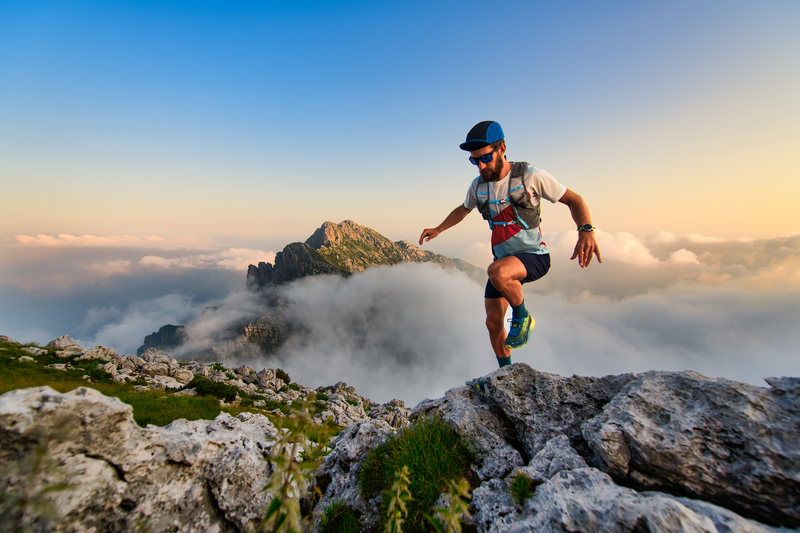
94% of researchers rate our articles as excellent or good
Learn more about the work of our research integrity team to safeguard the quality of each article we publish.
Find out more
ORIGINAL RESEARCH article
Front. Mar. Sci. , 01 December 2021
Sec. Marine Fisheries, Aquaculture and Living Resources
Volume 8 - 2021 | https://doi.org/10.3389/fmars.2021.736462
The maturity ogive is vital to defining the fraction of a population capable of reproduction. In this study, we proposed a novel approach, a Bayesian multilevel ordinal regression (i.e., Bayesian continuation ratio model), to model the maturity ogive. The model assumes that the observed maturity stage originates from the categorization of latent continuous variables. We demonstrated this approach by testing whether there are differences in the maturity ogive of skipjack tuna (Katsuonus pelamis) in the western and central Pacific Ocean between two school types, i.e., free-swimming and floating-object-associated schools. The model results show that K. pelamis, given the same fork length, are more likely to have a higher maturity stage in a free-swimming school than those associated with floating objects. The gonadosomatic index revealed the same conclusion. Our results indicate that fish aggregation devices (FADs) could negatively affect the maturity of K. pelamis and consequently reduce the population reproductive potential. This study provides (1) an alternative approach to analyze fisheries ordinal data; (2) important quantitative evidence to evaluate the existing ecological hypotheses; and (3) implications for tuna fisheries management.
The maturity ogive, which measures the proportion of mature fish at age or size, is instrumental to defining the fraction of the population that is capable of reproduction, commonly referred to as the spawning stock biomass (SSB) in a population dynamic context. It is highly influential upon the results of stock assessments and determining reference points for management (King and McFarlane, 2003). The adequate estimation of the maturity ogive is a critical step for assessment and management and typically requires (1) representative samples of ovaries from a well-designed sample adapted to a species-specific reproductive strategy; (2) precise determination of the stage of sexual maturation; (3) fitting a statistical model to the data from maturity staging. The maturity stage is commonly examined using either microscopic or macroscopic staging (Flores et al., 2019). Macroscopic staging is based on visual evaluation of the external gonad development and could be uncertain and inaccurate due to a lack of differentiating visible structures between stages; for instance, immature and regenerating/omitted spawning stages have very similar macroscopic aspects of the ovaries and are often misidentified. Microscopic staging is considered to be accurate and provides an unambiguous determination of maturity stages. However, histology is expensive, time consuming and impractical at sea during routine surveys (Flores et al., 2019). Other methods such as gonometric method that is based on the gonodasomatic index (GSI) has also been evaluated as an alternative to maturity staging (Flores et al., 2014, 2019).
