- 1School of Biological Sciences, University of Aberdeen, Aberdeen, United Kingdom
- 2National Oceanography Centre, Liverpool, United Kingdom
There is about to be an abrupt step-change in the use of our coastal seas, specifically by the addition of large-scale offshore renewable energy developments to combat climate change. Many trade-offs will need to be weighed up for the future sustainable management of marine ecosystems between renewables and other uses (e.g., fisheries, marine protected areas). Therefore, we need a much greater understanding of how different marine habitats and ecosystems are likely to change with both natural and anthropogenic transformations. This work will present a review of predictive Bayesian approaches from ecosystem level, through to fine scale mechanistic understanding of foraging success by individual species, to identify consistent physical (e.g., bottom temperature) and biological (e.g., chlorophyll-a) indicators of habitat and ecosystem change over the last 30 years within the North Sea. These combined approaches illuminate the feasibility of integrating knowledge across scales to be able to address the spatio-temporal variability of biophysical indicators to ultimately strengthen predictions of population changes at ecosystem scales across broadly different habitat types. Such knowledge will provide an effective baseline for more strategic and integrated approaches to both monitoring studies and assessing anthropogenic impacts to be used within marine spatial planning considerations.
Introduction
There is clear evidence of climate change within shallow seas, which are being affected by rising temperatures, for example, the well-studied United Kingdom waters north of Scotland and the North Sea have suffered rapid warming with temperatures increasing by up to 0.24°C per decade (Tinker et al., 2020). Latest projections imply increased coastal-flood risk due to sea-level rise (Horsburgh et al., 2020), reduced oxygen (Mahaffey et al., 2020; Wakelin et al., 2020), and exacerbating impacts from ocean acidification (Humphreys et al., 2020). These physical changes are having an impact throughout the food web, with effects on abundance, distribution, and biodiversity, seen in plankton (Edwards et al., 2020), fish (Wright et al., 2020), seabirds (Mitchell et al., 2020), and mammals (Evans and Waggitt, 2020).
One of the more likely solutions to combat climate change is the introduction of large-scale offshore renewable energy (ORE) developments (wind, tidal and wave) of 100 s of gigawatts (GW) (IRENA, 2019). However, the introduction of new structures and the extraction of more energy either from wind, wave or tides will have cumulative effects within the world’s shallow seas and therefore will also influence whole ecosystems. The size of these developments may also end up using more than 30% of coastal sea space (Figure 1), and some of the consequences that will most likely follow include physical habitat change (De Dominicis et al., 2018), displacement of fisheries (Kafas et al., 2017) and possible creation of de facto marine protected areas (MPAs) (Raoux et al., 2019).
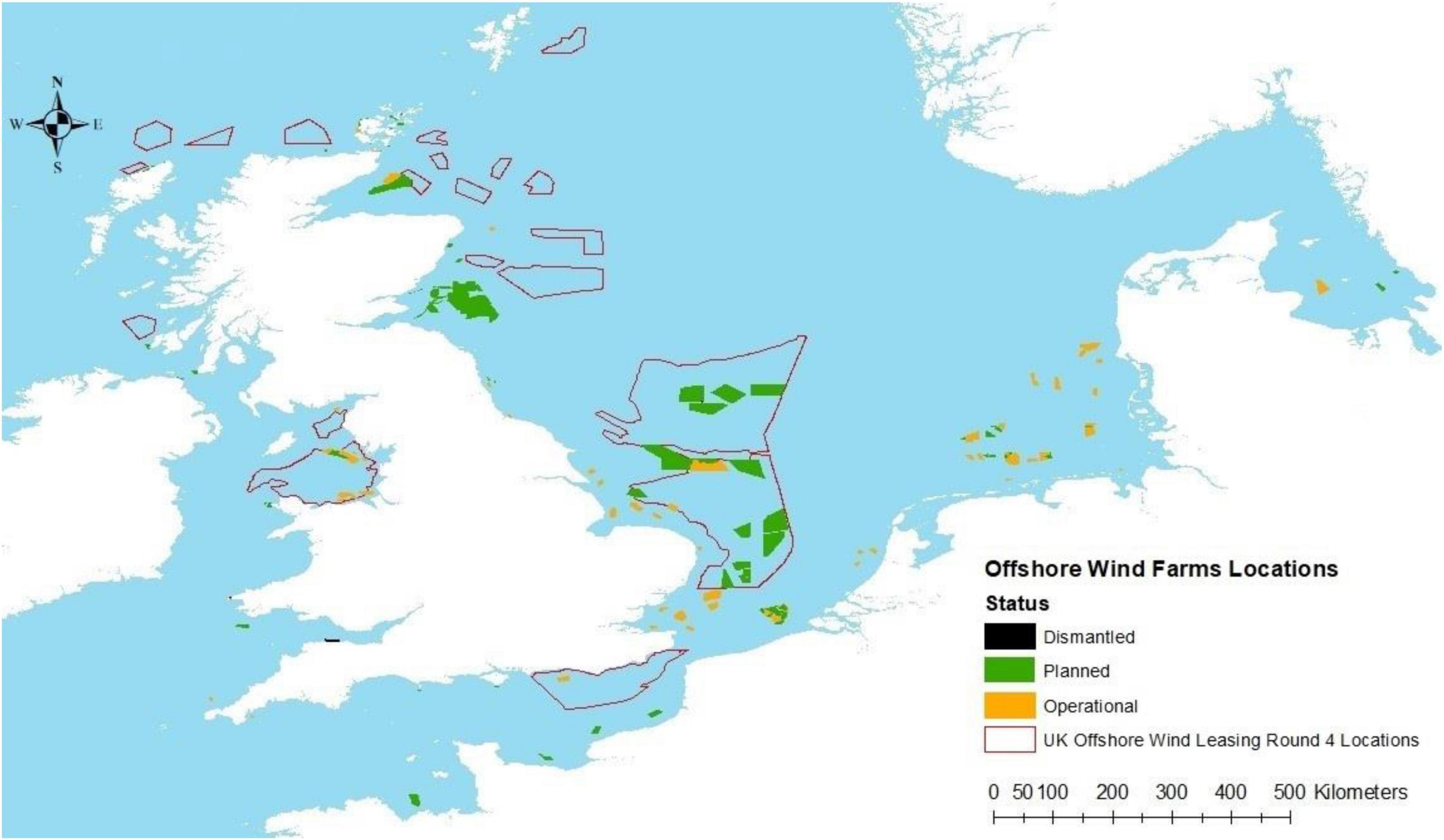
Figure 1. Offshore wind farms locations from past, currently operational and those planned, as well as the range of areas, available for use in leasing round 4.
However, to be able to move toward a much better evidence base for marine spatial planning (MSP), there is the need to identify and quantify the impacts separately from both climate change and ORE developments. To do this, we first need to understand how marine habitats are likely to change across different spatial scales and over time and so too their associated ecosystem characteristics, specifically their structures and function. To address this, we need to define indicators of habitat and ecosystem change but first we need to assess what makes a good indicator of change in marine ecosystems. It is generally assumed that visible top predators, such as seabirds and mammals, are sirens of ecosystem change. However, often nothing other than annual productivity and/or foraging trip durations are measured (Wanless et al., 2009; Russell et al., 2014), and without measured linkages to other physical and/or biological indicators at an ecosystem scale, it is not clear what exactly are they indicating that is changing. As an example, a series of studies that has tried to understand the details in the ecosystem linkages that drive kittiwake annual breeding success (Eerkes-Medrano et al., 2017) has shown that there is a need to understand more detail about other trophic levels and in this case, not only the biomass but the phenology of zooplankton is essential to include to predict kittiwake breeding success. Therefore, the increase in knowledge about which indicators to use and what they are telling us about how the ecosystem is functioning is necessary to add to the use of top predators and will ultimately guide future studies across a range of spatial and temporal scales and will reduce uncertainties of direct and indirect effects on populations across a range of trophic levels. Full understanding, due to the complex nature of ecosystems is challenging, however, we need to have pragmatic approaches to deal with the rapid expansion of ORE that is happening now (IRENA, 2019).
In this review, we suggest making the direct use of physical (e.g., bottom temperature) and biological (e.g., net primary production) indicators, that will be impacted by both predicted climate change and large-scale ORE extraction (Boon et al., 2018; Sadykova et al., 2020; Figure 2). An indicator is a physical and/or biological ecosystem component, that could be seen described as an environmental predictor, a response, or a pressure but we choose to use the terminology “indicator” to describe ecosystem components that have been found to help predict (“indicate”) habitat and/or ecosystem change over the last 30 years within the North Sea. The indicators are defined based on the literature reviewed for this study scoping the range of variables found to be significant drivers of variation, or direction of change, in either behaviors, distributions and/or population dynamics of the highly mobile top predator marine species from very fine spatial scales through to large ecosystem scales, thus delivering an understanding of the indicators of habitat and ecosystem change in the North Sea over the last 30 years. We present evidence from a range of case studies from large ecosystem-level through to fine spatial scales which show that the defined physical and biological indicators are effective predictors of habitat and ecosystem change. The results presented are based on recent novel modeling approaches and outputs, with the aim to effectively integrate and combine knowledge across spatial scales (i.e., individuals to populations and meta-populations). We reviewed the outputs to ensure that those are the models that have the highest confidence and are the most consistently predictable indicators of ecosystem change to ultimately increase certainty of predictions of future population changes and ecosystem dynamics. We synthesize what we have learned from marine ecosystem modeling approaches with respect to understanding the impacts of climate change and large-scale ORE developments, with the aim to effectively use and communicate their combined outputs to increase confidence in model projections and obtain more holistic knowledge of complex ecological systems. Finally, we discuss the implications from our synthesis and propose possible directions for future research and approaches with the aim to better utilize knowledge from existing data sources, survey techniques and modeling formulations to help guide future multitrophic studies to be performed at the most meaningful ecological scales for the types of investigations being proposed.
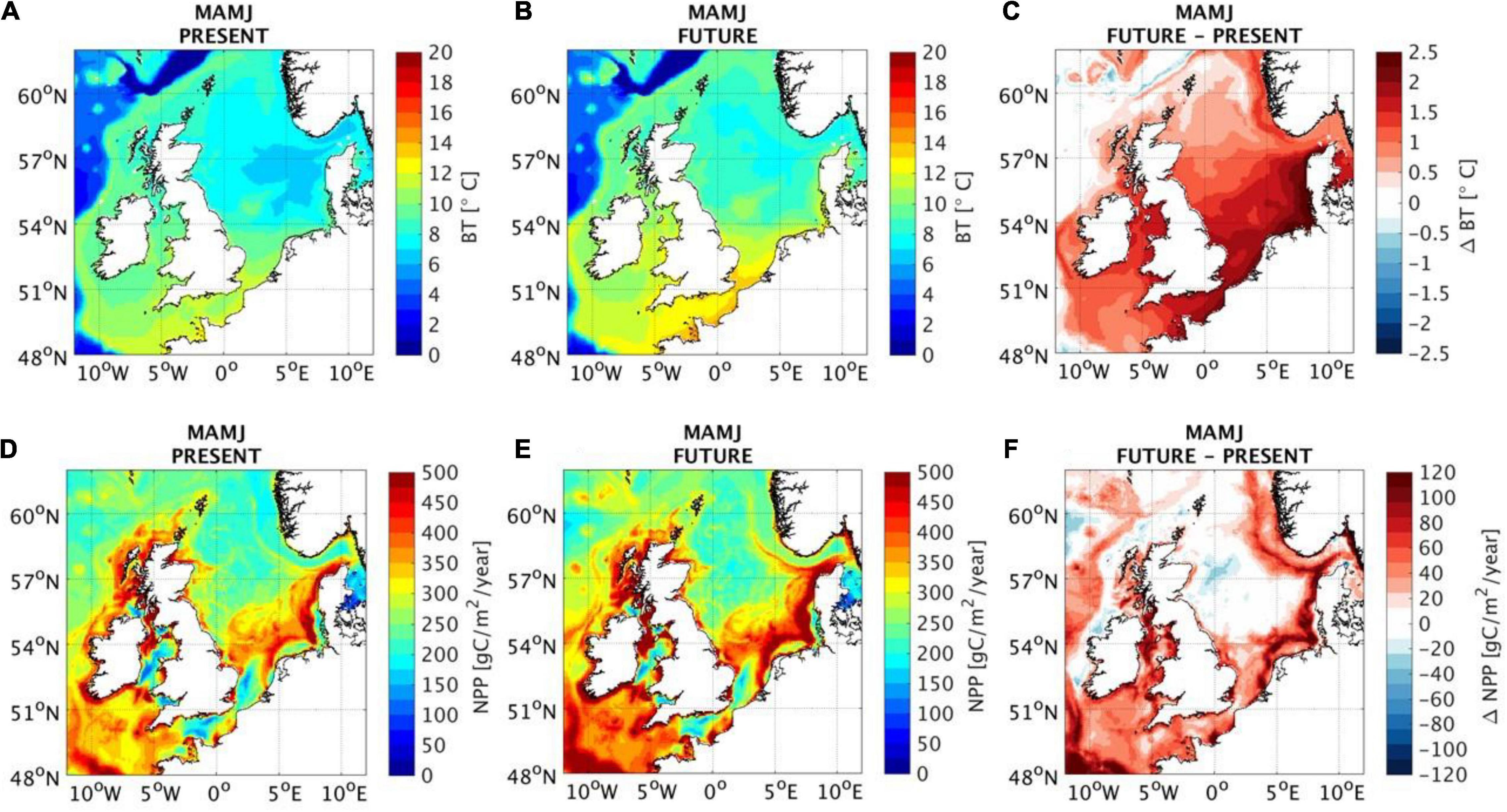
Figure 2. Present spring-summer (March–April–May–June: MAMJ) from a mean of 25 years (1989–2014) (A,D), future under “business as usual” (worst case) greenhouse-gas emissions scenario in 2050 from a mean of projected yearly outcomes from 2037 to 2062 (B,E) and difference between present and future (C,F) for bottom temperature (BT) and net primary production (NPP). Data provided from the Atlantic Margin Model 7 × 7 km (AMM7-NEMO) 3D baroclinic, hydrodynamic model, coupled with an ecosystem model ERSEM (Wakelin et al., 2015); for more information, see Sadykova et al. (2020).
Background
The Importance of Spatial and Temporal Scale to Define Specific Indicators
The spatial and temporal scale of physical and biological indicators is a challenging issue with respect to understanding the multiplicity of mechanisms underlying observed patterns and variability changes (Wiens, 1989, 1990; Levin, 1992) and especially the trophic interactions of highly mobile marine animals (fish, seabirds, and marine mammals) within dynamic marine environments. Case studies from around the globe represent the complexity between scale and the need to define indicators to be able to support management and conservation: e.g., Bay of Fundy (Johnston et al., 2005; Johnston and Read, 2007), Florida Bay (Torres et al., 2008), Pacific coast (Zamon, 2001), south coast of Australia (Ropert-Coudert et al., 2009), southern oceans (Bost et al., 2009). Recognizing the spatial and temporal heterogeneity of physical and biological indicators to understand habitat suitability, species distribution and behavior has been discussed on a wider scale for different cetacean species (Chavez-Rosales et al., 2019), but also on a finer scale for fish in the California current (Sabal et al., 2020), elephant seals in the Pacific Ocean (Abrahms et al., 2018) and whales in the Mediterranean (Cotté et al., 2011). These examples illustrate the importance of scale-dependent interplay between the physical environment, individual fitness, predator-prey interactions, and the mechanisms that drive population dynamics at multiple scales.
The North Sea has been considered as a coastal biome (Longhurst, 2010), a large marine ecosystem (Sherman et al., 2009) and a specific ecoregion (Spalding et al., 2007), but several studies demonstrate the need for detailed physical and biological understanding at a finer scale (Embling et al., 2012; Jones et al., 2014; Scales et al., 2017; Cox et al., 2018; Sadykova et al., 2020). The high hydrodynamic variability of some central North Sea areas, due to the large variability in duration of mixed and stratified conditions, described by van Leeuwen et al. (2016), suggests the hypothesis that such areas might be characterized by a more heterogeneous spatial pattern on a finer scale.
Physical and biological processes exhibit high spatial variability in the oceans (Tweddle et al., 2018). For example, there are large differences over small spatial distances in vertical gradients in light levels (Falkowski and Raven, 2013), horizontal and vertical gradients in temperature and salinity (Boyer et al., 2013), turbulent mixing (Simpson and Sharples, 2012), nutrient concentrations (Hydes et al., 2001; Boyer et al., 2013; Tweddle et al., 2013), phytoplankton concentrations and primary productivity (Behrenfeld et al., 2006), as well as predator-prey species distributions (Scott et al., 2010; Kaltenberg and Benoit-Bird, 2013). Many of these processes also vary temporally in their occurrence and as such, the distributions of highly mobile marine top predators across oceanographically dynamic areas may vary over both large (seasonal) and short (bi-weekly) temporal scales, due to seasonal stratification and the bi-weekly formation of tidal-mixing fronts (Johnston et al., 2005; Scales et al., 2014; IJsseldijk et al., 2015; Cox et al., 2016). Therefore, temporal changes in highly mobile top predator distributional patterns are likely to reflect those of their preferred prey, which may, in turn, be bottom–up driven by dynamic oceanographic processes (Cox et al., 2018). Such spatio-temporal variability has implications for the ecosystem structure and function and ecosystem responses to disturbances, all of which are likely to be scale dependent (Fauchald et al., 2011; Trifonova et al., 2015, 2021; Preciado et al., 2019; Heim et al., 2021).
