- 1Key Laboratory of Sustainable Development of Marine Fisheries, Ministry of Agriculture and Rural Affairs, Shandong Provincial Key Laboratory of Fishery Resources and Ecological Environment, Yellow Sea Fisheries Research Institute, Chinese Academy of Fishery Sciences, Qingdao, China
- 2College of Fisheries, Ocean University of China, Qingdao, China
- 3Function Laboratory for Marine Fisheries Science and Food Production Processes, Qingdao National Laboratory for Marine Science and Technology, Qingdao, China
- 4National Field Observation and Research Center for Changdao Marine Ecosystem, Yantai, China
- 5School of Biosciences, The University of Melbourne, Parkville, VIC, Australia
For many fish stocks, such as Pampus argenteus and Setipinna taty in China, size composition data are more accessible than catch data. Varied results can arise when different length-based stock assessment models are applied to these data, and fishery managers often need to reconcile conflicting estimates of population status. Superensemble modeling, a relatively recent innovation in fish stock assessments commonly used in other fields, may provide an effective solution to resolving uncertainties among the results from multiple length-based models. To verify potential for this approach to improve estimates of population status, we applied ensemble modeling to fit simulated data of P. argenteus and S. taty in the Bohai and Yellow Seas using predictions from a length-based integrated mixed effects (LIME) and length-based spawning potential ratio (LB-SPR) models as covariables in a superensemble model developed in this study. All simulation modeling of P. argenteus and S. taty in the Bohai and Yellow Seas was conducted using the operating model in the R package LIME. Initially, the LIME and LB-SPR performances were tested separately under three scenarios of fishing mortality and recruitment variability (“equilibrium scenario,” “endogenous scenario,” and “one-way base scenario”). Then, estimates of spawning potential ratio (SPR) were combined with the superensemble models (a linear model, a support vector machines, a random forest and a boosted regression tree). We trained our superensemble models with 80% of the simulated data and tested them with the remaining 20%. Our results showed that superensemble modeling substantially improved the estimates of SPR, with support vector machines performing the best at estimating population status: precision improved by 12.7% for S. taty and 8% for P. argenteus on average (namely, median absolute proportional error decreased by 0.127 and 0.08 on average) compared to the individual models. This finding has important implications for fisheries management in the context of species for which catch data are unavailable. Applying the size composition survey data, the results from support vector machines superensemble model suggested that neither S. taty nor P. argenteus in the Bohai Sea in 2019 are overfished, but the stock status of P. argenteus warrants vigilant monitoring.
Introduction
Stock assessment involves providing scientific, quantitative evaluations to objectively inform fisheries management (Hilborn and Walters, 1992). Globally, only about 50 percent of exploited fish species have been assessed due to limitations imposed by the data requirements of traditional or conventional stock assessment methods (Ricard et al., 2012; RAM Legacy Stock Assessment Database, 2018). Evidence-based fisheries management decisions rely on stock assessments to provide crucial insights into the status of fish stocks and fisheries. Due to an urgent need for managing an increasing number of smaller less productive or marginal fish stocks as well as halting depletion and promoting recovery of long established once highly productive stocks, in recent years, data-limited methods to estimate stock status and exploitation have developed rapidly (MacCall, 2009; Dick and MacCall, 2011; Free et al., 2017). Most of these methods can be divided into catch-based methods and length-based methods.
Not all the catch histories for various fish species can be obtained from fisheries statistical records. In many fisheries with limited monitoring capacity, it is often easier to collect length measurements from scientific surveys or catch sampling, such as for Pampus argenteus and Setipinna taty in the Bohai and Yellow Seas of China, than to quantify total catch. P. argenteus is a warm water fish species which aggregates to form a spatially clustered distribution of schools and is one of the main commercial species in China coastal waters (Liu et al., 1990). The P. argenteus population in China can be divided into the Bohai-Yellow Sea stock and the eastern China Sea stock (Liu et al., 1990). The Bohai-Yellow Sea stock has been the main target of trawl and mass drift net fishing for many years (Jin et al., 2005a,2006; Tang, 2006). Setipinna taty is distributed in spatially clustered schools along the coast of China (Liu et al., 1990; Jin et al., 2006, 2015). In recent years, with the decline of traditional economically remunerative fish resources, S. taty has become one of the main targets of all kinds of commercial net fishing adjacent to the coasts of the Bohai and Yellow Seas (Jin et al., 2006, 2015). Length-based assessment methods, which simply require mean length or length composition of the catch and estimates of life history parameters, have become increasingly prevalent for evaluating the status of data-limited or data-poor fish stocks (Thorson and Cope, 2014; Hordyk et al., 2015; Then et al., 2015), and have great potential for application in fish species assessment where catch data are unavailable.
Exploratory analysis of existing data and some background research (Rudd and Thorson, 2018; Pons et al., 2019) found that different results are obtained when applying different stock assessment models that rely solely on length measurements. Rational design of management strategies must consider the uncertainty in model outputs (Schnute and Hilborn, 1993) and fisheries managers must often reconcile conflicting estimates of population status and trend. Taking the average or weighted average of several model predictions, is one often tried and effective solution (e.g., Burnham and Anderson, 2002; Anderson et al., 2017). In population assessments where the input data and error assumptions are compatible, there are many successful examples in which model averaging has objectively combined the results of different models (Brodziak and Piner, 2010; Millar et al., 2015). However, some studies (Schnute and Hilborn, 1993; Anderson et al., 2017) have demonstrated that when model or data errors are incompatible, the most likely parameter values are not intermediary to conflicting values; instead, they occur toward one of the apparent extremes.
Superensemble modeling, often simply referred to as ensemble modeling, has been commonly used with success for climate and weather forecasting It provides a technical framework for drawing predictions from a group of models as inputs into a separate statistical model (Krishnamurti et al., 1999; Hamill et al., 2012). This technique has been used to improve estimates of population status by optimally leveraging multiple catch-based model predictions (Anderson et al., 2017). This approach may overcome the shortcomings of model-averaging methods and provide an effective solution for reducing the uncertainties that arise in the results from multiple length-based models to identify the most plausible combination of model parameters.
In fisheries without catch data or information on relative or absolute abundance, stock assessments typically use spawning potential ratio (SPR) as an alternative reference point to biomass at maximum sustainable yield (BMSY) (ICCAT, 2017; Pons et al., 2019). The ensemble-method framework developed in this study will provide appropriate options to inform the management of fish stocks for which catch data are unavailable.
Materials and Methods
To verify the potential of ensemble methods for improving estimates of population status, we fitted simulated data for stocks of P. argenteus and S. taty in the Bohai and Yellow Seas using predictions from a Length-based Integrated Mixed Effects (LIME, Rudd and Thorson, 2018) model and a length-based spawning potential ratio (LB-SPR, Hordyk et al., 2015) model as covariables in the superensemble models developed in this study, and compared their predictive performance against each other and their individual component models. LIME and LB-SPR are length-based methods that are commonly used in contemporary stock assessments, and are based on non-equilibrium and equilibrium principles, respectively. Both models require a minimum of 1 year of length composition data together with assumptions about growth, natural mortality, and maturity to estimate the spawning potential ratio (SPR) biological reference point, defined as the proportion of unfished reproductive potential at a given level of fishing pressure (Goodyear, 1993; Hordyk et al., 2015; Rudd and Thorson, 2018).
Firstly, a comparison was undertaken between the two methods using an operating model to produce data for simulated populations (P. argenteus and S. taty in the Bohai and Yellow Seas) under different length data groupings with three scenarios of fishing mortality and recruitment variability combinations (Figure 1). All simulation modeling of populations of the two species were conducted using the operating model in the R package LIME from which estimates of SPR were obtained for each scenario. The performance of the ensemble models using the simulated datasets was evaluated by using 80% of the simulated data for training and the remaining 20% for testing. Finally, based on insights about the robustness of the methods, the stock status of P. argenteus and S. taty in the Bohai Sea were estimated (Figure 2).
