- 1Ethology Unit, Department of Biology, University of Pisa, Pisa, Italy
- 2UMR CNRS 6249 Chrono-Environnement, Université Bourgogne Franche-Comté, Besançon, France
- 3School of Biological Sciences, University of Auckland, Auckland, New Zealand
- 4Institute of Marine Science, University of Auckland, Auckland, New Zealand
Multi-species feeding associations (MSFAs) are temporary communities of animals exploiting the same or co-occurrent resources. Their dynamics are species dependent, often creating competitive interactions, but they can also increase foraging efficiency and ultimately individual fitness. The foraging behaviors of some species can enhance prey capture by others, with different roles depending on the species present. Here, we use the Hauraki Gulf, New Zealand, as a model system to quantitatively characterize the principal types of MSFAs between 2011 and 2020. We determine the foraging associations of a large generalist predator, the Bryde’s whale, and how shifts in its prey preference change the dynamics between seabird competitors. Hierarchical clustering from influential predator groups identified three types of MSFAs. Two of mainly fish-feeding predators, one including and one lacking Bryde’s whales, and one involving (although not limited to) plankton-feeders associated with Bryde’s whales. Cluster frequencies featured significant temporal trends, whereas MSFA diversity and whale association rate showed no significant changes. Bryde’s whales’ increasing reliance on zooplankton highlights their foraging plasticity, with changes in cluster frequencies and resource competition related to this shift from fish to zooplankton. The role of Bryde’s whales varies from joiners and terminators in fish-feeding aggregations to initiators with plankton-feeding seabird associations, thereby changing the MSFA dynamics. MSFAs tend toward a diversity equilibrium, i.e., a maximum number of species involved before competitive effects exceed the benefits of interaction. Functional MSFAs where heterospecific interactions are important to foraging success can be affected by changing composition. Future work should focus on the behavioral interactions between key predators, prey availability and their effect on MSFAs.
Introduction
The complexity of community interactions around resource use has typically focused on competition and exclusion, but the importance of beneficial interactions such as facilitation are increasingly considered in population- and community-level processes (Stachowicz, 2001; Bruno et al., 2003). Animals that join heterospecific groups can gain multiple benefits through reciprocal attraction to resources and complementary predation strategies increasing feeding success for species and individuals (Lang and Farine, 2017). Multi-species feeding associations (MSFAs) are temporary ensembles of predators jointly exploiting patches of prey (Goodale et al., 2010). They differ from other interspecific foraging phenomena such as intraguild predation, where interactions between species consuming the same prey may shift as one of the species becomes predated upon (Holt and Polis, 1997). Intraguild predation may limit energy flows between trophic levels, but if sufficient biomass and biodiversity are provided it can play an important functional role in marine systems (Wang et al., 2019). The feeding associations of cetaceans, seabirds and large fish predators addressed in our study do not involve intraguild predation, but rather represent interactions between large marine predators ranging from competition to mutualism. The ubiquity of heterospecific foraging across marine and terrestrial habitats is strong evidence for its ecological importance even in the presence of competition for resources (Veit and Harrison, 2017). According to the feeding efficiency theory, species using the same resource with different strategies, even when competing for shared prey, can complement each other, maximizing their feeding efficiency and reducing interspecific competition (Monkkonen et al., 1996; Camphuysen and Webb, 1999; Zuluaga, 2013). Consequently, species that participate in MSFAs are likely to increase their fitness (Goodale et al., 2010; Lett et al., 2014).
Multi-species feeding associations occur in all marine ecosystems, from coastal to pelagic environments, and involve many top predator species including marine mammals, seabirds and large predatory fish (e.g., Orgeira, 2004; Anderson and Lovvorn, 2008; Hebshi et al., 2008; Goyert et al., 2014). Although these associations may seem coincidental co-occurrences around a shared food resource, recent studies suggest they involve some sort of commensalism or mutualism (e.g., Anderwald et al., 2011; Lett et al., 2014; Jourdain and Vongraven, 2017). Although exploitation of other individuals and species does exist within these communities and the benefits for some species are not always obvious, some suggest MSFAs should generally be considered mutualistic (Lett et al., 2014; Thiebault et al., 2016). Previous studies have attempted to classify species based on their role in MSFAs, defining categories such as initiators, catalysts, joiners, scroungers, disruptors or terminators (Camphuysen and Webb, 1999; Anderwald et al., 2011). The cooperation between species, beneficial for at least one and with no negative effect on either species, as shown when cetacean or large fish predators aggregate prey at the surface making it more accessible to seabirds, is an example of facilitation (Au and Pitman, 1986; Degrati et al., 2014). Plankton feeding seabirds such as fulmars (Fulmarus spp.) and storm petrels (Hydrobatinae and Oceanitinae) (Heather et al., 2015) associate with baleen whales as their commensals, taking amounts of food negligible for the whale, although, with reference to the ecosystem overall, competition does exist through the shared prey preferences.
There are many examples of local enhancement in MSFAs, whereby cues to locate prey are provided by the presence or behaviors of other animals (Goyert et al., 2014). Surface-feeding cetaceans and large seabird flocks are likely visual attractors to prey patches (Mehlum et al., 1998; Grunbaum and Veit, 2003). While specialist predators such as obligate fish-feeders are tied to one or few prey types and thus tend to associate with a specific range of other predators, generalist feeders with more flexible diets may be receptive to more cues and can participate in a wider range of associations. This also facilitates the potential for different or switching roles in the ephemeral communities formed around foraging events. Baleen whales can act as facilitators by aggregating prey, thereby making it available to other species (Sharpe and Dill, 1997), but they also often terminate feeding events by consuming most of the available prey (Haynes et al., 2011; Kahane-Rapport and Goldbogen, 2018). Flexible foraging can influence indirect trophic interactions such as bottom-up and top-down control, but our understanding of how predator species with shifting feeding strategies interact and how their reciprocal adaptations may shape their evolutionary paths is still limited (Abrams, 2010). Bryde’s whales (Balaenoptera edeni spp.) have some of the most diverse foraging behaviors and a flexible diet (Constantine et al., 2018) but their role in MSFAs has rarely been investigated (e.g., Haynes et al., 2011; Jourdain and Vongraven, 2017).
Although participating species have been attributed functional roles in previous MSFA studies (e.g., Diamond, 1981; Anderwald et al., 2011), to our knowledge no quantitative characterization of different types of MSFAs has been attempted, making it difficult to provide a general framework to be used when describing these associations. Furthermore, despite the importance of marine MSFAs for the ecological interactions within marine communities, no previous work has analyzed temporal trends in MSFA composition and diversity.
The Hauraki Gulf – Tīkapa Moana – Te Moananui-ā-Toi hosts resident and transitory large marine vertebrates including cetaceans, pinnipeds and sharks (Gaskin and Rayner, 2013; Colbert, 2019; Hamilton, 2020), and is a global hotspot for seabird biodiversity with ∼25% of the known seabird taxa in the region, including four endemics (Gaskin and Rayner, 2013). Temporary concentration of prey favors the formation of feeding aggregations, often characterized by common dolphins (Delphinus delphis), Bryde’s whales and seabirds such as Australasian gannets (Morus serrator), shearwaters, petrels (Procellariidae) and terns (Sterninae). There are four cetacean species present year-round (Colbert, 2019), including semi-resident Bryde’s whales (Baker and Madon, 2007; Tezanos-Pinto et al., 2017; Izadi, 2018). Bryde’s whales exhibit behavioral plasticity using lunge- and sometimes skim-feeding techniques when feeding on fish (Izadi, 2018), and when feeding on zooplankton they use a novel behavior known as head slapping to concentrate prey in the water before engulfing it (Izadi, 2018). Recent work has suggested a possible shift toward targeting more planktonic prey (Carroll et al., 2019). Their versatility in diet and feeding strategies allows associations with a wide range of predators, which make them an interesting species in MSFA studies.
Here, we aim to (1) identify types of MSFAs based on seabird and cetacean species through hierarchical clustering; (2) reveal temporal trends in MSFA diversity; (3) test for temporal shifts in species composition of MSFAs by autoregressive modeling; and (4) determine whether such shifts are related to changes in prey preference. We hypothesize that a generalist predator, the Bryde’s whale, will play different roles within MSFAs dependent on their prey preference and that this will influence competition dynamics within the MSFAs.
Materials and Methods
Study Area
The Hauraki Gulf – Tīkapa Moana – Te Moananui-ā-Toi (hereafter the Gulf) is a shallow embayment (maximum depth ∼ 60 m) on the northeast coast of Aotearoa New Zealand (Figure 1). During the austral summer and autumn (warm seasons), the East Auckland Current maintains a southward flow of warm water and onshore winds prevail. In austral winter and spring (cold seasons), offshore winds promote upwelling of cool, nutrient-rich water from the continental shelf, resulting in increased productivity (Chang et al., 2003). Sea surface temperature ranges from 12°C in August/September to 25°C in January/February (Shears and Bowen, 2017).
