- 1Marine Mammal and Turtle Division, SWFSC, National Marine Fisheries Service, La Jolla, CA, United States
- 2U. S. Marine Mammal Commission, Bethesda, MD, United States
- 3School of Aquatic and Fishery Sciences, University of Washington, Seattle, WA, United States
- 4Puget Sound Institute, University of Washington Tacoma, Tacoma, WA, United States
- 5Sea Mammal Research Unit, Scottish Oceans Institute, University of St Andrews, St Andrews, United Kingdom
- 6Office of Protected Resources, NOAA, National Marine Fisheries Service, Silver Spring, MD, United States
- 7Okapi Wildlife Associates, Hudson, QC, Canada
- 8Facultad de Ciencias, Universidad de Valparaíso, Valparaíso, Chile
- 9Pelagic Division, Marine and Freshwater Research Institute, Hafnarfjörður, Iceland
- 10Resource Assessment and Conservation Engineering Division, NOAA, National Marine Fisheries Service, Seattle, WA, United States
- 11Marine Mammal Laboratory, AFSC, NOAA Alaska Fisheries Science Center (AFSC), Seattle, WA, United States
- 12Oceans Initiative, Seattle, WA, United States
- 13Cooperative Institute for Climate, Ocean, and Ecosystem Studies (CICOES), University of Washington, Seattle, WA, United States
- 14Marine Ecology and Telemetry Research, Seabeck, WA, United States
Fisheries bycatch is the greatest current source of human-caused deaths of marine mammals worldwide, with severe impacts on the health and viability of many populations. Recent regulations enacted in the United States under the Fish and Fish Product Import Provisions of its Marine Mammal Protection Act require nations with fisheries exporting fish and fish products to the United States (hereafter, “export fisheries”) to have or establish marine mammal protection standards that are comparable in effectiveness to the standards for United States commercial fisheries. In many cases, this will require estimating marine mammal bycatch in those fisheries. Bycatch estimation is conceptually straightforward but can be difficult in practice, especially if resources (funding) are limiting or for fisheries consisting of many, small vessels with geographically-dispersed landing sites. This paper describes best practices for estimating bycatch mortality, which is an important ingredient of bycatch assessment and mitigation. We discuss a general bycatch estimator and how to obtain its requisite bycatch-rate and fisheries-effort data. Scientific observer programs provide the most robust bycatch estimates and consequently are discussed at length, including characteristics such as study design, data collection, statistical analysis, and common sources of estimation bias. We also discuss alternative approaches and data types, such as those based on self-reporting and electronic vessel-monitoring systems. This guide is intended to be useful to managers and scientists in countries having or establishing programs aimed at managing marine mammal bycatch, especially those conducting first-time assessments of fisheries impacts on marine mammal populations.
Introduction
Fisheries bycatch is the greatest current source of human-caused deaths of marine mammals worldwide (Lewison et al., 2004; Read et al., 2005; Avila et al., 2018). Bycatch occurs when species not targeted by fishers are incidentally and unintentionally hooked, entangled or entrapped by fishing gear (Hall et al., 2000). Most species of marine mammals—cetaceans, pinnipeds, sirenians and sea otters—are affected by bycatch (Reeves et al., 2013), with hundreds of thousands or perhaps millions of individuals killed annually (Read et al., 2006). Most bycatch occurs in gillnet fisheries (Read, 2008; Reeves et al., 2013), but there is notable bycatch in other types of gear as well, including but not limited to longlines, set nets, stow nets, seines, trawls, and pot or trap gear. Before the 1990s—prior to the enactment of key amendments to the United States Marine Mammal Protection Act (MMPA)—hundreds of thousands of dolphins were killed each year in Eastern Tropical Pacific tuna purse-seine fisheries alone (Hall, 1998). Fisheries-related mortality has been the dominant factor, or at least a major contributing factor, in causing population decline or preventing population recovery (e.g., from historical whaling and sealing impacts) of many marine mammal species. Examples of species highly affected by bycatch include the North Atlantic right whale (Eubalaena glacialis), Hector’s dolphin (Cephalorhynchus hectori), New Zealand sea lion (Phocarctos hookeri), the franciscana (Pontoporia blainvillei), the nearly-extinct vaquita (Phocoena sinus), and the extinct baiji (Lipotes vexillifer) (Wang et al., 2006; Slooten, 2007; Turvey et al., 2007; Chilvers, 2008; Secchi, 2010; Rolland et al., 2016; Taylor et al., 2017; Jaramillo-Legoretta et al., 2019; also see Brownell et al., 2019).
In 2016, the United States enacted regulations under the MMPA aimed at reducing marine mammal bycatch in international fisheries1. The regulations, stemming from the Fish and Fish Product Import Provisions of the MMPA (hereafter, “MMPA Import Provisions”)2, require fisheries exporting fish and fish products to the United States (hereafter, “export fisheries”) to have or establish marine mammal protection standards that are comparable in effectiveness to the standards for United States commercial fisheries. To continue exporting their products to the United States, nations must apply for and receive a “comparability finding” from the United States National Oceanic and Atmospheric Administration3. To achieve a comparability finding, the harvesting nation’s program regulating an export fishery must: (1) prohibit the intentional killing or serious injury4 of marine mammals in the fishery, and (2) conduct marine mammal stock (population) assessments that establish bycatch limits for those marine mammal populations interacting with export fisheries, estimating marine mammal bycatch in those fisheries, and taking measures if necessary to reduce total bycatch below the bycatch limits. Alternatively, harvesting nations may adopt other approaches, such as the implementation of bycatch mitigation measures, that are comparable in effectiveness to United States standards for export fisheries [Food and Agriculture Organization of the United Nations [FAO], 2021]. These comparability requirements are conceptually straightforward, but can be difficult to achieve in practice, especially for the most economically challenged countries (Williams et al., 2016). In the United States, the Guidelines for Assessing Marine Mammal Stocks [GAMMS; National Marine Fisheries Service [NMFS], 2016] provide guidance on the key assessment elements: estimating stock abundance, estimating bycatch mortality and serious injury, and comparing the latter to conservation reference points derived from the former. For example, in the United States, bycatch is compared to the conservation reference point call the Potential Biological Removal (PBR) level5, which is calculated from an estimate of the minimum population size and other parameters. PBR is defined conceptually in the MMPA and operationalized from a management strategy evaluation study by Wade (1998). More generally, comprehensive reviews of protected species reference point estimation and assessment frameworks have been conducted by Lonergan (2011), Moore et al. (2013), Curtis et al. (2015), and Moore and Curtis (2016).
The objective of this paper is to describe best practices for estimating bycatch mortality, which is a key ingredient for population or stock assessment, whereby the mortality estimates are compared to a conservation or limit reference point. Reference point estimation is tied to estimating population size, a topic thoroughly reviewed by Hammond et al. (2021), this issue. An in-depth description of the broader assessment framework is found in Wade et al. (2021), this issue. Readers should examine these papers to understand the broader management context within which bycatch estimation takes place under the MMPA Import Provisions and how estimating marine mammal bycatch and population size relate to each other.
There are important precursors to designing a program to estimate bycatch in a fishery (discussed more thoroughly by Wade et al., 2021). The first is making use of exploratory data (which may need to be collected anew) to characterize the fishery (number of vessels, vessel types, gears used, when and where fished, target species, etc.) and identify marine mammal populations that might interact with it. Our use of the term “fishery” is consistent with its usage under the United States List of Fisheries and List of Foreign Fisheries. That is, a fishery is characterized by a collection of fishers using similar methods (e.g., vessel and gear types), fishing for certain target species, operating in a certain place and time. Examples include the United States drift gillnet fishery for swordfish and thresher shark off the United States West Coast, or the Mexican demersal longline fishery for deepwater snappers in the Gulf of Mexico. Our use of marine mammal “population” is consistent with definitions provided by the GAMMS [National Marine Fisheries Service [NMFS], 2016], i.e., a group of interbreeding individuals that is more or less demographically independent from other groups. The United States marine mammal Stock Assessment Reports provide numerous examples of defined population “stocks” (e.g., Carretta et al., 2021). A marine mammal population may occur entirely within the geographic range of a fishery or the fishery and marine mammal population may only slightly overlap in space or time.
In the absence of data, inferences about the likelihood of bycatch occurrence can be made through exploring the spatial overlap of marine mammal populations and fishing gears known to catch or entangle marine mammals. If bycatch is known or expected to occur, and if negligible impacts to the population cannot be ruled out, then this points to the need to mitigate or undertake a formal bycatch estimation program, which is the focus of the remainder of this paper. Estimation should be prioritized for high-risk gears and fisheries that interact with marine mammal populations at particular risk (see risk categories in Box 1 in Wade et al., 2021, this issue). In the context of complying with MMPA Import Provisions, priority should be given to those fisheries categorized as “Export Fisheries” on NOAA’s List of Foreign Fisheries6. Once obtained, bycatch estimates can then be compared to conservation reference points that depend on the population’s size and growth rate to assess the likely or potential impacts of the fishery on the population’s viability and whether mitigation actions are needed.
We proceed by discussing a general bycatch estimator and how to obtain the bycatch-rate and fisheries-effort data needed to apply the estimator. Scientific observer programs provide the most robust source of information for estimation and should be used when possible. Observer programs are therefore discussed at length, including program-design considerations, data collection, statistical analysis, and common sources of estimation bias. In addition to their value for directly estimating bycatch, scientific observer programs can also be used to assess and improve compliance of required mitigation measures, estimating the efficacy of such measures (e.g., comparing bycatch rate estimates before and after mitigation, or in sectors of the fisheries with vs. without mitigation), and improve the quality of information provided by fishermen (Cox et al., 2007; Porter, 2010; Snyder and Erbaugh, 2020). Because scientific observer programs tend to be expensive and logistically difficult to implement, we also discuss alternative approaches and data types for making bycatch inferences, and the caveats associated with these. This document is intended to be useful to managers and scientists in harvesting nations maintaining or establishing regulatory programs aimed at reducing marine mammal bycatch, including for the purposes of achieving a comparability finding under the MMPA Import Provisions.
Bycatch Mortality Estimator
Bycatch mortality is the total number of animals that die (or are expected to die) in a fishery from interacting with fishing gear. Bycatch mortality is typically estimated annually for each gear-specific fishery affecting a defined population. Summing across all fisheries interacting with the population provides a total annual estimate for the population. A general point estimator of bycatch mortality for population i is:
where the expected bycatch mortality in year t, μit, is the product of animal abundance in the population (Nit), total fishing effort (Et), a scaling parameter referred to as catchability (cit) (which has the unit: bycatch N–1 effort–1, and can be thought of as the likelihood that a single animal in the population would be caught by a single unit of fishing effort), and the fraction of bycaught animals that are dead or expected to die (mit; the bycatch mortality rate, BMR), noting that for some types of gear, animals may be released or escape alive after being fatally injured. Nit and cit are correlated and in practice will often be difficult to estimate separately. For example, cit will lower if Nit is defined as the entire population (including potentially large numbers that never overlap with the fishery), whereas cit will be higher if Nit refers to just those animals in the area of the fishery, which may be difficult to estimate. Although there may be cases where cit is explicitly estimated (e.g., from a concurrent time series of abundance and bycatch data; Moore and Curtis, 2016), more typically the product Nit cit is estimated as a single parameter referred to as “bycatch per unit effort” (BPUE), or b, where
The sections below give details on how these terms may be estimated, and Figure 1 illustrates the associated decisions that must be made.