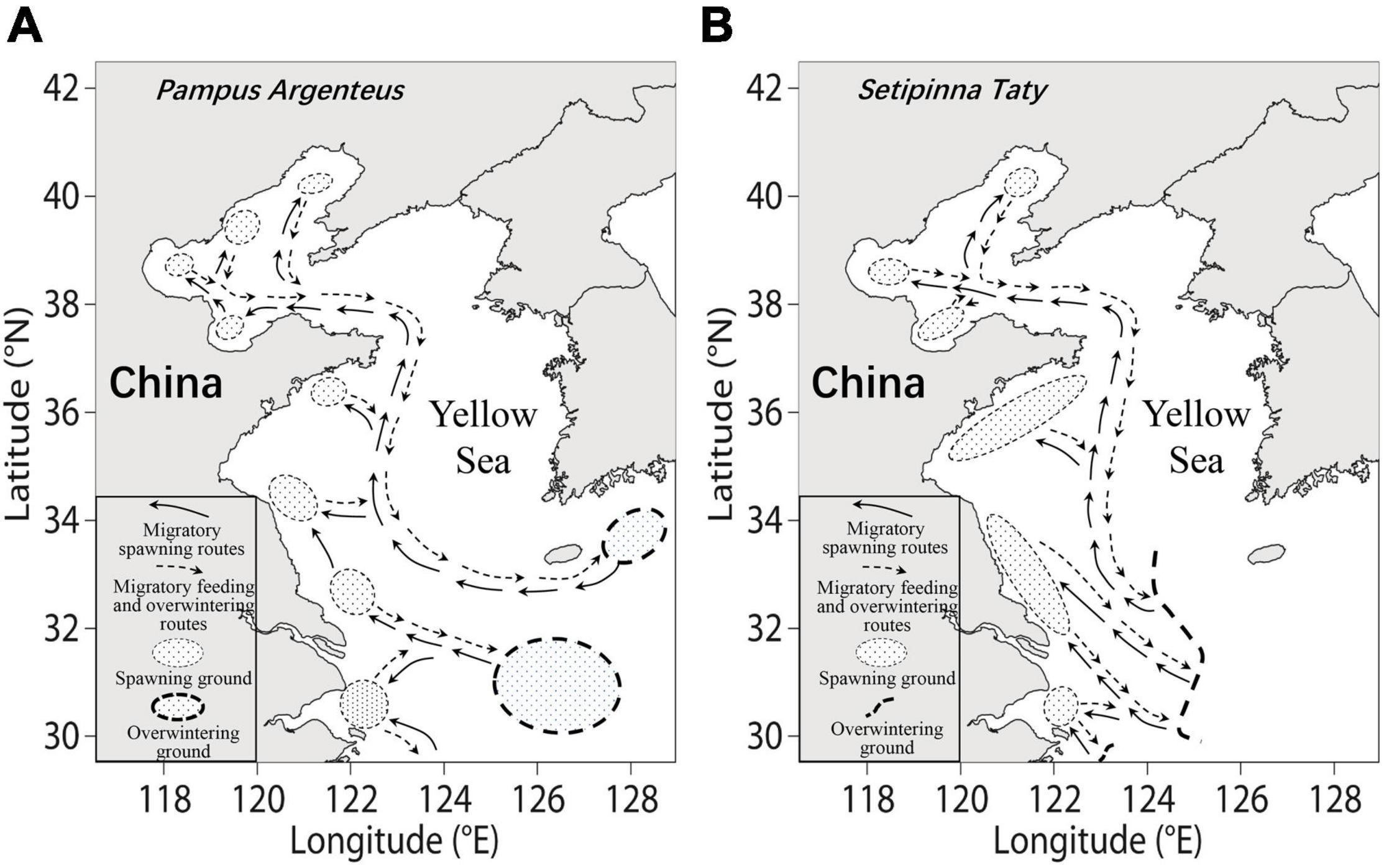
Figure 1. Migration routes of (A) P. argenteus and (B) S. taty in the Bohai and Yellow Seas. Refer to Liu et al. (1990).
Operating Models and Data Generation
Population estimates for simulation were generated by an operating model (all estimation was completed via the R package Template Model Builder, TMB, Kristensen et al., 2016) and developed in the LIME package (Rudd and Thorson, 2018;1) in the statistical software R (R Development Core Team, 2019). Rudd and Thorson (2018, Table 2 and Table 3 in their manuscript) described the population dynamic equations used in the operating model and functions for generating data. For natural mortality of P. argenteus and S. taty in the Bohai and Yellow Seas, the respective values (Table 1) were assumed to be constant so that in each instance the median value of natural mortality was chosen from estimates made using multiple methods (the Barefoot Ecologist’s Toolbox hosts a tool; Cope, 2017). For other biological and fisheries parameters of P. argenteus and S. taty, values were taken from local studies (Zhao, 1987; Liu et al., 1990; Tang and Ye, 1990; Chen, 1991; Jin et al., 2005a; Chen et al., 2018; Xu et al., 2019). Life history parameters for growth and length-at maturity are also listed in Table 1.

Table 1. Life history parameters for each species assumed in the models; where L∞ and K are the von Bertalanffy asymptotic length and Brody growth coefficient, respectively and L50 is the length at which 50% of the population is mature.
Levels of initial biomass depletion compared to carrying capacity of each simulated population were drawn from a uniform distribution between 0.35 and 0.95, and three scenarios involving combinations of fishing mortality and recruitment variability were explored (Figure 3):
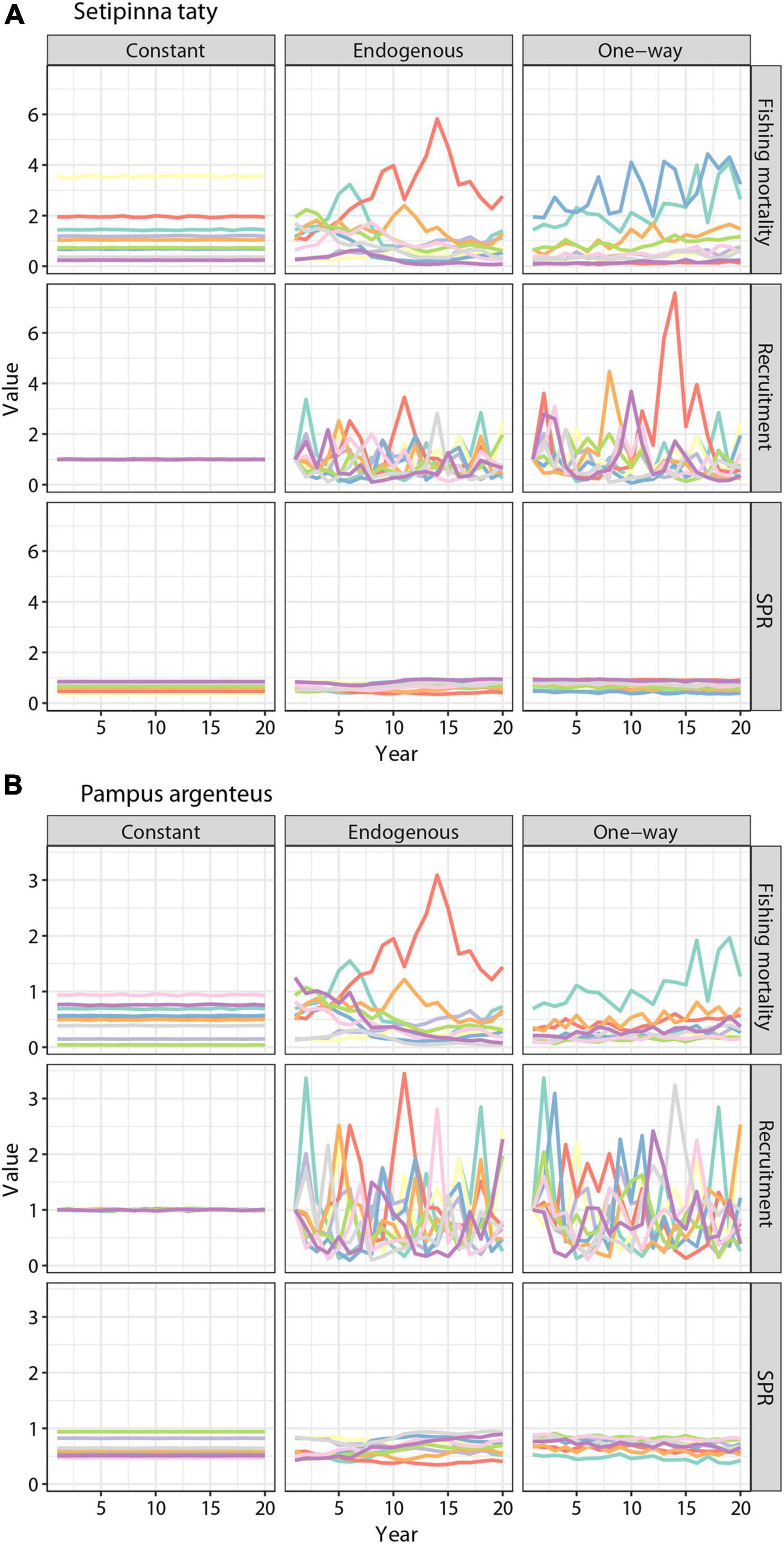
Figure 3. Scenarios of fishing mortality and recruitment under equilibrium and variable conditions for the three life-history types. The lines show 10 randomly chosen iterations out of 100 as examples. (A) S. taty. (B) P. argenteus.
