- 1College of Fisheries and Ocean Sciences, University of Alaska Fairbanks, Fairbanks, AK, United States
- 2College of Fisheries and Ocean Sciences, University of Alaska Fairbanks, Juneau, AK, United States
- 3Department of Arctic and Marine Biology, UiT – The Arctic University of Norway, Tromsø, Norway
Community assembly theory states that species assemble non-randomly as a result of dispersal limitation, biotic interactions, and environmental filtering. Strong environmental filtering likely leads to local assemblages that are similar in their functional trait composition (high trait convergence) while functional trait composition will be less similar (high trait divergence) under weaker environmental filters. We used two Arctic shelves as case studies to examine the relationship between functional community assembly and environmental filtering using the geographically close but functionally and environmentally dissimilar epibenthic communities on the Chukchi and Beaufort Sea shelves. Environmental drivers were compared to functional trait composition and to trait convergence within each shelf. Functional composition in the Chukchi Sea was more strongly correlated with environmental gradients compared to the Beaufort Sea, as shown by a combination of RLQ and fourth corner analyses and community-weighted mean redundancy analyses. In the Chukchi Sea, epibenthic functional composition, particularly body size, reproductive strategy, and several behavioral traits (i.e., feeding habit, living habit, movement), was most strongly related to gradients in percent mud and temperature while body size and larval development were most strongly related to a depth gradient in the Beaufort Sea. The stronger environmental filter in the Chukchi Sea also supported the hypothesized relationship with higher trait convergence, although this relationship was only evident at one end of the observed environmental gradient. Strong environmental filtering generally provides a challenge for biota and can be a barrier for invading species, a growing concern for the Chukchi Sea shelf communities under warming conditions. Weaker environmental filtering, such as on the Beaufort Sea shelf, generally leads to communities that are more structured by biotic interactions, and possibly representing partitioning of resources among species from intermediate disturbance levels. We provide evidence that environmental filtering can structure functional community composition, providing a baseline of how community function could be affected by stressors such as changes in environmental conditions or increased anthropogenic disturbance.
Introduction
A central question of community ecology is why species from a regional pool form similar or distinct local species compositions (Weiher et al., 1998). In other words, what are the driving forces in the assembly of local biological communities? Three main filters have been proposed for species to pass through in order to be part of a local community based on their functional traits. These include dispersal limitation, biotic interactions, and environmental filters (Keddy, 1992; Pearson et al., 2018). This idea of filtering based on functional traits was first tested with terrestrial vegetation (Weiher et al., 1998; Götzenberger et al., 2012), terrestrial invertebrates (de Bello et al., 2009), and freshwater invertebrates (Conti et al., 2014), but has been used throughout all ecological systems in the framework of community assembly theory (Keddy, 1992; Weiher et al., 2011). This theory assumes species have a suite of measurable functional traits that allow them to persist in a given environment over time (Kraft et al., 2015). Conversely, community assembly theory assumes that a series of hierarchical filters can prevent a prospective species to enter a community if a species’ functional traits would not perform well in the specific local ecosystem conditions (Keddy, 1992; Menegotto et al., 2019). The combined traits of community members should reflect the ability of communities to maintain ecosystem functions within a given environment (McGill et al., 2006; Sutton et al., 2020). If the functional traits represented by a local community do not optimally fill ecological niches and ecosystem functions, missing traits could predict the success of new species invasions (Webb et al., 2010; Pearson et al., 2018).
One of the primary filters of species into a local community is the environment. The relationship between abiotic conditions and functional traits can differ depending on the strength of environmental filtering occurring within a system (Blonder et al., 2015). While the effects of environmental filtering on community assembly can be seen worldwide in many different systems, the Arctic provides an excellent region to closely examine environmental filtering where narrow environmental ranges and highly seasonal conditions affect the species and their traits’ ability to persist (Peck et al., 2004). Most Arctic shelves, including the Alaskan Arctic shelves, are considered benthic-driven systems where tight pelagic-benthic coupling, a result of the timing of sea-ice retreat, supports a high benthic biomass (Grebmeier et al., 2006). These benthic systems, in turn, support many higher trophic level fish (Whitehouse et al., 2017) and marine mammal consumers (Bluhm and Gradinger, 2008). Many Arctic epibenthic invertebrates are long-lived (e.g., Bluhm et al., 1998; Ravelo et al., 2017) and relatively slow moving as adults; they are, thus, mostly confined to local environmental conditions. Consequently, Arctic epibenthic invertebrates must express a range of functional traits that allow them to thrive in those local conditions (Sutton et al., 2020). Environmental variables acting as filters on Arctic benthic community assembly have been well studied from a taxonomic perspective (Bluhm et al., 2009; Blanchard et al., 2013; Ravelo et al., 2014; Grebmeier et al., 2015a) but few studies explicitly looked at these filters from a functional trait perspective (but see Rand et al., 2018; Jørgensen et al., 2019; Liu et al., 2019). Conversely, Arctic benthic taxa can be quite plastic in their environmental tolerance ranges and may be more robust to environmental changes than previously assumed (Renaud et al., 2015, 2019); this could lessen the influence of environmental filters. In light of the strong environmental changes due to climate impacts, specifically in the Arctic, as well as likely increased anthropogenic influence, there is urgency to better understand the relationship between benthic invertebrate functional traits and environmental conditions (Renaud et al., 2019).
We used two Alaskan Arctic shelves as case studies in which to test and compare the effect of environmental filtering on functional community assembly. The Alaskan marine Arctic comprises the Chukchi Sea to the west and the Beaufort Sea to the north (Figure 1), two regions that are geographically close but vary considerably in their environmental influences. The Chukchi Sea is a seasonally ice-covered, broad and shallow inflow shelf (Carmack and Wassmann, 2006). It is influenced by a diverse set of water masses entering through Bering Strait: the fresh and warm Alaska Coastal Current, and the Chukchi Shelf Currents that are cold and nutrient-rich (Danielson et al., 2020). These water masses and the food supply they provide have previously been linked to Chukchi Sea benthic community composition and biomass (Bluhm et al., 2009; Ravelo et al., 2014; Grebmeier et al., 2015b), benthic larval meroplankton distribution (Ershova et al., 2019), and benthic food web structure (Iken et al., 2010). In addition, Chukchi Sea epibenthic assemblages are also correlated with sediment grain size (Bluhm et al., 2009), which in turn is related to current speed of these water masses (Pisareva et al., 2015). Similar to the Chukchi Sea, the Beaufort Sea is also seasonally ice-covered, with much of the narrow shelf covered by landfast ice and pack ice (Mahoney et al., 2014). As an interior Arctic shelf, the Beaufort Sea is exposed to temperature and salinity ranges from downstream influences from the colder and fresher Alaska Coastal Current from the west (Danielson et al., 2020) and from warmer and saline upwelled water from the Beaufort Sea slope (Pickart et al., 2013; Bluhm et al., 2020). In addition, the Beaufort Sea shelf benthos is impacted by riverine input from the Colville and Mackenzie rivers (Weingartner et al., 2017), plus receives additional terrestrial organic material from coastal erosion (Divine et al., 2015; Doxaran et al., 2015). The Beaufort Sea shelf has a distinct depth gradient from on- to offshore, which reflects a gradient for landfast ice, and depth drops rapidly into a steep slope after the shelf break (Bluhm et al., 2020). These Beaufort Sea shelf characteristics have been previously correlated with patterns in taxonomic diversity of epibenthic (Ravelo et al., 2020) and zooplankton communities (Smoot and Hopcroft, 2017), and benthic food web structure (Divine et al., 2015; Bell et al., 2016). Here, we build on previous knowledge of these two shelf systems to investigate if and how environmental variables related to sediment properties (i.e., sediment grain size), food supply (i.e., sediment chlorophyll), and elements of hydrography (i.e., depth, salinity, temperature) affect the filtering of functional traits of epibenthic invertebrates both within each of the two shelves as well as how processes compare between the two shelves.
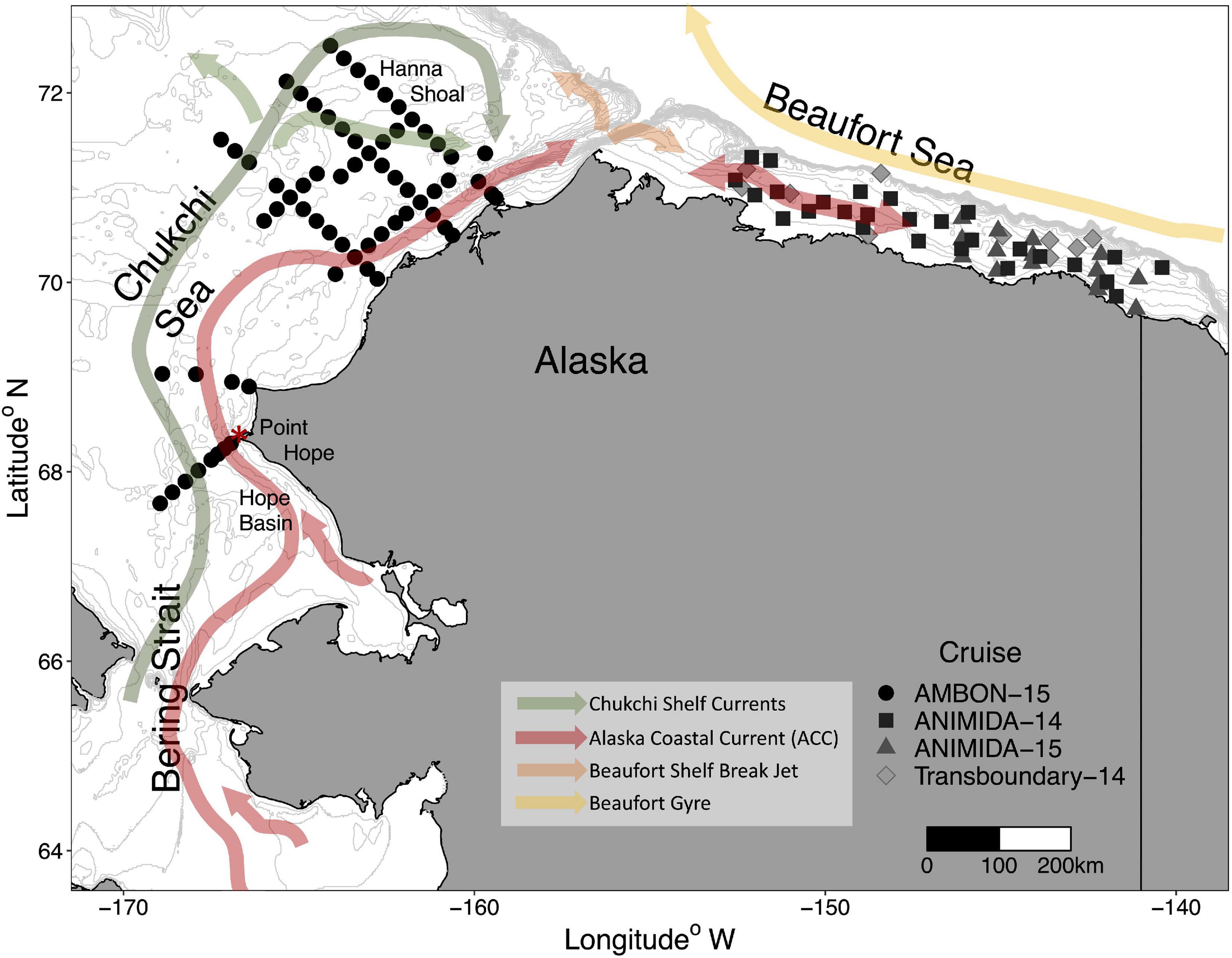
Figure 1. Stations sampled for epibenthic communities during four cruises in the Chukchi and Beaufort seas in 2014 and 2015. Depth contours in the study regions are shown in gray at 10 m intervals. Stations are designated by symbols for different cruises.
If filtering on these two shelves is indeed driven by strong gradients of distinct environmental conditions, we would expect the functional traits of local assemblages to be more similar at the more extreme values of these gradients. This means we expect local assemblages to express high local functional trait convergence at the more extreme values of the existing gradients. Across an environmental gradient, assemblages should exhibit a bell-shaped relationship between functional dissimilarity and environmental gradients (Muscarella and Uriarte, 2016; Denelle et al., 2019; Figure 2). For example, within the realized niche of the physiological range of many benthic invertebrates living in the Arctic, one might expect fewer functional traits to be competitive at either very low or very high temperatures, meaning that the traits of community members would exhibit high trait convergence at these more extreme values of the local environmental spectrum. Measuring trait convergence is a way to aggregate the complexities of different trait responses to environmental filtering into a single value; however, trait convergence can also inform further about the role of biological interactions and niche complementarity in a system after species and their traits have passed through the environmental filter (Pillar et al., 2009). In short, trait convergence not only represents the assembly of traits that were able to overcome the environmental filter, it also informs about the subsequent ecological sorting on the community level (Webb et al., 2010). Strong environmental filtering should provide less hospitable conditions for invading species unless the invaders possess the functional traits that are necessary to survive at the more extreme values of the local environmental gradients (Andersen et al., 2015). Alternatively, in locations where environmental filtering is relaxed, functional divergence within that local assemblage should increase, meaning the species are more dissimilar (more diverse) in their functional trait composition (Spasojevic and Suding, 2012). This weaker environmental filtering leads to increased niche complementarity, where species differ in their realized niches and resource partitioning allows species to coexist (MacArthur and Levins, 1967; Mason et al., 2005; Baltar et al., 2019).
