- 1Department of Integrative Biology, University of South Florida, Tampa, FL, United States
- 2Plant Evolutionary Ecology Group, University of Tübingen, Tübingen, Germany
- 3Department of Biology, The University of New Mexico, Albuquerque, NM, United States
Many coastal foundation plant species thrive across a range of environmental conditions, often displaying dramatic phenotypic variation in response to environmental variation. We characterized the response of propagules from six populations of the foundation species Rhizophora mangle L. to full factorial combinations of two levels of salinity (15 ppt and 45 ppt) reflecting the range of salinity measured in the field populations, and two levels of nitrogen (N; no addition and amended at approximately 3 mg N per pot each week) equivalent to comparing ambient N to a rate of addition of 75 kg per hectare per year. The response to increasing salinity included significant changes, i.e., phenotypic plasticity, in succulence and root to shoot biomass allocation. Propagules also showed plasticity in maximum photosynthetic rate and root to shoot allocation in response to N amendment, but the responses depended on the level of salinity and varied by population of origin. In addition, propagules from different populations and maternal families within populations differed in survival and all traits measured except photosynthesis. Variation in phenotypes, phenotypic plasticity and propagule survival within and among R. mangle populations may contribute to adaptation to a complex mosaic of environmental conditions and response to climate change.
Introduction
Many plant species thrive across an extensive range of environmental conditions, often displaying dramatic phenotypic variation (McKee, 1995; Smith and Snedaker, 1995; Richards et al., 2005; Feller et al., 2010). This is particularly true in coastal ecosystems that are characterized by temporal cycles and spatial variation in tidal inundation, temperature, nutrient availability, and salinity (Pennings and Bertness, 2001; Krauss et al., 2008; IPCC, 2014; Proffitt and Travis, 2014; Wuebbles et al., 2014). In addition to the naturally dynamic nature of coastal habitats, anthropogenic activities can increase the input of nutrients and alter watersheds, further contributing to environmental variation (Bertness et al., 2002; Barbier et al., 2008; Gedan et al., 2009, 2011; Crotty et al., 2020). Within these dynamic conditions, foundation plant species such as mangroves provide ecosystem services. These services include providing habitat for many juvenile fish species, biotic filtering of pollutants, and buffering of storms (Ellison et al., 2005; Zedler and Kercher, 2005; IUCN, 2007; Alongi, 2008, 2013; Costanza et al., 2008; Gedan et al., 2011; Bertness, 2020). Foundation species are defined not only as those that dominate a community assemblage numerically or in biomass, but they also determine diversity of associated taxa through a variety of interactions (Ellison, 2019). Further, foundation species modulate fluxes of nutrients and energy in their ecosystem (Ellison, 2019). Hence, these species disproportionately contribute to maintaining habitat integrity and ecosystem resilience (Bertness and Callaway, 1994; Keith et al., 2017; Ellison, 2019; Bertness, 2020; Qiao et al., 2021). Understanding how these species cope with challenges from anthropogenic impacts is key to preserving the ecosystems they create and define (Gedan et al., 2009, 2011; Guo et al., 2021).
Understanding the mechanisms of response in coastal foundation species has become increasingly important for conservation and management strategies as these species must cope with rising sea levels, increased warming, and anthropogenic disturbances (Gedan et al., 2011; Kirwan and Megonigal, 2013; Osland et al., 2013, 2017). The Food and Agriculture Organization of the United Nations (FAO) estimates that as much as 35% of global mangrove forest habitat has been destroyed since the early 1980’s for the development of human settlements, agriculture and aquaculture, and industrial shipping harbors, although the rate of loss appears to have slowed in the last decade (Food and Agriculture Organization of the United Nations, 2007; Polidoro et al., 2010; Ellison et al., 2015; FAO, 2020). In some regions, mangrove trees are also harvested for wood and charcoal (Ellison et al., 2015), resulting in habitat fragmentation and isolation of existing remnant fragments (Friess et al., 2012; Haddad et al., 2015). The resultant loss of diversity could pose risks for these coastal foundation species in the future, particularly as sea levels are projected to rise between 0.2 and 2 m over the next century due to anthropogenic climate change (Melillo et al., 2014).
The vulnerability of coastal foundation plant communities to global change has been debated. Several authors have suggested that the combination of eutrophication and sea-level rise may have a synergistic effect that results in enhanced losses of coastal habitats, and requires further research (Deegan et al., 2012; Kirwan and Megonigal, 2013; Kirwan et al., 2016; Crosby et al., 2017; Schuerch et al., 2018). While coastal eutrophication may enhance growth of foundation species, nutrient enrichment studies have reported a range of impacts on coastal systems depending on the local conditions (Anisfeld and Hill, 2012; Kirwan and Megonigal, 2013). For example, nutrient cycling and marsh stability were affected by local sediment characteristics, soil nutrients, microbial processes, and shifts in allocation of the plant species (McKee et al., 2007; Turner, 2011; Deegan et al., 2012; Lewis et al., 2021).
Predicting species level responses to environmental challenges requires an understanding of the amount of phenotypic variation within and among populations, which may reflect both phenotypic plasticity and heritable differences in phenotype (Richards et al., 2006; Nicotra et al., 2010; Banta and Richards, 2018). Several studies have shown that plant species harbor heritable differences in eco-physiological traits (Arntz and Delph, 2001; Geber and Griffen, 2003; Caruso et al., 2005), and variation in plasticity of traits (Sultan, 2001; Matesanz and Sultan, 2013; Nicotra et al., 2015; Matesanz et al., 2021). However, the amount of variation in natural populations for traits that are important for response to future climates is not well known (Davis and Shaw, 2001; Parmesan, 2006; Lovelock et al., 2016). Plants that inhabit coastal and intertidal zones have putative physiological adaptations that enable them to grow and reproduce in the anoxic and saline conditions that characterize these habitats. These adaptations include adjustment of water required by the plant (Antlfinger and Dunn, 1979, 1983; Glenn and O’Leary, 1984; Donovan et al., 1996; Ball et al., 1997), adjustment of carbon uptake and nutrient absorption (Donovan et al., 1997; Lovelock et al., 2006, 2016; Flowers and Colmer, 2008), and changes in resource allocation (Cavalieri and Huang, 1979; Glenn and O’Leary, 1984; Donovan et al., 1996, 1997; Flowers and Colmer, 2008; Lovelock et al., 2016). In addition, mangrove species can moderate anoxia that results from flooding via root growth, and altered peat formation has allowed mangrove communities to keep pace with sea level rise (McKee et al., 2007). Mangroves have been shown to respond to changes in nitrogen (N) availability by altering relative growth rate, photosynthetic rate, and resource allocation (Feller, 1995; McKee, 1995; Feller et al., 2003), which could be an important response to anthropogenic activities, such as runoff from agriculture and other types of land use change (Feller et al., 2003; Alongi, 2013).
Given spatial differences in salinity, anoxia, and N in the intertidal habitat, plasticity of traits that allow for tolerating such conditions may be adaptive. We expect intertidal plants like mangroves to show plasticity in response to salinity and N conditions (Antlfinger, 1981; Pennings and Richards, 1998; Richards et al., 2010b; Vovides et al., 2014; Lovelock et al., 2016). Proffitt and Travis (2010) found plasticity in growth rate and reproductive output within and among natural Rhizophora mangle mangrove populations in the Tampa Bay region of Florida in the United States. However, they also found both site of origin and maternal tree of origin affected R. mangle growth and survival, and that these effects varied by intertidal position (significant maternal family by elevation interaction; Proffitt and Travis, 2010). On the other hand, in a study of other Tampa Bay populations, we found low genetic diversity, and little population differentiation (Mounger et al., 2021). Although genetic diversity was low, we discovered high epigenetic diversity based on DNA methylation polymorphisms (Mounger et al., 2021). This type of epigenetic diversity has been associated with phenotypic and functional diversity, and could be a mechanism underlying phenotypic plasticity (Zhang et al., 2013; Nicotra et al., 2015; Herrera et al., 2017; Jueterbock et al., 2020). Several studies have suggested that epigenetic diversity could be particularly important in genetically depauperate species, providing a non-genetic source of response to the diverse conditions experienced by natural populations (Gao et al., 2010; Verhoeven et al., 2010; Richards et al., 2012; Jueterbock et al., 2020). Further, some epigenetic differences have been shown to be heritable. In fact, we discovered that the differences in DNA methylation in R. mangle propagules were predicted by maternal tree indicating a high degree of inheritance of differences in DNA methylation (Mounger et al., 2021).
In this study, we used plants from the same Tampa Bay populations to characterize within and among population level variation in putative adaptive traits in response to combinations of salinity and N in a full factorial design. Given the dynamic environment inhabited by R. mangle and the evidence of heritable non-genetic differences among populations, we hypothesized that propagules collected from different populations would respond differently to salinity and N amendment treatments. Our study was designed to test three predictions. First, R. mangle seedlings will be plastic in response to salinity and N amendment in putative adaptive traits that conserve water and adjust allocation of N. Second, response to salinity and N amendment will co-vary as plants shift resources to maintain osmotic balance. Finally, populations will vary in putative adaptive traits, and in plasticity of these traits, due to population differentiation.
Materials and Methods
Study Species
The red mangrove, Rhizophora mangle L. 1753 (Malpighiales, Rhizophoraceae), is an evergreen shrub or tree found along tropical and subtropical coastlines across the Americas, East Africa, Bermuda, and on a number of outlying islands across the South Pacific (Proffitt and Travis, 2014; Tomlinson, 2016; DeYoe et al., 2020) that can grow to heights of 24 m (Bowman, 1917). Poleward expansion of the species is limited by freezing events (its current northern range limit is roughly 29°N latitude; Proffitt and Travis, 2014; Kennedy et al., 2017). Rhizophora mangle is considered a self-compatible species, with selfing rates in Tampa Bay estimated to be as high as 80–100% (Proffitt and Travis, 2005; Nadia and Machado, 2014). However, colder temperatures and contaminants from anthropogenic sources correspond with increased flowering and outcrossing, potentially resulting in higher genetic diversity particularly in the smaller populations at range limits from 28 to 30°N (Proffitt and Travis, 2005, 2014). Rhizophora mangle stands in our study area have a mean number of about 600 reproducing trees per kilometer of estuary (Proffitt and Travis, 2014). Pollinated R. mangle flowers mature in approximately 95 days, producing the buoyant hypocotyl also known as a propagule (Raju Aluri, 2013). The viviparous propagule germinates and matures on the maternal tree before it drops off, is dispersed pelagically, and becomes established as a seedling (McKee, 1995). Rhizophora mangle excludes salt in the root system through selective uptake of potassium (K+) to sodium (Na+) ions (Wise and Juncosa, 1989; Flowers and Colmer, 2008; Krauss et al., 2008; Medina et al., 2015), and allocates resources to manage osmotic potential (Bowman, 1917; Flowers and Colmer, 2008).
