- 1CAS and Shandong Province Key Laboratory of Experimental Marine Biology, Center for Ocean Mega-Science, Institute of Oceanology, Chinese Academy of Sciences, Qingdao, China
- 2Laboratory for Marine Fisheries Science and Food Production Processes, Pilot National Laboratory for Marine Science and Technology, Qingdao, China
- 3University of Chinese Academy of Sciences, Beijing, China
- 4Laboratory for Marine Biology and Biotechnology, Pilot National Laboratory for Marine Science and Technology, Qingdao, China
- 5National and Local Joint Engineering Laboratory of Ecological Mariculture, Institute of Oceanology, Chinese Academy of Sciences, Qingdao, China
Understanding the genetic basis of trait variations and their coordination between relative species or populations distributing in different environmental conditions is important in evolutionary biology. In marine ectotherms, growth-defense trade-offs are a common ecological and evolutionary phenomenon. However, the biochemical and molecular mechanisms that govern these trade-offs in marine ectotherms in the evolutionary perspective remain poorly investigated. Oysters are among the most important species in global aquaculture. Crassostrea gigas (C. gigas) and Crassostrea angulata (C. angulata) are two allopatric congeneric dominant oyster species that inhabit the northern and southern intertidal areas of China. Wild C. gigas and C. angulata were spawned, and their F1 progeny were cultured in the same sites to reduce the environmental effects. Untargeted metabolomics and transcriptomics, together with phenotypic parameters including morphological traits (growth performance), nutritional content (glycogen, crude fat, and fatty acid content), physiology (normalized oxygen consumption rate and total antioxidant capacity) were applied to assess metabolic and transcript divergences between C. gigas and C. angulata. Integrated analyses of metabolites and transcriptomes showed that C. gigas allocated more energy to storage and defense by suppressing glycolysis, fatty acid oxidation and by upregulating fatty acid synthesis, antioxidant gene expression, and related metabolites. The metabolic and transcript results were further confirmed by the phenotypic data that C. gigas has higher glycogen and crude fat content and fatty acid unsaturation and stronger antioxidant capacity than C. angulata. In contrast, C. angulata exhibited better growth performance and a higher oxygen consumption rate. These findings suggest that C. angulata allocates more energy to growth, which is embodied in its stronger aerobic capacity and higher levels of protein synthesis genes, metabolites, and growth-related biomarkers. This study will help to enlighten the evolutionary patterns and genetic basis of growth-defense trade-offs in marine ectotherms and the biochemical and molecular mechanisms underlying energy allocation. Also, the key genes and metabolites of glycogen and fatty acids pathway identified in this study will be applied for meat quality improvement in the oyster industry.
Introduction
Understanding the genetic divergence between relative species or different populations that are distributed in different environments strongly influences our views on adaptation, speciation, and geographic distribution (Metcalfe and Monaghan, 2001; Ma et al., 2019; Matos et al., 2020). It has long been recognized that populations and relative species occupying different environments vary in their fitness-related traits, including those related to growth, survival, defense, and physiology (Prunier et al., 2012; Yuan et al., 2016; Zuniga-Vega et al., 2017; Pigot et al., 2020). Fortunately, common garden experiments, which used to alleviate environmental effects through culturing individuals from different genetic background under identical conditions, can reveal the genetic influences that shape phenotypic variation and the coordination of various traits (Kelly, 2019; Ma et al., 2019).
Trade-offs between traits are common ecological and evolutionary phenomenon in nature, as they ensure the best use of outside resources (Wang J. et al., 2021). Trait trade-offs are particularly common in plants and sessile marine ectotherms because these types of organisms cannot shift their locality to avoid environmental variation (Karasov et al., 2017; Zust and Agrawal, 2017; Chauvin et al., 2018; Min et al., 2018; Caignard et al., 2021). Plenty of studies demonstrated that the trade-offs between reproduction, growth, and survival are the key forces that structure life-history variation (Culina et al., 2019; Cohen et al., 2020; Roach and Smith, 2020). This has been validated in many plants, such as ribwort plantain (Plantago lanceolata) (Quarles and Roach, 2019), alpine wildflower (Polemonium viscosum) (Galen, 2000), orchids (Sletvold and Agren, 2015), and Oenothera L. (Vilela et al., 2008), as well as in marine ectotherms such as octocoral (Paramuricea clavata) (Linares et al., 2008), mussels (Petes et al., 2008), the Pacific oyster (Crassostrea gigas) (Ernande et al., 2004), Northeast Arctic cod (Gadus morhua) (Folkvord et al., 2014), and wavy-turban snails (Megastraea undosa) (Martone and Micheli, 2012). In addition to the cost of reproduction related to life-history trade-offs (Petes et al., 2008; Wingfield and Sapolsky, 2010), the energy allocation and associated physiological costs related to growth-defense trade-offs also play a major role in shaping large-scale geographic distribution and functional trait variation (Stier et al., 2014; Min et al., 2018). In this regard, there are ample experimental evidences available regarding growth-defense trade-offs (Roach and Smith, 2020; Coll et al., 2021), particularly for plants, such as Leymus chinensis (Min et al., 2018), Douglas-fir (Darychuk et al., 2012), and sunflower (Mayrose et al., 2011). Additionally, it also has been extensively observed in marine shellfish, such as mussels (Petes et al., 2008; Sherker et al., 2017), oysters (Crassostrea gigas) (Ernande et al., 2003), and intertidal snails (Littorina obtusata) (Trussell, 2000).
Many studies have suggested that an increased investment in body size leads to a reduction in individual-level energy allocation toward defense against enemies and environmental stress (Albrecht and Argueso, 2017; Zust and Agrawal, 2017; Gallardo-Hidalgo et al., 2021). Additionally, the allocation of energy toward growth occurs at the expense of other life-history components (Metcalfe and Monaghan, 2001). In particular, the energy invested in growth seems to be balanced against allocation toward physical self-maintenance processes (Lee et al., 2013; Lind et al., 2013). Therefore, energy allocation is an essential driving mediator for the coupling of growth and defense (Sokolova et al., 2012). The biochemical and molecular mechanisms underlying the growth-defense trade-offs that are mediated by energy allocation have been widely explored in plants (Karasov et al., 2017; Zust and Agrawal, 2017; Min et al., 2018). Many pathways for these mechanisms, such as antagonistic crosstalk among hormones, have been identified (Karasov et al., 2017; Cortleven et al., 2019; Margalha et al., 2019). A large number of growth-defense trade-offs have also been observed in marine ectotherms, such as ark shells (Scapharca subcrenata) (Jiang et al., 2020), sea cucumbers (Huo et al., 2019), blue crabs (Callinectes sapidus), clams (Venus verrucosa) (Feidantsis et al., 2021), and oysters (Crassostrea gigas) (Li et al., 2018). However, in marine ectotherms, the molecular mechanisms that control growth-defense trade-offs, the theoretical basis of traits, and the metabolic energy compensation in relative species are still poorly understood from the evolutionary perspective. Therefore, regulation of energy expenditure between species and the relative species distributing in various environments and its link with growth-defense trade-offs deserve to be studied further.
Oysters are common marine invertebrates that inhabit intertidal zones, which are the most physically demanding habitats on earth because of the existence of extreme temperatures, drought and starvation (Judge et al., 2018). Therefore, intertidal marine ectotherms have long been considered as ideal study subjects for quantifying the relationship between physiological variation and differential energy allocation in specimens that experience environmental variation (Sebens, 2002; Guofan et al., 2012). Oysters are among the most important species in global aquaculture. Crassostrea gigas is native to East Asia, now became major economic specie worldwide (Guo, 2009). And Crassostrea angulata distributes mainly in China, Vietnam, Europe (Guo et al., 2018). In China, C. gigas and C. angulata are the most two cultured oyster species and two allopatric congeneric dominant oyster species that inhabit in the northern and southern coast (Wang et al., 2010). Based on data, the production of two species accounts for more than 60% of the total oyster production (Fisheries and Fisheries Administration of the Ministry of Agriculture and Rural Affairs, 2020). Phylogenetic analysis has revealed that they diverged approximately 2.7 Mya (Ren et al., 2010). It has been reported that C. angulata grows faster than C. gigas in the first year of development, regardless of the location in which they are reared (Guo et al., 1999). Previous studies have demonstrated that the two species show divergence of multiple traits, including growth, survival following heat shock, respiration rate, metabolism-related gene expression, and nutrient content (Li A. et al., 2017; Li et al., 2018, 2019; Ghaffari et al., 2019). The balance of growth, defense, and energy metabolism may play an important role in the adaptive evolution between the two species. However, the coordination between the multiple traits have not been fully investigated, and the understanding of the related biochemical and molecular mechanisms remains poorly explored.
In recent years, rapid advances in technology have made next-generation sequencing suitable to conduct large-scale research to identify candidate genes related to trait variations (Li Z. et al., 2021; Wang X. et al., 2021). However, trait changes are not only regulated by specific genes but also regulated at multiple levels, including at the metabolite level. Metabolomics, in combination with the transcriptomics, can further confirm the critical pathways responsible for regulating specific traits by quantifying the real metabolites to explore physiological and biochemical status of biosystem. Recently, the integration of metabolomics and transcriptomics has been extensively applied in aquatic organisms to study the genetic basis of glycogen content in the Pacific oyster (C. gigas) (Li B. et al., 2017), unsynchronized growth and the larval metamorphosis in pearl oyster (Pinctada fucata martensii) (Hao et al., 2019; Zhang J. et al., 2021), and responses to abiotic stress in kuruma shrimp (Marsupenaeus japonicus) (Ren et al., 2020) and biotic stress in mud crab (Scylla paramamosain) (Kong et al., 2020).
In this study, we utilized an untargeted metabolome and transcriptome analysis in attempt to reveal the energy allocation that underlies the growth-defense trade-offs between C. gigas and C. angulata, which were sampled in situ following a common garden culture. Growth data, glycogen, crude fat, fatty acid contents, the normalized oxygen consumption rate, total antioxidant capacity, and anti-oxidative gene expression were determined to further validate biochemical and molecular findings. This study will provide new insights into the biochemical and molecular mechanisms underlying energy allocation-mediated growth-defense trade-offs and highlights the significance of an integrated approach for studying this topic.
Materials and Methods
Experimental Animals
The experimental design of spawning referred to our previous study (Li A. et al., 2017). In short, we collected two congeneric oyster species, C. gigas and C. angulata, from their natural habitat in Qingdao (35°44′ N) and Xiamen (24°33′ N), respectively. According to our measurements, the mean air and sea surface temperatures at the southern site were 8.7°C and 6.6°C higher than those at the northern site over the past 2 years, respectively (Li A. et al., 2017). To alleviate environmental affect, we conducted a one-generation common garden experiment (Sanford and Kelly, 2011). For each species, we mixed eggs from 30 mature females and divided them into 30 beakers. Sperm from each of the 30 mature males was crossed separately with the eggs in each beaker. Zygotes were fertilized and larvae were cultured in indoor hatchery of Laizhou city (37°18′N, Shandong province, China). The fertilization was conducted in a 100 L bucket at 20 ∼ 22°C and salinity for 28 ∼ 30‰. The larvae were cultured in 20 m3 indoor pond at 22 ∼ 24°C and 28 ∼ 30‰ for salinity, feeding with 1.5 L 30,000 ∼ 40,000 cells/ml mixed algae [golden algae (Chrysophyta) and Platymonas subcordiformis] five times a day. One month later, 3-month intermediate rearing at the sea of Laizhou city were conducted, during which the cultured density was decreased gradually. Then, 4-month-old F1 progenies were transported to the sea of Muping city (37°39′N, Shandong province, China) for grow-out culture. After 2 months of acclimatization, we sampled 15 adult progeny oysters, gill tissues, and other tissues of each species in situ for subsequent experiments. In addition, we collected C. gigas and C. angulata from Muping and brought them back to Qingdao to measure their oxygen consumption rates.
RNA Sequencing Data Analysis
The gill tissues were used for RNA extraction. To increase the accuracy of the sequencing, we increased the sample number to eight individuals of each species. RNA from the 16 oyster tissues were extracted using TRIzol (Invitrogen, United Kingdom) for RNA sequencing by BMK (Biomarker Technologies, China). Each sample was used to generate an independent library with NEBNext UltraTM RNA Library Prep Kit for Illumina (NEB, United States). RNA was enriched using Oligo(dT) beads. Then, the enriched mRNA was divided into short fragments using a fragmentation buffer, and it was reverse transcribed into cDNA using random primers. Second-strand cDNA was synthesized using DNA polymerase I, RNase H, dNTP, and buffer solution. Afterward, the cDNA fragments were purified using AMPure XP beads (Beckman Coulter, Beverly, MA, United States), the ends were repaired, poly(A) tails were added, and the fragments were ligated with Illumina adapter sequences. The ligation products were size-selected using AMPure XP beads, amplified by PCR, and sequenced using an Illumina NovaSeq 6000. To obtain high-quality clean reads, we filtered the reads by removing the adapter sequences, reads with >10% unknown nucleotides (N), and those with >50% low-quality bases (Q-value ≤ 20).