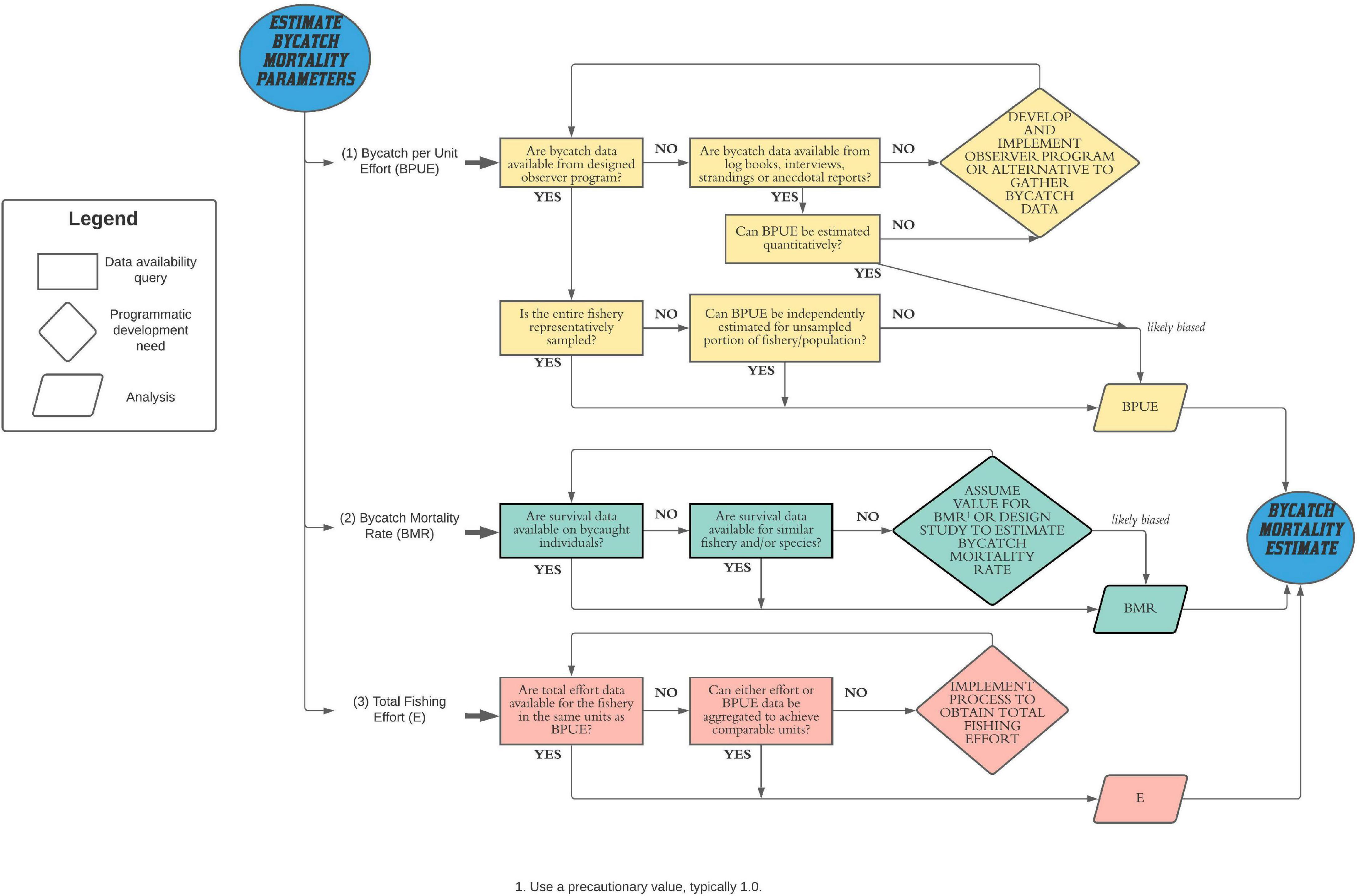
Figure 1. Decision tree for estimating the components of bycatch mortality: bycatch per unit effort (BPUE), bycatch mortality rate (BMR), and total fishery effort (E). For example, one sex or age class, or a portion of the fishing grounds, missed or incompletely covered by the sampling design.
Estimating b, Bycatch-Per-Unit-Of-Effort From Fisheries Observer Data
The most accurate way to estimate BPUE is with data from scientific fisheries observer programs, whereby a representative sample of fishing effort is directly observed by independent observers aboard fishing vessels, and the number of marine mammals bycaught (and killed or injured) is recorded. A simple point estimate for BPUE can be calculated as bycatch observed divided by effort observed. For example, if researchers observed 100 gillnet sets and two dolphins were captured, the BPUE would be two dolphins per 100 sets, or 0.02 dolphins per set. If instead of observing and recording sets, the researchers observed 10 complete fishing trips (which might last many days and include multiple sets and retrievals of one or multiple gear types) and counted six dolphins captured, then BPUE would be 0.6 dolphins per trip.
The fraction of a fishing fleet’s effort that is observed is referred to as the “observer coverage.” BPUE is more precisely estimated for populations in which animals are caught in greater numbers (because either N or c is higher), and in fisheries with higher observer coverage. Small populations for which bycatch is an infrequent or rare event pose particular bycatch 1estimation challenges (Martin et al., 2015; Gray and Kennelly, 2018; Wakefield et al., 2018) and require fairly high observer coverage levels to avoid severe biases due to small sample size. Curtis and Carretta (2020) developed the observer coverage calculator ObsCovgTools in R (R Core Team, 2019) that calculates coverage levels required to meet user-defined bycatch estimation objectives. Objectives include estimating bycatch to a desired precision level, estimating the probability of observing bycatch when it exists in a fishery, and providing an upper confidence limit for bycatch, even if no bycatch is observed. Outputs are conditioned on inputs, such as total effort in the fishery and expected BPUE and sampling variance, which can be obtained from a pilot study or borrowed from a similar study, or based on expert opinion. Under the MMPA, performance tests of the PBR control rule used for setting conservation reference points are based on bycatch estimation coefficients of variation (CVs) of 0.3 or better (Wade, 1998), so we suggest this as a reasonable default input for the target precision.
In addition to having adequate levels of observer coverage, statistically valid BPUE estimates demand the use of well-trained observers and an appropriate survey design. Designing an observer training program and prescribing field protocols (datasheets, etc.) are beyond the scope of this paper, but numerous resources address these topics in detail and should be consulted when designing an observer program [e.g., Pacific Islands Regional Office Observer Program [PIRO-OP], 2017; Northeast Fisheries Science Center [NEFSC], 2019]. As for survey design, the goal is to obtain observer data from a representative sample of the fishery with respect to the suite of attributes that characterize fishing effort, such as the geographic distribution of effort, temporal distribution on diurnal and seasonal timescales, vessel and gear characteristics, and types of effort (e.g., sets, hooks, etc.). For example, a gillnet fishery might have the following hypothetical characteristics: 30% of sets in July, 60% of sets in August, and 10% of sets in September; 30% of sets over the continental shelf and 70% of sets offshore; 50% of sets using long nets deployed from large vessels, and 50% of sets using shorter nets deployed from smaller vessels. Ideally, to avoid a biased estimate the observer dataset would have effort in roughly the same proportions; i.e., the observed component of the fishery would be a microcosm of the whole fishery. If this is not possible, stratification of the sampling and effort can potentially reduce problems associated with non-proportional sampling, as long as the important strata are identified and adequately sampled (see below under discussion of biases). Sampling does not need to be exactly proportional to the effort in each stratum, indeed strata sample sizes can be adjusted to the variance in BPUE in each stratum to increase the estimate’s precision without introducing significant bias.
The most statistically valid estimates typically are achieved by stratified random sampling, whereby the fishing effort is subdivided into relatively homogenous subgroups with respect to a particular variable (e.g., by area or season) (e.g., Liggins et al., 1997; Cotter and Pilling, 2007; Benoît and Allard, 2009). Precision is improved especially by sampling more intensively in strata where variance of the bycatch is higher (if this is known), for example if bycatch rates in 1 month tend to be more variable than bycatch rates in other months. This would be the recommended approach if there were sufficient knowledge of all the fishing vessels and their schedule of fishing deployments, and if all vessels could accommodate observers [e.g., bunk and deck space for the observer(s)]. In this scenario, one would randomly select a certain percentage of gear deployments ahead of time and place observers on the vessels expecting to make those deployments. But this is rarely practical in fisheries because of, among other things, uncertainties about who is fishing when and where, and the unwillingness (if observer program participation is voluntary) or inability to accommodate observers (see section “Sources of Bias in Bycatch Estimation”). Whatever the circumstances, the observer program must be diligent about obtaining a sample that accurately and precisely represents the fishery as well as possible (Benoît and Allard, 2009; Benoît et al., 2012; Mangi et al., 2015; Fernandes et al., 2021). It would also be beneficial to representatively sample in relation to spatial-temporal variation in animal density, although this information will often not be available. In situations where the effort is well characterized (e.g., how much fishing effort is occurring when, where, and how), but the observed fishing effort is extremely non-representative (e.g., zero or very small sample sizes in one or more strata), statistical approaches can be used in some cases to eliminate bias in bycatch estimates. Statistical approaches are discussed further below in the “Biases in Bycatch Estimation …” section.
Estimating m, Bycatch Mortality Rate
Observers typically document bycaught marine mammals as “dead” or “released/escaped alive,” often with an assessment of the type of gear interaction, observation of any gear remaining on the animal, and characterization of any injuries. Animals that escape or are released alive might be uninjured or, if injured, could die later or recover and survive. Thus, an unbiased estimate of bycatch mortality, the bycatch mortality rate (BMR), requires an estimate of the proportion of bycaught individuals that die, whether immediately or eventually (i.e., post-release mortality). In the United States, following a bycatch event and based on data collected at the time of detection and observation, the bycaught individual is categorized as “dead,” “seriously injured,” or “not seriously injured.” Those categories are based on guidelines developed through scientific analyses of data on injury severity and outcome, where “seriously injured” was defined as an animal having a greater than 50 percent chance of dying after release, and “not seriously injured” as the animal having a less than 50 percent chance (National Marine Fisheries Service [NMFS], 2012a, b).
Ideally, to determine the post-release mortality rate, bycaught individuals would be tagged prior to release and monitored afterward. Although this approach has been used for marine fish and sharks (e.g., Davis, 2002; Cadigan and Brattey, 2006; Campana et al., 2009; Carruthers et al., 2009; Patterson et al., 2014) and marine turtles (e.g., Álvarez de Quevedo et al., 2013; Stacy et al., 2016; Maxwell et al., 2018; Parga et al., 2020), it has not been employed with marine mammals. Punt et al. (2021) used a modeling approach to estimate post-release mortality rate of two pinniped species bycaught in Chilean purse seine and trawl fisheries. In practice, most bycatch mortality rate estimates are based on small data sets, categorical assignments (e.g., Andersen et al., 2008; Olaya-Ponzone et al., 2020), or expert assessments. The United States injury guidelines are based on either analyses of scarring data or subsequent observations documenting the condition, health, and fate of known individuals following the detection of injuries due to interactions with fishing gear (see case studies in Andersen et al., 2008). Only a small number of published studies provide estimates of BMR (e.g., Wells et al., 2008; Cassoff et al., 2011; Dolman and Moore, 2017; Pettis et al., 2017; Olaya-Ponzone et al., 2020).