(1) “constant” scenario: a constant exploitation rate and recruitment assumptions;
(2) “endogenous” scenario: an exploitation rate coupled with biomass, and a Beverton–Holt spawner-recruit function (Beverton and Holt, 1957); and
(3) “one-way” scenario: a Beverton–Holt spawner-recruit function and an exploitation rate coupled with fishing mortality changing linearly from the rate that would result in the randomly chosen initial depletion to F20% which was calculated deterministically based on the biological information and selectivity associated with each life-history type (Rudd and Thorson, 2018; see text footnote 1).
Scenario (2) and (3) included a standard deviation for fishing mortality equal to 0.2 (Rudd and Thorson, 2018), a standard deviation of recruitment residuals equal to 0.737 and a first-order autoregressive coefficient equal to 0.426, the mean of the predictive distribution from a meta-analysis of recruitment variability in global fish orders (Thorson et al., 2014). Selectivity at length was assumed to follow a two-parameter logistic model (eq. 4 in the Rudd and Thorson, 2018), with an estimated parameter length at 50% selectivity and a second parameter representing the difference between length at 95 and 50% selectivity. We repeated this process running 100 iterations or replicates for each combination for each population to generate the simulation data. More information can be found on Github (see text footnote 1) and in Rudd and Thorson (2018).
A sample size of 250 individuals was assumed, which approximates the more realistic sample sizes of P. argenteus and S. taty measured annually in the Bohai Sea. Although our ensemble models were only fitted to estimated values obtained from individual length-based models with length data as the sole input, the performance of the LIME model with rich data assumptions was nevertheless tested. This helped to gauge the level of results that could be deemed acceptable when individual length-based models were fitted solely to length data. Therefore, a total of 13 data-availability scenarios for LIME were set up: (1): “Rich20” with 20 years of catch, abundance index, and length data; (2) “Rich10” with 10 years of total catch, abundance index, and length data; (3) “Rich5” with 5 years of total catch, abundance index, and length data; (4) “I_LC20” with 20 years of abundance index, and length data; (5) “I_LC10” with 10 years of abundance index, and length data; (6) “I_LC5” with 5 years of abundance index, and length data; (7) “C_LC20” with 20 years of total catch, and length data; (8) “C_LC10” with 10 s of total catch, and length data; (9) “C_LC5” with 5 years of total catch, and length data; (10) “LC20” with 20 years of length data; (11) “LC10” with 10 years of length data; (12) “LC5” with 5 years of length data; and (13) “LC1” with 1 years of length data. Only one data availability scenario was set up for LB-SPR: “LBSPR” with 1 year of length data.
Individual Length-Based Models and Testing Performance
Two individual data-limited length-based models that use simulated data and basic life-history and/or fishery parameters to estimate SPR were fitted. These length-based models were chosen because they can be fitted to a majority of fisheries around the world, are well established in the literature, and have been extensively simulation-tested (Rudd and Thorson, 2018; Chong et al., 2019; Pons et al., 2019, 2020; Halim et al., 2020).
Length-based spawning potential ratio is one of the prominent length-based methods for estimating reference points in data-limited fisheries and enables a rapid assessment of stock status relative to unfished levels. This model assumes equilibrium conditions (invariant recruitment and mortality) by using a static, equilibrium-based relative age-structured model (Hordyk et al., 2015; Prince et al., 2015). Since the status determination of the LB-SPR model is based on 1 year of data at a time, we only used the length data of the final year to calculate the SPR of that year. The inputs to the LB-SPR included the ratio of the natural mortality coefficient to the von Bertalanffy growth coefficient; length data in each year; the von Bertalanffy asymptotic length parameter; the lengths where 50 and 95% of the fish are mature; and length–weight parameters. LB-SPR estimates the ratio of fishing mortality to natural mortality and the lengths at 50 and 95% selectivity to best fit the predicted and observed length composition proportions, and derives SPR, outputting estimates for these four values for each year with length data (Hordyk et al., 2015). In this study, we used the LB-SPR package version 0.1.5 in R (Hordyk, 2017).
Length-based integrated mixed effects is an age-structured population dynamics mixed model and can account for variable fishing mortality and recruitment when there are only length data from a single year as well as assumed life-history parameters; including the length-at-age relationship, von Bertalanffy growth parameters, allometric length-weight parameters, natural mortality coefficient, and length at 50% maturity (Rudd and Thorson, 2018). It can also accommodate multiple years and types of data, including both length data and index data and/or catch data, in an integrated manner to improve the estimation of changes in fishing mortality over time (Rudd and Thorson, 2018). LIME estimates lengths at 50 and 95% selectivity to the fishing gear; the Dirichlet-multinomial parameter; the recruitment standard deviation and annual fishing mortality coefficient as fixed effects; and estimates of annual recruitment as random effects. Compared with LB-SPR, when fitting length data from more than 1 year, LIME does not assume equilibrium conditions when recruitment is estimable. In our model runs, when the final gradient for all parameters was less than 0.001, the model converged. For each combination of life-history type, data availability scenario, fishing mortality pattern, and recruitment dynamics, we obtained 100 iterations of generated data and ran the estimation model for each set. In this study, we used the LIME package 2.1.3 version (Rudd and Thorson, 2018).
To assess the performance of the LIME and LB-SPR models under different scenarios we compared the outputs to the simulated “truth” from the operating models, and used the relative error (RE, [estimated-true]/true) as an evaluation indicator.
Building the Ensemble Models and Testing Performance
The individual models combined as ensembles were intended to provide estimates of recent stock status (SPR). Therefore, the ensembles were applied to estimate SPR in the last year of the data series, which was of interest for both of management and conservation purposes. The SPR were used in the last year as the response variable and the predictions from the individual models (LIME and LB-SPR) as predictors in our ensemble models (Figure 2).
A model average for each population and four ensembles of varying complexity were compared: a random forest (RF), a support vector machine (SVM), a linear model (LM), and a boosted regression tree (generalized boosted regression modeling, GBM). The four ensemble models were fitted for each population separately (e.g., RF_PA for P. argenteus only, RF_ST for S. taty only), as well as fitting the models for a set comprising data from populations of both species (e.g., RF for both P. argenteus and S. taty).