Read Mapping and Gene Expression Calculation
The HISAT2 v2.0.4 package (Kim et al., 2015) was used to map reads to the oyster genome (GenBank accession No., GCA_011032805.1) with a parameter setting of “–dta -p 6 –max-intronlen 5000000.” Then, the SAM files were converted to BAM files using SAMtools v0.1.18 (Li et al., 2009). The StringTie v1.3.4d package (Pertea et al., 2015) was used to process the read alignments and annotations with a parameter setting of “–merge -F 0.1 -T 0.1.” StringTie was then used to estimate abundance and create a new transcript table for input to the R package “ballgown” (Pertea et al., 2016). Gene expression levels were quantified using “ballgown” based on the expected number of fragments per kilobase of transcript sequence per million base pairs sequenced (FPKM) method (Florea et al., 2013).
Analysis of Differentially Expressed Genes
Differential expression analysis of C. gigas and C. angulata was performed using the “DESeq2” R package (version 1.6.3) (Love et al., 2014). The Benjamini-Hochberg method was used to control the false discovery rate (FDR) by adjusting the P-values. Genes with FDR ≤ 0.01 and a log2 fold change (FC) of |log2FC| > 1 were identified as differentially expressed genes (DEGs). Principal component analysis (PCA) and hierarchical cluster analysis (HCA) were performed using the “ropls” R package (version 3.3.2). The DEGs were enriched by Gene Ontology (GO) and Kyoto Encyclopedia of Genes and Genomes (KEGG) using the clusterProfiler R package (version 3.10.1) (Yu et al., 2012). GO is an international standardized gene function classification system consisting of terms that use controlled vocabulary to provide a comprehensive representation of gene function. KEGG is a major public pathway-related database (Kanehisa et al., 2008) that can identify significantly enriched metabolic pathways in DEGs from the background of the whole genome1. DEGs with a Q-value ≤ 0.05 were considered to be significantly enriched pathways.
Metabolite Extraction and Detection
Twenty gill tissues (i.e., ten from C. gigas and ten from C. angulata) were used for metabolite extraction and detection. Metabolites were extracted according to the protocol described by BMK (Biomarker Technologies, China). Approximately (50 ± 1 mg) of each oyster tissue was extracted using 1 mL extraction liquid (VMethanol:VAcetonitrile:VWater = 2:2:1) and 2 μL 2-Chloro-L-phenylalanine, which was used as an internal standard. The extract was vortexed for 30 s. Subsequently, the extract was homogenized using a ball mill for 10 min at 45 Hz and then ultrasonicated for 5 min at 4°C. The solution was incubated for 1 h at −20°C. Then, the mixture was centrifuged for 15 min at 12,000 rpm and 4°C. Approximately 500 μL of the supernatant was transferred into 1.5 mL microcentrifuge tubes. The extracts were dried completely in a vacuum concentrator without heating. Then, 160 μL of extraction liquid (VAcetonitrile:VWater = 1:1) was added to the dried metabolites, vortexed for 30 s, and ultrasonicated for 15 min at 4°C. The solution was centrifuged for 15 min at 12,000 rpm and 4°C. Approximately 120 μL of the supernatant was transferred into 2 mL sample bottles, and then 10 μL of the extract from each sample was mixed with a QC sample for detection. All samples were analyzed using a liquid chromatograph coupled with a quadrupole time-of-flight mass spectrometer (LC-QTOF-MS).
LC-QTOF-MS analysis was performed using an Acquity UPLC I-Class PLUS liquid chromatograph system (Waters, Milford, MA, United States) and a Waters UPLC Xevo G2-XS QTof mass spectrometer. Each sample was injected into an Acquity UPLC HSS T3 column (1.8 μm, 2.1 mm × 100 mm; Waters, Milford, MA, United States). The column was maintained at 50°C at a flow rate of 0.4 mL/min. The mobile phases consisted of (A) formic acid:water (1:999, v/v) and (B) formic acid:acetonitrile (1:999, v/v). The chromatographic separation was obtained using the following gradient: 0–0.25 min, 98% A and 2% B; 0.25–10 min, 98% A and 2% B; 10–13 min, 2% A and 98% B; 13–13.1 min, 2% A and 98% B; and 13.1–15 min, 98% A and 2% B. The analytical setup was operated in both positive and negative ion modes with the following parameters: capillary voltage of 2,000 V for positive ion mode and −1,500 V for negative ion mode; cone voltage, 30 V; source temperature, 150°C; desolvation temperature, 500°C; desolvation gas flow, 500 L/h; cone gas flow, 50 L/h.
Progenesis QI software (Waters, Milford, MA, United States), the METLIN database (Want, 2005) and the BMK Self-built Database (Biomarker Technologies, China) were used for raw peak exacting, data baseline filtering, calibration of the baseline, peak alignment, deconvolution analysis, peak identification, and integration of the peak area.
Analysis of Significantly Different Metabolites
Principal component analysis and orthogonal projections to latent structures–discriminate analysis (OPLS-DA) were conducted using the “ropls” R package (version 3.3.2). PCA was used to determine the distribution of the original data. OPLS-DA was performed to obtain group separation and identify the most discriminant variables. A permutation test was used to estimate the robustness and predictive ability of the model. Then, OPLS-DA was used to identify the significantly different metabolites between C. gigas and C. angulata. The variable importance for projection (VIP) values along the predictive component was obtained to explain the results. First, VIP values exceeding 1.0 were selected as changed metabolites. Then, a Student’s t-test was used to evaluate the remaining variables. The metabolites with P-values > 0.05 were discarded. Subsequently, the FC value of each metabolite was calculated to further filter the variables. Taken together, metabolites with VIP values > 1, P < 0.05, and | log2FC| > 1 were identified as significantly different metabolites (SDMs). The SDMs were enriched with KEGG using the “clusterProfiler” R package (version 3.10.1) (Yu et al., 2012).
Integrative Analysis of Metabolomes and Transcriptomes
Differentially expressed genes (FDR ≤ 0.01 and | log2FC| > 1) and SDMs (VIP > 1, P < 0.05, and | log2FC| > 1) were used for the integrative analysis of C. gigas and C. angulata. Pearson correlation coefficients were used to integrate metabolome and transcriptome data. A heatmap was used to show the connections between genes and metabolites.
Growth Performance Measurement
Growth parameters was measured before we sampled in situ. The shell length, width, and height of each sample were measured using a digital caliper (0.02 mm accuracy). The wet weight of each sample was measured using an electronic balance (0.01 g accuracy).
Nutrient Measurements
The glycogen content in the gill tissues of three oysters was determined using a kit for detecting liver and muscle glycogen content (Nanjing Jiancheng Bioengineering Institute, Nanjing, China). Soxhlet’s method was used to measure the crude fat content of all tissues excluding the gills from five oysters, and the fatty acid content and composition of these samples were measured using gas chromatography. The protocol was as follows: C19:0 fatty acid methyl ester (Sigma, United States) was added as an internal standard to the sample, and 0.01% butylhydroxytoluene (BHT) methanol solution was added as an antioxidant. After extracting the total fat using dichloromethane:methanol, the sample was dried using high-purity nitrogen. Then, 1 mL 0.5 M KOH methanol solution was added and the mixture was saponified in a water bath at 80°C for 2 h under the protection of nitrogen. After cooling, 1 mL of 14% BF3 methanol solution was added to the sample, and a methyl esterification reaction was carried out in a water bath at 80°C for 1 h. The fatty acid methyl esters were extracted using n-hexane. The sample volume was adjusted to 0.5 mL, and then the fatty acid composition and content was analyzed using an Agilent 7890A gas chromatograph (Agilent Technologies, United States). The chromatographic conditions were as follows: capillary column: DB-FFAP (30 m × 0.32 mm × 0.25 μm); inlet temperature: 220°C; detector temperature: 280°C; column temperature: Program heating 150°C (1 min), 3°C/min, 220°C (33 min).
Normalized Oxygen Consumption Rate Measurement
Ten 6-month-old individuals for each species were used for the normalized oxygen consumption rate measurements. To avoid the impact of periphyton, all of twenty oysters were cleaned and reared temporarily in a 500 L tank for 2 weeks. Averagely, 4 g/m3 commercial spirulina powder was added once per day as a food source (Zhao et al., 2021). An air pump (Super Silent Power Air Pump, China, V-10) was used to ensure a sufficient and stable oxygen supply.
The oysters were placed in sealed bottles (0.35 L) filled with air-saturated seawater. The temperature was regulated using a water bath at 20°C. The seawater was refreshed every 2 h and mixed using a magnetic stirring bar placed beneath the chamber to ensure adequate and uniform distribution of oxygen. The dissolved oxygen levels were measured using a ten-channel optical fiber oxygen meter (OXY-10 ST Prototype, PreSens Precision Sensing GmbH, Germany). The dissolved oxygen content was measured every 3 s using fiber optic probes. Due to the initial violent fluctuation of the dissolved oxygen and unstable water temperature, the data points obtained in the first 30 min were ignored. The subsequent 1,200 data points were used to obtain the fitted line and the slope coefficient K. The normalized oxygen consumption rate of the oysters was calculated as (K× V)/W, where V was the volume of the sealed bottle (L), K was the slope of the fitted line, and W was the dry weight (g) of the oysters (Zhao et al., 2021).
Antioxidant Genes Expression and the Total Antioxidant Capacity Measurement
Gills from 15 C. gigas and 15 C. angulata were sampled in situ, frozen in liquid nitrogen, and stored at −80°C for subsequent RNA extraction. RNA extraction was conducted using the TreliefTM RNAprep FastPure Tissue and Cell Kit (Tsingke Biotechnology, China). cDNA was synthesized using 1 μg of mixed RNA from three oysters, and qRT-PCR was performed using three technical replicates for each cDNA and five biological replicates for each group. The primers that were used are shown in Supplementary Table 1. The relative expression of antioxidant genes [glutathione S-transferase (GST), catalase (CAT), superoxide dismutase (SOD), glutathione (GSH), and xanthine dehydrogenase (XOD)] were determined using the 2–ΔΔCT method (Livak and Schmittgen, 2001). A comparison between the two species has been described in detail in a previous study (Li et al., 2019). The total antioxidant capacity in the gill tissues of five oysters was measured using a total antioxidant capacity assay kit (Nanjing Jiancheng Bioengineering Institute, Nanjing, China).
Statistical Analysis
All statistical analyses were performed with GraphPad Prism version 8.0.2 for Windows. Statistical analyses were conducted after confirming normality of the distributions using the Shapiro–Wilk test and homogeneity of variance using Bartlett’s test. The shell height, shell length, and shell width of C. gigas and C. angulata were compared using the Mann–Whitney U test. The comparison of wet weight, relative gene expression, fatty acid, crude fat, and glycogen content, oxygen consumption rate, and total antioxidant capacity between the two oyster congeners was carried out using an unpaired t-test. Significant differences between groups were marked with “∗” for P < 0.05, “∗∗” for P < 0.01, and “∗∗∗” for P < 0.001.
Results
Transcriptome Analysis of C. gigas and C. angulata
Based on the sequencing results of the cDNA from the 16 oysters representing the two species, an average of 41.3 and 44.7 million high-quality 150 bp paired-end reads (107.83 Gb reads in total) were mapped to the genomes of C. gigas (Supplementary Table 2). Quality analysis showed that C. gigas and C. angulata had an average guanine-cytosine content of 42.36 and 42.36% of clean reads, a Q30 of 93.52 and 93.43%, and a map rate of 72.63 and 69.63%, respectively (Supplementary Table 2). The data showed a high sequencing quality, indicating that the subsequent transcriptome analysis results were reliable. According to the HCA of gene expression, outlier samples of C. gigas-1 and C. gigas-7 were removed to obtain accurate results for differential genes (Supplementary Figure 1). The transcriptomes of the two oyster species were compared using PCA, which allowed the species to be visually and quantitatively compared in an unbiased format. The percentages of explained values of PC1 and PC2 in the transcriptome were 24.14 and 9.53%, respectively. Figure 1A shows that C. gigas and C. angulata were perfectly separated.