Sampling Design
We collected propagules from six populations of R. mangle between June 9 and June 26 of 2015, on the west coast of central Florida (USA) within the following county and state parks: Anclote Key Preserve State Park (AC), Fort De Soto Park (FD), Honeymoon Island State Park (HI), Upper Tampa Bay Conservation Park (UTB), Weedon Island Preserve (WI), and Werner-Boyce Salt Springs State Park (WB) (Figure 1). The sites varied in salinity, mean tidal range, and neighboring species. We measured salinity at each site with a refractometer finding that salinity ranged from 20 to 40 parts per thousand (ppt) across the sites at the time of collection. This area has a humid subtropical climate with mean monthly temperatures ranging from 15.6°C in January to 28.5°C in August (1991–2020 monthly normals, U.S. NOAA National Centers for Environmental Information, station GHCND:USC00088824, Tarpon Springs, FL, USA), and annual precipitation of 1379 mm (annual mean 1991–2020, Tarpon Springs station). Precipitation falls as rain, with 60% falling during June through September, and 40% evenly distributed among other months. Monthly mean relative humidity ranges from 67% in April and May to 76% in August and September (1948–2018, U.S. NOAA Comparative Climate Data for the USA through 2018, station GHCND:USW00012842, Tampa International Airport). Tides are semi-diurnal, with 0.57 m median amplitudes (U.S. NOAA National Ocean Service, Clearwater Beach, Florida, station 8726724). Sea-level rise is 4.0 ± 0.6 mm per year (1973–2020 trend, mean ± 95% confidence interval, NOAA NOS Clearwater Beach station).
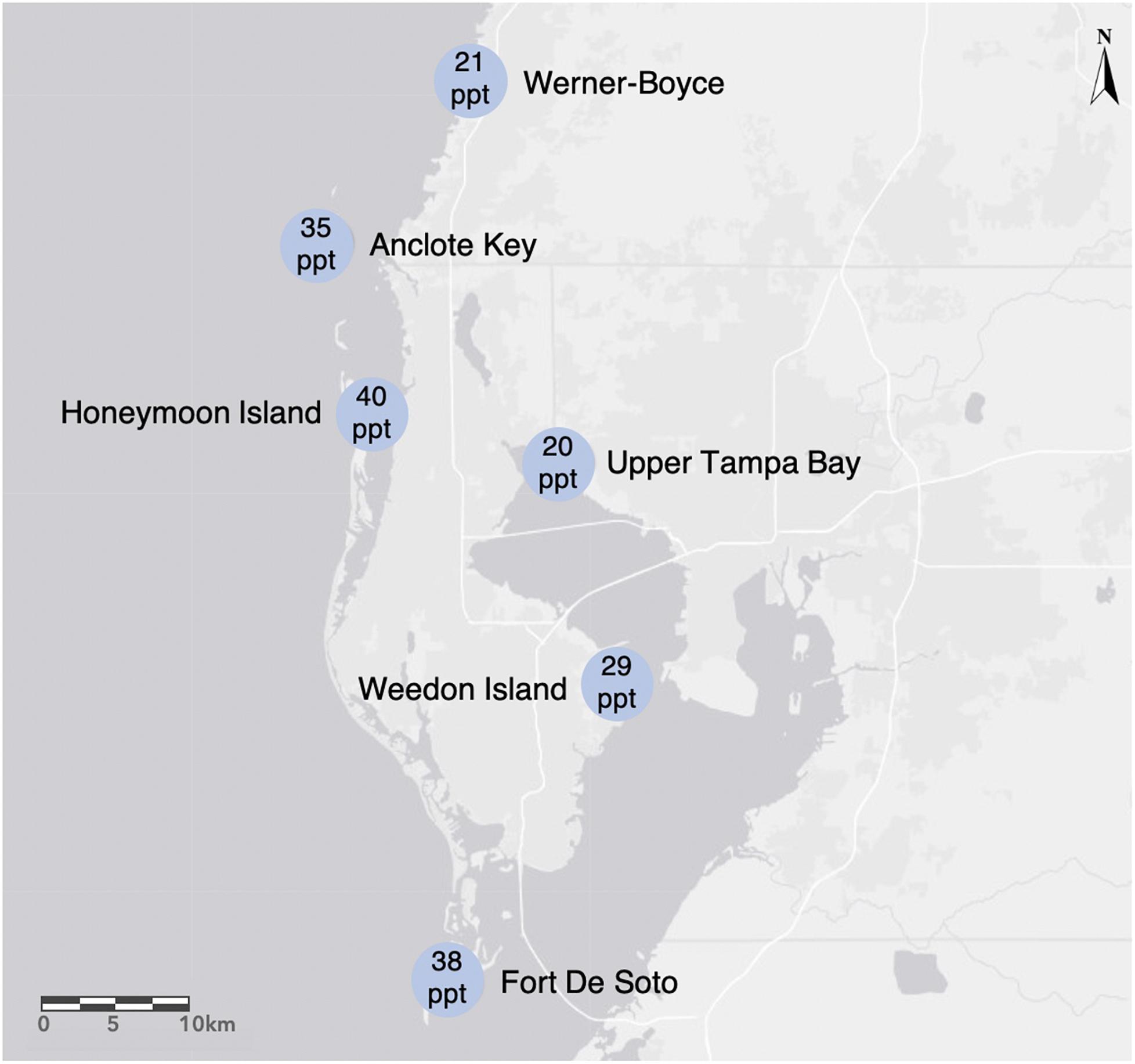
Figure 1. We collected Rhizophora mangle propagules from the following sites in the Tampa Bay area on the west coast of Florida, USA: Werner-Boyce Salt Springs State Park, Anclote Key Preserves State Park, Honeymoon Island State Park, Upper Tampa Bay Hillsborough County Park, and Weedon Island Preserve Pinellas County Park. Salinity levels (ppt) on the date of collection for each site are indicated within the site location markers.
Honeymoon Island had a near monoculture of R. mangle while the remaining sites contained mixtures of two other mangrove species that are common in Florida: Laguncularia racemosa L. and Avicennia germinans L. We refer to plants from different sites as members of different populations based on our previous work which found differences among sites based on molecular markers (Mounger et al., 2021). At each population, we collected 20 propagules directly from each of 10 maternal trees separated by at least 10 m from each other to maximize the range of genetic variation sampled within each population (Albrecht et al., 2013). Propagules from each maternal tree were at least half-siblings but they could be more closely related due to the high selfing rate in the study area (Proffitt and Travis, 2005).
We refrigerated the propagules at 4°C for up to 14 days until we planted them in the greenhouse at the University of South Florida Botanical Gardens. The greenhouse temperature was maintained between 18 and 29°C. In the greenhouse, propagules from four of the maternal trees at AC and nine of the maternal trees at FD failed to establish, so we returned to sample propagules from eight new maternal trees at FD on August 12 and 29, and from the same original maternal trees at AC on October 17. Hence, while most of the propagules were in the greenhouse from the end of June until mid-October before they were exposed to treatments, some propagules from these FD and AC maternal families had less acclimation time before treatments began.
Experimental Treatments
We measured the length of each propagule and planted each in a 0.5-L pot with a 50:50 mixture of sand and peat soil. We watered the plants each day with tap water until we started applying the salinity and N amendment in mid-October. We set up the experiment in five spatial blocks. Within each block we randomized the position of plants such that each block had one replicate of each family for each treatment combination [i.e., a full factorial randomized complete block design with N = 6 populations × 10 maternal families × 4 environmental treatment combinations (2 salinity × 2 N fertilization) × 5 blocks × 1 replicate/block = 1200 plants]. The treatments were a factorial combination of low and high salinity with either no addition or addition of N. The salinity treatments were made with Morton solar salt (NaCl). The low salinity treatment was 15 ppt and the high salinity was 45 ppt, reflecting a slightly wider range of salinity than we measured in the field sites considering that we only measured salinity in the field at one time point and we expect the range to be slightly greater. The N treatments were made from equal amounts (in moles N) of urea and ammonium nitrate in tap water approximating 3 mg N per pot each week. This level is similar to a rate of 75 kg N per hectare per year, based on an estimated rate of loss by soil erosion and water runoff from corn crop residue in the USA (Pimentel et al., 1989). The source water for our irrigation solution meets drinking water standards, and thus has low mineral N and phosphorous (P) concentrations, with mineral N (nitrate-N plus ammonium-N) less than 1 mg N/L and P (phosphate) less than 0.1 mg P/L (Penuela Useche, 2015). Furthermore, the aquifer that provides our water had a median sulfate concentration of 8.2 mg/L per liter (interquartile range 2.3–17.8) (Berndt et al., 2015). This sulfur (S) would not be targeted for removal via water treatment, as the Environmental Protection Agency recommended S level is <250 mg S (as SO4)/L. This level of S would avoid plant S limitation since plants need about 1 mol S per 15–20 mol N.
At the start of treatments, we recorded seedling initial height from the soil to end of any growth. To avoid osmotic shock, the salinity treatment was applied twice a week and gradually increased by five ppt each treatment. The low salinity level (15 ppt) was reached in 2 weeks and the high salinity level (45 ppt) in 6 weeks. We started N treatments after the first week (when salinity treatments were 10 ppt), and applied N once per week from October 15, 2015 to May 1, 2016. We watered on non-treatment days with enough water to saturate the soil, but not flow through. Once per week, we watered with sufficient water to flow through the soil to prevent salt buildup. To determine if the N amendment was lost between treatments, we collected the flow-through leachate for a subset of eight plants, two of each combination of salinity and N treatment. We analyzed leachate for total dissolved N via combustion and luminescent detection (Skalar Formacs TN analyzer, Breda, Netherlands).
Traits Measured
We characterized each plant as alive, dormant, or dead at the end of the experimental treatments. We assigned plants that showed no growth and no desiccation to the dormant category. We measured five traits related to salt tolerance and overall performance for alive plants: change in height from beginning to end of treatments (cm) (hereafter, height growth), leaf mass per area or LMA (dry leaf mass in g/total leaf area cm2), succulence (grams of water in all leaves/total leaf area cm2), root to shoot ratio based on dry biomass, and total dry biomass (g) at harvest. We only used healthy leaves for succulence and LMA. We defined healthy leaves as attached, a minimum of 50% green, and fully developed. For dry above ground biomass, we included leaves that were attached, but not 50% green or fully developed. We measured the biomass of above and below ground tissues of all harvested plants after the tissues were dried at 60°C until they maintained constant mass. Finally, we measured the total dry mass of leaves after drying in silica desiccant beads for a minimum of 7 days to constant mass.