These models can be described in terms of, the ensemble estimated SPR. The individual model estimates of SPR are represented as . The model average for each population was calculated as:
The linear model for each individual population or both populations combined was calculated as:
Support vector machines, based on mapping input space to a high-dimensional feature space where linear separation is easier than input space, provide a popular machine learning method and yet also represent a powerful technique for general (nonlinear) classification, regression and outlier detection with an intuitive model representation (Cortes and Vapnik, 1995; Bennett and Campbell, 2000; Chang and Lin, 2011). SVMs were initially used to train a data set (SPR in the last year of individual models fitting 80% of the iterations for simulated data under all fishing and recruitment scenarios) to obtain a model and secondly, the resultant ensemble model was used to predict information from a testing data set (SPR in the last year of individual models from fitting the remaining 20% iterations of simulated data under all fishing and recruitment scenarios). SVMs, based the pre-processing strategy in learning by mapping input space to a high-dimensional feature space, are used to find an optimal hyper-plane which maximizes the margin between itself and the nearest training examples in the new high-dimensional space and minimizes the expected generalization error (Seo, 2007). We fit SVMs with the e1071 package (Meyer et al., 2021) for R.
Generalized Boosted Regression Modeling (GBM), based on regression trees, is a type of regression model that is highly flexible, and has considerable success in predictive accuracy by maintaining a monotonic relationship between the response and each predictor (Friedman and Tibshirani, 2000; Ridgeway, 2007; Al-Mudhafar et al., 2016). GBM via the R gbm package (Ridgeway, 2007) was fitted using the default argument values.
Random forest (RF) modeling is also based on regression trees. In RF, each tree depends on the values of a random vector sampled independently and with the same distribution for all trees in the forest (Breiman, 2001). We fitted RF using the random Forest package (Liaw and Wiener, 2002) for R with the default argument values.
Repeated onefold cross-validation was used by randomly dividing the dataset into two sets, then building the ensemble model on eight-tenths of the data and evaluating its predictive performance on the remaining two-tenths. To assess the ability of the ensemble models to accurately and precisely estimate quantities of management interest i.e., SPR, the median absolute relative error (MARE) was used as an evaluation indicator to quantify precision between estimated and true SPR in the last year of data across the 20% iterations of simulated data.
Applying the Ensemble Models in the Assessment of Pampus argenteus and Setipinna taty in the Bohai Sea
As an example, the LIME and LB-SPR models were applied to stocks of species of interest (i.e., P. argenteus and S. taty in the Bohai Sea) and then the resultant SPR estimates of stock status were used as data in the previously built ensemble models (i.e., RF, RF_PA, RF_ST, SVM, SVM_PA, SVM_ST, GBM, GBM_PA, GBM_ST, LM, LM_PA, LM_ST, and Model average). The Bohai Sea is an important spawning ground for the Bohai-Yellow Sea stocks of P. argenteus and S. taty thereby playing an important role in future years’ stock recruitment (Liu et al., 1990; Jin et al., 2005a,2006, 2015). May to July is the spawning period for the Bohai-Yellow Sea stock of P. argenteus and July to November is the main feeding period. At the end of November, the stock gradually migrates toward the Yellow Sea for overwintering (Liu et al., 1990; Jin et al., 2005a,2006). In the Bohai Sea, S. taty has been the dominant species since the 1980s and plays an important role in the nearshore fish community (Jin et al., 2006). Setipinna taty spawn in the Bohai Sea from mid-May to June, and then return to the wintering ground in the Yellow Sea until the end of November (Liu et al., 1990; Jin et al., 2006, 2015).
The application of ensemble models in this study was based on P. argenteus and S. taty length data that were collected by fixed-station bottom trawl surveys conducted in the Bohai Sea during spring (May), summer (August- September), autumn (October- November) and winter (December) of 2016–2019 by the Yellow Sea Fisheries Research Institute, Chinese Academy of Fishery Sciences (Figure 4).
The modeling results can potentially inform a harvest strategy that targets a fishing mortality rate that is expected to result in 40% of unfished spawning output (termed “SPR40%”), which is considered risk-averse for many stocks with very low resilience (Clark, 2002). SPR30% is considered a threshold below which a stock is considered to have been overfished (Clark, 2002; Nadon et al., 2015; Rudd and Thorson, 2018). Therefore, these values were calculated as possible fishing mortality reference points to evaluate the status of the P. argenteus and S. taty in the Bohai Sea to inform future fishery management decisions.
Results
Simulation Testing: Individual Length-Based Model Performance Across Fishing Mortality, Recruitment Variability, and Data Scenarios
Simulation testing demonstrated that the RE distributions of the LIME and LB-SPR methods under varying conditions were relatively dispersed compared with those under equilibrium conditions (Figure 5). In addition, the RE distribution for P. argenteus was relatively dispersed compared with that for S. taty. When the time-period of data becomes shorter, the bias (bias is measured as MRE) of the LIME estimator for both P. argenteus and S. taty will become larger.
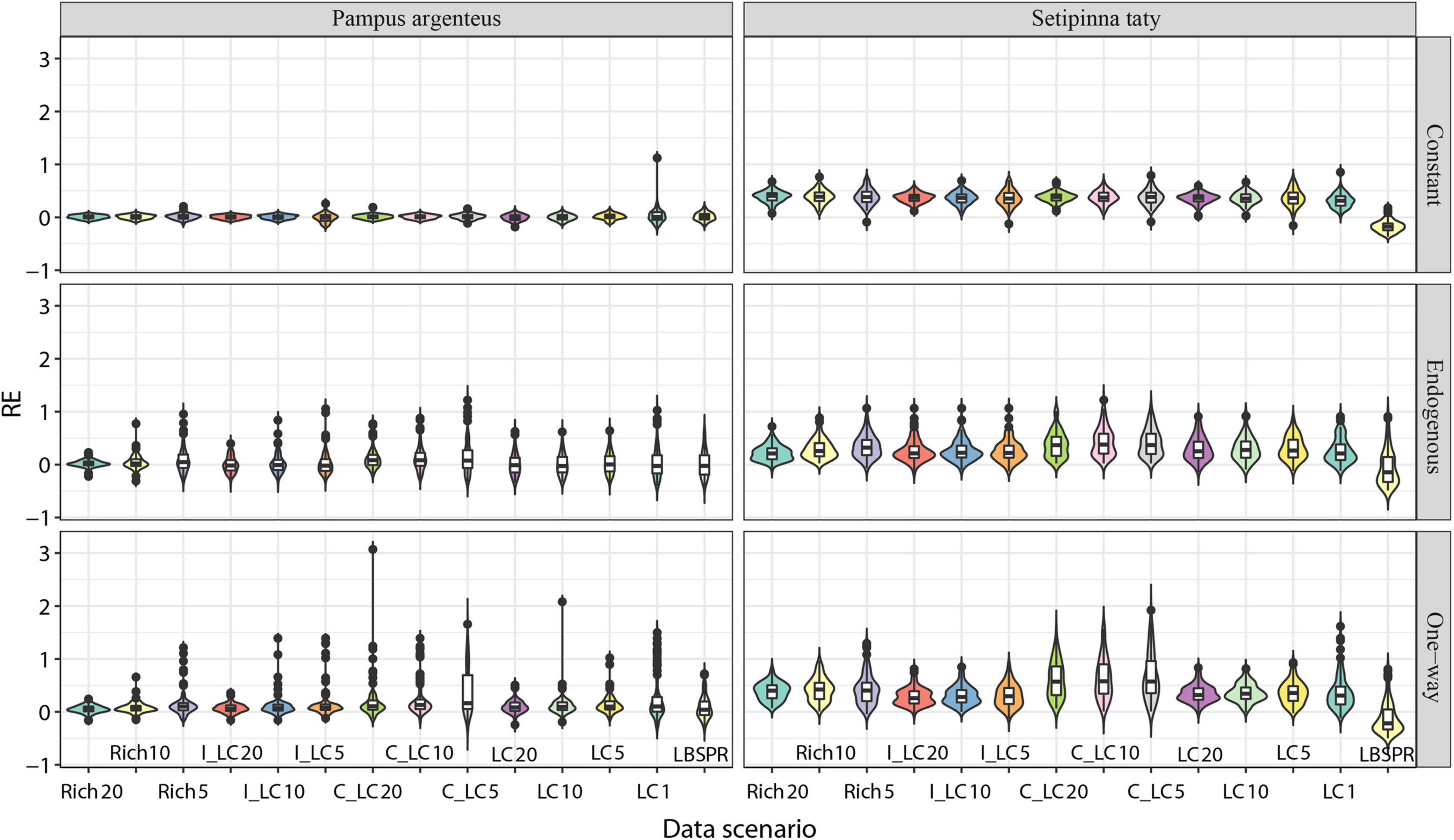
Figure 5. Distribution of relative error ([estimated – true]/true) for SPR in the last year of the data series for 100 iterations of simulated populations (S. taty and P. argenteus in the Bohai and Yellow Seas) across LIME and LB-SPR data availability scenarios for the two life-history types and scenarios of equilibrium and variable fishing mortality and recruitment with 250 length measurements annually.