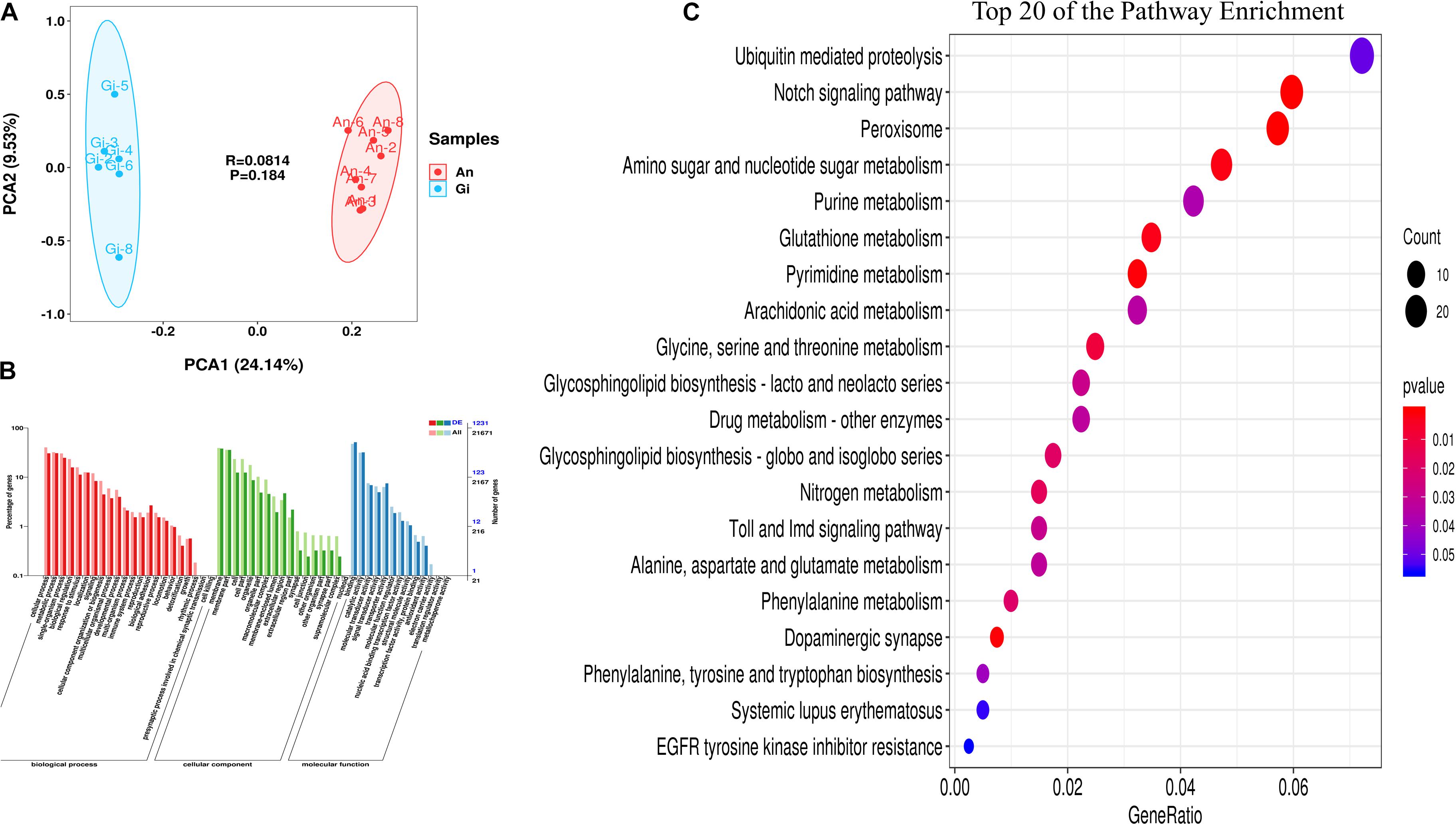
Figure 1. Transcriptome analysis of Crassostrea gigas and C. angulata. (A) Principle component analysis of genes differentially expressed between the two groups. The points represent scores of biological replicates (n = 14). (B) Gene Ontology (GO) terms enriched in genes that were differentially expressed between the two groups. The bars of the left Y-axis denote the proportion of the differentially expressed genes (DEGs) relative to the total number of genes in the C. gigas genome mapped to each term. The bars of the right Y-axis denote the number of the DEGs and the total genes. The red, green, and blue bars represent the GOTERM_BP, GOTERM_CC and, GOTERM_MF categories, respectively. (C) Scatterplot of the KEGG pathway enriched by the DEGs. The vertical axis represents the name of the pathway and the horizontal axis shows the gene ratio. The size of the plot denotes the number of DEGs while the color corresponds to the P-value. A deeper color represents a smaller P-value and indicates a more significant pathway enrichment.
A total of 2,006 DEGs were identified between the two oyster species (FDR ≤ 0.01 and | log2FC| > 1). The expression levels of these genes are shown in Supplementary Table 3. Compared with C. angulata, a total of 935 genes were upregulated and 1,071 genes were downregulated in C. gigas.
The DEGs were annotated using GO and KEGG. The majority (1,231) of the DEGs were mapped to at least one GO term, and 52 GO terms were significantly enriched. Among these, “cellular process,” “metabolic process,” “membrane,” “membrane part,” “binding,” and “catalytic activity,” were the most strongly represented (Figure 1B).
Pathway enrichment analysis identified significantly enriched metabolic pathways in the DEGs and showed significantly altered pathways in the transcriptome. We mapped all DEGs to KEGG pathways, and 17 significant specific pathways (P < 0.05) were found (Supplementary Table 5 and Figure 1C). Among these, “peroxisome,” “pyrimidine metabolism,” “glutathione metabolism,” “glycine, serine, and threonine metabolism,” “phenylalanine metabolism,” and “alanine, aspartate, and glutamate metabolism” were the most represented pathways. Enrichment of the antioxidant and amino acid metabolism pathways showed that the two oyster species may exhibit differences in stress resistance and protein synthesis.
Metabolome Analysis of C. gigas and C. angulata
A total of 3,149 metabolites (2,464 in positive and 685 in negative ionization modes) were identified using the LC-QTOF-MS platform. Gross changes in metabolite composition were determined using PCA and HCA. The percentages of explained values in the metabolome analysis of PC1 and PC2 were 62.52 and 11.3% in positive ionization mode and 47.51 and 18.06% in negative ionization mode, respectively. Based on the PCA (Supplementary Figures 2A,B) and the heatmap analysis (Supplementary Figures 2C,D), there was a slight separation between C. gigas and C. angulata. To maximize the discrimination between the two species, we used OPLS-DA to elucidate the different metabolic patterns. The parameters of the models were R2X = 0.492 and 0.637, R2Y = 0.965 and 0.961, and Q2 = 0.784 and 0.86 for positive and negative ionization modes, respectively (Figures 2A,B). All of them displayed an adequate goodness-of-fit and reliable prediction. In addition, pQ2 = 0.005 and pR2Y = 0.005 for the two modes, which demonstrated the robustness of the models, low overfitting, and low reliability risks (Figures 2C,D). All samples in the score plots were within the 95% Hotelling’s T-squared ellipse, and effective discrimination and separation were found between C. gigas and C. angulata. The OPLS-DA model was determined to be useful for subsequent analyses.
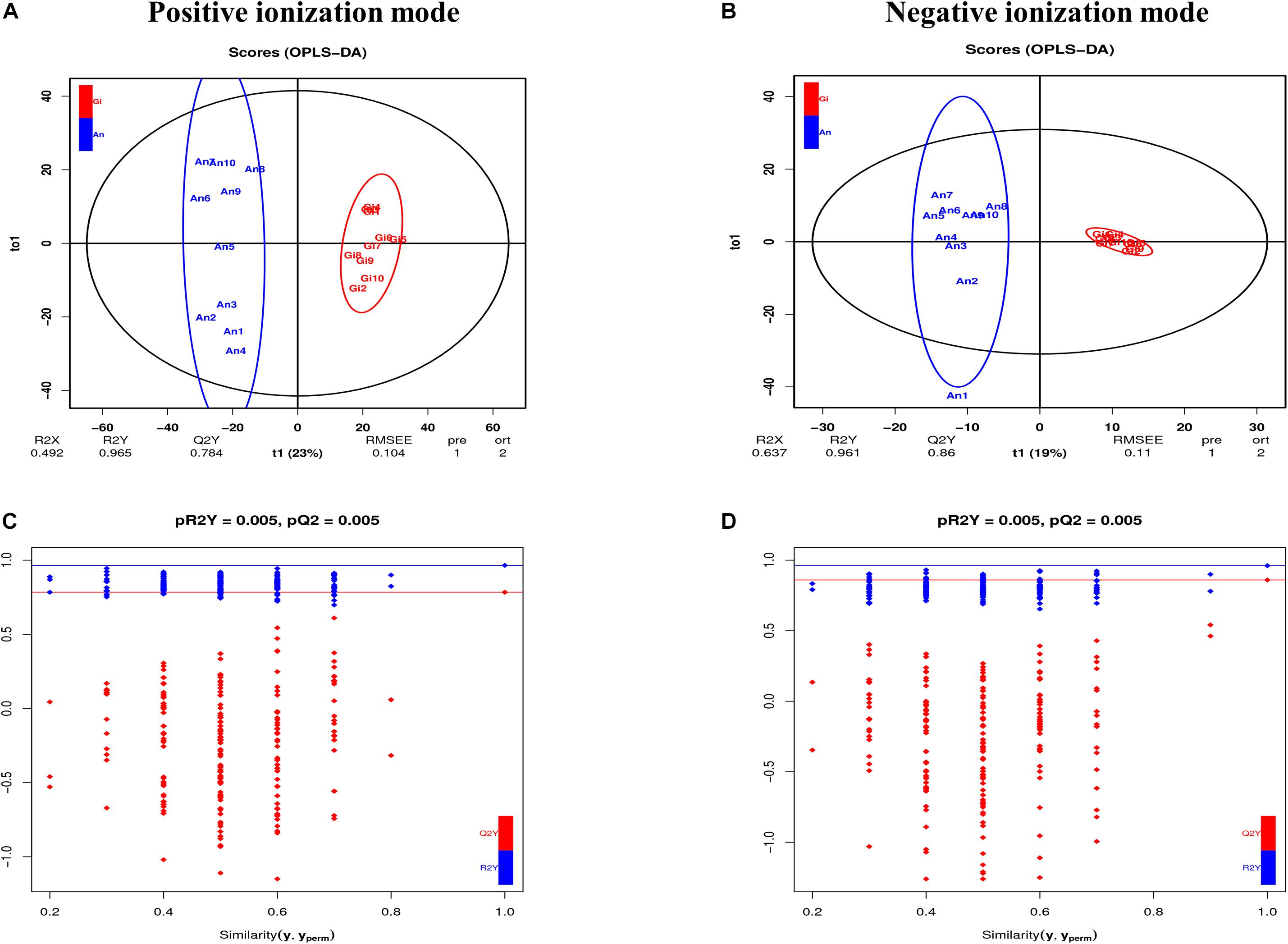
Figure 2. (A) OPLS-DA score plots in the positive ionization mode. (B) OPLS-DA score plots in the negative ionization mode. (C) Validation plots of OPLS-DA in the positive ionization mode. (D) Validation plots of OPLS-DA in the negative ionization mode.
In total, 1,312 (1,094 in positive and 218 in negative ionization modes) SDMs were identified between the two oyster species (P-value ≤ 0.05, VIP > 1, and | log2FC| > 1) (Supplementary Table 6). In the C. gigas group, 157 and 29 SDMs were significantly upregulated in the positive and negative ionization modes, respectively, and 937 and 189 SDMs were downregulated compared with the C. angulata group (Supplementary Table 6). A total of 189 pathways (135 in positive and 54 in negative ionization modes) were identified when the SDMs between C. gigas and C. angulata were introduced into KEGG (Supplementary Table 7). Based on the P-values and metabolite ratio, we identified that the most significantly enriched pathways were “carbon metabolism,” “purine metabolism,” “bile secretion,” “arachidonic acid metabolism,” “alpha-linolenic acid metabolism,” and “glutathione metabolism” (Figures 3A,B).
