landsberg, J. H., et al. (2021). Characterization of the microbiome of corals with stony coral tissue loss disease along Florida’s coral reef. Microorganisms 9:2181. doi: 10.3390/microorganisms9112181
Combs, I. R., Studivan, M. S., Eckert, R. J., and Voss, J. D. (2021). Quantifying impacts of stony coral tissue loss disease on corals in Southeast Florida through surveys and 3D photogrammetry. PLoS One 16:e0252593. doi: 10.1371/journal.pone.0252593
Cook, G. M., Greening, C., Hards, K., and Berney, M. (2014). Energetics of pathogenic bacteria and opportunities for drug development. Adv. Microb. Physiol. 65, 1–62. doi: 10.1016/bs.ampbs.2014.08.001
Davidson, A. L., Dassa, E., Orelle, C., and Chen, J. (2008). Structure, function, and evolution of bacterial ATP-binding cassette systems. Microbiol. Mol. Biol. Rev. 72, 317–364. doi: 10.1128/MMBR.00031-07
Eaton, K. R., Landsberg, J. H., Kiryu, Y., Peters, E. C., and Muller, E. M. (2021). Measuring stony coral tissue loss disease induction and lesion progression within two intermediately susceptible species, Montastraea cavernosa and Orbicella faveolata. Front. Mar. Sci. 8:717265. doi: 10.3389/fmars.2021.717265
Emms, D. M., and Kelly, S. (2019). OrthoFinder: phylogenetic orthology inference for comparative genomics. Genome Biol. 20:238. doi: 10.1186/s13059-019-1832-y
Escobar-Zepeda, A., Godoy-Lozano, E. E., Raggi, L., Segovia, L., Merino, E., Gutiérrez-Rios, R. M., et al. (2018). Analysis of sequencing strategies and tools for taxonomic annotation: defining standards for progressive metagenomics. Sci. Rep. 8:12034. doi: 10.1038/s41598-018-30515-5
Fong, J. N. C., and Yildiz, F. H. (2015). Biofilm matrix proteins. Microbiol. Spectr. 3, 1–16. doi: 10.1128/microbiolspec.MB-0004-2014
Franzosa, E. A., McIver, L. J., Rahnavard, G., Thompson, L. R., Schirmer, M., Weingart, G., et al. (2018). Species-level functional profiling of metagenomes and metatranscriptomes. Nat. Methods 15, 962–968.
Fuche, F., Vianney, A., Andrea, C., Doublet, P., and Gilbert, C. (2015). Functional Type 1 secretion system involved in Legionella pneumophila virulence. J. Bacteriol. 197, 563–571. doi: 10.1128/JB.02164-14
Gintert, B. E., Precht, W. F., Fura, R., Rogers, K., Rice, M., Precht, L. L., et al. (2019). Regional coral disease outbreak overwhelms impacts from a local dredge project. Environ. Monit. Assess. 191:630. doi: 10.1007/s10661-019-7767-7
Gruber-Vodicka, H. R., Seah, B. K. B., and Pruesse, E. (2020). phyloFlash: rapid small-subunit rRNA profiling and targeted assembly from metagenomes. mSystems 5:e00920-20. doi: 10.1128/mSystems.00920-20