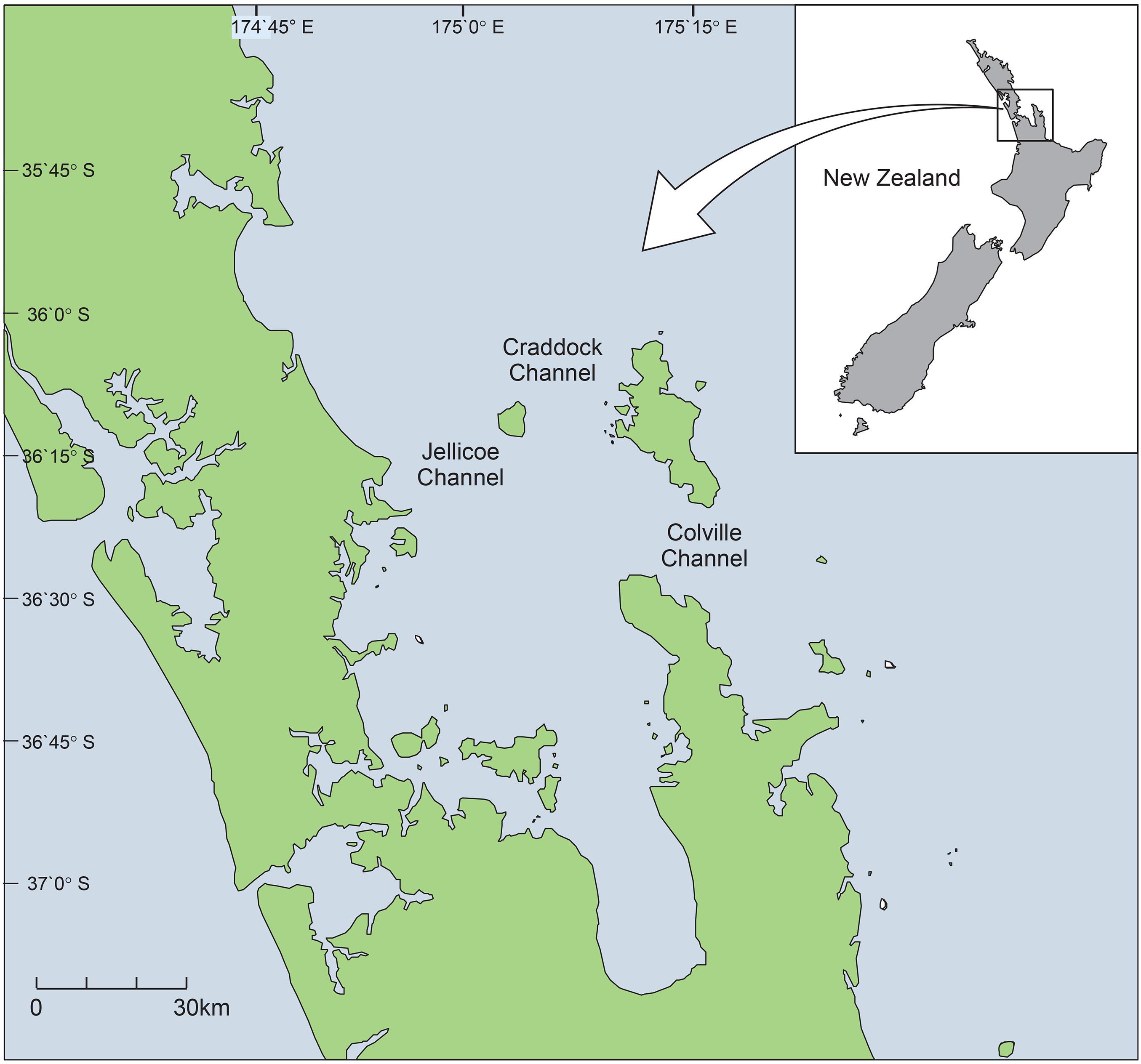
Figure 1. Map of the study area, the Hauraki Gulf, and its location on the North Island, New Zealand.
Data Collection and Organization
Observations were opportunistically collected between 2011 and 2020 from the 20 m Dolphin Explorer whale watch vessel operated by Auckland Whale and Dolphin Safari (AWADS). AWADS ran one 4.5 h trip per day during the cold season (June to November) when weather (<20 kts wind) and passenger numbers allowed, and one to two trips per day in the warm season. Average vessel speed was 20 knots while searching for cetaceans and <5 knots during encounters. Between September 2019 and January 2020, we conducted additional surveys from the University of Auckland 15 m vessel RV Hawere on 2 to 3 days per month during favorable weather conditions (Beaufort ≤ 2).
When cetaceans were encountered, at least two observers collected information about location (GPS coordinates), species of cetaceans, seabirds, sharks and/or pinnipeds, predominant behavioral state, and prey (zooplankton or fish) when foraging occurred. Prey type was identified visually or based on the feeding tactic used by Bryde’s whales, which feature a peculiar head-slapping behaviour when feeding on zooplankton (Izadi, 2018). A total of 1,658 multi-species feeding events were recorded: 880 in the warm season and 778 in the cold season.
Data management was conducted in R Studio (Version 1.2.1335) using R software version 4.0.2. We audited the AWADS data to include only observations reporting foraging behavior and created a meta-dataset of all cetacean sightings from 2011 to 2020.
Species Composition of Multi-Species Feeding Associations
To investigate MSFA composition, we created a species presence-absence matrix including all records from September 2011 to March 2020 featuring two or more predator species where at least one of them was foraging. Rarely sighted cetacean taxa were grouped based on functional similarities (i.e., similar prey and feeding strategies) (Table 1). Due to changes in the AWADS sampling protocol, taxonomic resolution for seabirds was inconsistent, so functionally similar seabird species were also grouped in higher taxonomic units. Petrels and shearwaters, both procellariids, were kept separate as they are functionally different [shearwaters more actively hunting prey, petrels overall more inclined to scavenging, or plankton feeding in the case of storm or diving petrels (Gaskin et al., 2019)].
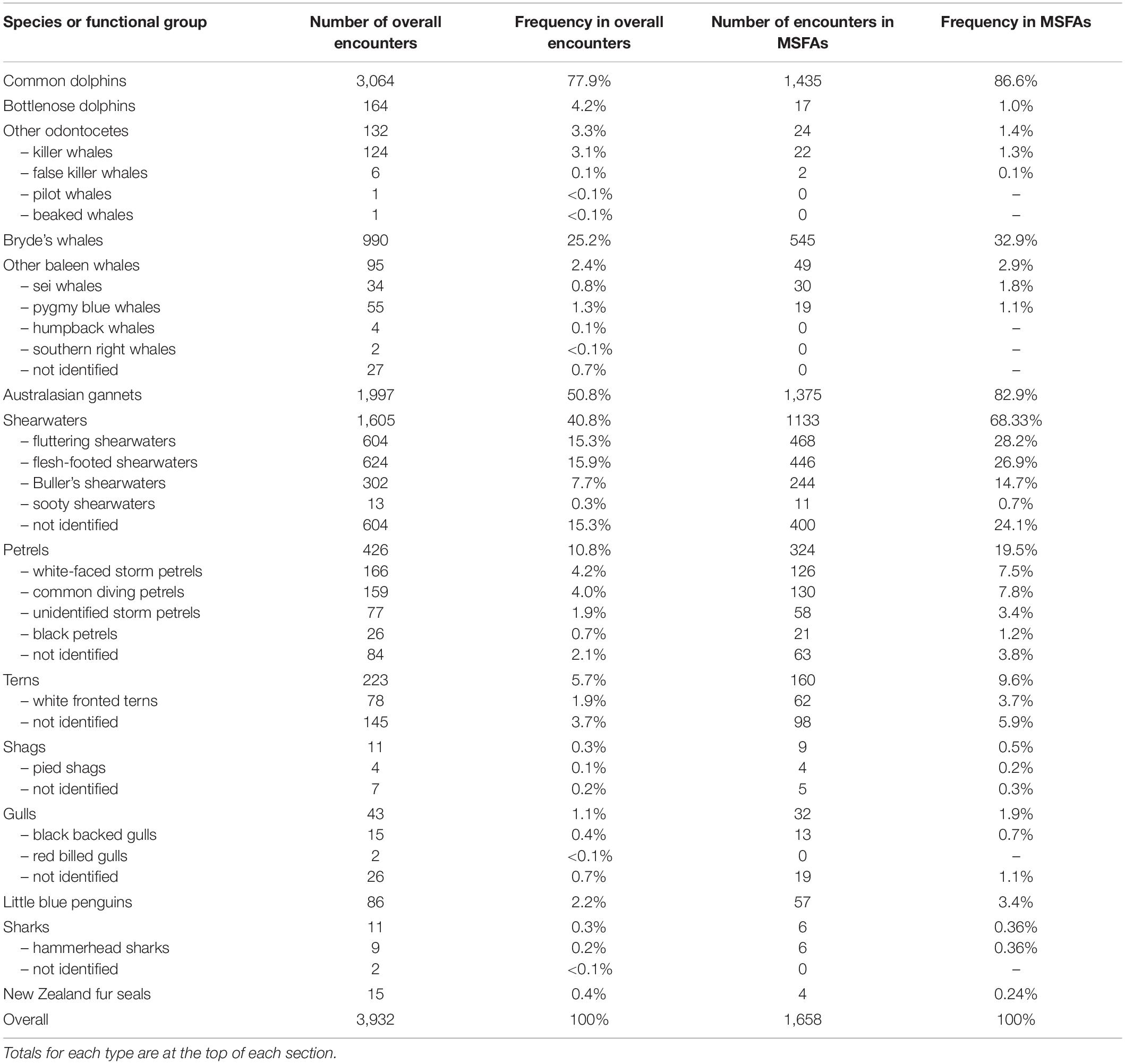
Table 1. The number and frequency (%) of different species and functional groups observed in multi-species feeding aggregations in the Hauraki Gulf, from 2011 to 2020.