It may not always be necessary to estimate BMR. For example, small cetaceans and pinnipeds caught in gillnets and some trawl fisheries are typically found dead. Conservatively, in the absence of data specific to a study population and fishery, it is prudent to set BMR to 1.0 for marine mammals captured in gillnet fisheries, as suffocating or drowning in the nets is by far the most likely outcome. For bycatch in other types of gear (e.g., purse seine, longline, some trawl), approximate values for BMR might be taken from the literature for similar species and gears, but in fact few such estimates are available.
Estimating E, Fishing Effort
General Principles for Estimating E
Scaling the observed-sample estimate of BPUE to an estimate of total bycatch in a fishery requires knowing the total amount of effort in the fishery (this is the sampling frame within which a subset of effort has been observed). Critically, the effort metric used for estimating BPUE and for characterizing the whole fishery must be the same. For example, if observers collect data for a random sample of fishing trips with an estimate of how many marine mammals on average are caught per trip, then the total number of trips made by the fleet must be quantified to properly extrapolate to the whole fishery. Similarly, if BPUE is quantified for a gillnet fishery by observing a random number of gillnet deployments (sets), then the total number of gillnet sets made by the fishery must also be known or estimated. In some observer programs, observers monitor all fishing activity over the course of a particular period (e.g., 24 h) and BPUE is measured as the number of bycatch events per effort-period (e.g., per-day); in this case, the number of effort-days (# boats x the # days each boat operates) would need to be known for the fleet. If the sampling frame is incomplete because the size and extent of the fleet has not been accurately determined, then the total bycatch mortality will be underestimated (i.e., negatively biased). Therefore, diligence is needed to identify all of the vessels operating in a particular fishery throughout its range.
Ideally, the units of fishing effort measured should be those most directly related to the amount of bycatch that occurs. For example, for a longline fishery, one might quantify the number of longline sets, or more coarsely, the number or total duration of longline fishing trips. However, the number of hooks on the line and their soak time (e.g., “hook-hours”) more closely relates to the likelihood of an animal being bycaught. This distinction is relatively unimportant if large numbers of longline sets or trips (i.e., effort units) are randomly sampled. However, if the size of the observed sample is small or the sampling is biased, then bycatch mortality may be more accurately estimated by measuring the number of bycatch events per hook-hour and scaling this to hook-hours in the fleet (rather than quantifying bycatch per longline trip and the number of trips). Effort recorded in finer units can always be re-scaled into coarser units as needed, whereas data recorded in coarse units cannot be more finely resolved. Of course, there are trade-offs to how finely one measures effort. Obtaining fleet-wide information about the number of vessels and trips is easier and less costly than monitoring the number of hook-hours, for example. In addition, coarser units tend to be more statistically independent. For example, observations of bycatch-per-trip are more likely to be statistically independent than observations of bycatch-per-set, since set data will be correlated in time and space within the same trips. Observations at coarser scales thus tend to give more valid estimates of precision unless autocorrelations in hierarchical or nested datasets are properly taken into account.
Estimating Fishing Effort in Practice
Measures of fishing effort vary greatly, as do the methods for quantifying those measures. McCluskey and Lewison (2008) reviewed the types of effort measures available for different types of fishing fleets around the world, including artisanal or small-scale and industrial fleets (as well as recreational and IUU7 fleets, not discussed further here). Though not well-defined (Tietze, 2016; Smith and Basurto, 2019), our use of “small-scale” refers to fleets that tend toward having lower capital or technological investment, being operated at the household/family level (rather than by companies), and having smaller vessel sizes. In the extreme, these fleets can consist of thousands of such vessels dispersed across vast geographic areas. Effort in small-scale fleets is usually poorly documented and rarely quantified, due to factors such as lack of awareness, funds and infrastructure, and institutional capacity. Interviews with a large, representative sample of small-scale fishers may be the most practical way to get useful estimates of effort (e.g., Gómez-Muñoz, 1990; Moore et al., 2010), and often measures of fishing-effort will necessarily be crude. For example, Lewison and Moore (2012), working with Nigerian colleagues, identified the number of fishing villages in each of three Nigerian states. For each state, they randomly sampled the villages, counted the number of fishing vessels on the beach in these villages and interviewed fishers there (stratified by boat or gear type) to obtain information about fishing methods, gears, seasonality, fish catch, and bycatch of marine mammals and sea turtles. The fishing effort metric was the average number of boats per village, multiplied by the number of fishing villages along the entire coast to estimate the number of boats per state. BPUE, also obtained from the interview data, was quantified in terms of animals caught per vessel per year. Rough total bycatch estimates were derived as catch per vessel (per year) multiplied by the number of active vessels in the state.
For industrial fisheries, a greater variety of methods for quantifying fishing effort data are generally available. In addition to interview approaches, industrial fleets are more amenable to implementing observer programs. Fleet-wide effort can be quantified through complete dockside monitoring when all vessels return to one central port or a few main ports, or using logbook data, whereby data are recorded on when, where, how and how much they fish (e.g., Roman et al., 2014). Collecting spatially and temporally explicit information about fishing effort (e.g., through logbook data) is extremely valuable, especially if total fishing effort (or BPUE) needs to be modeled rather than estimated using design-based approaches (McCluskey and Lewison, 2008). Inaccuracy is a potential pitfall of both interview-based and logbook data due to response bias (e.g., Cosgrove et al., 2016; Northridge et al., 2017; Luck et al., 2020).
The most accurate data on fishing effort are obtained from electronic logbooks that provide spatial and temporal fishing effort data, such as via a vessel monitoring system (VMS) in which data are uploaded via satellite on a regular schedule. A challenge to this approach is the resistance commonly shown by fishers to being monitored. Nevertheless, the availability of VMS and Automatic Identification Systems (AIS) data had led to recent advances in the ability to make inferences about fishing activity (effort levels and distribution) using computer science algorithms such as Global Fishing Watch8 (e.g., Kroodsma et al., 2018).
In the absence of data to estimate effort directly, effort can sometimes be predicted or inferred from other characteristics of the fishery using models (e.g., McCluskey and Lewison, 2008; Greenstreet et al., 2009; Soykan et al., 2014; Johnson et al., 2017; Adibi et al., 2020), although their accuracy may be difficult to validate and may rely on unrealistic or unsupported assumptions or inaccurate information. For example, fish catch (landings) has been used as a proxy for fishing effort, either directly or through models, but landings data themselves are often inaccurate (e.g., Batista et al., 2015; Pauly and Zeller, 2016).
Estimating μ, Total Mortality, and Its Uncertainty
Given (1) BPUE (b) and mortality (m) estimates obtained from an unbiased sample of observer data from a fishery and (2) an estimate of that fishery’s total fishing effort (E) in comparable units, the simplest and most common estimator for total bycatch is a ratio estimator, whereby b*m is multiplied by E. Equivalently, if e is the amount of effort observed, so that observer coverage P = e/E is the proportion of the fleet observed, then the bycatch estimator can also be expressed as the bycatch mortality in the observed sample divided by P (Julian and Beeson, 1998; Carretta et al., 2004). For example, if 100 effort units out of 1,000 in the fleet are observed (P = 0.1), then estimated total bycatch mortality, μ = b × m × 1,000 = observed bycatch mortality/0.1. Variance in this estimate is commonly calculated using resampling (e.g., bootstrapping) or delta methods (e.g., Zhou, 2002; Manly, 2011; Cruz et al., 2018). An advantage of bootstrapping is that it facilitates the accounting of variance on appropriate (independent) observational units. Often the independent sampling unit in an observer program is the fishing trip (e.g., it might be possible, given a rough schedule of fishing trips, to sample these randomly) whereas the multiple gear deployments observed within that trip are correlated (occurring in similar time and space and with similar methods). Treating each day or gear deployment as the observational unit would likely over-estimate the precision (underestimate the variance) of the estimates, whereas resampling fishing trips in the bootstrap analysis provides a valid variance estimate. Precision of the bycatch estimate is typically reported using coefficients of variation (CVs), along with other standard precision measures, such as 95% confidence intervals. As noted above, in the United States, performance tests of the PBR framework are based on the assumption that the CV for bycatch in an individual year 0.3 or less.
The above “design-based” methods for estimating bycatch and bycatch mortality assume that bycatch in the observed portion of the fishery can be extrapolated to the whole fishery, because the study is designed in a representative way. In many situations, bycatch is better estimated using a model-based approach, rather than simple ratio estimators. Examples include when the sample data are biased (not collected using a random or other representative sampling scheme), when multiple years of data have been collected and inferences about current bycatch levels can be informed by data from prior years, when multi-year datasets include years when no bycatch was observed (CVs cannot be calculated for these years using a simple ratio estimator), or when one desires to make probabilistic or predictive inferences about the likelihood of bycatch mortality exceeding a bycatch-limit reference point (e.g., in the current or a future year; Martin et al., 2015; Cruz et al., 2018; Carretta et al., 2019; Stock et al., 2019). Model-based approaches are discussed further in the next section.
Biases in Bycatch Estimation—Sources and Solutions
Non-representative Sampling
Biased sampling (e.g., extreme over- or under-sampling the fishing fleet with respect to characteristics such as area, season, gear, or vessel type) should be avoided if possible, but if the total fishing effort is well characterized, then stratifying the sample of observer data can help address some biases. For example, if a fishery operates over a 3-month period, with most effort occurring in the second month, but most of the observed effort comes from the first month, then bycatch (and variance) can be estimated separately for each month (stratum) and the stratum estimates combined to obtain the total bycatch mortality. However, it is important in this scenario that sampling within each stratum is largely representative of the fishing occurring within the stratum. Ideally, stratification should be built into the study design, to ensure sufficient representativeness and to ensure the adequacy of within-stratum sample sizes. “Post hoc stratification” may not overcome severe design biases, such as when sample sizes are very small or absent within some strata, or when sampling biases exist across multiple attributes of the fishery (e.g., sampling in the third month under-represented an important fishing area).