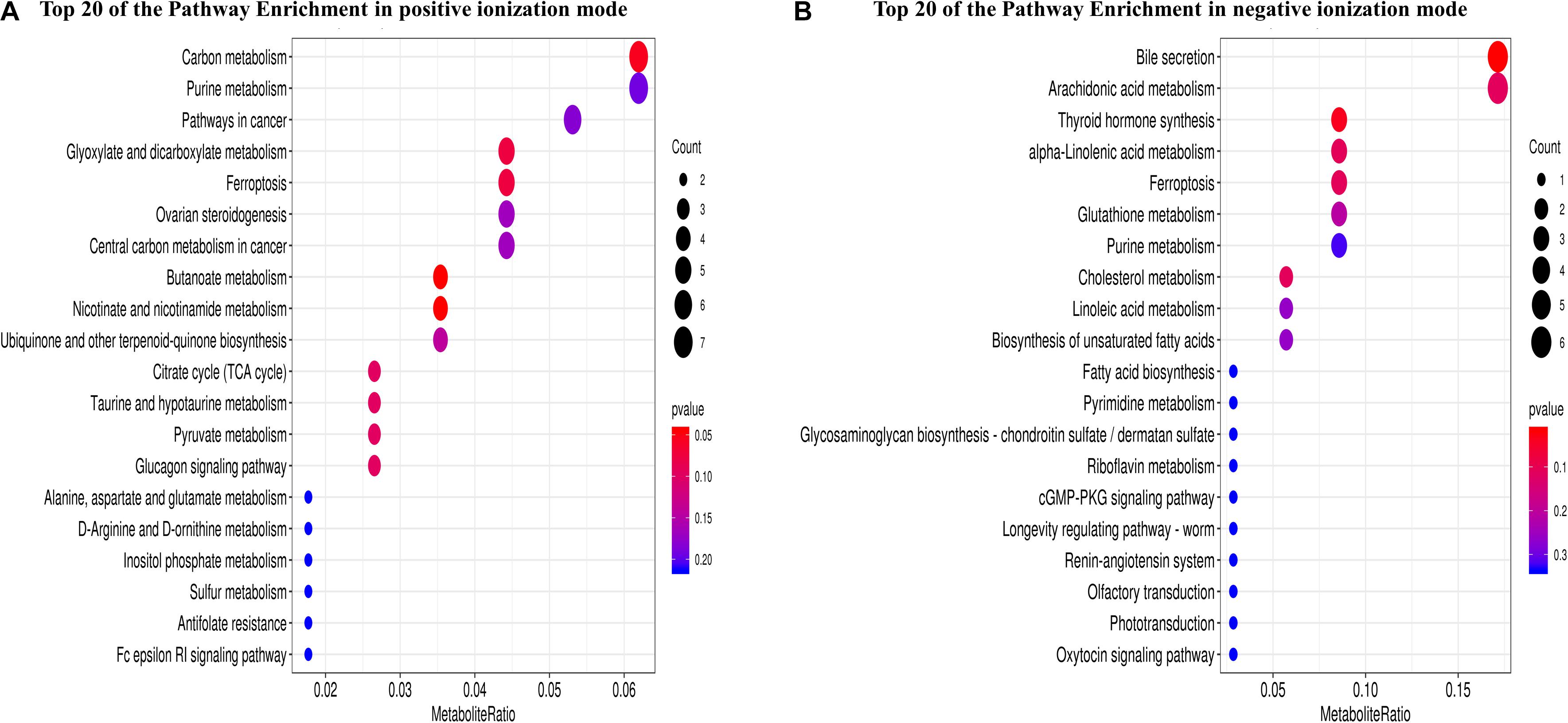
Figure 3. Metabolome analysis of Crassostrea gigas and C. angulata, represented as scatterplots of the KEGG pathway enriched by the significantly different metabolites. The vertical axis represents the name of the pathway and the horizontal axis shows the metabolite ratio. The size of the plot denotes the number of significantly different metabolites, while the color corresponds to the P-value. A deeper color represents a smaller P-value and indicates a more significant enrichment of the pathway. (A) Positive ionization mode. (B) Negative ionization mode.
Integrated Analysis of the Metabolome and Transcriptome
Pearson correlation coefficients were used to display the correlation between the transcriptome and the metabolomic data. An overview of the DEGs and metabolites found in C. gigas and C. angulata is shown in Figure 4. The pathways that were enriched between the two groups are shown in Supplementary Figures 3A,B.
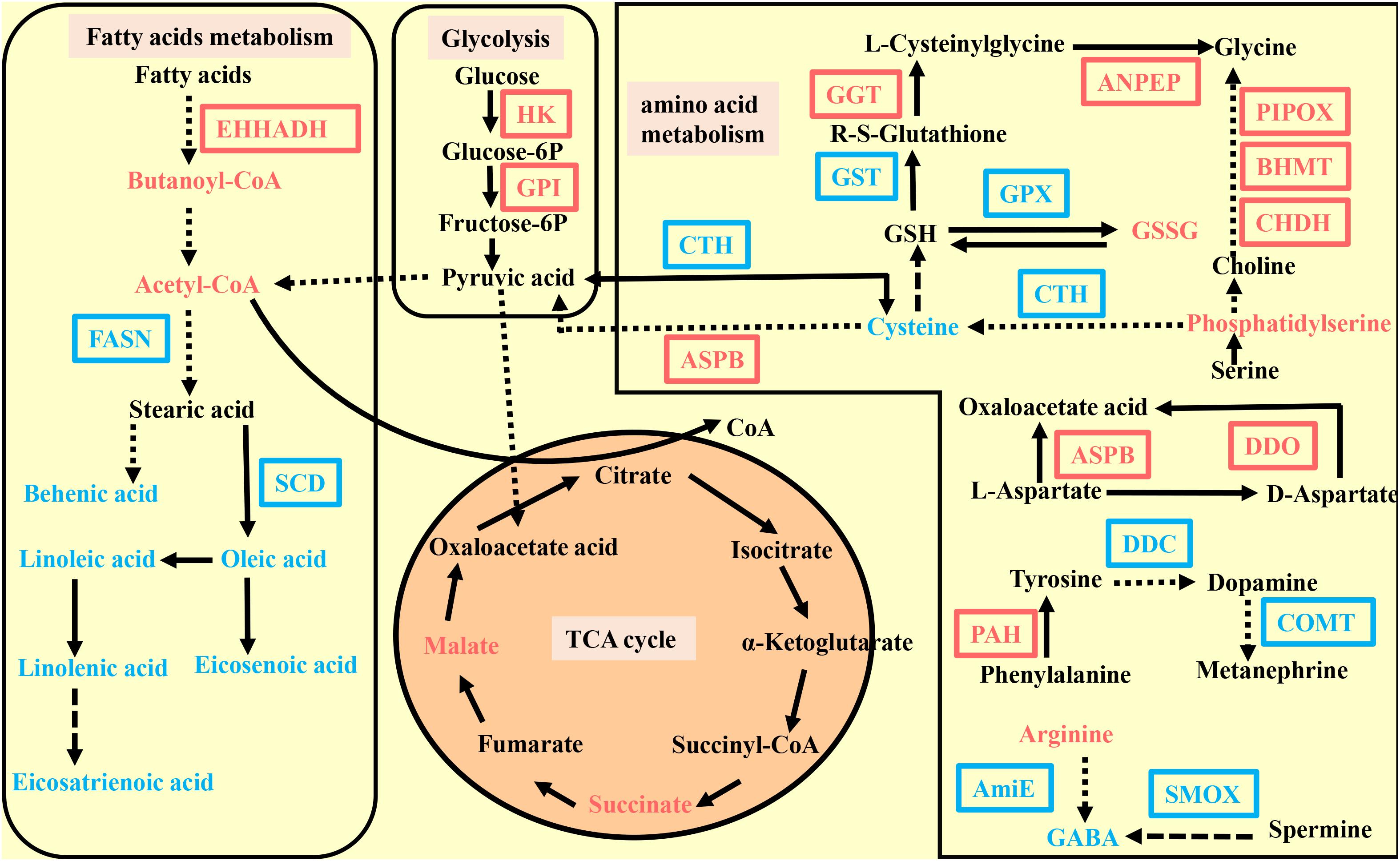
Figure 4. Pathway overview enriched by the metabolome and transcriptome in Crassostrea gigas and C. angulata. The differentially expressed gene abbreviations identified by the transcriptome analysis are shown in either blue (highly expressed in C. gigas) or red (highly expressed in the C. angulata) boxes. Differentially abundant metabolites identified by the metabolome analysis are shown either in blue (highly expressed in C. gigas) or red (highly expressed in C. angulata) letters. Solid arrows represent a direct effect, and dotted arrows an indirect effect. EHHADH, peroxisomal bifunctional enzyme; FASN, fatty acid synthase; SCD, stearoyl-CoA desaturase; HK, hexokinase; GPI, glucose-6-phosphate isomerase; GGT, gamma-glutamyl transpeptidase; ANPEP, aminopeptidase N; GST, glutathione S-transferase; GPX, glutathione peroxidase; BHMT, betaine-homocysteine S-methyltransferase; PIPOX, sarcosine oxidase; CHDH, choline dehydrogenase; ASPB, aspartate aminotransferase; DDC, aromatic-L-amino-acid decarboxylase; COMT, catechol O-methyltransferase; PAH, phenylalanine-4-hydroxylase; AMIE, amidase; SMOX, spermine oxidase; DDO, D-aspartate oxidase; CYP2U, long-chain fatty acid omega-monooxygenase; CYP2J, cytochrome P450 family 2 subfamily J; ALOX15B, arachidonate 15-lipoxygenase.
We found that the glycolysis pathway was downregulated in C. gigas (Figure 4). Hexokinase (HK) and glucose-6-phosphate isomerase (GPI) were significantly downregulated in C. gigas (Supplementary Table 4). Additionally, acetyl-CoA, an important oxidation product of pyruvic acid that enters the tricarboxylic acid (TCA) cycle (the final product in the glycolysis pathway), was also downregulated in C. gigas (Supplementary Table 6). HK phosphorylates glucose to produce glucose-6-phosphate, which is the first essential step in most glucose metabolism pathways. This phosphorylation event directly associates extramitochondrial glycolysis with intramolecular oxidative phosphorylation. GPI interconverts glucose-6-phosphate and fructose-6-phosphate, which is the second step in glycolysis.
We also found that the gene expression of enoyl-CoA hydratase and 3-hydroxyacyl CoA dehydrogenase (EHHADH) and butanoyl-CoA in the fatty acid degradation pathway were lower in C. gigas than in C. angulata (Figure 4 and Supplementary Tables 4, 6). In addition to the fatty acid degradation pathway, the fatty acid synthesis pathway was upregulated along with high expression of fatty acid synthase (FASN) and high contents of behenic acid (C22:0) and pentadecanoic acid (C15:0) (Figures 4, 5C and Supplementary Table 4). Moreover, stearoyl-CoA desaturase (SCD) involved in fatty acid desaturation pathway was also higher in C. gigas (Figure 4 and Supplementary Table 4).
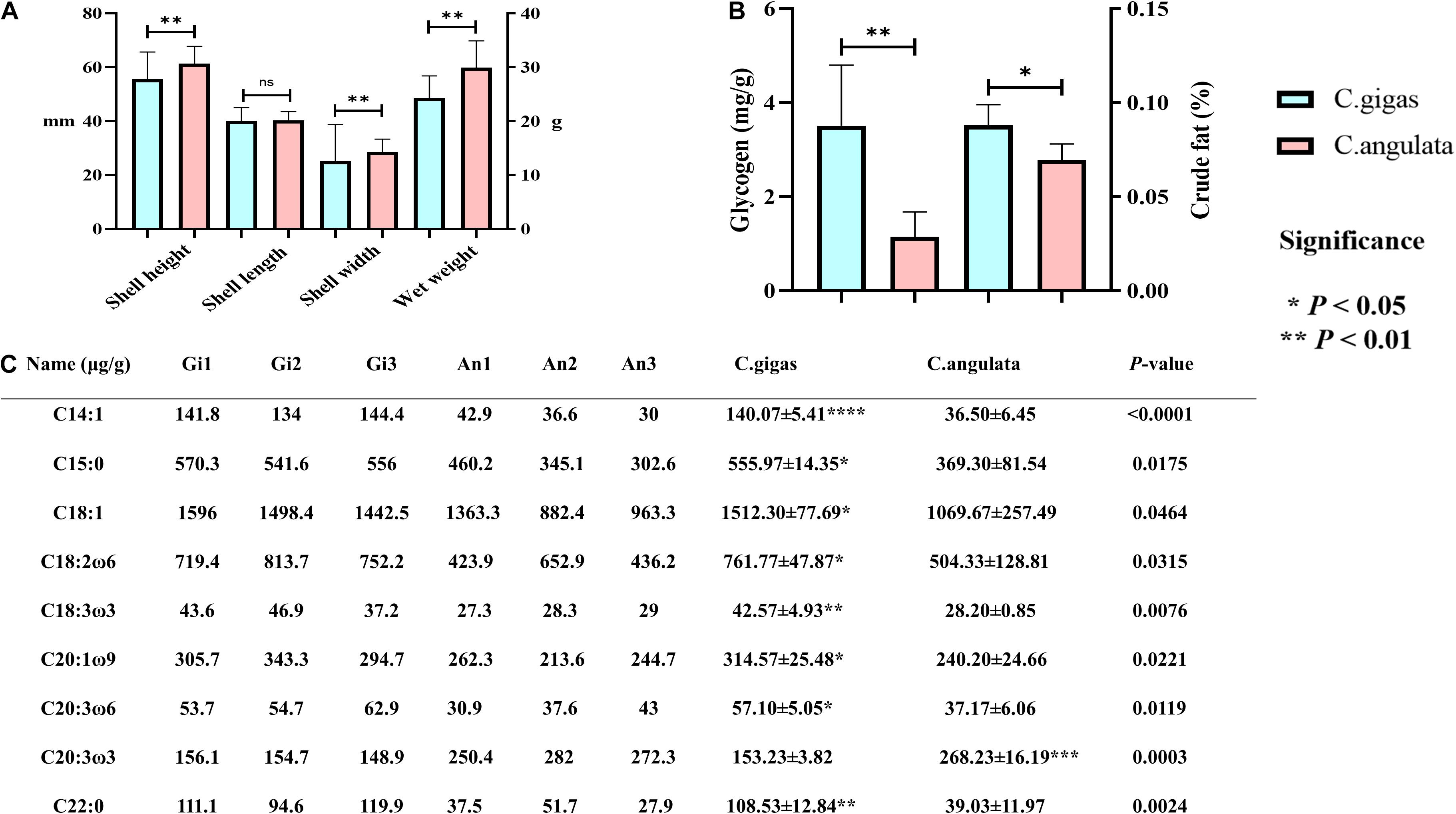
Figure 5. Morphological and nutritional measurement of Crassostrea gigas and C. angulata. (A) Growth trait comparison; from left to right are the shell height, shell length, shell width, and wet weight. (B) Comparison of glycogen and crude fat; left is glycogen, and right is crude fat. (C) Comparison of fatty acid content. Light blue represents C. gigas, and light red represents C. angulata. Significant differences among groups were marked with “*” at P < 0.05, “**” at P < 0.01, “***” at P < 0.001, and “****” at P < 0.0001. “ns” indicates a non-significant difference. The Mann–Whitney U test was used to analyze the differences in the shell height, shell length, and shell width between the two groups, and a t-test was used to analyze the differences in the wet weight, glycogen, crude fat, and fatty acids. C14:1, myristoleic acid; C15:0, pentadecanoic acid; C18:1, oleic acid; C18:2ω6, linoleic acid; C18:3ω3, linolenic acid; C20:1ω9, eicosenoic acid; C20:3ω6, eicosatrienoic acid; C20:2ω3, icosatrienoic acid; C22:0, behenic acid.