In addition, we used a LI-COR 6400 to measure maximum photosynthetic rate (micromoles CO2/m2 s) for a subset of the plants just prior to harvest. We determined that the appropriate photosynthetically active radiation (PAR) for saturation in these plants was 1000 micromoles PAR/m2 s based on light curves generated from six data points from each of two plants (one low salinity – no N and one high salinity – high N). We then measured maximum photosynthetic rate on one plant with at least two healthy leaves for each surviving maternal line for each treatment (n = 29 low salinity – no N; n = 31 high salinity – no N; n = 26 low salinity – high N; and n = 32 high salinity – high N, for total N = 118 total plants). We took maximum photosynthetic rate measurements at a CO2 rate of 400 micromoles/(m2 s) and a flow rate of 500 micromoles/s. We took the measurements on a healthy leaf from the second node of each plant after the leaf had been clamped in the LI-COR for 1 min to ensure conditions had stabilized. We measured maximum photosynthetic rate for the 118 plants in random order by block over six consecutive days from April 23 to 28, 2016, between 8:30 and 11:30 in the morning.
Statistical Analysis
We performed all statistical analyses in R, version 4.0.3 (R Core Team, 2020). All analyses reported here used either the General Linear Model (GLM) or Generalized Linear-Mixed-Model (GLMM) frameworks. GLMs are a generalization of the familiar analysis of variance framework, which allow one to use error distributions other than the restrictive Gaussian used in ANOVA. Moreover, the GLM link function allows one to fit a linear model to a dependent variable that has an essentially non-linear relationship with the predictors. For example, using a log link, one can model the log of the expected value of the data as a linear function; this is distinct from ANOVA on log-transformed data, which models the expected value of the log. GLMMs are a further generalization that allow one to model the variances, rather than expected values, of some predictors. These predictors are sometimes (incorrectly) assumed to be sampled randomly; conceptually the real distinction between these predictors and the others is whether one is interested in the mean values or variances. We fit all models as GLMMs because we were interested in the variance among the maternal families, rather than in their particular means (Bolker, 2015). However, we also fit GLMs that excluded the family terms, to determine whether maternal family contributed to improved model fits. We also treated Block as a “random” term where possible, but when such models failed to converge numerically (due to the small number of blocks) we fit the analogous model with Block as a fixed term (Bolker, 2015).
We checked the residuals to assess normality on traits as appropriate; we did not transform height growth or photosynthesis (lmer and glm), but we used the log link function (glmer) for analysis of succulence, LMA, root to shoot ratio, and total biomass. We used the function lmer or glmer implemented within the lme4 package (Bates et al., 2015) to fit a series of models and identify the best fit model for survival and for each trait (Table 1).
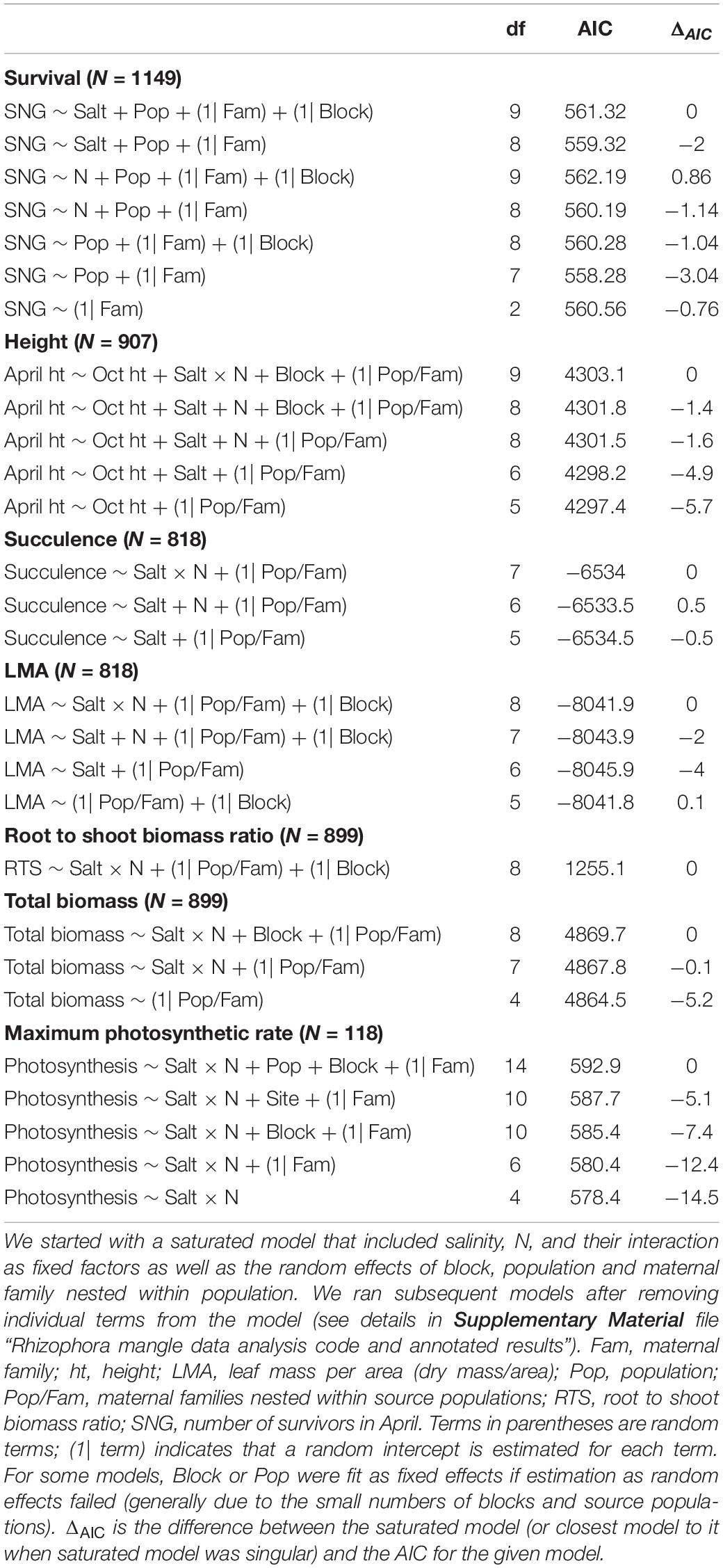
Table 1. Model selection based on a series of models using the General Linear Model (GLM) or Generalized Linear-Mixed-Model (GLMM) framework.
For survival and each trait, we began with a saturated model that included as fixed factors salinity, N, and their interaction as well as the random effects of block, population and maternal family nested within population. We ran subsequent models removing individual terms from the model (see details in the Supplementary Material file “Rhizophora mangle data analysis code and annotated results”). In several cases, models with random terms for block or population failed to converge (Table 1), most likely because there were relatively few blocks and populations. In these cases, we proceeded by treating these as fixed effects. We used AIC to evaluate the fit among all of the models that we ran for survival and for each trait. The model with the lowest AIC was considered to have the best fit. We used ANOVA (type III) in the package car (Fox and Weisberg, 2019) to evaluate the significance of fixed effects when they were included in the best model. When the random terms were found to be significant, we provided graphics of the relative differences among the maternal families, populations or blocks in the Supplementary Material file “Rhizophora mangle data analysis code and annotated results” to visualize the relative differences among these groups.
For survival, we assessed three states that were coded as 0 for live plants, 1 for dormant plants, and 2 for plants that died during the experiment. We modeled survival using random effects logistic regression. In one set of models, we included dormant plants as alive, and in another we excluded them. The results were qualitatively similar, so we report only the case where dormant plants were treated as alive (see results for both in Supplementary Material file “Rhizophora mangle data analysis code and annotated results”). For change in height, we included the covariate of height at the beginning of treatments in October.
To gain insight about variance explained by models, we calculated the R2 approximations proposed by Nakagawa and Schielzeth (2013). As these authors explained, in the context of GLMMs this leads to two different sorts of R2, a marginal R2 that reflects variance explained by fixed factors only, and a conditional R2 that reflects variance explained by both fixed and random factors. We reported each of these as appropriate, e.g., where the best model included only fixed factors we reported only the marginal R2.
Results
We found that some combination of salinity and N treatments were included in the best models for three of the six traits we measured: succulence, root to shoot biomass ratio, and maximum photosynthetic rate (Table 1). In addition, maternal families and populations were significantly different for survival and all traits measured with the exception of photosynthesis (Table 1). Below we report the results of model selection and assess significance of effects retained in the best model.
Treatment Validation
To ensure that our N amendment treatments were not flushed out during the once weekly flow through watering we measured the total dissolved N in leachate from a subsample of the seedlings. We found that N was not significantly different between the low salinity – no N and the high salinity – high N amended plants, and therefore, confirmed that we did not lose the N due to watering between treatments [Mean Square = 0.11, F ndf 3/ddf 48 = 0.23, Pr(>F) = 0.88].
Survival
Of 1,149 plants, 76 were unequivocally dead in April, 907 unequivocally alive, and 166 dormant. The number of plants that showed active growth ranged from 63% in HI to 92% in FD (Figure 2). The number of plants that did not grow, but also did not appear to be dead ranged from 3% in WB and WI to 10% in HI. We modeled survival by including these dormant plants alternatively as either alive or dead. In both cases, the best-supported model was one including a fixed effect for population and a random effect for maternal family (Table 1; Supplementary Figure 1). The model treating dormant plants as alive had conditional R2 = 0.11 and marginal R2 = 0.08. The model with dormant plants as dead had conditional R2 = 0.28 and marginal R2 = 0.13. Because there is no residual in the equation defining logistic regression, no variance component for a random residual is calculated, and thus it is not possible to calculate a meaningful scaled variance component for family here.