To identify recurring combinations of predator species or functional groups in MSFAs, we performed hierarchical clustering using Ward’s (1963) linkage method (see also Latasa and Bidigare, 1998; Kramer et al., 2020) implemented in the function hclust (R Core Team, 2013). As a dissimilarity measure for predator associations we used Jaccard distance, commonly employed for presence-absence data (Borcard et al., 2018) implemented in the dist function. To determine the number of clusters we used the NbClust function (package NbClust), which calculates a spectrum of 26 indices for the significance of differences among clusters (Charrad et al., 2014). We set the maximum number of clusters (k) to 10 to obtain a division of meaningful biological interpretation. The most supported choices were a 10-cluster partition (optimizing five indices) and a three-cluster partition optimizing four, namely Marriot (based on optimum stratification; Marriott, 1970), TraceW (trace of the within-cluster scatter matrix, equivalent to the error sum of squares based on within-cluster differences; Milligan and Cooper, 1985; Maulik and Bandyopadhyay, 2002), TrCovW (trace of within-clusters pooled covariance matrix; Milligan and Cooper, 1985), and Ball (average distance of the observations to their cluster centroids; Milligan and Cooper, 1985). For the benefit of interpretability and manageability of the subsequent analysis steps, we adopted the three-cluster partition.
Once the clusters were defined, we calculated the frequencies of the different predator groups in each cluster along with confidence intervals based on the Agresti-Coull algorithm (Agresti and Coull, 1998; Brown et al., 2001). Influential species for between-cluster dissimilarities were identified by SIMPER (similarity percentages) analysis implemented in the package vegan (Oksanen et al., 2019). We then calculated the contribution of each cluster to the overall number of MSFAs by year and season. To ensure a sufficient number of observations per time step, data were pooled by two seasons, warm (December-May) and cold (June-November). Subsequently, we transformed the cluster proportions into time series objects (function ts, package stats) and modeled their behavior over time using autoregressive integrated moving average (ARIMA) modeling (Chatfield, 1995) allowing for seasonal (SARIMA) models, implemented in the function auto.arima (package forecast; Hyndman and Khandakar, 2008). This allowed us to compare all possible models within a range of values for each parameter [namely: lag order of autoregression (p), degree of differencing (d), moving average order (q), lag order of seasonal autoregression (P), degree of seasonal differencing (D) and seasonal moving average order (Q)], and for a maximum overall order (defined as p + q + P + Q), and select the best-fitting model based on AIC, AICc or BIC (i.e., Akaike, corrected Akaike or Bayesian Information Criterion) according to the method chosen (for details on ARIMA model parameters, we invite the reader to refer to Cowpertwait and Metcalfe, 2009). We set the maximum d to 2 to allow for both constant or constantly changing trends, and D to 1 to allow for seasonal differences. All other parameters were left flexible with an overall maximum order of 16 (allowing, for example, for a SARIMA (4,2,4) (4,1,4) [S] model, where S is the seasonal period).
The best model for each cluster identified through the ARIMA approach was then validated by visualizing the autocorrelation of the model residuals in an ACF-plot and the correspondence of sample and model quantiles by a QQ-plot, and by conducting a Ljung-Box test on the residuals (Hassani and Yeganegi, 2019). We also calculated p-values for the autoregressive and moving average terms to test their significance. Finally, the trend component of each time series was extracted with the decompose function and its significance verified through a Cox-Stuart test [function cox.stuart.test, package randtests (Caeiro and Mateus, 2014)].
Diversity of Species or Functional Groups in Multi-Species Feeding Associations
To analyze the temporal behavior of MSFA diversity, we used a relative “richness index” (the number of functional groups involved in each aggregation; Moore, 2013), as we were interested in changes in diversity over time and the taxonomically inconsistent sampling of seabirds made standard diversity indices unsuitable for our data. After taking the mean of this value for the warm and cold season of each year from 2011 to 2020, we performed ARIMA modeling on the resulting time series.
Bryde’s Whale Foraging Associations and Prey Preference
To test for changes in Bryde’s whale foraging associations, single-species (whales only) and multi-species (whales and associated predators) foraging events were aggregated by season (warm and cold) and year. The proportion of multi-species feeding for each time-step was transformed into a time series object and the best fitting model was selected through ARIMA modeling. We calculated confidence intervals for these proportions based on the Agresti-Coull algorithm (Agresti and Coull, 1998; Brown et al., 2001).
To investigate temporal trends in Bryde’s whale prey preference, we used data from 2011 to 2020 featuring Bryde’s whale foraging (lunging or head slaps). With few sightings of other baleen whales (Table 1), whales not identified to the species level (27 observations) were considered as Bryde’s whales (as per Wiseman, 2008) and included in the analysis.
For records without information on prey type, this was inferred from the presence or absence of indicative species. Obligate fish feeders (dolphins, Australasian gannets, flesh-footed shearwaters, white-fronted terns; Gaskin et al., 2019) engaged in foraging behavior indicate the presence of fish. Zooplankton was inferred from the presence of plankton feeding birds [storm petrels, diving petrels (Pelecanoides urinatrix)] and from the head slapping of Bryde’s whales. When no other species were associated with foraging whales, we categorized this as feeding on zooplankton, as Bryde’s whales feeding on fish are always associated with other piscivorous predators (Rochelle Constantine, personal observation) and they lunge-feed without head slaps (Izadi, 2018). Therefore, for records containing indications of both fish and zooplankton (e.g., Australasian gannets and storm petrels), but not head slapping, we deduced the prey type was fish.
We calculated the percentages of fish and zooplankton feeding for each season and year. For better visualization of temporal patterns, we initially calculated a time series using four seasons and the corresponding ACF. For more robust numbers of observations per time-step, we used two seasons (warm and cold) for the subsequent analysis. An ARIMA model was fitted to the data and model performance evaluated via diagnostic plots (ACF and Q-Q-plot) and the Ljung-Box test. Model coefficients were tested for significance and the trend component was validated by the Cox-Stuart test.
Results
Species Composition of Multi-Species Feeding Associations
Cetacean species in these MSFAs were mainly common dolphins (observed on 1,435 events; 86.5%) and Bryde’s whales (n = 545; 32.9%), less frequently bottlenose dolphins (Tursiops truncatus), killer whales (Orcinus orca), false killer whales (Pseudorca crassidens), pygmy blue whales (Balaenoptera musculus brevicauda), and sei whales (Balaenoptera borealis) (<2% each; Table 1). The seabirds most frequently recorded were Australasian gannets (82.9%), shearwaters (68.3%), and petrels (19.5%). Terns, little blue penguins (Eudyptula minor), gulls (Laridae), and shags (Phalacrocoracidae) occurred less frequently in MSFAs (<10% each; Table 1). Six records included hammerhead sharks (Sphyrna zygaena) and four included New Zealand fur seals (Arctocephalus forsteri).
Hierarchical clustering assigned 979 records to the first cluster (henceforth Fish1N), 352 to the second (PlankW), and 327 to the third (Fish2W) (Figure 2A). Cluster Fish1N represented almost exclusively fish-feeding events (99.7%; Figure 2B), with a predominance of piscivorous predators such as common dolphins (100%), Australasian gannets (97.8%) and shearwaters (73.3%), and occasionally killer and false killer whales (4.2%), whilst rarely involving Bryde’s whales (1.2%; Figure 2C). Cluster PlankW included both fish (54.5%) and zooplankton feeding events (45.5%) and a variety of predator groups, with a dominance of petrels (84.7%) and Bryde’s whales (58.9%), and occasionally other baleen whales (5.4%) and toothed cetacean (11.4%) species. Of the 206 Bryde’s whale observations in this cluster, 192 (93%) were zooplankton feeding events. Comparing the frequencies of single procellariid species by cluster using the subset of data containing species-specific information on seabirds (2013 to 2020) revealed a dominance of storm and diving petrels (present in 15.1 and 33.8% of the observations in this cluster, respectively). In cluster Fish2W, Bryde’s whales were present in 100% of the observations (92.4% feeding on fish) and common dolphins almost as frequent (99.9%), followed by Australasian gannets (76.5%) and shearwaters (64.2%). Confidence intervals for the estimates of prey type proportion and of predator frequency estimates can be found in Supplementary Tables 1, 2, respectively.