Stratification of the water column is a key control for coastal shelf seas, as it is the main determinant in the spatial distribution of habitat types (Sharples et al., 2020). The timing and strength of stratification, which is mainly determined by annual season weather patterns, tidal forcing, and depth (Simpson and Sharples, 2012), is a main mechanistic driver for primary and secondary production, can influence the distribution of marine animals (Scott et al., 2010; Carroll et al., 2015; Cox et al., 2018) and cause implications to the breeding success of seabirds (Durant et al., 2006; Scott et al., 2006; Borstad et al., 2011). A warming climate is likely to alter the timing and length of summer stratification (Holt et al., 2010), which is a key physical control for the formation and level of production of the spring plankton bloom (Sharples et al., 2020). Change in the timing of blooms can lead to a temporal mismatch between fish larvae and their plankton prey, with implications for recruitment (Wright et al., 2020), fisheries production and other marine life (Edwards et al., 2020), highlighting the complex relationship between the change in physical habitat, following climate warming and predator-prey interactions. Worryingly, it has been found that both climate change and very large-scale tidal ORE developments can act in the same direction in terms of increasing stratification and consequently impact primary production. However, in the one direct comparison study that has been done, the effect of climate change is an order of magnitude higher than tidal effects by as early as 2050 and the tidal extraction effects are extremely location specific (De Dominicis et al., 2018; Figure 3).
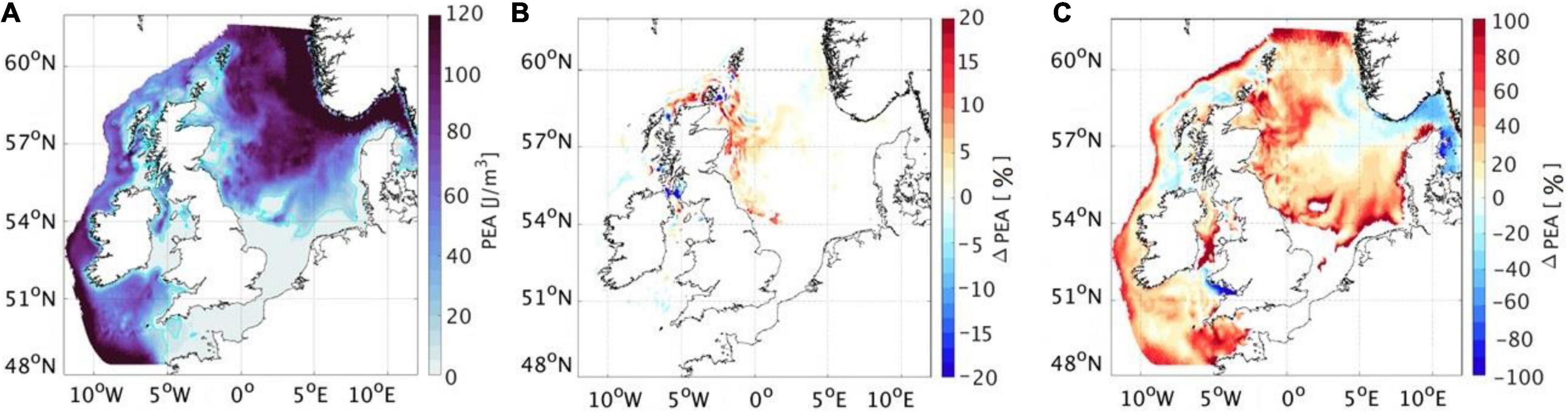
Figure 3. Present June–July–August potential energy anomaly (PEA, defined as an indicator to measure stratification and mixing rate) (A), future PEA under 6 GW energy extraction (B) and future PEA under “business as usual” (worst case) greenhouse-gas emissions scenario in 2050 (C). Data provided from the unstructured grid coastal ocean model, FVCOM (Finite-Volume Community Ocean Model, Chen et al., 2003), using an implementation known as the Scottish Shelf Model (SSM, Wolf et al., 2016); for more information, see De Dominicis et al. (2018).
Definitions of Scale and Types of Offshore Renewable Energy Extraction
In this section, we provide reason for and define the range of spatial scales (Figure 4) used in this study along with the background information on the major ecosystem level changes over the last 30 years in the North Sea. The largest scale is the entire shallow shelf sea area (in this specific example, defined as covering the North Sea and the United Kingdom continental shelf between 48° and 62° North and 10° West and 12° East; Figure 4A). The whole shallow shelf sea scale is relevant for numerous issues in the management and conservation of mobile marine species such as migratory fish species, seabirds, and marine mammals (Hammond et al., 2013; Cook et al., 2014b; Rutterford et al., 2015; Sadykova et al., 2017). The next scale down is the regional spatial scale and it can encompass populations and/or meta-populations of mobile species and each region consists of contrasting habitat types, which are more or less suitable for different ORE industries, based on depth and exposer/or not to wider oceanic influences. In the North Sea, these are four regions [each sized approximately 3500 km2; see Trifonova et al. (2021) and Figure 4A, that shows the Shetland and Orkney region] and range from the western Scottish waters, that are mainly influenced by western Atlantic Ocean conditions and targeted for wave energy developments (Davies et al., 2012); the Shetland and Orkney region with shelf edge habitats and some of the best tidal resources in the world (Davies et al., 2012); the northern North Sea that represents an expanse of deep (>60 m) areas, suitable for floating wind (Sectoral Marine Plan for Offshore Wind Energy, 2020) and shallow southern North Sea areas with depths < 50 m, suitable for static offshore wind (Offshore Renewable Energy Strategy European Commission, 2020).
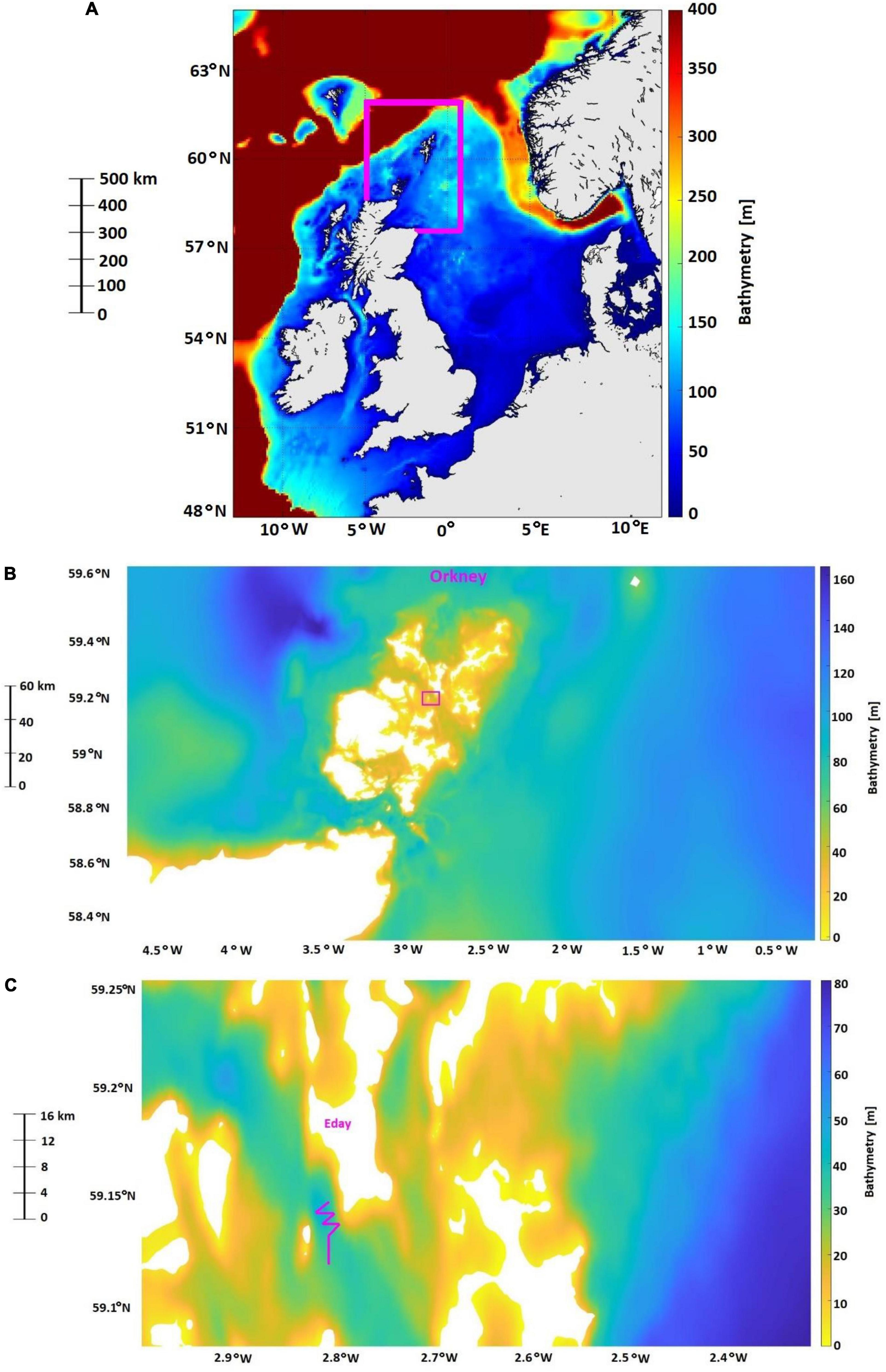
Figure 4. The North Sea and the United Kingdom continental shelf and the spatial boundaries of Shetland and Orkney region (highlighted in purple) to represent the shallow shelf sea and regional scales (A), respectively, as defined in this work, the archipelago of Orkney as a representation of the local scale (B) and the island of Eday and an example of vessel-based transects, based on Waggitt et al. (2016b), as a representation of the fine scale (C).
The size of the local spatial scale (in the range of 100s km; Figure 4B) is defined based on the characteristics at the base of marine food chains: phytoplankton and primary productivity (Holt et al., 2012; Tweddle et al., 2018). This approach allows knowledge about the base of ecosystems to be used to assist assessment of environmental status within MSP (including MPAs) and within sectoral licensing to support ecosystem-based sustainable management (Tweddle et al., 2018). In addition, recognizing the importance of inter-annual variability in the timing of spring stratification and plankton blooms is a key component to the understanding of the mechanisms behind variations in marine animal populations on a local scale (Sharples et al., 2006). The fine spatial scale (<1 km; Figure 4C) is defined to provide a more mechanistic understanding of which habitat variables are more important to an individual of a given species and most importantly, to achieve an understanding of preferred foraging habitats, including preferences for local hydrodynamic conditions, which can then be conveyed at an ecosystem level (Sharples et al., 2013; Waggitt et al., 2016a; Williamson et al., 2019).
Physical and Biological Indicators for Multiple Spatial and Temporal Scales
Physical Indicators: Stratification
One of several possible physical indicators to measure stratification is the potential energy anomaly (PEA, J/m3). PEA represents the amount of work required to bring about complete vertical mixing per unit of volume (Simpson and Bowers, 1981) and indicates the level of stratification and mixing rate (De Boer et al., 2008; De Dominicis et al., 2018). Recent modeling outcomes reveal that PEA plays a significant role in predicting the abundance changes of both lower (e.g., sandeel larvae) and higher trophic level (e.g., harbor porpoise, black-legged kittiwake) marine species on a regional spatial scale (Carroll et al., 2015; Trifonova et al., 2021) and to a lesser extent, in determining habitat preferences on a North Sea scale (Sadykova et al., 2017; Wakefield et al., 2017). PEA can reflect more subtle spatial and temporal changes within a habitat type (van Leeuwen et al., 2016) and season (Simpson and Bowers, 1981), thus further highlighting the importance of spatial and seasonal distribution of physical processes as good indicators up through the entire trophic chain and any changes that are affecting ecosystem functioning.
On a local spatial scale, work by Sharples et al. (2013), has shown how seabed topography, in particular, during the period of established stratification, can have a significant effect on the levels of primary production, which were correlated with fish behavior, foraging seabirds and fisheries response and activity. On a fine spatial scale, stratification was also a key indicator and specifically when looking at the impacts of offshore wind farm structures (for example, by reducing vertical ocean mixing: Afsharian and Taylor, 2019 or reducing wind speeds and resultant wind stresses at the sea surface: Miles et al., 2020) that will have consequences for primary production (Carpenter et al., 2016; Schultze et al., 2020). Few studies have included analyses of the wind farm and/or tidal turbine impacts on primary production, and their results differ among regions due to their unequal topographic and oceanographic conditions. However, the studies showed that physical processes controlling rates of mixing and stratification are of key importance to understanding changes in the level of primary production on a relatively fine to local scale (van der Molen et al., 2014, 2016; Schultze et al., 2020) and ecosystem-wide spatial scales (Ludewig, 2015). Thus, using the PEA indicator to capture changes in stratification is important as changes in primary production may lead to further impacts on ecosystem services, such as fisheries, but also mobile marine predator populations.
Physical Indicators: Bottom Temperature and Sea Surface Temperature
Temperature is a major driver of marine ecosystems and one of the key factors affecting the physiology and ecology of all marine organisms (Simpson et al., 2011; Edwards et al., 2020; Evans and Waggitt, 2020). On a North Sea spatial scale, bottom temperature (BT, °C) was found to be important only for some top predator species in Sadykova et al. (2017). However, it has been shown that warming BTs can induce changes in the distribution, abundance, and richness of fish species in the North Sea, including the west of Scotland region (Dulvy et al., 2008; Ter Hofstede et al., 2010), Celtic Sea (Mérillet et al., 2020) and other large shelf marine ecosystems (Hunt et al., 2008; Sagarese et al., 2014). Similarly, BT was found to be a better physical indicator of abundance and/or biomass change on a regional spatial scale, compared to the sea surface temperature (SST, °C), except in the southern North Sea where the water column is generally fully mixed (Trifonova et al., 2021). Daily to seasonal SST values have higher variability, as SST is more rapidly affected by atmospheric variation, whereas changes in BT reflect longer-term, integrated trends in warming/cooling. Therefore, it is likely that changes in SST reflect a wider range of the daily/seasonal extremes, whilst the BT reflects steady changes over longer periods of time. Thus, it is not surprising that SST has been used as one of the most important physical parameters influencing marine ecosystems linked to ecosystem changes in the North Sea due to climate variability in the North Atlantic Oscillation (Simpson et al., 2011; Pitois et al., 2012; Lynam et al., 2017; Edwards et al., 2020; Evans and Waggitt, 2020).
On a local to fine spatial scales, there has been a lot of work to relate SST with breeding success of kittiwakes (Frederiksen et al., 2005, 2007; Scott et al., 2006; Cook et al., 2014a), with most recent studies suggesting that temperature affects the abundance and timing of sandeels and their prey, which in turn has complex implications for kittiwakes, which are surface-feeders specializing on sandeels (Eerkes-Medrano et al., 2017; Wanless et al., 2018; Régnier et al., 2019). The relationships between SST and breeding success have been documented for other seabirds as well, including northern fulmar, Arctic tern, Atlantic puffin, and European shag (Durant et al., 2006; Borstad et al., 2011; Burthe et al., 2014; Cook et al., 2014a; Howells et al., 2017). However, this mechanism of “trophic mismatch,” where warming SSTs may affect seabirds though temporal mismatch between prey availability and energy demands during breeding is still unclear and poses challenges, due to the interacting effects from other factors (e.g., weather events, ORE developments) (Mitchell et al., 2020).
Physical Indicators: Horizontal Currents Speed
Local and regional hydrodynamic characteristics and processes can influence primary productivity and occupancy patterns of mobile animals by increasing food availability and abundance (Skov and Prins, 2001; Johnston et al., 2005; Ballance et al., 2006; Markones, 2007; Embling et al., 2012; Thompson, 2013; Scales et al., 2014; Cox et al., 2016; Sadykova et al., 2017; Scherelis et al., 2020; Trifonova et al., 2021). Specifically, depth-averaged (entire water column) horizontal currents speed (Hspeed, m/s) was found to be a key variable determining the abundance of mobile top predators, as stronger currents can promote foraging opportunities (Pierpoint, 2008; Embling et al., 2010; Gilles et al., 2011; IJsseldijk et al., 2015; Lambert et al., 2017; Diaz-López and Methion, 2018), specifically within tidal stream environments (Waggitt et al., 2016a,b, 2018b; Bouveroux et al., 2020), which has important implications with respect to mitigating population-level impacts from tidal stream energy extraction. Hydrodynamic characteristics have been hypothesized to aggregate, disaggregate, and disorient prey, affecting prey availability and foraging efficiency (Scott et al., 2014; Benjamins et al., 2015; Waggitt et al., 2016a), leading to effects on the energetics of individuals with possible effects on population-level across trophic levels (Zamon, 2003; Fraser et al., 2018; Williamson et al., 2019). Such findings are important for top predators, specifically when changes in fish behavior and distribution have been found to be more important than changes in abundance (Hastie et al., 2016; Waggitt et al., 2016b). Recent findings on very fine spatial scales (<500 m) demonstrate that man-made structures (e.g., decommissioned tidal turbine) and physical features (e.g., eddies and boils) generate wake features that attract top predators, which has implications for the distribution of species and identification of foraging hotspots (Embling et al., 2012; Waggitt et al., 2016a,b; Benjamins et al., 2017; Lieber et al., 2019). Therefore, quantifying spatio-temporal variation in animal site usage in relation to hydrodynamic features may help to identify which and when areas may be used (Bertrand et al., 2014; Jones et al., 2014; Waggitt and Scott, 2014; Benjamins et al., 2015; Hastie et al., 2016; Waggitt et al., 2017).