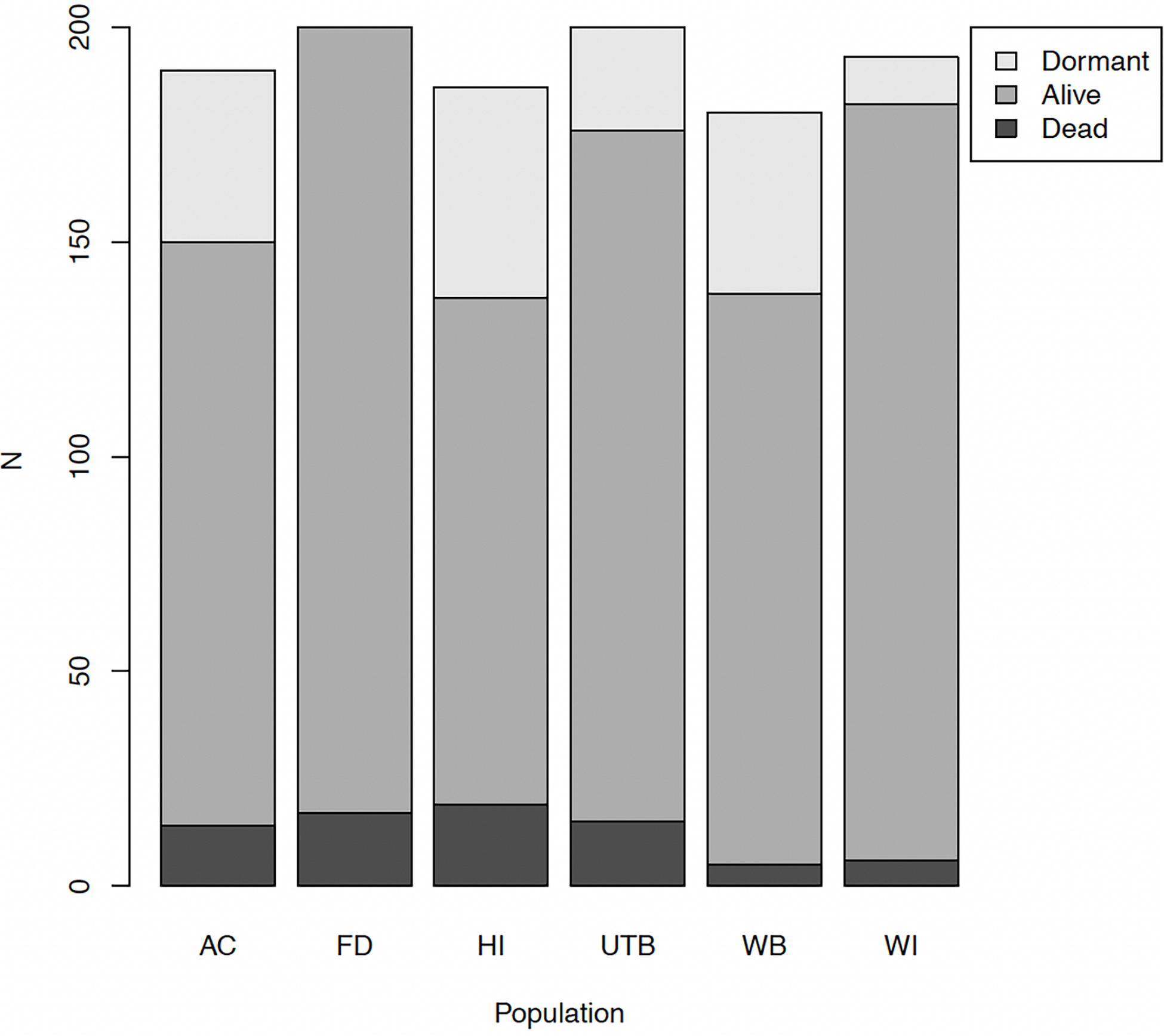
Figure 2. Absolute numbers of plants in each growth state at harvest by population of origin. Dormant plants (light gray) showed no signs of desiccation or growth. Seedlings from the HI population had the lowest survival overall, while WI seedlings had the highest survival. Plants alive at harvest are medium gray, plants that had died by harvest are dark gray.
Trait Responses to Treatment
The only useful predictor among the fixed effects for the change in height was the height at the start of treatments in October (Table 1; marginal R2 = 0.82; ANOVA chisq = 4432.67; p < 0.0001). The conditional R2 was 0.92, and the marginal R2 was 0.82, indicating that 82% of the variance in change in height was explained by a positive relationship with the fixed effect of height at the beginning of the experimental treatments (estimate = 0.9009). The variance components (scaled to sum to 1) for population and maternal family within population were 23 and 37% of the random variance, respectively. A plot of the random effects suggested that the populations were largely similar, with the exception of FD which was very different (Supplementary Figure 2). It is not obvious that any population had more among-family variability, but our design was limited to determine this.
For LMA (log link), the best model included only the random effects of block, population and maternal family within population. The conditional R2 was 0.99. In this model, maternal family explained 51% of the variance, population explained 30% of the variance and block explained 17% (Supplementary Figure 3).
For succulence (log link) the best model included the fixed effect of salinity and the random effects of population and maternal family nested within population. The marginal R2 = 0.39, while the conditional R2 = 0.99. The scaled variance components for maternal family within population and population were, respectively, 0.91 and 0; in other words there was very large variance among maternal families within populations, but not among populations (Supplementary Figure 3). While increased salinity resulted in a statistically meaningful reduction in succulence, it was only when conditioned on family that it added to the explanatory power of the model.
Root to shoot biomass ratio (log link) was the only variable for which the data supported the saturated model as the best model (Figure 3). The conditional R2 = 0.206, while the marginal R2 = 0.017. An ANOVA to evaluate the fixed effects revealed that the main effect of salinity was significant (chisq = 7.38; p = 0.007) indicating increased root to shoot allocation in response to salinity, but not the main effect of N. In addition, the interaction of salinity × N was significant (chisq = 6.19; p = 0.01). At ambient N levels (no N added), root to shoot ratio was not affected by increasing salt concentration. With the addition of N fertilizer, root to shoot ratio increased in response to high salinity (Figure 3B). However, the small size of the marginal R2 suggested that these effects were mainly meaningful when conditioned on the random terms. The random terms population, maternal family nested within population, and block account for 13, 24, and 6% of the random variance, respectively (Supplementary Figure 5).
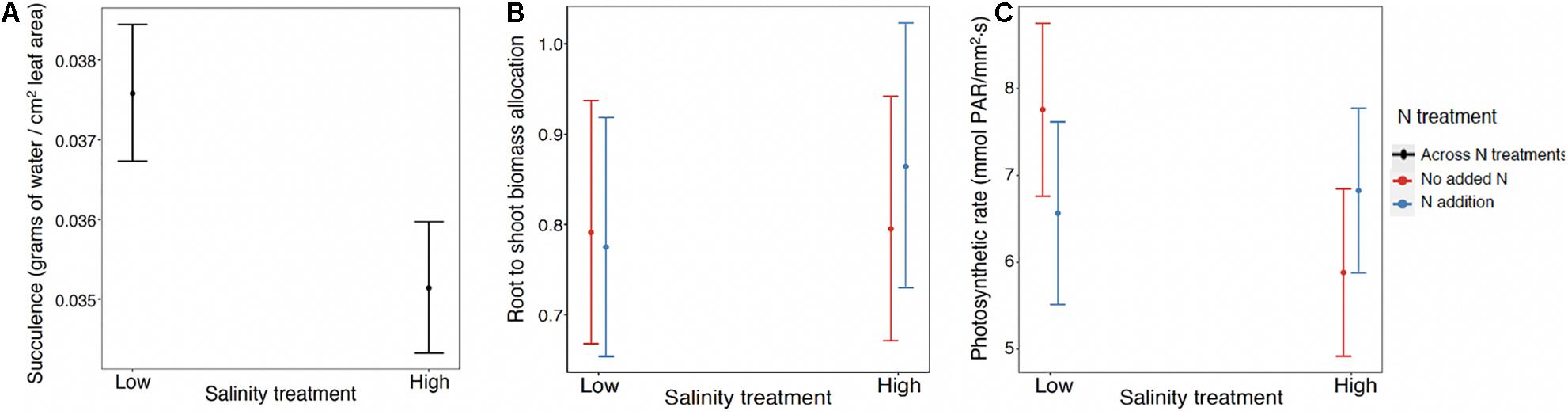
Figure 3. (A) Succulence response to salt across populations with no N effect, including the random effects of maternal families within populations and populations (N = 818). Interaction effect for (B) root to shoot biomass allocation and (C) photosynthetic rate where response to N depends on salt treatment across all mangrove propagules. The best model for root to shoot biomass allocation (N = 899) also includes the fixed effect of salt and random effects of population, maternal family within population and block. The best model for photosynthetic rate (N = 118) does not include any of the random effects.
The best model for total biomass (log link) included only the random effects of population and maternal family within population. The conditional R2 = 0.12. The terms for population and family nested within population accounted for 13 and 21% of the random variance, respectively (Supplementary Figure 6).
The photosynthesis data were the most limited in sample size since we were only able to assess one individual with at least two healthy leaves for each surviving maternal line for each treatment (N = 118). The best model was one including the fixed effects salinity, N, and their interaction, but no random effects (Table 1). An ANOVA to evaluate these fixed effects showed the main effects of salinity and N were not significant but the interaction was (chisq = 4.46; p = 0.035). At ambient N levels (no N added), maximum photosynthetic rate declined with increasing salt concentration, but this negative impact of salinity was absent or reversed upon the addition of N fertilizer (Figure 3C).
In summary, we found plasticity to our treatments for three of the six traits we measured. Succulence decreased in response to higher salinity (Figure 3A), but this response varied largely by family (Supplementary Figure 3). For root to shoot biomass ratio and maximum photosynthetic rate the responses to experimental treatments depended on changes in both salinity and N (Figures 3B,C). Root to shoot ratio also varied overall in response to salinity as well as by family and population (Supplementary Figure 4).
Discussion
We assessed the growth and survival of R. mangle propagules to full factorial combinations of two salt and two N levels because these are two important abiotic properties that potentially have important impacts on mangrove survival and traits, and by extension, on the biodiversity and ecosystem function of coastal wetlands. In addition to natural variation in these conditions, anthropogenic activities may result in more extreme levels of these conditions from runoff and flooding (Ellison et al., 2005; Krauss et al., 2006; Gedan et al., 2009; Kirwan and Megonigal, 2013; Lewis et al., 2014). We predicted that R. mangle seedlings would be plastic in response to salinity and N amendment in putative adaptive traits that conserve water and adjust allocation of N. Our study showed that only succulence and root to shoot ratio were plastic in response to salt, regardless of N treatment. Allocation to root and shoot biomass and maximum photosynthetic rate were also plastic, but response of both traits to N amendment depended on the level of salt. This supported our second prediction that response to salinity and N amendment will co-vary as plants shift resources to maintain osmotic balance. We also found support for our third prediction that populations would vary in putative adaptive traits, and in plasticity of these traits, due to population differentiation. Importantly, every trait except for photosynthesis varied among population and maternal families within populations. This was also true of survival. In fact, maternal family and population were the most consistent predictors for variation in traits and survival.
Phenotypic Plasticity in Response to Treatments
We expected that R. mangle propagule survival and growth would decrease in response to high salinity and increase in response to N fertilization. We also expected that N could alleviate some of the effects of salinity as indicated by an interaction of the two conditions. However, we found no response to treatments in survival, height growth, LMA or total dry biomass. This may be because the propagules were supported by resources provided by the maternal tree, which in R. mangle can support growth for at least a year (Ball, 2002; Proffitt and Travis, 2010). If the seedlings were supported by these maternal reserves, height growth would likely be more correlated to propagule length at collection which would be corrected for in the start-of-experiment (time zero) height measurements that we included as a covariate. Because our treatment duration was only 6 months, the lack of growth response to treatments was consistent with dependence on maternal reserves. However, we did see seedling response to treatment in succulence, allocation to root and shoot biomass and maximum photosynthetic rate.
