- 1Department of Biology, University of West Florida, Pensacola, FL, United States
- 2Department of Earth, Ocean and Atmospheric Science, Florida State University, Tallahassee, FL, United States
- 3Department of Fisheries, Wildlife and Conservation Sciences, Oregon State University, Corvallis, OR, United States
Population size estimates are key parameters used in assessments to evaluate and determine a species’ conservation status. Typically, sea turtle population estimates are made from nesting beach surveys which capture only hatchling and adult female life stages and can display trends opposite of the full population. As such, in-water studies are critical to improve our understanding of sea turtle population dynamics as they can target a broader range of life stages – though they are more logistically and financially challenging to execute compared to beach-based surveys. Stereo-video camera systems (SVCS) hold promise for improving in-water assessments by removing the need to physically capture individuals and instead extract 3D measurements from video footage, thereby simplifying monitoring logistics and improving safety for the animals and surveyors. To demonstrate this potential, snorkel surveys were conducted at artificial habitats in the northeastern Gulf of Mexico (neGOM) to collect size and photo-identification data on sea turtles in situ using a SVCS. Over 29.86 survey hours, 35 sea turtles were observed across three species (Caretta caretta, Chelonia mydas, and Lepidochelys kempii) and all neritic life stages (juvenile, sub-adult, and adult) utilizing different habitats, including artificial reefs, jetties, and fishing piers. Greens straight carapace length ranged from 28.55 to 66.96 cm (n = 23, mean 43.07 cm ± 11.26 cm standard deviation; SD) and loggerheads ranged from 59.71 to 91.77 cm (n = 10, mean 74.50 cm ± 11.35 cm SD), and Kemp’s ridleys ranged from 42.23 cm to 44.98 cm (mean 43.61 cm ± 1.94 cm SD). Using a linear mixed model, we found that species and habitat type were the most important predictors of sea turtle body length distribution. Overall, this case study demonstrates the potential of SVCS surveys to enhance our understanding of the population structure of sea turtle species within the neGOM and elsewhere.
Introduction
Population assessments are crucial to determine population trends and status (i.e., trends in size class distribution; Crouse et al., 1987; Summers et al., 2017). For a population assessment to be considered robust it requires demographic data on all life stages, survival rates, habitat distribution, species-specific size data, and movement patterns (Heppell et al., 2003). Typically, only abundance data are used to assess population size and to estimate extinction risk for sea turtles and other endangered species (Schroeder and Murphy, 1999; Caswell, 2002; Morris and Doak, 2002; National Research Council (U.S.) et al., 2010). While abundance-centered assessments are essential, these data alone are insufficient to predict sea turtle population trends (Heppell et al., 2003). Abundance-based data alone can be misleading and lead to potentially erroneous conclusions about the direction and severity of population decline or recovery, especially if the population index is based on only one life stage, e.g., reproductive females (Esteban et al., 2017; Piacenza et al., 2019; Casale and Ceriani, 2020; Ceriani et al., 2021). Incorporating demographic data, specifically morphometric data, would lead to more effective modeling of populations and allow for researchers to estimate age at maturity, growth rates, and survival rates (Heppell et al., 2003; Casale et al., 2011). These vital rates allow researchers and conservation management agencies to determine if a population is declining or recovering and which, if any, conservation management actions are needed to aid in recovery (Bjorndal et al., 2011; Redfoot and Ehrhart, 2013). Collecting morphometric data in addition to abundance data can also be used to predict recruitment to reproductive life stages, particularly when populations are unstable, and the population structure is transient (O’Farrell and Botsford, 2006; White et al., 2013; Froese et al., 2018; Rudd and Thorson, 2018). Moreover, size-frequency distributions which encompass juvenile sizes can be powerful tools to understand population recovery that may not yet be reflected in adults, who are more commonly monitored (Hilborn and Walters, 2001; Ault et al., 2008; Heppell et al., 2012).
Sea turtle populations in general are commonly monitored by observing the number of females nesting or numbers of nests laid [National Research Council (U.S.) et al., 2010]. While nesting surveys provide readily accessible data for population assessments, sea turtles only spend 1% of their life on nesting beaches (as embryos to hatchlings and as nesters), yet 90% of sea turtle literature is derived from these surveys (Bjorndal, 1999; Wildermann et al., 2018). Studying a single life stage, such as nesting females, has been equated to studying human maternity wards with the assumption that the results represent the entire species (Bjorndal et al., 2011). In addition, a stage-based matrix model of loggerhead sea turtles (Caretta caretta) suggests juvenile life stages of sea turtles can have the largest impact on population growth and recovery (Crouse et al., 1987). However, due to their highly migratory behavior and difficulty to capture, studying in-water life stages presents a unique set of challenges (Wildermann et al., 2018).
Traditional methods for studying turtles in-water are to capture them via rodeo or tangle netting (Limpus and Walter, 1980; Ehrhart and Ogren, 1999; Fuentes et al., 2006). Both methods are time- and labor-intensive, which could result in additional stress on the animal, the turtle evading capture, and can leave researchers with small sample sizes, especially on short-term projects. However, observing turtles in situ using a stereo-video camera system (SVCS) can allow researchers to expand efforts to study different demographic classes while eliminating difficulty related to capturing turtles, thus improve the accuracy of population status estimates (Goetze et al., 2015; Araujo et al., 2016, 2019; Logan et al., 2017; Boldt et al., 2018). The SVCS is a non-invasive, remote method that allows for 3D measurements to be extracted from video footage (Harvey et al., 2002). The SVCS requires no handling of sea turtles and is highly accurate when compared to traditional hand measurements. Mean percent bias of the SVCS across three species of sea turtles ranged from −0.61% (±0.11 SE) to −4.46 % (±0.31 SE; Siegfried et al., 2021). Body size data is incorporated into length-based population assessment models, such as length-frequency analysis, which can be used to estimate growth rates, size at maturity, survival rates, and abundance of sea turtle populations (Casale et al., 2011). Length-frequency analysis requires relatively high sample numbers of turtles (Casale et al., 2011). Fortunately, since SVCSs do not require time-intensive capture methods, it is possible to achieve a larger sample size than methods that require capture.
The nearshore estuarine habitats and artificial reefs of the northeastern Gulf of Mexico (neGoM) have been recognized as geographic gaps in in-water sea turtle research in Florida (Eaton et al., 2008). For sea turtle species in these coastal waters, fewer studies have been conducted in situ to assess population structure and size-class distributions for loggerhead (C. caretta), green (C. mydas), and Kemp’s ridley (L. kempii) sea turtles known to use this region (but see: Avens et al., 2012; Hart et al., 2012, 2013, 2014, 2020; Metz and Landry, 2013; Lamont et al., 2015; Lamont and Iverson, 2018; Wildermann et al., 2019; Broadbent et al., 2020; Chabot et al., 2021; Lamont and Johnson, 2021). These existing studies have either focused on satellite telemetry studies, or have used in-water capture methods for other regions in the northern GOM, i.e., the lower Texas Coast, St. Joseph’s Bay, Florida, or off Crystal River, Florida, and only one included a study site in northwestern Florida. To demonstrate how SVCS surveys could be used to fill these data gaps and improve the accuracy and completeness of sea turtle population assessments, this study sought to (1) record sea turtle population size distributions, and (2) relate this distribution to artificial habitat preferences in the western Florida Panhandle as a case study for the application of SVCS.
Materials and Methods
Study Sites
The neGOM is a dynamic coastal environment composed mostly of soft, sandy bottom interspersed with inlets of estuarine seagrass beds and sparse natural hard-bottom or reefs (Locker et al., 2000) used by loggerhead, green, and Kemp’s ridley sea turtles (Lamont and Iverson, 2018; Wildermann et al., 2019). However, since the Deepwater Horizon oil spill in 2010, local and state authorities began adding additional artificial reef habitats to aid in fish recovery (Nelson, 2017). As of 2019, there have been 1,065 artificial reefs installed in the region from the Alabama border to Mexico Beach, Florida to attract marine wildlife and sustain ecotourism (Barnette, 2017; FWC Database, 2019).