Model-based approaches can be useful when sampling biases cannot be remedied by stratification, and in some other contexts. Model-based estimators use statistical relationships between potential explanatory variables (e.g., properties of a fishing deployment in a certain time and place) and a response variable (e.g., bycatch mortality) to make predictions about bycatch mortality in the unobserved component of the fishery. If the sample data capture the range of variation in the important explanatory variables, then these relationships can be described (modeled) and used to predict bycatch throughout the fishery provided that the covariate values are known for all the fishing effort (e.g., from fishery logbooks). For example, Carretta et al. (2019) used a random-forest machine learning approach to estimate marine mammal, sea turtle and seabird bycatch in the California drift-gillnet fishery based on quantified relationships between observed bycatch and a suite of fishing-set characteristics (location, diurnal and seasonal time variables, bathymetry, oceanography, gear characteristics, etc.) (also see Stock et al., 2019 for a random forest example). Authier et al. (2021, this issue) showed how regularized multilevel regression with post-stratification could be used to estimate bycatch from non-representative sampling. Another common framework for estimating bycatch mortality using covariate data is generalized linear or generalized additive modeling (GLMs or GAMs), which can be implemented in a frequentist (Orphanides, 2009; Cruz et al., 2018; Stock et al., 2019) or Bayesian estimation framework (e.g., Martin et al., 2015; Moore and Curtis, 2016). Models can be particularly useful when multiple years of data exist, allowing information-rich years to inform bycatch estimates in more data-limited years, to resolve the problem of unestimable CVs in years when no bycatch is observed, and to evaluate longitudinal relationships to bycatch mortality such as a change in management actions (e.g., Carretta et al., 2019). Bayesian methods in particular are useful for obtaining probabilistic inferences, such as the probability that the bycatch rate has changed in response to a management action or that bycatch mortality exceeds a limit or other threshold (Martin et al., 2015; Moore and Curtis, 2016).
Taking a model-based approach may be the only option for obtaining valid estimates of bycatch if a sampling design is non-representative. Importantly, however, a model-based design cannot always provide unbiased estimation if the survey design is poor. In particular, if important covariates are not adequately sampled across their range of variation, or if many observations are not statistically independent, then the covariate relationships can be incorrect. As described earlier, there is no good substitute for a well-designed survey and fishery observer program.
Inaccurate Counts by Observers
Bycatch mortality estimates can be biased due to inaccurate counts of observed bycatch events (typically undercounts). Undercounts occur when observers are unable to record every bycatch event that occurs during a watch period. Observers may be engaged in other data collection tasks and not detect bycaught individuals, particularly those not brought on deck. The number of bycaught animals recorded by an observer can be less than the number that were actually bycaught because marine mammals caught on hooks can “drop-off,” or those entangled in nets can “drop-out,” at any time during the fishing or retrieval of the gear (e.g., Hamer et al., 2011). These problems can be exacerbated if the crew inadvertently or deliberately fail to inform the observer of the presence of bycaught individuals, or surreptitiously release or shake an animal out, or off, the gear. This source of bias can be minimized by assuring the cooperation of crews, although in practice it cannot be eliminated because it is very difficult to estimate the frequency of drop-offs and drop-outs that occur out of sight from the vessel.
Deployment Effects
“Deployment effects” refer to factors that make it logistically infeasible to carry out the planned sampling design, forcing non-representative sampling of the fishery (Benoît and Allard, 2009; Faunce and Barbeaux, 2011; Cahalan and Faunce, 2020; Fernandes et al., 2021). These factors (Table 1) include unequal ability to observe different vessel types in the fishery, non-participation in the observer program by fishers, inability to observe the fleet operating in certain locations and periods, sub-optimal allocation of observer effort due to incomplete knowledge of the fleet, and other logistical restraints (Table 1).
Observer Effects
“Observer effects” occur when fishers use different gear or fishing methods, target different fish species, fish in different areas or at different times, reduce effort per trip, or handle bycatch differently when observers are on board (Liggins et al., 1997; Benoît and Allard, 2009; Faunce and Barbeaux, 2011), presumably to reduce the chance of bycatch occurring (or being detected and reported). Observer effects result in observer data that are not representative of the entire fleet and may not accurately reflect the bycatch that occurred on the observed trips. Subsequently, bycatch mortality estimates are biased and likely more precise than is warranted (Cotter and Pilling, 2007). Observer effects and the resulting “observer biases” are difficult to confirm [National Marine Fisheries Service [NMFS], 2011]. In some studies, observer effects have been inferred based on catch statistics that differed significantly between observed and unobserved portions of a fleet (e.g., Wahlen and Smith, 1985; Walsh et al., 2002; Cotter and Pilling, 2007; Burns and Kerr, 2008; Faunce and Barbeaux, 2011; Kirkwood et al., 2020); some other studies failed to find such differences (e.g., Liggins et al., 1997). It is widely assumed that observer effects are common (e.g., Faunce and Barbeaux, 2011), especially when (i) captains and/or crew believe that observer data can be used against them (e.g., have enforcement consequences or lead to disadvantageous management changes) [National Marine Fisheries Service [NMFS], 2011], that an onboard observer requires additional effort on their part, or that having an observer on board constrains their behavior in some way (Cotter and Pilling, 2007), or (ii) fishers believe that greater profits can be made without observers on board (Furlong and Martin, 2000). One way to address this challenge is for managers to identify and provide incentives for fishers to cooperate, thereby helping to ensure the safety of observers and the integrity of their data. Potential incentives might include financial compensation, increased quota allocation, access to closed areas or seasons, permit fee relief, or access to restricted access fisheries.
If significant observer effects have been documented or are suspected, the problem can be handled in several ways, although the best approach is to address potential biases in the initial design of the observer program (Benoît and Allard, 2009). Theoretically, bycatch mortality estimates could be corrected if there is an estimate of the bias introduced by the observer effects, although such an estimate is rarely if ever available (Punt, 1999). Bias can be reduced by increasing observer coverage or deploying electronic monitoring devices on the unobserved portion of the fleet, although the latter may introduce its own sources of error (see “Electronic vessel monitoring as an alternative to observer programs” below) and may not be economically feasible. The bias should decrease to zero as observer coverage increases to 100%, although there is still the potential for bias due to unrepresentative sampling within trips or to fishers influencing the ability of observers to conduct their duties as required by the observer program (Benoît and Allard, 2009). Finally, a fishery can be stratified such that unbiased bycatch estimates are obtained from the observable vessels, thus confining the problems and bias to just a portion of the fishery, which can be subject to targeted monitoring to account for the under-representation (Furlong and Martin, 2000; Benoît and Allard, 2009).
Cryptic Bycatch Mortality
In general, “cryptic mortality” refers to human-caused mortality that is not, or cannot be, observed. Bycatch should be estimated across all fisheries for a given marine mammal population. However, it is relatively uncommon that all fisheries are observed, and IUU fisheries are, of course, unobserved. Cryptic deaths and injuries can (1) occur in observed fisheries when deaths and injuries are not detected by observers (e.g., drop-offs and drop-outs), (2) go undetected because some fisheries are not observed, or (3) result from “ghost-fishing” (Gilman et al., 2013). Several methods have been developed to estimate the magnitude of overall cryptic mortality (e.g., Williams et al., 2011; Peltier et al., 2012; Barbieri et al., 2013; Gilman et al., 2013; Prado et al., 2013; Wells et al., 2015; Carretta et al., 2016), from which it may be possible to estimate cryptic bycatch mortality. The most common approach estimates the recovery rate of carcasses as the ratio of the number of known deaths due to all causes (obtained, for example, from stranding data) to the estimated total number of deaths in the population (e.g., from a population model). The product of the inverse of the recovery rate and the number of known deaths due to fisheries interactions, excluding those documented by observers, provides an estimate of the undetected (i.e., cryptic) fisheries related mortality. This approach depends strongly on the assumption that the detection rate of deaths due to fisheries interactions is not different from the overall detection rate. Cryptic mortality from all sources, not just bycatch in fisheries, has been estimated to be one-half to two-thirds, and in extreme cases up to and exceeding 90%, of total mortality for marine mammal populations (see references above).
Alternative Approaches for Inferring Bycatch Impacts
Designing and implementing a new observer program is challenging conceptually, logistically and financially [National Marine Fisheries Service [NMFS], 2011]. For any given level of observer coverage, logistics will often increase in complexity with the size of the fishing area and the fleet, and the number of observers deployed [National Marine Fisheries Service [NMFS], 2011]. Observers need to be well trained and competent in several skill areas, including species identification, collecting scientific measurements and samples, and data security, which can require substantial funding [National Marine Fisheries Service [NMFS], 2011]. Complete (100%) observer coverage, while undeniably providing the best bycatch mortality estimates, is the most expensive and difficult option. Gains in precision diminish as observer coverage approaches 100%. The cost per unit increase in precision increases as observer coverage increases [National Marine Fisheries Service [NMFS], 2011], forcing an “optimal” observer coverage level typically much less than 100%.
While observer programs are generally regarded as the most accurate approach for estimating bycatch, some less-than-ideal alternatives exist that, under favorable circumstances and if implemented well, can provide information to support cruder assessments of marine mammal bycatch in a fishery.
Self-Reporting (Logbooks or Interview Data) as an Alternative to Observer Programs
Vessel logbook data and data collected through “dock-side” interviews, in addition to providing information about fishing effort, can provide information about marine mammal bycatch. Bycatch data collected by these methods are generally incomplete and inaccurate, usually in the direction of under-reporting (e.g., Walsh et al., 2002; Emery et al., 2019; see Mangi et al., 2016 for discussion of the efficacy of self-reporting). It is widely assumed that logbook data are incomplete and inaccurate because fishers are not skilled at collecting fisheries data (e.g., Faunce, 2011; Faunce and Barbeaux, 2011; Sampson, 2011; Mangi et al., 2016), or that they withhold information they believe could have negative consequences for them. Gilman et al. (2019) suggested that fishers “may have an economic or regulatory disincentive to record accurate data.” This problem may be less severe where there are strict legal requirements to report bycatch in logbooks, with surveillance, enforcement and punishments in place. Indeed, the use of electronic monitoring (see below) has been shown to improve the quality of logbook data (Emery et al., 2019). If logbook reporting can be assumed to be consistent throughout the fishery, then such data can be useful for extrapolating/estimating from more reliable data (observer program) that are limited in time and/or space.
Many interview-based assessments have been conducted to obtain semi-quantitative or qualitative information for characterizing fisheries in terms of describing gears and vessel-types, spatial or temporal patterns of fishing effort, and interactions with target and bycatch species, and for doing risk mapping, spatial planning and understanding socio-economic drivers of fisheries management issues (e.g., Moore et al., 2010; Liu et al., 2016; Whitty, 2016; Pilcher et al., 2017; Braulik et al., 2018). An advantage of using interview-based approaches to quantify bycatch mortality is the relatively low cost and relative logistical ease of talking with fishers compared to implementing an observer program. However, as is the case for logbook data, interview data are likely to provide biased inference if fishers are not forthcoming and honest (e.g., for fear of regulations that will limit their fishing opportunities), and interview responses are prone to memory error and interviewer effects. Conducting interviews is itself an art that requires skill and training (e.g., Moore et al., 2010; Lewison and Moore, 2012).
Nevertheless, there can be circumstances where self-reporting from logbooks or interviews provides useful information, at the very least providing information on minimum bycatch levels and on when and where at least some bycatch is occurring, in which gear types, and for which species (although accurate species identification can also be a problem with logbook and interview data, as fishers are unlikely to have been trained in species identification). Information from self-reporting can be useful for determining whether an observer program is needed, and, if it is, for guiding initial planning (e.g., prioritizing which fisheries or areas to observe first).