Gunasekera, S. P., Meyer, J. L., Ding, Y., Abboud, K. A., Luo, D., Campbell, J. E., et al. (2019). Chemical and metagenomic studies of the lethal black band disease of corals reveal two broadly distributed, redox-sensitive mixed polyketide/peptide macrocycles. J. Nat. Prod. 82, 111–121. doi: 10.1021/acs.jnatprod.8b00804
Haas, A. F., Fairoz, M. F. M., Kelly, L. W., Nelson, C. E., Dinsdale, E. A., Edwards, R. A., et al. (2016). Global microbialization of coral reefs. Nat. Microbiol. 1:16042. doi: 10.1038/nmicrobiol.2016.42
Hartman, T., Weinrick, B., Vilchèze, C., Berney, M., Tufariello, J., Cook, G. M., et al. (2014). Succinate dehydrogenase is the regulator of respiration in Mycobacterium tuberculosis. PLoS Pathog. 10:e1004510. doi: 10.1371/journal.ppat.1004510
Heres, M. M., Farmer, B. H., Elmer, F., and Hertler, H. (2021). Ecological consequences of stony coral tissue loss disease in the Turks and Caicos Islands. Coral Reefs 40, 609–624. doi: 10.1007/s00338-021-02071-4
Hiron, A., Posteraro, B., Carrière, M., Remy, L., Delporte, C., La Sorda, M., et al. (2010). A nickel ABC-transporter of Staphylococcus aureus is involved in urinary tract infection: nickel uptake and virulence in Staphylococcus aureus. Mol. Microbiol. 77, 1246–1260. doi: 10.1111/j.1365-2958.2010.07287.x
Huang, Y., Hsieh, H., Huang, S., Meng, P., Chen, Y., Keshavmurthy, S., et al. (2011). Nutrient enrichment caused by marine cage culture and its influence on subtropical coral communities in turbid waters. Mar. Ecol. Prog. Ser. 423, 83–93. doi: 10.3354/meps08944
Iwanowicz, D. D., Schill, W. B., Woodley, C. M., Bruckner, A., Neely, K., and Briggs, K. M. (2020). Exploring the stony coral tissue loss disease bacterial pathobiome. Microbiology doi: 10.1101/2020.05.27.120469
Kanehisa, M., Sato, Y., and Morishima, K. (2016). BlastKOALA and GhostKOALA: KEGG tools for functional characterization of genome and metagenome sequences. J. Mol. Biol. 428, 726–731. doi: 10.1016/j.jmb.2015.11.006
Ken-ichi, Y., Kazuo, K., Yasuhiko, M., Choong-Min, K., Masayuki, M., Hirotake, Y., et al. (2001). Combined transcriptome and proteome analysis as a powerful approach to study genes under glucose repression in Bacillus subtilis. Nucleic Acids Res. 29, 683–692. doi: 10.1093/nar/29.3.683
Keohane, C. E., Steele, A. D., Fetzer, C., Khowsathit, J., Van Tyne, D., Moynié, L., et al. (2018). Promysalin elicits species-selective inhibition of Pseudomonas aeruginosa by targeting succinate dehydrogenase. J. Am. Chem. Soc. 140, 1774–1782. doi: 10.1021/jacs.7b11212
Kline, D. I, Kuntz, N. M., Breitbart, M., Knowlton, N., and Rohwer, F. (2006). Role of elevated organic carbon levels and microbial activity in coral mortality. Mar. Ecol. Prog. Ser. 314, 119–125.
Kramer, P. R. (2021). Map of Stony Coral Tissue Loss Disease Outbreak in the Caribbean. Available online at: https://www.agrra.org/ (accessed August 14, 2021).