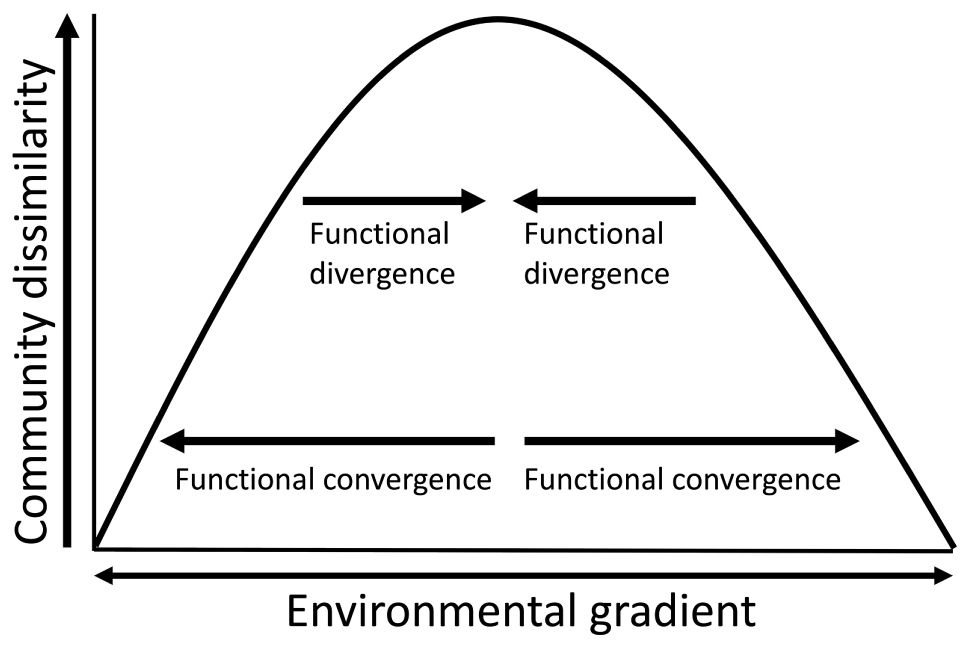
Figure 2. Environmental filtering can be expressed as the relationship between community dissimilarity and environmental gradients. The hypothesized relationship predicts that community dissimilarity decreases toward the more extreme values of an environmental gradient (i.e., strong environmental filtering). Adapted from Muscarella and Uriarte (2016).
The goal of this study was to investigate how environmental filtering influences functional composition and trait convergence within the community assembly framework. We use two geographically close but environmentally dissimilar Arctic epibenthic shelf systems as case studies to address four primary questions: (1) Is there a relationship between epibenthic functional trait composition and environmental gradients? (2) Is the strength of this environmental filtering similar on both the Chukchi and Beaufort Sea shelves? (3) If relationships between functional composition and environmental gradients exist, are they driven by the same trait modalities on the two shelves? (4) Do epibenthic communities follow the expected relationship of higher trait convergence with stronger environmental filtering within each shelf?
Materials and Methods
Study Sites and Sample Collection
Epibenthic taxa used in this study were collected from the Chukchi and Beaufort Sea shelves from research cruises in 2014 to 2015 (Figure 1). Stations in the Chukchi Sea were sampled as part of the Arctic Marine Biodiversity Observing Network survey in 2015 (AMBON, 2015). Stations in the Beaufort Sea were sampled as part of the US-Canada Transboundary Project 2014 and the Arctic Nearshore Impact Monitoring in Development Area project III (ANIMIDA, 2014, 2015). Bottom depth ranged from 11 to 54 m for the 67 stations in the Chukchi Sea and 10 to 64 m for the 46 stations in the Beaufort Sea. Epibenthic invertebrates were collected with a plumb-staff beam trawl with a mouth opening of 2.6 m wide and 1.2 m high as well as a 7 mm mesh and 4 mm codend liner (modified after Gunderson and Ellis, 1986). Environmental variables previously shown to influence epibenthic taxonomic composition (Bluhm et al., 2009; Blanchard et al., 2013; Ravelo et al., 2014, 2015) were collected at each station to reflect hydrography (depth, bottom water salinity, bottom water temperature; all from CTD profiles taken with a Seabird Model SBE911), sediment properties (percent gravel, sand, and mud), and food supply (sediment chlorophyll-a). Sediment properties and food supply were both sampled from top 1 cm sediments from van Veen grab samples, following analyses described in Grebmeier et al. (1989) and Cooper et al. (2012), respectively (also see Iken et al., 2019). Environmental variables for each shelf were standardized to a mean of zero and a variance of one, and a matrix of pairwise Euclidean distances between stations was created. Environmental variables were evaluated for collinearity via Pearson correlations. When collinearity existed [absolute value (Pearson) >0.70 see Supplementary Figure 1 for correlations of environmental variables] we chose one of the environmental variables. For example, percent sand was removed from the Chukchi Sea and Beaufort Sea models prior to analysis due high collinearity between percent sand and percent mud. Variability of environmental variables between the Chukchi and the Beaufort Sea was compared using a Levene’s test. All environmental data are available through the MBON Data Portal1, the Alaska Ocean Observing System (AOOS) Data Portal2, and the National Centers for Environmental Information (Kasper et al., 2017)3.
Epibenthic Functional Composition
Epibenthic invertebrate functional composition was expressed through a combination of biological traits that describe the taxa’s life history, morphology, and behavior, following commonly accepted definitions and categories (Degen and Faulwetter, 2019). Nine biological traits for a total of 327 taxa were assessed, with almost identical taxon richness in the Chukchi and Beaufort seas (n = 246, n = 245, respectively) at the same levels of taxonomic identification (Sutton et al., 2020). Each trait was categorized by modalities, which are specific categories within each trait (see Table 1). Modalities within each trait were assigned to each taxon using a fuzzy coding approach, where a minimum value of 0 indicated no affiliation with that modality and a maximum value of 3 indicated a strong affiliation of a taxon with a modality (Chevenet et al., 1994; Sutton et al., 2020). This process created unique biological trait profiles for all taxa, which were standardized between 0 and 1 to create a trait by taxon matrix (Q matrix). Trait information for all epibenthic taxa in this study can be accessed in Supplementary Material for this manuscript or via The Arctic Traits Database4 for more detailed descriptions.
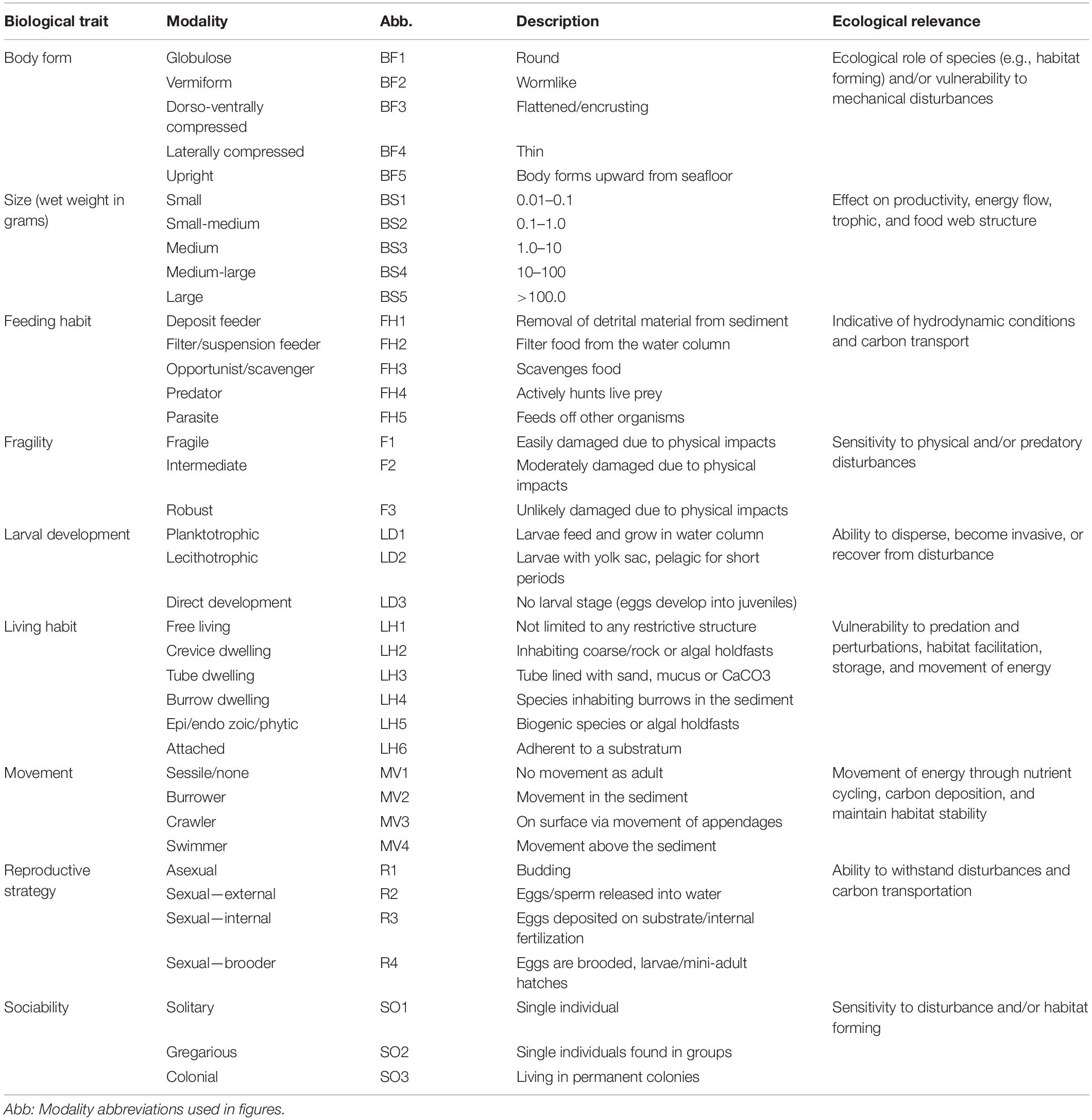
Table 1. Biological traits, modalities, descriptions, and ecological relevance are shown for epibenthic invertebrates collected in the Chukchi and Beaufort seas, following Degen and Faulwetter (2019).
Relationships Between Functional Composition and Environmental Variables
The relationship between functional composition and environmental variables, expressed as covariance at each station, was tested with combinations of RLQ and fourth corner analyses (Dolédec et al., 1996; Legendre et al., 1997; Dray et al., 2014). RLQ is an ordination-based multivariate technique that relates a sites by environmental matrix (R-matrix) to a Q-matrix, which are linked by a biomass-weighted species by site matrix (L-matrix; Dolédec and Chessel, 1994). It provides ordination scores that describe the relationship of linear combinations of trait modalities to environmental variables that are most covariant. A global significance value was obtained through Monte-Carlo tests via 4,999 permutations of the L matrix rows (stations; model 2) and L matrix columns (taxa; model 4) for each shelf and was used to assess if there was a significant relationship between functional composition and environmental conditions (Dray et al., 2014). Prior to the RLQ analysis for each shelf, separate correspondence analyses were performed for the fourth-root transformed L-matrix, and separate principal component analyses were performed for the standardized R-matrix and Q-matrix. The summary RLQ table, thus, compares the RLQ output to these separate ordinations to compare how much variance was preserved for each part of the RLQ.
Fourth corner analysis investigates the bivariate relationships of trait modalities to each environmental variable. When RLQ and fourth corner analyses are used in tandem, two associations are investigated: the first association investigates the significance of trait composition (individual modalities) to environmental gradients. The second association investigates the significance of environmental variables to trait composition (combination of modalities) (Dray and Legendre, 2008). To account for multiple testing, a false discovery rate correction was implemented for all RLQ and fourth corner analyses. We set a significance alpha value at 0.05 for all RLQ and fourth corner analyses to reduce our rate of type 1error.
Significant modalities with the highest relative Pearson correlation values for each shelf with environmental axes from the RLQ-fourth corner were selected to further investigate spatial relationships in each shelf. Cut-off Pearson correlation values were chosen for modalities with absolute Pearson values >0.15 in the Chukchi Sea and modalities with absolute Pearson values > 0.10 in the Beaufort Sea, based on the overall range of Pearson correlation values for each shelf.
Trait Convergence
Trait convergence was evaluated using the measure of functional dispersion (FDis), where a lower dispersion value indicates more similar functional composition and, therefore, higher trait convergence of taxa at a station. To calculate FDis, the Q matrix was multiplied with a fourth root transformed, biomass-weighted L matrix to create a trait by station matrix (LQ matrix). The LQ matrix represented the functional composition at a station as community-weighted-means (CWM) (Lavorel et al., 2008; Sutton et al., 2020). Next, trait space for each station was calculated using a principal coordinate analysis (PCoA), where PCoA axes were used as the derived “traits” quantified from the CWM matrix (Laliberté and Legendre, 2010). FDis was then calculated as the mean distance of all taxa at a station to the biomass-weighted centroid of the stations in trait space (Anderson et al., 2006; Laliberté and Legendre, 2010). Average FDis was compared between the Chukchi and Beaufort epibenthic shelf communities using a generalized least squares (GLS) linear model while accounting for spatial autocorrelation:
where y is FDis, the intercept αk denotes the mean value of y for the Beaufort Sea (k = 1) and Chukchi Sea (k = 2). The error, ε, was modeled as a spatial random process with a correlation structure that declined exponentially with distance between stations to account for spatial autocorrelation. Models were fit using a generalized least squares approach (Pinheiro et al., 2020) when autocorrelation significantly improved the model; otherwise, ordinary least squares were used.