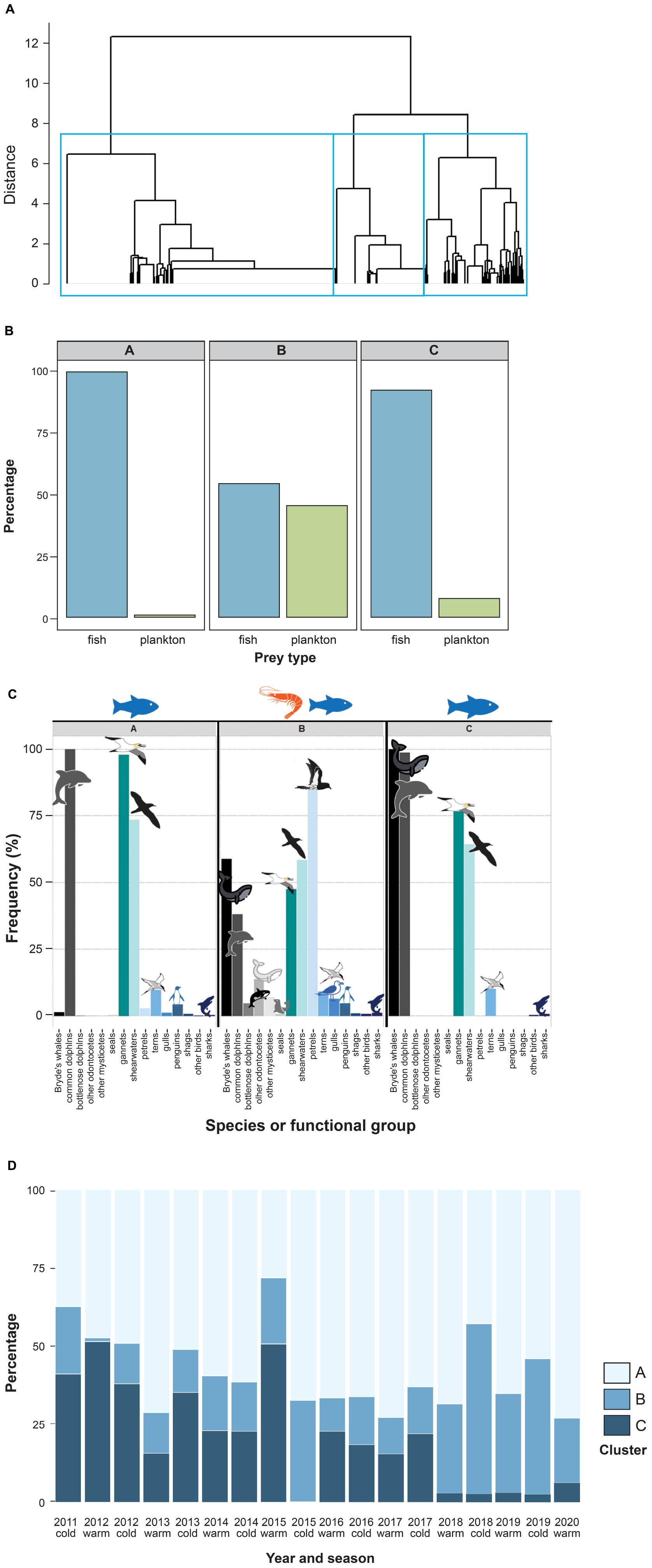
Figure 2. Results of cluster analysis on multi-species feeding aggregations from 2011 to 2020 in the Hauraki Gulf. (A) Cluster dendrogram of MSFA observations (n = 1,658), obtained by hierarchical clustering using Jaccard distances and Ward’s clustering algorithm. The rectangles show the three-cluster partition. The lowest level of the tree has been cut to avoid visual overlap due to the large dataset. (B) Frequency of main prey types (plankton vs. fish) by cluster. (C) Frequency of different functional groups within the clusters identified by the hierarchal clustering. (D) Temporal patterns of the cluster frequencies by season and year; top – frequency of cluster Fish1N; center – frequency of cluster PlankW; bottom – frequency of cluster Fish2W.
Similarity percentage analysis (SIMPER) identified petrels, common dolphins, Bryde’s whales and Australasian gannets as the most influential species for differences between clusters Fish1N and PlankW, Bryde’s whales and shearwaters for clusters Fish1N and Fish2W and petrels, common dolphins, Australasian gannets and shearwaters for clusters PlankW and Fish2W (Table 2).
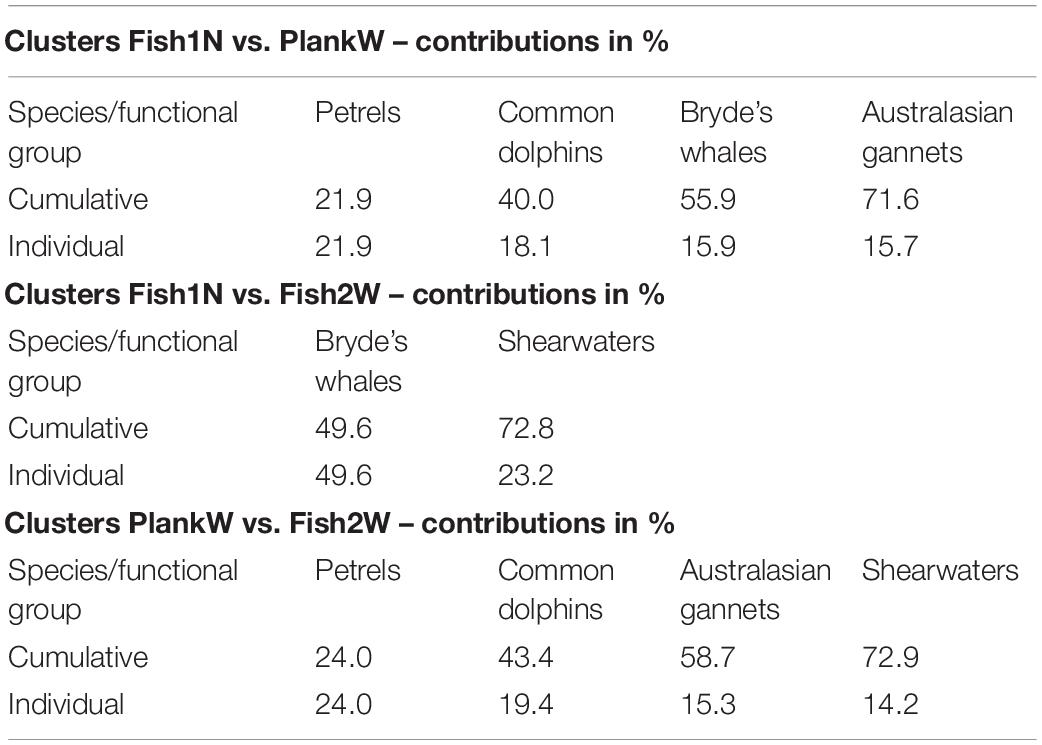
Table 2. Percentage of main species or functional groups contributing to pairwise dissimilarities between clusters of multi-species feeding aggregations identified by similarity percentages analysis (SIMPER) from 2011 to 2020 in the Hauraki Gulf.
The frequencies of all clusters revealed strong temporal variation (Figure 2D). Clusters Fish1N and PlankW became more frequent over the 10-year period, while cluster Fish2W decreased in frequency. Cluster Fish1N predominated throughout, with frequencies between 37 and 73% (except for summer-autumn 2015, 28%). In the time series of the three clusters, cluster Fish2W showed no significant autocorrelation (Supplementary Figure 1). The same models were selected regardless the information criterion used (AIC, AICc or BIC).
The cluster Fish1N time series was identified as a first-order moving average process without seasonality but with a linear trend [ARIMA (0,1,1)]. Diagnostic plots (Supplementary Figure 2) displayed good correspondence of observed and predicted values, with no significant residual autocorrelation. The Cox-Stuart test supported the existence of an increasing trend (Table 3).
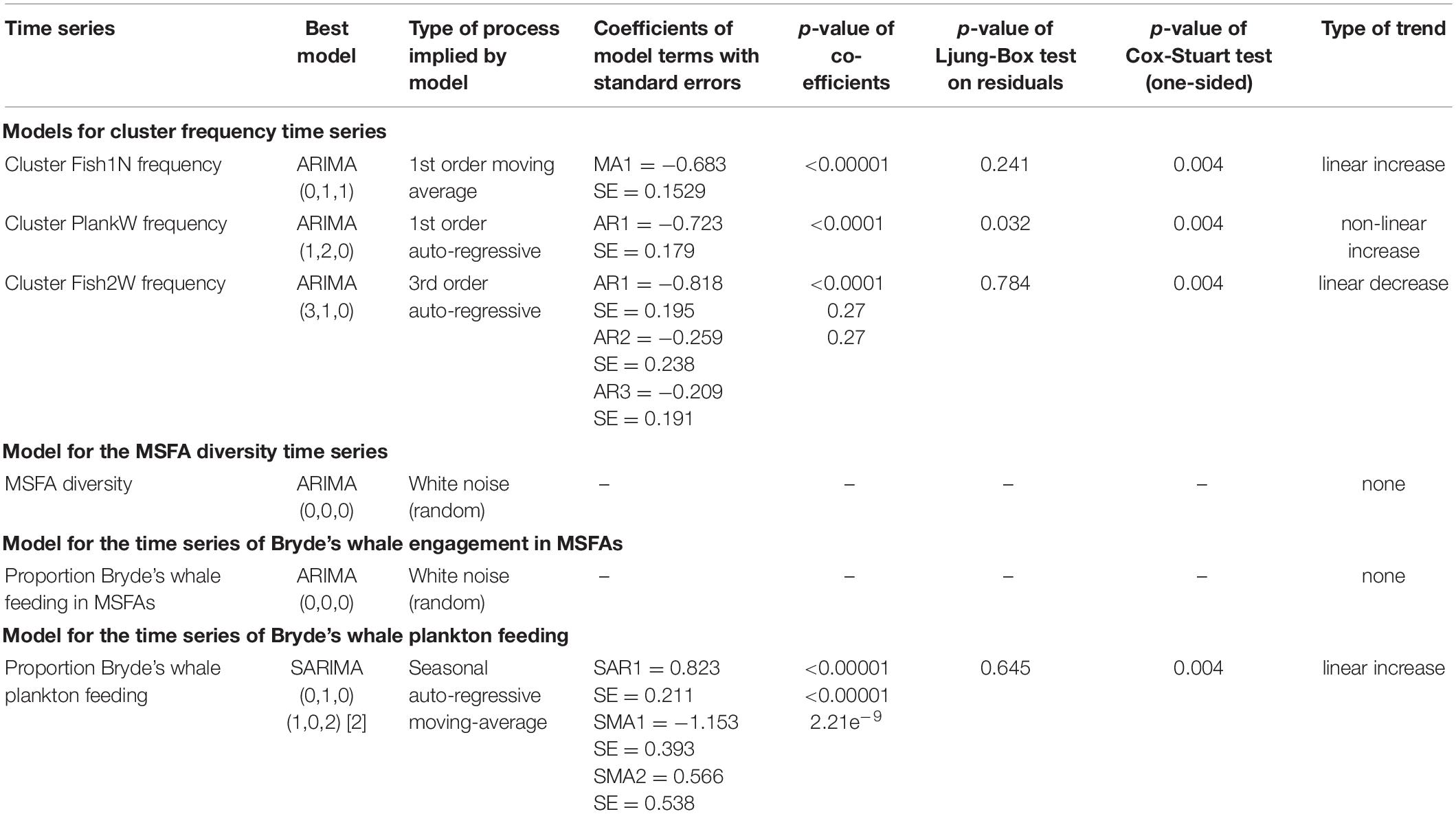
Table 3. Model parameters and diagnostics (where applicable) of models selected through auto.arima on multi-species feeding aggregations (MSFAs) from 2011 to 2020 in the Hauraki Gulf.