Shore-based dive surveys on local artificial reefs, piers, and jetties from Santa Rosa to South Walton Counties of Florida with a SVCS were conducted weekly, weather permitting, from May 2019 to August 2020 with locations selected on an opportunistic rotating basis (Figure 1). We conducted a total of 58 dive surveys (29.86 total observation hours, surveys typically took ∼ 30 min to complete) at 14 sites from the Florida-Alabama border to just west of Panama City, FL, United States (Table 1 and Figure 1). Artificial habitats included artificial reefs, fishing piers, and rocky jetties. We attempted to survey each site an equal number of times; however, certain conditions, such as sea state, water visibility, or reef accessibility influenced the number of dive surveys at each site.
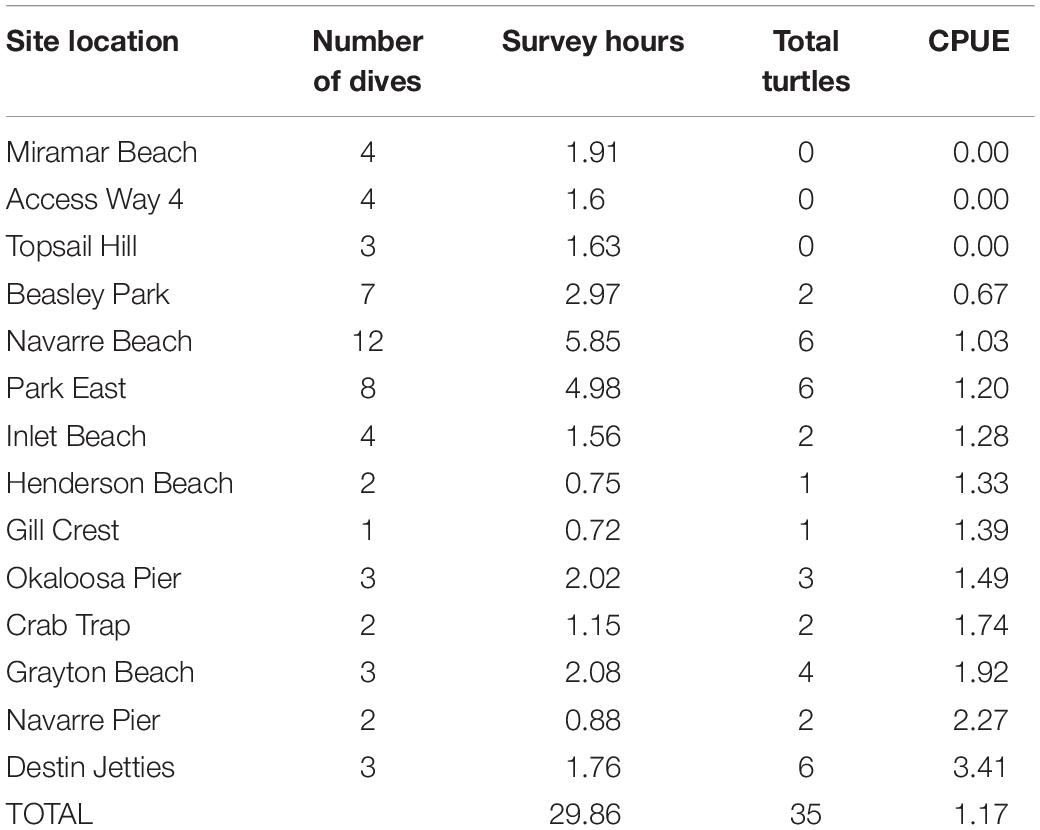
Table 1. Relative filming catch per unit effort (CPUE) of all species of sea turtles (n) per survey hour among locations during snorkel surveys. CPUE calculation includes Kemp’s ridleys.
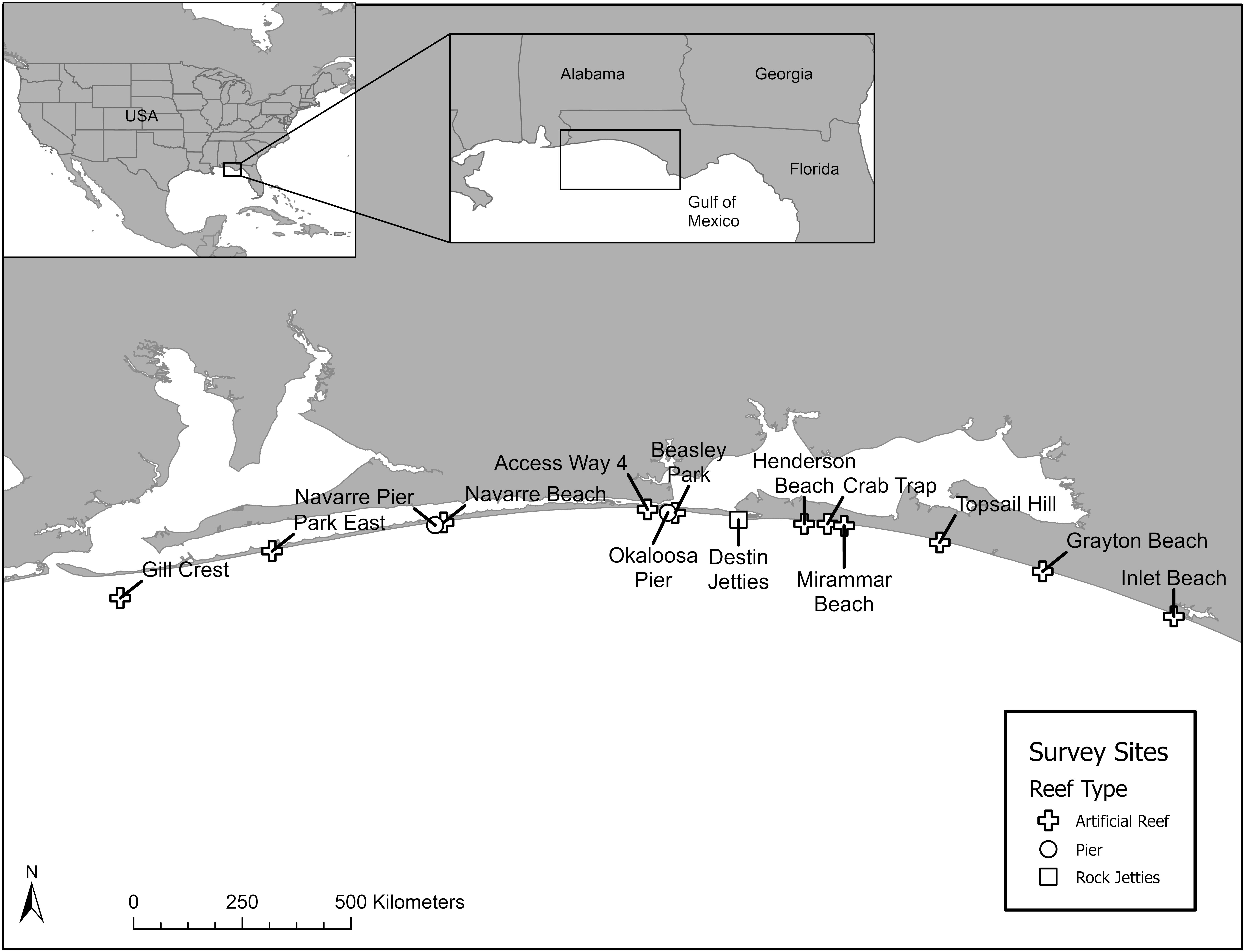
Figure 1. Northeastern Gulf of Mexico survey sites from Escambia County to South Walton County, Florida.