Biological Indicators: Net Primary Production, Maximum Chlorophyll-a, and Phytoplankton Patchiness
Net primary production (NPP, mg C/m2; depth averaged NPP, that is the total carbon uptake by phytoplankton minus the phytoplankton respiration in a day) is a key driver of species dynamics from zooplankton through to top predators. Thus, it is through energy transfer along the food web, that NPP provides bottom–up control on fisheries production, identified within the North Sea and across other large marine ecosystems around the globe (Chassot et al., 2007; Conti and Scardi, 2010; Blanchard et al., 2012; Barange et al., 2014; Bentley et al., 2019; Hernvann et al., 2020). Specifically, across different regional spatial scales within the North Sea, the magnitude of NPP and its high temporal variability focused attention on NPP as a good indicator of changes felt throughout the trophic chain. The effect of NPP has enormous implications for fisheries production, given future climate and fisheries management changes (Capuzzo et al., 2018; Trifonova et al., 2021). NPP plays a significant role in determining habitat preferences and facilitating foraging for both fish prey and top predator species, highlighting that prey and predators are selecting aspects of the habitat type very differently and that might be a reflection of prey species avoiding areas with predators (Sadykova et al., 2017, 2020; Cox et al., 2018). This further supports the Longhurst Provinces approach (Vichi et al., 2011), that defining the predictability of primary production throughout the trophic chain should be taken into consideration in terms of future spatial management of anthropogenic aspects that can change mixing (Tweddle et al., 2018).
Similarly, maximum chlorophyll-a at any depth (Chl-a, mg C/m3; the highest biomass of chlorophyll found at any vertical location in the water column but generally found at the base of the pycnocline; Ross and Sharples, 2007; Scott et al., 2010; Cullen, 2015), has also been identified as a good indicator of changes felt throughout the food web and specifically, its potential role to the structure and dynamics of marine ecosystems, including the North Sea (Trifonova et al., 2021), but also other shelf seas e.g., Celtic Sea (Mérillet et al., 2020) and other large marine ecosystems (Ware and Thomson, 2005; Frank et al., 2006; Friedland et al., 2012; Kidé et al., 2015). The Chl-a can be influenced by a greater variety of weather and physical variables, enhancing the importance of specific processes of bottom-up control of the planktonic food web (Molinero et al., 2013). This suggests that locations of Chl-a are very ecologically important (Scott et al., 2010; Scales et al., 2014), as they can represent new and/or aggregated primary production, but they may not have high values of biomass and hence, do not show up as areas of high, depth integrated NPP (Hickman et al., 2012). There is evidence that increased Chl-a can be used as an indicator of areas of importance to top predators (Suryan et al., 2012; Sabarros et al., 2014; Bennison and Jessopp, 2015; Grecian et al., 2016; Nowak et al., 2020), potentially indicating that the dynamics of top predators can be predicted using a combination of temperature and first level trophic indicators (Lynam et al., 2017; Trifonova et al., 2021). In particular, on a local to fine scale, foraging behavior of Manx shearwater and guillemots has been associated with areas of high concentrations of Chl-a off the west coast of Ireland (Kane et al., 2020) and off the east coast of Scotland, respectively (Cox et al., 2013). However, the processes behind this association are yet to be fully explained (Grémillet et al., 2008; Sharples et al., 2013).
The marine environment is subject to spatial and temporal phytoplankton “patchiness,” driven by physical processes across spatial scales, e.g., upwelling along the continental shelf break or tidal mixing fronts (MacIsaac et al., 1985; Videau, 1987; Joint et al., 2001; Sharples et al., 2013). Patchiness in phytoplankton is extremely important as it affects predators’ ability to find food, therefore spatial and temporal changes in locations and/or quantity of patchiness will impact the entire trophic web (Scott et al., 2010; Benoit-Bird and McManus, 2012). Periodic phytoplankton and subsurface blooms leading to accumulated new “patchy” production, greater than usually assumed in some regions (e.g., Dogger Bank) might explain the high fish production in the North Sea, compared to other temperate seas (Richardson et al., 2000; Weston et al., 2005). Such high production areas have been reported along the shelf edge in the north-eastern North Sea, coincidental with high species richness and fishing activity, which could have implications for the ecosystem structure (Bendtsen and Richardson, 2020). In addition, the timing (phenology) of the phytoplankton blooms has been highlighted important for the function of food webs on a global scale, specifically affecting energy flow pathways in ecosystems and the productivity of different species (Friedland et al., 2018).
Biological Indicators: Predator–Prey Interactions
Studying predator–prey interactions should bring extra knowledge about consequences for the dynamics of marine ecosystems in a bio/physical environment that is changing rapidly. In marine ecosystems, top predator populations are influenced by environmental factors that affect their prey abundance and distribution at multiple spatial scales and are often used as indicators (Bertrand et al., 2008; Wolf et al., 2009; Boyd et al., 2017; Carroll et al., 2017; Figure 5). Sadykova et al. (2020) showed the complex relationship between the change in physical habitat, following climate warming and predator–prey interactions (including competition for prey between gray and harbor seals), with the more severe population declines predicted in harbor seals (SCOS, 2019; Evans and Waggitt, 2020). The spatio-temporal overlap between cod and its predators increased with increasing temperature, indicating that food web processes might reduce the recovery potential of cod during warm periods (Kempf et al., 2010). Seasonal changes in migration patterns, stock sizes and locations of prey, due to climate warming, has had an influential role in controlling the movements of killer whales off northern Scotland (Samarra and Foote, 2015) and the large north to south distribution shift of the harbor porpoise within the southern North Sea (Camphuysen, 2004; Camphuysen and Peet, 2006; Evans and Waggitt, 2020) and might also be partly responsible for the increase in sightings of humpback whales in the north-western North sea (Evans and Bjørge, 2013). At the same time, prey may be abundant but not accessible due to removal by fisheries, which has often resulted in population-level effects (Cury et al., 2011; Bertrand et al., 2012; Barbraud et al., 2018), ecosystem shifts and consequent reversal of predator–prey roles, where adult prey attack vulnerable young predators (Frank et al., 2005; Heithaus et al., 2008; Fauchald, 2010; Estes et al., 2011). For example, the North Sea cod recruitment has been negatively related to the spawning-stock biomass of herring, as they are found to prey on cod eggs and larvae (Fauchald, 2010), and also exacerbated by climate-induced declines in the plankton production, which is a major food source for cod larvae (Beaugrand et al., 2003; Edwards et al., 2020). The identified impact of predators (e.g., cod, saithe, and gray seals) on juvenile cod and whiting advocated the use of ecosystem modeling alongside single stock assessments to better inform fisheries management in the west of Scotland region (Baudron et al., 2019).
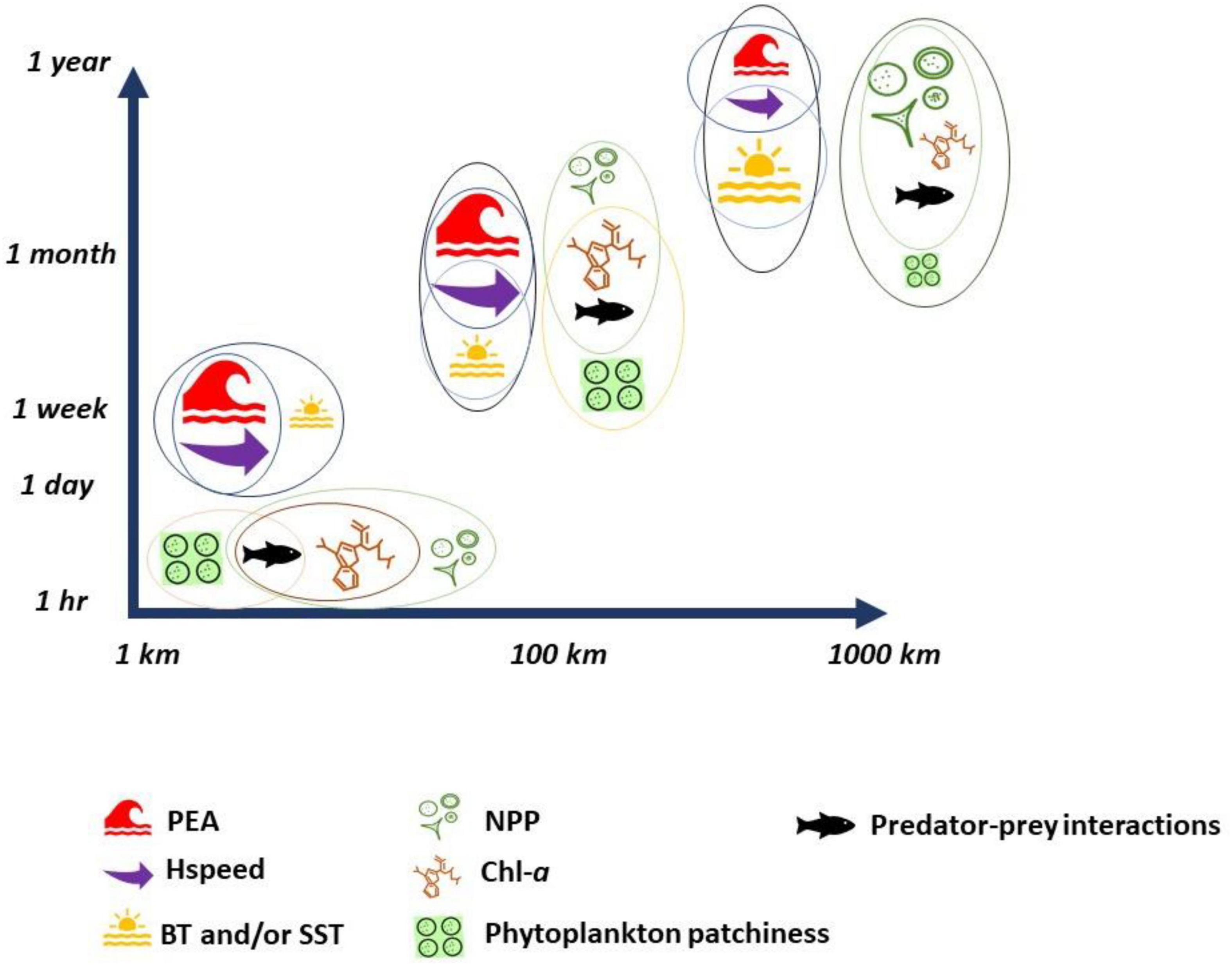
Figure 5. Physical and biological indicators and their overlap (represented by colored circles) across spatial and temporal scales. The size of the symbol represents the relevant “importance” of each indicator at each scale, which was determined, based on all the reviewed literature for this study.
It is evident that changes in the population dynamics of higher trophic levels are likely to reflect those of their preferred prey, which may, in turn, be bottom–up driven by dynamic bio-physical oceanographic processes across spatial and temporal scales (Shealer, 2002; Bertrand et al., 2014; Boyd et al., 2015; Woodson and Litvin, 2015; McInnes et al., 2017; Cox et al., 2018; Figure 5). For example, on a local scale, by accounting for the influence of tidal state and stratification on foraging habitat, seabird–fish interactions were identified, highlighting that critical marine habitats occur at limited spatial locations but also within specific temporal intervals (Cox et al., 2013; Calle et al., 2018). The extent to which spatial and tidal temporal oceanographic features are important to seabirds is dependent on the use of such features by their prey (Daunt et al., 2006; Stevick et al., 2008; Heim et al., 2019). On a fine spatial scale, prey behavior and availability and/or predictability in tidal stream environments may be more important in attracting predators than absolute prey abundance (Zamon, 2002; Grémillet et al., 2008; Skov and Thomsen, 2008; Benoit-Bird et al., 2013; Wilson et al., 2013; Benjamins et al., 2016) and that memory of profitable areas can also play an important role in deterministic selection of foraging habitat (Nabe-Nielsen et al., 2013; Regular et al., 2013). This might explain why the relationship between foraging seabirds and harbor porpoise with current speeds sometimes differs between studies (Booth et al., 2013; Jones et al., 2014; Scott et al., 2014; Benjamins et al., 2015, Benjamins et al., 2017) and highlights the importance of including prey when looking at the behavior and distribution of top predators. For example, prey transitional behavior moving between habitats, due to mixed and stratified water, has been found important for top predators, suggesting that predators may select foraging habitats by trading-off several measurements of prey availability (Waggitt et al., 2018a,b).
What Have We Learned From Marine Ecosystem Modeling Approaches for Climate Change and Offshore Renewable Energy Developments?
Introduction of the Different Modeling Approaches
Significant progress has been made in developing ecosystem models that use traditional statistical approaches to understand the relationships between a number of variables (Lynam et al., 2017), including “end-to-end” dynamic ecosystem models to predict impacts of environmental change on the structure and function of marine food webs and the services they provide (Marine Ecosystems Research Programme1; Spence et al., 2018). The model developed in Lynam et al. (2017) is a particularly good example as it uses a non-deterministic approach that incorporated different trophic levels from over 40 years on a North Sea spatial scale. The model linked top–down and bottom–up effects simulating complex long-term changes under a combination of stressor scenarios for the North Sea marine system, providing useful outcomes to support the ecosystem approach to management. Size-based ecosystem modeling is also emerging as a powerful way to assess ecosystem-level impacts of natural and anthropogenic changes from individual-level processes (Barange et al., 2014; Blanchard et al., 2017). In particular, a size-based food web model coupled with a physical-biogeochemical model was used to investigate the impacts of climate change on fish biomass and primary production (Blanchard et al., 2012).
Ecopath with Ecosim (EwE) models (Polovina, 1984; Christensen and Walters, 2000) are also useful to capture the impacts, on a relatively local spatial scale, assessing wind farm “before” and “after” effects and one was developed for the Bay of Seine ecosystem (Raoux et al., 2017). This was the first holistic approach to examine the offshore wind farm impacts on ecosystems functioning using trophic web modeling tools. The approach successfully determined how the local food web structure and function may change 30 years after the installation of the offshore wind farm, examining changes from the “reef effect” on apex predators and highlighting the “wasp-waist” controlled food webs (Raoux et al., 2017).
Species-specific individual-based models (IBMs) have been developed to quantify impacts from ORE developments (DEPONS, Nabe-Nielsen et al., 2018; SeabORD, Searle et al., 2014), however, they require highly detailed spatial information and are currently under improvement. Models, such as AgentSeal, are the first step in providing solid understanding of animals’ physiology, movement, and behavior, that can be integrated into IBMs to understand cumulative effects of stressors (Chudzinska et al., 2021). At the population-level, a promising avenue for future research includes the use of IBMs with telemetry data to better understand population dynamics and predict population-level distribution, following changes to species environment (McClintock et al., 2013; Carroll et al., 2020).
An EwE approach was also applied to model ecosystem effects of fishing and climate warming in the North Sea, highlighting the interactions between predator-prey and climate change, which will have significant implications for fisheries management (Lynam and Mackinson, 2015). The EwE approach was also developed on a much finer spatial scale for the west coast of Scotland to assess the combined impacts of fishing and rising temperature on selected species. Modeling outcomes revealed that gray seals, cod, herring, and haddock were facing severe declines, whilst some species (e.g., whiting) were not affected, highlighting the importance of including rising temperatures in the ecosystem approach to achieve sustainable fisheries management (Serpetti et al., 2017). While the EwE model cannot, in its present state, be used for short-term decisions due to too many uncertainties relating to the numerous processes it encompasses, the approach might be useful in terms of informing managers on likely future long-term trends in biomass and catches under various “what if” scenarios, such that this approach is currently being used to investigate small-scale impacts of multi-purpose platforms (Serpetti et al., 2017).
Bayesian network models have also been applied to investigate the consequences of fisheries catch, temperature and primary productivity scenarios on different fish and zooplankton species, outlining the importance of trophic interactions and the spatial relationship between neighboring areas (Trifonova et al., 2017). The success of using a hidden variable to identify indicator species of key importance to the ecosystem dynamics that has also been helpful in illuminating the possible mechanisms behind functional ecosystem changes has been shown for the North Sea (Trifonova et al., 2015) but also for other systems, e.g., Barents Sea (Uusitalo et al., 2018) and Gulf of Mexico (Trifonova et al., 2019). Bayesian hierarchical hurdle and zero-inflated joint models with integrated nested Laplace approximation (INLA) have been used to model the common spatial habitat between predators and prey and more recently, to predict the “ecological costs” in population terms, following the projected effects of climate change (Sadykova et al., 2020).
New Modeling Formulations, How to Effectively Combine Outputs From Different Approaches, Do Not Create New Models but Use Different Approaches Effectively Together
New modeling formulations might be needed to be able to assess multiple stressors, account for behavior of species, demographic data, as well as predator-prey interactions occurring at different spatial and temporal scales. Machine-learning and bioinformatics techniques can be greatly utilized to aid such work due to their “crossover potential” in predictive ecology (Tucker and Duplisea, 2012), including animal behavior and movement (Mattei et al., 2018; Pirotta et al., 2018). Coupling existing models (e.g., physical, biological, economic) is another avenue that has proven successful (Planque et al., 2011; Robinson et al., 2011; Kempf et al., 2013; Peck et al., 2018). Mechanistic food-web model and Bayesian hierarchical species distribution model have been used complementary to take a more holistic approach to estimating species distributions (Coll et al., 2019). Another alternative could be nested sub-models, based on principles from graph and network theory that can be used to overcome scale issues more easily by linking up to existing ecosystem or species-based models. For example, by using bipartite network principles to create species-habitat networks, the scale of the ecological information was matched to the scale of the landscape management intervention to assist landscape management and multispecies conservation (Marini et al., 2019).