The model for the cluster PlankW time series showed a first-order autoregressive process with a non-linear trend [ARIMA (1,2,0)]. The model and the increasing trend were supported by the diagnostic tests (Table 3), despite relatively weak correspondence of sample and model quantiles (Supplementary Figure 2).
For cluster Fish2W, a third-order autoregressive process with a (downward) linear trend [ARIMA (3,1,0)] was detected. Of the three autoregressive terms, only the first was (highly) significant. Diagnostics presented a good fit to the data and the Cox-Stuart test supported the decreasing trend (Table 3; Supplementary Figure 2).
Diversity of Species or Functional Groups in Multi-Species Feeding Associations
Diversity analysis employed the same MSFA records as used in cluster analysis (n = 1,658). The number of functional groups involved ranged from two to seven, with a relatively constant seasonal mean (range = 2.96 to 3.39 except for summer-autumn 2015 (2.52); Figure 3A). With the low variability of this time series, the auto.arima approach delivered an ARIMA (0,0,0) model, i.e., a constant phenomenon with random oscillations, but no trend or periodicity (Supplementary Figure 3).
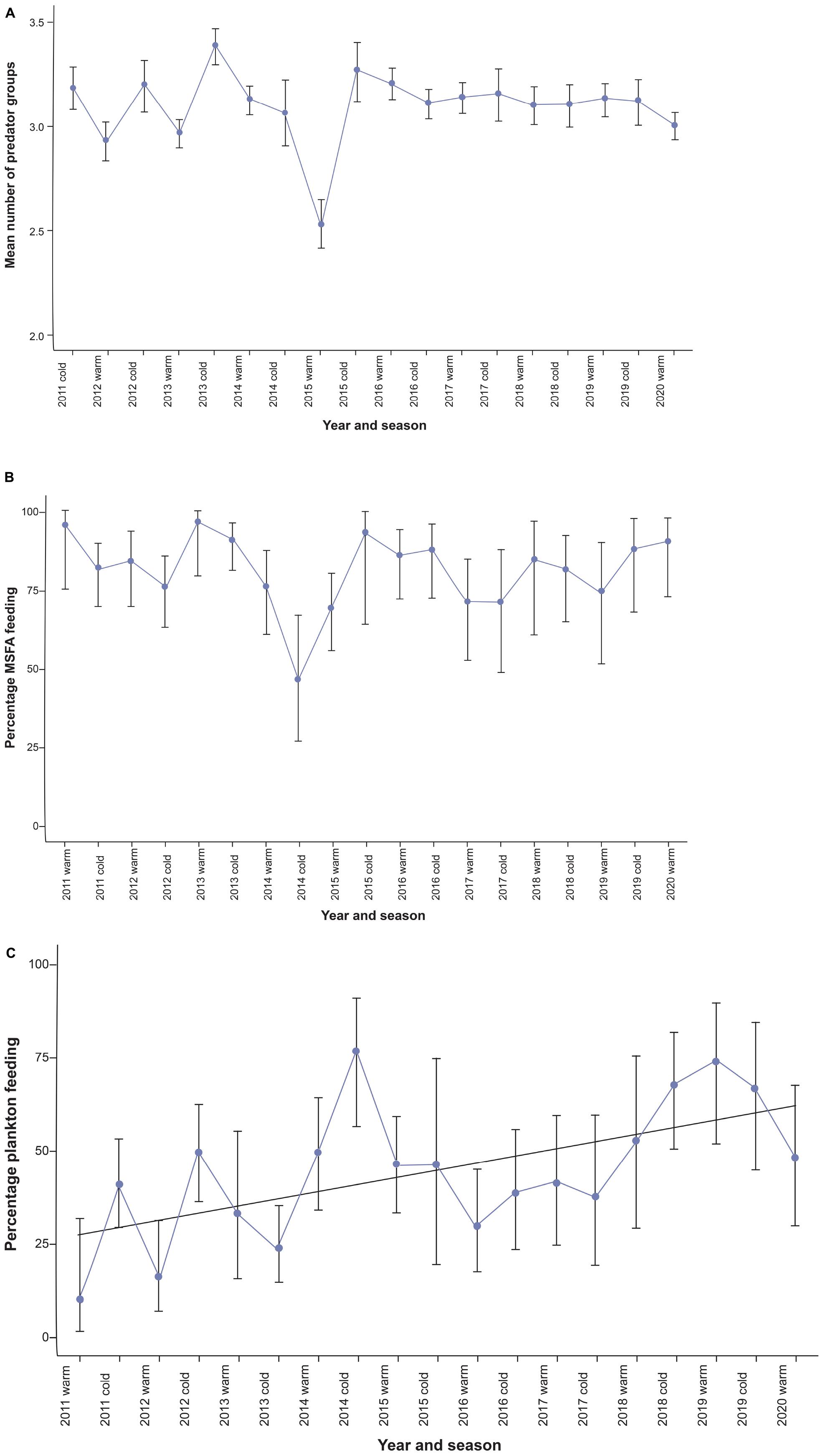
Figure 3. (A) Seasonal (cold vs. warm seasons) time series of diversity of multi-species feeding aggregations (black bars represent the standard error). (B) seasonal time series of the proportion of Bryde’s whales feeding within MSFAs (black bars show the Clopper-Pearson confidence intervals). (C) Bryde’s whales’ reliance on plankton as a food resource from 2011 to 2020 in the Hauraki Gulf (black bars show the Clopper-Pearson confidence intervals).
Bryde’s Whale Foraging Associations
Foraging behavior was recorded during 710 (69.9%) out of 1,017 Bryde’s whale observations (which included 27 unidentified baleen whale sightings counted as Bryde’s whales). Other baleen whale species were identified in 95 encounters (Table 1). Bryde’s whales associated with other species in 80.8% (SE = 2.7%; range = 70–96%) of the cases of each seasonal time-step, except for one outlier in winter-spring 2014 (46%).
The time series of the proportion of multi-species foraging events (Figure 3B and Supplementary Table 3) did not show significant autocorrelation (Supplementary Figure 4) and the best-fitting model was again a “null model” [ARIMA (0,0,0)]. Thus, based on our data, there was no evidence for either a trend or seasonality in the tendency of Bryde’s whales to engage in heterospecific foraging events (Table 3) (see discussion). What did change was the cluster they were associated with. Bryde’s whales in the Gulf prevalently fed in association with other predators, and the proportion of observations with Bryde’s whales feeding alone (overall = 19.3%) could be slightly overestimated due to incomplete reporting of birds, seals or sharks present in low numbers and/or for a short time during a foraging event.
Bryde’s Whale Prey Preference
To determine Bryde’s whale prey preference we analyzed the same 710 whale feeding records as above, which included 353 (49.7%) fish-feeding and 302 (42.5%) plankton-feeding observations, as well as 55 (7.7%) records with indications of the presence of both fish and zooplankton. Based on the whales’ foraging behavior (Izadi, 2018), we counted these 55 records as fish feeding events increasing the total to 408 (52.2%). The time plot of percent zooplankton feeding over four seasons suggested an upward trend and seasonal oscillations, with increased reliance on plankton during the colder months (Supplementary Figure 6). The latter were supported by the time series showing significant autocorrelation at lag 4 (Supplementary Figure 5A).
For the warm and cold season time series, a SARIMA (0,1,0) (1,0,2) [2] was selected. Coefficients were highly significant (p < 0.0001 for both SMA1 for SMA2). Supported by good diagnostics (Table 3 and Supplementary Figure 5), this model identified the prey preference time series as a seasonal (warm – cold) autoregressive moving-average process with a linear upward trend.