Trait Convergence and Environmental Gradients
The relationship between functional trait convergence and environmental gradients was tested by comparing the axes from CWM redundancy analyses (CWM-RDA, Nygaard and Ejrnaes, 2004) to our trait convergence metric (FDis) for each shelf. The reason for using CWM-RDA over the RLQ and fourth corner analyses to evaluate the relationship of functional dispersion to environmental gradients is that spatial coordinates could be included in the CWM-RDA model as a condition matrix of station latitude and longitude. Predictor variables in the CWM-RDA were represented by environmental variables that were retained after testing for collinearity (see above). We used the CWM-RDA axes that accounted for the majority of variance from the Chukchi Sea and Beaufort Sea, respectively, as proxies for overall environmental gradients (Lavorel et al., 2008). The relationships of FDis to these overall environmental gradients (i.e., CWM-RDA axes) were tested using a GLS model of FDis as a linear or quadratic function of the first two axes from the CWM-RDA, with autocorrelated residuals as in Equation (1). We chose quadratic relationships due to the expected bell-shaped relationship for environmental filtering (see Figure 2). The best model was represented by the lowest corrected AIC (AICc) that accounts for small sample size. In the Beaufort Sea, latitude and longitude were collinear with depth, so depth was removed from the CWM-RDA in the Beaufort Sea. Model outputs were considered significant at alpha = 0.05 for the relationship between RDA axes and environmental variables. All analyses were performed in R version 4.0.2 (R Core Team, 2020) using the ade4 (Dray and Dufour, 2007), vegan (Oksanen et al., 2020), FD (Laliberté and Legendre, 2010), and nlme (Pinheiro et al., 2020) packages.
Results
Functional Composition and Relationship to Environmental Conditions
Individual modalities had much closer relationships in pairwise comparisons with environmental variables in the Chukchi Sea compared to the Beaufort Sea where only two significant bivariate modality-environment relationships occurred (fourth-corner analysis, Figure 3). Temperature and mud in the Chukchi Sea had the most significant relationships with modalities, either positively or negatively (Figure 3A). Temperature had many positive relationships and only a few negative relationships with the modalities small/medium body size (BS2), fragile (F1), medium fragility (F2), and free-living (LH1). Percent mud was mostly negatively correlated with modalities except for the modalities free-living (LH1), crawler (MV3), and sexual-internal (R3). In the Beaufort Sea, depth was the only environmental variable with any significant relationship to modalities with two negative associations with the modalities medium/large (BS4) and lecithotrophic (LD2) (Figure 3B). These relationships were well expressed when RLQ and fourth-corner analyses were combined (Table 2).
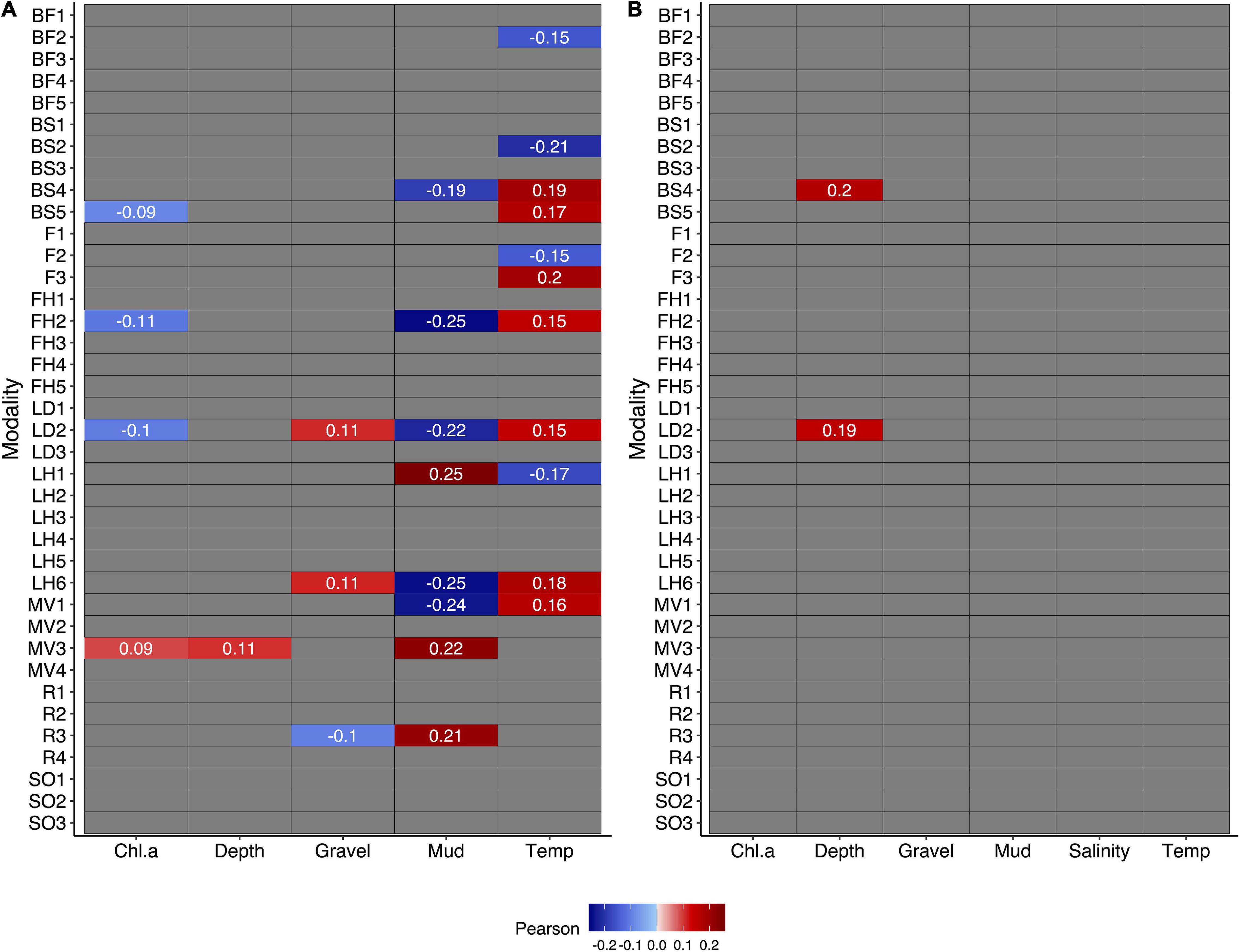
Figure 3. Fourth corner tests show significant bivariate relationships between modalities and environmental variables for the Chukchi Sea (A) and the Beaufort Sea (B). Significant positive relationships are represented by red colors and significant negative relationships are represented by blue colors. Gray colors indicate no significant relationships. Pearson correlation values are included for significant bivariate relationships with darker colors indicating stronger relationships.
When modalities were compared to environmental gradients along the RLQ axes, as opposed to individual environmental variables, the relationship between functional composition of epibenthic communities was globally significant for the Chukchi Sea (p < 0.001, inertia = 1.486) and for the Beaufort Sea (p = 0.007, inertia = 0.484) (Table 2). The RLQ analysis in the Chukchi Sea took a higher proportion of the variability for the three R, L, and Q matrices into account, indicating higher explanatory power compared to the Beaufort Sea (Table 2 and Supplementary Figure 2). The first RLQ axis of the Chukchi Sea represented the vast majority of the total cross-variance between traits and the environmental gradients with 91%, while only 5% was represented by the second axis (Figure 4A). The first RLQ axis in the Chukchi Sea represented a gradient of muddier and high chlorophyll-a content environmental conditions associated with negative R-loadings to more gravelly, and warmer conditions associated with positive R-loadings (RLQ analysis; Figure 4A). The first and second RLQ axes of the Beaufort Sea represented 82% and 9% of the of the total cross-variance between traits and the environmental gradients (Figure 4B). Based on the R-loadings, the first RLQ axis in the Beaufort Sea represented a gradient of shallow and fresher conditions associated with negative R-loadings to more saline and deeper conditions associated with positive R-loadings (RLQ analysis; Figure 4B). Environmental variable R-loadings of the RLQ analysis mirrored the significant associations between environmental variables and RLQ trait axes in both seas (RLQ and fourth corner analysis; p < 0.05; Figures 4A,B).
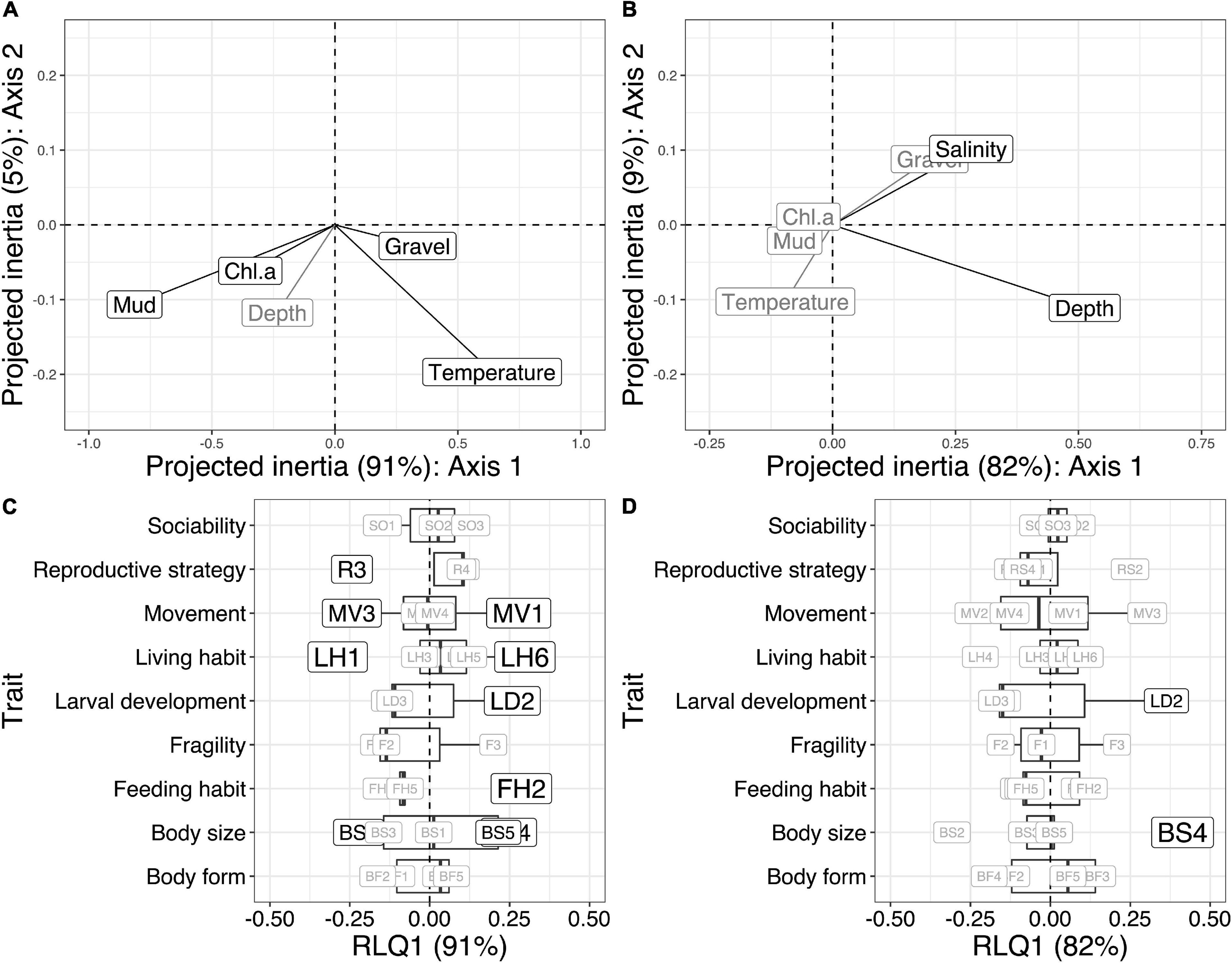
Figure 4. The covariation of the first two RLQ axes of the environmental variables are shown for the Chukchi Sea (A) and Beaufort Sea (B). Significant relationships of environmental variables to RLQ trait axes (p < 0.05) are shown in black while environmental variables with non-significant relationships to RLQ trait axes are shown in gray for the Chukchi Sea (A) and Beaufort Sea (B). Significant relationships of modalities (p < 0.05) to the first RLQ environmental axes are shown for the Chukchi and Beaufort Sea (C,D). Size of the modality boxes correspond to the strength of the Pearson correlation values. Modality abbreviations are shown in Table 1 and RLQ outputs are shown in Table 2.