A useful future approach would be to use the interaction web as an emulator for a more complex model (Lynam et al., 2017) and consider an ensemble modeling approach (Gårdmark et al., 2013; Spence et al., 2018). Rather than trying to choose the “best” model, or taking some weighted average, it is important to exploit the strengths of each of the types of models, whilst learning from the differences between them, as it was shown in an ensemble model that was developed based on the relationships between a collection of mechanistic models (e.g., EwE), allowing for uncertainty to be included when making predictions under different scenarios (Spence et al., 2018; Figure 6). The approach was conducted to evaluate the impact of fishing on demersal fish species; however, the framework is not exclusive to ecosystem simulators in fisheries and is potentially applicable over a wide scope of complex ecological systems.
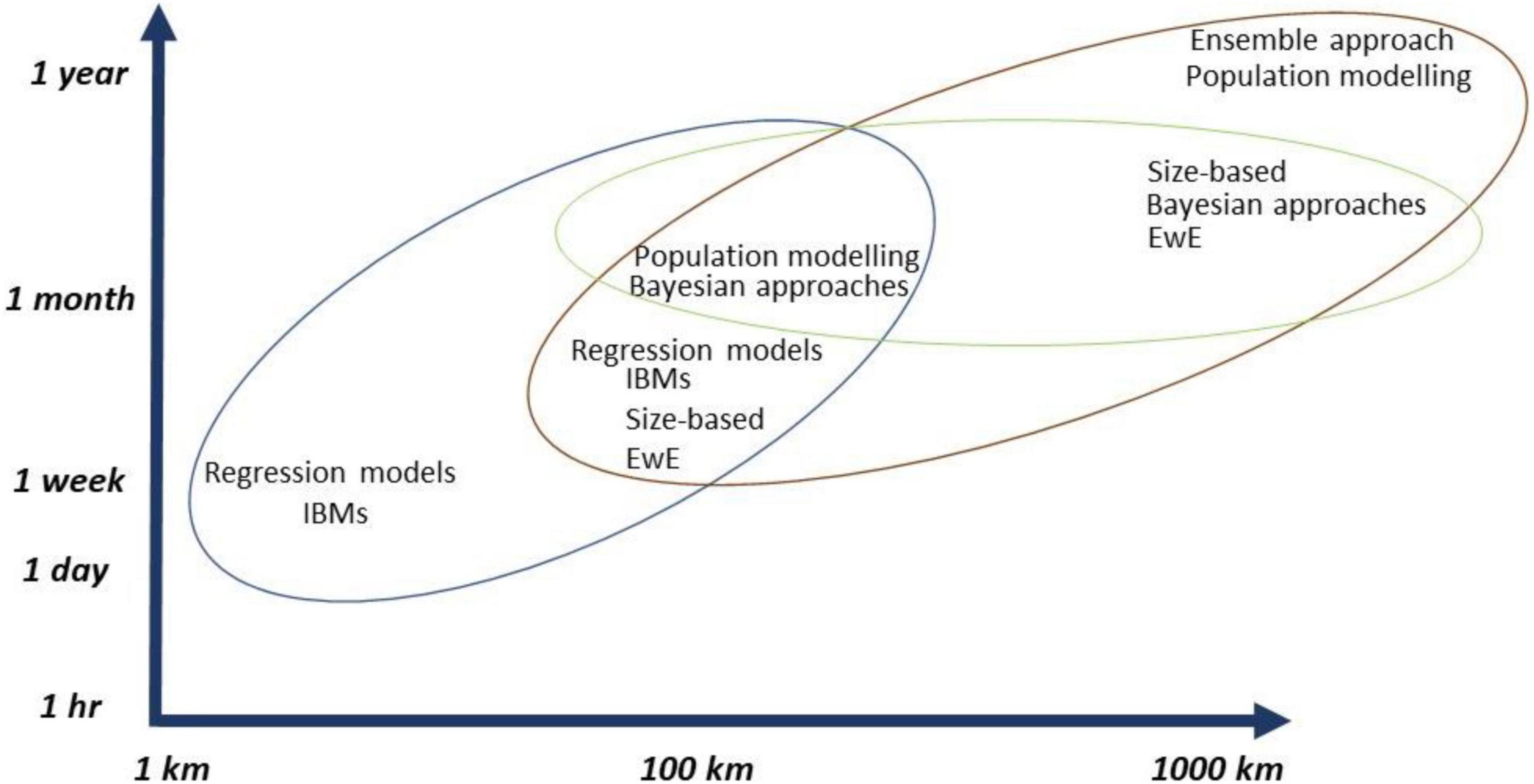
Figure 6. Marine ecosystem modeling approaches for climate change and ORE developments and their overlap across spatial and temporal scales. The colored circles are used to represent which groups of models work best together across spatial and temporal scales, which was determined, based on all the reviewed literature for this study.
How to Improve on Integration Across Spatial and Temporal Scales
Greater integration of both physical and biological indicators will potentially allow identification of the important biophysical interactions within different habitats that will allow evaluation of how ecosystems might change in the future. The optimal approach is to utilize knowledge across a range of spatial and temporal scales to be able to reduce uncertainties of direct and indirect effects on individuals and subsequently populations and ultimately, to provide sensible strategic advice for future sustainable spatial management. Most importantly, studies need to be performed at appropriate ecological scales, considering the spatial and temporal physical and biological variability, and recognizing the pragmatic importance of asking the “right question” at the “right scale” (Mannocci et al., 2017; Scales et al., 2017).
How to Improve Connection Between Scales (Better Use of Data, Fine Scale Studies Versus Large-Scale, Generalization Across Regions and Scales)
Focusing on explanatory physical and/or biological indicators, which can be quickly quantified across spatial and temporal scales, can be a pragmatic approach to generalize relationships (e.g., predator–prey and/or biophysical interactions) across marine systems but from different geographic regions, where data is limited (Tucker and Duplisea, 2012). In this way, local and regional studies from data rich areas (e.g., Moray Firth in northeast Scotland, ScotMER and MREF MASTS Workshop October 5, 20192; coastal monitoring sites3) should be explored in more detail to provide a robust understanding of the biophysical interactions across trophic levels and any changes that are affecting the ecosystem dynamics. By relying on the efficient and robust use of data from such well-studied areas, biophysical relationships can be confirmed and predicted for other habitats to generalize the relative influence from climate and other factors to support population-level studies across larger scales. Therefore, finer, local scale and site-specific surveys and monitoring only need to be conducted on few key demonstrator habitats focusing on interactions with few key species to build a baseline for the understanding of the North Sea (Bailey et al., 2014).
Data obtained from few key demonstrator sites can be integrated with site-specific data at proposed ORE development sites to provide a more robust understanding of population-level consequences and potentially identify alternative more suitable potential sites (Bailey et al., 2014). Hydrodynamic models are routinely used to assess energy resources and environmental impacts on hydrodynamics, which in combination with site-specific data, would allow useful information to be collected relatively cheaply and easily. The challenge for modeling studies is to validate the models sufficiently for present-day conditions, so that we can have confidence in the results under assumptions about the response to deployment of ORE devices, which cannot yet be validated from observations (Wolf et al., 2021). If effort and resources were to be focused on selected species and seasons, post-installation monitoring and mitigation might be more clearly and easily managed. In this way, knowledge can be used to optimize site development and purpose, balancing benefits and trade-offs between the rapidly growing ORE industry and conservation.
Individual-based studies and short-term interactions can serve as a baseline to assess how particular pressures interact with broader scale variation in environmental conditions (Votier et al., 2005) or over time (Veran et al., 2007) to support a more general understanding of the long-term population consequences. Such baseline studies will also contribute to determine the spatial scales at which to further investigate more detailed prey and predator behaviors within sites of interest. For example, demographic and fecundity data can be used to assess the longer-term impact of any behavioral responses, following natural or anthropogenic changes (Boyd et al., 2015, 2017; Carroll et al., 2017; Barbraud et al., 2018). Then, quantification of fine scale usage can be utilized within population level models through generalized functional response methods and/or influences on energetic changes to understand how these individual effects translate into population-level changes at a wider spatial scale and ecosystem level (Matthiopoulos et al., 2015; Figure 6).
Different Sources of Data, Different Survey Techniques
With a greater mechanistic understanding of how and why fish and top predators use fine scale dynamic marine habitats (i.e., high-energy tidal sites, Hastie et al., 2018; use of wind turbine structures, Whyte et al., 2020) for foraging, predictable changes from the installation of ORE developments can be used to estimate cumulative effects at a population level and guide strategic approach to the monitoring and management of such devices (Williamson et al., 2017, 2019). However, very few studies are performed on a fine spatial scale and concurrent measurements of physical and biological data are lacking. Achieving this will require adopting novel methodological techniques (e.g., acoustics, underwater photography, cameras, gliders, telemetry) to improve our understanding of habitat use by marine animals to be able to link biophysical processes at a population level (Cotter and Polagye, 2020; Polagye et al., 2020; Whitt et al., 2020). However, given the cost and capacity related to data collection, existing sources of information should be optimized and their relevance to new data evaluated.
Existing data (e.g., Copernicus4; INSITE5; MEDIN6; Seabird and mammal population data, Waggitt et al., 2020; Oil and gas environment interactive7) should be integrated more and used to populate multiple approaches (from IBMs to population-level approaches) across spatio-temporal scales. Similarly, datasets such as ScotMap (Kafas et al., 2014) should be utilized more to investigate forage fish distribution across scales due to their central place in the North Sea marine system, linking bottom–up and top–down processes.
Remote sensing data (e.g., SeaWiFS8) offer a much extended spatial and temporal coverage but presenting some limitations related to image resolution and targets (Patil et al., 2002). Still, analyses of such data can be used in combination with field observations and expertise knowledge as an effective tool to assist the understanding of complex interactive biophysical effects within the environment and minimize uncertainty with future climate change to aid the evidence-based decision-making process (Patil et al., 2002; Moufaddal, 2005). In addition, physical and biological variables from remote sensors could be used as proxies to better understand foraging behavior and habitat preferences of animals across scales and how these vary with weather conditions and climate change (Medina-Lopez et al., 2021). Data from GPS and accelerometers of top predators can be used to investigate fine scale foraging locations and consequently determine prey behavior and distribution across trophic levels and scales that can advance the understanding of marine ecosystem dynamics (Ohman et al., 2013; Suberg et al., 2014).
New hydrodynamic modeling products, like the Scottish Shelf Waters Reanalysis Service9 are becoming available. The outstanding feature of the Service is the use of an unstructured mesh, which allows the reproduction of Scottish shelf waters dynamics that have a diverse character, from deep, almost enclosed coastal lochs on the west coast, with many islands, to the outer shelf. It fills the gap of large-scale modeling that does not have sufficient spatial resolution to resolve ORE sites. The multi-year run enables the analysis of inter-annual natural changes of the system to be compared with ORE impacts.
Moving Forward
Many of the physical and biological indicators, presented in this work, show similar levels of importance from fine through to larger spatial scales, highlighting indicators such as stratification with mechanisms via bottom-up control driving the marine ecosystem dynamics in the North Sea. This study illuminates the feasibility of generalizing knowledge from such a well-studied region and the potential to translate it across other large marine ecosystems to be able to address the importance of indicators and to ultimately strengthen predictions of population changes at ecosystem scales in other shallow shelf seas. It is with the added baseline knowledge from this study, on what ecosystem components are more consistently found as the physical and biological indicators of habitat and ecosystem change and what they are telling us about how the ecosystem is functioning, that much more informed choices for MSP decisions should be made. The knowledge presented in this study can be used for future guidance on the most sensible unit of measure (mean, median, or percentile) for both physical and biological indicators, given the specific spatial and temporal scales. Although, the mean is the mostly used unit across studies, examples illustrate that in some cases, other units have a more meaningful interpretation, for example maximum values of currents speed (Trifonova et al., 2021), maximum Chl-a (Scott et al., 2010). This work has also shown that the strength of the biophysical relationships may vary due to both strong climate and anthropogenic pressures such as fisheries, which is most important when interpreting and extrapolating relationships between animals and environmental variables (Mannocci et al., 2017; Scales et al., 2017). Thus, considering the variability of biophysical relationships at multiple spatial and temporal scales throughout different trophic levels is key to providing more accurate predictions of breeding success and survival of contrasting seabird, mammal and fish populations and ecosystem level changes these differences are driving (Figure 7).
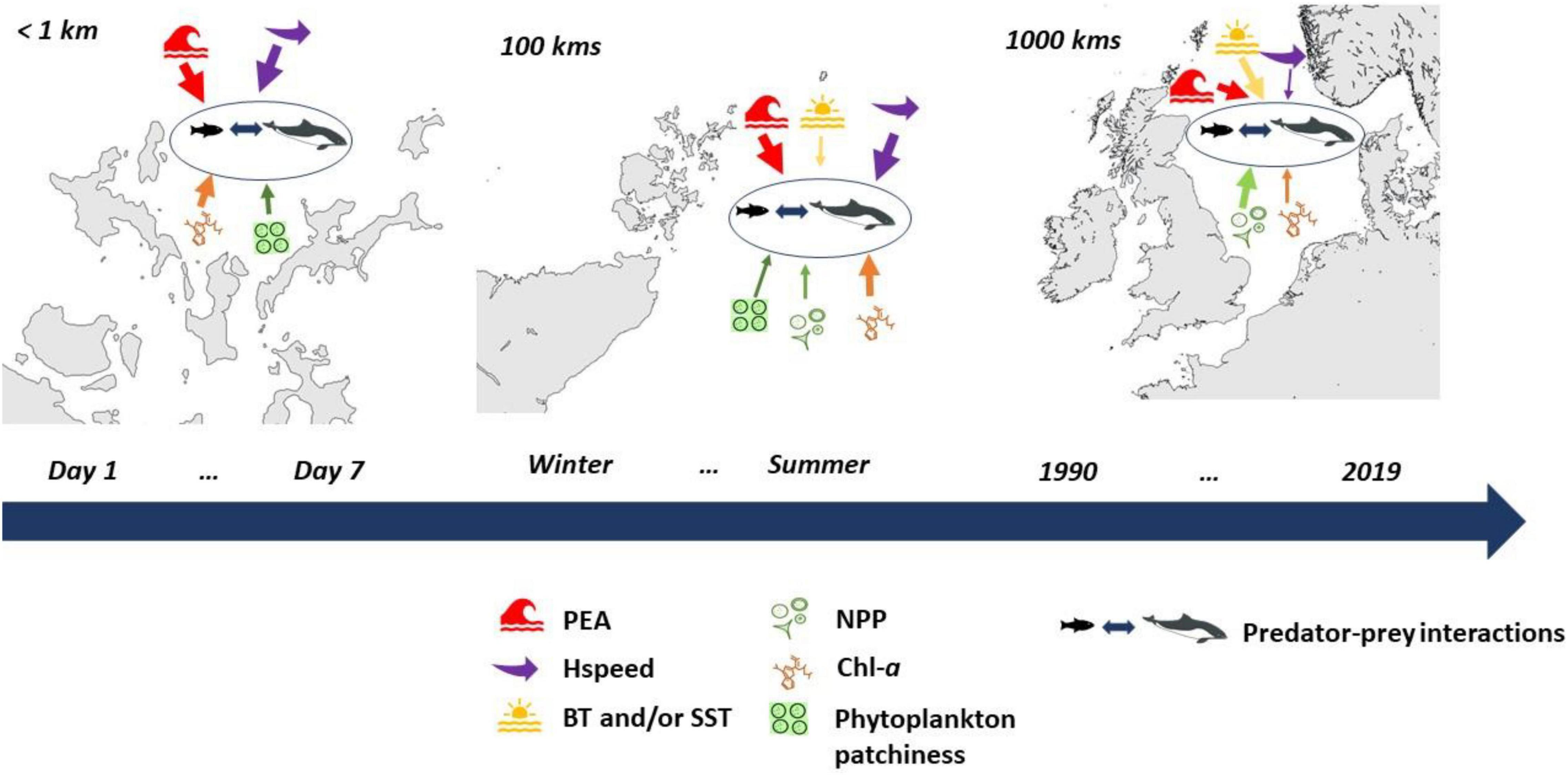
Figure 7. Illustration of the variability of biophysical relationships (represented by the arrows) across spatial and temporal scales. Note, the width of the arrows represents the relevant “importance” of the indicator on the relationship at each scale, which was determined based on all the reviewed literature for this study.
Therefore, it is important that fine scale site-specific surveys and multidisciplinary research with simultaneous collections of oceanographic data, prey and predator characteristics are performed on key demonstrator sites and then, quantified across spatial scales to support a more general understanding of the important biophysical relationships to populations and regional wide ecosystem scale. We suggest that more general patterns of relationships should be aimed at, whilst effort should be emphasized on the integral “habitat” use and specifically, determine what aspects of the habitat are relevant to marine animals to be able to address a wider range of ecological questions. For example, by quantifying the effects of habitat preference and spatial usage, the effects of species interactions (e.g., competition, predation) on populations can be better understood. By investigating locations, where predator and prey or competing species overlap, we can better monitor and mitigate the potential natural and anthropogenic consequences on the marine environment (Williamson et al., 2021). This will allow for future-proofing of the locations of MPAs and improve decisions, such as where ORE developments should be placed.