Discussion
Here, we reveal three primary types of MSFAs of large marine predators - cetaceans, seabirds, sharks and seals, in a productive shallow marine habitat. The relative frequencies of these aggregation types were not constant over time. Clusters Fish1N, dominated by fish feeding birds and dolphins and virtually lacking Bryde’s whales, and PlankW, containing the majority of plankton feeding events, showed increasing trends, whereas the frequency of cluster Fish2W, characterized by whale-dolphin associations feeding on fish, significantly decreased over time. The majority of Bryde’s whale feeding events occurred within MSFAs throughout the 10-year study period, and no significant trend or seasonality was detected in their engagement in MSFAs, but their interspecific associations shifted toward a prevalence of plankton feeders. Feeding associations comprised a relatively constant number of functional groups of cetaceans and seabirds, which have not undergone major changes over the last 10 years, suggesting a diversity equilibrium within MSFAs in the Gulf, i.e., a maximum number of species that can be involved before competitive effects exceed the benefits of interspecific interaction.
Species Composition and Diversity in Multi-Species Feeding Associations
In terms of MSFA clusters, we expected different combinations of predators based on the type of prey; fish and zooplankton attracting piscivorous and planktivorous species, respectively, plus generalist feeders in both cases. Fish-based MSFAs might or might not include the generalist feeders Bryde’s whales, and this was reflected by clusters Fish2W and Fish1N, respectively. Potentially the same holds for aggregations of plankton feeders; however, given the rare presence of other planktivorous cetaceans such as pygmy blue and sei whales in the Gulf (Olson et al., 2015; Barlow et al., 2018), plankton-feeder associations not including Bryde’s whales are composed of seabirds only and thus not included in the data set. We therefore expected to find a single cluster of zooplankton based MSFAs, which is represented by cluster PlankW. As expected, this cluster revealed a very high frequency of petrels, with a dominance of the planktivorous common diving petrels and storm petrels, a high occurrence of Bryde’s whales and occasionally other baleen whales. However, this cluster also included obligate fish feeders like Australasian gannets and common dolphins, and occasionally coastal species (shags and bottlenose dolphins). This overlap may be due to opportunistic feeders such as gulls and terns, or seabirds feeding on zooplankton co-occurring with schooling fish (Zeldis and Willis, 2015), blurring the boundaries between fish- and zooplankton-feeding events. The association of shearwaters and common dolphins is an example of facultative commensalism, with the shearwaters benefitting from the dolphins’ activity but not critically depending on it (Degrati et al., 2014).
Given the competition between common dolphins, Bryde’s whales and gannets for fish, their high co-occurrence in MSFAs (especially in cluster Fish2W) was interesting. Although previous research identified Australasian gannets and common dolphins as predictors of Bryde’s whale presence (Baker and Madon, 2007; Stockin et al., 2009; Purvin, 2014; Izadi, 2018), direct observations of MSFA dynamics show Bryde’s whale feeding lunges often disrupt and terminate the feeding event (Rochelle Constantine, personal observation), as observed elsewhere for other species such as humpback whales (Megaptera novaeangliae) (Haynes et al., 2011).
Our three clusters could easily be generalized to other contexts for comparative studies of MSFA dynamics, provided the predator species are classified in functional groups based on their foraging ecology. Where additional groups are present, integration of additional MSFA types may be necessary. For instance, where two or more baleen whale species are common, different plankton-feeder clusters and corresponding competitive interactions could emerge, and other MSFA types would be expected where foraging behaviors differ strongly from those in the Gulf, such as associations between bottom-feeding gray whales (Eschrichtius robustus) and scavenger birds (Harrison, 1979).
Even though we did not have fine-scale records of the seabird taxa in feeding aggregations available, assigning functional groups enabled robust designation of clusters. Feeding associations comprised a relatively stable number of functional groups. This absence of a trend in diversity was not surprising. Given the trade-off between costs and benefits in heterospecific foraging, it is likely that a maximum number of functional groups exists which can be involved in a feeding aggregation before competitive effects outweigh the benefits of interspecific interactions. MSFAs in the Gulf are not a recent phenomenon and these predators have evolved long-term strategies that exploit the advantages and drawbacks of heterospecific foraging strategies, so this sort of diversity optimum had certainly been reached before our observations started. Nonetheless, in our study the number of groups involved became more constant over time, supporting further stabilization of an equilibrium (Figure 3A).
Bryde’s Whale Foraging and Associations
The majority of Bryde’s whales observed feeding in the Gulf were involved in MSFAs, consistent with observations by Penry et al. (2011) in South Africa. Bryde’s whales accounted for ∼50% of the differences between clusters A and C, implying that whales tended to associate with some species whilst avoiding (or being avoided by) others. The high overall association rate raises the question whether Bryde’s whales engage in MSFAs because they benefit from them, or if it is mainly the other species joining them to increase feeding success or through shared prey preference. Baleen whales rely on multiple sensory systems for foraging at different spatial scales (Torres, 2017). Given the acoustic detection abilities of Bryde’s whales (Constantine et al., 2018) and the overlap in frequency ranges with dolphin and seabird acoustics, the whales may use acoustic cues to detect foraging aggregations. They possibly exploit olfactory cues to detect patches of zooplankton, as found in humpback whales (Bouchard et al., 2019). Surface feeding activity is a useful visual cue for scavenger species and plankton feeding birds like storm and diving petrels, which become commensals of whales by taking small amounts of food without representing relevant competitors (Harrison, 1979). This is supported by the importance of petrels in explaining cluster dissimilarities (Table 2), resulting from the strong association with Bryde’s whales.
By virtue of their generalist feeding habits and year-round presence, Bryde’s whales and potentially other baleen whales may play multiple roles in MSFAs depending on the species they associate with. They are joiners of aggregations initiated by dolphins, and often terminate them by engulfing large amounts of prey (Kahane-Rapport and Goldbogen, 2018). In addition, they likely act as catalysts attracting other species to these events, and as initiators when they are the first to detect prey and attract others through surface-active behaviors. Thus, cetaceans with flexible diet and foraging strategies (i.e., generalist feeders) may be key players in the complex interactions within MSFAs depending on the target prey and on their associates.
Our findings revealed seasonality in Bryde’s whales’ reliance on zooplankton, with a higher frequency of MSFAs feeding on zooplankton in the cold season, when upwelling enhances its availability (Chang et al., 2003; Carroll et al., 2019). Furthermore, 12 years ago fish used to be the predominant prey (Wiseman, 2008), but preference for zooplankton is increasing (see also Carroll et al., 2019). The prey shifts highlight Bryde’s whales’ foraging plasticity both over the long- and short-term including seasonal shifts between fish and zooplankton, within a single population. This may be important for the whales’ tolerance to environmental shifts potentially affecting prey availability (Colbert, 2019; Hauraki Gulf Forum, 2020).
The relative frequencies of the three MSFA types changed over time, with a marked decline of cluster Fish2W characterized by common dolphins feeding on fish in association with Bryde’s whales, whereas the frequencies of clusters Fish1N - mainly seabirds and dolphins feeding on fish, and PlankW - high occurrence of Bryde’s whales feeding on plankton, are increasing (Figure 2D). With the growing reliance of Bryde’s whales on zooplankton, they are progressively abandoning the association with dolphins feeding on fish (cluster Fish2W) in favor of zooplankton (i.e., increasingly contributing to cluster PlankW), leaving dolphins and associated seabirds to feed on fish as shown by the increased frequency of cluster Fish1N. A recent study using DNA metabarcoding to determine zooplankton communities in the Gulf revealed that even though there were seasonal shifts in community structure, preferred whale prey, i.e., copepods, salps and krill-like zooplankton, were present year-round (Carroll et al., 2019). Stocks of pilchards (Sardinops neopilchardus), anchovies (Engraulis australis), and mackerels (Trachurus spp.) are poorly understood (Hauraki Gulf Forum, 2020), and stock assessments are needed to clarify whether the shifts in whale prey preference and in the frequencies of MSFA types are related to prey depletion exacerbating the competition between whales and dolphins and making their foraging association less advantageous for the whales. Whether the whales are foraging on zooplankton as part of a prey availability driven response or as preferred prey remains unknown. Future efforts should aim to identify specific prey via underwater cameras, drone imagery, or the use of eDNA (see Carroll et al., 2019 for prey identification using scat and matched environmental controls), although the high phytoplankton productivity in the Gulf can make visual observations challenging.
Concluding Remarks and Recommendations
Prey consumption by cetaceans in the Gulf is shifting (see also Peters et al., 2020 on common dolphins), which is of interest for future studies of MSFAs. The effective role of seabirds in functional ecosystems within the Gulf requires reliable access to prey, critical for survival and reproduction. Seabirds are ecosystem indicators of nutritional stress (Dunphy et al., 2020), and any impact on prey availability should be considered alongside restoration efforts (Borrelle et al., 2015). Increased competition as a consequence of reduced prey availability is one of the most likely factors contrasting heterospecific foraging associations (Lang and Farine, 2017). With enhanced knowledge of prey dynamics, conservation measures can be adjusted to protect less-flexible foragers, which are more vulnerable to the depletion or redistribution of prey.
Put aside habitat related differences, MSFAs are ubiquitous in marine ecosystems, and the fitness of most participating species depends on these associations to some extent. Focused analyses of the short-term MSFA dynamics will enhance our understanding of the role each species plays in a particular association and reveal behavioral and relational complexities of MSFAs in time and space. To ensure the functioning of these temporary communities and their respective ecosystems, key initiator and catalyst species need to be safeguarded, and maintaining the abundance of prey organisms at their base is equally important. A better understanding of how they change over time will provide important insights on the effects of global and local changes in marine ecosystems.
Data Availability Statement
The raw data supporting the conclusions of this article will be made available by the authors, without undue reservation.