Ordinal data like maturity stages arise when underlying continuous processes are censored in the observational process, where measurements are limited to discrete categories. This type of data is not uncommon in fisheries science. However, ordinal regression, which models the influence of latent effects on an ordinal outcome, is not commonly used in fisheries science. There are different types of ordinal regressions to model an ordinal response depending upon how their logits are formed and underlying assumptions (Bürkner and Vuorre, 2019): proportional odds model (POM), continuation ratio model (CRM), and adjacent category model (ACM). POMs, considered as cumulative higher categories verses cumulative remaining lower categories, are often used when assuming responses are from categorization of a latent continuous variable. However, CRMs (considered as cumulative higher category verses just lower category alone) and ACMs (between any of two consecutive categories) can assume that for every category there is a latent continuous variable that determines the transition of successive categories. The POM focuses on the cumulative odds of grouped categories, whereas the CRM estimates the conditional odds of being in a particular category, given that an individual has reached that category or above (Liu et al., 2011).
The maturity ogive is conventionally modeled as a function of age and/or size using logistic regression, often collapsing maturity stages into two levels (i.e., immature and mature). Such a dichotomization throws away important information in the data that are ordinal measurements, i.e., maturity stages, and the resulting estimates of the maturity ogive can be sensitive to the maturity scales and “cut point” used to determine whether an individual is matured or not (Núñez et al., 2015). Ordinal regression models making full use of the ordinality of the data is an approach that could fit maturity stages in a more natural way and potentially improve the accuracy of SSB calculation. However, to our knowledge, this method has not been used for modeling fish maturity data.
Skipjack tuna (Katsuwonus pelamis) are widely distributed in tropical and subtropical waters throughout the Western and Central Pacific Ocean (WCPO) and support important fisheries. In 2018, the catch of world’s major commercial tunas was 5.2 million tons, of which the majority was skipjack tuna and caught via purse seining (ISSF, 2020). There are two types of tuna schools which purse seiners target: schools associated with floating objects including fish aggregation devices (FADs) (hereinafter referred to as an associated school) and free-swimming schools. About half of the total tuna catches in the WCPO were from associated schools, although the number of sets around FADs was much less than that on free-swimming schools (WCPFC, 2019). Capturing schools of tunas associated with floating objects was pioneered by tuna purse seiners operating in the eastern Pacific Ocean during the late 1950s (Hampton, 1993). Artificial FADs have been increasingly constructed and released to the global ocean since 1980s because floating objects significantly increase the catchability of tunas, i.e., purse seine sets around floating objects yield much higher success rate than those made on free-swimming schools (Suzuki et al., 2003).
The potential negative impacts of using FADs have gained a significant amount of scientific attention, including growth overfishing of tuna stocks (FAD catches are usually characterized by small individuals, whereas catches on free-swimming schools are typically dominated by large tuna), increased bycatch, and alteration of the life history traits of the species associated with FADs (Fonteneau et al., 2000; Bromhead, 2003; Hallier and Gaertner, 2008). The potential effects of floating objects on the behavior and biology of tunas have been widely studied, and hypotheses have been developed to explain ecological consequences, e.g., “ecological trap” (Marsac et al., 2000; Hallier and Gaertner, 2008). This hypothesis contends that releasing a large number of FADs in the ocean could prevent tuna from moving to places where they would go normally, lead them to low-quality habitats and consequently negatively impact their biology. For example, Jaquemet et al. (2011) found that K. pelamis and small yellowfin tuna (Thunnus albacores) caught around drifting FADs had a significantly higher frequency of empty stomachs and concluded that FADs could negatively impact the growth of K. pelamis and small T. albacores. Ashida et al. (2017) found that the relative condition factor and proportion of mature fish significantly differ between associated and free-swimming schools (lower in associated schools). However, K. pelamis and T. albacores are considered as income breeders (Zudaire et al., 2014; Grande et al., 2016), which means they rely on concurrent energy income from feeding to support the cost of reproduction. Therefore, the condition factor might not be directly related to maturation. Assuming the “ecological trap” hypothesis is true and considering tunas are income breeders, one would hypothesize that fish associated with floating objects for an adequate period of time would have a lower maturity stage compared with those in free-swimming schools, given the same age/size. However, there are few studies that have compared the size-at-maturity between tuna associated with FADs and free-swimming schools. This may be because most of the tuna catches (e.g., bigeye tuna (Thunnus obesus) and T. albacores) in floating object sets consist of juvenile individuals (Bromhead, 2003; Dagorn et al., 2013). Ashida et al. (2017) compared the difference in reproductive traits of K. pelamis females between the two schools, but they did not examine whether there was a difference in size-at-maturity between the two schools.