A step-change is occurring in the use of our shallow seas, specifically by the addition of large-scale ORE developments, in part driven by the United Kingdom government aspiration to achieve Net Zero by 2050 and the Green Industrial Revolution. To ensure the compatibility of ORE developments with other marine management sectors, we need to evaluate cumulative effects through cumulative impact assessments (Cavallo et al., 2017; Willsteed et al., 2018). However, cumulative assessments currently fail to include the linkages between different trophic levels, and the interactions between pressures, such as changes in the physical environment from the introduction of structures and extraction of GWs of energy up through the entire marine ecosystem. The cumulative environmental effects of ORE developments remain highly uncertain and require a multidisciplinary approach to provide more holistic and structured assessments (Willsteed et al., 2017). Using ecosystem models at ecologically meaningful scales to understand how ecosystems respond to multiple stressors will support the cumulative assessment process (Piroddi et al., 2015). To address that and to incorporate both direct and indirect effects on the ecosystem, ecosystem models need to be linked with a hydrodynamic-biogeochemical-sediments modeling system (Schuchert et al., 2018; Wolf et al., 2021). The study by van der Molen et al. (2016) is the only study that has integrated hydrodynamic-sediments-biogeochemistry effects. They found that a very large array of 8 GW located in the Pentland Firth (United Kingdom) can lead to changes in ecosystem variables of up to 10% at more than 100 km away from the array.
Ideally, holistic modeling approaches should be integrated at ecologically meaningful spatial and temporal scales in response to natural variability and /or anthropogenic pressures. Ecological and socio-economic effects need to be combined in one modeling framework to enable assessment of trade-offs between different uses of coastal seas. In fact, a common “currency” to communicate modeling outputs could be developed that works across different disciplines and stakeholder groups. Most ecosystem models use some aspect of biological biomass or numbers of animals, or ratios of size of predator to prey as the currency in the model. However, many industries and regulators use the level of risk as the central currency in decision making. A coming together of currencies could be used to communicate modeling outputs with different groups for a more coherent and comprehensive understanding of environmental perturbations following ORE developments and climate change. This way, a common “currency” could be the NPP or the amount of energy per kg (the energy flow or availability of energy, derived from key trophic interactions).
Natural capital is a method that has been adopted to achieve the use of financial currency across modeling frameworks. The natural capital approach provides a more holistic perspective of socio-ecological systems than traditional environmental impact assessment (Hooper et al., 2017). It is a useful tool to support impact assessment on the goods and services people receive from nature (Arkema et al., 2015; Guerry et al., 2015). Such a framework will also provide the likely outcomes from alternative management and climate scenarios, allowing the user to make judgments and decisions about the ecological and socio-economic benefits and trade-offs within spatial scales, among sectors, and between users. Such information will ideally support the communication with other sectors, both the conservation and financial sectors, and guide policy decisions and planning.
Lastly, a focus should be placed on the development of interactive visualization tools (such as InVEST10; MSP Simulation Platform11; Virtual Ecosystem Scenario Viewer12) that assist in conveying complex outputs from state-of-the-art modeling tools, discussed already in the text. Modeling outputs should be brought together and better integrated for better communication and interactions with stakeholders to be able to represent and understand current and future trade-offs, following natural and/or anthropogenic changes across spatial scales for whole ecosystem levels. Therefore, different expertise can have a quantitative indication of the range of possible scenarios consistent with the data to give strategic advice on potential ecosystem response. In this way, we can proceed more rapidly than before by utilizing knowledge and using existing extensive data sources, predictive modeling approaches and visualization tools that will ultimately lead to more strategic and integrated approaches to both monitoring studies and assessing anthropogenic impacts to enable evaluation of trade-offs and benefits to provide the most sustainable future spatial use of our seas at whole ecosystem scales.
Author Contributions
NT designed the study, performed the research, and wrote the manuscript. BS designed the study, wrote, reviewed, and edited the manuscript. MD, and JW wrote, reviewed, and edited the manuscript. All authors contributed to the article and approved the submitted version.
Funding
This work was supported by the Supergen Offshore Renewable Energy (ORE) Hub, funded by the Engineering and Physical Sciences Research Council (EPSRC EP/S000747/1).
Conflict of Interest
The authors declare that the research was conducted in the absence of any commercial or financial relationships that could be construed as a potential conflict of interest.
Publisher’s Note
All claims expressed in this article are solely those of the authors and do not necessarily represent those of their affiliated organizations, or those of the publisher, the editors and the reviewers. Any product that may be evaluated in this article, or claim that may be made by its manufacturer, is not guaranteed or endorsed by the publisher.
Acknowledgments
The authors would like to thank the following people for providing original images, incorporated in this work: Rory O’Hara Murray (Marine Scotland Science, United Kingdom), Ella-Sophia Benninghaus and Morgane Declerck (University of Aberdeen, United Kingdom).
Footnotes
- ^ https://www.marine-ecosystems.org.uk/Research_outcomes/Modelling
- ^ https://tethys.pnnl.gov/sites/default/files/publications/working-across-sector-groups_scotmer-masts-workshop_2019_final.pdf
- ^ http://marine.gov.scot/information/coastal-monitoring-site-stonehaven
- ^ https://www.copernicus.eu/en/access-data
- ^ https://www.insitenorthsea.org/data/
- ^ https://portal.medin.org.uk/portal/start.php
- ^ https://storymaps.arcgis.com/stories/4b6d3b5867b14bb7aecf6f6557a5fb82
- ^ https://oceancolor.gsfc.nasa.gov/SeaWiFS/
- ^ https://sites.google.com/view/ssw-rs/home
- ^ https://naturalcapitalproject.stanford.edu/software/invest
- ^ https://www.mspchallenge.info/
- ^ https://www.fisheries.noaa.gov/resource/tool-app/virtual-ecosystem-scenario-viewer-ves-v
References
Abrahms, B., Scales, K. L., Hazen, E. L., Bograd, S. J., Schick, R. S., Robinson, P. W., et al., (2018). Mesoscale activity facilitates energy gain in a top predator. Proc. R. Soc. B 285:20181101. doi: 10.1098/rspb.2018.1101
Afsharian, S., and Taylor, P. A. (2019). On the potential impact of lake erie wind farms on water temperatures and mixed-layer depths: some preliminary 1-D modelling using COHERENS. J. Geophys. Res. Oceans 124, 1736–1749.
Arkema, K. K., Verutes, G. M., Wood, S. A., Clarke-Samuels, C., Rosado, S., Canto, M., et al., (2015). Embedding ecosystem services in coastal planning leads to better outcomes for people and nature. Proc. Natl. Acad. Sci. 112, 7390–7395. doi: 10.1073/pnas.1406483112
Bailey, H., Brookes, K. L., and Thompson, P. M. (2014). Assessing environmental impacts of offshore wind farms: lessons learned and recommendations for the future. Aquatic Biosyst. 10, 1–13. doi: 10.1186/2046-9063-10-8
Ballance, L. T., Pitman, R. L., and Fiedler, P. C. (2006). Oceanographic influences on seabirds and cetaceans of the eastern tropical pacific: a review. Prog. Oceanogr. 69, 360–390. doi: 10.1016/j.pocean.2006.03.013
Barange, M., Merino, G., Blanchard, J. L., Scholtens, J., Harle, J., Allison, E. H., et al., (2014). Impacts of climate change on marine ecosystem production in societies dependent on fisheries. Nat. Clim. Change 4, 211–216. doi: 10.1111/gcb.14512
Barbraud, C., Bertrand, A., Bouchón, M., Chaigneau, A., Delord, K., Demarcq, H., et al., (2018). Density dependence, prey accessibility and prey depletion by fisheries drive Peruvian seabird population dynamics. Ecography 41, 1092–1102.
Baudron, A. R., Serpetti, N., Fallon, N. G., Heymans, J. J., and Fernandes, P. G. (2019). Can the common fisheries policy achieve good environmental status in exploited ecosystems: the west of Scotland demersal fisheries example. Fisher. Res. 211, 217–230.
Beaugrand, G., Brander, K. M., Lindley, J. A., Souissi, S., and Reid, P. C. (2003). Plankton effect on cod recruitment in the north sea. Nature 426, 661–664. doi: 10.1038/nature02164
Behrenfeld, M. J., O’Malley, R. T., Siegel, D. A., McClain, C. R., Sarmiento, J. L., Feldman, G. C., et al., (2006). Climate-driven trends in contemporary ocean productivity. Nature 444, 752–755. doi: 10.1038/nature05317
Bendtsen, J., and Richardson, K. (2020). New production across the shelf-edge in the northeastern north sea during the stratified summer period. J. Mar. Syst. 211:103414.
Benjamins, S., Dale, A., van Geel, N., and Wilson, B. (2016). Riding the tide: use of a moving tidal-stream habitat by harbour porpoises. Mar. Ecol. Prog. Ser. 549, 275–288. doi: 10.3354/meps11677
Benjamins, S., Dale, A. C., Hastie, G., Waggitt, J. J., Lea, M. A., Scott, B., et al., (2015). Confusion reigns? A review of marine megafauna interactions with tidal-stream environments. Oceanogr. Mar. Biol. Ann. Rev. 53, 1–54. doi: 10.1201/b18733-2
Benjamins, S., van Geel, N., Hastie, G., Elliott, J., and Wilson, B. (2017). Harbour porpoise distribution can vary at small spatiotemporal scales in energetic habitats. Deep Sea Res. Part II Top. Stud. Oceanogr. 141, 191–202. doi: 10.1016/j.dsr2.2016.07.002
Bennison, A., and Jessopp, M. (2015). At-sea surveys confirm a North Atlantic biodiversity hotspot. Bird Study 62, 262–266. doi: 10.1080/00063657.2015.1011601
Benoit-Bird, K. J., Battaile, B. C., Heppell, S. A., Hoover, B., Irons, D., Jones, N., et al., (2013). Prey patch patterns predict habitat use by top marine predators with diverse foraging strategies. PLoS one 8:e53348. doi: 10.1371/journal.pone.0053348
Benoit-Bird, K. J., and McManus, M. A. (2012). Bottom-up regulation of a pelagic community through spatial aggregations. Biol. Lett. 8, 813–816. doi: 10.1098/rsbl.2012.0232
Bentley, J., Serpetti, N., Fox, C., Reid, D., and Heymans, J. J. (2019). Modelling the Food Web in the Irish Sea in the Context of a Depleted Commercial Fish Community.: Part 2: ICES Ecopath with Ecosim Key Run, SAMS Report No. 294. Oban: Scottish Association for Marine Science.
Bertrand, A., Gerlotto, F., Bertrand, S., Gutiérrez, M., Alza, L., Chipollini, A., et al., (2008). Schooling behaviour and environmental forcing in relation to anchoveta distribution: an analysis across multiple spatial scales. Prog. Oceanogr. 79, 264–277. doi: 10.1016/j.pocean.2008.10.018
Bertrand, A., Grados, D., Colas, F., Bertrand, S., Capet, X., Chaigneau, A., et al., (2014). Broad impacts of fine-scale dynamics on seascape structure from zooplankton to seabirds. Nat. Commun. 5, 1–9. doi: 10.1038/ncomms6239
Bertrand, S., Joo, R., Arbulu Smet, C., Tremblay, Y., Barbraud, C., and Weimerskirch, H. (2012). Local depletion by a fishery can affect seabird foraging. J. Appl. Ecol. 49, 1168–1177. doi: 10.1111/j.1365-2664.2012.02190.x
Blanchard, J. L., Heneghan, R. F., Everett, J. D., Trebilco, R., and Richardson, A. J. (2017). From bacteria to whales: using functional size spectra to model marine ecosystems. Trends Ecol. Evolu. 32, 174–186. doi: 10.1016/j.tree.2016.12.003
Blanchard, J. L., Jennings, S., Holmes, R., Harle, J., Merino, G., Allen, J. I, et al., (2012). Potential consequences of climate change for primary production and fish production in large marine ecosystems. Philos. Trans. R. Soc. B Biol. Sci. 367, 2979–2989. doi: 10.1098/rstb.2012.0231
Boon, A., Caires, S. I, Wijnant, L., Verzijlbergh, R., Zijl, F., Schouten, J. J., et al., (2018). The Assessment of System Effects of Large-Scale Implementation of Offshore Wind in the Southern North Sea Technical Report number: 11202792-002-ZKS-0006.
Booth, C. G., Embling, C., Gordon, J., Calderan, S. V., and Hammond, P. S. (2013). Habitat preferences and distribution of the harbour porpoise phocoena phocoena west of scotland. Mar. Ecol. Prog. Ser. 478, 273–285. doi: 10.3354/meps10239
Borstad, G., Crawford, W., Hipfner, J. M., Thomson, R., and Hyatt, K. (2011). Environmental control of the breeding success of rhinoceros auklets at triangle Island. Br. Columbia Mar. Ecol. Prog. Ser. 424, 285–302. doi: 10.3354/meps08950
Bost, C. A., Cotté, C., Bailleul, F., Cherel, Y., Charrassin, J. B., Guinet, C., et al., (2009). The importance of oceanographic fronts to marine birds and mammals of the southern oceans. J. Mar. Syst. 78, 363–376. doi: 10.1038/s41598-018-35975-3
Bouveroux, T., Waggitt, J. J., Belhadjer, A., Cazenave, P. W., Evans, P. G., and Kiszka, J. J. (2020). Modelling fine-scale distribution and relative abundance of harbour porpoises in the southern bight of the north sea using platform-of-opportunity data. J. Mar. Biol. Assoc. U.K. 100, 481–489.
Boyd, C., Castillo, R., Hunt, G. L. Jr., Punt, A. E., VanBlaricom, G. R., Weimerskirch, H., et al., (2015). Predictive modelling of habitat selection by marine predators with respect to the abundance and depth distribution of pelagic prey. J. Animal Ecol. 84, 1575–1588. doi: 10.1111/1365-2656.12409
Boyd, C., Grünbaum, D., Hunt, G. L. Jr., Punt, A. E., Weimerskirch, H., and Bertrand, S. (2017). Effects of variation in the abundance and distribution of prey on the foraging success of central place foragers. J. Appl. Ecol. 54, 1362–1372. doi: 10.1111/1365-2664.12832
Boyer, T. P., Antonov, J. I., Baranova, O. K., Coleman, C., Garcia, H. E., Grodsky, A., et al. (2013). World Ocean Database 2013, NOAA Atlas NESDIS 72, eds S. Levitus and A. Mishonov (Silver Spring, MD: National Oceanic and Atmospheric Administration), 209. doi: 10.7289/V5NZ85MT
Burthe, S. J., Wanless, S., Newell, M. A., Butler, A., and Daunt, F. (2014). Assessing the vulnerability of the marine bird community in the western North Sea to climate change and other anthropogenic impacts. Mar. Ecol. Prog. Ser. 507, 277–295. doi: 10.3354/meps10849
Calle, L., Green, L., Strong, A., and Gawlik, D. E. (2018). Time-integrated habitat availability is a resource attribute that informs patterns of use in intertidal areas. Ecol. Monogr. 88, 600–620. doi: 10.1002/ecm.1305
Camphuysen, C. J. (2004). The return of the harbour porpoise (phocoena phocoena) in Dutch coastal waters. Lutra 47, 113–122.
Camphuysen, C. J., and Peet, G. (2006). Whales and Dolphins of the North Sea. Kortenhoef: Fontaine Uitgevers.
Capuzzo, E., Lynam, C. P., Barry, J., Stephens, D., Forster, R. M., Greenwood, N., et al., (2018). A decline in primary production in the North sea over 25 years, associated with reductions in zooplankton abundance and fish stock recruitment. Global Change Biol. 24, e352–e364. doi: 10.1111/gcb.13916
Carpenter, J. R., Merckelbach, L., Callies, U., Clark, S., Gaslikova, L., and Baschek, B. (2016). Potential impacts of offshore wind farms on North sea stratification. PLoS One 11:e0160830. doi: 10.1371/journal.pone.0160830
Carroll, E. L., Hall, A., Olsen, M. T., Onoufriou, A. B., Gaggiotti, O. E., and Russell, D. J. (2020). Perturbation drives changing metapopulation dynamics in a top marine predator. Proc. R. Soc. 287:20200318. doi: 10.1098/rspb.2020.0318
Carroll, G., Cox, M., Harcourt, R., Pitcher, B. J., Slip, D., and Jonsen, I. (2017). Hierarchical influences of prey distribution on patterns of prey capture by a marine predator. Funct. Ecol. 31, 1750–1760. doi: 10.1006/anbe.2000.1427
Carroll, M. J., Butler, A., Owen, E., Ewing, S. R., Cole, T., Green, J. A., et al., (2015). Effects of sea temperature and stratification changes on seabird breeding success. Climate Res. 66, 75–89. doi: 10.3354/cr01332
Cavallo, M., Elliott, M., Touza, J., and Quintino, V. (2017). Benefits and impediments for the integrated and coordinated management of European seas. Mar. Policy 86, 206–213. doi: 10.1016/j.marpol.2017.09.035
Chassot, E., Mélin, F., Le Pape, O., and Gascuel, D. (2007). Bottom-up control regulates fisheries production at the scale of eco-regions in European seas. Mar. Ecol. Prog. Ser. 343, 45–55.
Chavez-Rosales, S., Palka, D. L., Garrison, L. P., and Josephson, E. A. (2019). Environmental predictors of habitat suitability and occurrence of cetaceans in the western North Atlantic Ocean. Sci. Rep. 9, 1–11. doi: 10.1038/s41598-019-42288-6
Chen, C., Liu, H., and Beardsley, R. C. (2003). An unstructured grid, finite-volume, three-dimensional, primitive equations ocean model: application to coastal ocean and estuaries. J. Atmospheric Oceanic Technol. 20, 159–186.
Christensen, V., and Walters, C. (2000). Ecopath with ecosim: methods, capabilities and limitations. Fish. Cent. Res. Rep. 8, 79–105.
Chudzinska, M., Nabe-Nielsen, J., Smout, S., Aarts, G., Brasseur, S., Graham, I., et al., (2021). Agent seal: agent-based model describing movement of marine central-place foragers. Ecol. Mod. 440:109397.