Our results showed that for P. argenteus, both LIME and LB-SPR can estimate unbiased SPR when length data are available and biological characteristics are correctly specified across various scenarios of fishing mortality and recruitment patterns (Figure 5). Compared with P. argenteus, the SPR estimated for S. taty by both LIME and LB-SPR had a large relative error. For P. argenteus, the mean bias in SPR of LIME and LB-SPR was 0.008 (from −0.005 to 0.017) across all data scenarios under equilibrium conditions (constant scenario). Under an endogenous scenario, the mean bias in SPR of LIME and LB-SPR for P. argenteus was 0.014 (from −0.026 to 0.085) across all data scenarios, and the SPR from LIME demonstrated relatively large deviations under scenarios with only length and catch data (C_LC). Under a one-way scenario, the mean bias in the SPR of LIME and LB-SPR for P. argenteus was 0.088 (from 0.039 to 0.163) across all data scenarios, and the SPR of LIME also demonstrated relatively large deviations under scenarios with only length and catch data (C_LC). In general, LB_SPR and LIME with length only data had a similar bias for P. argenteus.
For S. taty, the mean bias in the SPR of LIME and LB-SPR was 0.331 (from −0.176 to 0.405) across all data scenarios under equilibrium conditions. Under endogenous scenarios, the mean bias in SPR of LIME and LB-SPR for S. taty was 0.243 (from −0.146 to 0.379) across all data scenarios. But those RE distributions had relatively large deviations compared with those obtained under equilibrium conditions. Under a one-way scenario, the mean bias in SPR of LIME and LB-SPR for S. taty was 0.348 (from −0.217 to 0.576) across all data scenarios. In general, for S. taty, the LB-SPR estimate was lower than its true value, while the LIME estimate was higher than its true value. It seems that a persistent bias exists for both individual length-based assessment methods compared with the true values in the OM.
Performance of the Ensemble Models
Ensemble methods, and in particular the machine-learning ensemble models (support vector machines, SVMs), generally improved the estimates of stock status beyond those of any individual model (Table 2). Compared to the LIME models with LC20, LC10, LC5, LC1, and SVM ensembles (SVM20, SVM10, SVM5, and SVM1) decreased the MARE (median absolute relative error to quantify precision) by averages of 0.257, 0.252, 0.243, 0.130, respectively for S. taty and −0.014, 0.004, 0.011, 0.036, respectively for P. argenteus. Compared to the LB-SPR model, SVM ensembles (SVM20, SVM10, SVM5, and SVM1) decreased the MARE by averages of 0.161, 0.150, 0.148, 0.136, respectively for S. taty and 0.021, 0.019, 0.011, −0.006, respectively for P. argenteus. Compared to the LB-SPR model, only SPR from individual models with the simulation of individual species (S. taty or P. argenteus) can be used to build ensemble models with higher accuracy than those fitted by both species in combination.
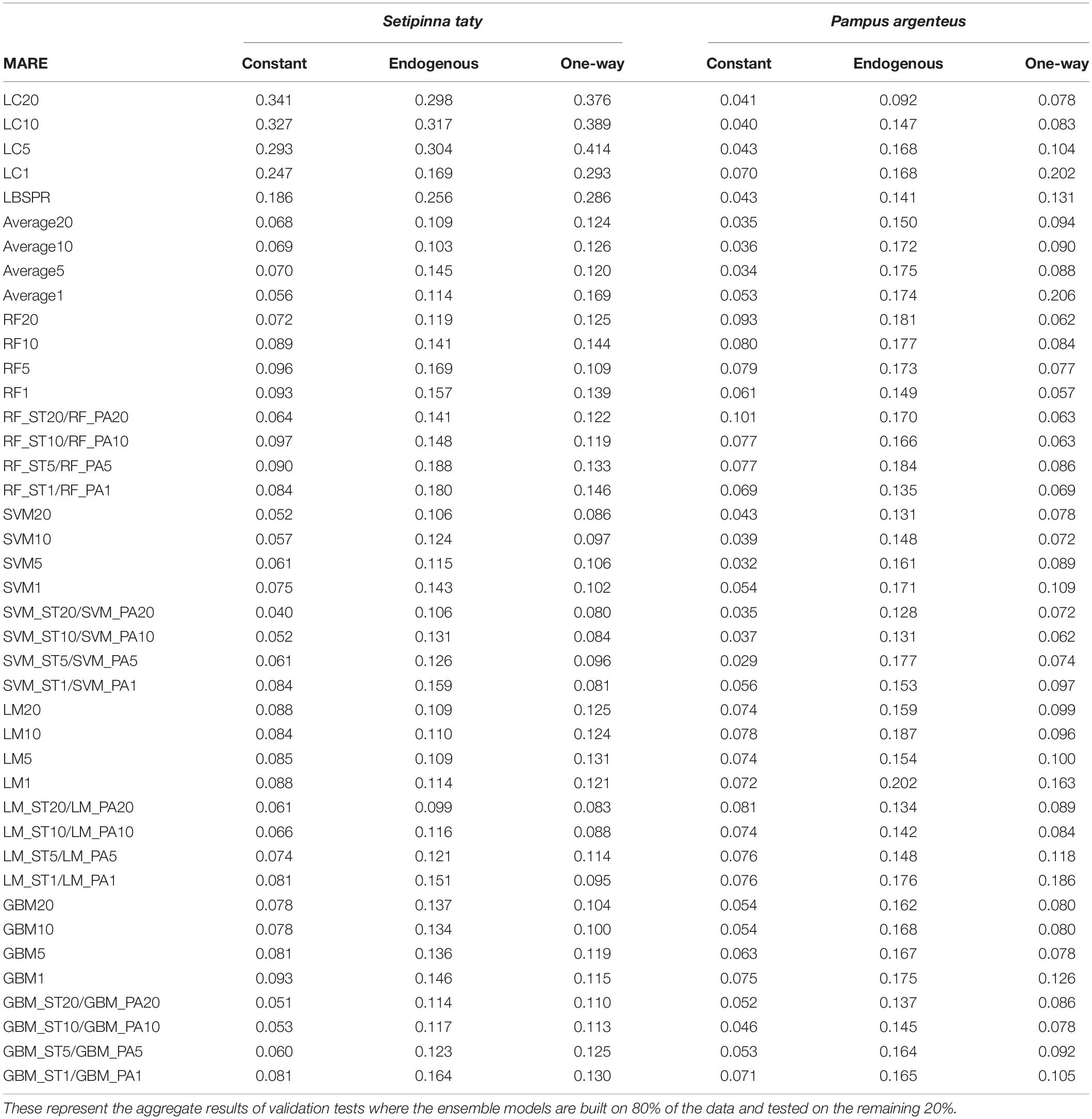
Table 2. Ensemble models performance in estimates of status (SPR in the last year) compared with LB-SPR and LIME.
Assessments of Setipinna taty and Pampus argenteus in the Bohai Sea Using Ensemble Models
We applied ensemble models using the SPR from LIME and LB-SPR with the length composition data from S. taty and P. argenteus from the surveys conducted in the Bohai Sea during 2016–2019 (Table 3). The optimal ensemble model (SVM) showed that the SPR estimates for P. argenteus and S. taty in 2019 were 0.388 and 0.525, respectively. These results (> SPR30%) indicated that there was no evidence of overfishing for P. argenteus and S. taty in the Bohai Sea. Notwithstanding this result, the SPR of P. argenteus (0.388< SPR40%) indicated that extra monitoring attention is needed, and further protection warranted to reduce the risk of this stock becoming overfished. Most ensemble models tested produced consistent results.