The metabolome data showed that malate and succinate, which are intermediate products of the TCA cycle, were significantly lower in C. gigas (Figure 4 and Supplementary Table 6). And there was also a lower content of flavin mononucleotide (FMN) which is an important component of electron transport chain in C. gigas (Supplementary Table 6).
According to the transcriptome and metabolome data, we found that many amino acid metabolic pathways were significantly enriched, including glycine, serine, and threonine metabolism; alanine, aspartate, and glutamate metabolism; tyrosine metabolism; cysteine and methionine metabolism; phenylalanine metabolism; and arginine and proline metabolism (Supplementary Figure 3). In the glycine, serine, and threonine metabolism pathways, we observed higher gene expression of betaine-homocysteine S-methyltransferase (BHMT), sarcosine oxidase (PIPOX), choline dehydrogenase (CHDH), and higher content of phosphatidylserine in C. angulata relative to those in C. gigas (Figure 4 and Supplementary Table 4). Interestingly, the expressions of gamma-glutamyl transpeptidase and aminopeptidase N, which catalyze the conversion of glutathione to glycine, were higher in C. angulata (Figure 4 and Supplementary Table 4). In the alanine, aspartate, and glutamate metabolism pathways, we found that aspartate aminotransferase (ASPB) and d-aspartate oxidase (DDO) which mediate aspartic acid into the TCA cycle to supply ATP were upregulated in C. angulata (Figure 4 and Supplementary Table 4). The same result was also identified for cysteine and methionine metabolism; there was higher expression of ASPB and lower expression of cystathionine gamma-lyase in C. angulata, which also mediates the conversion of cysteine and pyruvate (Figure 4 and Supplementary Table 4).
Moreover, we observed that many antioxidant and resistance-related pathways were significantly enriched. In the glutathione metabolism pathway, C. gigas had a higher content of cysteine, greater expressions of glutathione peroxidase and glutathione S-transferase, and a lower content of oxidized glutathione (Figure 4 and Supplementary Tables 4, 6). In the arginine and proline metabolism pathways, we observed that C. gigas had a higher content of gamma aminobutyric acid (GABA) and a higher level of amidase and spermine oxidase (Figure 4 and Supplementary Tables 4, 6), which catalyze arginine and spermine to GABA (Wang et al., 2003). In the tyrosine metabolism pathway, increased levels of aromatic-L-amino-acid/L-tryptophan decarboxylase, catechol O-methyltransferase, and phenylalanine-4-hydroxylase in C. gigas implied that catecholamines such as dopamine and epinephrine were upregulated (Figure 4 and Supplementary Table 4). Moreover, the integration analysis showed that the linoleic acid metabolism pathways were significantly enriched. In the linoleic acid metabolism pathway, we found that linoleic acid and linolenic acid were higher in C. gigas (Figure 4 and Supplementary Table 6).
Phenotype Measurement
In morphological data, our results showed that the mean shell height, shell width, and wet weight of C. angulata were significantly higher than those of C. gigas (P < 0.01; Figure 5A and Supplementary Table 8).
In nutritional data, C. gigas had higher glycogen and crude fat contents (Figure 5B and Supplementary Table 9). Additionally, we found that C14:1 (tetradecanoic acid), C15:0 (pentadecanoic acid), C18:1 (oleic acid), C18:2ω6 (linoleic acid), C18:3ω3 (linolenic acid), C20:1ω9 (eicosenoic acid), C20:3ω6 (eicosatrienoic acid), and C22:0 (behenic acid) were significantly higher in C. gigas (Figure 5C). And C20:3ω3 (icosatrienoic acid) was found to be higher in C. angulata (Figure 5C).
In physiological data, the normalized oxygen consumption rate in C. angulata under normal conditions was also higher than that in C. gigas (Figure 6A). Additionally, total antioxidant capacity (Figure 6B) and five anti-oxidation genes (glutathione S-transferase, catalase, superoxide dismutase, glutathione, and xanthine dehydrogenase) expression (1.97–11.44 fold change; P < 0.05; Figure 6C) were higher in C. gigas.
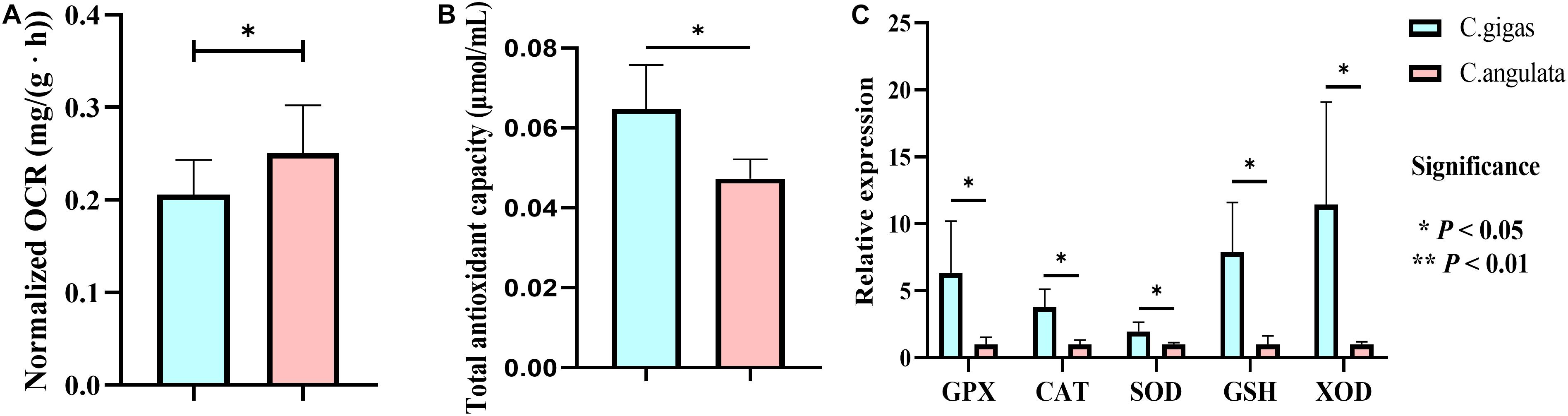
Figure 6. Physiological measurement of Crassostrea gigas and C. angulata. (A) Normalized oxygen consumption rate comparison. (B) Total antioxidant capacity. (C) Relative expression of antioxidant genes. Light blue represents Crassostrea gigas, and light red represents C. angulata. Asterisks indicate significant differences between these two species (t-test). Vertical bars represent standard errors. GPX, glutathione peroxidase; CAT, catalase; GST, glutathione S-transferase; SOD, superoxide dismutase; XDH, xanthine dehydrogenase.
Discussion
Crassostrea gigas Allocates More Energy to Storage
On the basis of the pathway enrichment analysis of DEGs and SDMs, we found that the glycolysis pathway and fatty acids degradation pathway were downregulated in C. gigas. And fatty acids synthesis pathway was upregulated comparatively.
In the glycolysis pathway, HK and GPI were lower in C. gigas. It has been reported that HK expression can be affected by environmental stressors, such as hypoxia (Hall et al., 2009). The glycogen content assays further validated that C. gigas inhibited the glycolysis pathway to increase glycogen content. As for lipids, lower EHHADH expression and butanoyl-CoA content suggested that C. gigas suppressed the fatty acid degradation pathway, which was also confirmed by the crude fat assay. Additionally, higher expression of fatty acid synthase and an increase in the contents of behenic acid and pentadecanoic acid suggested that the fatty acid synthesis pathway was upregulated. Fatty acid synthase is a multifunctional protein, and its main function is to catalyze the synthesis of palmitate from acetyl-CoA and malonyl-CoA into long-chain saturated fatty acids in the presence of NADPH. These results suggest that C. gigas allocates more energy to store glycogen and lipids than does C. angulata.
Interestingly, our data showed that C. gigas had less fatty acid saturation than did C. angulata. It has been widely recognized that membrane lipid unsaturation is closely related to membrane fluidity, which plays an essential role in the cold resistance of organisms (Nishida and Murata, 1996; Guderley, 2004). Higher expression of SCD in C. gigas further illustrated that the unsaturated fatty acid synthesis pathway was upregulated. When on the membrane of the endoplasmic reticulum, SCD is the rate-limiting enzyme that catalyzes the formation of monounsaturated fatty acids from saturated fatty acids. This process is especially important for palmitoyl-CoA (C16:0) and stearoyl-CoA (C18:0), which form palmitoleate (C16:1) and oleic acid (C18:1), respectively, as they are important targets for fatty acid metabolism (Stukey et al., 1990; Wang et al., 2005). Palmitoleate and oleate are the main components of monounsaturated fatty acids in membrane phospholipids, triglycerides, cholesterol esters, and ester waxes, which are closely related to membrane fluidity (Nakamura and Nara, 2004). It has been reported that SCD-1 was significantly induced by low temperature stress in orange-spotted grouper (Epinephelus coioides) (Sun et al., 2019) and large yellow croaker (Larimichthys crocea) (Xu et al., 2015). This is consistent with the relatively low temperatures of the natural habitats in Northern China. Therefore, we speculate that C. gigas upregulated fatty acid unsaturation to maintain membrane fluidity and adapt to the relatively cold environment in Northern China.
Moreover, lower content of malate and succinate reflect that the TCA cycle is downregulated in C. gigas (Figure 6). The TCA cycle is the final oxidative pathway in aerobic metabolism, which links carbohydrates, fats, and amino acids (Akram, 2014). Oxidation of acetyl-CoA by the TCA cycle accounts for 2/3 of total oxygen consumption and ATP production. In addition, low content of flavin mononucleotide (FMN) in C. gigas indicates downregulated oxidative phosphorylation (Supplementary Table 8). FMN is the active form of the flavoprotein prosthetic group in complex I of the mitochondrial electron transport chain and it participates in the electron transfer process during biological oxidation (Liu et al., 2002). The suppression of the TCA cycle and oxidative phosphorylation indicates that C. gigas reduces the ATP supply via aerobic metabolism, which is consistent with previous finding that C. angulata had higher ATP content than C. gigas (Li A. et al., 2021). And the reduction of ATP supply may influence the costs of reproduction, growth, activity, and maintenance (Sokolova et al., 2012).
Crassostrea angulata Allocates More Energy to Growth
There were many significantly enriched amino acid metabolism pathways in the integrated analysis. Glycine is an important structural amino acid in the glycine, serine, and threonine metabolism pathways, and its synthesis pathway was upregulated in C. angulata. It has been reported that glycine plays an important role in the metabolism and nutrition of organisms and 80% of all glycine is used for protein synthesis, which means that it has a crucial function in body growth (Razak et al., 2017). Based on a growth improvement experiment, it has also been reported that the glycine, serine, and threonine metabolism pathways are enriched in Pacific white shrimp (Litopenaeus vannamei) (Yu et al., 2021). In C. angulata, the genes that mediate the alanine, aspartate, and glutamate metabolism pathways and the cysteine and methionine metabolism pathways (such as ASPB and DDO) into the TCA cycle to supply ATP were upregulated. Furthermore, we found that C. angulata had higher contents of arginine and histidine than did C. gigas (Supplementary Table 8). The oxygen consumption rate is a physiological and metabolic indicator frequently used to reflect aerobic respiration and energy metabolism levels (Prins et al., 1997), and as a basic physiological activity of energy metabolism, it indicates the physiological state of organisms and the influence of environmental factors (Lannig et al., 2006; Sokolova et al., 2012; Juárez et al., 2018). Therefore, we suggest that the higher normalized oxygen consumption rate in C. angulata than in C. gigas reflects its stronger aerobic metabolism capacity and higher allocation of energy to growth. We infer that C. angulata upregulates amino acid synthesis (such as glycine) and amino acids oxidation (such as aspartate and cysteine) to supply ATP for other life-history components.