In this study, we proposed a novel approach: a Bayesian multilevel ordinal regression, to model the maturity ogive using maturity stage data. We demonstrated this approach by testing the hypothesis that there are differences in maturity characteristic between the associated and free-swimming schools. We also compared the results of ordinal regressions with those obtained from the conventional logistic regressions.
A total of 2013 K. pelamis were sampled on Chinese commercial tuna fishing vessels in the WCPO during 2007–2014 from 154 fishing locations (Figure 1). The sampling date, location (latitude and longitude) and the school type (i.e., free swimming school or associated school) were recorded for all specimens. All specimens were measured for fork length (FL, mm to the nearest 0.1 mm) and gonads were removed and weighed. Body weight was measured when possible. The majority of the specimens were collected from associated schools (n = 1655). Gonadal stage of maturity was assigned for each specimen based on observation of the morphological features of the gonad within the body cavity. We followed the general maturity criteria for large pelagic gonads and established six developmental stages for both female and male (Table 1): stage-1: Gonads are small ribbon-like, not possible to determine sex by gross examination; stage-2: Immature; stage-3: Early maturing; stage-4: Late maturing; stage-5: Ripe; and stage-6: Spent/Spawned. Sex was determined for each individual based on visual examination of the gonad. Of the total specimens, 929 specimens were female, 830 specimens were male. The sex of the rest 254 specimens (stage-1) could not be identified. We excluded the data of individuals identified as Spent/Spawned (i.e., stage-6) in this analysis due to the high sprobability of misidentification (Figure 2).
Figure 1. Fishing locations of Chinese commercial tuna fishing vessels in the Western and Central Pacific Ocean during 2007–2014 targeting free-swimming (red filled squares) and floating-object-associated (blue dots) schools.
Table 1. Description of macroscopic characteristics of the gonadal phases in the reproductive cycle of Katsuonus pelamis.
Figure 2. Histogram of fork length (FL, cm) of Katsuonus pelamis samples collected in the Western and Central Pacific Ocean during 2007–2014 by maturity stage (A) and school type (B).
The ordinal regression model is motivated by assuming underlying latent continuous variables that are related to the ordinal response through the threshold concept (Hedeker and Gibbons, 1994). Because the maturity data are ordinal in nature, instead of a threshold value such as the cut-point to separate mature from immature fish as in the conventional logistic regression approach, there are several thresholds (depending on the number of maturity stages) that separate individuals into the various response categories, i.e., maturity stages. Gonadal maturation is a sequential biological process throughout the reproductive cycle, i.e., individuals must pass through lower stages in order to reach higher stages. Therefore, a CRM was considered in this study.