Fewer associations were present for the Chukchi Sea between modalities and environmental gradients (RLQ-axes) compared to bivariate associations of modalities and environmental variables (Figure 3) while the same associations were present in the Beaufort Sea (Figures 4C,D). The Chukchi Sea modalities with the strongest relationships with the first RLQ environmental axis included those from behavioral traits (feeding habit, living habit, movement) and body size (Figures 4A,C). Weaker relationships occurred with life history traits (larval development and reproductive strategy). Specifically, proportions of the modalities small/medium (BS2), free-living (LH1), crawler (MV3), and sexual-internal (R3) were negatively associated with RLQ environmental axis 1, representing increased proportions of these modalities with greater percent mud and chlorophyll-a and decreased proportions with temperature. Likewise, the proportion of the modalities medium/large (BS4), large (BS5), filter-feeding (FH2), lecithotrophic (LD2), attached (LH6), and sessile (MV1) were positively related to RLQ environmental axis 1, decreasing with percent mud and chlorophyll-a and increasing with warmer temperatures (Figures 4A,C). These associations well matched the spatial distribution of these environmental variables on the shelf, mostly in an on- to offshore pattern (Figures 5A, 6A). Similar to the bivariate associations between modalities and environmental variables, the modalities medium/large (BS4) and lecithotrophic (LD2) had positive associations with the first RLQ environmental axis in the Beaufort Sea (Figure 4D). The proportion of these modalities expressed at stations subsequently followed the depth and salinity gradients along the Beaufort Sea shelf (Figures 5B, 6A). All modality heatmaps can be found in Supplementary Figure 3.
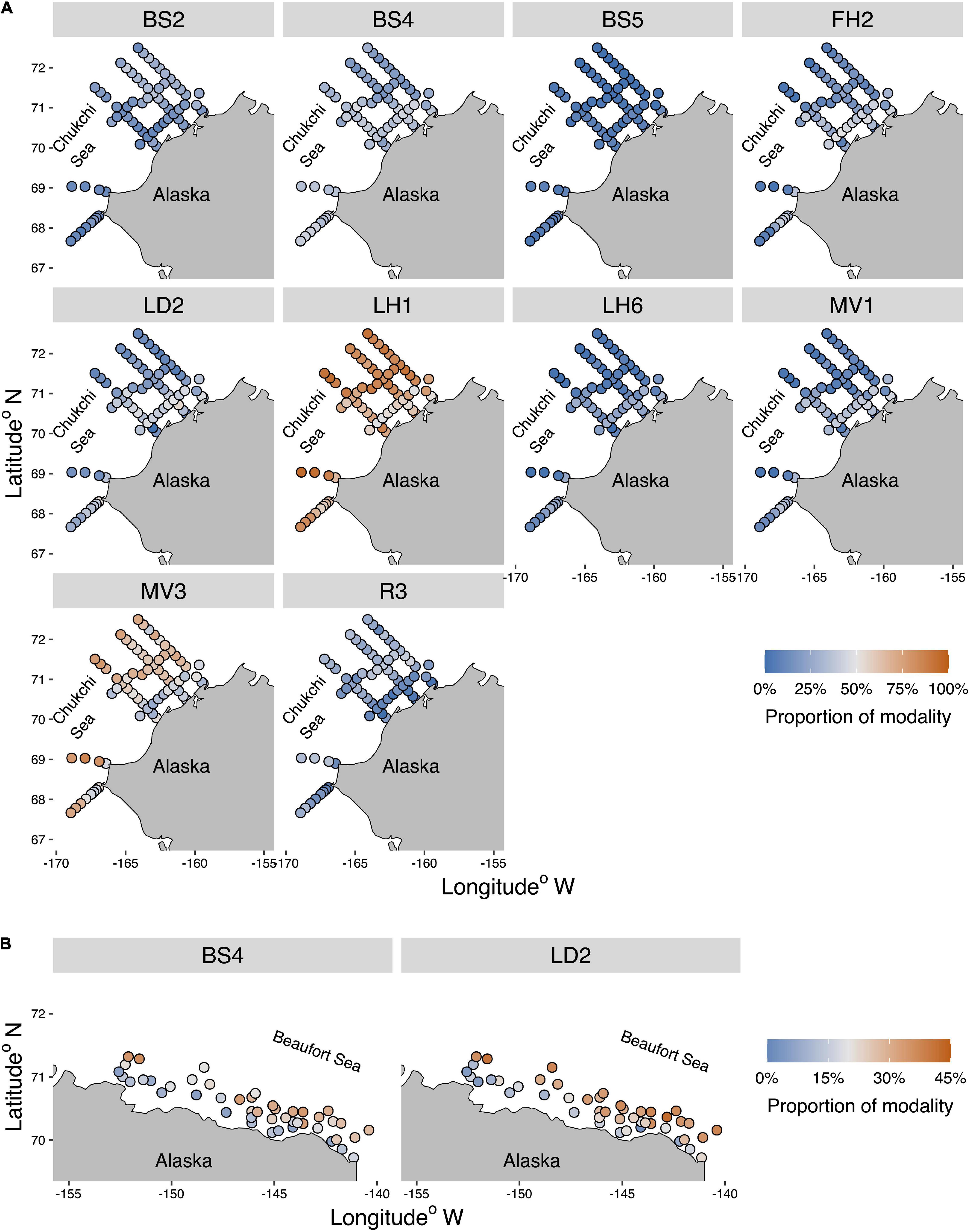
Figure 5. Spatial representation of the significant modalities with absolute Pearson correlations > 0.15 for the first axis of the RLQ plot in the Chukchi Sea (A) and modalities with absolute Pearson correlations > 0.1 for the first axis of the RLQ plot in the Beaufort Sea (B) (see Figure 4). Pearson correlation cutoff levels were chosen based on the highest relative correlations on the first axis for the RLQ among all traits for each shelf. The full suite of spatial representations for all modalities in both seas is shown in Supplementary Figure 3.
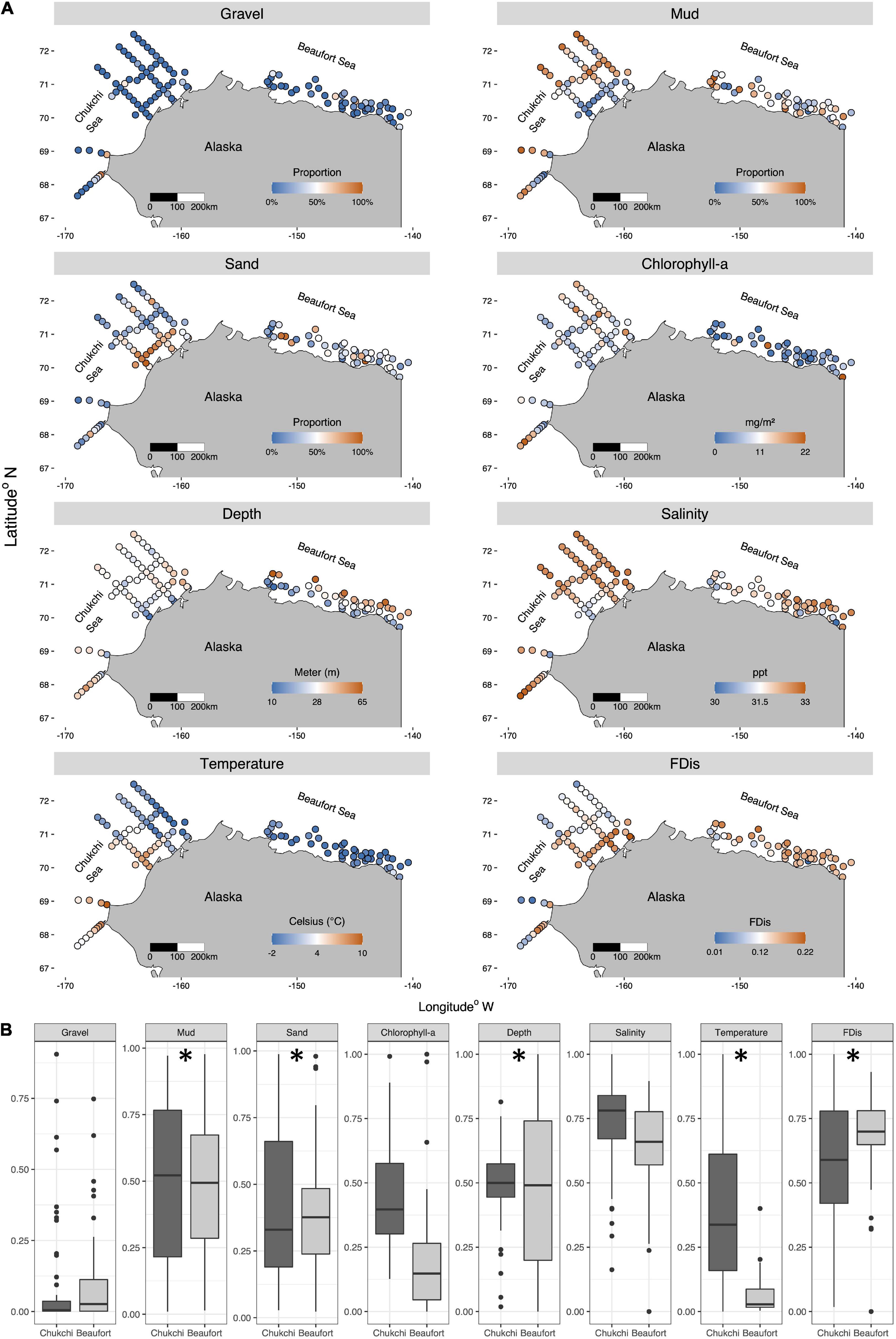
Figure 6. Spatial representation of the Chukchi and Beaufort Sea environmental variables and of functional dispersion (FDis) (A). Variance of environmental variables and FDis were compared between the Chukchi Sea (dark gray) and the Beaufort Sea (light gray) (B). Horizontal lines: median, boxes: inter-quartile range (IQR); whiskers: highest/lowest values ≤ 1.5x IQR above/below box; black points: outliers; *: significance (p < 0.05) of variance of environmental variables and FDis using Levene’s Tests.
Relationship Between Environmental Gradients and Trait Convergence
FDis in the Chukchi Sea decreased along an on- to offshore gradient, largely following a similar trajectory of temperature and opposite the trajectory of percent mud (Figure 6A). This represented higher trait convergence in the offshore and northern study region and higher trait divergence in onshore regions. Greater trait convergence on the Beaufort Sea shelf was found in the shallow western, nearshore stations, characterized by lower salinities, while trait divergence was greater at the deeper offshore and eastern sites (Figure 6A). Variability in both the environmental variables and in FDis differed between the Chukchi and Beaufort seas (Levene’s test; p < 0.05; Supplementary Table 1). The Chukchi Sea had larger variability in percent sand, percent mud, temperature, and functional dispersion while the variability in depth was larger in the Beaufort Sea (Figure 6B). Mean FDis was not significantly different between the two seas (p = 0.284, adj. R2 = 0.009; Figure 6B) however, the much larger variability in FDis in the Chukchi Sea indicated that stations there covered a greater range of trait convergence. This reflected a range of some stations with very similar functional composition to some stations with very dissimilar functional composition in the Chukchi Sea. In contrast, Beaufort Sea stations exhibited more consistent levels of higher trait divergence.
The relationship between FDis and environmental variables were assessed with CWM-RDA ordinations between functional composition and environmental variables after removing collinear associations (Supplementary Figure 1). The environmental matrix for the analysis included latitude and longitude to account for spatial autocorrelation. The overall CWM-RDA model was significant in the Chukchi Sea and mud, depth and temperature were significant terms in the model. RDA1 represented a gradient of warmer and larger sediment grain size associated with negative RDA1 values to cooler and muddier conditions with positive RDA1 values. The second RDA axis represented a gradient of deeper, muddier conditions associated with negative axis values to shallower and larger sediment grain size conditions associated with more positive values (Table 3 and Figure 7A). In the Beaufort Sea, two environmental variables, depth and salinity, were removed from the CWM-RDA due to their disproportionately strong influence on the model variance. The overall CWM-RDA was not significant in the Beaufort Sea and no environmental variables were significant in the model (Figure 7B). This was likely due to the high percentage of the variance explained by the conditioned (spatial associations) and unconstrained matrices (35 and 56%, respectively) compared to the variance that the constrained matrix explained (9%).
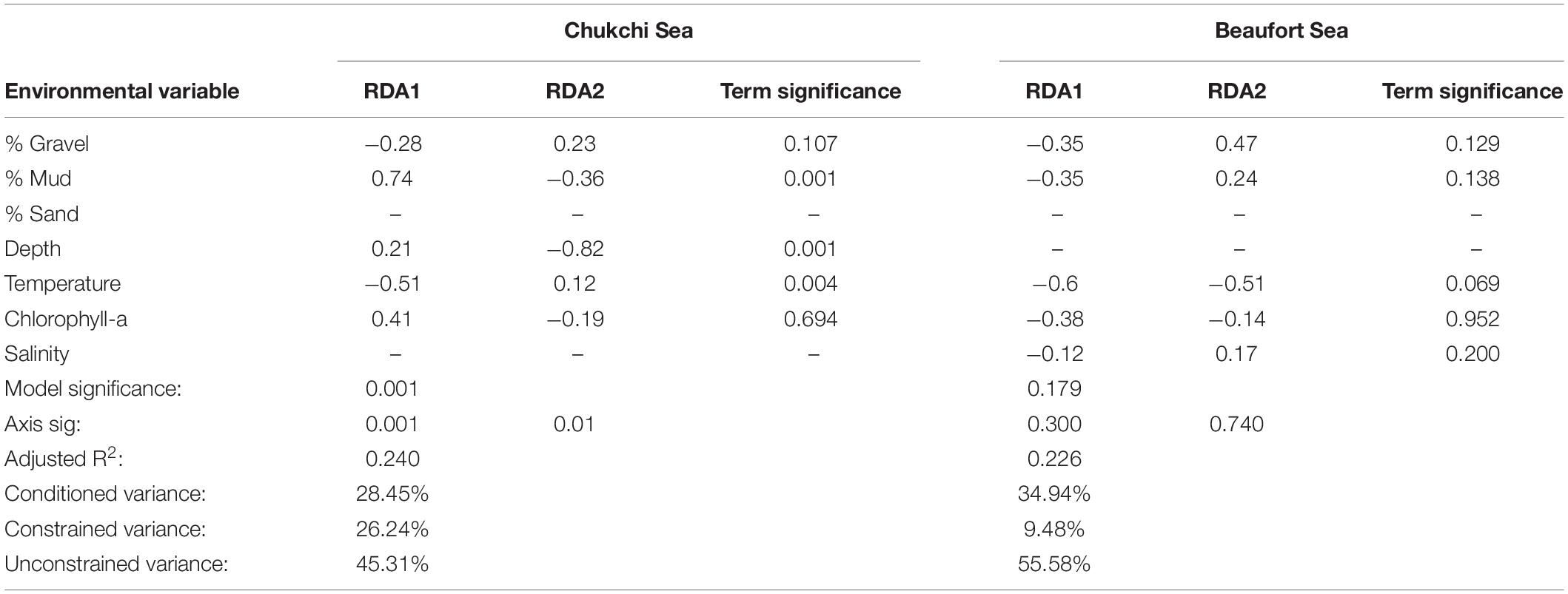
Table 3. CWM-RDA outputs are shown as the loadings of environmental variables for the first two axes.