Coll, M., Pennino, M. G., Steenbeek, J., Solé, J., and Bellido, J. M. (2019). Predicting marine species distributions: complementarity of food-web and Bayesian hierarchical modelling approaches. Ecol. Mod. 405, 86–101. doi: 10.1016/j.ecolmodel.2019.05.005
Conti, L., and Scardi, M. (2010). Fisheries yield and primary productivity in large marine ecosystems. Mar. Ecol. Prog. Ser. 410, 233–244. doi: 10.3354/meps08630
Cook, A. S., Ross-Smith, V. H., and Robinson, R. A. (2014b). Development of MSFD Indicators, Baselines and Target for Seabird Breeding Failure Occurrence in the UK. JNCC Report No. 539.
Cook, A. S., Dadam, D., Mitchell, I., Ross-Smith, V. H., and Robinson, R. A. (2014a). Indicators of seabird reproductive performance demonstrate the impact of commercial fisheries on seabird populations in the North Sea. Ecol. Indic. 38, 1–11. doi: 10.1016/j.ecolind.2013.10.027
Cotté, C., d’Ovidio, F., Chaigneau, A., Lévy, M., Taupier-Letage, I., Mate, B., et al., (2011). Scale-dependent interactions of mediterranean whales with marine dynamics. Limnol. Oceanogr. 56, 219–232. doi: 10.4319/lo.2011.56.1.0219
Cotter, E., and Polagye, B. (2020). Automatic classification of biological targets in a tidal channel using a multibeam sonar. J. Atmospheric Oceanic Technol. 37, 1437–1455. doi: 10.1175/jtech-d-19-0222.1
Cox, S., Embling, C. B., Hosegood, P. J., Votier, S. C., and Ingram, S. N. (2018). Oceanographic drivers of marine mammal and seabird habitat-use across shelf-seas: a guide to key features and recommendations for future research and conservation management. Estuarine Coastal Shelf Sci. 212, 294–310. doi: 10.1016/j.ecss.2018.06.022
Cox, S. L., Miller, P. I., Embling, C. B., Scales, K. L., Bicknell, A. W. J., Hosegood, P. J., et al., (2016). Seabird diving behaviour reveals the functional significance of shelf-sea fronts as foraging hotspots. R. Soc. Open Sci. 3:160317. doi: 10.1098/rsos.160317
Cox, S. L., Scott, B. E., and Camphuysen, C. J. (2013). Combined spatial and tidal processes identify links between pelagic prey species and seabirds. Mar. Ecol. Prog. Ser. 479, 203–221.
Cullen, J. J. (2015). Subsurface chlorophyll maximum layers: enduring enigma or mystery solved? Ann. Rev. Mar. Sci. 7, 207–239. doi: 10.1146/annurev-marine-010213-135111
Cury, P. M., Boyd, I. L., Bonhommeau, S., Anker-Nilssen, T., Crawford, R. J., Furness, R. W., et al., (2011). Global seabird response to forage fish depletion—one-third for the birds. Science 334, 1703–1706. doi: 10.1126/science.1212928
Daunt, F., Wanless, S., Peters, G., Benvenuti, S., Sharples, J., Grémillet, D., et al., (2006). Impacts of Oceanography on the Foraging Dynamics of Seabirds in the North Sea. Top Predators in Marine Ecosystems: Their Role in Monitoring and Management. Cambridge: Cambridge University Press, 177–190.
Davies, I., Gubbins, M., and Watret, R. (2012). Scoping Study for Offshore Wave Energy Development in Scottish Waters, Vol. 3. Edinburgh: Scottish Government.
De Boer, G. J., Pietrzak, J. D., and Winterwerp, J. C. (2008). Using the potential energy anomaly equation to investigate tidal straining and advection of stratification in a region of freshwater influence. Ocean Mod. 22, 1–11. doi: 10.1016/j.ocemod.2007.12.003
De Dominicis, M., Wolf, J., and O’Hara Murray, R. (2018). Comparative effects of climate change and tidal stream energy extraction in a shelf sea. J. Geophys. Res. Oceans 123, 5041–5067.
Diaz-López, B. D., and Methion, S. (2018). Does interspecific competition drive patterns of habitat use and relative density in harbour porpoises? Mar. Biol. 165, 1–11.
Dulvy, N. K., Rogers, S. I., Jennings, S., Stelzenmüller, V., Dye, S. R., and Skjoldal, H. R. (2008). Climate change and deepening of the North sea fish assemblage: a biotic indicator of warming seas. J. Appl. Ecol. 45, 1029–1039.
Durant, J. M., Anker-Nilssen, T., and Stenseth, N. C. (2006). Ocean climate prior to breeding affects the duration of the nestling period in the Atlantic puffin. Biol. Lett. 2, 628–631. doi: 10.1098/rsbl.2006.0520
Edwards, M., Atkinson, A., Bresnan, E., Helaouet, P., McQuattersGollup, A., Ostle, C., et al., (2020). Plankton, jellyfish and climate in the North-East Atlantic. MCCIP Sci. Rev. 2020, 322–353.
Eerkes-Medrano, D., Fryer, R. J., Cook, K. B., and Wright, P. J. (2017). Are simple environmental indicators of food web dynamics reliable: exploring the kittiwake–temperature relationship. Ecol. Indic. 75, 36–47. doi: 10.1016/j.ecolind.2016.12.019
Embling, C. B., Gillibrand, P. A., Gordon, J., Shrimpton, J., Stevick, P. T., and Hammond, P. S. (2010). Using habitat models to identify suitable sites for marine protected areas for harbour porpoises (phocoena phocoena). Biol. Conserv. 143, 267–279. doi: 10.1016/j.biocon.2009.09.005
Embling, C. B., Illian, J., Armstrong, E., van der Kooij, J., Sharples, J., Camphuysen, K. C., et al., (2012). Investigating fine-scale spatio-temporal predator–prey patterns in dynamic marine ecosystems: a functional data analysis approach. J. Appl. Ecol. 49, 481–492. doi: 10.1111/j.1365-2664.2012.02114.x
Estes, J. A., Terborgh, J., Brashares, J. S., Power, M. E., Berger, J., Bond, W. J., et al., (2011). Trophic downgrading of planet earth. Science 333, 301–306. doi: 10.1126/science.1205106
Offshore Renewable Energy Strategy European Commission (2020). Offshore Renewable Energy Strategy. Available online at: https://ec.europa.eu/energy/sites/ener/files/offshore_renewable_energy_strategy.pdf (accessed November 19, 2020).
Evans, P. G., and Bjørge, A. (2013). Impacts of climate change on marine mammals. Mar. Clim. Change Impacts Partnership Sci. Rev. 2013, 134–148.
Evans, P. G. H., and Waggitt, J. J. (2020). Impacts of climate change on marine mammals, relevant to the coastal and marine environment around the UK. MCCIP Sci. Rev. 2020, 421–455. doi: 10.1007/s00248-013-0204-5
Falkowski, P. G., and Raven, J. A. (2013). Aquatic Photosynthesis. Princeton: Princeton University Press.
Fauchald, P. (2010). Predator–prey reversal: a possible mechanism for ecosystem hysteresis in the North Sea? Ecology 91, 2191–2197. doi: 10.1890/09-1500.1
Fauchald, P., Skov, H., Skern-Mauritzen, M., Johns, D., and Tveraa, T. (2011). Wasp-waist interactions in the North sea ecosystem. PLoS One 6:e22729. doi: 10.1371/journal.pone.0022729
Frank, K. T., Petrie, B., Choi, J. S., and Leggett, W. C. (2005). Trophic cascades in a formerly cod-dominated ecosystem. Science 308, 1621–1623. doi: 10.1126/science.1113075
Frank, K. T., Petrie, B., Shackell, N. L., and Choi, J. S. (2006). Reconciling differences in trophic control in mid-latitude marine ecosystems. Ecol. Lett. 9, 1096–1105. doi: 10.1111/j.1461-0248.2006.00961.x
Fraser, S., Williamson, B. J., Nikora, V., and Scott, B. E. (2018). Fish distributions in a tidal channel indicate the behavioural impact of a marine renewable energy installation. Energy Rep. 4, 65–69.
Frederiksen, M., Edwards, M., Mavor, R. A., and Wanless, S. (2007). Regional and annual variation in black-legged kittiwake breeding productivity is related to sea surface temperature. Mar. Ecol. Prog. Ser. 350, 137–143.
Frederiksen, M., Wright, P. J., Harris, M. P., Mavor, R. A., Heubeck, M., and Wanless, S. (2005). Regional patterns of kittiwake rissa tridactyla breeding success are related to variability in sandeel recruitment. Mar. Ecol. Progr. Ser. 300, 201–211. doi: 10.3354/meps300201
Friedland, K. D., Mouw, C. B., Asch, R. G., Ferreira, A. S. A., Henson, S., Hyde, K. J., et al., (2018). Phenology and time series trends of the dominant seasonal phytoplankton bloom across global scales. Global Ecol. Biogeogr. 27, 551–569. doi: 10.1111/geb.12717
Friedland, K. D., Stock, C., Drinkwater, K. F., Link, J. S., Leaf, R. T., Shank, B. V., et al., (2012). Pathways between primary production and fisheries yields of large marine ecosystems. PLoS One 7:e28945. doi: 10.1371/journal.pone.0028945
Gårdmark, A., Lindegren, M., Neuenfeldt, S., Blenckner, T., Heikinheimo, O., Müller-Karulis, B., et al., (2013). Biological ensemble modelling to evaluate potential futures of living marine resources. Ecol. Appl. 23, 742–754. doi: 10.1890/12-0267.1
Gilles, A., Adler, S., Kaschner, K., Scheidat, M., and Siebert, U. (2011). Modelling harbour porpoise seasonal density as a function of the german bight environment: implications for management. Endangered Species Res. 14, 157–169. doi: 10.3354/esr00344
Grecian, W. J., Witt, M. J., Attrill, M. J., Bearhop, S., Becker, P. H., Egevang, C., et al., (2016). Seabird diversity hotspot linked to ocean productivity in the canary current large marine ecosystem. Bio. Lett. 12:20160024. doi: 10.1098/rsbl.2016.0024
Grémillet, D., Lewis, S., Drapeau, L., van Der Lingen, C. D., Huggett, J. A., Coetzee, J. C., et al., (2008). Spatial match–mismatch in the benguela upwelling zone: should we expect chlorophyll and sea-surface temperature to predict marine predator distributions? J. Appl. Ecol. 45, 610–621. doi: 10.1111/j.1365-2664.2007.01447.x
Guerry, A. D., Polasky, S., Lubchenco, J., Chaplin-Kramer, R., Daily, G. C., Griffin, R., et al., (2015). Natural capital and ecosystem services informing decisions: from promise to practice. Proc. Natl. Acad. Sci. U.S.A. 112, 7348–7355. doi: 10.1073/pnas.1503751112
Hammond, P. S., Macleod, K., Berggren, P., Borchers, D. L., Burt, L., Cañadas, A., et al., (2013). Cetacean abundance and distribution in European Atlantic shelf waters to inform conservation and management. Biol. Conserv. 164, 107–122. doi: 10.1016/j.biocon.2013.04.010
Hastie, G. D., Russell, D. J., Benjamins, S., Moss, S., Wilson, B., and Thompson, D. (2016). Dynamic habitat corridors for marine predators; intensive use of a coastal channel by harbour seals is modulated by tidal currents. Behav. Ecol. Sociobiol. 70, 2161–2174. doi: 10.1007/s00265-016-2219-7
Hastie, G. D., Russell, D. J., Lepper, P., Elliott, J., Wilson, B., Benjamins, S., et al., (2018). Harbour seals avoid tidal turbine noise: implications for collision risk. J. Appl. Ecol. 55, 684–693. doi: 10.1111/1365-2664.12981
Heim, K. C., McMahon, T. E., Calle, L., Wipfli, M. S., and Falke, J. A. (2019). A general model of temporary aquatic habitat use: water phenology as a life history filter. Fish Fisher. 20, 802–816.
Heim, K. C., Thorne, L. H., Warren, J. D., Link, J. S., and Nye, J. A. (2021). Marine ecosystem indicators are sensitive to ecosystem boundaries and spatial scale. Ecol. Indic. 125:107522. doi: 10.1016/j.ecolind.2021.107522
Heithaus, M. R., Frid, A., Wirsing, A. J., and Worm, B. (2008). Predicting ecological consequences of marine top predator declines. Trends Ecol. Evolu. 23, 202–210. doi: 10.1016/j.tree.2008.01.003
Hernvann, P. Y., Gascuel, D., Grüss, A., Druon, J. N., Kopp, D., Perez, I., et al., (2020). The celtic sea through time and space: ecosystem modeling to unravel fishing and climate change impacts on food-web structure and dynamics. Front. Mar. Sci. 7:1018.
Hickman, A. E., Moore, C. M., Sharples, J., Lucas, M. I., Tilstone, G. H., Krivtsov, V., et al., (2012). Primary production and nitrate uptake within the seasonal thermocline of a stratified shelf sea. Mar. Ecol. Prog. Ser. 463, 39–57. doi: 10.3354/meps09836
Holt, J., Butenschön, M., Wakelin, S. L., Artioli, Y., and Allen, J. I. (2012). Oceanic controls on the primary production of the northwest European continental shelf: model experiments under recent past conditions and a potential future scenario. Biogeosciences 9, 97–117. doi: 10.5194/bg-9-97-2012
Holt, J., Wakelin, S., Lowe, J., and Tinker, J. (2010). The potential impacts of climate change on the hydrography of the northwest European continental shelf. Prog. Oceanogr. 86, 361–379. doi: 10.1016/j.pocean.2010.05.003
Hooper, T., Beaumont, N., and Hattam, C. (2017). The implications of energy systems for ecosystem services: a detailed case study of offshore wind. Renewable Sust. Energy Rev. 70, 230–241.
Horsburgh, K., Rennie, A., and Palmer, M. (2020). Impacts of climate change on sea-level rise relevant to the coastal and marine environment around the UK. MCCIP Sci. Rev. 2020, 116–131.
Howells, R. J., Burthe, S. J., Green, J. A., Harris, M. P., Newell, M. A., Butler, A., et al., (2017). From days to decades: short-and long-term variation in environmental conditions affect offspring diet composition of a marine top predator. Mar. Ecology Prog. Ser.583, 227–242.
Humphreys, M. P., Artioli, Y., Bakker, D. C. E., Hartman, S. E., León, P., Wakelin, S., et al., (2020). Air-sea CO2 exchange and ocean acidification in UK seas and adjacent waters. MCCIP Sci. Rev. 2020, 54–75.
Hunt, G. L. Jr., Stabeno, P. J., Strom, S., and Napp, J. M. (2008). Patterns of spatial and temporal variation in the marine ecosystem of the southeastern bering sea, with special reference to the pribilof domain. Deep Sea Res. Part II Top. Stud. Oceanogr. 55, 1919–1944.
Hydes, D. J., Le Gall, A. C., Miller, A. E. J., Brockmann, U., Raabe, T., Holley, S., et al., (2001). Supply and demand of nutrients and dissolved organic matter at and across the NW European shelf break in relation to hydrography and biogeochemical activity. Deep Sea Res. Part II Top. Stud. Oceanogr. 48, 3023–3047.
IJsseldijk, L. L., Camphuysen, K. C., Nauw, J. J., and Aarts, G. (2015). Going with the flow: tidal influence on the occurrence of the harbour porpoise (phocoena phocoena) in the marsdiep area, the Netherlands. J. Sea Res. 103, 129–137.
IRENA (2019). Global Energy Transformation: A Roadmap to 2050. Abu Dhabi: International Renewable Energy Agency.
Johnston, D. W., and Read, A. J. (2007). Flow-field observations of a tidally driven island wake used by marine mammals in the bay of fundy, Canada. Fisher. Oceanogr. 16, 422–435.
Johnston, D. W., Westgate, A. J., and Read, A. J. (2005). Effects of fine-scale oceanographic features on the distribution and movements of harbour porpoises phocoena phocoena in the bay of fundy. Mar. Ecol. Progr. Ser. 295, 279–293. doi: 10.3354/meps295279
Joint, I., Wollast, R., Chou, L., Batten, S., Elskens, M., Edwards, E., et al., (2001). Pelagic production at the celtic sea shelf break. Deep Sea Res. Part II Top. Stud. Oceanogr. 48, 3049–3081.
Jones, A. R., Hosegood, P., Wynn, R. B., De Boer, M. N., Butler-Cowdry, S., and Embling, C. B. (2014). Fine-scale hydrodynamics influence the spatio-temporal distribution of harbour porpoises at a coastal hotspot. Prog. Oceanogr. 128, 30–48. doi: 10.1016/j.pocean.2014.08.002
Kafas, A., Davies, I., McLay, A., Gubbins, M., and Scott, B. (2014). “New perspectives on fisheries: combining the distribution of inshore and offshore commercial fisheries in scotland,” in Proceeding of the 2nd International Conference on Environmental Interactions of Marine Renewables Energy Technologies, Stornoway, Scotland.
Kafas, A., McLay, A., Chimienti, M., Scott, B. E., Davies, I., and Gubbins, M. (2017). ScotMap: Participatory mapping of inshore fishing activity to inform marine spatial planning in Scotland. Mar. Policy 79, 8–18. doi: 10.1016/j.marpol.2017.01.009
Kaltenberg, A. M., and Benoit-Bird, K. J. (2013). Intra-patch clustering in mysid swarms revealed through multifrequency acoustics. ICES J. Mar. Sci. 70, 883–891.
Kane, A., Pirotta, E., Wischnewski, S., Critchley, E. J., Bennison, A., Jessopp, M., et al., (2020). Spatio-temporal patterns of foraging behaviour in a wide-ranging seabird reveal the role of primary productivity in locating prey. Mar. Ecol. Progr. Ser. 646, 175–188.