Ethics Statement
The animal study was reviewed and approved by the University of Auckland Animal Ethics Committee.
Author Contributions
RC initiated the long-term research project. JG, AM, and RC designed the study and analysis. JG led the writing of the manuscript. All authors critically reviewed the final manuscript.
Funding
JG was partially funded by a University of Pisa scholarship for international mobility. This research was partially funded by G.I.F.T – Foundation North, the Chisholm Whitney Charitable Trust, the Auckland Council, University of Auckland Alumni, Department of Conservation, and the Waiheke Brewing Company.
Conflict of Interest
The authors declare that the research was conducted in the absence of any commercial or financial relationships that could be construed as a potential conflict of interest.
Publisher’s Note
All claims expressed in this article are solely those of the authors and do not necessarily represent those of their affiliated organizations, or those of the publisher, the editors and the reviewers. Any product that may be evaluated in this article, or claim that may be made by its manufacturer, is not guaranteed or endorsed by the publisher.
Acknowledgments
We thank all the AWADS crew and management, in particular Cat Lea and Andy Light, for collecting and curating the data and for many years of close collaboration. Many thank to the tangata whenua (Indigenous Māori) of Tīkapa Moana – Te Moananui-ā-Toi for supporting our mahi. Many thanks to Peter Browne, Jessie Colbert, Brady Doak, Olivia Hamilton, Leena Riekkola, Peter Schlegel, Esther Stuck, Edin Whitehead (University of Auckland), Vivian Ward and Federica Spina and Luca Ruggirello (University of Pisa), and to the late Richelle Kahui-McConnell for their help with fieldwork, analysis, graphics, and many valuable discussions about this work. This research was conducted under permits to RC from the New Zealand Department of Conservation – Te Papa Atawhai and University of Auckland Animal Ethics Committee.
Supplementary Material
The Supplementary Material for this article can be found online at: https://www.frontiersin.org/articles/10.3389/fmars.2021.739894/full#supplementary-material
References
Abrams, P. A. (2010). Implications of flexible foraging for interspecific interactions: lessons from simple models. Funct. Ecol. 24, 7–17. doi: 10.1111/j.1365-2435.2009.01621.x
Agresti, A., and Coull, B. (1998). Approximate is better than “exact” for interval estimation of binomial proportions. Am. Statist. 52, 119–126. doi: 10.1080/00031305.1998.10480550
Anderson, E. M., and Lovvorn, J. R. (2008). Gray whales may increase feeding opportunities for avian benthivores. Mar. Ecol. Progr. Ser. 360, 291–296. doi: 10.3354/meps07359
Anderwald, P., Evans, P. G. H., Gygax, L., and Hoelzel, A. R. (2011). Role of feeding strategies in seabird-minke whale associations. Mar. Ecol. Progr. Ser. 424, 219–227. doi: 10.3354/meps08947
Au, D. W. K., and Pitman, R. L. (1986). Seabird interactions with dolphins and tuna in the Eastern Tropical Pacific. Condor 88, 304–317. doi: 10.2307/1368877
Baker, A. N., and Madon, B. (2007). Bryde’s Whales (Balaenoptera cf. Brydei Olsen 1913) in the Hauraki Gulf and Northeastern New Zealand Waters. Wellington: Department of Conservation.
Barlow, D. R., Torres, L. G., Hodge, K. B., Steel, D., Baker, C. S., Chandler, T. E., et al. (2018). Documentation of a New Zealand blue whale population based on multiple lines of evidence. Endanger. Species Res. 36, 27–40. doi: 10.3354/esr00891
Borcard, D., Gillet, F., and Legendre, P. (2018). Numerical Ecology With R, 2 Edn. USA: Springer International Publishing. doi: 10.1007/978-3-319-71404-2
Borrelle, S. B., Buxton, R. T., Jones, H. P., and Towns, D. R. (2015). A GIS-based decision-making approach for prioritizing seabird management following predator eradication. Restor Ecol. 23, 580–587. doi: 10.1111/rec.12229
Bouchard, B., Barnagaud, J. Y., Poupard, M., Glotin, H., Gauffier, P., Ortiz, S. T., et al. (2019). Behavioural responses of humpback whales to food-related chemical stimuli. PLoS One 14:e0212515. doi: 10.1371/journal.pone.0212515
Brown, L. D., Cai, T. T., and DasGupta, A. (2001). Interval estimation for a binomial proportion. Statist. Sci. 16, 101–107. doi: 10.1214/ss/1009213286
Bruno, J. F., Stachowicz, J. J., and Bertness, M. D. (2003). Inclusion of facilitation into ecological theory. Trends Ecol. Evol. 18, 119–125. doi: 10.1016/S0169-5347(02)00045-9
Caeiro, F., and Mateus, A. (2014). Randtests: Testing Randomness in R. R package version 1.0. Available online at: https://CRAN.R-project.org/package=randtests (accessed 09 October 2020).
Camphuysen, K., and Webb, A. (1999). Multi-species feeding associations in North Sea seabirds: jointly exploiting a patchy environment. Ardea 87, 177–198.
Carroll, E. L., Gallego, R., Sewell, M. A., Zeldis, J., Ranjard, L., Ross, H. A., et al. (2019). Multi-locus DNA metabarcoding of zooplankton communities and scat reveal trophic interactions of a generalist predator. Sci. Rep. 9:281. doi: 10.1038/s41598-018-36478-x
Chang, F. H., Zeldis, J., Gall, M., and Hall, J. (2003). Seasonal and spatial variation of phytoplankton assemblages, biomass and cell size from spring to summer across the north-eastern New Zealand continental shelf. J. Plankton Res. 25, 737–758. doi: 10.1093/plankt/25.7.737
Charrad, M., Ghazzali, N., Boiteau, V., and Niknafs, A. (2014). Nbclust: an R package for determining the relevant number of clusters in a data set. J. Statist. Softw. 61, 1–36. doi: 10.18637/jss.v061.i06
Chatfield, C. (1995). The Analysis of Time Series - an Introduction (C. Hall/CRC Ed, 5 Edn. United Kingdom: University of Bath.
Colbert, J. (2019). Sea Surface Temperature and Cetacean Distribution in the Hauraki Gulf, New Zealand. New Zealand: University of Auckland.
Constantine, R., Iwata, T., Nieukirk, S. L., and Penry, G. S. (2018). Future directions in research on Bryde’s whales. Front. Mar. Sci. 5:333. doi: 10.3389/fmars.2018.00333
Cowpertwait, P. S. P., and Metcalfe, A. V. (2009). Introductory Time Series With R. New York, NY: Springer Science+Business Media. doi: 10.1007/978-0-387-88698-5_1
Degrati, M., Dans, S. L., Garaffo, G. V., and Crespo, E. A. (2014). Seabird and dolphin associations: do seabirds benefit from feeding in association with dusky dolphins in Patagonia? J. Mar. Biol. Assoc. U. K. 94, 1147–1153. doi: 10.1017/s0025315413000945
Dunphy, B. J., Vickers, S. I., Zhang, J., Sagar, R. L., Landers, T. J., Bury, S. J., et al. (2020). Seabirds as environmental indicators: foraging behaviour and ecophysiology of common diving petrels (Pelecanoides urinatrix) reflect local-scale differences in prey availability. Mar. Biol. 167:53. doi: 10.1007/s00227-020-3672-4
Gaskin, C., Adams, N., Kozmian-Ledward, L., and Jeffs, A. (2019). Indirect Effects on Seabirds in the Northern North Island Region. Final Report on Comparison of Fish Shoals and Seabird Diet Prepared by NNZST for the Conservation Services Programme POP2017-06. New Zealand: Department of Conservation.
Gaskin, C. P., and Rayner, M. J. (2013). Seabirds of the Hauraki Gulf. Natural History, Research and Conservation. Auckland: Hauraki Gulf Forum.
Goodale, E., Beauchamp, G., Magrath, R. D., Nieh, J. C., and Ruxton, G. D. (2010). Interspecific information transfer influences animal community structure. Trends Ecol. Evol. 25, 354–361. doi: 10.1016/j.tree.2010.01.002
Goyert, H. F., Manne, L. L., and Veit, R. R. (2014). Facilitative interactions among the pelagic community of temperate migratory terns, tunas and dolphins. Oikos 123, 1400–1408. doi: 10.1111/oik.00814
Grunbaum, D., and Veit, R. R. (2003). Black-browed albatrosses foraging on Antarctic krill: density-dependence through local enhancement? Ecology 84, 3265–3275. doi: 10.1890/01-4098
Hamilton, O. (2020). The Ecology of Large Marine Predators in the Hauraki Gulf. Auckland: University of Auckland.
Harrison, C. S. (1979). Association of marine birds and feeding gray whales. Condor 81, 93–95. doi: 10.2307/1367866
Hassani, H., and Yeganegi, M. R. (2019). Sum of squared ACF and the Ljung-Box statistics. Physica A 520, 81–86. doi: 10.1016/j.physa.2018.12.028
Hauraki Gulf Forum (2020). “State of our Gulf 2020” in Hauraki Gulf/Tīkapa Moana/Te Moananui-ā-Toi State of the Environment Report. (Auckland: Hauraki Gulf Forum).
Haynes, T. B., Nelson, S. K., and Padula, V. M. (2011). Dynamics of multi-species feeding associations in marine waters near Juneau. Alaska. Mar. Ornithol. 39, 227–233.