Electronic Vessel Monitoring as an Alternative to Observer Programs
An alternative to using fisheries observers is electronic monitoring using various technologies, such as GPS or AIS, video cameras, and gear sensors, that capture information on fishing location, catch, bycatch, and discards. Electronic monitoring systems can be used to monitor compliance with catch retention requirements or bycatch of protected species. Systems are now available that can monitor fishing activities on a vessel, and they are starting to supplement data collected by observers or to obtain data from previously unmonitored fisheries (Gilman et al., 2019). These systems integrate GPS units, hard disks, gear sensors and video cameras that provide a visual record of what was caught when and where, including bycatch (Mangi et al., 2015; van Helmond et al., 2020). Gear sensors can improve the efficiency of data collection and storage. For example, a reel sensor can determine when a longline is being retrieved and turn the system on only at those times. van Helmond et al. (2020) reviewed 100 pilot studies and 12 operational implementations, as of 2018, to monitor catch from around the world. As electronic monitoring systems are in the early stages of development and use, it is not yet clear how effective they will be at detecting and accurately recording data on marine mammal bycatch. Nonetheless, a number of systems deployed to monitor protected-species bycatch have reported marine mammal or seabird bycatch (McElderry et al., 2007; Evans and Molony, 2011; Kindt-Larsen et al., 2012; Bartholomew et al., 2018; Emery et al., 2019; Glemarec et al., 2020; van Helmond et al., 2020).
While these systems may collect data on numbers of species with relatively high precision, they cannot yet match observers in many tasks (e.g., species identification, measuring and weighing, sample collection) (Gilman et al., 2020). On the other hand, electronic monitoring systems can collect some data that observers cannot necessarily collect consistently (e.g., precise time and location of individual events, nature of handling and disposition of animals), and they can collect data on 100% of the effort during a fishing trip; observers miss some effort when they are off duty or ill, or weather prevents them from being on deck. Important costs associated with electronic monitoring systems include the often substantial time and funding needed to review and analyze the video streams, although advances in machine learning software hold promise for addressing this issue, and the need for video storage, which can be expensive (Margolis and Alger, 2020).
Several authors have identified strengths and weaknesses of electronic monitoring systems and compared the technology to traditional methods (e.g., Mangi et al., 2015; Suuronen and Gilman, 2020; Table 2). Because these systems can be “on” all the time, or started and stopped remotely or automatically based on sensor input, the fishers do not know when the system is collecting data. Further, the data are likely to be subsampled later, which also prevents fishers from knowing when they are being monitored. Therefore, the use of these systems could eliminate an observer effect, or discourage fishers from attempting to influence the data collected by an observer, when electronic monitoring is used to supplement observer data. Further, a sampling design applied to the recorded data could be completely representative and would not suffer from a deployment effect. Electronic monitoring can create a record that, for the duration that it is stored, can be revisited to verify information or resampled to address new questions, although most current applications retain raw data only for finite periods because of high data storage costs and infrastructure requirements.
Impediments to deploying and implementing electronic monitoring include resistance from fishers out of concern about the upfront cost, difficulties of installation, especially on small vessels, and privacy issues (McElderry et al., 2007; Mangi et al., 2015). Fishers may consider electronic monitoring an intrusion into their private workspace (Plet-Hansen et al., 2017) and may argue that camera surveillance reflects a governmental mistrust against them (Mangi et al., 2015). There are also concerns that some bycaught marine mammals may not be brought close enough to the vessel to be seen on camera, and regarding the capability of the video cameras to record sufficient detail to confirm the species identification of marine mammals in the water alongside the vessel and determine the extent of their injuries, particularly at night.
What Can Be Inferred Without Bycatch Monitoring Data?
There is no substitute for bycatch monitoring, but in the complete absence of a bycatch data collection system, there are indirect ways to infer whether bycatch is occurring and whether the impacts are likely to be trivial or worse. For example, beach-stranded and at-sea carcasses can provide information on interactions with fisheries and be used to help determine the need for an observer program. In the United States for example, stranding-network volunteers document human-caused injuries and deaths (e.g., as evidenced by vessel strikes, gunshot wounds, hooks, line, or net, or knife marks), and the data from strandings are used in marine mammal stock assessments [National Marine Fisheries Service [NMFS], 2016]. Often, carcasses bear clear evidence of a fishery interaction, although it is often not possible to link each case to a specific fishery or type of fishing activity. Stranding data can rarely be used to estimate bycatch mortality directly, but in some cases, models applied to stranding data have been used to infer estimates of the proportion of carcasses likely to strand ashore or minimum bycatch levels (e.g., Moore and Read, 2008; Williams et al., 2011; Carretta et al., 2016; Peltier et al., 2016, 2020). For pinnipeds, animals at rookeries can show direct evidence of entanglement; Page et al., 2004 used such data to calculate minimum entanglement mortality estimates. If a minimum estimate itself approaches or exceeds a bycatch-limit reference point (e.g., PBR) that may be sufficient to conclude that a management problem exists that needs to be addressed through an active effort to collect bycatch data more directly to inform mitigation.
It is widely understood that certain gear types represent a predictable threat to particular groups of marine mammals (Wade et al., 2021). For example, vertical buoy lines used to mark and retrieve fixed gear such as crab, lobster or fish traps have the potential to entangle large whales, and to result in their serious injury and death, but may not be a threat to smaller species. In contrast, gill nets are a serious threat to most marine mammals, including porpoises, dolphins and pinnipeds, as well as whales and sirenians. Similar to buoy lines, trawls, seines and longlines can be significant threats to particular marine mammals. Careful comparison by experts of the characteristics of an unobserved fishery with those of similar fisheries with known bycatch rates, combined with consideration of the extent of spatial-temporal overlap between the fishery and the distribution of marine mammal populations, can be used to make qualitative inferences about the likelihood of a population-level problem. Inferences of any kind can be made stronger by drawing upon multiple lines of information.
Concluding Remarks
This paper is especially intended for fisheries managers and researchers attempting to conduct first-time assessments of fisheries impacts on marine mammal populations. We have tried to break down the daunting challenge of estimating bycatch mortality, highlighting key central concepts, best practices, and typical impediments to obtaining good estimates. Bycatch estimates need to be compared to conservation reference points, which are derived for marine mammal populations mainly from estimates of population size. Population size and reference point estimation are not covered here, but we have provided references on these topics, and a more complete treatment of estimating abundance and reference points can be found in Hammond et al. (2021) and Wade et al. (2021), respectively, in this issue. Scientific observer programs are the only known way to obtain the data needed to estimate bycatch accurately. We therefore place considerable emphasis on this topic and hope the principles discussed in this paper will be useful for those developing fledgling observer programs. Importantly, the main principles—e.g., estimators and measurement units, survey design and statistical considerations, sources of bias—should be useful for the application of alternative bycatch estimation approaches (e.g., using logbooks, interviews) to the extent that these can be incorporated. Alternatives to observer programs have the key advantage of cost-effectiveness. If done well, they can provide useful information for the assessment process and in some cases may be sufficient for determining whether bycatch mitigation is required.
Author Contributions
JM and DH designed and wrote the manuscript. TF helped design and produced the figures. All co-authors developed the concept, contributed to writing and editing, and approved its publication. All authors contributed to the article and approved the submitted version.
Author Disclaimer
The views expressed are those of the authors and do not necessarily reflect the views of the Lenfest Ocean Program or the Pew Charitable Trusts.
Funding
Support for this project was provided by the Lenfest Ocean Program (Contract ID: #31008).
Conflict of Interest
The authors declare that the research was conducted in the absence of any commercial or financial relationships that could be construed as a potential conflict of interest.
Publisher’s Note
All claims expressed in this article are solely those of the authors and do not necessarily represent those of their affiliated organizations, or those of the publisher, the editors and the reviewers. Any product that may be evaluated in this article, or claim that may be made by its manufacturer, is not guaranteed or endorsed by the publisher.
Acknowledgments
The authors would like to thank Jim Carretta for reviewing and improving the manuscript.
Footnotes
- ^ United States Federal Register: 81 FR 54389.
- ^ United States Federal Code: 16 U.S.C. 1371(a)(2).
- ^ https://media.fisheries.noaa.gov/dam-migration/mmpa_import_rule_compliance_guide_april_2019_eng_508.pdf
- ^ The term “serious injury” is defined in United States regulations as “any injury that will likely result in mortality” (50 CFR 229.2), and by policy directive (https://media.fisheries.noaa.gov/dam-migration/02-238.pdf) “likely” is defined as “more likely than not” (i.e., greater than a probability of 0.5).
- ^ The MMPA defines PBR as the “maximum number of animals, not including natural mortalities, that may be removed from a marine mammal stock [population] while allowing that stock to reach or maintain its optimum sustainable population.” PBR is calculated as the product of the minimum population estimate of the stock (Nmin), one-half the maximum, theoretical or estimated net productivity rate of the stock at a small population (Rmax), and a recovery factor (Fr) that ranges from 0.1 to 1.0.
- ^ https://www.fisheries.noaa.gov/foreign/international-affairs/list-foreign-fisheries
- ^ Illegal, unreported and unregulated.
- ^ globalfishingwatch.org
References
Adibi, P., Pranovi, F., Raffaetà, A., Russo, E., Silvestri, C., Simeoni, M., et al. (2020). “Predicting fishing effort and catch using semantic trajectories and machine learning,” in Multiple-Aspect Analysis of Semantic Trajectories. MASTER 2019. Lecture Notes in Computer Science, vol 11889, eds K. Tserpes, C. Renso, and S. Matwin (Cham: Springer), 83–99. doi: 10.1007/978-3-030-38081-6_7
Álvarez de Quevedo, I., San Félix, M., and Cardona, L. (2013). Mortality rates in by-caught loggerhead turtle Caretta caretta in the Mediterranean Sea and implications for the Atlantic populations. Mar. Ecol. Prog. Ser. 489, 225–234. doi: 10.3354/meps10411
Andersen, M. S., Forney, K. A., Cole, T. V. N., Eagle, T., Angliss, R., Long, K., et al. (2008). Differentiating serious and non-serious injury of marine mammals: Report Of The Serious Injury Technical Workshop, 10-13 September 2007, Seattle, Washington. Washington: NOAA, 94. https://repository.library.noaa.gov/view/noaa/4389.