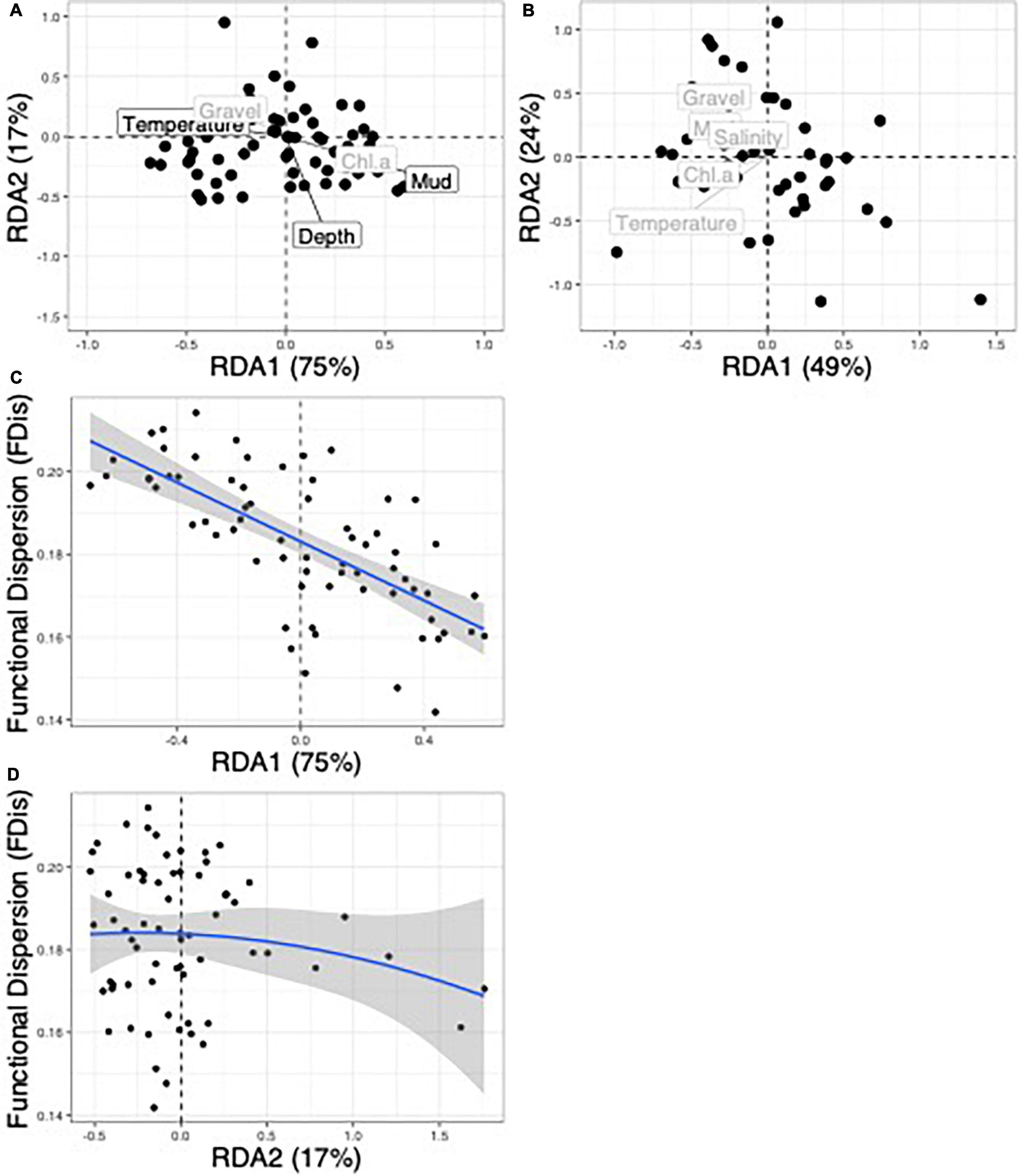
Figure 7. Community-weighted-mean redundancy analysis (CWM-RDA) of functional traits and environmental variables in the Chukchi Sea (A) and the Beaufort Sea (B). Length of vectors represent the loadings of environmental variables. The relationship of functional dispersion (FDis) with environmental gradients via a generalized-least-squares polynomial linear model is shown for the Chukchi Sea for the first CWM-RDA axis (from left to right along the RDA axis) representing high percent mud to high temperature (C) and for the second axis (from bottom to top) representing a decreasing depth gradient (D).
The relationship of FDis to significant environmental gradients expressed in CWM-RDA axes in the Chukchi Sea did not follow the expected bell-shaped relationship with the first axis and only very weakly with the second axis (Figures 7A,C,D). The best AIC selection model in the Chukchi Sea for FDis included both CWM-RDA axes (Figures 7C,D) and implied a negative (linear) relationship of FDis with the first axis (dominated by a gradient of warmer, low percent mud to cooler, muddier conditions) and a weak negative (quadratic) relationship with the second axis (representative of a gradient of deeper, muddier conditions to shallower, low percent mud conditions). In other words, functional trait convergence was greatest (low FDis) at high percent mud and smallest (high FDis) at warmer temperatures.
Discussion
This study explored whether and how the concepts of environmental filtering in community assembly theory applied to the functional composition of epibenthic communities using the Arctic Chukchi and Beaufort Sea epibenthic shelf communities as case studies. The Chukchi Sea, for the most part, had larger environmental gradients, and exhibited higher trait convergence in relation to environmental gradients compared to the Beaufort Sea. This provided evidence for the community assembly concept that aligns more extreme ends of an environmental gradient with fewer functional traits (higher trait convergence) (Figure 2) for the Chukchi Sea, although only part of this relationship was represented.
Drivers of Functional Composition and Environmental Variables
Environmental filtering, exhibited through the relationships of functional composition and environmental conditions, was evident on the Chukchi Sea but less so for the Beaufort Sea shelf. Functional composition on the Chukchi Sea shelf, namely several behavioral traits (i.e., feeding habit, living habit, movement) and body size, were correlated to some water mass characteristics (temperature) and sediment grain size, specifically percentage of mud. Sediment grain size is globally an important driver of soft-bottom benthic community composition and diversity (Snelgrove et al., 2001; Thrush et al., 2004) as well as on the Chukchi Sea shelf (Feder et al., 1994; Bluhm et al., 2009; Iken et al., 2019). Our results expand our understanding of this relationship beyond taxonomic composition to epibenthic functional traits in the study regions. Similarly, variation in sediment grain size has been linked to behavioral traits such as mobility and feeding habit in the Canadian Arctic (Krumhansl et al., 2016), as well as larval settlement selection along the eastern seaboard of the United States (Snelgrove et al., 1999). Sediment grain size can be a proxy for water flow intensity and deposition (Snelgrove and Butman, 1994). Patterns of distribution of finer sediments on the Chukchi Sea shelf are the result of variation in current velocities that carry finer sediment particles downstream of the Bering Strait, then deposit them in regions of reduced water flow such as in Hope Basin in the south-central Chukchi Sea and the northern and offshore regions of the shelf (Darby et al., 2009; Grebmeier et al., 2015b; Pisareva et al., 2015). Temperature and salinity are generally representative of dominant water masses; in the Chukchi Sea, these are most notably the mid-shelf cold, saline, and high-nutrient Chukchi Shelf Currents, while the Alaska Coastal Current (ACC) is warm, fresh, and low in nutrients (Danielson et al., 2020; Figure 1).
Areas with a high mud content on the Chukchi shelf were positively associated with small/medium body size, crawling movements, free-living habits, and sexual-internal reproductive strategy. These results are similar to the relationships of benthic traits and sediment grain size in the Canadian Arctic (Krumhansl et al., 2016) and seminal work on the US East Coast on the interaction between feeding habits and movement types with sediment grain size (Rhoads and Young, 1970). In the south-central Chukchi Sea, Hope Basin (Figure 1) is known for the especially high deposition rates of small particle sizes when water slows after transitioning the narrow Bering Strait. This deposition supplies ample food for deposit feeding clams, which in turn act as prey for many epibenthic invertebrates or marine mammals (Grebmeier et al., 2015a,b). The middle shelf in the northern Chukchi Sea was also particularly characterized by high percent mud in our study. This may be the result of converging water masses around Hanna Shoal, which leads to high deposition rates and reduced current flow (Blanchard et al., 2013; Weingartner et al., 2013), resulting in a shallow biological hotspot known to support many higher trophic levels (Young et al., 2017). The invertebrate movement type crawler and free-living habit that were most common in these areas are indicative of taxa that can easily move on fine-grained sediments, often pursuing predatory feeding habits (Grebmeier et al., 2006). These behavioral traits provide important ecosystem functions in muddy areas because crawling movement types and free-living habits foster bioturbation of the sediment, increasing oxygenation of the sediments and the resuspension of organic material (Snelgrove et al., 2000; Queiros et al., 2013). This especially benefits the lower trophic level food web. This effect can be seen in the fact that some of these areas coincide with “hotspots” in macrobenthic biomass that benefit from the deposition of fine-particle organic matter (Grebmeier et al., 2015b) but also high biomass of highly mobile epibethic invertebrates (Bluhm et al., 2009; Iken et al., 2019). At first glance, the strong association between sexual-internal reproduction (i.e., eggs deposited on the substrate and/or internal fertilization) is somewhat surprising given that egg laying tends to be more common on hard substrates. However, regions of high percent mud in the Chukchi Sea also have high instances of gastropods, many of which can lay their egg masses on other gastropods or shells or as large, protected masses on top of the sediment (e.g., Naticidae, Buccinidae).
Lecithotrophic, larger, filter feeding, sessile, and attached living habit had a strong negative relationship with mud in the Chukchi Sea. Filter-feeding taxa are generally limited by such finer sediments as they tend to clog filter-feeding appendages (Rhoads and Young, 1970). This process forces filter feeders to allocate energy to clearing feeding apparatuses instead of growth and reproduction (Ellis et al., 2002). Many epifaunal filter-feeding taxa are sessile and, thus, require hard substrate for attachment (e.g., Almond et al., 2021), which would create better habitat suitability for this feeding type in the more coastal Chukchi Sea waters, where faster water flow creates coarser sediments (Pisareva et al., 2015). This may also explain the positive relationship of robust, filter-feeding, lecithotrophic taxa with increasing temperature, as the coastal Chukchi waters are also characterized by higher temperatures (Weingartner et al., 2005; Danielson et al., 2020), rather than a causal relationship between filter feeding with temperature per se. Although lecithotrophic larval development has previously been documented to follow warmer temperatures along the southern California and Florida coast (Goddard, 2004), the negative relationship to chlorophyll-a content is likely the result of taxa that can survive in areas of low food availability for their planktonic stage. Larger, more robust taxa in coastal Chukchi Sea regions may be more likely to withstand an increased risk of disturbance along the dynamic coastal environment.
The relationships of functional composition to environmental gradients were much weaker in the Beaufort Sea compared to the Chukchi Sea with few significant trait-environmental relationships driven mostly by depth and to a lesser degree, salinity. Depth has been identified as a driving force of functional traits in inflow Arctic shelves such as the Bering Sea (Liu et al., 2019) and the Barents Sea (Cochrane et al., 2012) and has long been suggested as a general key environmental variable to track functional traits (Costello et al., 2015). Depth increases unidirectionally with distance from shore across the narrow Beaufort Sea shelf; it often is considered a proxy for many environmental drivers (Jakobsson et al., 2012) such as salinity, temperature, and, in polar seas, influences of ice cover and scour. For example, in the shallower coastal Beaufort Sea, environmental conditions are especially related to the degree and extent of nearshore ice scouring (Mahoney et al., 2014), input of sediments and terrestrial matter from coastal erosion (Doxaran et al., 2015), and riverine influence from major rivers such as the Mackenzie and Colville rivers (Rachold et al., 2005). This highly dynamic coastal environment might select against modalities such as lecithotrophic which was associated with deeper conditions. In deeper Beaufort shelf regions close to the shelf edge, physical disturbance may be less common but there is increased influx of nutrients and warmer water from upwelling from the deep slope that add environmental variability (Pickart et al., 2013). This allows for taxa to exploit more diverse resources and likely leads to the presence of taxa that have predominantly a larger body size, and pelagic larvae such as ophiuroids, holothurians, or gastropods that thrive in more established soft-bottom Artic communities (Ravelo et al., 2020).