Stereo-Video Camera System Surveys
The SVCS was used to conduct video surveys throughout the neGOM. The SVCS was comprised of two GoPro® cameras attached at a fixed distance apart (0.8 m) that were inwardly converged at an angle of ∼4°. The SVCS was calibrated following the procedure described by Harvey and Shortis (1998) at the University of West Florida Aquatic center in <1 m depth of water using the SeaGIS CAL software v.3.23 (SeaGIS, 2008a Pty., Ltd., Bacchus Marsh, VIC, Australia). In previous work, the SVCS measurements were validated by comparing hand-captured measurements to stereo measurements and percent error was between −0.61% (±0.11 SE) and −4.46% (±0.31 SE) across three sea turtle species (Siegfried et al., 2021).
Opportunistic searches for sea turtles were conducted via snorkel, covering the entire artificial reef site while visually inspecting around and under each reef module at least once per survey. Surveys at all reefs were conducted at equivalent times of the day, typically between 10 am and 3 pm. The survey methodology was modified slightly for the fishing piers and jetties, where we swam linearly along the center of the pier pilings or along the edge of the jetty, rather than systematically swimming around the reef pilings. One researcher swam with the SVCS while the other researcher carried a secondary GoPro® camera to assist in obtaining facial identification photos. At each field site, water temperature, maximum depth, visibility, and weather conditions were recorded. We also noted if flipper tags were apparent, however, we usually could not read the tag identification codes, due to distance of turtle or bioaccumulation on tags. To be included in the data set, a survey was considered successful when the entire artificial reef assemblage was inspected and visibility was ≥2 m to allow for adequate detection of sea turtles. Visibility was visually estimated based on the divers’ experience. If these conditions were not met, the survey was not considered part of the sample set and was not included in the analysis.
Video footage was analyzed using SeaGIS EventMeasure software, v.5.22 (SeaGIS, 2008b Pty., Ltd., Bacchus Marsh, VIC, Australia) to record straight carapace length (SCL; cm). The measurement points for SCL were selected at the nuchal scute and the tip of one of the supracaudal scutes (Bolten, 1999) when both were clearly visible in the same frame. To reduce measurement error, the average of ten SCL measurements from separate video frames was calculated for each turtle (Harvey et al., 2001).
Catch per unit effort (CPUE) was calculated as the number of turtles filmed (i.e., “caught”) per dive time:
Where Nt is the number of turtles filmed and t is the time (in hours) at a given location (Table 1). When applicable, photo-identification using the I3S software with the random pattern search was used to check for re-sighting events (Calmanovici et al., 2018). I3S has a high success rate for positively identifying resighted individuals; for free-swimming turtles I3S has an 85% success rate (Calmanovici et al., 2018). Additionally, all matches identified by I3S were visually inspected to confirm potential match. To avoid pseudoreplication, all resighted turtles were treated as an individual average measurement as resightings happened <1 year apart and no substantial growth was observed.
Statistical Analysis
To examine factors that may be influencing size distribution, we evaluated individual body size (i.e., SCL) as a function of water temperature, species, and habitat type in a linear mixed effects model (LMM). Survey site was used as a repeated effect to account for spatial autocorrelation as we made multiple visits to each site. We ran model diagnostic tests to evaluate which model type was appropriate for the data and model residuals were assessed for homoscedasticity and normality. Visual inspection of the quantile-quantile plot and fitted values vs. residuals plot conformed to the model assumptions. Therefore, we evaluated factors influencing body size using LMM using R package lme4 (Bates et al., 2015).
We used the information-theoretic approach for model selection based on Akaike Information Criterion correction (AICc) for small sample sizes (Burnham and Anderson, 2002; Johnson and Omland, 2004) to identify explanatory variables that influence the size distribution using the dredge function in the R package MuMin (Barton, 2020). In the dredge function, we limited the number of allowed explanatory variables to 2 due to our small sample size. Models with ΔAICc < 2 from the top-ranked model were retained in the confidence model set. Lastly, we examined the 95% confidence intervals of all explanatory parameters to identify uninformative parameters, i.e., parameters that had confidence intervals crossing zero (Burnham and Anderson, 2002; Arnold, 2010; Leroux, 2019). All candidate models were tested against our global model:
Where SCL is the predicted mean body length at site i, β0 is the intercept, and εi ∼ N(0, σ2) of site i. All analyses were performed in R v.3.5.2 (R Development Core Team, 2021) and R Studio v.1.0.153 (RStudio Team, 2021 Inc.).
Results
Throughout our study, 35 sea turtles were recorded, but only 33 sea turtles were measured using the SVCS. CPUE among the different site locations varied considerably (Table 1). Destin Jetties had the highest CPUE with 3.41 turtles/h, while three sites (Dolphin Reef, Access Way 4, and Topsail Hill) had zero observed turtles despite over 5 h of surveying, combined. Overall, the average filming frequency across all dive surveys were 1.17 turtles/h. Three turtles were resighted at the same artificial reefs. Two individuals were resighted once, while the third individual was resighted three times.
We observed body lengths of green turtles (n = 23) ranging from 28.55 to 66.96 cm (mean 43.07 cm ± 11.26 cm standard deviation; SD) and loggerhead turtles (n = 10) ranging from 59.71 to 91.77 cm (mean 74.50 cm ± 11.35 cm SD) across all sites and locations (Figure 2). Green turtles were primarily juveniles, with only one subadult (defined as 65 < SCL < 90 cm; Bresette et al., 2010) observed (Table 2). Of the loggerhead turtles observed, 26% were classified as adults (SCL > 82 cm) and 74% were subadults (SCL < 82 cm; Márquez, 1990), and no juveniles were filmed. It should be noted that recent research indicates that the size at maturity cut-off for adult loggerhead and green turtles may be lower than previously thought (Phillips et al., 2021). However, we decided to use a more broadly accepted cutoffs for these species as this study was just recently published and was based on data from one nesting beach, albeit with very high numbers of nesters. In the future, it may be worthwhile to use these lower cut-offs for size-at-maturity, as well as to consider regionally specific cut-offs. One adult male loggerhead turtle was confirmed based on tail length. Kemp’s ridley turtles were observed as well (Figures 3A-D), but because their sample size was small (n = 2), they were excluded from the statistical analysis, and their size ranged from 42.23 cm to 44.98 cm (mean 43.61 cm ± 1.94 cm SD).