Authier, M., Rouby, E., and MacLeod, K. (2021). Estimating cetacean bycatch from non-representative samples (I): a simulation study with regularized multilevel regression and post-stratification. Front. Mar. Sci. 8:719956. doi: 10.3389/fmars.2021.719956
Avila, I. C., Kaschner, K., and Dormann, C. F. (2018). Current global risks to marine mammals: taking stock of the threats. Biol. Conserv. 221, 44–58. doi: 10.1016/j.biocon.2018.02.021
Barbieri, M. M., Raverty, S., Hanson, M. B., Venn-Watson, S., Ford, J. K. B., and Gaydos, J. K. (2013). Spatial and temporal analysis of killer whale (Orcinus orca) strandings in the North Pacific Ocean and the benefits of a coordinated stranding response protocol. Mar. Mamm. Sci. 29, E448–E462. doi: 10.1111/mms.12044
Bartholomew, D. C., Mangel, J. C., Alfaro-Shigueto, J., Pingo, S., Jimenez, A., and Godley, B. J. (2018). Remote electronic monitoring as a potential alternative to on-board observers in small-scale fisheries. Biol. Conserv. 219, 35–45. doi: 10.1016/j.biocon.2018.01.003
Batista, M. I., Horta e Costa, B., Gonçalves, L., Henriques, M., Erzini, K., Caselle, J. E., et al. (2015). Assessment of catches, landings and fishing effort as useful tools for MPA management. Fish. Res. 172, 197–208. doi: 10.1016/j.fishres.2015.07.020
Benoît, H. P., and Allard, J. (2009). Can the data from at-sea observer surveys be used to make general inferences about catch composition and discards? Can. J. Fish. Aquat. Sci. 66, 2025–2039. doi: 10.1139/F09-116
Benoît, H. P., Hurlburt, T., Chassé, J., and Jonsen, I. D. (2012). Estimating fishery-scale rates of discard mortality using conditional reasoning. Fish. Res. 125-126, 318–330. doi: 10.1016/j.fishres.2011.12.004
Braulik, G. T., Kasuga, M., Wittich, A., Kiszka, J. J., MacCaulay, J., Gillespie, D., et al. (2018). Cetacean rapid assessment: an approach to fill knowledge gaps and target conservation across large data deficient areas. Aquat. Conserv. 28, 216–230. doi: 10.1002/aqc.2833
Brownell, R. L. Jr., Reeves, R. R., Read, A. J., Smith, B. D., Thomas, P. O., Ralls, K., et al. (2019). Bycatch in gillnet fisheries threatens Critically Endangered small cetaceans and other aquatic megafauna. Endanger. Species Res. 40, 285–296. doi: 10.3354/esr00994
Burns, R. J., and Kerr, G. N. (2008). Observer effect on fisher bycatch reports in the New Zealand ling (Genypterus blacodes) bottom longlining fishery. N. Z. J. Mar. Freshw. Res. 42, 23–32. doi: 10.1080/00288330809509933
Cadigan, N. G., and Brattey, J. (2006). Reporting and shedding rate estimates from tag recovery experiments on Atlantic cod (Gadus morhua) in coastal Newfoundland. Can. J. Fish. Aquat. Sci. 63, 1944–1958. doi: 10.1139/f06-096
Cahalan, J., and Faunce, C. (2020). Development and implementation of a fully randomized sampling design for a fishery monitoring program. Fish. Bull. 118, 87–99. doi: 10.7755/FB.118.1.8
Campana, S. E., Joyce, W., and Manning, M. J. (2009). Bycatch and discard mortality in commercially caught blue sharks Prionace glauca assessed using archival satellite pop-up tags. Mar. Ecol. Prog. Ser. 387, 241–253. doi: 10.3354/meps08109
Carretta, J. V., Danil, K., Chivers, S. J., Weller, D. W., Janiger, D. S., Berman-Kowalewski, M., et al. (2016). Recovery rates of bottlenose dolphin (Tursiops truncatus) carcasses estimated from stranding and survival rate data. Mar. Mamm. Sci. 32, 349–362. doi: 10.1111/mms.12264
Carretta, J. V., Moore, J. E., and Forney, K. A. (2019). Estimates of Marine Mammals, Sea Turtle, and Seabird Bycatch from the California Large-Mesh Drift Gillnet Fishery: 1990-2017. Washington: NOAA, 76.
Carretta, J. V., Oleson, E. M., Forney, K. A., Muto, M. M., Weller, D. W., Lang, A. R., et al. (2021). U.S. Pacific marine mammal stock assessments: 2020. Washington: NOAA, 394.
Carretta, J. V., Price, T., Petersen, D., and Read, R. (2004). Estimates of marine mammal, sea turtle, and seabird mortality in the California Drift Gillnet Fishery for swordfish and thresher shark, 1996-2002. Mar. Fish. Rev. 66, 21–30.
Carruthers, E. H., Schneider, D. C., and Neilson, J. D. (2009). Estimating the odds of survival and identifying mitigation opportunities for common bycatch in pelagic longline fisheries. Biol. Conserv. 142, 2620–2630. doi: 10.1016/j.biocon.2009.06.010
Cassoff, R. M., Moore, K. M., McLellan, W. A., Barco, S. G., Rotstein, D. S., and Moore, M. J. (2011). Lethal entanglement in baleen whales. Dis. Aquat. Organ. 96, 175–185. doi: 10.3354/dao02385
Chilvers, B. L. (2008). New Zealand sea lions Phocarctos hookeri and squid trawl fisheries: bycatch problems and management options. Endanger. Species Res. 5, 193–204. doi: 10.3354/esr00086
R Core Team. (2019). R: A Language and Environment for Statistical Computing. Vienna: R Foundation for Statistical Computing.
Cosgrove, R., Gosch, M., Reid, D., Sheridan, M., Chopin, N., Jessopp, M., et al. (2016). Seal bycatch in gillnet and entangling net fisheries in Irish waters. Fish. Res. 183, 192–199. doi: 10.1016/j.fishres.2016.06.007
Cotter, A. J. R., and Pilling, G. M. (2007). Landings, logbooks and observer surveys: improving the protocols for sampling commercial fisheries. Fish Fish. 8, 123–152. doi: 10.1111/j.1467-2679.2007.00241.x
Cox, T. M., Lewison, R. L., Žydelis, R., Crowder, L. B., Safina, C., and Read, A. J. (2007). Comparing effectiveness of experimental and implemented bycatch reduction measures: the ideal and the real. Conserv. Biol. 21, 1155–1164. doi: 10.1111/j.1523-1739.2007.00772.x
Cruz, M. J., Machete, M., Menezes, G., Rogan, E., and Silva, M. A. (2018). Estimating common dolphin bycatch in the pole-and-line tuna fishery in the Azores. PeerJ 6:e4285. doi: 10.7717/peerj.4285
Curtis, K. A., and Carretta, J. V. (2020). ObsCovgTools: assessing observer coverage needed to document and estimate rare event bycatch. Fish. Res. 225:105493. doi: 10.1016/j.fishres.2020.105493
Curtis, K. A., Moore, J. E., Boyd, C., Dillingham, P. W., Lewison, R. L., Taylor, B. L., et al. (2015). Managing catch of marine megafauna: guidelines for setting limit reference points. Mar. Policy 61, 249–263. doi: 10.1016/j.marpol.2015.07.002
Davis, M. W. (2002). Key principles for understanding fish bycatch discard mortality. Can. J. Fish. Aquat. Sci. 59, 1834–1843. doi: 10.1139/f02-139
Dolman, S. J., and Moore, M. J. (2017). “Welfare implications of cetacean bycatch and entanglements,” in Marine Mammal Welfare, ed. A. Butterworth (Cham: Springer), 41–65. doi: 10.1007/978-3-319-46994-2
Emery, T. J., Noriega, R., Williams, A. J., and Larcombe, J. (2019). Changes in logbook reporting by commercial fishers following the implementation of electronic monitoring in Australian Commonwealth fisheries. Mar. Policy 104, 135–145. doi: 10.1016/j.marpol.2019.01.018
Evans, R., and Molony, B. (2011). Pilot Evaluation Of The Efficacy Of Electronic Monitoring On A Demersal Gillnet Vessel As An Alternative To Human Observers. Fisheries Research Report No. 221. Western Australia: Department of Fisheries, 20.
Faunce, C. H. (2011). A comparison between industry and observer catch compositions within the Gulf of Alaska rockfish fishery. ICES J. Mar. Sci. 68, 1769–1777. doi: 10.1093/icesjms/fsr093
Faunce, C. H., and Barbeaux, S. J. (2011). The frequency and quantity of Alaskan groundfish catcher-vessel landings made with and without an observer. ICES J. Mar. Sci. 68, 1757–1763. doi: 10.1093/icesjms/fsr090
Fernandes, A. C., Oroszlányová, M., Silva, C., Azevedo, M., and Coelho, R. (2021). Investigating the representativeness of onboard sampling trips and estimation of discards based on clustering. Fish. Res. 234:105778. doi: 10.1016/j.fishres.2020.105778
Food and Agriculture Organization of the United Nations [FAO] (2021). Fishing Operations. Guidelines To Prevent And Reduce Bycatch Of Marine Mammals In Capture Fisheries. Rome: FAO, doi: 10.4060/cb2887en
Furlong, W. J., and Martin, P. M. (2000). “Observer deployment in the fishery and regulatory self-enforcement,” in Microbehavior and Macroresults: Proceedings of the 10th Biennial Conference of the International Institute of Fisheries Economics and Trade, eds A. L. Shriver and R. S. Johnston (Corvallis: International Institute of Fisheries Economics and Trade), 1–8.
Gilman, E., Castejón, V. D. R., Loganimoce, E., and Chaloupka, M. (2020). Capability of a pilot fisheries electronic monitoring system to meet scientific and compliance monitoring objectives. Mar. Policy 113:103792.
Gilman, E., Legorburu, G., Fedoruk, A., Heberer, C., Zimring, M., and Barkai, A. (2019). Increasing the functionalities and accuracy of fisheries electronic monitoring systems. Aquat. Conserv. Mar. Freshw. Ecosyst. 29, 901–926. doi: 10.1002/aqc.3086
Gilman, E., Suuronen, P., Hall, M., and Kennelly, S. (2013). Causes and methods to estimate cryptic sources of fishing mortality. J. Fish Biol. 83, 766–803. doi: 10.1111/jfb.12148
Glemarec, G., Kindt-Larsen, L., Lundgaard, L. S., and Larsen, F. (2020). Assessing seabird bycatch in gillnet fisheries using electronic monitoring. Biol. Conserv. 243:108461. doi: 10.1016/j.biocon.2020.108461
Gómez-Muñoz, V. M. (1990). A model to estimate catches from a short fishery statistics survey. Bull. Mar. Sci. 46, 719–722.
Gray, C. A., and Kennelly, S. J. (2018). Bycatches of endangered, threatened and protected species in marine fisheries. Rev. Fish Biol. Fish. 28, 521–541. doi: 10.1007/s11160-018-9520-7
Greenstreet, S. P. R., Holland, G. J., Fraser, T. W. K., and Allen, V. J. (2009). Modelling demersal fishing effort based on landings and days absence from port, to generate indicators of “activity”. ICES J. Mar. Sci. 66, 886–901. doi: 10.1093/icesjms/fsp068
Hall, M. A. (1998). An ecological view of the tuna–dolphin problem: impacts and trade-offs. Rev. Fish Biol. Fish. 8, 1–34. doi: 10.1023/A:1008854816580
Hall, M. A., Alverson, D. L., and Metuzals, K. I. (2000). By-catch: problems and solutions. Mar. Pollut. Bull. 41, 204–219. doi: 10.1016/S0025-326X(00)00111-9
Hamer, D. J., Ward, T. M., Shaughnessey, P. D., and Clark, S. R. (2011). Assessing the effectiveness of the Great Australian Bight Marine Park in protecting the endangered Australian sea lion Neophoca cinerea from bycatch mortality in shark gillnets. Endanger. Species Res. 14, 203–216. doi: 10.3354/esr00353
Hammond, P. S., Francis, T. B., Heinemann, D., Long, K. J., Moore, J. E., Punt, A. E., et al. (2021). Estimating the abundance of marine mammal populations. Front. Mar. Sci. 8:735770. doi: 10.3389/fmars.2021.735770
Jaramillo-Legoretta, A. M., Cardenas-Hinojosa, G., Nieto-Garcia, E., Rojas-Bracho, L., Thomas, L., Ver Hoef, J. M., et al. (2019). Decline towards extinction of Mexico’s vaquita porpoise (Phocoena sinus). R. Soc. Open Sci. 6:190598. doi: 10.1098/rsos.190598
Johnson, A. F., Moreno-Báez, M., Giron-Nava, A., Corominas, J., Erisman, B., Ezcurra, E., et al. (2017). A spatial method to calculate small-scale fisheries effort in data poor scenarios. PLoS One 12:e0174064. doi: 10.1371/journal.pone.0174064
Julian, F., and Beeson, M. (1998). Estimates of marine mammal, turtle, and seabird mortality for two California gillnet fisheries: 1990-1995. Fish. Bull. 96, 271–284.