The Influence of Environmental Filters on Trait Convergence
We assumed that optimal suites of functional modalities of species should be more similar (higher trait convergence) at the more extreme local values of an environmental gradient and should be dissimilar (higher trait divergence) at more moderate levels of a local environmental gradient (Figure 2). Overall, we saw that environmental drivers in the Chukchi Sea with greater variability, i.e., covering a greater range of an environmental gradient, had a greater correlation with functional composition and trait convergence. Specifically, we found higher trait convergence toward the more extreme values of a gradient of percent mud and water mass characteristics. However, we did not see the expected relationship of higher trait divergence at more intermediate levels of percent mud and temperature. This may be a result of using ordinated environmental variables as the gradient, where the influence of one variable becoming more extreme may be offset by the effect of other variables. In the natural system, taxa with their functional traits are not exposed to individual environmental conditions but to this combination of conditions (Everatt et al., 2015). This suggests that while environmental filters exist and may affect individual trait modalities strongly, the responses of other modalities in this complex environment may continue to result in high trait dispersion.
If we postulate that multiple environmental variables can offset their effects on FDis, why did we then see high trait convergence (low Fdis) at one end of the combined environmental gradient but not the other? We suggest that the specific environmental variables at play (grain size, temperature, and depth in the Chukchi Sea) only caused a sufficient filter at one end of the realized gradient, i.e., the actual conditions experienced on the shelf. For example, polar taxa in general are known to be highly adapted in their physiological tolerance to low temperatures (Peck et al., 2004). The higher temperatures experienced in the more coastal Chukchi waters may, therefore, present an effective environmental filter at the edge of the tolerance window, but the colder temperatures on the offshore shelf may not have been functionally limiting.
The relationship of the environmental gradients with trait convergence was not significant in the Beaufort Sea. Likely, the removal of the variable depth was a cause for non-significant relationships, which was the strongest variable in the RLQ analysis with traits. Since depth was highly correlated with latitude and longitude, it was removed to account for spatial autocorrelation. Depth across the Beaufort Sea shelf increases steadily to 100 m at the shelfbreak (Jakobsson et al., 2012) and depth-related patterns across the entire shelf depth range have been found in epibenthic taxonomic diversity (Ravelo et al., 2020). Given that functional composition patterns generally match those of taxonomic composition (Sutton et al., 2020), it is possible that changes in trait convergence may occur if deeper Beaufort Sea shelf regions would be included.
The lack of apparent environmental filtering on the Beaufort Sea shelf could also represent a system where biotic interactions act as a second filter that may shape community function more prominently rather than the environment. This interpretation matches well with our previous findings that epibenthic functional composition on the Beaufort shelf had higher functional dissimilarity and evenness than that in the Chukchi Sea (Sutton et al., 2020). This more balanced interplay of environmental and biological filters (Weiher et al., 2011) that we propose for the Beaufort Sea may promote higher trait divergence and the coexistence of many functional roles (Cornwell et al., 2006). The environmental conditions in the Beaufort Sea may be more representative of disturbances, such as seasonal ice-scouring and spring freshet, rather than continuous environmental gradients that would exert sufficient sustained (e.g., year-round) pressure to drive trait convergence. Intermediate disturbance regimes promote functional richness (Grime, 2006; Cadotte et al., 2011), where more diverse functional traits are used to exploit more diverse resources, a process known as disturbance-induced niche complementarity (Roxburgh et al., 2004). This niche complementarity resulting from intermediate disturbance is different from strong environmental filtering, where the latter would be limiting the functions that are able to thrive at the more extreme values of a local gradient, ultimately limiting niche complementarity (Valdivia et al., 2017).
Conclusion
The influence of environmental filtering on the functional community assembly was much more evident for the Chukchi Sea, although we did not see the expected bell-curve relationship of functional trait convergence in response to environmental gradients. In the Chukchi Sea as the system with stronger environmental filters, behavioral traits (i.e., feeding habit, living habit, movement) had the strongest associations to environmental gradients. These traits may, therefore, be good indicators of environmental filtering in regions that are experiencing strong changes of environmental conditions due to climate change such as the Arctic. In the Chukchi Sea, these changes include an increasing influx of warmer water from the North Pacific (Woodgate, 2018), conditions that may negatively affect smaller-sized taxa with characteristics of crawling movement types, sexual-internal reproduction, and free-living habits. In addition to changes in hydrographic conditions, increased current speed through Bering Strait (Woodgate, 2018) could lead to changes in sediment grain size on the southern Chukchi Sea shelf (Abe et al., 2019). While these environmental changes may challenge the fitness of some traits currently abundant in this Arctic shelf system, they likely contribute to a northward shift of boreal benthic taxa into the Arctic (Mueter and Litzow, 2008; Grebmeier et al., 2015b; Renaud et al., 2015). This study suggests that these northward-moving benthic taxa will have a competitive advantage over current Chukchi Sea shelf inhabitants if they possess favorable behavioral and life history functional traits that can pass through the local environmental filters. It is unknown if expected environmental changes on the Beaufort Sea shelf may affect environmental filters in that system. It is expected that warmer temperatures will lead to less seasonal sea ice and, therefore, less ice-scour on the shallow shelf (Wadhams, 2012), along with increased wave action along the coast (Pickart et al., 2009). This will also lead to more disturbances from increased terrestrial input from coastal erosion (Rachold et al., 2000; Doxaran et al., 2015) and increased sedimentation from river outflow (Weingartner et al., 2017; Bonsell and Dunton, 2018). It remains to be seen if these changing conditions would strengthen or further weaken environmental filters, or if they would remain part of a changing disturbance regime in which biological interactions play a greater role in structuring epibenthic functional composition.
One caveat to consider in our study, which is typical for most polar field studies, is the fact that the environmental measurements used were all taken during a relatively short sampling period. In our case that was during summer when these Arctic shelf systems are ice-free and accessible. The local range for some dynamic variables, such as temperature and salinity, will be much larger than used here if year-round ranges would be considered (Danielson et al., 2017; Hauri et al., 2018). Hence, the mostly long-lived Arctic invertebrates are exposed to larger local environmental gradients of some variables than applied in this study. Applying full seasonal ranges of environmental gradients would likely even strengthen the relationship with certain functional traits or with trait convergence. Since those environmental data are often not available at the spatial resolution of a specific station distribution, modeling approaches will be needed to more fully predict future scenarios of functional changes in such benthic systems. Here, we provided a benchmark to predict which functional traits will have competitive advantages by evaluating community assembly through an environmental filtering lens. While we focused on two Arctic shelf regions, we postulate that our framework of examining functional trait-environment relationships is applicable to many other systems. We suggest that this environmental filter lens will be particularly critical in regions of rapid environmental change such as the Arctic.
Data Availability Statement
The datasets presented in this study can be found in online repositories. The names of the repository/repositories and accession number(s) can be found in the article/Supplementary Material.
Author Contributions
LS conceived the ideas, carried out all analyses, and wrote the first draft of the manuscript. BB and KI contributed data. FM contributed to the analyses and statistics. All authors contributed to development of this study and ideas and commented on and edited the manuscript.
Funding
This work was part of the Arctic Marine Biodiversity Observing Network (AMBON) project, funded through a National Ocean Partnership Program (NOPP Grant No. NA14NOS0120158) by the National Oceanographic and Atmospheric Administration (NOAA), the Bureau of Ocean Management and Shell Exploration & Production, under management of the Integrated Ocean Observing System (IOOS), and the North Pacific Research Board (Project number: 1912). BB appreciates support from the US Department of the Interior, Bureau of Ocean Energy Management (BOEM), Alaska Outer Continental Shelf Region, Anchorage, Alaska, as part of the BOEM Environmental Studies Program, Contract No. M13PC00019 [Arctic Nearshore Impact Monitoring in Development Area (ANIMIDA) III: Contaminants, Sources, and Bioaccumulation]. Data for the Chukchi Sea were collected by the Arctic Marine Biodiversity Observing Network (AMBON) project, funded through a National Ocean Partnership Program (NOPP Grant No. NA14NOS0120158). Data for the Beaufort Sea were collected under BOEM Cooperative Agreement No. M12AC00011 “US-Canada Transboundary Fish and Lower Trophic Communities,” and the Arctic Nearshore Impact Monitoring in Development Area (ANIMIDA; Contract No. M13PC00019), US Department of the Interior, BOEM, Alaska Outer Continental Shelf Region, Anchorage, Alaska, as part of the BOEM Environmental Studies Program.
Conflict of Interest
The authors declare that the research was conducted in the absence of any commercial or financial relationships that could be construed as a potential conflict of interest.
Publisher’s Note
All claims expressed in this article are solely those of the authors and do not necessarily represent those of their affiliated organizations, or those of the publisher, the editors and the reviewers. Any product that may be evaluated in this article, or claim that may be made by its manufacturer, is not guaranteed or endorsed by the publisher.
Acknowledgments
We are grateful to Drs. Brenda Konar and Claudine Hauri (both UAF) for constructive comments on this manuscript. We are grateful to two journal reviewers who provided substantial and excellent input that improved the quality of this manuscript.
Supplementary Material
The Supplementary Material for this article can be found online at: https://www.frontiersin.org/articles/10.3389/fmars.2021.736917/full#supplementary-material
Footnotes
- ^ https://mbon.ioos.us/#search?type_group=all&tag—tag=ambon-projects&page=1
- ^ https://portal.aoos.org/#module-metadata/af3a4323-b854-4bce-890e-793c02b24394/cd3ada75-3897-444f-9d9c-42f8840f1018
- ^ https://www.ncei.noaa.gov/access/metadata/landing-page/bin/iso?id=gov.noaa.nodc:0162530
- ^ https://www.univie.ac.at/arctictraits/
References
Abe, H., Sampei, M., Hirawake, T., Waga, H., Nishino, S., and Ooki, A. (2019). Sediment-associated phytoplankton release from the seafloor in response to wind-induced barotropic currents in the Bering Strait. Front. Mar. Sci. 6:97. doi: 10.3389/fmars.2019.00097
Almond, P. M., Linse, K., Dreutter, S., Grant, S. M., Griffiths, H. J., Whittle, R. J., et al. (2021). In-situ image analysis of habitat heterogeneity and benthic biodiversity in the Prince Gustav Channel, Eastern Antarctic Peninsula. Front. Mar. Sci. 8:614496. doi: 10.3389/fmars.2021.614496
Andersen, K. M., Naylor, B. J., Endress, B. A., and Parks, C. G. (2015). Contrasting distribution patterns of invasive and naturalized non-native species along environmental gradients in a semi-arid montane ecosystem. Appl. Veg. Sci. 18, 683–693. doi: 10.1111/avsc.12185
Anderson, M., Ellingsen, K., and McArdle, B. (2006). Multivariate dispersion as a measure of beta diversity. Ecol. Lett. 9, 683–693. doi: 10.1111/j.1461-0248.2006.00926.x
Baltar, F., Bayer, B., Bednarsek, N., Deppeler, S., Escribano, R., Gonzalez, C. E., et al. (2019). Towards integrating evolution, metabolism, and climate change studies of marine ecosystems. Trends Ecol. Evol. 34, 1022–1033. doi: 10.1016/j.tree.2019.07.003
Bell, L. E., Bluhm, B. A., and Iken, K. (2016). Influence of terrestrial organic matter in marine food webs of the Beaufort Sea shelf and slope. Mar. Ecol. Prog. Ser. 550, 1–24. doi: 10.3354/meps11725
Blanchard, A. L., Parris, C. L., Knowlton, A. L., and Wade, N. R. (2013). Benthic ecology of the northeastern Chukchi Sea. Part I. Environmental characteristics and macrofaunal community structure, 2008-2010. Cont. Shelf Res. 67, 52–66. doi: 10.1016/j.csr.2013.04.021
Blonder, B., Nogués-Bravo, D., Borregaard, M. K., Donoghue, J. C., Jørgensen, P. M., Kraft, N. J. B., et al. (2015). Linking environmental filtering and disequilibrium to biogeography with a community climate framework. Ecology 96, 972–985. doi: 10.1890/14-0589.1
Bluhm, B. A., and Gradinger, R. (2008). Regional variability in food availability. Ecol. Appl. 18, S77–S96. doi: 10.1890/06-0562.1
Bluhm, B. A., Iken, K., Mincks Hardy, S., Sirenko, B. I., and Holladay, B. A. (2009). Community structure of epibenthic megafauna in the Chukchi Sea. Aquat. Biol. 7, 269–293. doi: 10.3354/ab00198
Bluhm, B. A., Janout, M. A., Danielson, S. L., Ellingsen, I., Gavrilo, M., Grebmeier, J. M., et al. (2020). The Pan-Arctic continental slope: sharp gradients of physical processes affect pelagic and benthic ecosystems. Front. Mar. Sci. 7:544386. doi: 10.3389/fmars.2020.544386
Bluhm, B. A., Piepenburg, D., and Von Juterzenka, K. (1998). Distribution, standing stock, growth, mortality and production of Strongylocentrotus pallidus (Echinodermata: echinoidea) in the northern Barents Sea. Polar Biol. 20, 325–334. doi: 10.1007/s003000050310
Bonsell, C., and Dunton, K. H. (2018). Long-term patterns of benthic irradiance and kelp production in the central Beaufort sea reveal implications of warming for Arctic inner shelves. Prog. Oceanogr. 162, 160–170. doi: 10.1016/j.pocean.2018.02.016
Cadotte, M. W., Carscadden, K., and Mirotchnick, N. (2011). Beyond species: functional diversity and the maintenance of ecological processes and services. J. Appl. Ecol. 48, 1079–1087. doi: 10.1111/j.1365-2664.2011.02048.x
Carmack, E., and Wassmann, P. (2006). Food webs, and physical-biological coupling on pan-Arctic shelves: unifying concepts and comprehensive perspectives. Prog. Oceanogr. 71, 446–477. doi: 10.1016/j.pocean.2006.10.004
Chevenet, F., Doleadec, S., and Chessel, D. (1994). A fuzzy coding approach for the analysis of long-term ecological data. Freshw. Biol. 31, 295–309. doi: 10.1111/j.1365-2427.1994.tb01742.x
Cochrane, S. K. J., Pearson, T. H., Greenacre, M., Costelloe, J., Ellingsen, I. H., Dahle, S., et al. (2012). Benthic fauna and functional traits along a Polar Front transect in the Barents Sea - Advancing tools for ecosystem-scale assessments. J. Mar. Syst. 94, 204–217. doi: 10.1016/j.jmarsys.2011.12.001
Conti, L., Schmidt-kloiber, A., Grenouillet, G., and Graf, W. (2014). A trait-based approach to assess the vulnerability of European aquatic insects to climate change. Hydrobiologia 721, 297–315. doi: 10.1007/s10750-013-1690-7
Cooper, L. W., Janout, M. A., Frey, K. E., Pirtle-Levy, R., Guarinello, M. L., Grebmeier, J. M., et al. (2012). The relationship between sea ice break-up, water mass variation, chlorophyll biomass, and sedimentation in the northern Bering Sea. Deep Sea Res. Part II Top. Stud. Oceanogr. 6, 141–162. doi: 10.1016/j.dsr2.2012.02.002
Cornwell, W. K., Schwilk, D. W., and Ackerly, D. D. (2006). A trait-based test for habitat filtering: convex hull volume. Ecology 87, 1465–1471.