Increased succulence is a common response to water deficiency under high salinity conditions (Rosenthal et al., 2002; Vendramini et al., 2002; Ottow et al., 2005; Karrenberg et al., 2006; Richards et al., 2008, 2010a), but in our experiment we detected reduced succulence in response to high salt. However, this could be because R. mangle excludes salt. Many halophytes absorb high concentrations of salt for osmotic adjustment that would normally be toxic. One strategy is to compartmentalize these ions along with increased succulence (Flowers and Colmer, 2008). On the other hand, many plants avoid this toxicity by excluding salt at the roots (Cavalieri and Huang, 1979; Donovan et al., 1996; Tester and Davenport, 2003). For example, several other halophytes that are salt excluders, including the succulent plant Salicornia europaea L., and another member of Rhizophoraceae, Kandelia candel (L.) Druce, do not increase succulence or leaf thickness in response to high salinity (Glenn and O’Leary, 1984; Kao et al., 2001). In fact, with N fertilization K. candel decreased leaf thickness when salinity was increased (Kao et al., 2001). Thus, one possible explanation for our results is that the N fertilized seedlings were able to reallocate resources (e.g., increased N) to salt tolerance mechanisms like compatible solutes, and still maintain positive water balance in the high salt condition without increased succulence.
Although we saw a statistically significant response to our treatments in three of the six traits, the interaction between salt and N fertilization only effected R:S and maximum photosynthetic rate. We expected maximum photosynthetic rate to increase in response to N fertilization because the enzyme RuBisCO, which catalyzes the dark reactions in photosynthesis, requires a large amount of N (Sage et al., 1987; Andersson, 2008). Further, a meta-analysis across 356 diverse species representing most biomes and growth forms showed increased maximum photosynthetic rate with increased N is a general phenomenon (Walker et al., 2014). This could lead to increased biomass allocation to shoots relative to roots. Despite this expectation, there was no overall response to high N level independent of salt treatment. One reason might be that photosynthesis overall was limited by other nutrients, not just N, and thus increasing N alone might not have been enough to elicit a response (see e.g., Lovelock et al., 2006). In a field study, dwarf R. mangle did not respond to N alone, presumably because other nutrients were limiting (Feller, 1995; Lovelock et al., 2006). Dwarf mangroves did increase biomass in response to fertilization with all three nutrients: N, phosphorus (P), and potassium ions (K+) (Feller, 1995), and in response to P addition alone (Lovelock et al., 2006). We also expected that response to N amendment would depend on salinity. We found that in plants treated with high salt, maximum photosynthetic rate was slightly enhanced by high N. Possibly, the additional N enabled the plants to synthesize N-rich compatible solutes for osmotic regulation and continued photosynthetic gain of carbon (Flowers and Colmer, 2008). Plants in high salt also responded differently in allocation of biomass with increased N; instead of increasing shoot biomass, they increased root biomass on average.
Variation Within and Among Sites
Phenotypic variation that is maintained in common garden from within and among populations would indicate R. mangle has heritable trait diversity which could allow for response to changing environmental conditions. Seedling survival depended on population and varied among maternal families within the six populations. We found variation in height growth, succulence, LMA, root to shoot allocation, and total dry biomass was largely determined by maternal families within populations. Proffitt and Travis (2010) also found seedling survival varied among maternal families, as well as by location in the intertidal zone. But in their study after 3 years, growth and survival did not reflect initial propagule size (Proffitt and Travis, 2010). Our results support these previous findings that propagule length is positively correlated to short term performance. This findings suggests that maternal reserves in the R. mangle propagule can help the seedling survive, and larger propagules contain more maternal reserves than smaller propagules (Ball, 2002; Proffitt and Travis, 2010). Because our study was a short-term, controlled greenhouse study, maximum photosynthetic rate might be the best indicator as an immediate response. Variation in maximum photosynthetic rate can ultimately manifest as variation in growth and allocation of resources, particularly once the seedling has depleted maternal reserves. The seedlings did not show significant differences in height growth or total dry biomass in response to treatments. But given the plasticity we saw in maximum photosynthetic rate, and the 1-year and 3-year growth results found in a previous study of nearby populations (Proffitt and Travis, 2010), we might have found a more dramatic response to these treatments with more time (Ball and Farquhar, 1984). However, previous work in several different systems have argued that greenhouse studies are often unable to recreate relevant field conditions, so such responses may only be registered in the field (Schittko et al., 2016; Rinella and Reinhart, 2017; Forero et al., 2019; Dostál et al., 2020).
The amount of heritable phenotypic diversity and differentiation we discovered in this study is an important indicator of the potential for this species to respond to changing conditions. The high level of diversity in response among populations and maternal families within populations may be surprising since we previously reported low genetic diversity among these plants based on molecular markers. Low genetic diversity is expected to limit the potential for different responses among individuals. On the other hand, we discovered high epigenetic diversity (Mounger et al., 2021), which could contribute to phenotypic and functional diversity, and could be a mechanism underlying the type of phenotypic differences and plasticity we found here (Zhang et al., 2013; Nicotra et al., 2015; Herrera et al., 2017; Jueterbock et al., 2020). In addition, we know very little about the interactions with the microbiome in the species, but microbes have been highlighted as important symbionts in these and other challenging environments (Bowen et al., 2017; Angermeyer et al., 2018; Jung et al., 2021). Soil microbial activity could have dramatic impacts on the future nutrient availability and stability of these coastal sediments (Deegan et al., 2012; Bowen et al., 2017; Hughes et al., 2020; Lewis et al., 2021). In fact, a recent study suggested that bacterial community composition differed among R. mangle maternal genotypes but not with genetic diversity (Craig et al., 2020).
Conclusion
Previous work suggested that R. mangle growth and survival depended on an interaction of intertidal elevation and maternal genotype, suggesting variation in response to flooding conditions (Proffitt and Travis, 2010). However, in addition to changes in flooding, anthropogenic activities are causing changes in salinity and N level in R. mangle ecosystems (McKee et al., 2007). The interacting effects of salinity, N level, and elevation are complex and potentially non-additive (McKee et al., 2007). Our experimental findings suggest that the important traits of succulence, root to shoot biomass allocation and photosynthetic rate respond to salinity, N level, or the combination of these conditions. We also discovered that seedling survival and the magnitude of almost all responses varied among populations and even maternal families within populations.
This variation in survival and important traits among families and among populations is particularly interesting considering the importance of this foundation species for the functioning of the coastal ecosystem. Our previous work showed a lack of genetic diversity, which might be alarming considering the expected limitations of low genetic variation (Allendorf et al., 2010; Estoup et al., 2016). However, accumulating studies provide important evidence that genetic variation must be interpreted with caution (Hufford and Mazer, 2003; Estoup et al., 2016) and that the emphasis on only variation in DNA sequence can be misguided (Keller, 2002, 2014; Sultan, 2015; Bonduriansky and Day, 2018). Non-genetic sources of response may contribute to the phenotypic diversity we report here that is particularly relevant under different salinity and N conditions. This may provide another source of resilience for R. mangle and other critical species to changing environmental conditions and contribute to future adaptation to a complex mosaic of environmental conditions.
Data Availability Statement
All of the data collected and analyzed for this study along with R code are included in the Supplementary Material, Further inquiries can be directed to the corresponding author.
Author Contributions
CR, KL, GF, and DL conceived the study and designed the experiments. KL and JM collected plants and maintained the experiments. KL and GF analyzed the data. CR and KL wrote the first draft of the manuscript. All authors provided input and revisions to the manuscript.
Funding
This work was supported by funding from the National Science Foundation (United States) IOS-1556820 (to CR), DUE-1930451 (to DL), and the Federal Ministry of Education and Research (BMBF, Germany; MOPGA Project ID 306055 to CR).
Conflict of Interest
The authors declare that the research was conducted in the absence of any commercial or financial relationships that could be construed as a potential conflict of interest.
Publisher’s Note
All claims expressed in this article are solely those of the authors and do not necessarily represent those of their affiliated organizations, or those of the publisher, the editors and the reviewers. Any product that may be evaluated in this article, or claim that may be made by its manufacturer, is not guaranteed or endorsed by the publisher.
Acknowledgments
We thank Bert Anderson, Sandy Voors, Viviana Penuela Useche, M. Teresa Boquete, Mariano Alverez, and Marta Robertson for their help with analyses and review of earlier versions of this manuscript. We are grateful to Christine Brubaker, Racquel Pancho, Brianna Jerman, Vernetta Williams, Alan Franck, and Mary Mangiapia for their guidance, and Charley’s Pizza for great food during greenhouse and plant processing work. We also thank Samantha Blonder, Jordan Dollbaum, Maria Nikolopoulos, Shane Palmer, Bradley Biega, Bryan Lotici, Harper Cassidy, Jelena Dosen, Nancy Sheridan, and Dawei Tang for their help in the field and the greenhouse, and our friends and family for constant support and encouragement. Finally, we acknowledge support by the Open Access Publishing Fund of University of Tübingen.
Supplementary Material
The Supplementary Material for this article can be found online at: https://www.frontiersin.org/articles/10.3389/fmars.2021.756683/full#supplementary-material
Supplementary Data Sheet 1 | SD1_ Code and Results.pdf = Rhizophora mangle data analysis code and annotated results (including Supplementary Figures 1–6).
Supplementary Data Sheet 2 | SD2_List of variable names.pdf = used in Rhizophora mangle data analysis code and annotated results.
Supplementary Data Sheet 3 | SD3_2017011 All No Calcs.csv data used to calculate survivorship.
Supplementary Data Sheet 4 | SD4_20170211 Survivors Pairs.csv data used with all plants to examine correlation.
Supplementary Data Sheet 5 | SD5_20170211 Survivors No Calcs.csv data used used for height, R:S and biomass.
Supplementary Data Sheet 6 | SD6_20160420 Final R25.csv data used for succulence and LMA data.
Supplementary Data Sheet 7 | SD7_20170728 licor FDFC.txt data used for photosynthetic rate.
References
Albrecht, M., Kneeland, K. M., Lindroth, E., and Foster, J. E. (2013). Genetic diversity and relatedness of the mangrove Rhizophora mangle L. (Rhizophoraceae) using amplified fragment polymorphism (AFLP) among locations in Florida, USA and the Caribbean. J. Coast. Conserv. 17, 483–491. doi: 10.1007/s11852-013-0246-3
Allendorf, F. W., Hohenlohe, P. A., and Luikart, G. (2010). Genomics and the future of conservation genetics. Nat. Rev. Genet. 11, 697–709. doi: 10.1038/nrg2844
Alongi, D. (2013). Cycling and global fluxes of nitrogen in mangroves. Glob. Environ. Res. 17, 173–182.