Fisheries data, including maturity data, are often structured in a hierarchical manner. For example, observations of the maturity stage taken from an individual fish are nested within sampling sites. Maturity stages within a sampling site are likely to be more similar to each other compared to observations between sampling sites. Such multilevel structure would violate the assumption of independence and should be accounted for in the analyses. Multilevel models have been extensively developed to model hierarchical data (Wagner et al., 2011). In this analysis, we proposed a multilevel CRM to estimate the maturity ogive. The CRM estimates the odds of being in a particular stage k relative to being above that stage and can be formulated as:
where P(Y = k|Xβ + Zμ) is the conditional probability of being in stage k, conditional on being that stage or beyond, given the linear predictor (Xβ + Zμ), k = 1, 2, 3,…,K−1, τk are the thresholds or cut points, β are the regression coefficients at population-level (i.e., fixed effects), X are population-level covariates, Z are grouping variables, μ are group-level coefficients, which are also known as random effects that follow a normal distribution with a mean of zero. We assumed that β are constant across all response categories, i.e., the effect of each predictor is invariant across the ordinal responses.
The covariates we considered in the ordinal regression were fork length (FL), school type (School), fishing location (location), year (Year) and month (Month). We excluded sex as a covariate in the model because sex could not be identified for stage-1 fish. The linear predictor of the full model was specified as
where FL and School are population-level effects, and location, Year and Month are group-level effects, and Month is nested within Year. We included an interaction term to examine whether the effect of fork length on maturity is independent of school type. We conducted model comparisons of the full model to reduced models to better understand the relative importance of model terms (Supplementary Table 1).
All models were fitted with the “Bayesian Regression Models using Stan” (brms) R package (Bürkner, 2017). We chose a weakly informative prior, i.e., Normal(0,10) for β and τk, and a Half-Cauchy prior, i.e., Cauchy(0,5) for the random effect standard deviations. We ran 4 chains for 1,500 warm-up iterations followed by an additional 3,000 iterations. We confirmed algorithm convergence with visual checks (e.g., traceplots) and the Rhat statistic. We chose the leave-one-out information criterion (LOOIC), a Bayesian information criterion based on out-of-sample predictive performance, to compare models fitted to the same data (Vehtari et al., 2017). The model with a lower LOOIC is expected to have better predictive accuracy (Hooten and Hobbs, 2015). We used the “LOO” R package to compute the LOOIC (v2.4.1; Vehtari et al., 2020). To test our hypothesis that fish in free-swimming schools on average have a higher maturity stage compared with those associated with floating objects given the same age/size, we calculated the evidence ratio of the posterior probability of School > 0 (i.e., school type has a positive effect on maturity) and the posterior probability of School < 0.
We tested the sensitivity of the estimated maturity ogive to different types of models and data. First, we tested the sensitivity of results to the potential misidentification of maturity stages by running the analysis using the full maturity dataset including individuals identified as stage 6. Second, we fitted both ordinal and conventional logistic models with the same covariates to the same datasets. We chose a parsimonious model, M7, for the sensitivity analyses. The sensitivity analyses resulted in four model runs: ordinal and logistic regression fitted to full dataset and the dataset with stage-6 fish excluded, respectively. We considered fish in stages 4–6 to be matured fish. We also investigated that whether the logistic model with the same covariates as the full model would lead to the same conclusion regarding the effect of school type based on ordinal regression.
The gonadosomatic index (GSI), measuring the ratio between gonad weight and body weight, can indicate maturity and stage of spawning and is commonly used to compare reproductive condition among individuals (Flores et al., 2014, 2019). If there are differences in the maturity condition between the two schools, it is expected that the GSI also differs between the two schools. We therefore tested this hypothesis using a Bayesian generalized linear model
where GSI is the ratio between gonad weight and gutted weight, β0, β1, β2 and β3 are population-level effects and are given a weakly informative prior, i.e., Normal(0,10); αlocation is the site-level effect, which follows a normal distribution with a mean of zero. The standard deviation is given a Half-Caughy prior, i.e., Caughy(0,5). The model was also fitted with the “Bayesian Regression Models using Stan” (brms) R package (Bürkner, 2017).
A total of 1,501 specimens (stages 1–5) were used in the analyses. The majority of them were from associated schools (1,202 specimens). The range of FL of skipjack tuna from associated and free-swimming schools were (27–73.3 cm) and (22.8–74.6 cm), respectively. The median FLs of skipjack tuna from associated and free-swimming schools were 44.8 and 53.2 cm, respectively (Figure 2). The median FL increased as tuna progressed to higher maturity stages, except stage 6 (Figure 2). This indicates that the likelihood of misidentifying spawned tuna is high.