Kempf, A., Dingsør, G. E., Huse, G., Vinther, M., Floeter, J., and Temming, A. (2010). The importance of predator–prey overlap: predicting North sea cod recovery with a multispecies assessment model. ICES J. Mar. Sci. 67, 1989–1997. doi: 10.1093/icesjms/fsq114
Kempf, A., Stelzenmüller, V., Akimova, A., and Floeter, J. (2013). Spatial assessment of predator–prey relationships in the North sea: the influence of abiotic habitat properties on the spatial overlap between 0-group cod and grey gurnard. Fish. Oceanogr. 22, 174–192. doi: 10.1111/fog.12013
Kidé, S. O., Manté, C., Dubroca, L., Demarcq, H., and Mérigot, B. (2015). Spatio-temporal dynamics of exploited groundfish species assemblages faced to environmental and fishing forcings: insights from the mauritanian exclusive economic zone. PLoS One 10:e0141566. doi: 10.1371/journal.pone.0141566
Lambert, C., Pettex, E., Dorémus, G., Laran, S., Stéphan, E., Van Canneyt, O., et al., (2017). How does ocean seasonality drive habitat preferences of highly mobile top predators? Part II Eastern North Atlantic Deep Sea Res. Part II Top. Stud. Oceanogr. 141, 133–154. doi: 10.1016/j.dsr2.2016.06.011
Levin, S. A. (1992). The problem of pattern and scale in ecology: the robert H. MacArthur award lecture. Ecology 73, 1943–1967. doi: 10.2307/1941447
Lieber, L., Nimmo-Smith, W. A. M., Waggitt, J. J., and Kregting, L. (2019). Localised anthropogenic wake generates a predictable foraging hotspot for top predators. Commun. Biol. 2, 1–8. doi: 10.1038/s42003-019-0364-z
Ludewig, E. (2015). On the effect of offshore wind farms on the atmosphere and ocean dynamics. Switzerland: Springer.
Lynam, C. P., Llope, M., Möllmann, C., Helaouët, P., Bayliss-Brown, G. A., and Stenseth, N. C. (2017). Interaction between top-down and bottom-up control in marine food webs. Proc. Natl. Acad. Sci. U.S.A. 114, 1952–1957. doi: 10.1073/pnas.1621037114
Lynam, C. P., and Mackinson, S. (2015). How will fisheries management measures contribute towards the attainment of good environmental status for the North sea ecosystem? Global Ecol. Conserv. 4, 160–175.
MacIsaac, J. J., Dugdale, R. C., Barber, R. T., Blasco, D., and Packard, T. T. (1985). Primary production cycle in an upwelling center. Deep Sea Res. Part A Oceanogr. Res. Papers 32, 503–529. doi: 10.1016/0198-0149(85)90042-1
Mahaffey, C., Palmer, M., Greenwood, N., and Sharples, J. (2020). Impacts of climate change on dissolved oxygen concentration relevant to the coastal and marine environment around the UK. MCCIP Sci. Rev. 2020, 31–53.
Mannocci, L., Boustany, A. M., Roberts, J. J., Palacios, D. M., Dunn, D. C., Halpin, P. N., et al., (2017). Temporal resolutions in species distribution models of highly mobile marine animals: recommendations for ecologists and managers. Div. Distribut. 23, 1098–1109. doi: 10.1111/ddi.12609
Marini, L., Bartomeus, I., Rader, R., and Lami, F. (2019). Species–habitat networks: a tool to improve landscape management for conservation. J. Appl. Ecol. 56, 923–928.
Markones, N. (2007). Habitat Selection of Seabirds in a Highly Dynamic Coastal Sea: Temporal Variation and Influence of Hydrographic Features Ph. D, Thesis. Christian-Albrechts Universität Kiel.
Mattei, F., Franceschini, S., and Scardi, M. (2018). A depth-resolved artificial neural network model of marine phytoplankton primary production. Ecol. Mod. 382, 51–62.
Matthiopoulos, J., Fieberg, J., Aarts, G., Beyer, H. L., Morales, J. M., and Haydon, D. T. (2015). Establishing the link between habitat selection and animal population dynamics. Ecol. Monogr. 85, 413–436. doi: 10.1890/14-2244.1
McClintock, B. T., Russell, D. J., Matthiopoulos, J., and King, R. (2013). Combining individual animal movement and ancillary biotelemetry data to investigate population-level activity budgets. Ecology 94, 838–849. doi: 10.1890/12-0954.1
McInnes, A. M., Ryan, P. G., Lacerda, M., Deshayes, J., Goschen, W. S., and Pichegru, L. (2017). Small pelagic fish responses to fine-scale oceanographic conditions: implications for the endangered African penguin. Mar. Ecol. Prog. Ser. 569, 187–203.
Medina-Lopez, E., McMillan, D., Lazic, J., Hart, E., Zen, S., Angeloudis, A., et al., (2021). Satellite data for the offshore renewable energy sector: synergies and innovation opportunities. Remote Sensing Environ. 264:112588. doi: 10.1016/j.rse.2021.112588
Mérillet, L., Kopp, D., Robert, M., Mouchet, M., and Pavoine, S. (2020). Environment outweighs the effects of fishing in regulating demersal community structure in an exploited marine ecosystem. Global Change Biol. 26, 2106–2119. doi: 10.1111/gcb.14969
Miles, T., Murphy, S., Kohut, J., Borsetti, S., and Munroe, D. (2020). Could Federal Wind Farms Influence Continental Shelf Oceanography and Alter Associated Ecological Processes? A Literature Review, 1–22. Available online at: https://scemfis.org/wp-content/uploads/2021/01/ColdPoolReview.pdf
Mitchell, I., Daunt, F., Frederiksen, M., and Wade, K. (2020). Impacts of climate change on seabirds, relevant to the coastal and marine environment around the UK. MCCIP Sci. Rev. 2020, 382–399.
Molinero, J. C., Reygondeau, G., and Bonnet, D. (2013). Climate variance influence on the non-stationary plankton dynamics. Mar. Environ. Res. 89, 91–96. doi: 10.1016/j.marenvres.2013.04.006
Moufaddal, W. M. (2005). Use of satellite imagery as environmental impact assessment tool: a case study from the NW egyptian red sea coastal zone. Environ. Monit. Assess. 107, 427–452. doi: 10.1007/s10661-005-3576-2
Nabe-Nielsen, J., Tougaard, J., Teilmann, J., Lucke, K., and Forchhammer, M. C. (2013). How a simple adaptive foraging strategy can lead to emergent home ranges and increased food intake. Oikos 122, 1307–1316.
Nabe-Nielsen, J., van Beest, F. M., Grimm, V., Sibly, R. M., Teilmann, J., and Thompson, P. M. (2018). Predicting the impacts of anthropogenic disturbances on marine populations. Conserv. Lett.11:e12563.
Nowak, B. V. R., Bowen, W. D., Whoriskey, K., Lidgard, D. C., Flemming, J. M., and Iverson, S. J. (2020). Foraging behaviour of a continental shelf marine predator, the grey seal (Halichoerus grypus), is associated with in situ, subsurface oceanographic conditions. Movement Ecol. 8, 1–14. doi: 10.1186/s40462-020-00225-7
Ohman, M. D., Rudnick, D. L., Chekalyuk, A., Davis, R. E., Feely, R. A., Kahru, M., et al., (2013). Autonomous ocean measurements in the california current ecosystem. Oceanography 26, 18–25. doi: 10.5670/oceanog.2013.41
Patil, A. A., Annachhatre, A. P., and Tripathi, N. K. (2002). Comparison of conventional and geo-spatial EIA: a shrimp farming case study. Environ. Impact Assess. Rev. 22, 361–375. doi: 10.1016/s0195-9255(02)00013-6
Peck, M. A., Arvanitidis, C., Butenschön, M., Canu, D. M., Chatzinikolaou, E., Cucco, A., et al., (2018). Projecting changes in the distribution and productivity of living marine resources: a critical review of the suite of modelling approaches used in the large European project VECTORS. Estuarine Coastal Shelf Sci. 201, 40–55. doi: 10.1016/j.ecss.2016.05.019
Pierpoint, C. (2008). Harbour porpoise (phocoena phocoena) foraging strategy at a high energy, near-shore site in south-west Wales, UK. J. Mar. Biol. Assoc. U.K. 88, 1167–1173.
Piroddi, C., Teixeira, H., Lynam, C. P., Smith, C., Alvarez, M. C., Mazik, K., et al., (2015). Using ecological models to assess ecosystem status in support of the european marine strategy framework directive. Ecol. Indic. 58, 175–191. doi: 10.1016/j.ecolind.2015.05.037
Pirotta, E., Edwards, E. W., New, L., and Thompson, P. M. (2018). Central place foragers and moving stimuli: a hidden-state model to discriminate the processes affecting movement. J. Animal Ecol. 87, 1116–1125. doi: 10.1111/1365-2656.12830
Pitois, S. G., Lynam, C. P., Jansen, T., Halliday, N., and Edwards, M. (2012). Bottom-up effects of climate on fish populations: data from the continuous plankton recorder. Mar. Ecol. Prog. Ser. 456, 169–186.
Planque, B., Loots, C., Petitgas, P., Lindstrøm, U. L. F., and Vaz, S. (2011). Understanding what controls the spatial distribution of fish populations using a multi-model approach. Fish. Oceanogr. 20, 1–17. doi: 10.1111/j.1365-2419.2010.00546.x
Polagye, B., Joslin, J., Murphy, P., Cotter, E., Scott, M., Gibbs, P., et al., (2020). Adaptable monitoring package development and deployment: lessons learned for integrated instrumentation at marine energy sites. J. Mar. Sci. Eng. 8:553.
Polovina, J. J. (1984). The ECOPATH model and its application to french frigate shoals. Coral Reefs 3, 1–11. doi: 10.1007/bf00306135
Preciado, I., Arroyo, N. L., González-Irusta, J. M., López-López, L., Punzón, A., Muñoz, I., et al., (2019). Small-scale spatial variations of trawling impact on food web structure. Ecol. Indic. 98, 442–452. doi: 10.1016/j.ecolind.2018.11.024
Raoux, A., Lassalle, G., Pezy, J. P., Tecchio, S., Safi, G., Ernande, B., et al., (2019). Measuring sensitivity of two OSPAR indicators for a coastal food web model under offshore wind farm construction. Ecol. Indic. 96, 728–738. doi: 10.1016/j.ecolind.2018.07.014
Raoux, A., Tecchio, S., Pezy, J. P., Lassalle, G., Degraer, S., Wilhelmsson, D., et al., (2017). Benthic and fish aggregation inside an offshore wind farm: which effects on the trophic web functioning? Ecol. Indic. 72, 33–46. doi: 10.1016/j.ecolind.2016.07.037
Régnier, T., Gibb, F. M., and Wright, P. J. (2019). Understanding temperature effects on recruitment in the context of trophic mismatch. Sci. Rep. 9, 1–13. doi: 10.1038/s41598-019-51296-5
Regular, P. M., Hedd, A., and Montevecchi, W. A. (2013). Must marine predators always follow scaling laws? Memory guides the foraging decisions of a pursuit-diving seabird. Animal Behav. 86, 545–552. doi: 10.1016/j.anbehav.2013.06.008
Richardson, K., Visser, A. W., and Pedersen, F. B. (2000). Subsurface phytoplankton blooms fuel pelagic production in the North sea. J. Plankton Res. 22, 1663–1671. doi: 10.1093/plankt/22.9.1663
Robinson, L. M., Elith, J., Hobday, A. J., Pearson, R. G., Kendall, B. E., Possingham, H. P., et al., (2011). Pushing the limits in marine species distribution modelling: lessons from the land present challenges and opportunities. Global Ecol. Biogeogr. 20, 789–802. doi: 10.1111/j.1466-8238.2010.00636.x
Ropert-Coudert, Y., Kato, A., and Chiaradia, A. (2009). Impact of small-scale environmental perturbations on local marine food resources: a case study of a predator, the little penguin. Proc. R. Soc. B Biol. Sci. 276, 4105–4109. doi: 10.1098/rspb.2009.1399
Ross, O. N., and Sharples, J. (2007). Phytoplankton motility and the competition for nutrients in the thermocline. Mar. Ecol. Prog. Ser. 347, 21–38.
Russell, D. J., Brasseur, S. M., Thompson, D., Hastie, G. D., Janik, V. M., Aarts, G., et al., (2014). Marine mammals trace anthropogenic structures at sea. Curr. Biol. 24, R638–R639. doi: 10.1016/j.cub.2014.06.033
Rutterford, L. A., Simpson, S. D., Jennings, S., Johnson, M. P., Blanchard, J. L., Schön, P. J., et al., (2015). Future fish distributions constrained by depth in warming seas. Nat. Climate Change 5, 569–573.
Sabal, M. C., Hazen, E. L., Bograd, S. J., MacFarlane, R. B., Schroeder, I. D., Hayes, S. A., et al., (2020). California current seascape influences juvenile salmon foraging ecology at multiple scales. Mar. Ecol. Prog. Ser. 634, 159–173. doi: 10.3354/meps13185
Sabarros, P. S., Grémillet, D., Demarcq, H., Moseley, C., Pichegru, L., Mullers, R. H., et al., (2014). Fine-scale recognition and use of mesoscale fronts by foraging cape gannets in the benguela upwelling region. Deep Sea Res. Part II Top. Stud. Oceanogr. 107, 77–84.
Sadykova, D., Scott, B. E., De Dominicis, M., Wakelin, S. L., Sadykov, A., and Wolf, J. (2017). Bayesian joint models with INLA exploring marine mobile predator-prey and competitor species habitat overlap. Ecol. Evol. 7, 5212–5226. doi: 10.1002/ece3.3081
Sadykova, D., Scott, B. E., De Dominicis, M., Wakelin, S. L., Wolf, J., and Sadykov, A. (2020). Ecological costs of climate change on marine predator–prey population distributions by 2050. Ecol. Evol. 7, 5212–5226. doi: 10.1002/ece3.5973
Sagarese, S. R., Frisk, M. G., Cerrato, R. M., Sosebee, K. A., Musick, J. A., and Rago, P. J. (2014). Application of generalized additive models to examine ontogenetic and seasonal distributions of spiny dogfish (squalus acanthias) in the northeast (US) shelf large marine ecosystem. Can. J. Fish. Aquatic Sci. 71, 847–877.
Samarra, F. I. P., and Foote, A. D. (2015). Seasonal movements of killer whales between iceland and scotland. Aquatic Biol. 24, 75–79. doi: 10.3354/ab00637
Scales, K. L., Hazen, E. L., Jacox, M. G., Edwards, C. A., Boustany, A. M., Oliver, M. J., et al., (2017). Scale of inference: on the sensitivity of habitat models for wide-ranging marine predators to the resolution of environmental data. Ecography 40, 210–220. doi: 10.1111/ecog.02272
Scales, K. L., Miller, P. I., Embling, C. B., Ingram, S. N., Pirotta, E., and Votier, S. C. (2014). Mesoscale fronts as foraging habitats: composite front mapping reveals oceanographic drivers of habitat use for a pelagic seabird. J. R. Soc. Interface 11:20140679. doi: 10.1098/rsif.2014.0679
Scherelis, C., Penesis, I., Hemer, M. A., Cossu, R., Wright, J. T., and Guihen, D. (2020). Investigating biophysical linkages at tidal energy candidate sites: a case study for combining environmental assessment and resource characterisation. Renewable Energy 159, 399–413. doi: 10.1016/j.dib.2020.105873
Schuchert, P., Kregting, L., Pritchard, D., Savidge, G., and Elsäßer, B. (2018). Using coupled hydrodynamic biogeochemical models to predict the effects of tidal turbine arrays on phytoplankton dynamics. J. Mar. Sci. Eng. 6:58. doi: 10.3390/jmse6020058
Schultze, L. K. P., Merckelbach, L. M., Horstmann, J., Raasch, S., and Carpenter, J. R. (2020). Increased mixing and turbulence in the wake of offshore wind farm foundations. J. Geophys. Res. Oceans 125:e2019JC015858.
Scott, B. E., Langton, R., Philpott, E., and Waggitt, J. J. (2014). Seabirds and Marine Renewables: Are We Asking The Right Questions? In Marine Renewable Energy Technology and Environmental Interactions. Dordrecht: Springer, 81–92.
Scott, B. E., Sharples, J., Ross, O. N., Wang, J., Pierce, G. J., and Camphuysen, C. J. (2010). Sub-surface hotspots in shallow seas: fine-scale limited locations of top predator foraging habitat indicated by tidal mixing and sub-surface chlorophyll. Mar. Ecol. Prog. Ser. 408, 207–226. doi: 10.3354/meps08552
Scott, B. E., Sharples, J., Wanless, S., Ross, O. N., Frederiksen, M., and Daunt, F. (2006). The use of biologically meaningful oceanographic indices to separate the effects of climate and fisheries on seabird breeding success. Conserv. Biol. Ser. Cambridge 12:46.
Searle, K., Mobbs, D., Butler, A., Bogdanova, M., Freeman, S., Wanless, S., et al., (2014). Population Consequences of Displacement from Proposed Offshore Wind Energy Developments for Seabirds Breeding at Scottish SPAs: Final Report to Marine Scotland Science CR/2012/03. Marine Scotland Science, 1–145. Available online at: https://tethys.pnnl.gov/sites/default/files/publications/searleetal.pdf
Sectoral Marine Plan for Offshore Wind Energy (2020). Available online at: https://www.gov.scot/publications/sectoral-marine-plan-offshore-wind-energy/ (accessed November 19, 2020).