Landsberg, J. H., Kiryu, Y., Peters, E. C., Wilson, P. W., Perry, N., Waters, Y., et al. (2020). Stony coral tissue loss disease in Florida is associated with disruption of host–zooxanthellae physiology. Front. Mar. Sci. 7:576013. doi: 10.3389/fmars.2020.576013
Li, D., Luo, R., Liu, C.-M., Leung, C.-M., Ting, H.-F., Sadakane, K., et al. (2016). MEGAHIT v1.0: a fast and scalable metagenome assembler driven by advanced methodologies and community practices. Methods 102, 3–11. doi: 10.1016/j.ymeth.2016.02.020
Lin, H., and Peddada, S. D. (2020). Analysis of compositions of microbiomes with bias correction. Nat. Commun. 11:3514. doi: 10.1038/s41467-020-17041-7
Linhartová, I., Bumba, L., Mašín, J., Basler, M., Osička, R., Kamanová, J., et al. (2010). RTX proteins: a highly diverse family secreted by a common mechanism. FEMS Microbiol. Rev. 34, 1076–1112. doi: 10.1111/j.1574-6976.2010.00231.x
Lusi, E. A., Patrissi, T., and Guarascio, P. (2017). Nickel-resistant bacteria isolated in human microbiome. New Microbes New Infect. 19, 67–70. doi: 10.1016/j.nmni.2017.06.001
Maier, R., and Benoit, S. (2019). Role of nickel in microbial pathogenesis. Inorganics 7:80. doi: 10.3390/inorganics7070080
Meiling, S., Muller, E. M., Smith, T. B., and Brandt, M. E. (2020). 3D photogrammetry reveals dynamics of stony coral tissue loss disease (SCTLD) lesion progression across a thermal stress event. Front. Mar. Sci. 7:597643. doi: 10.3389/fmars.2020.597643
Mera, H., and Bourne, D. G. (2018). Disentangling causation: complex roles of coral-associated microorganisms in disease: disentangling coral disease causation. Environ. Microbiol. 20, 431–449. doi: 10.1111/1462-2920.13958
Meyer, J. L., Castellanos-Gell, J., Aeby, G. S., Häse, C. C., Ushijima, B., and Paul, V. J. (2019). Microbial community shifts associated with the ongoing stony coral tissue loss disease outbreak on the Florida reef tract. Front. Microbiol. 10:2244. doi: 10.3389/fmicb.2019.02244
Miller, A. W., and Richardson, L. L. (2011). A meta-analysis of 16S rRNA gene clone libraries from the polymicrobial black band disease of corals: meta-analysis of BBD clone libraries. FEMS Microbiol. Ecol. 75, 231–241. doi: 10.1111/j.1574-6941.2010.00991.x
Miller, M., Karazsia, J., Groves, C. E., Griffin, S., Moore, T., Wilber, P., et al. (2016). Detecting sedimentation impacts to coral reefs resulting from dredging the Port of Miami, Florida USA. PeerJ 4:e2711. doi: 10.7717/peerj.2711
Mirdita, M., Steinegger, M., Breitwieser, F., Söding, J., and Levy Karin, E. (2021). Fast and sensitive taxonomic assignment to metagenomic contigs. Bioinformatics 37, 3029–3031. doi: 10.1093/bioinformatics/btab184
Muller, E. M., Sartor, C., Alcaraz, N. I., and van Woesik, R. (2020). Spatial epidemiology of the stony-coral-tissue-loss disease in Florida. Front. Mar. Sci. 7:163. doi: 10.3389/fmars.2020.00163
Naikare, H., Palyada, K., Panciera, R., Marlow, D., and Stintzi, A. (2006). Major role for FeoB in Campylobacter jejuni ferrous iron acquisition, gut colonization, and intracellular survival. Infect. Immun. 74, 5433–5444. doi: 10.1128/IAI.00052-06
Neely, K. L., Macaulay, K. A., Hower, E. K., and Dobler, M. A. (2020). Effectiveness of topical antibiotics in treating corals affected by stony coral tissue loss disease. PeerJ 8:e9289. doi: 10.7717/peerj.9289
Neely, K. L., Shea, C. P., Macaulay, K. A., Hower, E. K., and Dobler, M. A. (2021). Short- and long-term effectiveness of coral disease treatments. Front. Mar. Sci. 8:675349. doi: 10.3389/fmars.2021.675349