Discussion
Applications of ensemble models were firstly applied to empirical data acquired from two data-poor Chinese stocks (P. argenteus and S. taty), which were then extended in a combination of equilibrium-based (LB-SPR) and relaxed equilibrium-based (LIME) length-based methods. As a mixed model, LIME attributed changes in the length composition data to variability in recruitment, keeping selectivity constant over time (Rudd and Thorson, 2018). LB-SPR allowed selectivity to change between years, but assumed recruitment was constant over time (Hordyk et al., 2015). Combined with simulation testing, the empirical examples provide a pathway for application of these ensemble methods to more data-limited stock assessments of Chinese fish species for which catch data are unavailable.
Application of the simulated dataset of known stock status showed that the individual models had variable success at estimating SPR in the last year of the data series, but with large deviations. Simulation testing comparing the performance of the LIME and LB-SPR methods in estimating SPR showed that LIME and LB-SPR performed better for P. argenteus (maximum age 6, asymptotic length (cm) 30.4, growth coefficient 0.25, and Natural mortality 0.817) across a range of life history, fishing mortality, and recruitment scenarios compared with that for S. taty (Maximum age 4, asymptotic length (cm) 20, growth coefficient 0.62, and Natural mortality 1.35). The results indicate that LIME overestimates SPR and LB-SPR underestimates SPR, thereby conferring poorer ability to estimate stock status for the rapidly growing, short-lived, small Engraulidae fish. Therefore, the conclusion of Rudd and Thorson (2018) that “with only length data, LIME performs well for the shorter-lived fish” which they inferred from studying only one species of short-lived fish needs further study for verification.
Ensemble methods provide a useful approach to situations where environmental resource management decisions must be made on the basis of multiple, potentially contrasting estimates of status (Anderson et al., 2017). Our results suggest that choosing a support vector machine ensemble model that allows for nonlinear relationships provides additional insight into individual model behavior and generally performed the best among all the models that we tested. However, not all ensemble models performed at their best in a variety of situations. The number of models included, and the structural forms of those individual component models, can greatly influence the performance of ensemble models (Ali and Pazzani, 1996; Dietterich, 2000; Tebaldi and Knutti, 2007). Ensemble models can exploit the best predictive performance of each of their individual component models which perform well under different conditions (Anderson et al., 2017). Whether the training dataset represents the dataset of interest will affect the performance of ensemble models (Knutti et al., 2009; Weigel et al., 2010), which is why we trained our ensemble models by considering the datasets produced by the various scenarios described in this paper. Inclusion of the dataset of interest in our training dataset, which improved the objectivity of the predictive performance of our models during their verification (Hastie et al., 2009).
Ensemble methods do not require theoretical information, or the further development of multi-model inference, because they can combine different types of models and model predictions via nonlinear functions that are tuned to known data (Stewart and Martell, 2015; Anderson et al., 2017). The results of our simulation testing illustrated that ensemble models can improve population status estimates of fish species in situations where catch data are unavailable. This is consistent with the results from ensemble modeling when compared with catch-based models worldwide (Anderson et al., 2017). The ensemble approach can provide a framework to enable managers to focus on decision-making rather than selecting from among assessment models or assumptions within those models (Stewart and Martell, 2015). The results also suggested that fundamentally different stock assessment modeling paradigms, LIME and LB-SPR, can be included in such an approach, and that it is more robust and convenient for assessing the stock status of Chinese fish species without catch data than attempting to select a single best length-based model. The challenges associated with diagnosing why different models (i.e., LIME and LB-SPR) yield different results, and the implications of these differences on management decisions is all the more reason for us to pursue ensemble modeling.
Over the past 30 years, many scholars have focused on the stock status of S. taty and P. argenteus in the Bohai Sea (for example, Tang and Ye, 1990; Jin et al., 2005b,2006). Based on the composition data of the fork length of S. taty from 1983 to 1985, Tang and Ye (1990) used a cohort analysis (Jones, 1981) to estimate that the resource utilization rate of S. taty in the Bohai and Yellow Seas is at an intermediary level of utilization. Compared with the 1980s, the biomass of P. argenteus and S. taty estimated from area-sweeping and acoustic survey methods has greatly reduced during the early 21st century (Jin et al., 2005b,2006). The concomitant limited availability of data has hindered the development of fish stock assessment and fishery management for both species. A lack of catch records for S. taty has limited the use of conventional assessment methods, so that this species cannot be assessed quickly and accurately. Recorded catches of P. argenteus have often included several related species, thereby confounding previous evaluations of its stock status (see Liu et al., 2013). Our application of ensemble methods by combining length-based models provides a solution which facilitates accurate evaluation of the state of stocks in which mixing of species in catches or other deficiencies in reported catches may occur. Through the application of survey data, the results from the ensemble modeling indicated that neither S. taty nor P. argenteus in the Bohai Sea was at an overfished level (of stock biomass) in 2019, but that the stock status of P. argenteus at an SPR <40% indicated that this species deserves special attention to ensure that it does not become more depleted. However, Jin et al. (2005b,2006) reported that the population structures of P. argenteus and S. taty have been trending toward younger cohorts of smaller fish during recent years (Jin et al., 2005b,2006). Results from the present study (SPR of 39% for P. argenteus and 53% for S. taty) when considered in conjunction with the previous work cited in this paper lend support to a risk-averse argument that managers should take measures to protect the stocks of both species to ensure their sustainable use and their role in the ecosystem (i.e., to increase the SPR of P. argenteus above 40% and maintain the SPR of S. taty well above 40%).
The ensemble modeling based on individual length-based models was initially used for the assessment of fishery species for which catch data were unavailable, but there is certainly ample scope for improvement. Ensemble models provide scope to combine a larger number of length-based models with differing structures and assumptions (Stewart and Martell, 2015) to explore whether these combinations can further improve the estimation performance of the ensemble.
Performance of ensemble models could be improved by optimizing the estimation of their individual component models. For LIME, this means collecting more years of length data; taking more independent length measurements during each year of length data collection; and conducting more surveys to acquire monitoring index data from which to derive estimates of variability in fishing mortality and recruitment. Sampling costs can be weighed against model performance to ascertain an appropriate number of fishery independent length measurements.
The impact of mis-specifying parameters should also be considered during future application of these models. A next step for ensemble models would be to apply Bayesian priors on biological parameters to more thoroughly represent the uncertainties in population parameter estimates relevant to management (e.g., from FishLife; Thorson et al., 2017). Sensitivity tests and likelihood profiles should be conducted on different levels of shaped selectivity to understand how SPR may become biased if the model structure is mis-specified.
Data Availability Statement
The datasets that support the findings of this study are available from the corresponding author upon reasonable request.
Ethics Statement
The animal study was reviewed and approved by Animal Ethics Committee, Yellow Sea Fisheries Research Institute.
Author Contributions
QH made substantial contributions to conceptualization, methodology, software, validation, formal analysis, data curation, writing – original draft, and visualization. XS made substantial contribution to conceptualization, methodology, data curation, and writing – review and editing. XJ, TY, and CS made substantial contribution to conceptualization, data curation, and writing – review and editing. HG made substantial contribution to conceptualization, writing – review and editing. All authors contributed to the article and approved the submitted version.
Funding
This work was supported in part by National Key R&D Program of China (2017YFE0104400), The David and Lucile Packard Foundation, The Innovation Team of Fishery Resources and Ecology in the Yellow Sea and Bohai Sea (2020TD01), and The Special Funds for Taishan Scholars Project of Shandong Province and Central Public-interest Scientific Institution Basal Research Fund, YSFRI, CAFS (NO. 20603022020017).