Kindt-Larsen, L., Dalskov, J., Stage, B., and Larsen, F. (2012). Observing incidental harbour porpoise Phocoena phocoena bycatch by remote electronic monitoring. Endanger. Species Res. 19, 75–83. doi: 10.3354/esr00455
Kirkwood, R. J., Goldsworthy, S. D., and Ward, T. M. (2020). Assessment of dolphin interactions, effectiveness of the Code of Practice and fishing behavior in the South Australian sardine fishery: 2019-20. SARDI Research Report Series No. 1077. Adeliaide: South Australian Research and Development Institute (Aquatic Sciences), 34.
Kroodsma, D. A., Mayorga, J., Hochberg, T., Miller, N. A., Boerder, K., Ferretti, F., et al. (2018). Tracking the global footprint of fisheries. Science 359, 904–908. doi: 10.1126/science.aao5646
Lewison, R. L., and Moore, J. E. (2012). Improving Interview-Based Assessments of Sea Turtle and Marine Mammal Bycatch in West Africa: Putting Fishing Activity into a Socio-Economic Context. Project Report. Washington, DC: NOAA Office of International Affairs.
Lewison, R. L., Crowder, L. B., Read, A. J., and Freeman, S. A. (2004). Understanding impacts of fisheries bycatch on marine megafauna. Trends Ecol. Evol. 19, 598–604. doi: 10.1016/j.tree.2004.09.004
Liggins, G. W., Bradley, M. J., and Kennelly, S. J. (1997). Detection of bias in observer-based estimates of retained and discarded catches from a multi species trawl fishery. Fish. Res. 32, 133–147. doi: 10.1016/S0165-7836(97)00053-2
Liu, M., Lin, M., Turvey, S., and Li, S. (2016). Fishers’ knowledge as an information source to investigate bycatch of marine mammals in the South China Sea. Anim. Conserv. 20, 182–192. doi: 10.1111/acv.12304
Lonergan, M. (2011). Potential biological removal and other currently used management rules for marine mammal populations: a comparison. Mar. Policy 35, 584–589. doi: 10.1016/j.marpol.2011.01.020
Luck, C., Jessopp, M., Tully, O., Cosgrove, R., Rogan, E., and Cronin, M. (2020). Estimating protected species bycatch from limited observer coverage: a case study of seal bycatch in static net fisheries. Glob. Ecol. Conserv. 24:e01213. doi: 10.1016/j.gecco.2020.e01213
Mangi, S. C., Dolder, P. J., Catchpole, T. L., Rodmell, D., and de Rozarieux, N. (2015). Approaches to fully documented fisheries: practical issues and stakeholder perceptions. Fish Fish. 16, 426–452. doi: 10.1111/faf.12065
Mangi, S. C., Smith, S., and Catchpole, T. L. (2016). Assessing the capability and willingness of skippers towards fishing industry-led data collection. Ocean Coast Manag. 134, 11–19. doi: 10.1016/j.ocecoaman.2016.09.027
Manly, B. F. (2011). Bootstrapping with models for count data. J. Biopharm. Stat. 21, 1164–1176. doi: 10.1080/10543406.2011.607748
Margolis, S., and Alger, B. (2020). The National Electronic Monitoring Workshop Report 2019| 2020. Washington: NOAA Office of Science and Technology, 46.
Martin, S. L., Stohs, S. M., and Moore, J. E. (2015). Bayesian inference and assessment for rare-event bycatch in marine fisheries: a drift gillnet fishery case study. Ecol. Appl. 25, 416–429. doi: 10.1890/14-0059.1
Maxwell, S. M., Witt, M. J., Abitsi, G., Aboro, M. P., Agamboue, P. D., Asseko, G. M., et al. (2018). Sea turtles and survivability in demersal trawl fisheries: do comatose olive ridley sea turtles survive post-release? Anim. Biotelemetry 6:11. doi: 10.1186/s40317-018-0155-1
McCluskey, S. M., and Lewison, R. L. (2008). Quantifying fishing effort: a synthesis of current methods and their applications. Fish Fish. 9, 188–200. doi: 10.1111/j.1467-2979.2008.00283.x
McElderry, H., McCullough, D., Schrader, J., and Illingworth, J. (2007). Pilot Study To Test The Effectiveness Of Electronic Monitoring In Canterbury Fisheries. Wellington: Science & Technical Publishing, 27.
Moore, J. E., Cox, T. M., Lewison, R. L., Read, A. J., Bjorkland, R., McDonald, S. L., et al. (2010). An interview-based approach to assess marine mammal and sea turtle captures in artisanal fisheries. Biol. Conserv. 143, 795–805. doi: 10.1016/j.biocon.2009.12.023
Moore, J. E., and Curtis, K. A. (2016). “Developing control rules for threatened bycatch species,” in Management Science in Fisheries: An Introduction to Simulation-Based Methods, 278-297, eds C. T. T. Edwards and D. J. Dankel (London: Routledge), 20. doi: 10.4324/9781315751443-23
Moore, J. E., Curtis, K. A., Lewison, R. L., Dillingham, P. W., Cope, J. M., Fordham, S. V., et al. (2013). Evaluating sustainability of fisheries bycatch mortality for marine megafauna: a review of conservation reference points for data-limited populations. Environ. Conserv. 40, 329–344. doi: 10.1017/S037689291300012X
Moore, J. E., and Read, A. J. (2008). A Bayesian uncertainty analysis of cetacean demography and bycatch mortality using age-at-death data. Ecol. Appl. 18, 1914–1931. doi: 10.1890/07-0862.1
National Marine Fisheries Service [NMFS] (2011). U.S. National Bycatch Report First Edition. Washington: NOAA, 508.
National Marine Fisheries Service [NMFS] (2012a). Guidelines for Distinguishing Serious from Non-Serious Injury of Marine Mammals Pursuant to the Marine Mammal Protection Act. Maryland: National Marine Fisheries Service.
National Marine Fisheries Service [NMFS] (2012b). Protected Resources Management Process for Distinguishing Serious from Non-Serious Injury of Marine Mammals. Maryland: National Marine Fisheries Service.
National Marine Fisheries Service [NMFS] (2016). Guidelines for Preparing Stock Assessment Reports Pursuant to Section 117 of the Marine Mammal Protection Act. Maryland: National Marine Fisheries Service.
Northeast Fisheries Science Center [NEFSC] (2019). Northeast Fisheries Observer Program Handbook for Fisheries Observers and Providers. Maryland: NOAA Fisheries.
Northridge, S., Coram, A., Kingston, A., and Crawford, R. (2017). Disentangling the causes of protected-species bycatch in gillnet fisheries. Conserv. Biol. 31, 686–695. doi: 10.1111/cobi.12741
Olaya-Ponzone, L., Espada, R., Moreno, E. M., Marcial, I. C., and García-Gómez, J. C. (2020). Injuries, healing and management of common dolphins (Delphinus delphis) in human-impacted waters in the south Iberian Peninsula. J. Mar. Biol. Assoc. U. K. 100, 315–325. doi: 10.1017/S0025315420000090
Orphanides, C. D. (2009). Protected species bycatch estimating approaches: estimating harbor porpoise bycatch in U. S. northwestern Atlantic gillnet fisheries. J. Northw. Atl. Fish. Sci. 42, 55–76. doi: 10.2960/J.v42.m647
Pacific Islands Regional Office Observer Program [PIRO-OP] (2017). Hawaii Longline Observer Program Field Manual, Version LM.17.02. Washington: National Oceanic and Atmospheric Administration.
Page, B., McKenzie, J., McIntosh, R., Baylis, A., Morrissey, A., Calvert, N., et al. (2004). Entanglement of Australian sea lions and New Zealand fur seals in lost fishing gear and other marine debris before and after Government and industry attempts to reduce the problem. Mar. Pollut. Bull. 49, 33–42. doi: 10.1016/j.marpolbul.2004.01.006
Parga, M. L., Crespo-Picazo, J. L., Monteiro, D., García-Párraga, D., Hernandez, J. A., Swimmer, Y., et al. (2020). On-board study of gas embolism in marine turtles caught in bottom trawl fisheries in the Atlantic Ocean. Sci. Rep. 10:5561. doi: 10.1038/s41598-020-62355-7
Patterson, H., Hansen, S., and Larcombe, J. (2014). A review of shark bycatch mitigation in tuna longline fisheries. Western and Central Pacific Fisheries Commission, Scientific Committee report, WCPFC-SC10-2014/EB-WP-05. Kolonia: Western and Central Pacific Fisheries Commission.
Pauly, D., and Zeller, D. (2016). Catch reconstructions reveal that global marine fisheries catches are higher than reported and declining. Nat. Commun. 7:10244. doi: 10.1038/ncomms10244
Peltier, H., Authier, M., Dabin, W., Dars, C., Demaret, F., Doremus, G., et al. (2020). Can modelling the drift of bycaught dolphin stranded carcasses help identify involved fisheries? An exploratory study. Glob. Ecol Conserv. 21:e00843. doi: 10.1016/j.gecco.2019.e00843
Peltier, H., Authier, M., Deaville, R., Dabin, W., Jepson, P. D., van Canneyt, O., et al. (2016). Small cetacean bycatch as estimated from stranding schemes: the common dolphin case in the northeast Atlantic. Environ. Sci. Policy 63, 7–18. doi: 10.1016/j.envsci.2016.05.004
Peltier, H., Dabin, W., Daniel, P., Van Canneyt, O., Dorémus, G., Huon, M., et al. (2012). The significance of stranding data as indicators of cetacean populations at sea: modelling the drift of cetacean carcasses. Ecol. Ind. 18, 278–290. doi: 10.1016/j.ecolind.2011.11.014
Pettis, H. M., Rolland, R. M., Hamilton, P. K., Knowlton, A. R., Burgess, E. A., and Kraus, S. D. (2017). Body condition changes arising from natural factors and fishing gear entanglements in North Atlantic right whales Eubalaena glacialis. Endanger. Species Res. 32, 237–249. doi: 10.3354/esr00800
Pilcher, N. J., Adulyanukosol, K., Das, H., Davis, P., Hines, E., Kwan, D., et al. (2017). A low-cost solution for documenting distribution and abundance of endangered marine fauna and impacts from fisheries. PLoS One 12:e0190021. doi: 10.1371/journal.pone.0190021
Plet-Hansen, K. S., Eliasen, S. Q., Mortensen, L. O., Bergsson, H., Olesen, H. J., and Ulrich, C. (2017). Remote electronic monitoring and the landing obligation–some insights into fishers’ and fishery inspectors’ opinions. Mar. Policy 76, 98–106. doi: 10.1016/j.marpol.2016.11.028
Porter, R. D. (2010). Fisheries observers as enforcement assets: lessons from the North Pacific. Mar. Policy 34, 583–589. doi: 10.1016/j.marpol.2009.11.005
Prado, J. H. F., Secchi, E. R., and Kinas, P. G. (2013). Mark-recapture of endangered franciscana dolphin (Pontoporia blainvillei) killed in gillnet fisheries to estimate past bycatch time series of stranded carcasses in southern Brazil. Ecol. Ind. 32, 35–41. doi: 10.1016/j.ecolind.2013.03.005
Punt, A. E. (1999). “Evaluating the costs and benefits of alternative monitoring programmes for fisheries management,” in Proceedings of the International Conference on Integrated Fisheries Monitoring, Sydney, Australia, 1-5 February 1999, ed. C. P. Nolan (Rome: FAO).