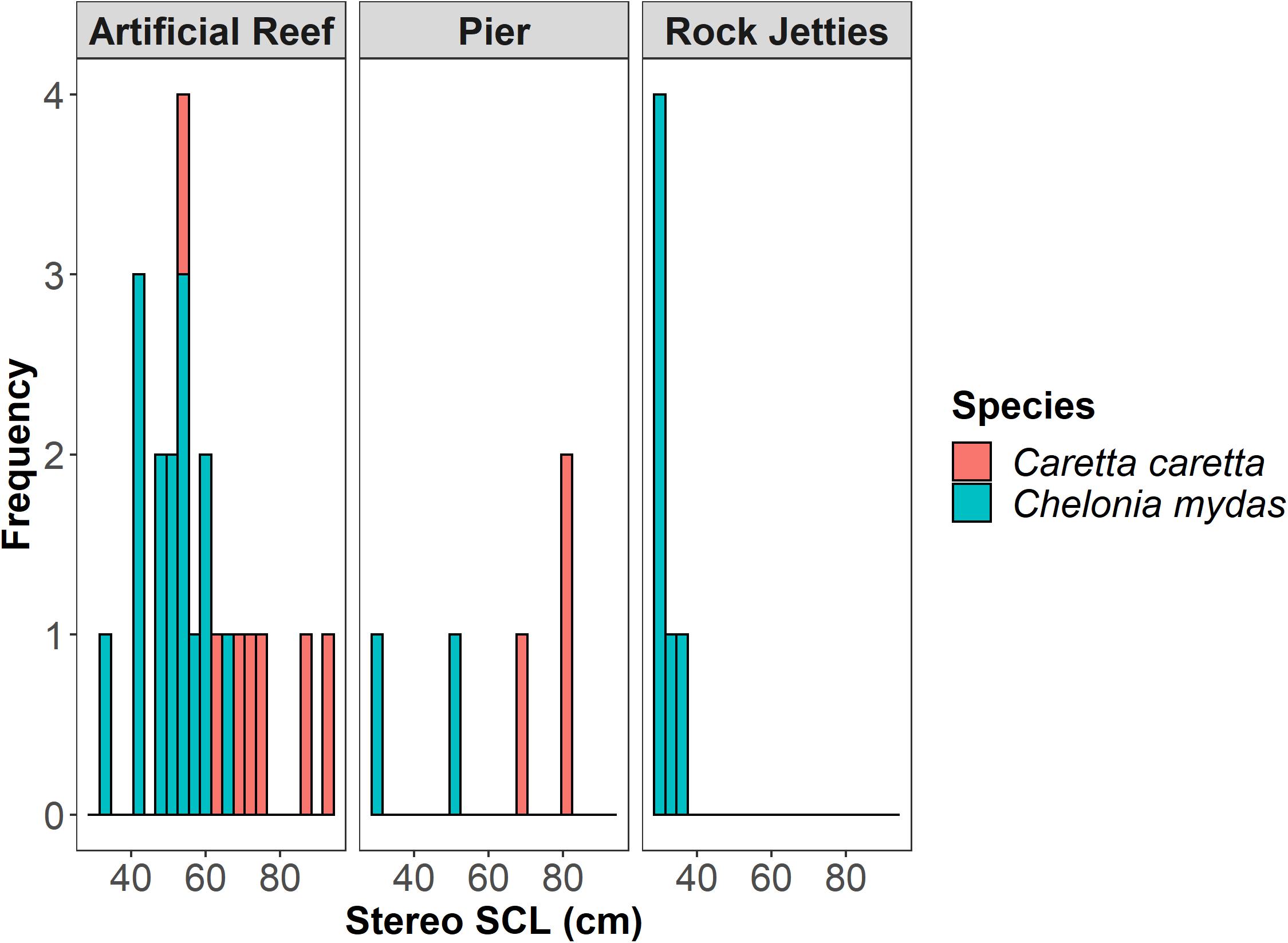
Figure 2. Size-frequency distribution of sea turtle species across our study sites in the northeastern Gulf of Mexico.
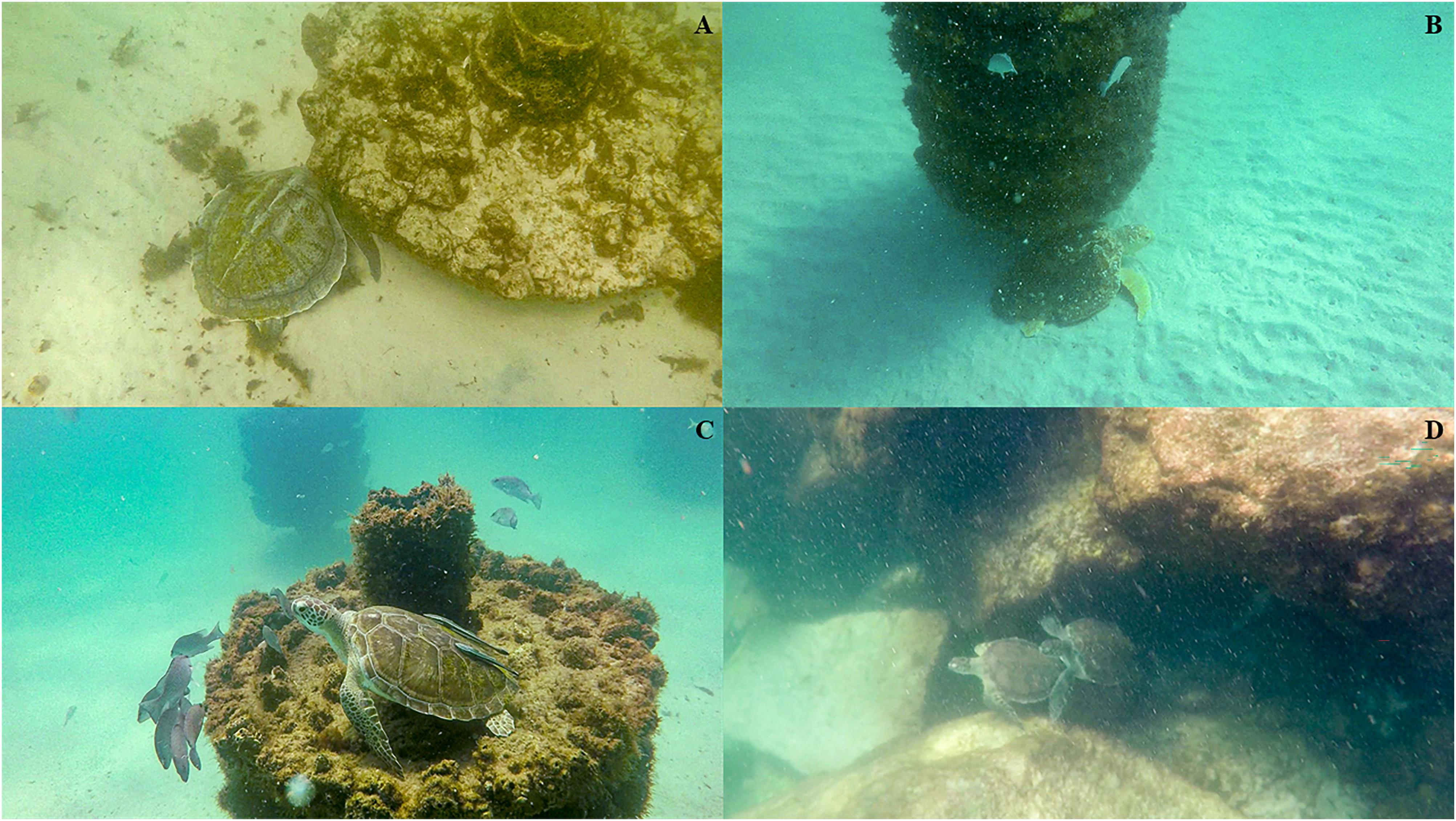
Figure 3. All species of sea turtles using artificial habitats for resting and protection in the northeastern Gulf of Mexico. (A) Kemp’s ridley (Lepidochelys kempii), (B) Loggerhead (Caretta caretta), (C) green (Chelonia mydas), observed sitting on top of reef module, and (D) two juvenile green turtles observed resting and swimming together at the Destin East Pass Jetties.
When evaluating the effect of water temperature, habitat, and species on the size distribution, the model confidence set included one top ranked model (Table 3). In the top ranked model, habitat type and species best predicted SCL of sea turtles in the neGOM. Upon evaluating the explanatory variables in the confidence set, only one variable’s confidence intervals crossed zero: the fishing pier habitat type. Overall, the rock jetties supported the smallest green turtles, with the fishing pier and artificial habitat supporting a wider range size of this species (Figure 4). The artificial reefs supported a wider range of loggerhead turtles, subadult to adult, while we observed only sub-adult loggerhead turtles at the fishing piers (Figure 4).

Table 3. Model confidence set (ΔAICc < 2) for LMM analysis of body length and environmental correlates.