Alongi, D. M. (2008). Mangrove forests: resilience, protection from tsunamis, and responses to global climate change. Estuar. Coast. Shelf Sci. 76, 1–13. doi: 10.1016/j.ecss.2007.08.024
Andersson, I. (2008). Catalysis and regulation in Rubisco. J. Exp. Bot. 59, 1555–1568. doi: 10.1093/jxb/ern091
Angermeyer, A., Crosby, S. C., and Huber, J. A. (2018). Salt marsh sediment bacterial communities maintain original population structure after transplantation across a latitudinal gradient. PeerJ 6:e4735. doi: 10.7717/peerj.4735
Anisfeld, S. C., and Hill, T. D. (2012). Fertilization effects on elevation change and belowground carbon balance in a Long Island sound tidal marsh. Estuar. Coast. 35, 201–211. doi: 10.1007/s12237-011-9440-4
Antlfinger, A. E. (1981). The genetic basis of microdifferentiation in natural and experimental populations of Borrichia frutescens in relation to salinity. Evolution 35, 1056–1068. doi: 10.1111/j.1558-5646.1981.tb04974.x
Antlfinger, A. E., and Dunn, E. L. (1979). Seasonal patterns of CO2 and water vapor exchange of three salt marsh succulents. Oecologia 43, 249–260. doi: 10.1007/BF00344952
Antlfinger, A. E., and Dunn, E. L. (1983). Water use and salt balance in three salt marsh succulents. Am. J. Bot. 70:561. doi: 10.2307/2443167
Arntz, M. A., and Delph, L. F. (2001). Pattern and process: evidence for the evolution of photosynthetic traits in natural populations. Oecologia 127, 455–467. doi: 10.1007/s004420100650
Ball, M. C. (2002). Interactive effects of salinity and irradiance on growth: implications for mangrove forest structure along salinity gradients. Trees 16, 126–139. doi: 10.1007/s00468-002-0169-3
Ball, M. C., Cochrane, M. J., and Rawson, H. M. (1997). Growth and water use of the mangroves Rhizophora apiculata and R. stylosa in response to salinity and humidity under ambient and elevated concentrations of atmospheric CO2. Plant Cell Environ. 20, 1158–1166. doi: 10.1046/j.1365-3040.1997.d01-144.x
Ball, M. C., and Farquhar, G. D. (1984). Photosynthetic and stomatal responses of the grey mangrove, avicennia marina, to transient salinity conditions. Plant Physiol. 74, 7–11. doi: 10.1104/pp.74.1.7
Banta, J. A., and Richards, C. L. (2018). Quantitative epigenetics and evolution. Heredity 121, 210–224. doi: 10.1038/s41437-018-0114-x
Barbier, E. B., Koch, E. W., Silliman, B. R., Hacker, S. D., Wolanski, E., Primavera, J., et al. (2008). Coastal ecosystem-based management with nonlinear ecological functions and values. Science 319, 321–323. doi: 10.1126/science.1150349
Bates, D., Mächler, M., Bolker, B., and Walker, S. (2015). Fitting linear mixed-effects models Usinglme4. J. Stat. Softw. 67:1. doi: 10.18637/jss.v067.i01
Berndt, M. P., Katz, B. G., Kingsbury, J. A., and Crandall, C. A. (2015). The quality of our Nation’s waters: water quality in the Upper Floridan aquifer and overlying surficial aquifers, southeastern United States, 1993-2010. Circular 1355:72. doi: 10.3133/cir1355
Bertness, M. (2020). A Brief Natural History of Civilization: Why a Balance Between Cooperation and Competition Is Vital to Humanity. Yale: Yale University Press.
Bertness, M. D., and Callaway, R. (1994). Positive interactions in communities. Trends Ecol. Evol. 9, 191–193. doi: 10.1016/0169-5347(94)90088-4
Bertness, M. D., Ewanchuk, P. J., and Silliman, B. R. (2002). Anthropogenic modification of New England salt marsh landscapes. Proc. Natl. Acad. Sci. U.S.A. 99, 1395–1398. doi: 10.1073/pnas.022447299
Bolker, B. M. (2015). “Linear and generalized linear mixed models,” in Ecological Statistics, eds G. A. Fox, S. Negrete-Yankelevich, and V. J. Sosa (Oxford: Oxford University Press), 309–333.
Bowen, J. L., Kearns, P. J., Byrnes, J. E. K., Wigginton, S., Allen, W. J., Greenwood, M., et al. (2017). Lineage overwhelms environmental conditions in determining rhizosphere bacterial community structure in a cosmopolitan invasive plant. Nat. Commun. 8:433. doi: 10.1038/s41467-017-00626-0
Bowman, H. H. M. (1917). Ecology and physiology of the red mangrove. Proc. Am. Philos. Soc. 56, 589–672.
Caruso, C. M., Maherali, H., Mikulyuk, A., Carlson, K., and Jackson, R. B. (2005). Genetic variance and covariance for physiological traits in Lobelia: are there constraints on adaptive evolution? Evolution 59, 826–837. doi: 10.1111/j.0014-3820.2005.tb01756.x
Cavalieri, A. J., and Huang, A. H. C. (1979). Evaluation of proline accumulation in the adaptation of diverse species of marsh halophytes to the saline environment. Am. J. Bot. 66, 307–312. doi: 10.1002/j.1537-2197.1979.tb06228.x
Costanza, R., Pérez-Maqueo, O., Martinez, M. L., Sutton, P., Anderson, S. J., and Mulder, K. (2008). The value of coastal wetlands for hurricane protection. Ambio 37, 241–248.
Craig, H., Kennedy, J. P., Devlin, D. J., Bardgett, R. D., and Rowntree, J. K. (2020). Effects of maternal genotypic identity and genetic diversity of the red mangrove Rhizophora mangle on associated soil bacterial communities: a field-based experiment. Ecol. Evol. 10, 13957–13967. doi: 10.1002/ece3.6989
Crosby, S. C., Angermeyer, A., Adler, J. M., Bertness, M. D., Deegan, L. A., Sibinga, N., et al. (2017). Spartina alterniflora biomass allocation and temperature: implications for salt marsh persistence with sea-level rise. Estuar. Coast. 40, 213–223. doi: 10.1007/s12237-016-0142-9
Crotty, S. M., Ortals, C., Pettengill, T. M., Shi, L., Olabarrieta, M., Joyce, M. A., et al. (2020). Sea-level rise and the emergence of a keystone grazer alter the geomorphic evolution and ecology of southeast US salt marshes. Proc. Natl. Acad. Sci. U.S.A. 117, 17891–17902. doi: 10.1073/pnas.1917869117
Davis, M. B., and Shaw, R. G. (2001). Range shifts and adaptive responses to Quaternary climate change. Science 292, 673–679. doi: 10.1126/science.292.5517.673
Deegan, L. A., Johnson, D. S., Warren, R. S., Peterson, B. J., Fleeger, J. W., Fagherazzi, S., et al. (2012). Coastal eutrophication as a driver of salt marsh loss. Nature 490, 388–392. doi: 10.1038/nature11533
DeYoe, H., Lonard, R. I., Judd, F. W., Stalter, R., and Feller, I. (2020). Biological flora of the tropical and subtropical intertidal zone: literature review for Rhizophora mangle L. J. Coast. Res. 36:857. doi: 10.2112/jcoastres-d-19-00088.1
Donovan, L. A., Richards, J. H., and Muller, M. W. (1996). Water relations and leaf chemistry of Chrysothamnus nauseosus ssp. Consimilis (Asteraceae) and Sarcobatus vermiculatus (Chenopodiaceae). Am. J. Bot. 83:1637. doi: 10.2307/2445840
Donovan, L. A., Richards, J. H., and Schaber, E. J. (1997). Nutrient relations of the halophytic shrub, Sarcobatus vermiculatus, along a soil salinity gradient. Plant Soil 190, 105–117. doi: 10.1023/A:1004211207079
Dostál, P., Fischer, M., and Prati, D. (2020). Comparing experimental and field-measured traits and their variability in Central European grassland species. J. Veg. Sci. 31, 561–570. doi: 10.1111/jvs.12875
Ellison, A., Farnsworth, E., and Moore, G. (2015). Rhizophora Mangle. IUCN Red List of Threatened Species. Gland: IUCN.
Ellison, A. M. (2019). Foundation species, non-trophic interactions, and the value of being common. iScience 13, 254–268. doi: 10.1016/j.isci.2019.02.020
Ellison, A. M., Bank, M. S., Clinton, B. D., Colburn, E. A., Elliott, K., Ford, C. R., et al. (2005). Loss of foundation species: consequences for the structure and dynamics of forested ecosystems. Front. Ecol. Environ. 3, 479–486.
Estoup, A., Ravigné, V., Hufbauer, R., Vitalis, R., Gautier, M., and Facon, B. (2016). Is there a genetic paradox of biological invasion? Annu. Rev. Ecol. Evol. Syst. 47, 51–72. doi: 10.1146/annurev-ecolsys-121415-032116
Feller, I. C. (1995). Effects of nutrient enrichment on growth and herbivory of dwarf red mangrove (Rhizophora mangle). Ecol. Monogr. 65, 477–505. doi: 10.2307/2963499
Feller, I. C., Lovelock, C. E., Berger, U., McKee, K. L., Joye, S. B., and Ball, M. C. (2010). Biocomplexity in mangrove ecosystems. Ann. Rev. Mar. Sci. 2, 395–417. doi: 10.1146/annurev.marine.010908.163809
Feller, I. C., Whigham, D. F., McKee, K. L., and Lovelock, C. E. (2003). Nitrogen limitation of growth and nutrient dynamics in a disturbed mangrove forest, Indian River Lagoon, Florida. Oecologia 134, 405–414. doi: 10.1007/s00442-002-1117-z
Flowers, T. J., and Colmer, T. D. (2008). Salinity tolerance in halophytes. New Phytol. 179, 945–963. doi: 10.1111/j.1469-8137.2008.02531.x
Food and Agriculture Organization of the United Nations (2007). The World’s Mangroves 1980-2005. A Thematic Study Prepared in the Framework of the Global Forest Resources Assessment 2005 [2007]. Rome: FAO.
Forero, L. E., Grenzer, J., Heinze, J., Schittko, C., and Kulmatiski, A. (2019). Greenhouse- and field-measured plant-soil feedbacks are not correlated. Front. Environ. Sci. 7:184. doi: 10.3389/fenvs.2019.00184
Fox, J., and Weisberg, S. (2019). An R Companion to Applied Regression, Third edition. Thousand Oaks, CA: Sage.