Olm, M. R., Brown, C. T., Brooks, B., and Banfield, J. F. (2017). dRep: a tool for fast and accurate genomic comparisons that enables improved genome recovery from metagenomes through de-replication. ISME J. 11, 2864–2868. doi: 10.1038/ismej.2017.126
Parks, D. H., Chuvochina, M., Chaumeil, P.-A., Rinke, C., Mussig, A. J., and Hugenholtz, P. (2020). A complete domain-to-species taxonomy for Bacteria and Archaea. Nat. Biotechnol. 38, 1079–1086. doi: 10.1038/s41587-020-0501-8
Parks, D. H., Chuvochina, M., Waite, D. W., Rinke, C., Skarshewski, A., Chaumeil, P.-A., et al. (2018). A standardized bacterial taxonomy based on genome phylogeny substantially revises the tree of life. Nat. Biotechnol. 36, 996–1004. doi: 10.1038/nbt.4229
Parks, D. H., Imelfort, M., Skennerton, C. T., Hugenholtz, P., and Tyson, G. W. (2015). CheckM: assessing the quality of microbial genomes recovered from isolates, single cells, and metagenomes. Genome Res. 25, 1043–1055. doi: 10.1101/gr.186072.114
Parks, D. H., Rinke, C., Chuvochina, M., Chaumeil, P.-A., Woodcroft, B. J., Evans, P. N., et al. (2017). Recovery of nearly 8,000 metagenome-assembled genomes substantially expands the tree of life. Nat. Microbiol. 2, 1533–1542. doi: 10.1038/s41564-017-0012-7
Passalacqua, K. D., Charbonneau, M.-E., and O’Riordan, M. X. D. (2016). Bacterial metabolism shapes the host–pathogen interface. Microbiol. Spectr. 4, 1–31. doi: 10.1128/microbiolspec.VMBF-0027-2015
Pratte, Z., and Richardson, L. (2016). Possible links between white plague-like disease, scleractinian corals, and a cryptochirid gall crab. Dis. Aquat. Organ. 122, 153–161. doi: 10.3354/dao03074
Precht, W. F., Gintert, B. E., Robbart, M. L., Fura, R., and van Woesik, R. (2016). Unprecedented disease-related coral mortality in Southeastern Florida. Sci. Rep. 6:31374. doi: 10.1038/srep31374
Robinson, M. D., McCarthy, D. J., and Smyth, G. K. (2010). edgeR: a Bioconductor package for differential expression analysis of digital gene expression data. Bioinformatics 26, 139–140. doi: 10.1093/bioinformatics/btp616
Romano, A. H., and Conway, T. (1996). Evolution of carbohydrate metabolic pathways. Res. Microbiol. 147, 448–455. doi: 10.1016/0923-2508(96)83998-2
Rosales, S. (2021). Shotgun Metagenome Analsysis to Understand SCTLD in Epidemic Reefs. doi: 10.6084/M9.FIGSHARE.C.5562255.V1
Rosales, S. M., Clark, A. S., Huebner, L. K., Ruzicka, R. R., and Muller, E. M. (2020). Rhodobacterales and rhizobiales are associated with stony coral tissue loss disease and its suspected sources of transmission. Front. Microbiol. 11:681. doi: 10.3389/fmicb.2020.00681
Rosales, S. M., Miller, M. W., Williams, D. E., Traylor-Knowles, N., Young, B., and Serrano, X. M. (2019). Microbiome differences in disease-resistant vs. susceptible Acropora corals subjected to disease challenge assays. Sci. Rep. 9:18279. doi: 10.1038/s41598-019-54855-y
Samant, S., Lee, H., Ghassemi, M., Chen, J., Cook, J. L., Mankin, A. S., et al. (2008). Nucleotide biosynthesis is critical for growth of bacteria in human blood. PLoS Pathog. 4:e37. doi: 10.1371/journal.ppat.0040037
Schliep, K. P. (2011). phangorn: phylogenetic analysis in R. Bioinformatics 27, 592–593. doi: 10.1093/bioinformatics/btq706