Discussion
The SVCS successfully collected length-based data on sea turtle populations at nearshore artificial habitats. Research on artificial reefs in Florida’s nearshore coastal waters is generally lacking due to the difficulty in capturing and accessing the turtles on these reefs with traditional research methods. However, the use of SVCS provided a snapshot of the local sea turtle populations in the neGOM, with minimal cost and without the need for direct capture methods. The SVCS allowed us to remotely measure SCL from each turtle encounter, separate each animal into the appropriate size class, and then examine the size class distribution among different habitat types.
Our LMM analysis indicated that habitat type and species greatly influenced size distribution of sea turtles throughout the neGOM. In our study, all green turtles except one were considered juveniles (SCL < 65 cm), and were observed at almost all sites, except Beasley Park and Okaloosa fishing pier. A reasonably high density of juvenile green turtles may suggest that the area is serving as an important foraging and recruitment area for this species (León and Diez, 1999). Once green turtles reach SCL ≥ 35 cm, they undergo an ontogenetic habitat shift from the open ocean to the nearshore reefs (Summers et al., 2017). Often, these smaller juvenile green turtles can be found at rock jetties as they transition from open ocean to nearshore foraging habitats (Figure 3D; Coyne, 1994; Metz and Landry, 2013). Rock jetties serve as resting grounds, providing juvenile green turtles shelter and adequate food, primarily algae, during this transition (Coyne, 1994; Metz and Landry, 2013). In our study, the Destin Jetties supported the smallest size range of green turtles (range 30.76 cm–34.57 cm; mean 31.81 ± 1.51 cm). The observed size distribution may be a result of size-specific habitat requirements and predation risk (Bresette et al., 2010).
Loggerhead turtles observed in the study area were sub-adults and adults, with no observations of juveniles. Loggerhead turtles are highly migratory and travel between foraging grounds and breeding grounds (Hart et al., 2014) and may be attracted to fish and encrusting invertebrates, such as sponges and cnidarians, present at the artificial reefs (Mendonça et al., 1982). Loggerhead turtles may use the artificial reefs as resting grounds while migrating into the neGOM for breeding and nesting. Notably, most of the loggerhead turtles observed coincided with the nesting season (May–October), which may be because several beaches in the neGOM are known nesting beaches for loggerhead turtles (Fuentes et al., 2016 and Silver-Gorges et al., 2021). However, only 26% of the loggerhead turtles observed during our surveys were classified as adults, so this also suggests that the Florida panhandle is important habitat for sub-adult loggerheads.
Flipper-tagged green turtles were present at Navarre Beach, Park East, and the Navarre Beach fishing pier; however, untagged green and loggerhead turtles were present at all site locations. Most local tagging efforts in the region occur at nearby sea turtle rehabilitation centers, rather than in-water research tagging efforts, although sustained in-water capture and tagging programs exist in St. Joseph’s Bay and in the Big Bend area (Lamont and Johnson, 2020; Wildermann et al., 2020; Chabot et al., 2021). Regardless, the SVCS allows researchers to collect data on turtles that have not yet been tagged and of various size classes. Green turtles were observed year-round, with three individuals being re-sighted at the same artificial reef, which may suggest site fidelity and residency. Juvenile hawksbills have fidelity to specific sites (Limpus, 1992; van Dam and Diez, 1998), thus, it is not unlikely that juvenile green turtles may experience this same sort of site fidelity. Past studies have confirmed that green turtles tend to overwinter in the neGOM (Lamont et al., 2018), so, perhaps, it should not be unexpected that green turtles inhabit artificial reefs year-round even at such northerly sites. This could be discerned with longer-term monitoring programs at artificial reefs in the neGOM. If conditions in an area are favorable (i.e., feeding, protection, adequate temperatures, and nesting for adults), despite the higher latitude location, then there would be no need for migration elsewhere (Carr, 1980).
In considering the efficacy of using SVCS to obtain morphometric data, we calculated catch per unit effort. Notably, our survey sites differed significantly in survey effort and was heavily influenced by weather and sea state, and therefore sample sizes were higher at some artificial reefs and between habitat types (Table 1). Anecdotally, there is probably also an observer effect, which we did not calculate as our survey team was consistent throughout the study period. It seems likely that observers that conduct surveys at different swim speeds, or other variables, may have different CPUE. In practice, swimming with the SVCS slows the diver down, and thus this individual would set the pace for the survey. In the future, it may be useful to physically measure distance traveled during the survey (e.g., using GPS) or include survey team as a random effect, when evaluating patterns in carapace length and SVCS CPUE. In addition, it is likely that sites with higher turtle abundance would also have higher CPUE. Future surveys that compared different types of surveys, e.g., in-water SVC and aerial surveys with unmanned aerial vehicles, in locations with high water visibility and calm conditions may help to better ascertain sighting efficiency and CPUE for the SVCS.
Our CPUE (range: 0.0–3.41 turtles/h) was comparable to capture frequencies seen in the Dominican Republic (range: 0.0–3.43 turtles/h; León and Diez, 1999) and Mona Island, Puerto Rico (range: 0.48–2.38 turtles/h) during snorkel surveys. For example, our maximum CPUE was slightly less than the maximum sighting frequency observed in the Dominican Republic (sighting frequency (3.41 vs. 3.43 turtle/h) and slightly less than maximum capture frequency (3.41 vs. 3.43 turtles/h; León and Diez, 1999). Our average CPUE was 1.17 turtles/h, which is comparable to the sighting frequency in the Dominican Republic (1.67 turtles/h) and slightly less than their capture frequency (1.42 turtles/h; León and Diez, 1999). During our dive surveys, only four sea turtles were sighted in the water, but not successfully filmed. This is substantially less than snorkel capture surveys in the Dominican Republic, where they sighted 324 turtles and successfully captured 275 of those turtles (León and Diez, 1999). Ultimately, incorporating the use of SVCS to conduct dive surveys at artificial habitats would greatly increase the amount of data collected on a given sea turtle population.
Conclusion
Many mark-recapture studies are commonly conducted in seagrass beds, which are important habitats for sea turtles. However, few mark-recapture studies have been conducted in the coastal waters of the neGOM, and even fewer at artificial habitats (i.e., fishing piers, jetties, and reefs), due to logistical and financial challenges associated with direct capture methods (but see Coleman et al., 2016). SVCS allows researchers to study sea turtle population structure in areas where it is otherwise difficult to capture a range of size classes. Importantly, SVCS may be used in various locations, such as deep offshore artificial reefs, nearshore habitats, seagrass beds, and mangrove creeks (Santana-Garcon et al., 2014; Cundy et al., 2017; Logan et al., 2017; Siegfried et al., 2021); however, decent water visibility is required for turtle detection (Siegfried et al., 2021). With the implementation of the SVCS over time, residency and site fidelity traits may be monitored at selected sites. The use of SVCS gives researchers a greater chance to study sea turtles in-water, where they spend most of their lives, without the need to capture or to physically tag. Therefore, this methodology may give scientists a more comprehensive understanding of the sea turtle populations in each area. Through our remote in-water study, we have observed three species and all neritic life stages using artificial habitats. Thus, by implementing and collecting demographic data in-water using novel approaches, such as the SVCS, we demonstrate the use of an in-water non-invasive monitoring study while also collecting baseline population data and size structure for loggerhead and green sea turtles.
Data Availability Statement
The raw data supporting the conclusions of this article will be made available by the authors, without undue reservation.
Ethics Statement
Ethical review and approval was not required for this animal study because no handling of animals occurred. Therefore, no ethical committee evaluation was required.
Author Contributions
TS contributed to the conception and design of study, data collection, data analysis, grant writing, and writing of the manuscript. CN and JR contributed to data collection, post-processing video footage, and extensively reviewing the manuscript. MW contributed R code analysis support, GIS mapping consultation, and extensively reviewing the manuscript. MF contributed to an extensive review of the manuscript. SP was the advisor of TS and contributed data analysis, aiding in grant writing, aiding in research development, and manuscript writing. All authors contributed to manuscript revision, read, and approved the submitted version.