Moreover, we found that 40S ribosomal protein S19 (RPS19) and 60S acidic ribosomal protein P1 gene expression was higher in C. angulata (Supplementary Table 4). These proteins participate in the ribosome pathway. Ribosomes, which are vital organelles in all living organisms, are involved in the translation process. The abundance of ribosomes depends on the level of ribosomal protein-encoding mRNAs (RP-mRNAs) and the subsequent translation process (Jesús et al., 2015; Dermit et al., 2019). Therefore, higher expression of RP-mRNAs suggests that more ribosomes are present and that protein synthesis is upregulated. There is experimental evidence to suggest that chitinase negatively regulates biomineralization (Wang et al., 2017; Zhou et al., 2020). Our data showed a significantly lower level of chitinase (Supplementary Table 4) in C. angulata, indicating an upregulated biomineralization process.
In addition, we found some growth performance-related differential metabolites, including uridine diphosphate-glucose in the pyrimidine metabolism pathway, creatinine in the arginine and proline metabolism pathway, and taurocholate and taurocyamine in the taurine and hypotaurine metabolism pathway (Supplementary Table 8). In a study on the effects of temperature on the growth of European seabass (Dicentrarchus labrax), Zhang Z. et al. (2021) found that uridine diphosphate-glucose and taurocholate are potential markers of growth (Zhang Z. et al., 2021). It has been reported that creatinine levels are positively correlated with muscle quality and bone mineral density (Schiffrin et al., 2007; Yamada et al., 2017).
Our data showed that C. angulata has better growth performance than C. gigas, demonstrated by significantly greater shell height, shell weight, and wet weight. Based on our results and those of previous studies, we propose that C. angulata upregulates the amount of available energy by increasing the aerobic metabolism of carbohydrates and lipids and then distributing more energy to synthesize amino acids and proteins.
Crassostrea gigas Allocates More Energy to Defense
Glutathione is essential for antioxidant defense, energy metabolism, and regulation of cellular events (Wu et al., 2004; Jones, 2008; Forman et al., 2009). It has been reported that cysteine is the amino acid that is limiting for glutathione synthesis (Lyons et al., 2000; Lu, 2009), and its antioxidant function is related to its sulfhydryl group (Valko et al., 2016). Therefore, a higher cysteine content and higher expression of glutathione peroxidase and glutathione-S-transferase in C. gigas indicated a stronger antioxidant ability. Furthermore, the lower oxidized glutathione content further validated our hypothesis. It has been reported that the glutathione oxidation-reduction product is a signal molecule that participates in the defense responses of organisms (Jones, 2006; Locato et al., 2017). Importantly, antioxidant gene expression and the total antioxidant capacity data were consistent with our results.
Gamma aminobutyric acid is a four-carbon amino acid that is an important inhibitory neurotransmitter in animals (Kinnersley and Turano, 2000; Bouche et al., 2003). Previous studies have mostly focused on the functions of GABA in the growth, development, and resistance of plants (Guo et al., 2021; Wang P. K. et al., 2021). However, it has also been reported that GABA content is higher in more hypoxia-tolerant species such as C. gigas (Haider et al., 2020) and it is helpful for mitigating heat stress in the green-lipped mussel Perna canaliculus Gmelin (Dunphy et al., 2015) and soft corals (Farag et al., 2018). In the tyrosine metabolism pathway of C. gigas, the catecholamine synthesis pathway is upregulated. Under environmental stress, catecholamines and adrenaline can be induced in mammals (Gatzke-Kopp, 2011; Vindas et al., 2017) and Drosophila melanogaster (Adamo, 2017). Linolenic acid (C18:3n-3) is an eighteen-carbon polyunsaturated fatty acid that is a precursor of synthetic eicosanoids, such as prostaglandins and leukotrienes, and it can activate immune function and improve the ability of antioxidants (Geay et al., 2015; Chen et al., 2016).
Previous research has found that C. gigas has more glycogen and lipids than C. angulata. Under environmental stress, glycogen and lipids can be used to quickly supply energy to maintain the basic functions of organisms (Rajamanickam and Muthuswamy, 2008; Ferreira et al., 2011; Wu et al., 2013). Therefore, based on the increased levels of antioxidant-related genes and metabolites, as well as the content of resistant metabolites, we speculate that C. gigas allocates more energy to basal maintenance of some cellular processes related to defense than does C. angulata. Considering the common garden experiment was conducted in the region of the native inhabit of C. gigas (Northern of China), our result was consistent with previous study that C. gigas has higher adaptive capacity than C. angulata in its native inhabit (Li A. et al., 2021).
Conclusion
In this study, untargeted metabolomic and transcriptomic approaches applied together with phenotypic parameters were used to assess the metabolite and transcript divergences between C. gigas and C. angulata oysters. The integrated analysis showed that C. gigas allocated more energy to storage and defense by suppressing glycolysis and the fatty acid oxidation pathway, as well as by upregulating fatty acid synthesis, antioxidant genes, and related metabolites. In addition, we observed that C. gigas has higher glycogen and crude fat content and fatty acid unsaturation and stronger antioxidant capacity. However, C. angulata exhibited better growth performance and a higher oxygen consumption rate. These findings suggest that C. angulata allocates more energy to growth, which is embodied in its stronger aerobic capacity and higher levels of protein synthesis genes, metabolites, and growth-related biomarkers. This study revealed the energy allocation-mediated mechanisms of growth-defense trade-offs between the two relative oyster species, which provides new insight into how the adaptive traits coordinate and the mechanisms underlying biochemical and molecular mechanism. Additionally, energetic metabolism-related genes identified in this study will serve as target for the meat quality improvement in the oyster industry.
Data Availability Statement
The datasets presented in this study can be found in online repositories. The names of the repository/repositories and accession number(s) can be found below: https://www.ncbi.nlm.nih.gov/, SRP326737.
Author Contributions
LL and GZ conceived the study. CW carried out the field and laboratory work, participated in the data analysis, and drafted the manuscript. CW and AL collected the oyster samples. AL produced the F1 progeny. AL and WW contributed to cultural management. CW, LL, and GZ revised the manuscript. All authors approved the manuscript for publication.
Funding
This research was funded by the National Key R&D Program of China (No. 2018YFD0900304), National Natural Science Foundation of China (32101353), Strategic Priority Research Program of the Chinese Academy of Sciences (Grant No. XDA23050402), Key Deployment Project of the Centre for Ocean Mega-Research of Science, Chinese Academy of Sciences (COMS2019Q06), Distinguished Young Scientists Research Fund of the Key Laboratory of Experimental Marine Biology, Chinese Academy of Sciences (No. KLEMB-DYS04), Blue Life Breakthrough Program of LMBB of Qingdao National Laboratory for Marine Science and Technology (MS2018NO02), and Modern Agroindustry Technology Research System (No. CARS-49).
Conflict of Interest
The authors declare that the research was conducted in the absence of any commercial or financial relationships that could be construed as a potential conflict of interest.
Publisher’s Note
All claims expressed in this article are solely those of the authors and do not necessarily represent those of their affiliated organizations, or those of the publisher, the editors and the reviewers. Any product that may be evaluated in this article, or claim that may be made by its manufacturer, is not guaranteed or endorsed by the publisher.
Acknowledgments
The authors would like to thank Xuegang Wang, Kexin Zhang, and Ziyan Zhang for their support and assistance in oyster breeding and sample collection.
Supplementary Material
The Supplementary Material for this article can be found online at: https://www.frontiersin.org/articles/10.3389/fmars.2021.744626/full#supplementary-material
Footnotes
References
Adamo, S. A. (2017). Stress responses sculpt the insect immune system, optimizing defense in an ever-changing world. Dev. Comput. Immunol. 66, 24–32. doi: 10.1016/j.dci.2016.06.005
Akram, M. (2014). Citric acid cycle and role of its intermediates in metabolism. Cell. Biochem. Biophys. 68, 475–478.
Albrecht, T., and Argueso, C. T. (2017). Should I fight or should I grow now? The role of cytokinins in plant growth and immunity and in the growth-defence trade-off. Ann. Bot. 119, 725–735.
Bouche, N., Lacombe, B., and Fromm, H. (2003). GABA signaling: a conserved and ubiquitous mechanism. Trends Cell Biol. 13, 607–610.
Caignard, T., Kremer, A., Bouteiller, X. P., Parmentier, J., Louvet, J.-M., Venner, S., et al. (2021). Counter-gradient variation of reproductive effort in a widely distributed temperate oak. Funct. Ecol. 35, 1745–1755. doi: 10.1111/1365-2435.13830
Chauvin, K. M., Asner, G. P., Martin, R. E., Kress, W. J., Wright, S. J., and Field, C. B. (2018). Decoupled dimensions of leaf economic and anti-herbivore defense strategies in a tropical canopy tree community. Oecologia 186, 765–782. doi: 10.1007/s00442-017-4043-9
Chen, C., Sun, B., Guan, W., Bi, Y., Li, P., Ma, J., et al. (2016). N-3 essential fatty acids in Nile tilapia, Oreochromis niloticus: effects of linolenic acid on non-specific immunity and anti-inflammatory responses in juvenile fish. Aquaculture 450, 250–257. doi: 10.1016/j.aquaculture.2015.08.005
Cohen, A. A., Coste, C. F. D., Li, X. Y., Bourg, S., and Pavard, S. (2020). Are trade-offs really the key drivers of ageing and life span? Functional. Ecol. 34, 153–166.
Coll, Y., Peralta, K. D., Olea, A. F., and Espinoza, L. (2021). Plant growth-defense trade-offs: molecular processes leading to physiological changes. Int. J. Mol. Sci. 22:693. doi: 10.3390/ijms22020693
Cortleven, A., Leuendorf, J. E., Frank, M., Pezzetta, D., Bolt, S., and Schmulling, T. (2019). Cytokinin action in response to abiotic and biotic stresses in plants. Plant Cell Environ. 42, 998–1018.
Culina, A., Linton, D. M., Pradel, R., Bouwhuis, S., and Macdonald, D. W. (2019). Live fast, don’t die young: survival-reproduction trade-offs in long-lived income breeders. J. Animal Ecol. 88, 746–756. doi: 10.1111/1365-2656.12957
Darychuk, N., Hawkins, B. J., and Stoehr, M. (2012). Trade-offs between growth and cold and drought hardiness in submaritime Douglas-fir.Can. J. For. Res. 42, 1530–1541. doi: 10.1002/ece3.3796
Dermit, M., Dodel, M., Lee, F., Azman, M. S., and Mardakheh, F. K. (2019). Subcellular mRNA localization regulates ribosome biogenesis in migrating cells. Dev. Cell. 55, 298–313.e10. doi: 10.1016/j.devcel.2020.10.006
Dunphy, B. J., Watts, E., and Ragg, N. L. C. (2015). Identifying thermally-stressed adult green-lipped mussels (Perna canaliculus Gmelin, 1791) via metabolomic profiling. Am. Malacol. Bull. 33, 127–135.
Ernande, B., Boudry, P., Clobert, J., and Haure, J. (2004). Plasticity in resource allocation based life history traits in the Pacific oyster, Crassostrea gigas. I. spatial variation in food abundance. J. Evolu. Biol. 17, 342–356. doi: 10.1046/j.1420-9101.2003.00674.x
Ernande, B., Clobert, J., Mccombie, H., and Boudry, P. (2003). Genetic polymorphism and trade-offs in the early life-history strategy of the Pacific oyster, Crassostrea gigas (Thunberg, 1795): a quantitative genetic study. J. Evolu. Biol. 16, 399–414. doi: 10.1046/j.1420-9101.2003.00543.x
Farag, M. A., Meyer, A., Ali, S. E., Salem, M. A., Giavalisco, P., Westphal, H., et al. (2018). Comparative metabolomics approach detects stress-specific responses during coral bleaching in soft corals. J. Proteome Res. 17, 2060–2071. doi: 10.1021/acs.jproteome.7b00929
Feidantsis, K., Michaelidis, B., Raitsos, D. E., and Vafidis, D. (2021). Seasonal metabolic and oxidative stress responses of commercially important invertebrate species-correlation with their habitat. Mari. Ecol. Prog. Ser. 658, 27–46.
Ferreira, M., Valenti, V. E., Cisternas, J. R., Ferreira, C., Meneghini, A., Filho, C. F., et al. (2011). Memantine effects on liver and adrenal gland of rats exposed to cold stress. Int. Arch. Med. 4:5.
Florea, L., Song, L., and Salzberg, S. L. (2013). Thousands of exon skipping events differentiate among splicing patterns in sixteen. Hum. Tissues 2:188. doi: 10.12688/f1000research.2-188.v2
Folkvord, A., Jorgensen, C., Korsbrekke, K., Nash, R. D. M., Nilsen, T., and Skjaeraasen, J. E. (2014). Trade-offs between growth and reproduction in wild Atlantic cod. Can. J. Fish. Aquatic Sci. 71, 1106–1112. doi: 10.1890/08-1404.1
Forman, H. J., Zhang, H. Q., and Rinna, A. (2009). Glutathione: overview of its protective roles, measurement, and biosynthesis. Mol. Aspects Med. 30, 1–12.