Model selection indicated that models with the random effect grouping variables year and sampling station tended to fit the data much better than models without them (Supplementary Table 2). This suggested that maturity of skipjack tuna varies across time and space. The full model had the lowest LOOIC, suggesting the most parsimonious fit. The mean and standard deviation of the posterior distribution for each parameter of the full model are summarized in Table 2. Rhat values suggested that the model had converged. The thresholds (Table 2) describe the four cut points of the model applied to the maturity stage data and indicate where the continuous latent variables were partitioned to produce the observed responses. The estimated mean of the coefficient of FL indicated that fork length had a positive effect on maturity stage, and its 95% credible interval did not contain zero. The effect of FL does not tend to be different between the two types of school as indicated by the posterior distribution of the coefficient of the interaction between FL and school type.
Because school type is coded as a factor with associated school as the reference category, the coefficient of school type indicates the extent to which fish of free-swimming schools differ from those of associated schools on the latent scale maturity stage. The estimated mean indicates that there was a strong effect of school type on the maturity of skipjack tuna, i.e., the maturity of fish from free-swimming schools appeared to be higher than that of fish from associated schools (Table 2 and Figure 3). The 95% credible interval of this parameter was between 0.78 and 7.83, which did not contain zero. The evidence ratio suggested that the posterior probability of School>0 (i.e., free school has a positive effect on maturity) was 99%. We can therefore conclude with 99% probability that fish from free-swimming schools exist at a higher maturity stage than those associated with floating objects given the other covariates in the model. For any given fork length, K. pelamis from a free-swimming school are more likely to be in higher maturity stage than those associated with floating objects (Figure 3). For example, given a length of 50 cm, K. pelamis from free-swimming schools are more likely to be in mature stages (i.e., stages 4 and 5) than those from associated schools (Figures 4, 5).
Figure 3. Comparison of predicted maturity stage of Katsuonus pelamis based on fork length (FL) between the floating-object-associated and free-swimming school. The lines represent the means, and the shaded areas are their 95% credible interval.
Figure 4. Predicted probability of each of the five modeled maturity stages of 500 mm Katsuonus pelamis for floating-object-associated and free-swimming schools. Error bars indicate 95% credible intervals.
Figure 5. Predicted probability of each of the five modeled maturity stages of Katsuonus pelamis given fork length for floating-object-associated and free-swimming schools. The lines represent the means, and the shaded areas are their 95% credible interval.
Estimated maturity ogives were sensitive to the data used in the analyses. Excluding stage 6 fish in both ordinal and logistic regression analyses steepened the slope of the curve; proportion mature increased faster as fork length increased (Figure 6). This suggested that some of the fish in stage 3 (immature) were likely misidentified as stage 6 (mature). Therefore, the predicted proportion for small fish, e.g., 40 cm, was higher when stage 6 fish were included in the analysis. The ordinal and logistic regressions yielded very similar maturity ogives when using data without stage 6 fish. However, when stage 6 fish were included in the analyses, the logistic regression produced a higher proportion mature for large fish (e.g., >45 cm) compared with the ordinal regression. The logistic regression with full covariates (i.e., M0) did not detect a significant difference between the two school types (Supplementary Table 3).
Figure 6. Fitted maturity ogives based on ordinal and logistic regressions using data with and without stage 6 fish.
The GSI model results indicated a significant difference in GSI between the two schools, i.e., the point estimate of the coefficient of school type is 1.04 and its 95% credible interval did not contain zero (Table 3). The evidence ratio suggested that the posterior probability of School>0 (i.e., free school has a positive effect on GSI) is 99%. For a given fork length, fish from free-swimming schools have a larger GSI than those associated with floating objects (Figure 7). As expected, fork length had a significant effect on GSI. However, this effect is independent of school type, as the 95% credible interval of the coefficient of the interaction term between fork length and school type contained zero.