Funding
Funding was provided by Sigma XI grant, Florida Sea Turtle Grants Program, HMCSE Graduate Research Grant, UWF Office of Undergraduate Research, UWF Department of Biology, and UWF Honors Program.
Conflict of Interest
The authors declare that the research was conducted in the absence of any commercial or financial relationships that could be construed as a potential conflict of interest.
Publisher’s Note
All claims expressed in this article are solely those of the authors and do not necessarily represent those of their affiliated organizations, or those of the publisher, the editors and the reviewers. Any product that may be evaluated in this article, or claim that may be made by its manufacturer, is not guaranteed or endorsed by the publisher.
Acknowledgments
We would like to thank Emma Roberto for assisting in snorkel surveys, as well as the undergraduate students who contributed to the project. We would also like to thank the funding sources that helped make this research possible: UWF Hal Marcus College of Science and Engineering, University of West Florida (UWF) Biology Department, Office of Undergraduate Research, UWF Honors Program, Sigma XI grant, and Sea Turtle Grants Program. Opportunistic stereo-filming of sea turtles did not require a permit for scientific take, under guidance of NMFS and FWC. When sea turtles were observed, we followed them for <5 min and took care not to cause startle flight responses, per guidance from Florida Fish and Wildlife Commission (FWC) and National Oceanic and Atmospheric Administration (NOAA).
References
Araujo, G., Legaspi, C., Ferber, S., Murray, R., Burdett, K., Grundy, S., et al. (2019). In-water methods reveal population dynamics of a green turtle Chelonia mydas foraging aggregation in the Philippines. Endanger. Species Res. 40, 207–218. doi: 10.3354/esr00989
Araujo, G., Montgomery, J., Pahang, K., Labaja, J., Murray, R., and Ponzo, A. (2016). Using minimally invasive techniques to determine green sea turtle Chelonia mydas life-history parameters. J. Exp. Mar. Biol. Ecol. 483, 25–30. doi: 10.1016/j.jembe.2016.06.004
Arnold, T. W. (2010). Uninformative parameters and model selection using Akaike’s information criterion. J. Wildl. Manag. 74, 1175–1178. doi: 10.2193/2009-367
Ault, J. S., Smith, S. G., Luo, J., Monaco, M. E., and Appeldoorn, R. S. (2008). Length-based assessment of sustainability benchmarks for coral reef fishes in Puerto Rico. Environ. Conserv. 35, 221–231. doi: 10.1017/S0376892908005043
Avens, L., Goshe, L. R., Harms, C. A., Anderson, E. T., Hall, A. G., Cluse, W. M., et al. (2012). Population characteristics, age structure, and growth dynamics of neritic juvenile green turtles in the northeastern Gulf of Mexico. Mar. Ecol. Prog. Ser. 458, 213–229. doi: 10.3354/meps09720
Barnette, M. C. (2017). Potential Impacts of Artificial Reef Development on Sea Turtle Conservation in Florida. 1.1 MB. St. Petersburg, FL: U.S. Department of Commerce,Google Scholar
Barton, K. (2020). MuMIn: Multi-Model Inference. R package version 1.43.17. Available online at: https://CRAN.R-project.org/package=MuMIn (accessed April15, 2020).
Bates, D., Maechler, M., Bolker, B., and Walker, S. (2015). Fitting linear mixed-effects models using lme4. J. Stat. Softw. 67, 1–48. doi: 10.18637/jss.v067.i01
Bjorndal, K. A. (1999). “Priorities for research in foraging habitats,” in Research and Management Techniques for the Conservation of Sea Turtles, eds S. A. Eckert, K. A. Bjorndal, F. A. Abreu-Grobois, and M. Donnelly (Washington, DC: IUCN).
Bjorndal, K. A., Bowen, B. W., Chaloupka, M., Crowder, L. B., Heppell, S. S., Jones, C. M., et al. (2011). Better science needed for restoration in the Gulf of Mexico. Science 331, 537–538. doi: 10.1126/science.1199935
Boldt, J. L., Williams, K., Rooper, C. N., Towler, R. H., and Gauthier, S. (2018). Development of stereo camera methodologies to improve pelagic fish biomass estimates and inform ecosystem management in marine waters. Fish. Res. 198, 66–77. doi: 10.1016/j.fishres.2017.10.013
Bolten, A. B. (1999). “Techniques for measuring sea turtles,” in Research and Management Techniques for the Conservation of Sea Turtles, eds S. A. Eckert, K. A. Bjorndal, F. A. Abreu-Grobois, and M. Donnelly (Washington, DC: IUCN), 110–114.
Bresette, M. J., Witherington, B. E., Herren, R. M., Bagley, D. A., Gorham, J. C., Traxler, S. L., et al. (2010). Size-class partitioning and herding in a foraging group of green turtles Chelonia mydas. Endanger. Species Res. 9, 105–116. doi: 10.3354/esr00245
Broadbent, H. A., Grasty, S. E., Hardy, R. F., Lamont, M. M., Hart, K. M., Lembke, C., et al. (2020). West Florida shelf pipeline serves as sea turtle benthic habitat based on in situ towed camera observations. Aquat. Biol. 29, 17–31. doi: 10.3354/ab00722
Burnham, K., and Anderson, D. (2002). Model Selection and Multimodel Inference: A Practical Information-Theoretic Approach. New York, NY: Springer.
Calmanovici, B., Waayers, D., Reisser, J., Clifton, J., and Proietti, M. (2018). I3S Pattern as a mark-recapture tool to identify captured and free-swimming sea turtles: an assessment. Mar. Ecol. Prog. Ser. 589, 263–268. doi: 10.3354/meps12483
Carr, A. (1980). Some problems of sea turtle ecology. Am. Zool. 20, 489–498. doi: 10.1093/icb/20.3.489
Casale, P., and Ceriani, S. (2020). Sea turtle populations are overestimated worldwide from remigration intervals: correction for bias. Endanger. Species Res. 41, 141–151. doi: 10.3354/esr01019
Casale, P., Mazaris, A., and Freggi, D. (2011). Estimation of age at maturity of loggerhead sea turtles Caretta caretta in the Mediterranean using length-frequency data. Endanger. Species Res. 13, 123–129. doi: 10.3354/esr00319
Caswell, H. (2002). Matrix Population Models: Construction, Analysis, and Interpretation, 2nd Edn. Sunderland, MA: Sinauer Associates, Inc.
Ceriani, S. A., Brost, B., Meylan, A. B., and Casale, P. (2021). Bias in sea turtle productivity estimates: error and factors involved. Mar. Biol. 168:41. doi: 10.1007/s00227-021-03843-w
Chabot, R., Welsh, R., Mott, C., Guertin, J., Shamblin, B., and Witherington, B. (2021). A sea turtle population assessment for Florida’s big bend, Northeastern Gulf of Mexico. Gulf Caribb. Res. 32, 19–33. doi: 10.18785/gcr.3201.05
Coleman, A. T., Pulis, E. E., Pitchford, J. L., Crocker, K., Heaton, A. J., Carron, A. M., et al. (2016). Population ecology and rehabilitation of incidentally captured kemp’s ridley sea turtles (lepidochelys kempii) in the mississippi sound, USA. Herpetol. Conserv. Biol. 11, 253–264. doi: 10.1002/aqc.2656
Coyne, M. S. (1994). Feeding Ecology of Subadult Green Sea Turtles in South Texas Waters. Ph.D Thesis. College Station, TX: Texas A&M University.