Friess, D. A., Krauss, K. W., Horstman, E. M., Balke, T., Bouma, T. J., Galli, D., et al. (2012). Are all intertidal wetlands naturally created equal? Bottlenecks, thresholds and knowledge gaps to mangrove and saltmarsh ecosystems. Biol. Rev. Camb. Philos. Soc. 87, 346–366. doi: 10.1111/j.1469-185x.2011.00198.x
Gao, L., Geng, Y., Li, B., Chen, J., and Yang, J. (2010). Genome-wide DNA methylation alterations of Alternanthera philoxeroides in natural and manipulated habitats: implications for epigenetic regulation of rapid responses to environmental fluctuation and phenotypic variation. Plant Cell Environ. 33, 1820–1827. doi: 10.1111/j.1365-3040.2010.02186.x
Geber, M. A., and Griffen, L. R. (2003). Inheritance and natural selection on functional traits. Int. J. Plant Sci. 164, S21–S42. doi: 10.1086/368233
Gedan, K. B., Kirwan, M. L., Wolanski, E., and Barbier, E. B. (2011). The present and future role of coastal wetland vegetation in protecting shorelines: answering recent challenges to the paradigm. Clim. Change 106, 7–29. doi: 10.1007/s10584-010-0003-7
Gedan, K. B., Silliman, B. R., and Bertness, M. D. (2009). Centuries of human-driven change in salt marsh ecosystems. Ann. Rev. Mar. Sci. 1, 117–141. doi: 10.1146/annurev.marine.010908.163930
Glenn, E. P., and O’Leary, W. (1984). Relationship between salt accumulation and water content of dicotyledonous halophytes. Plant Cell Environ. 7, 253–261. doi: 10.1111/1365-3040.ep11589448
Guo, J., Richards, C. L., Holsinger, K. E., Fox, G. A., Zhang, Z., and Zhou, C. (2021). Genetic structure in patchy populations of a candidate foundation plant: a case study of Leymus chinensis (Poaceae) using genetic and clonal diversity. Am. J. Bot. doi: 10.1002/ajb2.1771
Haddad, N. M., Brudvig, L. A., Clobert, J., Davies, K. F., Gonzalez, A., Holt, R. D., et al. (2015). Habitat fragmentation and its lasting impact on Earth’s ecosystems. Sci. Adv. 1:e1500052. doi: 10.1126/sciadv.1500052
Herrera, C. M., Medrano, M., and Bazaga, P. (2017). Comparative epigenetic and genetic spatial structure of the perennial herb Helleborus foetidus: isolation by environment, isolation by distance, and functional trait divergence. Am. J. Bot. 104, 1195–1204. doi: 10.3732/ajb.1700162
Hufford, K. M., and Mazer, S. J. (2003). Plant ecotypes: genetic differentiation in the age of ecological restoration. Trends Ecol. Evol. 18, 147–155. doi: 10.1016/s0169-5347(03)00002-8
Hughes, A. R., Moore, A. F. P., and Gehring, C. (2020). Plant response to fungal root endophytes varies by host genotype in the foundation species Spartina alterniflora. Am. J. Bot. 107, 1645–1653. doi: 10.1002/ajb2.1573
IPCC (2014). Climate change 2014: synthesis report. Paper Presented at the Contribution of Working Groups I, II and III to the Fifth Assessment Report of the Intergovernmental Panel on Climate Change, eds R. K. Pachauri and L. A. Meyer Geneva (Switzerland: IPCC).
IUCN (2007). Environmental and Socio Economic Value of Mangroves in Tsunami Affected Areas. Gland: IUCN.
Jueterbock, A., Boström, C., Coyer, J. A., Olsen, J. L., Kopp, M., Dhanasiri, A. K. S., et al. (2020). The seagrass methylome is associated with variation in photosynthetic performance among clonal shoots. Front. Plant Sci. 11:571646. doi: 10.3389/fpls.2020.571646
Jung, J. H., Reis, F., Richards, C. L., and Bossdorf, O. (2021). Understanding plant microbiomes requires a genotype × environment framework. Am. J. Bot. 108, 1–4. doi: 10.1002/ajb2.1742
Kao, W.-Y., Tsai, H.-C., and Tsai, T.-T. (2001). Effect of NaCl and nitrogen availability on growth and photosynthesis of seedlings of a mangrove species, Kandelia candel (L.) Druce. J. Plant Physiol. 158, 841–846. doi: 10.1078/0176-1617-00248
Karrenberg, S., Edelist, C., Lexer, C., and Rieseberg, L. (2006). Response to salinity in the homoploid hybrid species Helianthus paradoxus and its progenitors H. annuus and H. petiolaris. New Phytol. 170, 615–629. doi: 10.1111/j.1469-8137.2006.01687.x
Keith, A. R., Bailey, J. K., Lau, M. K., and Whitham, T. G. (2017). Genetics-based interactions of foundation species affect community diversity, stability and network structure. Proc. Biol. Sci. 284:20162703. doi: 10.1098/rspb.2016.2703
Keller, E. F. (2014). From gene action to reactive genomes. J. Physiol. 592, 2423–2429. doi: 10.1113/jphysiol.2014.270991
Kennedy, J. P., Garavelli, L., Truelove, N. K., Devlin, D. J., Box, S. J., Chérubin, L. M., et al. (2017). Contrasting genetic effects of red mangrove (Rhizophora mangleL.) range expansion along West and East Florida. J. Biogeogr. 44, 335–347. doi: 10.1111/jbi.12813
Kirwan, M. L., and Megonigal, J. P. (2013). Tidal wetland stability in the face of human impacts and sea-level rise. Nature 504, 53–60. doi: 10.1038/nature12856
Kirwan, M. L., Temmerman, S., Skeehan, E. E., Guntenspergen, G. R., and Fagherazzi, S. (2016). Overestimation of marsh vulnerability to sea level rise. Nat. Clim. Chang. 6, 253–260. doi: 10.1038/nclimate2909
Krauss, K. W., Lovelock, C. E., McKee, K. L., López-Hoffman, L., Ewe, S. M. L., and Sousa, W. P. (2008). Environmental drivers in mangrove establishment and early development: a review. Aquat. Bot. 89, 105–127. doi: 10.1016/j.aquabot.2007.12.014
Krauss, K. W., Twilley, R. R., Doyle, T. W., and Gardiner, E. S. (2006). Leaf gas exchange characteristics of three neotropical mangrove species in response to varying hydroperiod. Tree Physiol. 26, 959–968. doi: 10.1093/treephys/26.7.959
Lewis, D. B., Brown, J. A., and Jimenez, K. L. (2014). Effects of flooding and warming on soil organic matter mineralization in Avicennia germinans mangrove forests and Juncus roemerianus salt marshes. Estuar. Coast. Shelf Sci. 139, 11–19. doi: 10.1016/j.ecss.2013.12.032
Lewis, D. B., Jimenez, K. L., Abd-Elrahman, A., Andreu, M. G., Landry, S. M., Northrop, R. J., et al. (2021). Carbon and nitrogen pools and mobile fractions in surface soils across a mangrove saltmarsh ecotone. Sci. Total Environ. 798:149328. doi: 10.1016/j.scitotenv.2021.149328
Lovelock, C. E., Ball, M. C., Choat, B., Engelbrecht, B. M. J., Holbrook, N. M., and Feller, I. C. (2006). Linking physiological processes with mangrove forest structure: phosphorus deficiency limits canopy development, hydraulic conductivity and photosynthetic carbon gain in dwarf Rhizophora mangle. Plant Cell Environ. 29, 793–802. doi: 10.1111/j.1365-3040.2005.01446.x
Lovelock, C. E., Krauss, K. W., Osland, M. J., Reef, R., and Ball, M. C. (2016). “The physiology of mangrove trees with changing climate,” in Tree Physiology, eds G. Goldstein and L. S. Santiago (Cham: Springer International Publishing), 149–179.
Matesanz, S., Blanco-Sánchez, M., Ramos-Muñoz, M., de la Cruz, M., Benavides, R., and Escudero, A. (2021). Phenotypic integration does not constrain phenotypic plasticity: differential plasticity of traits is associated to their integration across environments. New Phytol. 231, 2359–2370. doi: 10.1111/nph.17536
Matesanz, S., and Sultan, S. E. (2013). High-performance genotypes in an introduced plant: insights to future invasiveness. Ecology 94, 2464–2474. doi: 10.1890/12-1359.1
McKee, K. L. (1995). Seedling recruitment patterns in a Belizean mangrove forest: effects of establishment ability and physico-chemical factors. Oecologia 101, 448–460. doi: 10.1007/BF00329423
McKee, K. L., Cahoon, D. R., and Feller, I. C. (2007). Caribbean mangroves adjust to rising sea level through biotic controls on change in soil elevation. Glob. Ecol. Biogeogr. 16, 545–556. doi: 10.1111/j.1466-8238.2007.00317.x
Medina, E., Fernandez, W., and Barboza, F. (2015). Element uptake, accumulation, and resorption in leaves of mangrove species with different mechanisms of salt regulation. Web Ecol. 15, 3–13. doi: 10.5194/we-15-3-2015
Melillo, J. M., Richmond, T. T. C., and Yohe, G. W. (2014). Climate Change Impacts in the United States: The Third National Climate Assessment. London: U.S. Global Change Research Program.
Mounger, J., Teresa Boquete, M., Schmid, M. W., Granado, R., Robertson, M. H., Voors, S. A., et al. (2021). Inheritance of DNA methylation differences in the mangrove Rhizophora mangle. Evol. Dev. 23, 351–374. doi: 10.1111/ede.12388
Nadia, T. L., and Machado, I. C. (2014). Wind pollination and propagule formation in Rhizophora mangle L. (Rhizophoraceae): resource or pollination limitation? An. Acad. Bras. Cienc. 86, 229–238. doi: 10.1590/0001-37652014101712
Nakagawa, S., and Schielzeth, H. (2013). A general and simple method for obtainingR2from generalized linear mixed-effects models. Methods Ecol. Evol. 4, 133–142. doi: 10.1111/j.2041-210x.2012.00261.x
Nicotra, A. B., Atkin, O. K., Bonser, S. P., Davidson, A. M., Finnegan, E. J., Mathesius, U., et al. (2010). Plant phenotypic plasticity in a changing climate. Trends Plant Sci. 15, 684–692. doi: 10.1016/j.tplants.2010.09.008
Nicotra, A. B., Segal, D. L., Hoyle, G. L., Schrey, A. W., Verhoeven, K. J. F., and Richards, C. L. (2015). Adaptive plasticity and epigenetic variation in response to warming in an Alpine plant. Ecol. Evol. 5, 634–647. doi: 10.1002/ece3.1329
Osland, M. J., Enwright, N., Day, R. H., and Doyle, T. W. (2013). Winter climate change and coastal wetland foundation species: salt marshes vs. mangrove forests in the southeastern United States. Glob. Chang. Biol. 19, 1482–1494. doi: 10.1111/gcb.12126
Osland, M. J., Griffith, K. T., Larriviere, J. C., Feher, L. C., Cahoon, D. R., Enwright, N. M., et al. (2017). Assessing coastal wetland vulnerability to sea-level rise along the northern Gulf of Mexico coast: gaps and opportunities for developing a coordinated regional sampling network. PLoS One 12:e0183431. doi: 10.1371/journal.pone.0183431
Ottow, E. A., Brinker, M., Teichmann, T., Fritz, E., Kaiser, W., Brosché, M., et al. (2005). Populus euphratica displays apoplastic sodium accumulation, osmotic adjustment by decreases in calcium and soluble carbohydrates, and develops leaf succulence under salt stress. Plant Physiol. 139, 1762–1772. doi: 10.1104/pp.105.069971
Parmesan, C. (2006). Ecological and evolutionary responses to recent climate change. Annu. Rev. Ecol. Evol. Syst. 37, 637–669. doi: 10.1146/annurev.ecolsys.37.091305.110100
Pennings, S. C., and Bertness, M. (2001). “Salt marsh communities,” in Marine Community Ecology, eds S. Bertness, D. Gaines, and M. Hay (Sunderland, MA: Sinauer Associates), 289–316.