Galen, C. (2000). High and dry: drought stress, sex-allocation trade-offs, and selection on flower size in the alpine wildflower. Polemonium Viscosum (Polemoniaceae) 156, 72–83. doi: 10.1086/303373
Gallardo-Hidalgo, J., Barria, A., Yoshida, G. M., and Yanez, J. M. (2021). Genetics of growth and survival under chronic heat stress and trade-offs with growth- and robustness-related traits in rainbow trout. Aquaculture 531:9.
Gatzke-Kopp, L. M. (2011). The canary in the coalmine: the sensitivity of mesolimbic dopamine to environmental adversity during development. Neurosci. Biobehav. Rev. 35, 794–803. doi: 10.1016/j.neubiorev.2010.09.013
Geay, F., Mellery, J., Tinti, E., Douxfils, J., Larondelle, Y., Mandiki, S. N. M., et al. (2015). Effects of dietary linseed oil on innate immune system of Eurasian perch and disease resistance after exposure to Aeromonas salmonicida achromogen. Fish Shellfish Immunol. 47, 782–796. doi: 10.1016/j.fsi.2015.10.021
Ghaffari, H., Wang, W., Li, A., Zhang, G., and Li, L. (2019). Thermotolerance divergence revealed by the physiological and molecular responses in two oyster subspecies of Crassostrea gigas in China. Front. Physiol. 10:1137. doi: 10.3389/fphys.2019.01137
Guderley, H. (2004). Metabolic responses to low temperature in fish muscle. Biol. Rev. 79, 409–427. doi: 10.1017/s1464793103006328
Guo, X. (2009). Use and exchange of genetic resources in molluscan aquaculture. Rev. Aquac. 1, 251–259. doi: 10.1111/j.1753-5131.2009.01014.x
Guo, X., Ford, S., Zhang, F., and Library, W. M. J. (1999). Molluscan aquaculture in China. J. Shellfish Res. 18, 19–31.
Guo, X., Li, C., Wang, H., and Xu, Z. (2018). Diversity and evolution of living oysters. J. Shellfish Res. 37, 755–771. doi: 10.2983/035.037.0407
Guo, Z., Lv, J., Dong, X., Du, N., and Piao, F. (2021). Gamma-aminobutyric acid improves phenanthrene phytotoxicity tolerance in cucumber through the glutathione-dependent system of antioxidant defense. Ecotoxicol. Environ. Safety 217, 112254–112254. doi: 10.1016/j.ecoenv.2021.112254
Guofan, Z., Xiaodong, F., Ximing, G., Li, L., Ruibang, L., Fei, X., et al. (2012). The oyster genome reveals stress adaptation and complexity of shell formation. Nature 490, 49–54. doi: 10.1038/nature11413
Haider, F., Falfushynska, H. I., Timm, S., and Sokolova, I. M. (2020). Effects of hypoxia and reoxygenation on intermediary metabolite homeostasis of marine bivalves Mytilus edulis and Crassostrea gigas. Comparat. Biochem. Physiol. A Mol. Integrat. Physiol. 242:16. doi: 10.1016/j.cbpa.2020.110657
Hall, J. R., Short, C. E., Petersen, L. H., Stacey, J., Gamperl, A. K., and Driedzic, W. R. (2009). Expression levels of genes associated with oxygen utilization, glucose transport and glucose phosphorylation in hypoxia exposed Atlantic cod (Gadus morhua). Comparat. Biochem. Physiol. D Genom. Proteomics 4, 128–138. doi: 10.1016/j.cbd.2008.12.007
Hao, R., Du, X., Yang, C., Deng, Y., Zheng, Z., and Wang, Q. (2019). Integrated application of transcriptomics and metabolomics provides insights into unsynchronized growth in pearl oyster Pinctada fucata martensii. Sci. Total Environ. 666, 46–56. doi: 10.1016/j.scitotenv.2019.02.221
Huo, D., Sun, L. N., Zhang, L. B., Ru, X. S., Liu, S. L., and Yang, H. S. (2019). Metabolome responses of the sea cucumber Apostichopus japonicus to multiple environmental stresses: heat and hypoxia. Mari. Pollut. Bull. 138, 407–420. doi: 10.1016/j.marpolbul.2018.11.063
Jesús, D., Karbstein, K., and Woolford, J. L. J. (2015). Functions of ribosomal proteins in assembly of eukaryotic ribosomes in vivo. Annu. Rev. Biochem. 84:93–129.
Jiang, Y. Z., Jiao, H. F., Sun, P., Yin, F., and Tang, B. J. (2020). Metabolic response of Scapharca subcrenata to heat stress using GC/MS-based metabolomics. PeerJ 8:20. doi: 10.7717/peerj.8445
Jones, D. P. (2008). Radical-free biology of oxidative stress. Am. J. Physiol. Cell Physiol. 295, C849–C868.
Juárez, O. E., Lafarga-De La Cruz, F., Leyva-Valencia, I., López-Landavery, E., García-Esquivel, Z., Díaz, F., et al. (2018). Transcriptomic and metabolic response to chronic and acute thermal exposure of juvenile geoduck clams Panopea globosa. Mar. Genomics 42, 1–13. doi: 10.1016/j.margen.2018.09.003
Judge, R., Choi, F., and Helmuth, B. (2018). Recent advances in data logging for intertidal ecology. Front. Ecol. Evolu. 6:18.
Kanehisa, M., Araki, M., Goto, S., Hattori, M., Hirakawa, M., Itoh, M., et al. (2008). KEGG for linking genomes to life and the environment. Nucleic Acids Res. 36, D480–D484.
Karasov, T. L., Chae, E., Herman, J. J., and Bergelson, J. (2017). Mechanisms to mitigate the trade-off between growth and defense. Plant Cell 29, 666–680.
Kelly, M. (2019). Adaptation to climate change through genetic accommodation and assimilation of plastic phenotypes. Philos Trans R Soc. Lond B Biol. Sci. 374:20180176. doi: 10.1098/rstb.2018.0176
Kim, D., Landmead, B., and Salzberg, S. L. (2015). HISAT: a fast spliced aligner with low memory requirements. Nat. Methods 12:357. doi: 10.1038/nmeth.3317
Kinnersley, A. M., and Turano, F. J. (2000). Gamma aminobutyric acid (GABA) and plant responses to stress. Crit. Rev. Plant Sci. 19, 479–509.
Kong, T. T., Lin, S. M., Ren, X., Li, S. K., and Gong, Y. (2020). Transcriptome and metabolome integration analysis of mud crab Scylla paramamosain challenged to Vibrio parahaemolyticus infection. Fish Shellfish Immunol. 103, 430–437. doi: 10.1016/j.fsi.2020.05.069
Lannig, G., Flores, J. F., and Sokolova, I. M. J. (2006). Temperature-dependent stress response in oysters, Crassostrea virginica: pollution reduces temperature tolerance in oysters. Aquat. Toxicol. 79, 278–287. doi: 10.1016/j.aquatox.2006.06.017
Lee, W.-S., Monaghan, P., and Metcalfe, N. B. (2013). Experimental demonstration of the growth rate–lifespan trade-off. Proc. Biol. Sci. 280:20122370.
Li, A., Li, L., Song, K., Wang, W., and Zhang, G. (2017). Temperature, energy metabolism, and adaptive divergence in two oyster subspecies. Ecol. Evol. 7, 6151–6162. doi: 10.1002/ece3.3085
Li, A., Li, L., Wang, W., and Zhang, G. (2019). Evolutionary trade-offs between baseline and plastic gene expression in two congeneric oyster species. Biol. Lett. 15:20190202. doi: 10.1098/rsbl.2019.0202
Li, A., Li, L., Zhang, Z., Li, S., Wang, W., Guo, X., et al. (2021). Noncoding variation and transcriptional plasticity promote thermal adaptation in oysters by altering energy metabolism. Mol. Biol. Evol. doi: 10.1093/molbev/msab241
Li, B., Song, K., Meng, J., Li, L., and Zhang, G. (2017). Integrated application of transcriptomics and metabolomics provides insights into glycogen content regulation in the Pacific oyster Crassostrea gigas. BMC Genom. 18:713. doi: 10.1186/s12864-017-4069-8
Li, H., Handsaker, B., Wysoker, A., Fennell, T., Ruan, J., Homer, N., et al. (2009). The sequence alignment/map format and SAMtools. Bioinformatics 25, 2078–2079.
Li, L., Ao, L., Kai, S., Jie, M., Ximing, G., Shiming, L., et al. (2018). Divergence and plasticity shape adaptive potential of the Pacific oyster. Nat. Ecol. Evolu. 2, 1751–1760.
Li, Z., Li, Q., Liu, S., Han, Z., Kong, L., and Yu, H. (2021). Integrated analysis of coding genes and non-coding RNAs associated with shell color in the Pacific oyster (Crassostrea gigas). Mar. Biotechnol. 23, 417–429. doi: 10.1007/s10126-021-10034-7
Linares, C., Doak, D. F., Coma, R., Díaz, D., and Zabala, M. (2007). Life history and viability of a long-lived marine invertebrate: the octocoral Paramuricea clavata. Ecology 88, 918–928. doi: 10.1890/05-1931
Lind, E. M., Borer, E., Seabloom, E., Adler, P., Bakker, J. D., Blumenthal, D. M., et al. (2013). Life-history constraints in grassland plant species: a growth-defence trade-off is the norm. Ecol. Lett. 16, 513–521. doi: 10.1111/ele.12078
Liu, Y. B., Fiskum, G., and Schubert, D. (2002). Generation of reactive oxygen species by the mitochondrial electron transport chain. J. Neurochem. 80, 780–787.
Livak, K. J., and Schmittgen, T. D. (2001). Analysis of relative gene expression data using real-time quantitative PCR and the 2(-Delta Delta C(T)) Method. Methods 25, 402–408. doi: 10.1006/meth.2001.1262
Locato, V., Cimini, S., and De Gara, L. (2017). “Glutathione as a key player in plant abiotic stress responses and tolerance,” in Glutathione in Plant Growth, Development, and Stress Tolerance, eds M. A. Hossain, M. G. Mostofa, P. Diaz-Vivancos, D. J. Burritt, M. Fujita, and L.-S. P. Tran (Cham: Springer International Publishing), 127–145. doi: 10.1007/978-3-319-66682-2_6
Love, M. I., Huber, W., and Anders, S. (2014). Moderated estimation of fold change and dispersion for RNA-seq data with DESeq2. Genome Biol. 15:550. doi: 10.1186/s13059-014-0550-8
Lyons, J., Rauh-Pfeiffer, A., Yu, Y. M., Lu, X. M., Zurakowski, D., Tompkins, R. G., et al. (2000). Blood glutathione synthesis rates in healthy adults receiving a sulfur amino acid-free diet. Proc. Natl. Acad. Sci. U.S.A. 97, 5071–5076. doi: 10.1073/pnas.090083297
Ma, L., Liu, P., Su, S., Luo, L. G., Zhao, W. G., and Ji, X. (2019). Life-history consequences of local adaptation in lizards: Takydromus wolteri (Lacertidae) as a model organism. Biol. J. Linnean Soc. 127, 88–99.
Margalha, L., Confraria, A., and Baena-Gonzalez, E. (2019). SnRK1 and TOR: modulating growth-defense trade-offs in plant stress responses. J. Exp. Bot. 70, 2261–2274. doi: 10.1093/jxb/erz066
Martone, R. G., and Micheli, F. (2012). Geographic variation in demography of a temperate reef snail: importance of multiple life-history traits. Mari. Ecol.Prog. Ser. 457, 85–99.
Matos, A. S., Matthews-Cascon, H., and Chaparro, O. R. (2020). Morphometric analysis of the shell of the intertidal gastropodEchinolittorina lineolata(d’Orbigny, 1840) at different latitudes along the Brazilian coast. J. Mari. Biol. Assoc. U.K. 100, 725–731.
Mayrose, M., Kane, N. C., Mayrose, I., Dlugosch, K. M., and Rieseberg, L. H. (2011). Increased growth in sunflower correlates with reduced defences and altered gene expression in response to biotic and abiotic stress. Mol. Ecol. 20, 4683–4694. doi: 10.1111/j.1365-294X.2011.05301.x
Metcalfe, N. B., and Monaghan, P. (2001). Compensation for a bad start: grow now, pay later? Trends Ecol. Evol. 16, 254–260. doi: 10.1016/s0169-5347(01)02124-3
Min, L., Jirui, G., and Ying, X. (2018). Growth-defense trade-off regulated by hormones in grass plants growing under different grazing intensities. Physiol Plant. 166, 553–569 doi: 10.1111/ppl.12802
Nakamura, M. T., and Nara, T. Y. (2004). Structure, function, and dietary regulation of delta 6, delta 5, and delta 9 desaturases. Ann. Rev. Nut. 24, 345–376.