Crouse, D. T., Crowder, L. B., and Caswell, H. (1987). A stage-based population model for loggerhead sea turtles and implications for conservation. Ecology 68, 1412–1423. doi: 10.2307/1939225
Cundy, M. E., Santana-Garcon, J., Ferguson, A. M., Fairclough, D. V., Jennings, P., and Harvey, E. S. (2017). Baited remote underwater stereo-video outperforms baited downward-facing single-video for assessments of fish diversity, abundance and size composition. J. Exp. Mar. Biol. Ecol. 497, 19–32. doi: 10.1016/j.jembe.2017.09.004
Eaton, C., McMichael, E., Witherington, B., Foley, A., Hardy, R., and Meylan, A. (2008). In-Water Sea Turtle Monitoring and Research in Florida. Review and Recommendations NOAA Technical Memorandum NMFS-OPR-38. St. Petersburg, FL: Florida Fish and Wildlife Conservation Commission.
Ehrhart, L. M., and Ogren, L. H. (1999). “Studies in Foraging habitats: capturing and handling turtles,” in Research and Management Techniques for the Conservation of Sea Turtles, eds K. L. Eckert, K. A. Bjorndal, F. A. Abreu-Grobois, and M. Donnelly (Gland: IUCN).
Esteban, N., Mortimer, J. A., and Hays, G. C. (2017). How numbers of nesting sea turtles can be overestimated by nearly a factor of two. Proc. R. Soc. B Biol. Sci. 284:20162581. doi: 10.1098/rspb.2016.2581
Froese, R., Winker, H., Coro, G., Demirel, N., Tsikliras, A. C., Dimarchopoulou, D., et al. (2018). A new approach for estimating stock status from length frequency data. ICES J. Mar. Sci. 75, 2004–2015. doi: 10.1093/icesjms/fsy078
Fuentes, M. M. P. B., Gredzens, C., Bateman, B. L., Boettcher, R., Ceriani, S. A., Godfrey, M. H., et al. (2016). Conservation hotspots for marine turtle nesting in the United States based on coastal development. Ecol. Appl. 26, 2706–2717. doi: 10.1002/eap.1386
Fuentes, M. M. P. B., Lawler, I. R., and Gyuris, E. (2006). Dietary preferences of juvenile green turtles (Chelonia mydas) on a tropical reef flat. Wildl. Res. 33:671. doi: 10.1071/WR05081
FWC Database (2019). Artificial Reefs in Florida. In: Geodata.myfwc.com. Available online at: http://geodata.myfwc.com/datasets/artificial-reefs-in-florida?geometry=-94.069%2C24.150%2C-73.393%2C30.962 (accessed Sep 8, 2020)
Goetze, J. S., Jupiter, S. D., Langlois, T. J., Wilson, S. K., Harvey, E. S., Bond, T., et al. (2015). Diver operated video most accurately detects the impacts of fishing within periodically harvested closures. J. Exp. Mar. Biol. Ecol. 462, 74–82. doi: 10.1016/j.jembe.2014.10.004
Hart, K. M., Lamont, M. M., Fujisaki, I., Tucker, A. D., and Raymond, R. C. (2012). Common coastal foraging areas for loggerheads in the Gulf of Mexico: opportunities for marine conservation. Biol. Conserv. 145, 185–194. doi: 10.1016/j.biocon.2011.10.030
Hart, K. M., Lamont, M. M., Iverson, A. R., and Smith, B. J. (2020). The importance of the Northeastern Gulf of Mexico to foraging loggerhead sea turtles. Front. Mar. Sci. 7:330. doi: 10.3389/fmars.2020.00330
Hart, K. M., Lamont, M. M., Sartain, A. R., and Fujisaki, I. (2014). Migration, foraging, and residency patterns for Northern Gulf loggerheads: implications of local threats and international movements. PLoS One 9:e103453. doi: 10.1371/journal.pone.0103453
Hart, K. M., Lamont, M. M., Sartain, A. R., Fujisaki, I., and Stephens, B. S. (2013). Movements and habitat-use of loggerhead sea turtles in the Northern Gulf of Mexico during the reproductive period. PLoS One 8:e66921. doi: 10.1371/journal.pone.0066921
Harvey, E., Fletcher, D., and Shortis, M. (2001). A comparison of the precision and accuracy of estimates of reef-fish lengths determined visually by divers with estimates produced by a stereo-video system. Fish. Bull. 99, 63–71.
Harvey, E., Fletcher, D., and Shortis, M. (2002). Estimation of reef fish length by divers and by stereo-video A first comparison of the accuracy and precision in the field on living fish under operational conditions. Fish. Res. 57, 255–265. doi: 10.1016/S0165-7836(01)00356-3
Harvey, E. S., and Shortis, M. R. (1998). Calibration stability of an underwater stereo video system: implications for measurement accuracy and precision. Mar. Technol. Soc. J. 32, 3–17.
Heppell, S. A., Semmens, B. X., Archer, S. K., Pattengill-Semmens, C. V., Bush, P. G., McCoy, C. M., et al. (2012). Documenting recovery of a spawning aggregation through size frequency analysis from underwater laser calipers measurements. Biol. Conserv. 155, 119–127. doi: 10.1016/j.biocon.2012.06.002
Heppell, S. S., Crowder, L. B., Crouse, D. T., Epperly, S. P., and Frazer, N. B. (2003). “Population models for atlantic loggerheads: past, present, and future,” in Loggerhead Sea Turtles, eds A. Bolten and B. Witherington (Washington, DC.: Smithsonian Institution Press), 255–273.
Hilborn, R., and Walters, C. J. (2001). Quantitative Fishers Stock Assessment: Choice, Dynamics, and Uncertainty. Boston, MA: Kluwer Academic Oublishers, 563.
Johnson, J. B., and Omland, K. S. (2004). Model selection in ecology and evolution. Trends Ecol. Evol. 19, 101–108. doi: 10.1016/j.tree.2003.10.013
Lamont, M. M., Fujisaki, I., Stephens, B. S., and Hackett, C. (2015). Home range and habitat use of juvenile green turtles (Chelonia mydas) in the northern Gulf of Mexico. Anim. Biotelemetry 3, 1–12. doi: 10.1186/s40317-015-0089-9
Lamont, M. M., and Iverson, A. (2018). Shared habitat use by juveniles of three sea turtle species. Mar. Ecol. Prog. Ser. 606, 187–200. doi: 10.3354/meps12748
Lamont, M. M., and Johnson, D. (2020). Growth rates for immature Kemp’s ridley sea turtles from a foraging area in the northern Gulf of Mexico. Mar. Ecol. Prog. Ser. 652, 145–155. doi: 10.3354/meps13469
Lamont, M. M., and Johnson, D. (2021). Variation in species composition, size and fitness of two multi-species sea turtle assemblages using different neritic habitats. Front. Mar. Sci 7:608740. doi: 10.3389/fmars.2020.608740
Lamont, M. M., Seay, D. R., and Gault, K. (2018). Overwintering behavior of juvenile sea turtles at a temperate foraging ground. Ecology 99, 2621–2624. doi: 10.1002/ecy.2439
León, Y. M., and Diez, C. E. (1999). Population structure of hawksbill turtles on a foraging ground in the dominican republic. Chelonian Conserv. Biol. 3, 230–236.
Leroux, S. J. (2019). On the prevalence of uninformative parameters in statistical models applying model selection in applied ecology. PLoS One 14:e0206711. doi: 10.1371/journal.pone.0206711
Limpus, C. J. (1992). The hawksbill turtle, Eremochelys imbricata, in Queensland: population structure within a southern great barrier reef feeding ground. Wildl. Res. 19, 489–506. doi: 10.1071/WR9920489
Limpus, C. J., and Walter, D. G. (1980). The growth of immature green turtles (Chelonia mydas) under natural conditions. Herpetologica 36, 162–165.