Conflict of Interest
The authors declare that the research was conducted in the absence of any commercial or financial relationships that could be construed as a potential conflict of interest.
Publisher’s Note
All claims expressed in this article are solely those of the authors and do not necessarily represent those of their affiliated organizations, or those of the publisher, the editors and the reviewers. Any product that may be evaluated in this article, or claim that may be made by its manufacturer, is not guaranteed or endorsed by the publisher.
Acknowledgments
The authors greatly appreciated support from the members of the Fishery Resources and Ecosystem Unit, Yellow Sea Fisheries Research Institute, Chinese Academy of Fishery Sciences.
Footnotes
References
Ali, K. M., and Pazzani, M. J. (1996). Error reduction through learning multiple descriptions. Mach. Learn. 24, 173–202. doi: 10.1007/bf00058611
Al-Mudhafar, W. J., Jaber, A. K., and Al-Mudhafar, A. (2016). “Integrating probabilistic neural networks and generalized boosted regression modeling for lithofacies classification and formation permeability estimation,” in Proceedings of the Offshore Technology Conference, Houston, TX. doi: 10.4043/27067-MS
Anderson, S. C., Cooper, A. B., Jensen, O. P., Minto, C., Thorson, J. T., Walsh, J. C., et al. (2017). Improving estimates of population status and trend with superensemble models. Fish Fish. 18, 1–10. doi: 10.1111/faf.12200
Bennett, K. P., and Campbell, C. (2000). Support vector machines: hype or hallelujah? SIGKDD Explor. Newslett. 2, 1–13. doi: 10.1145/380995.380999
Beverton, R. J. H., and Holt, S. J. (1957). On the Dynamics of Exploited Fish Populations. Fishery Investigations (Great Britain, Ministry of Agriculture, Fisheries, and Food). London: Her Majesty’s Stationery Office.
Brodziak, J., and Piner, K. R. (2010). Model averaging and probable status of North Pacific striped marlin, Tetrapturus audax. Can. J. Fish. Aquat. Sci. 67, 793–805. doi: 10.1139/f10-029
Burnham, K. P., and Anderson, D. R. (2002). Model Selection and Multimodel Inference: A Practical Information Theoretic Approach, 2nd Edn. New York, NY: Springer-Verlag.
Chang, C. C., and Lin, C. J. (2011). Libsvm: a library for support vector machines. ACM Trans. Intell. Syst. Technol. 2:27. doi: 10.1145/1961189.1961199
Chen, D. G. (1991). Fishery Ecology in the Yellow Sea and Bohai Sea. Beijing: Ocean Press. (in Chinese).
Chen, R. J., Li, X. S., Fan, G. Z., Zhao, X. Y., and Zhang, G. S. (2018). Minimal cod-end mesh of a pair trawl in the Yellow Sea. J. Dalian Ocean Univ. 33, 258–264. (in Chinese).
Chong, L. S., Mildenberger, T. K., Rudd, M. B., Taylor, M. H., Cope, J. M., Branch, T. A., et al. (2019). Performance evaluation of data-limited, length-based stock assessment methods. ICES J. Mar. Sci. 77, 97–108. doi: 10.1093/icesjms/fsz212
Clark, W. G. (2002). F35% revisited ten years later. N. Am. J. Fish. Manag. 22, 251–257. doi: 10.1577/1548-8675%282002%29022%3C0251%3AFRTYL%3E2.0.CO%3B2
Cope, J. M. (2017). Natural Mortality Tool. GitHub Repository. Available online at: https://github.com/shcaba/Natural-Mortality-Tool (accessed November 20, 2019).
Cortes, C., and Vapnik, V. (1995). Support-vector network. Mach. Learn. 20, 1–25. doi: 10.21275/v5i3.nov161719
Dick, E. J., and MacCall, A. D. (2011). Depletion-based stock reduction analysis: a catch-based method for determining sustainable yields for data-poor fish stocks. Fish. Res. 110, 331–341. doi: 10.1016/j.fishres.2011.05.007
Dietterich, T. G. (2000). “Ensemble methods in machine learning,” in Multiple Classifier Systems. MCS 2000. Lecture Notes in Computer Science (Berlin, Heidelberg: Springer), 1857. doi: 10.1007/3-540-45014-9_1
Free, C. M., Jensen, O. P., Wiedenmann, J., and Deroba, J. J. (2017). The refined orcs approach: a catch-based method for estimating stock status and catch limits for data-poor fish stocks. Fish. Res. 193, 60–70. doi: 10.1016/j.fishres.2017.03.017
Friedman, J. H. T., and Tibshirani, R. (2000). Additive logistic regression: a statistical view of boosting. Ann. Stat. 28, 337–407.
Goodyear, C. P. (1993). “Spawning stock biomass per recruit in fisheries management: foundation and current use,” in Risk Evaluation and Biological Reference Points for Fisheries Management. No. 120, eds S. J. Smith, J. J. Hunt, and D. Rivard (Ottawa, ON: Canadian Special Publication of Fisheries and Aquatic Sciences).
Halim, A., Loneragan, N. R., Wiryawan, B., Hordyk, A. R., and Yulianto, I. (2020). Evaluating data-limited fisheries for grouper (serranidae) and snapper (lutjanidae) in the coral triangle, eastern Indonesia. Reg. Stud. Mar. Sci. 38:101388. doi: 10.1016/j.rsma.2020.101388
Hamill, T. M., Brennan, M. J., Brown, B., Hamill, T. M., Brennan, M. J., Brown, B., et al. (2012). NOAA’s future ensemble-based hurricane forecast products. Bull. Am. Meteorol. Soc. 93, 209–220. doi: 10.1175/2011bams3106.1
Hastie, T., Tibshirani, R., and Friedman, J. (2009). The Elements of Statistical Learning: Data Mining, Inference, and Prediction, 2nd Edn. New York, NY: Springer.
Hilborn, R., and Walters, C. J. (1992). Quantitative Fisheries Stock Assessment: Choice, Dynamics, and Uncertainty. Berlin: Springer.
Hordyk, A., Ono, K., Valencia, S., Loneragan, N., and Prince, J. (2015). A novel length-based empirical estimation method of spawning potential ratio (SPR) and tests of its performance, for small-scale, data-poor fisheries. ICES J. Mar. Sci. 72, 217–231.
Hordyk, A. R. (2017). LBSPR: Length-Based Spawning Potential Ratio. R Package Version 0.1.5. Available online at: https://github.com/AdrianHordyk/LBSPR (accessed November 25, 2019).
ICCAT (2017). Report of the 2017 SMALL Tunas Species Group Intersessional Meeting, Miami, United States, 24–28 April 2017. Collective Volume of Scientific Papers of ICCAT, Vol. 74. Madrid: ICCAT, 1–75.
Jin, X. S., Cheng, J. S., Qiu, S. Y., Li, P. J., Cui, Y., Dong, J., et al. (2006). The General Study and Evaluation of Fisheries Resources in Yellow Sea and Bohai Sea. Beijing: Ocean Press. (in Chinese).
Jin, X. S., Dou, S. Z., Shan, X. J., Wang, Z. Y., Wan, Y. J., and Bian, X. D. (2015). Hot spots of frontiers in the research of sustainable yield of Chinese inshore fishery. Prog. Fish. Sci. 36, 124–131. (in Chinese),
Jin, X. S., Zhao, X. Y., Meng, T., Bohuan, X., and Cui, Y. (2005a). Biological Resource and Habitation Environment of the Bohai and Yellow Sea. Beijing: Science Press. (in Chinese).
Jin, X. S., Qiu, S. Y., Liu, X. Z., Wang, J., Zhang, B., and Shan, X. J. (2005b). Basis and Prospect of Fishery Resources Enhancement in the Bohai and Yellow Sea. Beijing: Science Press. (in Chinese).
Jones, R. (1981). The Use of Length Composition Data in Fish Stock Assessmentst (with notes on VPA and cohort analysis). FAO (Ed.), Fisheries Technical Paper. (256). Rome: FAO, 118.