Serpetti, N., Baudron, A. R., Burrows, M. T., Payne, B. L., Helaouet, P., Fernandes, P. G., et al., (2017). Impact of ocean warming on sustainable fisheries management informs the ecosystem approach to fisheries. Sci. Rep. 7, 1–15. doi: 10.1038/s41598-017-13220-7
Sharples, J., Holet, J., and Wakelin, S. (2020). Impacts of climate change on shelf sea stratification, relevant to the coastal and marine environment around the UK. MCCIP Sci. Rev. 2020, 103–115.
Sharples, J., Ross, O. N., Scott, B. E., Greenstreet, S. P., and Fraser, H. (2006). Inter-annual variability in the timing of stratification and the spring bloom in the North-western North sea. Continental Shelf Res. 26, 733–751. doi: 10.1016/j.csr.2006.01.011
Sharples, J., Scott, B. E., and Inall, M. E. (2013). From physics to fishing over a shelf sea bank: preface. Prog. Oceanogr. 117, 1–8.
Shealer, D. A. (2002). “Foraging behavior and food of seabirds,” in Biology of Marine Birds, eds A. E. Schreiber and J. Burger (Boca Raton, FL: CRC Press), 137–177. doi: 10.1201/9781420036305.ch6
Sherman, K., Belkin, I. M., Friedland, K. D., O’Reilly, J., and Hyde, K. (2009). Accelerated warming and emergent trends in fisheries biomass yields of the world’s large marine ecosystems. Ambio 38, 215–224. doi: 10.1579/0044-7447-38.4.215
Simpson, J. H., and Bowers, D. (1981). Models of stratification and frontal movement in shelf seas. Deep Sea Res. Part A Oceanogr. Res. Papers 28, 727–738. doi: 10.1016/0198-0149(81)90132-1
Simpson, J. H., and Sharples, J. (2012). Introduction to the Physical and Biological Oceanography of Shelf Seas. Cambridge: Cambridge University Press.
Simpson, S. D., Jennings, S., Johnson, M. P., Blanchard, J. L., Schön, P. J., Sims, D. W., et al., (2011). Continental shelf-wide response of a fish assemblage to rapid warming of the sea. Curr. Biol. 21, 1565–1570. doi: 10.1016/j.cub.2011.08.016
Skov, H., and Prins, E. (2001). Impact of estuarine fronts on the dispersal of piscivorous birds in the German Bight. Mar. Ecol. Prog. Ser. 214, 279–287. doi: 10.3354/meps214279
Skov, H., and Thomsen, F. (2008). Resolving fine-scale spatio-temporal dynamics in the harbour porpoise phocoena phocoena. Mar. Ecol. Prog. Ser. 373, 173–186. doi: 10.3354/meps07666
Spalding, M. D., Fox, H. E., Allen, G. R., Davidson, N., Ferdaña, Z. A., Finlayson, M. A. X., et al., (2007). Marine ecoregions of the world: a bioregionalization of coastal and shelf areas. BioScience 57, 573–583. doi: 10.1111/cla.12453
Spence, M. A., Blanchard, J. L., Rossberg, A. G., Heath, M. R., Heymans, J. J., Mackinson, S., et al., (2018). A general framework for combining ecosystem models. Fish Fisher. 19, 1031–1042.
Stevick, P. T., Incze, L. S., Kraus, S. D., Rosen, S., Wolff, N., and Baukus, A. (2008). Trophic relationships and oceanography on and around a small offshore bank. Mar. Ecol. Prog. Ser. 363, 15–28. doi: 10.3354/meps07475
Suberg, L., Wynn, R. B., Van Der Kooij, J., Fernand, L., Fielding, S., Guihen, D., et al., (2014). Assessing the potential of autonomous submarine gliders for ecosystem monitoring across multiple trophic levels (plankton to cetaceans) and pollutants in shallow shelf seas. Methods Oceanogr. 10, 70–89. doi: 10.1016/j.mio.2014.06.002
Suryan, R. M., Santora, J. A., and Sydeman, W. J. (2012). New approach for using remotely sensed chlorophyll a to identify seabird hotspots. Mar. Ecol. Prog. Ser. 451, 213–225.
Ter Hofstede, R., Hiddink, J. G., and Rijnsdorp, A. D. (2010). Regional warming changes fish species richness in the eastern North Atlantic Ocean. Mar. Ecol. Prog. Ser. 414, 1–9. doi: 10.3354/meps08753
Thompson, D. (2013). Studies of Harbour Seal Behaviour in Areas of High Tidal Energy: part 1. Movements and Diving Behaviour of Harbour Seals in Kyle Rhea. Report to Scottish Natural Heritage and Marine Scotland. St. Andrews: Sea Mammal Research Unit.
Tinker, J. P., Howes, E. L., Wakelin, S., Menary, M., Kent, E., and Berry, D. (2020). The impacts of climate change on temperature (air and sea), relevant to the coastal and marine environment around the UK. MCCIP Sci. Rev. 2020, 1–3.
Torres, L. G., Read, A. J., and Halpin, P. (2008). Fine-scale habitat modelling of a top marine predator: do prey data improve predictive capacity. Ecol. Appl. 18, 1702–1717. doi: 10.1890/07-1455.1
Trifonova, N., Karnauskas, M., and Kelble, C. (2019). Predicting ecosystem components in the gulf of mexico and their responses to climate variability with a dynamic bayesian network model. PLoS One 14:e0209257. doi: 10.1371/journal.pone.0209257
Trifonova, N., Kenny, A., Maxwell, D., Duplisea, D., Fernandes, J., and Tucker, A. (2015). Spatio-temporal Bayesian network models with latent variables for revealing trophic dynamics and functional networks in fisheries ecology. Ecol. Inform. 30, 142–158. doi: 10.1016/j.ecoinf.2015.10.003
Trifonova, N., Maxwell, D., Pinnegar, J., Kenny, A., and Tucker, A. (2017). Predicting ecosystem responses to changes in fisheries catch, temperature, and primary productivity with a dynamic Bayesian network model. ICES J. Mar. Sci. 74, 1334–1343.
Trifonova, N., Scott, B. E., De Dominicis, M., Waggitt, J., and Wolf, J. (2021). Bayesian network modelling provides Spatial and temporal understanding of ecosystem dynamics within coastal and shallow shelf seas. Ecol. Indic. 129:107997.
Tucker, A., and Duplisea, D. (2012). Bioinformatics tools in predictive ecology: applications to fisheries. Philos. Trans. R. Soc. B Biol. Sci. 367, 279–290. doi: 10.1098/rstb.2011.0184
Tweddle, J. F., Gubbins, M., and Scott, B. E. (2018). Should phytoplankton be a key consideration for marine management? Mar. Policy 97, 1–9.
Tweddle, J. F., Sharples, J., Palmer, M. R., Davidson, K., and McNeill, S. (2013). Enhanced nutrient fluxes at the shelf sea seasonal thermocline caused by stratified flow over a bank. Prog. Oceanogr. 117, 37–47.
Uusitalo, L., Tomczak, M. T., Müller-Karulis, B., Putnis, I., Trifonova, N., and Tucker, A. (2018). Hidden variables in a dynamic bayesian network identify ecosystem level change. Ecol. Inform. 45, 9–15.
van der Molen, J., Ruardij, P., and Greenwood, N. (2016). Potential environmental impact of tidal energy extraction in the pentland firth at large spatial scales: results of a biogeochemical model. Biogeosciences 13, 2593–2609.
van der Molen, J., Smith, H. C., Lepper, P., Limpenny, S., and Rees, J. (2014). Predicting the large-scale consequences of offshore wind turbine array development on a North sea ecosystem. Continental Shelf Res. 85, 60–72. doi: 10.1016/j.csr.2014.05.018
van Leeuwen, S. M., Le Quesne, W. F., and Parker, E. R. (2016). Potential future fisheries yields in shelf waters: a model study of the effects of climate change and ocean acidification. Biogeosciences 13, 441–454.
Veran, S., Gimenez, O., Flint, E., Kendall, W. L., Doherty, P. F. Jr., and Lebreton, J. D. (2007). Quantifying the impact of longline fisheries on adult survival in the black-footed albatross. J. Appl. Ecol. 44, 942–952.
Vichi, M., Allen, J. I, Masina, S., and Hardman-Mountford, N. J. (2011). The emergence of ocean biogeochemical provinces: a quantitative assessment and a diagnostic for model evaluation. Global Biogeochem. Cycles 25:3867.
Videau, C. (1987). Primary production and physiological state of phytoplankton at the ushant tidal front (west coast of brittany, france). Mar. Ecol. Prog. Ser. 35, 141–151. doi: 10.3354/meps035141
Votier, S. C., Hatchwell, B. J., Beckerman, A., McCleery, R. H., Hunter, F. M., Pellatt, J., et al., (2005). Oil pollution and climate have wide-scale impacts on seabird demographics. Ecol. Lett. 8, 1157–1164. doi: 10.1111/j.1461-0248.2005.00818.x
Waggitt, J. J., Dunn, H. K., Evans, P. G., Hiddink, J. G., Holmes, L. J., Keen, E., et al., (2018b). Regional-scale patterns in harbour porpoise occupancy of tidal stream environments. ICES J. Mar. Sci. 75, 701–710. doi: 10.1093/icesjms/fsx164
Waggitt, J. J., Cazenave, P. W., Howarth, L. M., Evans, P. G., van der Kooij, J., and Hiddink, J. G. (2018a). Combined measurements of prey availability explain habitat selection in foraging seabirds. Biol. Lett. 14:20180348. doi: 10.1098/rsbl.2018.0348
Waggitt, J. J., Cazenave, P. W., Torres, R., Williamson, B. J., and Scott, B. E. (2016b). Quantifying pursuit-diving seabirds’ associations with fine-scale physical features in tidal stream environments. J. Appl. Ecol. 53, 1653–1666. doi: 10.1111/1365-2664.12646
Waggitt, J. J., Cazenave, P. W., Torres, R., Williamson, B. J., and Scott, B. E. (2016a). Predictable hydrodynamic conditions explain temporal variations in the density of benthic foraging seabirds in a tidal stream environment. ICES J. Mar. Sci. 73, 2677–2686. doi: 10.1093/icesjms/fsw100
Waggitt, J. J., Evans, P. G., Andrade, J., Banks, A. N., Boisseau, O., Bolton, M., et al., (2020). Distribution maps of cetacean and seabird populations in the North-East Atlantic. J. Appl. Ecol. 57, 253–269.
Waggitt, J. J., Robbins, A. M., Wade, H. M., Masden, E. A., Furness, R. W., Jackson, A. C., et al., (2017). Comparative studies reveal variability in the use of tidal stream environments by seabirds. Mar. Policy 81, 143–152.
Waggitt, J. J., and Scott, B. E. (2014). Using a spatial overlap approach to estimate the risk of collisions between deep diving seabirds and tidal stream turbines: a review of potential methods and approaches. Mar. Policy 44, 90–97.
Wakefield, E. D., Owen, E., Baer, J., Carroll, M. J., Daunt, F., Dodd, S. G., et al., (2017). Breeding density, fine-scale tracking, and large-scale modelling reveal the regional distribution of four seabird species. Ecol. Appl. 27, 2074–2091. doi: 10.1002/eap.1591
Wakelin, S. L., Artioli, Y., Butenschön, M., Allen, J. I., and Holt, J. T. (2015). Modelling the combined impacts of climate change and direct anthropogenic drivers on the ecosystem of the northwest European continental shelf. J. Mar. Syst. 152, 51–63.
Wakelin, S. L., Artioli, Y., Holt, J. T., Butenschön, M., and Blackford, J. (2020). Controls on near-bed oxygen concentration on the Northwest European continental shelf under a potential future climate scenario. Prog. Oceanogr. 187:102400.
Wanless, S., Frederiksen, M., Walton, J., and Harris, M. P. (2009). Long-term changes in breeding phenology at two seabird colonies in the western North Sea. Ibis 151, 274–285.
Wanless, S., Harris, M. P., Newell, M. A., Speakman, J. R., and Daunt, F. (2018). Community-wide decline in the occurrence of lesser sandeels ammodytes marinus in seabird chick diets at a North Sea colony. Mar. Ecol. Prog. Ser. 600, 193–206. doi: 10.3354/meps12679
Ware, D. M., and Thomson, R. E. (2005). Bottom-up ecosystem trophic dynamics determine fish production in the Northeast Pacific. Science 308, 1280–1284. doi: 10.1126/science.1109049
Weston, K., Fernand, L., Mills, D. K., Delahunty, R., and Brown, J. (2005). Primary production in the deep chlorophyll maximum of the central North Sea. J. Plankton Res. 27, 909–922. doi: 10.1093/plankt/fbi064
Whitt, C., Pearlman, J., Polagye, B., Caimi, F., Muller-Karger, F., Copping, A., et al., (2020). Future vision for autonomous ocean observations. Front. Mar. Sci. 7:697.
Whyte, K. F., Russell, D. J., Sparling, C. E., Binnerts, B., and Hastie, G. D. (2020). Estimating the effects of pile driving sounds on seals: Pitfalls and possibilities. J. Acoustical Soc. Am. 147, 3948–3958. doi: 10.1121/10.0001408
Williamson, B., Fraser, S., Williamson, L., Nikora, V., and Scott, B. (2019). Predictable changes in fish school characteristics due to a tidal turbine support structure. Renewable Energy 141, 1092–1102.
Williamson, B. J., Fraser, S., Blondel, P., Bell, P. S., Waggitt, J. J., and Scott, B. E. (2017). Multisensor acoustic tracking of fish and seabird behaviour around tidal turbine structures in Scotland. IEEE J. Oceanic Eng. 42, 948–965.
Williamson, L. D., Scott, B. E., Laxton, M. R., Bachl, F. E., Illian, J. B., Brookes, K. L., et al., (2021). Spatiotemporal variation in harbour porpoise distribution and foraging across a landscape of fear. Mar. Mammal Sci. 2021: 12839.
Willsteed, E., Gill, A. B., Birchenough, S. N., and Jude, S. (2017). Assessing the cumulative environmental effects of marine renewable energy developments: establishing common ground. Sci. Total Environ. 577, 19–32. doi: 10.1016/j.scitotenv.2016.10.152
Willsteed, E. A., Jude, S., Gill, A. B., and Birchenough, S. N. (2018). Obligations and aspirations: a critical evaluation of offshore wind farm cumulative impact assessments. Renewable Sust. Energy Rev. 82, 2332–2345.
Wilson, B., Benjamins, S., and Elliott, J. (2013). Using drifting passive echolocation loggers to study harbour porpoises in tidal-stream habitats. Endangered Species Res. 22, 125–143.
Wolf, J., De Dominicis, M., Lewis, M., Neill, S., O’Hara Murray, R., Scott, B., et al., (2021). “Environmental issues for offshore renewable energy,” in Reference Module in Earth Systems and Environmental Sciences (Amsterdam: Elsevier).
Wolf, J., Yates, N., Brereton, A., Buckland, H., De Dominicis, M., Gallego, A., et al., (2016). The scottish shelf model. part 1: shelf-wide domain. Scottish Mar. Fresh. Sci. 7:151.
Wolf, S. G., Sydeman, W. J., Hipfner, J. M., Abraham, C. L., Tershy, B. R., and Croll, D. A. (2009). Range-wide reproductive consequences of ocean climate variability for the seabird Cassin’s Auklet. Ecology 90, 742–753. doi: 10.1890/07-1267.1
Woodson, C. B., and Litvin, S. Y. (2015). Ocean fronts drive marine fishery production and biogeochemical cycling. Proc. Natl. Acad. Sci. U.S.A. 112, 1710–1715. doi: 10.1073/pnas.1417143112
Wright, P. J., Pinnegar, J. K., and Fox, C. (2020). Impacts of climate change on fish, relevant to the coastal and marine environment around the UK. MCCIP Sci. Rev. 2020, 354–381.
Zamon, J. E. (2001). Seal predation on salmon and forage fish schools as a function of tidal currents in the San Juan Islands, Washington, USA. Fish. Oceanogr. 10, 353–366. doi: 10.1046/j.1365-2419.2001.00180.x
Zamon, J. E. (2002). Tidal changes in copepod abundance and maintenance of a summer coscinodiscus bloom in the southern san juan channel, san juan islands, USA. Mar. Ecol. Prog. Ser. 226, 193–210. doi: 10.3354/meps226193
Keywords: marine ecosystem, marine spatial planning, climate change, fisheries, marine protected areas, seabirds and marine mammals, potential energy anomaly, net primary production
Citation: Trifonova N, Scott B, De Dominicis M and Wolf J (2022) Use of Our Future Seas: Relevance of Spatial and Temporal Scale for Physical and Biological Indicators. Front. Mar. Sci. 8:769680. doi: 10.3389/fmars.2021.769680
Received: 02 September 2021; Accepted: 16 December 2021;
Published: 25 January 2022.
Edited by:
Rowan Trebilco, Centre for Marine Socioecology, AustraliaReviewed by:
Kurt Heim, United States Fish and Wildlife Service (USFWS), United StatesScott Large, National Oceanic and Atmospheric Administration (NOAA), United States
Copyright © 2022 Trifonova, Scott, De Dominicis and Wolf. This is an open-access article distributed under the terms of the Creative Commons Attribution License (CC BY). The use, distribution or reproduction in other forums is permitted, provided the original author(s) and the copyright owner(s) are credited and that the original publication in this journal is cited, in accordance with accepted academic practice. No use, distribution or reproduction is permitted which does not comply with these terms.
*Correspondence: Neda Trifonova, neda.trifonova@abdn.ac.uk