Punt, A. E., Sepúlveda, M., Siple, M. C., Moore, J., Francis, T. B., Hammond, P. S., et al. (2021). Assessing pinniped bycatch mortality with uncertainty in abundance and post-release mortality: a case study from Chile. Fish. Res. 235:105816. doi: 10.1016/j.fishres.2020.105816
Read, A. J. (2008). The looming crisis: interactions between marine mammals and fisheries. J. Mamm. 89, 541–548. doi: 10.1644/07-MAMM-S-315R1.1
Read, A. J., Drinker, P., and Northridge, S. (2006). Bycatch of marine mammals in US and global fisheries. Conserv. Biol 20, 163–169. doi: 10.1111/j.1523-1739.2006.00338.x
Read, A. J., Reynolds, J. E., Perrin, W. F., Reeves, R. R., Montgomery, S., and Ragen, T. J. (2005). “Bycatch and depredation,” in Marine Mammal Research: Conservation Beyond Crisis, eds T. J. Ragen, J. E. Reynolds, W. F. Perrin, R. R. Reeves, and S. Montgomery (Baltimore: The John Hopkins Univ. Press), 5–17.
Reeves, R. R., McClellan, K., and Werner, T. B. (2013). Marine mammal bycatch in gillnet and other entangling net fisheries, 1990 to 2011. Endanger. Species Res. 20, 71–97. doi: 10.3354/esr00481
Rolland, R. M., Schick, R. S., Pettis, H. M., Knowlton, A. R., Hamilton, P. K., Clark, J. S., et al. (2016). Health of North Atlantic right whales Eubalaena glacialis over three decades: from individual health to demographic and population health trends. Mar. Ecol. Prog. Ser. 542, 265–282. doi: 10.3354/meps11547
Roman, J., Estes, J. A., Morissette, L., Smith, C., Costa, D., McCarthy, J. B., et al. (2014). Whales as marine ecosystem engineers. Front. Ecol. Environ. 12, 377–385. doi: 10.1890/130220
Sampson, D. B. (2011). The accuracy of self-reported fisheries data: Oregon trawl logbook fishing locations and retained catches. Fish. Res. 112, 59–75. doi: 10.1016/j.fishres.2011.08.012
Secchi, E. R. (2010). “Review on the threats and conservation status of franciscana, Pontoporia blainvillei (Cetacea, Pontoporiidae),” in Biology, Evolution and Conservation of River Dolphins within South America and Asia, eds M. Ruiz-García and J. Shostell (New York: Nova Science), 323–339.
Slooten, E. (2007). Conservation management in the face of uncertainty: effectiveness of four options for managing Hector’s dolphin bycatch. Endanger. Species Res. 3, 169–179. doi: 10.3354/esr003169
Smith, H., and Basurto, X. (2019). Defining small-scale fisheries and examining the role of science in shaping perceptions of who and what counts: a systematic review. Front. Mar. Sci. 6:00236. doi: 10.3389/fmars.2019.00236
Snyder, H. T., and Erbaugh, J. T. (2020). Fishery observers address arctic fishery discards. Environ. Res. Lett. 15:0940c4. doi: 10.1088/1748-9326/aba57d
Soykan, C. U., Eguchi, T., Kohin, S., and Dewar, H. (2014). Prediction of fishing effort distributions using boosted regression trees. Ecol. Appl. 24, 71–83. doi: 10.1890/12-0826.1
Stacy, B. A., Keene, J. L., and Schroeder, B. A. (2016). Report of the technical expert workshop: Developing national criteria for assessing post-interaction mortality of sea turtles in trawl, net, and pot/trap fisheries: Shepherdstown, West Virginia, USA, 18-22 August 2015. Washington: NOAA.
Stock, B. C., Ward, E. J., Thorson, J. T., Jannot, J. E., and Semmens, B. X. (2019). The utility of spatial model-based estimators of unobserved catch. ICES J. Mar. Sci. 76, 255–267. doi: 10.1093/icesjms/fsy153
Suuronen, P., and Gilman, E. (2020). Monitoring and managing fisheries discards: new technologies and approaches. Mar. Policy 116:103554. doi: 10.1016/j.marpol.2019.103554
Taylor, B. L., Rojas-Bracho, L., Moore, J., Jaramillo-Legorreta, A., Ver Hoef, J. M., Cardenas-Hinojosa, G., et al. (2017). Extinction is imminent for Mexico’s endemic porpoise unless fishery bycatch is eliminated. Conserv. Lett. 10, 588–595. doi: 10.1111/conl.12331
Tietze, U. (2016). Technical And Socio-Economic Characteristics Of Small-Scale Coastal Fishing Communities, And Opportunities For Poverty Alleviation And Empowerment. Rome: FAO Fisheries and Aquaculture.
Turvey, S. T., Pitman, R. L., Taylor, B. L., Barlow, J., Akamatsu, T., Barrett, L. A., et al. (2007). First human-caused extinction of a cetacean species? Biol. Lett. 3, 537–540. doi: 10.1098/rsbl.2007.0292
van Helmond, A. T. M., Mortensen, L. O., Plet-Hansen, K. S., Ulrich, C., Needle, C. L., Oesterwind, D., et al. (2020). Electronic monitoring in fisheries: lessons from global experiences and future opportunities. Fish Fish. 21, 162–189. doi: 10.1111/faf.12425
Wade, P. R. (1998). Calculating limits to the allowable human-caused mortality of cetaceans and pinnipeds. Mar. Mamm. Sci. 14, 1–37. doi: 10.1111/j.1748-7692.1998.tb00688.x
Wade P. R., Long K. J., Francis T. B., Punt A. E., Hammond P. S., Heinemann D., et al. (2021). Best practices for assessing and managing bycatch of marine mammals. Front. Mar. Sci. 8:757330. doi: 10.3389/fmars.2021.757330
Wahlen, B. E., and Smith, T. D. (1985). Observer effect on incidental dolphin mortality in the Eastern Tropical Pacific tuna fishery. Fish. Bull. 83, 521–530.
Wakefield, C. B., Hesp, S. A., Blight, S., Molony, B. W., Newman, S. J., and Hall, N. G. (2018). Uncertainty associated with total bycatch estimates for rarely-encountered species varies substantially with observer coverage levels: informing minimum requirements for statutory logbook validation. Mar. Policy 95, 273–282. doi: 10.1016/j.marpol.2018.05.018
Walsh, W. A., Kleiber, P., and McCracken, M. (2002). Comparison of logbook reports of incidental blue shark catch rates by Hawaii-based longline vessels to fishery observer data by application of a generalized additive model. Fish. Res. 58, 79–94. doi: 10.1016/S0165-7836(01)00361-7
Wang, D., Zhang, X., Wang, K., Wei, Z., Würsig, B., Braulik, G. T., et al. (2006). Conservation of the baiji: no simple solution. Conserv. Biol. 20, 623–625. doi: 10.1111/j.1523-1739.2006.00471.x
Wells, R. S., Allen, J. B., Hofmann, S., Bassos-Hull, K., Fauquier, D. A., Barros, N. B., et al. (2008). Consequences of injuries on survival and reproduction of common bottlenose dolphins (Tursiops truncatus) along the west coast of Florida. Mar. Mamm. Sci. 24, 774–794. doi: 10.1111/j.1748-7692.2008.00212.x
Wells, R. S., Allen, J. B., Lovewell, G., Gorzelany, J., Delynn, R. E., Fauquier, D. A., et al. (2015). Carcass-recovery rates for resident bottlenose dolphins in Sarasota Bay, Florida. Mar. Mamm. Sci. 31, 355–368. doi: 10.1111/mms.12142
Whitty, T. S. (2016). Multi-methods approach to characterizing the magnitude, impact, and spatial risk of Irrawaddy dolphin (Orcaella brevirostris) bycatch in small-scale fisheries in Malampaya Sound, Philippines. Mar. Mamm. Sci. 32, 1022–1043. doi: 10.1111/mms.12322
Williams, R., Burgess, M. G., Ashe, E., Gaines, S. D., and Reeves, R. R. (2016). U.S. seafood import restriction presents opportunity and risk. Science 354, 1372–1374. doi: 10.1126/science.aai8222
Williams, R., Gero, S., Bejder, L., Calambokidis, J., Kraus, S. D., Lusseau, D., et al. (2011). Underestimating the damage: interpreting cetacean carcass recoveries in the context of the Deepwater Horizon/BP incident. Conserv. Lett. 4, 228–233. doi: 10.1111/j.1755-263X.2011.00168.x
Keywords: Potential Biological Removal (PBR), seafood import provisions, stock assessment, marine mammal, estimation, bycatch, Marine Mammal Protection Act
Citation: Moore JE, Heinemann D, Francis TB, Hammond PS, Long KJ, Punt AE, Reeves RR, Sepúlveda M, Sigurðsson GM, Siple MC, Víkingsson GA, Wade PR, Williams R and Zerbini AN (2021) Estimating Bycatch Mortality for Marine Mammals: Concepts and Best Practices. Front. Mar. Sci. 8:752356. doi: 10.3389/fmars.2021.752356
Received: 02 August 2021; Accepted: 09 November 2021;
Published: 10 December 2021.
Edited by:
Alastair Martin Mitri Baylis, South Atlantic Environmental Research Institute, Falkland IslandsReviewed by:
Simon David Goldsworthy, South Australian Research and Development Institute, AustraliaJustin Cooke, CEMS, Germany
Copyright © 2021 Moore, Heinemann, Francis, Hammond, Long, Punt, Reeves, Sepúlveda, Sigurðsson, Siple, Víkingsson, Wade, Williams and Zerbini. This is an open-access article distributed under the terms of the Creative Commons Attribution License (CC BY). The use, distribution or reproduction in other forums is permitted, provided the original author(s) and the copyright owner(s) are credited and that the original publication in this journal is cited, in accordance with accepted academic practice. No use, distribution or reproduction is permitted which does not comply with these terms.
*Correspondence: Jeffrey E. Moore, amVmZi5lLm1vb3JlQG5vYWEuZ292