Pennings, S. C., and Richards, C. L. (1998). Effects of wrack burial in salt-stressed habitats: Batis maritima in a southwest Atlantic salt marsh. Ecography 21, 630–638. doi: 10.1111/j.1600-0587.1998.tb00556.x
Penuela Useche, V. (2015). Influences of Yard Management Intensity on Urban Soil Biogeochemistry. Available online at: https://digitalcommons.usf.edu/cgi/viewcontent.cgi?article=6582&context=etd (accessed September 21, 2021).
Pimentel, D., Culliney, T., Butler, I., Reinemann, D., and Beckman, K. (1989). “Low-input sustainable agriculture using ecological management practices,” in Agricultural Ecology and Environment, eds M. G. Paoletti, B. R. Stinner, and G. G. Lorenzoni (Amsterdam: Elsevier).
Polidoro, B. A., Carpenter, K. E., Collins, L., Duke, N. C., Ellison, A. M., Ellison, J. C., et al. (2010). The loss of species: mangrove extinction risk and geographic areas of global concern. PLoS One 5:e10095. doi: 10.1371/journal.pone.0010095
Proffitt, C. E., and Travis, S. (2014). Red mangrove life history variables along latitudinal and anthropogenic stress gradients. Ecol. Evol. 4, 2352–2359. doi: 10.1002/ece3.1095
Proffitt, C. E., and Travis, S. E. (2005). Albino mutation rates in red mangroves (Rhizophora mangle L.) as a bioassay of contamination history in Tampa Bay, Florida, USA. Wetlands 25, 326–334.
Proffitt, C. E., and Travis, S. E. (2010). Red mangrove seedling survival, growth, and reproduction: effects of environment and maternal genotype. Estuar. Coast. 33, 890–901. doi: 10.1007/s12237-010-9265-6
Qiao, X., Zhang, J., Wang, Z., Xu, Y., Zhou, T., Mi, X., et al. (2021). Foundation species across a latitudinal gradient in China. Ecology 102:e03234. doi: 10.1002/ecy.3234
R Core Team (2020). R: A Language and Environment for Statistical Computing. Vienna: R Foundation for Statistical Computing.
Raju Aluri, J. S. (2013). Reproductive ecology of mangrove flora: conservation and management. Trans. Rev. Syst. Ecol. Res. 15, 133–184. doi: 10.2478/trser-2013-0026
Richards, C. L., Bossdorf, O., Muth, N. Z., Gurevitch, J., and Pigliucci, M. (2006). Jack of all trades, master of some? On the role of phenotypic plasticity in plant invasions. Ecol. Lett. 9, 981–993. doi: 10.1111/j.1461-0248.2006.00950.x
Richards, C. L., Pennings, S. C., and Donovan, L. A. (2005). Habitat range and phenotypic variation in salt marsh plants. Plant Ecol. 176, 263–273. doi: 10.1007/s11258-004-0841-3
Richards, C. L., Schrey, A. W., and Pigliucci, M. (2012). Invasion of diverse habitats by few Japanese knotweed genotypes is correlated with epigenetic differentiation. Ecol. Lett. 15, 1016–1025. doi: 10.1111/j.1461-0248.2012.01824.x
Richards, C. L., Walls, R. L., and Bailey, J. P. (2008). Plasticity in salt tolerance traits allows for invasion of novel habitat by Japanese knotweed sl (Fallopia japonica and F.×bohemica, Polygonaceae). Am. J. Bot. 95, 931–942. doi: 10.3732/ajb.2007364
Richards, C. L., White, S. N., McGuire, M. A., and Franks, S. J. (2010b). Plasticity, not adaptation to salt level, explains variation along a salinity gradient in a salt marsh perennial. Estuaries 33, 840–852. doi: 10.1007/s12237-009-9186-4
Richards, C. L., Wares, J. P., and Mackie, J. A. (2010a). Evaluating adaptive processes for conservation and management of estuarine and coastal resources. Estuar. Coasts 33, 805–810.
Rinella, M. J., and Reinhart, K. O. (2017). Mixing soil samples across experimental units ignores uncertainty and generates incorrect estimates of soil biota effects on plants: response to Cahill et al. (2017) No silver bullet: different soil handling techniques are useful for different research questions, exhibit differential type I and II error rates, and are sensitive to sampling intensity. New Phytol. 216, 15–17. doi: 10.1111/nph.14432
Rosenthal, D. M., Schwarzbach, A. E., Donovan, L. A., Raymond, O., and Rieseberg, L. H. (2002). Phenotypic differentiation between three ancient hybrid taxa and their parental species. Int. J. Plant Sci. 163, 387–398. doi: 10.1086/339237
Sage, R. F., Pearcy, R. W., and Seemann, J. R. (1987). The nitrogen use efficiency of C(3) and C(4) plants : III. Leaf nitrogen effects on the activity of carboxylating enzymes in Chenopodium album (L.) and Amaranthus retroflexus (L.). Plant Physiol. 85, 355–359. doi: 10.1104/pp.85.2.355
Schittko, C., Runge, C., Strupp, M., Wolff, S., and Wurst, S. (2016). No evidence that plant-soil feedback effects of native and invasive plant species under glasshouse conditions are reflected in the field. J. Ecol. 104, 1243–1249. doi: 10.1111/1365-2745.12603
Schuerch, M., Spencer, T., Temmerman, S., Kirwan, M. L., Wolff, C., Lincke, D., et al. (2018). Future response of global coastal wetlands to sea-level rise. Nature 561, 231–234. doi: 10.1038/s41586-018-0476-5
Smith, S. M., and Snedaker, S. C. (1995). Salinity responses in two populations of viviparous Rhizophora mangle L. seedlings. Biotropica 27:435. doi: 10.2307/2388955
Sultan, S. E. (2001). Phenotypic plasticity for fitness components in polygonum species of contrasting ecological breadth. Ecology 82, 328–343.
Sultan, S. E. (2015). Organism and Environment: Ecological Development, Niche Construction, and Adaptation. Oxford: Oxford University Press.
Tester, M., and Davenport, R. (2003). Na+ tolerance and Na+ transport in higher plants. Ann. Bot. 91, 503–527. doi: 10.1093/aob/mcg058
Turner, R. E. (2011). Beneath the salt marsh canopy: loss of soil strength with increasing nutrient loads. Estuar. Coast. 34, 1084–1093. doi: 10.1007/s12237-010-9341-y
Vendramini, F., Díaz, S., Gurvich, D. E., Wilson, P. J., Thompson, K., and Hodgson, J. G. (2002). Leaf traits as indicators of resource-use strategy in floras with succulent species. New Phytol. 154, 147–157. doi: 10.1046/j.1469-8137.2002.00357.x
Verhoeven, K. J. F., Van Dijk, P. J., and Biere, A. (2010). Changes in genomic methylation patterns during the formation of triploid asexual dandelion lineages. Mol. Ecol. 19, 315–324. doi: 10.1111/j.1365-294X.2009.04460.x
Vovides, A. G., Vogt, J., Kollert, A., Berger, U., Grueters, U., Peters, R., et al. (2014). Morphological plasticity in mangrove trees: salinity-related changes in the allometry of Avicennia germinans. Trees 28, 1413–1425. doi: 10.1007/s00468-014-1044-8
Walker, A. P., Beckerman, A. P., Gu, L., Kattge, J., Cernusak, L. A., Domingues, T. F., et al. (2014). The relationship of leaf photosynthetic traits - V cmax and J max - to leaf nitrogen, leaf phosphorus, and specific leaf area: a meta-analysis and modeling study. Ecol. Evol. 4, 3218–3235. doi: 10.1002/ece3.1173
Wise, R. R., and Juncosa, A. M. (1989). Ultrastructure of the transfer tissues during viviparous seedling development in Rhizophora mangle (Rhizophoraceae). Am. J. Bot. 76, 1286–1298. doi: 10.1002/j.1537-2197.1989.tb15110.x
Wuebbles, D., Meehl, G., Hayhoe, K., Karl, T. R., Kunkel, K., Santer, B., et al. (2014). CMIP5 climate model analyses: climate extremes in the United States. Bull. Am. Meteorol. Soc. 95, 571–583. doi: 10.1175/bams-d-12-00172.1
Zedler, J. B., and Kercher, S. (2005). Wetland resources: status, trends, ecosystem services, and restorability. Annu. Rev. Environ. Resour. 30, 39–74. doi: 10.1146/annurev.energy.30.050504.144248
Keywords: coastal ecosystem, conservation genetics, foundation species, mangroves, phenotypic plasticity, Rhizophora mangle
Citation: Richards CL, Langanke KL, Mounger J, Fox GA and Lewis DB (2021) Trait Response to Nitrogen and Salinity in Rhizophora mangle Propagules and Variation by Maternal Family and Population of Origin. Front. Mar. Sci. 8:756683. doi: 10.3389/fmars.2021.756683
Received: 10 August 2021; Accepted: 24 September 2021;
Published: 16 November 2021.
Edited by:
Lisa N. S. Shama, Alfred Wegener Institute, Helmholtz Centre for Polar and Marine Research (AWI), GermanyReviewed by:
Ketil Koop-Jakobsen, Alfred Wegener Institute, Helmholtz Centre for Polar and Marine Research (AWI), GermanyEmily Dangremond, Roosevelt University, United States
Copyright © 2021 Richards, Langanke, Mounger, Fox and Lewis. This is an open-access article distributed under the terms of the Creative Commons Attribution License (CC BY). The use, distribution or reproduction in other forums is permitted, provided the original author(s) and the copyright owner(s) are credited and that the original publication in this journal is cited, in accordance with accepted academic practice. No use, distribution or reproduction is permitted which does not comply with these terms.
*Correspondence: Christina L. Richards, Y2xyQHVzZi5lZHU=
†These authors share first authorship
‡These authors have contributed equally to this work and share senior authorship