Knutti, R., Furrer, R., Tebaldi, C., Cermak, J., and Meehl, G. A. (2009). Challenges in combining projections from multiple climate models. J. Clim. 23, 2739–2758.
Krishnamurti, T. N., Kishtawal, C. M., and LaRow, T. E. (1999). Improved weather and seasonal climate forecasts from multimodel superensemble. Science 285, 1548–1550. doi: 10.1126/science.285.5433.1548
Kristensen, K., Nielsen, A., Berg, C. W., Skaug, H., and Bell, B. M. (2016). TMB: automatic differentiation and Laplace approximation. J. Stat. Softw. 70, 1–21. doi: 10.18637/jss.v070.i05
Liu, J., Li, C., and Ning, P. (2013). A redescription of grey pomfret Pampus cinereus (Bloch, 1795) with the designation of a neotype (Teleostei: Stromateidae). Chin. J. Oceanol. Limnol. 31, 140–145. doi: 10.1007/s00343-013-2039-9
Liu, X. S., Wu, J. N., and Han, G. Z. (1990). Fishery Resources Investigation and Regionalization District in the Bohai Sea and the Yellow Sea. Beijing: China Ocean Press. (in Chinese).
MacCall, A. D. (2009). Depletion-corrected average catch: a simple formula for estimating sustainable yields in data-poor situations. ICES J. Mar. Sci. 66, 2267–2271. doi: 10.1093/icesjms/fsp209
Meyer, D., Dimitriadou, E., Hornik, K., Weingessel, A., Leisch, F., Chang, C. C., et al. (2021). e1071: Misc Functions of the Department of Statistics, Probability Theory Group (Formerly: E1071). Available online at: https://CRAN.R-project.org/package=e1071 (accessed November 28, 2019).
Millar, C. P., Jardim, E., Scott, F., Osio, G. C., Mosqueira, I., and Alzorriz, N. (2015). Model averaging to streamline the stock assessment process. ICES J. Mar. Sci. 72, 93–98. doi: 10.2196/26402
Nadon, M. O., Ault, J. S., Williams, I. D., Smith, S. G., Dinardo, G. T., and Ferse, S. (2015). Length-based assessment of coral reef fish populations in the main and northwestern Hawaiian Islands. PLoS One 10:e0133960. doi: 10.1371/journal.pone.0133960
Pons, M., Cope, J. M., and Kell, L. T. (2020). Comparing performance of catch-based and length-based stock assessment methods in data-limited fisheries. Can. J. Fish. Aquat. Sci. 77, 1026–1037. doi: 10.1139/cjfas-2019-0276
Pons, M., Kell, L., Rudd, M. B., Cope, J. M., and Flávia, L. F. (2019). Performance of length-based data-limited methods in a multifleet context: application to small tunas, mackerels, and bonitos in the Atlantic Ocean. ICES J. Mar. Sci. 4, 960–973. doi: 10.1093/icesjms/fsz004
Prince, J., Victor, S., Kloulchad, V., and Hordyk, A. (2015). Length based SPR assessment of eleven Indo-Pacific coral reef fish populations in Palau. Fish. Res. 171, 42–58. doi: 10.1016/j.fishres.2015.06.008
R Development Core Team (2019). R: A Language and Environment for Statistical Computing. Vienna: R Foundation for Statistical Computing.
RAM Legacy Stock Assessment Database (2018). Version 4.44-Assessment-Only. Released 2018-12-22. doi: 10.5281/zenodo.2542919
Ricard, D., Minto, C., Jensen, O. P., and Baum, J. K. (2012). Evaluating the knowledge base and status of commercially exploited marine species with the RAM Legacy Stock Assessment Database. Fish Fish. 13, 380–398. doi: 10.1111/j.1467-2979.2011.00435.x
Ridgeway, G. (2007). Generalized Boosted Models: A Guide to the gbm Package. Available online at: http://www.saedsayad.com/docs/gbm2.pdf (accessed November 28, 2019).
Rudd, M. B., and Thorson, J. T. (2018). Accounting for variable recruitment and fishing mortality in length-based stock assessments for data-limited fisheries. Can. J. Fish. Aquat. Sci. 75, 1019–1035. doi: 10.1139/cjfas-2017-0143
Schnute, J. T., and Hilborn, R. (1993). Analysis of contradictory data sources in fish stock assessment. Can. J. Fish. Aquat. Sci. 50, 1916–1923. doi: 10.1139/f93-214
Seo, K. K. (2007). An application of one-class support vector machines in content-based image retrieval. Expert Syst. Appl. 33, 491–498.
Stewart, I. J., and Martell, S. J. D. (2015). Reconciling stock assessment paradigms to better inform fisheries management. ICES J. Mar. Sci. 72, 2187–2196. doi: 10.1093/icesjms/fsv061
Tang, Q. S. (2006). Marine Living Resources and Habitat Environment in China’s Exclusive Economic Zone. Beijing: Science Press.
Tang, Q. S., and Ye, M. Z. (1990). Exploitation and Conservation of Fishery Resources in the Coastal Water of Shandong. Beijing: China Agriculture Press. (in Chinese).
Tebaldi, C., and Knutti, R. (2007). The use of the multi-model ensemble in probabilistic climate projections. Philos. Trans. R. Soc. Lond. A Math. Phys. Eng. Sci. 365, 2053–2075.
Then, A. Y., Hoenig, J. M., Gedamke, T., and Ault, J. S. (2015). Comparison of two length-based estimators of total mortality: a simulation approach. Trans. Am. Fish. Soc. 144, 1206–1219. doi: 10.1080/00028487.2015.1077158
Thorson, J. T., and Cope, J. M. (2014). Catch curve stock-reduction analysis: an alternative solution to the catch equations. Fish. Res. 171, 33–41.
Thorson, J. T., Jensen, O. P., and Zipkin, E. F. (2014). How variable is recruitment for exploited marine fishes? A hierarchical model for testing life history theory. Can. J. Fish. Aquat. Sci. 71, 973–983. doi: 10.1139/cjfas-2013-0645
Thorson, J. T., Munch, S. B., Cope, J. M., and Gao, J. (2017). Predicting life history parameters for all fishes worldwide. Ecol. Appl. 27, 2262–2276. doi: 10.1002/eap.1606
Weigel, A. P., Knutti, R., Liniger, M. A., and Appenzeller, C. (2010). Risks of model weighting in multimodel climate projections. J. Clim. 23, 4175–4191. doi: 10.1016/j.scitotenv.2019.135357
Xu, Q. C., Li, X. S., Sun, S., Fan, G. Z., Jiang, Z. W., and You, Z. B. (2019). On catch composition and selectivity of pair-trawling in the Yellow Sea. Mar. Fish. 41, 676–683.
Keywords: ensemble models, length-based, multimodel averaging, population dynamics, data-limited fisheries
Citation: Han Q, Shan X, Jin X, Gorfine H, Yang T and Su C (2021) Data-Limited Stock Assessment for Fish Species Devoid of Catch Statistics: Case Studies for Pampus argenteus and Setipinna taty in the Bohai and Yellow Seas. Front. Mar. Sci. 8:766499. doi: 10.3389/fmars.2021.766499
Received: 29 August 2021; Accepted: 03 November 2021;
Published: 24 November 2021.
Edited by:
Oscar Sosa-Nishizaki, Center for Scientific Research and Higher Education in Ensenada (CICESE), MexicoReviewed by:
Nor Azman Kasan, University of Malaysia Terengganu, MalaysiaJason Marc Cope, National Marine Fisheries Service (NOAA), Northwest Region, United States
Copyright © 2021 Han, Shan, Jin, Gorfine, Yang and Su. This is an open-access article distributed under the terms of the Creative Commons Attribution License (CC BY). The use, distribution or reproduction in other forums is permitted, provided the original author(s) and the copyright owner(s) are credited and that the original publication in this journal is cited, in accordance with accepted academic practice. No use, distribution or reproduction is permitted which does not comply with these terms.
*Correspondence: Xiujuan Shan, shanxj@ysfri.ac.cn