Nishida, I., and Murata, N. (1996). Chilling sensitivity in plants and cyanobacteria: the crucial contribution of membrane lipids. Annu. Rev. Plant Physiol. Plant Mol. Biol. 47, 541–568. doi: 10.1146/annurev.arplant.47.1.541
Pertea, M., Kim, D., Pertea, G. M., Leek, J. T., and Salzberg, S. L. (2016). Transcript-level expression analysis of RNA-seq experiments with HISAT. StringTie Ballgown 11, 1650–1667. doi: 10.1038/nprot.2016.095
Pertea, M., Pertea, G. M., Antonescu, C. M., Chang, T. C., Mendell, J. T., and Salzberg, S. L. (2015). StringTie enables improved reconstruction of a transcriptome from RNA-seq reads. Nat. Biotechnol. 33, 290–295. doi: 10.1038/nbt.3122
Petes, L. E., Menge, B. A., and Harris, A. L. (2008). Intertidal mussels exhibit energetic trade-offs between reproduction and stress resistance. Ecol. Monogr. 78, 387–402.
Pigot, A. L., Sheard, C., Miller, E. T., Bregman, T. P., Freeman, B. G., Roll, U., et al. (2020). Macroevolutionary convergence connects morphological form to ecological function in birds. Nat. Ecol. Evolu. 4, 230–239. doi: 10.1038/s41559-019-1070-4
Prins, T. C., Smaal, A. C., and Dame, R. F. J. (1997). A review of the feedbacks between bivalve grazing and ecosystem processes. Aquat. Ecol. 31, 349–359.
Prunier, R., Holsinger, K. E., and Carlson, J. E. (2012). The effect of historical legacy on adaptation: do closely related species respond to the environment in the same way? J. Evolu. Biol. 25, 1636–1649. doi: 10.1111/j.1420-9101.2012.02548.x
Quarles, B. M., and Roach, D. A. (2019). Ageing in an herbaceous plant: Increases in mortality and decreases in physiology and seed mass. J. Ecol. 107, 1409–1418.
Rajamanickam, V., and Muthuswamy, N. (2008). Effect of heavy metals on the level of vitamin E, total lipid and glycogen reserves in the liver of common carp (Cyprinus carpio L.). Maejo Int. J. Sci. Technol. 2, 391–399.
Razak, M. A., Begum, P. S., Viswanath, B., and Rajagopal, S. (2017). Multifarious beneficial effect of nonessential amino acid, glycine: a review. Oxidative Med. Cell. Long. 2017:8. doi: 10.1155/2017/1716701
Ren, J., Xiao, L., Feng, J., Guo, X., and Liu, B. (2010). Unusual conservation of mitochondrial gene order in Crassostrea oysters: evidence for recent speciation in Asia. BMC Evol. Biol. 10:394. doi: 10.1186/1471-2148-10-394
Ren, X. Y., Yu, Z. X., Xu, Y., Zhang, Y. B., Mu, C. M., Liu, P., et al. (2020). Integrated transcriptomic and metabolomic responses in the hepatopancreas of kuruma shrimp (Marsupenaeus japonicus) under cold stress. Ecotoxicol. Environ. Safety 206:9. doi: 10.1016/j.ecoenv.2020.111360
Roach, D. A., and Smith, E. F. (2020). Life-history trade-offs and senescence in plants. Functional Ecol. 34, 17–25.
Sanford, E., and Kelly, M. W. J. (2011). Local adaptation in marine invertebrates. Ann. Rev. Mar. Sci. 3, 509–535.
Schiffrin, E. L., Lipman, M. L., and Mann, J. F. E. (2007). Chronic kidney diseaseeffects on the cardiovascular system. Circulation 116, 85–97.
Sebens, K. P. (2002). Energetic constraints, size gradients, and size limits in benthic marine invertebrates. Int. Comparat. Biol. 42, 853–861. doi: 10.1093/icb/42.4.853
Sherker, Z. T., Ellrich, J. A., and Scrosati, R. A. (2017). Predator-induced shell plasticity in mussels hinders predation by drilling snails. Mar. Ecol. Prog. Ser. 573, 167–175.
Sletvold, N., and Agren, J. (2015). Climate-dependent costs of reproduction: survival and fecundity costs decline with length of the growing season and summer temperature. Ecol. Lett. 18, 357–364. doi: 10.1111/ele.12417
Sokolova, I. M., Frederich, M., Bagwe, R., Lannig, G., and Sukhotin, A. A. (2012). Energy homeostasis as an integrative tool for assessing limits of environmental stress tolerance in aquatic invertebrates. Mar. Environ. Res. 79, 1–15. doi: 10.1016/j.marenvres.2012.04.003
Stier, A., Delestrade, A., Zahn, S., Arrivé, M., Criscuolo, F., and Massemin-Challet, S. J. O. (2014). Elevation impacts the balance between growth and oxidative stress in coal tits. Oecologia 175, 791–800. doi: 10.1007/s00442-014-2946-2
Stukey, J. E., Mcdonough, V. M., and Martin, C. E. (1990). The OLE1 gene of Saccharomyces cerevisiae encodes the delta 9 fatty acid desaturase and can be functionally replaced by the rat stearoyl-CoA desaturase gene. J. Biol. Chem.. 265, 20144–20149.
Sun, Z. Z., Tan, X. H., Liu, Q. Y., Ye, H. Q., Zou, C. Y., Xu, M. L., et al. (2019). Physiological, immune responses and liver lipid metabolism of orange-spotted grouper (Epinephelus coioides) under cold stress. Aquaculture 498, 545–555.
Trussell, G. C. (2000). Phenotypic clines, plasticity, and morphological trade-offs in an intertidal snail. Evolution 54, 151–166. doi: 10.1111/j.0014-3820.2000.tb00016.x
Valko, M., Jomova, K., Rhodes, C. J., Kuca, K., and Musilek, K. (2016). Redox- and non-redox-metal-induced formation of free radicals and their role in human disease. Arch. Toxicol. 90, 1–37. doi: 10.1007/s00204-015-1579-5
Vilela, A., Cariaga, R., Gonzalez-Paleo, L., and Ravetta, D. (2008). Trade-offs between reproductive allocation and storage in species of Oenothera L. (Onagraceae) native to Argentina. Acta Oecol. Int. J. Ecol. 33, 85–92.
Vindas, M. A., Gorissen, M., Hoglund, E., Flik, G., Tronci, V., Damsgard, B., et al. (2017). How do individuals cope with stress? Behavioural, physiological and neuronal differences between proactive and reactive coping styles in fish. J. Exp. Biol. 220, 1524–1532. doi: 10.1242/jeb.153213
Wang, H., Qian, L., Liu, X., Zhang, G., and Guo, X. J. (2010). Classification of a common cupped oyster from southern China. J. Shellfish Res. 29, 857–866.
Wang, J., Lan, Y., Schmidt, R. E., Su, C., Huang, X., Gould, K., et al. (2005). Characterization of HSCD5, a novel human stearoyl-CoA desaturase unique to primates. Biochem. Biophys. Res. Commun. 332, 735–742. doi: 10.1016/j.bbrc.2005.05.013
Wang, J., Long, X. Y., Chern, M., and Chen, X. W. (2021). Understanding the molecular mechanisms of trade-offs between plant growth and immunity. Sci. China Life Sci. 64, 234–241.
Wang, P. K., Dong, Y. N., Zhu, L. M., Hao, Z. D., Hu, L. F., Hu, X. Y., et al. (2021). The role of gamma-aminobutyric acid in aluminum stress tolerance in a woody plant, Liriodendron chinense x tulipifera. Horticu. Res. 8:15. doi: 10.1038/s41438-021-00517-y
Wang, X., Wang, W. J., Li, Z., Sun, G. H., Xu, X. H., Feng, Y. W., et al. (2021). Comprehensive analysis of differentially expressed ncRNA, mRNA, and their ceRNA networks in the regulation of glycogen content in the Pacific oyster, Crassostrea gigas. Aquaculture 531:20.
Wang, X. F., Liu, Z. M., and Wu, W. J. (2017). Transcriptome analysis of the freshwater pearl mussel (Cristaria plicata) mantle unravels genes involved in the formation of shell and pearl. Mol. Genet. Genom. 292, 343–352. doi: 10.1007/s00438-016-1278-9
Wang, Y., Murray-Stewart, T., Devereux, W., Hacker, A., Frydman, B., Woster, P. M., et al. (2003). Properties of purified recombinant human polyamine oxidase, PAOh1/SMO. Biochem. Biophys. Res. Commun. 304, 605–611. doi: 10.1016/s0006-291x(03)00636-3
Wingfield, J. C., and Sapolsky, R. M. (2010). Reproduction and resistance to stress: when and how. J. Neuroendocrinol. 15, 711–724.
Wu, G. Y., Fang, Y. Z., Yang, S., Lupton, J. R., and Turner, N. D. (2004). Glutathione metabolism and its implications for health. J. Nutr. 134, 489–492.
Wu, H., Zhang, X., Wang, Q., Li, L., Ji, C., Liu, X., et al. (2013). A metabolomic investigation on arsenic-induced toxicological effects in the clam Ruditapes philippinarum under different salinities. Ecotoxicol. Environ. Saf. 90, 1–6. doi: 10.1016/j.ecoenv.2012.02.022
Xu, H., Zhang, D. L., Yu, D. H., Lv, C. H., Luo, H. Y., and Wang, Z. Y. (2015). Molecular cloning and expression analysis of scd1 gene from large yellow croaker Larimichthys crocea under cold stress. Gene 568, 100–108. doi: 10.1016/j.gene.2015.05.027
Yamada, S., Taniguchi, M., Tokumoto, M., Yoshitomi, R., Yoshida, H., Tatsumoto, N., et al. (2017). Modified creatinine index and the risk of bone fracture in patients undergoing hemodialysis: the Q-cohort study. Am. J. Kidney Dis. 70, 270–280. doi: 10.1053/j.ajkd.2017.01.052
Yu, G., Wang, L. G., Han, Y., and He, Q. Y. (2012). clusterProfiler: an R package for comparing biological themes among gene. Clusters 16, 284–287. doi: 10.1089/omi.2011.0118
Yu, Q., Fu, Z., Huang, M., Xu, C., and Li, E. (2021). Growth, physiological, biochemical, and molecular responses of pacific white shrimp litopenaeus vannamei fed different levels of dietary selenium. Aquaculture 535:736393
Yuan, C. M., Wu, T., Geng, Y. F., Chai, Y., and Hao, J. B. (2016). Phenotypic plasticity of lianas in response to altered light environment. Ecol. Res. 31, 375–384.
Zhang, J., Xiong, X., Deng, Y., Zheng, Z., Yang, C., and Du, X. (2021). Integrated application of transcriptomics and metabolomics provides insights into the larval metamorphosis of pearl oyster (Pinctada fucata martensii). Aquaculture 532:736067.
Zhang, Z., Zhou, C., Fan, K., Zhang, L., and Liu, P. (2021). Metabolomics analysis of the effects of temperature on the growth and development of juvenile. Eur. Seabass (Dicentrarchus labrax) 769:145155. doi: 10.1016/j.scitotenv.2021.145155
Zhao, Z., Cong, R., Zhang, K., Wang, W., Zhang, G., Pan, Y., et al. (2021). Illumination can change the periodic variation of the oxygen consumption rate of Crassostrea gigas. J. Mol. Stud. 63, 1–8.
Zhou, Y. P., Yan, Y., Yang, D., Zheng, G. L., Xie, L. P., and Zhang, R. Q. (2020). Cloning, characterization, and functional analysis of chitinase-like protein 1 in the shell of Pinctada fucata. Acta Bio. Et Biophys. Sin. 52, 954–966. doi: 10.1093/abbs/gmaa076
Zuniga-Vega, J. J., Fuentes, J. A., Zamora-Abrego, J. G., Garcia-Vazquez, U. O., De Oca, A. N. M., and Martins, E. P. (2017). Evolutionary patterns in life-history traits of lizards of the genus Xenosaurus. Herpetol. J. 27, 346–360.
Keywords: growth-defense trade-offs, energy allocation, transcriptome, metabolome, Crassostrea gigas, Crassostrea angulata, phenotypic variation
Citation: Wang C, Li A, Wang W, Cong R, Wang L, Zhang G and Li L (2021) Integrated Application of Transcriptomics and Metabolomics Reveals the Energy Allocation-Mediated Mechanisms of Growth-Defense Trade-Offs in Crassostrea gigas and Crassostrea angulata. Front. Mar. Sci. 8:744626. doi: 10.3389/fmars.2021.744626
Received: 20 July 2021; Accepted: 09 September 2021;
Published: 07 October 2021.
Edited by:
Daniel Rittschof, Duke University Marine Laboratory, Nicholas School of the Environment, United StatesReviewed by:
Danielle Ferraz Mello, Duke University, United StatesChuangye Yang, Guangdong Ocean University, China
Copyright © 2021 Wang, Li, Wang, Cong, Wang, Zhang and Li. This is an open-access article distributed under the terms of the Creative Commons Attribution License (CC BY). The use, distribution or reproduction in other forums is permitted, provided the original author(s) and the copyright owner(s) are credited and that the original publication in this journal is cited, in accordance with accepted academic practice. No use, distribution or reproduction is permitted which does not comply with these terms.
*Correspondence: Li Li, lili@qdio.ac.cn