Sekar, R., Kaczmarsky, L. T., and Richardson, L. L. (2009). Effect of freezing on PCR amplification of 16S rRNA genes from microbes associated with black band disease of corals. Appl. Environ. Microbiol. 75, 2581–2584. doi: 10.1128/AEM.01500-08
Séré, M., Wilkinson, D. A., Schleyer, M. H., Chabanet, P., Quod, J.-P., and Tortosa, P. (2016). Characterisation of an atypical manifestation of black band disease on Porites lutea in the Western Indian Ocean. PeerJ 4:e2073. doi: 10.7717/peerj.2073
Shaffer, M., Borton, M. A., McGivern, B. B., Zayed, A. A., La Rosa, S. L., Solden, L. M., et al. (2020). DRAM for distilling microbial metabolism to automate the curation of microbiome function. Nucleic Acids Res. 48, 8883–8900. doi: 10.1093/nar/gkaa621
Sharp, W. C., Shea, C. P., Maxwell, K. E., Muller, E. M., and Hunt, J. H. (2020). Evaluating the small-scale epidemiology of the stony-coral -tissue-loss-disease in the middle Florida Keys. PLoS One 15:e0241871. doi: 10.1371/journal.pone.0241871
Shilling, E. N., Combs, I. R., and Voss, J. D. (2021). Assessing the effectiveness of two intervention methods for stony coral tissue loss disease on Montastraea cavernosa. Sci. Rep. 11:8566. doi: 10.1038/s41598-021-86926-4
Shraim, R., Dieng, M. M., Vinu, M., Vaughan, G., McParland, D., Idaghdour, Y., et al. (2017). Environmental extremes are associated with dietary patterns in Arabian Gulf reef fishes. Front. Mar. Sci. 4:285. doi: 10.3389/fmars.2017.00285
Sunagawa, S., DeSantis, T. Z., Piceno, Y. M., Brodie, E. L., DeSalvo, M. K., Voolstra, C. R., et al. (2009). Bacterial diversity and white plague disease-associated community changes in the Caribbean coral Montastraea faveolata. ISME J. 3, 512–521. doi: 10.1038/ismej.2008.131
Sutherland, K. P., Shaban, S., Joyner, J. L., Porter, J. W., and Lipp, E. K. (2011). Human pathogen shown to cause disease in the threatened Eklhorn coral Acropora palmata. PLoS One 6:e23468. doi: 10.1371/journal.pone.0023468
Sweet, M. J., and Séré, M. G. (2016). Ciliate communities consistently associated with coral diseases. J. Sea Res. 113, 119–131. doi: 10.1016/j.seares.2015.06.008
Syberg-Olsen, M. J., Irwin, N. A. T., Vannini, C., Erra, F., Di Giuseppe, G., Boscaro, V., et al. (2016). Biogeography and character evolution of the ciliate genus Euplotes (Spirotrichea, Euplotia), with description of Euplotes curdsi sp. nov. PLoS One 11:e0165442. doi: 10.1371/journal.pone.0165442
Thome, P. E., Rivera-Ortega, J., Rodríguez-Villalobos, J. C., Cerqueda-García, D., Guzmán-Urieta, E. O., García-Maldonado, J. Q., et al. (2021). Local dynamics of a white syndrome outbreak and changes in the microbial community associated with colonies of the Scleractinian brain coral Pseudodiploria strigosa. PeerJ 9:e10695. doi: 10.7717/peerj.10695
Tout, J., Jeffries, T. C., Petrou, K., Tyson, G. W., Webster, N. S., Garren, M., et al. (2015). Chemotaxis by natural populations of coral reef bacteria. ISME J. 9, 1764–1777. doi: 10.1038/ismej.2014.261
Truong, D. T., Franzosa, E. A., Tickle, T. L., Scholz, M., Weingart, G., Pasolli, E., et al. (2015). MetaPhlAn2 for enhanced metagenomic taxonomic profiling. Nat. Methods 12, 902–903. doi: 10.1038/nmeth.3589
Typas, A., Banzhaf, M., Gross, C. A., and Vollmer, W. (2012). From the regulation of peptidoglycan synthesis to bacterial growth and morphology. Nat. Rev. Microbiol. 10, 123–136. doi: 10.1038/nrmicro2677
Ushijima, B., Meyer, J. L., Thompson, S., Pitts, K., Marusich, M. F., Tittl, J., et al. (2020). Disease diagnostics and potential coinfections by Vibrio coralliilyticus during an ongoing coral disease outbreak in Florida. Front. Microbiol. 11:569354. doi: 10.3389/fmicb.2020.569354