Locker, S., Doyle, L., and Logue, T. (2000). “Surface sediments of the NW Florida inner continental shelf: a review of previous results, assessment, and recommendations,” in Physical/Biological Oceanographic Integration Workshop for the DeSoto Canyon and Adjacent Shelf, eds W. W. Schroeder and C. F. Wood (Washington, DC: U.S. Department of the Interior).
Logan, J., Young, M., Harvey, E., Schimel, A., and Ierodiaconou, D. (2017). Combining underwater video methods improves effectiveness of demersal fish assemblage surveys across habitats. Mar. Ecol. Prog. Ser. 582, 181–200. doi: 10.3354/meps12326
Márquez, M. R. (1990). FAO Species Catalogue. Vol.11: Sea Turtles of the World. An Annotated and Illustrated Catalogue of Sea Turtle Species Known to Date. FAO Fisheries Synopsis No. 125, Vol. 11. Rome: FAO.
Mendonça, M. T., Ehrhart, L. M., and Mendonca, M. T. (1982). Activity, population size and structure of immature Chelonia mydas and Caretta caretta in mosquito lagoon, Florida. Copeia 1982:161. doi: 10.2307/1444280
Metz, T. L., and Landry, A. M. (2013). An assessment of green turtle (Chelonia mydas) stocks along tbe Texas Coast, witb emphasis on the lower Laguna Madre. Chelonian Conserv. Biol. 12:11. doi: 10.2744/CCB-1046.1
Morris, W. F., and Doak, D. F. (2002). Quantitative Conservation Biology. Sunderland, MA: Sinauer Associates.
National Research Council (U.S.), Committee on the Review of Sea-Turtle Population Assessment Methods, National Academy of Sciences (U.S.), Division on Earth and Life Studies, and Ocean Studies Board (2010). Assessment of Sea-Turtle Status and Trends: Integrating Demography and Abundance. Washington, DC: National Academies Press.
Nelson, B. (2017). Placement of Artificial Reef Modules off Mexico Beach Now Complete. In: Florida Fish and Wildlife Conservation Commission. Available online at: https://myfwc.com/news/all-news/artificial-reefs/ (accessed Sep 8, 2020).
O’Farrell, M. R., and Botsford, L. W. (2006). Estimating the status of nearshore rockfish (Sebastes Spp.) populations with length frequency data. Ecol. Appl. 16, 977–986. doi: 10.1890/1051-0761(2006)016[0977:ETSONR]2.0.CO;2
Phillips, K. F., Stahelin, G. D., Chabot, R. M., and Mansfield, K. L. (2021). Long-term trends in marine turtle size at maturity at an important Atlantic rookery. Ecosphere 12, 1–13 doi: 10.1002/ecs2.3631
Piacenza, S. E., Richards, P. M., and Heppell, S. S. (2019). Fathoming sea turtles: monitoring strategy evaluation to improve conservation status assessments. Ecol. Appl. 29:e01942. doi: 10.1002/eap.1942
R Development Core Team (2021). R: A Language and Environment for Statistical Computing. Vienna: R Foundation for Statistical Computing.
Redfoot, W., and Ehrhart, L. (2013). Trends in size class distribution, recaptures, and abundance of juvenile green turtles (Chelonia mydas) utilizing a rock riprap lined embayment at port Canaveral, Florida, USA, as developmental habitat. Chelonian Conserv. Biol. 12, 252–261. doi: 10.2744/CCB-0952.1
Rudd, M. B., and Thorson, J. T. (2018). Accounting for variable recruitment and fishing mortality in length-based stock assessments for data-limited fisheries. Can. J. Fish. Aquat. Sci. 75, 1019–1035. doi: 10.1139/cjfas-2017-0143
Santana-Garcon, J., Newman, S. J., and Harvey, E. S. (2014). Development and validation of a mid-water baited stereo-video technique for investigating pelagic fish assemblages. J. Exp. Mar. Biol. Ecol. 452, 82–90. doi: 10.1016/j.jembe.2013.12.009
Schroeder, B., and Murphy, S. (1999). “Population surveys (ground and aerial) on nesting beaches,” in Research and Management Techniques for the Conservation of Sea Turtles, eds K. L. Eckert, K. A. Bjorndal, F. A. Abreu-Grobois, and M. Donnelly (Gland: IUCN Marine Turtle Specialist Group).
SeaGIS (2008a). CAL. SeaGIS Pty, Bacchus Marsh. Available online at: www.seagis.com.au
SeaGIS (2008b). EventMeasure. SeaGIS Pty, Bacchus Marsh. Available online at: www.seagis.com.au
Siegfried, T. R., Fuentes, M. M. P. B., Ware, M., Robinson, N. J., Roberto, E., Piacenza, J. R., et al. (2021). Validating the use of stereo-video cameras to conduct remote measurements of sea turtles. Ecol. Evol. 11, 8226–8237. doi: 10.1002/ece3.7653
Silver-Gorges, I., Ceriani, S. A., Ware, M., Lamb, M., Lamont, M., Becker, J., et al. (2021). Using systems thinking to inform management of imperiled species: a case study with sea turtles. Biol. Conserv. 260:109201. doi: 10.1016/j.biocon.2021.109201
Summers, T. M., Jones, T. T., Martin, S. L., Hapdei, J. R., Ruak, J. K., and Lepczyk, C. A. (2017). Demography of marine turtles in the nearshore environments of the Northern Mariana Islands. Pacific Sci. 71, 269–286. doi: 10.2984/71.3.3
van Dam, R. P., and Diez, C. E. (1998). Home range of immature hawksbill turtles (Eretmochelys imbricata (Linnaeus)) at two Caribbean islands. J. Exp. Mar. Biol. Ecol. 220, 15–24. doi: 10.1016/S0022-0981(97)00080-4
White, M., Boura, L., and Venizelos, L. (2013). Population structure for sea turtles at drini bay: an important nearshore foraging and developmental habitat in Albania. Chelonian Conserv. Biol. 12, 283–292. doi: 10.2744/CCB-1002.1
Wildermann, N., Gredzens, C., Avens, L., Barrios-Garrido, I., Bell, J., Blumenthal, A. B., et al. (2018). Informing research priorities for immature sea turtles through expert elicitation. Endanger. Species Res. 37, 55–76. doi: 10.3354/esr00916
Wildermann, N., Sasso, C., Gredzens, C., and Fuentes, M. (2020). Assessing the effect of recreational scallop harvest on the distribution and behaviour of foraging marine turtles. Oryx 54, 307–314. doi: 10.1017/S0030605318000182
Keywords: green sea turtle, loggerhead sea turtle, Kemp’s ridley sea turtle, abundance, size distribution, photogrammetry, stereo-video camera system
Citation: Siegfried T, Noren C, Reimer J, Ware M, Fuentes MMPB and Piacenza SE (2021) Insights Into Sea Turtle Population Composition Obtained With Stereo-Video Cameras in situ Across Nearshore Habitats in the Northeastern Gulf of Mexico. Front. Mar. Sci. 8:746500. doi: 10.3389/fmars.2021.746500
Received: 23 July 2021; Accepted: 23 September 2021;
Published: 15 October 2021.
Edited by:
Kristen Marie Hart, United States Geological Survey (USGS), United StatesReviewed by:
Roldan A. Valverde, Southeastern Louisiana University, United StatesWendy Piniak, National Oceanic and Atmospheric Administration (NOAA), United States
Copyright © 2021 Siegfried, Noren, Reimer, Ware, Fuentes and Piacenza. This is an open-access article distributed under the terms of the Creative Commons Attribution License (CC BY). The use, distribution or reproduction in other forums is permitted, provided the original author(s) and the copyright owner(s) are credited and that the original publication in this journal is cited, in accordance with accepted academic practice. No use, distribution or reproduction is permitted which does not comply with these terms.
*Correspondence: Tabitha Siegfried, VHNpZWdmcmllZDk4QGdtYWlsLmNvbQ==