Costello, M. J., Claus, S., Dekeyzer, S., Vandepitte, L., Tuama, É, Lear, D., et al. (2015). Biological and ecological traits of marine species. PeerJ 3:e1201. doi: 10.7717/peerj.1201
Danielson, S. L., Ahkinga, O., Ashjian, C., Basyuk, E., Cooper, L. W., Eisner, L., et al. (2020). Manifestation and consequences of warming and altered heat fluxes over the Bering and Chukchi Sea continental shelves. Deep Sea Res. Part II Top. Stud. Oceanogr. 177, 1–22. doi: 10.1016/j.dsr2.2020.104781
Danielson, S. L., Iken, K., Hauri, C., Hopcroft, R. R., McDonnell, A. M. P., Winsor, P., et al. (2017). Collaborative Approaches to Multi-Disciplinary Monitoring of the Chukchi Shelf Marine Ecosystem: Networks of Networks for Maintaining Long-Term Arctic Observations. OCEANS 2017 - Anchorage. Anchorage, AK, USA: IEEE.
Darby, D. A., Ortiz, J., Polyak, L., Lund, S., Jakobsson, M., and Woodgate, R. A. (2009). The role of currents and sea ice in both slowly deposited central Arctic and rapidly deposited Chukchi-Alaskan margin sediments. Glob. Planet. Change 68, 58–72. doi: 10.1016/j.gloplacha.2009.02.007
de Bello, F., Thuiller, W., Leps, J., Cholor, P., Clement, J., Macek, P., et al. (2009). Partitioning of functional diversity reveals the scale and extent of trait convergence and divergence. J. Veg. Sci. 20, 475–486. doi: 10.1111/j.1654-1103.2009.01042.x
Degen, R., and Faulwetter, S. (2019). The Arctic Traits Database - A repository of arctic benthic invertebrate traits. Earth Syst. Sci. Data Discuss. 11, 1–25. doi: 10.5194/essd-2018-97
Denelle, P., Violle, C., and Munoz, F. (2019). Distinguishing the signatures of local environmental filtering and regional trait range limits in the study of trait–environment relationships. Oikos 128, 960–971. doi: 10.1111/oik.05851
Divine, L. M., Iken, K., and Bluhm, B. A. (2015). Regional benthic food web structure on the Alaska Beaufort Sea shelf. Mar. Ecol. Prog. Ser. 531, 15–32. doi: 10.3354/meps11340
Dolédec, S., and Chessel, D. (1994). Co-inertia analysis: an alternative method for studying species — environment relationships. Freshw. Biol. 31, 277–294.
Dolédec, S., Chessel, D., ter Braak, C. J. F., and Champely, S. (1996). Matching species traits to environmental variables: a new three-table ordination method. Environ. Ecol. Stat. 3, 143–166. doi: 10.1007/BF02427859
Doxaran, D., Devred, E., and Babin, M. (2015). A 50 % increase in the mass of terrestrial particles delivered by the Mackenzie River into the Beaufort Sea (Canadian Arctic Ocean) over the last 10 years. Biogeosciences 12, 3551–3565. doi: 10.5194/bg-12-3551-2015
Dray, S., Choler, P., Dolédec, S., Peres-Neto, P. R., Thuiller, W., Pavoine, S., et al. (2014). Combining the fourth-corner and the RLQ methods for assessing trait responses to environmental variation. Ecology 95, 14–21. doi: 10.1890/13-0196.1
Dray, S., and Dufour, A. B. (2007). The ade4 package: implementing the duality diagram for ecologists. J. Stat. Softw. 22, 1–20.
Dray, S., and Legendre, P. (2008). Testing the Species Traits-Environment Relationships: the Fourth-Corner Problem Revisited. Ecology 89, 3400–3412. doi: 10.1890/08-0349.1
Ellis, J., Cummings, V., Hewitt, J., Thrush, S., and Norkko, A. (2002). Determining effects of suspended sediment on condition of a suspension feeding bivalve (Atrina zelandica): results of a survey, a laboratory experiment and a field transplant experiment. J. Exp. Mar. Biol. Ecol. 267, 147–174. doi: 10.1016/S0022-0981(01)00355-0
Ershova, E., Descoteaux, R., Wangensteen, O., Iken, K., Hopcroft, R., Smoot, C., et al. (2019). Diversity and distribution of meroplanktonic larvae in the Pacific Arctic and connectivity with adult benthic invertebrate communities. Front. Mar. Sci. 6:490. doi: 10.3389/FMARS.2019.00490
Everatt, M. J., Convey, P., Bale, J. S., Worland, M. R., and Hayward, S. A. L. (2015). Responses of invertebrates to temperature and water stress: a polar perspective. J. Therm. Biol. 54, 118–132. doi: 10.1016/j.jtherbio.2014.05.004
Feder, H. M., Naidu, A. S., Jewett, S. C., Hameedi, J. M., Johnson, W. R., and Whitledge, T. E. (1994). The northeastern Chukchi Sea: benthos-environmental interactions. Mar. Ecol. Prog. Ser. 111, 171–190. doi: 10.3354/meps111171
Goddard, J. H. (2004). Developmental mode in benthic opisthobranch molluscs from the northeast Pacific Ocean: feeding in a sea of plenty. Can. J. Zool. 82, 1954–1968. doi: 10.1139/z05-008
Götzenberger, L., de Bello, F., Bråthen, K. A., Davison, J., Dubuis, A., Guisan, A., et al. (2012). Ecological assembly rules in plant communities-approaches, patterns and prospects. Biol. Rev. 87, 111–127. doi: 10.1111/j.1469-185X.2011.00187.x
Grebmeier, J. M., Bluhm, B. A., Cooper, L. W., Denisenko, S. G., Iken, K., Kedra, M., et al. (2015a). Time-series benthic community composition and biomass and associated environmental characteristics in the Chukchi Sea during the RUSALCA 2004-2012 Program. Oceanography 28, 116–133. doi: 10.5670/oceanog.2015.61
Grebmeier, J. M., Bluhm, B. A., Cooper, L. W., Danielson, S. L., Arrigo, K. R., Blanchard, A. L., et al. (2015b). Ecosystem characteristics and processes facilitating persistent macrobenthic biomass hotspots and associated benthivory in the Pacific Arctic. Prog. Oceanogr. 136, 92–114. doi: 10.1016/j.pocean.2015.05.006
Grebmeier, J. M., Cooper, L. W., Feder, H. M., and Sirenko, B. I. (2006). Ecosystem dynamics of the Pacific-influenced Northern Bering and Chukchi Seas in the Amerasian Arctic. Prog. Oceanogr. 71, 331–361. doi: 10.1016/j.pocean.2006.10.001
Grebmeier, J. M., Feder, H. M., and McRoy, P. C. (1989). Pelagic-benthic coupling on the shelf of the northern Bering and Chukchi Seas. II. Benthic community structure. Mar. Ecol. Prog. Ser. 51, 253–268. doi: 10.3354/meps048057
Grime, J. P. (2006). Trait convergence and trait divergence in herbaceous plant communities: mechanisms and consequences. J. Veg. Sci. 17, 255–260.
Gunderson, D. R., and Ellis, I. E. (1986). Development of a plumb staff beam trawl for sampling demersal fauna. Fish. Res. 4, 35–41. doi: 10.1016/0165-7836(86)90026-3
Hauri, C., Danielson, S., McDonnell, A. M. P., Hopcroft, R. R., Winsor, P., Shipton, P., et al. (2018). From sea ice to seals: a moored marine ecosystem observatory in the Arctic. Ocean Sci. 14, 1423–1433. doi: 10.5194/os-14-1423-2018
Iken, K., Bluhm, B., and Dunton, K. (2010). Benthic food-web structure under differing water mass properties in the southern Chukchi Sea. Deep Sea Res. Part II Top. Stud. Oceanogr. 57, 71–85. doi: 10.1016/j.dsr2.2009.08.007
Iken, K., Mueter, F., Grebmeier, J. M., Cooper, L. W., Danielson, S. L., and Bluhm, B. A. (2019). Developing an observational design for epibenthos and fish assemblages in the Chukchi Sea. Deep Sea Res. Part II Top. Stud. Oceanogr. 162, 180–190. doi: 10.1016/j.dsr2.2018.11.005
Jakobsson, M., Mayer, L., Coakley, B., Dowdeswell, J. A., Forbes, S., Fridman, B., et al. (2012). The International Bathymetric Chart of the Arctic Ocean (IBCAO) Version 3.0. Geophys. Res. Lett. 39, 1–6. doi: 10.1029/2012GL052219
Jørgensen, L. L., Primicerio, R., Ingvaldsen, R. B., Fossheim, M., Strelkova, N., Thangstad, T. H., et al. (2019). Impact of multiple stressors on sea bed fauna in a warming Arctic. Mar. Ecol. Prog. Ser. 608, 1–12. doi: 10.3354/meps12803
Kasper, J., Dunton, K. H., Schonberg, S. V., Trefry, J., Bluhm, B., Durell, G., et al. (2017). Arctic Nearshore Impact Monitoring in Development Area (ANIMIDA) III: Contaminants, Sources, and Bioaccumulation. U.S. Dept. of the Interior, Bureau of Ocean Energy Management, Alaska OCS Region, Anchorage, AK.
Keddy, P. A. (1992). Assembly and response rules: two goals for predictive community ecology. J. Veg. Sci. 3, 157–164. doi: 10.2307/3235676
Kraft, N. J. B., Adler, P. B., Godoy, O., James, E. C., Fuller, S., and Levine, J. M. (2015). Community assembly, coexistence and the environmental filtering metaphor. Funct. Ecol. 29, 592–599. doi: 10.1111/1365-2435.12345
Krumhansl, K., Jamieson, R., and Krkosek, W. (2016). Using species traits to assess human impacts on near shore benthic ecosystems in the Canadian Arctic. Ecol. Indic. 60, 495–502. doi: 10.1016/j.ecolind.2015.07.026
Laliberté, E., and Legendre, P. (2010). A distance-based framework for measuring functional diversity from multiple traits. Ecology 91, 299–305.
Lavorel, S., Grigulis, K., Mcintyre, S., Williams, N. S. G., Garden, D., Berman, S., et al. (2008). Assessing functional diversity in the field – methodology matters! Funct. Ecol. 22, 134–147. doi: 10.1111/j.1365-2435.2007.01339.x
Legendre, P., Galzin, R., and Harmelin-vivien, M. L. (1997). Relating Behavior to Habitat: solutions to the fourth-corner problem. Ecology 78, 547–562. doi: 10.2307/2266029
Liu, K., Lin, H., He, X., Huang, Y., Li, Z., Lin, J., et al. (2019). Functional trait composition and diversity patterns of marine macrobenthos across the Arctic Bering Sea. Ecol. Indic. 102, 673–685. doi: 10.1016/j.ecolind.2019.03.029
MacArthur, R., and Levins, R. (1967). The limiting similarity, convergence, and divergence of coexisting species. Am. Soc. Nat. 101, 377–385.