Vega Thurber, R., Mydlarz, L. D., Brandt, M., Harvell, D., Weil, E., Raymundo, L., et al. (2020). Deciphering coral disease dynamics: integrating host, microbiome, and the changing environment. Front. Ecol. Evol. 8:575927. doi: 10.3389/fevo.2020.575927
Walker, B. K., Turner, N. R., Noren, H. K. G., Buckley, S. F., and Pitts, K. A. (2021). Optimizing stony coral tissue loss disease (SCTLD) intervention treatments on Montastraea cavernosa in an endemic zone. Front. Mar. Sci. 8:666224. doi: 10.3389/fmars.2021.666224
Walton, C. J., Hayes, N. K., and Gilliam, D. S. (2018). Impacts of a regional, multi-year, multi-species coral disease outbreak in Southeast Florida. Front. Mar. Sci. 5:323. doi: 10.3389/fmars.2018.00323
Williams, S. D., Walter, C. S., and Muller, E. M. (2021). Fine scale temporal and spatial dynamics of the stony coral tissue loss disease outbreak within the lower Florida keys. Front. Mar. Sci. 8:631776. doi: 10.3389/fmars.2021.631776
Wu, Y.-W., Simmons, B. A., and Singer, S. W. (2016). MaxBin 2.0: an automated binning algorithm to recover genomes from multiple metagenomic datasets. Bioinformatics 32, 605–607. doi: 10.1093/bioinformatics/btv638
Yang, X., Koohi-Moghadam, M., Wang, R., Chang, Y.-Y., Woo, P. C. Y., Wang, J., et al. (2018). Metallochaperone UreG serves as a new target for design of urease inhibitor: a novel strategy for development of antimicrobials. PLoS Biol. 16:e2003887. doi: 10.1371/journal.pbio.2003887
Zaborina, O., Misra, N., Kostal, J., Kamath, S., Kapatral, V., El-Idrissi, M. E.-A., et al. (1999). P2Z-independent and P2Z receptor-mediated macrophage killing by Pseudomonas aeruginosa isolated from cystic fibrosis patients. Infect. Immun. 67, 5231–5242. doi: 10.1128/IAI.67.10.5231-5242.1999
Zrafi-Nouira, I., Guermazi, S., Chouari, R., Safi, N. M., Pelletier, E., Bakhrouf, A., et al. (2009). Molecular diversity analysis and bacterial population dynamics of an adapted seawater microbiota during the degradation of Tunisian zarzatine oil. Biodegradation 20, 467–486. doi: 10.1007/s10532-008-9235-x
Keywords: SCTLD, microbiomes, ciliates, Florida’s coral reef, Rhodobacterales, metagenomes
Citation: Rosales SM, Huebner LK, Clark AS, McMinds R, Ruzicka RR and Muller EM (2022) Bacterial Metabolic Potential and Micro-Eukaryotes Enriched in Stony Coral Tissue Loss Disease Lesions. Front. Mar. Sci. 8:776859. doi: 10.3389/fmars.2021.776859
Received: 14 September 2021; Accepted: 03 December 2021;
Published: 06 January 2022.
Edited by:
Lorenzo Alvarez-Filip, National Autonomous University of Mexico, MexicoReviewed by:
Zoe Adina Pratte, Montana State University, United StatesJulie L. Meyer, University of Florida, United States
Copyright © 2022 Rosales, Huebner, Clark, McMinds, Ruzicka and Muller. This is an open-access article distributed under the terms of the Creative Commons Attribution License (CC BY). The use, distribution or reproduction in other forums is permitted, provided the original author(s) and the copyright owner(s) are credited and that the original publication in this journal is cited, in accordance with accepted academic practice. No use, distribution or reproduction is permitted which does not comply with these terms.
*Correspondence: Stephanie M. Rosales, U3RlcGhhbmllLlJvc2FsZXNATk9BQS5nb3Y=; c3Jvc2FsZXM3MTJAZ21haWwuY29t