Heather, B., Robertson, H., and Onley, D. (2015). The Field Guide to the Birds of New Zealand, 4th Edn. New Zealand: Penguin Books.
Hebshi, A. J., Duffy, D. C., and Hyrenbach, K. D. (2008). Associations between seabirds and subsurface predators around Oahu. Hawaii. Aquat. Biol. 4, 89–98. doi: 10.3354/ab00098
Holt, R. D., and Polis, G. A. (1997). A theoretical framework for intraguild predation. Am. Nat. 149, 745–764. doi: 10.1086/286018
Hyndman, R. J., and Khandakar, Y. (2008). Automatic time series forecasting: the forecast package for R. J. Statist. Softw. 26, 1–22.
Jourdain, E., and Vongraven, D. (2017). Humpback whale (Megaptera novaeangliae) and killer whale (Orcinus orca) feeding aggregations for foraging on herring (Clupea harengus) in Northern Norway. Mamm. Biol. 86, 27–32. doi: 10.1016/j.mambio.2017.03.006
Kahane-Rapport, S. R., and Goldbogen, J. A. (2018). Allometric scaling of morphology and engulfment capacity in rorqual whales. J. Morphol. 279, 1256–1268. doi: 10.1002/jmor.20846
Kramer, S. J., Siegel, D. A., and Graff, J. R. (2020). Phytoplankton community composition determined from co-variability among phytoplankton pigments from the NAAMES field campaign. Front. Mar. Sci. 7:15. doi: 10.3389/fmars.2020.00215
Lang, S. D. J., and Farine, D. R. (2017). A multidimensional framework for studying social predation strategies. Nat. Ecol. Evol. 1, 1230–1239. doi: 10.1038/s41559-017-0245-0
Latasa, M., and Bidigare, R. R. (1998). A comparison of phytoplankton populations of the Arabian Sea during the Spring Intermonsoon and Southwest Monsoon of 1995 as described by HPLC-analyzed pigments. Deep Sea Res. Part 2 Top. Stud. Oceanogr. 45, 2133–2170. doi: 10.1016/s0967-0645(98)00066-6
Lett, C., Semeria, M., Thiebault, A., and Tremblay, Y. (2014). Effects of successive predator attacks on prey aggregations. Theor. Ecol. 7, 239–252. doi: 10.1007/s12080-014-0213-0
Marriott, F. H. C. (1970). A problem of optimum stratification. Biometrics 26, 845–847. doi: 10.2307/2528730
Maulik, U., and Bandyopadhyay, S. (2002). Performance evaluation of some clustering algorithms and validity indices. IEEE Transac. Patt. Anal. Mach. Intell. 24, 1650–1654. doi: 10.1109/TPAMI.2002.1114856
Mehlum, F., Hunt, G. L., Decker, M. B., and Nordlund, N. (1998). Hydrographic features, cetaceans and the foraging of thick-billed murres and other marine birds in the northwestern Barents Sea. Arctic 51, 243–252. doi: 10.14430/arctic1066
Milligan, G. W., and Cooper, M. C. (1985). An examination of procedures for determining the number of clusters in a data set. Psychometrika 50, 159–179. doi: 10.1007/bf02294245
Monkkonen, M., Forsman, J. T., and Helle, P. (1996). Mixed-species foraging aggregations and heterospecific attraction in boreal bird communities. Oikos 77, 127–136. doi: 10.2307/3545592
Moore, J. C. (2013). “Diversity, Taxonomic versus Functional” in Encyclopedia of Biodiversity 2nd Edn. ed. S. A. Levin (Waltham: Academic Press). 648–656. doi: 10.1016/b978-0-12-384719-5.00036-8
Oksanen, J., Blanchet, F. G., Friendly, M., Kindt, R., Legendre, P., McGlinn, D., et al. (2019). vegan: Community Ecology Package. R package version 2.5-6. Available online at: https://CRAN.R-project.org/package=vegan (accessed October 11, 2020).
Olson, P. A., Ensor, P., Olavarria, C., Bott, N., Constantine, R., Weir, J., et al. (2015). New Zealand blue whales: residency, morphology, and feeding behavior of a little-known population. Pacific Sci. 69, 477–485. doi: 10.2984/69.4.4
Orgeira, J. L. (2004). Associations between seabirds and cetaceans in the South Atlantic Ocean and Antarctica. Ornitol. Neotrop. 15, 163–171.
Penry, G. S., Cockcroft, V. G., and Hammond, P. S. (2011). Seasonal fluctuations in occurrence of inshore Bryde’s whales in Plettenberg Bay, South Africa, with notes on feeding and multispecies associations. Afr. J. Mar. Sci. 33, 403–414. doi: 10.2989/1814232X.2011.637617
Peters, K. J., Bury, S. J., Betty, E. L., Parra, G. J., Tezanos-Pinto, G., and Stockin, K. A. (2020). Foraging ecology of the common dolphin Delphinus delphis revealed by stable isotope analysis. Marine Ecology Progress Series 652, 173–186. doi: 10.3354/meps13482
Purvin, C. M. (2014). The Influence of Multi-species Feeding Associations on the Foraging Behaviour of Australasian Gannets (Morus serrator) in the Hauraki Gulf, New Zealand. New Zealand: Massey University.
R Core Team (2013). R: A Language and Environment for Statistical Computing. Vienna: R Foundation for Statistical Computing.
Sharpe, F. A., and Dill, L. M. (1997). The behavior of Pacific herring schools in response to artificial humpback whale bubbles. Can. J. Zool. 75, 725–730. doi: 10.1139/z97-093
Shears, N. T., and Bowen, M. M. (2017). Half a century of coastal temperature records reveal complex warming trends in western boundary currents. Sci. Rep. 7:14527. doi: 10.1038/s41598-017-14944-2
Stachowicz, J. J. (2001). Mutualism, facilitation, and the structure of ecological communities. Bioscience 51, 235–246. doi: 10.1641/0006-3568(2001)051[0235:MFATSO]2.0.CO;2
Stockin, K. A., Binedell, V., Wiseman, N., Brunton, D. H., and Orams, M. B. (2009). Behavior of free-ranging common dolphins (Delphinus sp.) in the Hauraki Gulf. New Zealand. Mar. Mamm. Sci. 25, 283–301. doi: 10.1111/j.1748-7692.2008.00262.x
Tezanos-Pinto, G., Hupman, K., Wiseman, N., Dwyer, S. L., Baker, C. S., Brooks, L., et al. (2017). Local abundance, apparent survival and site fidelity of Bryde’s whales in the Hauraki Gulf (New Zealand) inferred from long-term photo-identification. Endanger. Species Res. 34, 61–73. doi: 10.3354/esr00839
Thiebault, A., Semeria, M., Lett, C., and Tremblay, Y. (2016). How to capture fish in a school? Effect of successive predator attacks on seabird feeding success. J. Anim. Ecol. 85, 157–167. doi: 10.1111/1365-2656.12455
Torres, L. G. (2017). A sense of scale: foraging cetaceans’ use of scale-dependent multimodal sensory systems. Mar. Mamm. Sci. 33, 1170–1193. doi: 10.1111/mms.12426
Veit, R. R., and Harrison, N. M. (2017). Positive interactions among foraging seabirds, marine mammals and fishes and implications for their conservation. Front. Ecol. Evol. 5:121. doi: 10.3389/fevo.2017.00121
Wang, S., Brose, U., and Gravel, D. (2019). Intraguild predation enhances biodiversity and functioning in complex food webs. Ecology 100:e02616. doi: 10.1002/ecy.2616
Ward, J. H. (1963). Hierarchical grouping to optimize an objective function. J. Am. Stat. Assoc. 58, 236–244. doi: 10.1080/01621459.1963.10500845
Wiseman, N. (2008). Genetic Identity and Ecology of Bryde’s Whales in the Hauraki Gulf, New Zealand. New Zealand: University of Auckland.
Zeldis, J. R., and Willis, K. J. (2015). Biogeographic and trophic drivers of mesozooplankton distribution on the northeast continental shelf and in Hauraki Gulf, New Zealand. N. Zeal. J. Mar. Freshwater Res. 49, 69–86. doi: 10.1080/00288330.2014.955806
Keywords: foraging behavior and efficiency, heterospecific groups, feeding interactions, competition, cetacean, seabird
Citation: Gostischa J, Massolo A and Constantine R (2021) Multi-Species Feeding Association Dynamics Driven by a Large Generalist Predator. Front. Mar. Sci. 8:739894. doi: 10.3389/fmars.2021.739894
Received: 12 July 2021; Accepted: 30 September 2021;
Published: 20 October 2021.
Edited by:
Filipe Alves, Marine and Environmental Sciences Centre (MARE), PortugalReviewed by:
Jason Schmidt, University of Georgia, United StatesMarilia Bueno, State University of Campinas, Brazil
Copyright © 2021 Gostischa, Massolo and Constantine. This is an open-access article distributed under the terms of the Creative Commons Attribution License (CC BY). The use, distribution or reproduction in other forums is permitted, provided the original author(s) and the copyright owner(s) are credited and that the original publication in this journal is cited, in accordance with accepted academic practice. No use, distribution or reproduction is permitted which does not comply with these terms.
*Correspondence: Rochelle Constantine, ci5jb25zdGFudGluZUBhdWNrbGFuZC5hYy5ueg==
†These authors have contributed equally to this work
‡ORCID: Alessandro Massolo, orcid.org/0000-0002-6333-4281