Mahoney, A. R., Eicken, H., Gaylord, A. G., and Gens, R. (2014). Landfast sea ice extent in the Chukchi and Beaufort Seas: the annual cycle and decadal variability. Cold Reg. Sci. Technol. 103, 41–56. doi: 10.1016/j.coldregions.2014.03.003
Mason, N. W. H., Mouillot, D., Lee, W. G., and Wilson, J. B. (2005). Functional richness, functional evenness and functional divergence: the primary components of functional diversity. Oikos 111, 112–118. doi: 10.1111/j.0030-1299.2005.13886.x
McGill, B. J., Enquist, B. J., Weiher, E., and Westoby, M. (2006). Rebuilding community ecology from functional traits. Trends Ecol. Evol. 21, 178–185. doi: 10.1016/j.tree.2006.02.002
Menegotto, A., Dambros, C. S., and Netto, S. A. (2019). The scale-dependent effect of environmental filters on species turnover and nestedness in an estuarine benthic community. Ecology 100, 1–9. doi: 10.1002/ecy.2721
Mueter, F. J., and Litzow, M. A. (2008). Sea ice retreat alters the biogeography of the Bering Sea continental shelf. Ecol. Appl. 18, 309–320. doi: 10.1890/07-0564.1
Muscarella, R., and Uriarte, M. (2016). Do community-weighted mean functional traits reflect optimal strategies? Proc. R. Soc. B Biol. Sci. 283:20152434.
Nygaard, B., and Ejrnaes, R. (2004). A new approach to functional interpretation of vegetation data. J. Veg. Sci. 15, 49–56.
Oksanen, A. J., Blanchet, F. G., Friendly, M., Kindt, R., Legendre, P., Mcglinn, D., et al. (2020). Vegan: community Ecology Package. R Package Version 2.2-0.
Pearson, D. E., Ortega, Y. K., and Eren, Ö., and Hierro, J. L. (2018). Community assembly theory as a framework for biological invasions. Trends Ecol. Evol. 33, 313–325. doi: 10.1016/j.tree.2018.03.002
Peck, L. S., Webb, K. E., and Bailey, D. M. (2004). Extreme sensitivity of biological function to temperature in Antarctic marine species. Funct. Ecol. 18, 625–630. doi: 10.1111/j.0269-8463.2004.00903.x
Pickart, R. S., Moore, G. W. K., Torres, D. J., Fratantoni, P. S., Goldsmith, R. A., and Yang, J. (2009). Upwelling on the continental slope of the Alaskan Beaufort Sea: storms, ice, and oceanographic response. J. Geophys. Res. 114, 1–17.
Pickart, R. S., Schulze, L. M., Moore, G. W. K., Charette, M. A., Arrigo, K. R., Van Dijken, G., et al. (2013). Long-term trends of upwelling and impacts on primary productivity in the Alaskan Beaufort Sea. Deep Sea Res. Part I Oceanogr. Res. Pap. 79, 106–121. doi: 10.1016/j.dsr.2013.05.003
Pillar, V. D., Duarte, L. D. S., Sosinski, E. E., and Joner, F. (2009). Discriminating trait-convergence and trait-divergence assembly patterns in ecological community gradients. J. Veg. Sci. 20, 334–348. doi: 10.1111/j.1654-1103.2009.05666.x
Pinheiro, J., Bates, D., DebRoy, S., and Sarkar, D. (2020). Linear and Nonlinear Mixed Effects Models. R Package Version 3.1-338.
Pisareva, M. N., Pickart, R. S., Iken, K., Ershova, E. A., Grebmeier, J. M., Cooper, L. W., et al. (2015). The relationship between patterns of benthic fauna and zooplankton in the Chukchi Sea and physical forcing. Oceanography 28, 68–83. doi: 10.5670/oceanog.2015.58
Queiros, A. M., Birchenough, S. N. R., Bremner, J., Godbold, J. A., Parker, R. E., and Queir, A. M. (2013). A bioturbation classification of European marine infaunal invertebrates. Ecol. Evol. 3, 3958–3985. doi: 10.1002/ece3.769
R Core Team (2020). R: A Language and Environment For Statistical Computing. Vienna: R. Foundation for Statistical Computing. Available online at: https://www.R-project.org/
Rachold, V., Are, F. E., Atkinson, D. E., Cherkashov, G., and Solomon, S. M. (2005). Arctic Coastal Dynamics (ACD): an introduction. Geo-Mar. Lett. 25, 63–68. doi: 10.1007/s00367-004-0187-9
Rachold, V., Grigoriev, M. N., Are, F. E., Solomon, S., Reimnitz, E., Kassens, H., et al. (2000). Coastal erosion vs riverline sediment discharge in the Arctic shelf seas. Int. J. Earth Sci. 89, 450–460. doi: 10.1007/s005310000113
Rand, K., Logerwell, E., Bluhm, B., Chenelot, H., Danielson, S., Iken, K., et al. (2018). Using biological traits and environmental variables to characterize two Arctic epibenthic invertebrate communities in and adjacent to Barrow Canyon. Deep Sea Res. Part II Top. Stud. Oceanogr. 152, 154–169. doi: 10.1016/j.dsr2.2017.07.015
Ravelo, A. M., Bluhm, B. A., Foster, N., and Iken, K. (2020). Biogeography of epibenthic assemblages in the central Beaufort Sea. Mar. Biodivers. 50, 1–19. doi: 10.1007/s12526-019-01036-9
Ravelo, A. M., Konar, B., Bluhm, B., and Iken, K. (2017). Growth and production of the brittle stars Ophiura sarsii and Ophiocten sericeum (Echinodermata: ophiuroidea). Cont. Shelf Res. 139, 9–20. doi: 10.1016/j.csr.2017.03.011
Ravelo, A. M., Konar, B., and Bluhm, B. A. (2015). Spatial variability of epibenthic communities on the Alaska Beaufort Shelf. Polar Biol. 38, 1783–1804. doi: 10.1007/s00300-015-1741-9
Ravelo, A. M., Konar, B., Trefry, J. H., and Grebmeier, J. M. (2014). Epibenthic community variability in the northeastern Chukchi Sea. Deep Sea Res. Part II Top. Stud. Oceanogr. 102, 119–131. doi: 10.1016/j.dsr2.2013.07.017
Renaud, P., Sejr, M. K., Bluhm, B. A., Sirenko, B., and Ellingsen, I. H. (2015). The future of Arctic benthos: expansion, invasion, and biodiversity. Prog. Oceanogr. 139, 244–257. doi: 10.1016/j.pocean.2015.07.007
Renaud, P., Wallhead, P., Kotta, J., Włodarska-Kowalczuk, M., Bellerby, R. G. J., Rätsep, M., et al. (2019). Arctic sensitivity? Suitable habitat for benthic taxa is surprisingly robust to climate change. Front. Mar. Sci. 6:538. doi: 10.3389/fmars.2019.00538
Rhoads, D. C., and Young, D. K. (1970). The influences of deposit feeding benthos on bottom stability and community trophic structure. J. Mar. Res. 28, 150–178.
Roxburgh, S. H., Shea, K., and Wilson, J. B. (2004). The intermediate disturbance hypothesis: patch dynamics and mechanisms of species coexistence. Ecology 85, 359–371. doi: 10.1890/03-0266
Smoot, C. A., and Hopcroft, R. R. (2017). Cross-shelf gradients of epipelagic zooplankton communities of the Beaufort Sea and the influence of localized hydrographic features. J. Plankton Res. 39, 65–75. doi: 10.1093/plankt/fbw080
Snelgrove, P. V. R., Austen, M. C., Boucher, G., Heip, C., Hutchings, P. A., King, G. M., et al. (2000). Linking biodiversity above and below the marine sediment-water interface. BioScience 50, 1076–1088.
Snelgrove, P. V. R., and Butman, C. A. (1994). Animal sediment relationships revisited: cause versus effect. Oceanogr. Mar. Biol. 32, 111–177.
Snelgrove, P. V. R., Grassee, J. P., Grassle, J. F., Petrecca, R. F., and Hongguang, M. (1999). In situ habitat selection by settling larvae of marine soft-sediment invertebrates. Limnol. Oceanogr. 44, 1341–1347. doi: 10.4319/lo.1999.44.5.1341
Snelgrove, P. V. R., Grassle, J. F., Grassle, J. P., Petrecca, R. F., and Stocks, K. I. (2001). The role of colonization in establishing patterns of community composition and diversity in shallow-water sedimentary communities. J. Mar. Res. 59, 813–831. doi: 10.1357/002224001762674953
Spasojevic, M. J., and Suding, K. N. (2012). Inferring community assembly mechanisms from functional diversity patterns: the importance of multiple assembly processes. J. Ecol. 100, 652–661. doi: 10.1111/j.1365-2745.2011.01945.x
Sutton, L., Iken, K., Bluhm, B. A., and Mueter, F. J. (2020). Comparison of functional diversity of two Alaskan Arctic shelf epibenthic communities. Mar. Ecol. Prog. Ser. 651, 1–21. doi: 10.3354/meps13478
Thrush, A. S. F., Hewitt, J. E., Cummings, V. J., Ellis, J. I., Hatton, C., Lohrer, A., et al. (2004). Muddy waters: elevating sediment input to coastal and estuarine habitats. Front. Ecol. Environ. 2, 299–306. doi: 10.1890/1540-9295
Valdivia, N., Eliseo, V. S., César, F., Aguilera, M. A., and Broitman, B. R. (2017). Context-dependent functional dispersion across similar ranges of trait space covered by intertidal rocky shore communities. Ecol. Evol. 7, 1882–1891. doi: 10.1002/ece3.2762
Wadhams, P. (2012). New predictions of extreme keel depths and scour frequencies for the Beaufort Sea using ice thickness statistics. Cold Reg. Sci. Technol. 7, 77–82. doi: 10.1016/j.coldregions.2011.12.002
Webb, C. T., Hoeting, J. A., Ames, G. M., Pyne, M. I., and LeRoy Poff, N. (2010). A structured and dynamic framework to advance traits-based theory and prediction in ecology. Ecol. Lett. 13, 267–283. doi: 10.1111/j.1461-0248.2010.01444.x
Weiher, E., Clarke, G. D. P., and Keddy, P. A. (1998). Community assembly rules, morphological dispersion, and the coexistence of plant species. Oikos 81, 309–322.
Weiher, E., Freund, D., Bunton, T., Stefanski, A., Lee, T., and Bentivenga, S. (2011). Advances, challenges and a developing synthesis of ecological community assembly theory. Philos. Trans. R. Soc. B Biol. Sci. 366, 2403–2413. doi: 10.1098/rstb.2011.0056
Weingartner, T., Aagaard, K., Woodgate, R., Danielson, S., Sasaki, Y., and Cavalieri, D. (2005). Circulation on the north central Chukchi Sea shelf. Deep Sea Res. II Top. Stud. Oceanogr. 52, 3150–3174. doi: 10.1016/j.dsr2.2005.10.015
Weingartner, T. J., Aerts, L. A. M., Blanchard, A. L., Gall, A. E., Gallaway, B. J., Hannay, D. E., et al. (2013). The offshore northeastern Chukchi Sea, Alaska: a complex high-latitude ecosystem. Cont. Shelf Res. 67, 147–165. doi: 10.1016/j.csr.2013.02.002
Weingartner, T. J., Danielson, S. L., Potter, R. A., Trefry, J. H., Mahoney, A., Savoie, M., et al. (2017). Circulation and water properties in the landfast ice zone of the Alaskan Beaufort Sea. Cont. Shelf Res. 148, 185–198. doi: 10.1016/j.csr.2017.09.001
Whitehouse, G. A., Buckley, T. W., and Danielson, S. L. (2017). Diet compositions and trophic guild structure of the eastern Chukchi Sea demersal fish community. Deep Sea Res. Part II Top. Stud. Oceanogr. 135, 95–110. doi: 10.1016/j.dsr2.2016.03.010
Woodgate, R. A. (2018). Increases in the Pacific inflow to the Arctic from 1990 to 2015, and insights into seasonal trends and driving mechanisms from year-round Bering Strait mooring data. Prog. Oceanogr. 160, 124–154. doi: 10.1016/j.pocean.2017.12.007
Young, J. K., Black, B. A., Clarke, J. T., Schonberg, S. V., and Dunton, K. H. (2017). Abundance, biomass and caloric content of Chukchi Sea bivalves and association with Pacific walrus (Odobenus rosmarus divergens) relative density and distribution in the northeastern Chukchi Sea. Deep Sea Res. Part II Top. Stud. Oceanogr. 144, 125–141. doi: 10.1016/j.dsr2.2017.04.017
Keywords: community assembly, environmental filtering, functional traits, trait convergence, Arctic
Citation: Sutton L, Mueter FJ, Bluhm BA and Iken K (2021) Environmental Filtering Influences Functional Community Assembly of Epibenthic Communities. Front. Mar. Sci. 8:736917. doi: 10.3389/fmars.2021.736917
Received: 06 July 2021; Accepted: 14 September 2021;
Published: 10 November 2021.
Edited by:
Iñigo Muxika, Technological Center Expert in Marine and Food Innovation (AZTI), SpainReviewed by:
Gert Van Hoey, Institute for Agricultural, Fisheries and Food Research (ILVO), BelgiumVadim Khaitov, Saint Petersburg State University, Russia
Copyright © 2021 Sutton, Mueter, Bluhm and Iken. This is an open-access article distributed under the terms of the Creative Commons Attribution License (CC BY). The use, distribution or reproduction in other forums is permitted, provided the original author(s) and the copyright owner(s) are credited and that the original publication in this journal is cited, in accordance with accepted academic practice. No use, distribution or reproduction is permitted which does not comply with these terms.
*Correspondence: Lauren Sutton, lsutton7@alaska.edu