Figure 7. Comparison of predicted gonadosomatic index (GSI) of Katsuonus pelamis based on fork length (FL) between the floating-object-associated and free-swimming schools. The lines represent the means, and the shaded areas are their 95% credible interval.
In this study, we proposed a novel Bayesian multilevel ordinal regression model to model maturity ogives and applied it to K. pelamis in the WCPO to examine whether maturity characteristics of K. pelamis differs between two school types: schools associated with floating objects and free-swimming schools. The model results showed that there is a significant difference in maturity conditions between the two schools. K. pelamis from free-swimming school were more likely to have a higher maturity stage than those associated with floating objects given the same fork length. This was supported by the results of the GSI analysis, which also showed a significant difference in GSI between the two schools, i.e., that the GSI of K. pelamis in free-swimming school was significantly higher given the same fork length. Together, our results provide evidence that floating objects, including FAD, affect the maturity ogive of K. pelamis, and individuals in free-swimming schools may be maturing earlier. Therefore, it is important to account for such differences when estimating maturity ogive for stock assessment and management. Our results also suggest that estimates of maturity ogive are sensitive to maturity data as well as models used in the analysis. The conventional logistic regression model did not detect a significant difference in maturity between the two school types and could underestimate the L50 (length at which 50% of the fish are maturity), which leads to overestimation of maturity ogive, compared with the ordinal regression model.
A previous study also found that reproductive biology of Red Snapper in the Gulf of Mexico differs significantly between natural and artificial habitats (Glenn et al., 2017). Red Snapper at natural habitats may be maturing earlier than those at artificial habitats because they can afford to trade somatic growth for reproductive potential. The impact of floating objects on K. pelamis’s maturity could be a result of the negative impacts of FADs on the rates of food intake and the breeding strategy that K. pelamis utilize. Studies based on lipid and stable isotope analyses suggest that K. pelamis are income breeders (Grande et al., 2016). A income breeding strategy can adjust the reproductive schedule; for instance, the spawning season of K. pelamis in the western Indian Ocean matches with monsoon periods when food availability is high (Roger, 1994; Schott et al., 2002; Potier et al., 2008; Sardenne et al., 2016), and subsequent egg production of T. albacares increased in response to increasing daily rations (Margulies et al., 2007; Zudaire et al., 2015) relative to the current food availability. Studies have found that batch fecundity, egg size, and number of eggs spawned can change depending on the food availability (Ashida et al., 2017).
Aggregation around FADs is believed to decrease the food intake for tuna species (Ménard et al., 2000; Hallier and Gaertner, 2008). In all the specimens that we have examined for stomach contents during 2007–2014, the percentage of empty stomachs for K. pelamis from associated schools was 94.7% (1,566 out of 1,653). This was far higher than that from free-swimming schools, which was 29.6% (106 out of 358). Such a difference between the two school types has also been observed in other studies (Ménard et al., 2000; Potier et al., 2001; Hallier and Gaertner, 2008; Jaquemet et al., 2011). However, such a large difference in percentages might be due in part to the time of day when the fishing operations were conducted. The commercial fishing vessels targeted associated schools at dawn when the food in stomach had already been digested because tuna have exceptionally high digestion rates (Olson and Boggs, 1986), whereas the fishing vessels targeted unassociated tuna schools primarily during the day and often during their feeding time. Therefore, the percentage of empty stomachs in this case might not accurately reflect the difference in food intake between these two types of schools. Our results suggested that K. pelamis associated with floating objects need more time for gonad development. This is likely due to poorer feeding conditions where FADs are typically deployed. There is a tendency for some fishermen deploy FADs in areas where productivity is low (Bromhead, 2003) or using materials other than natural logs (Leroy et al., 2013).
One caveat of this study is the absence of data on residence time around FADs. For tropical tunas, some tagging experiments revealed that the residence time around individual FADs are short (i.e., 2–8 days) (Hilborn, 1991; Schaefer and Fuller, 2013; Matsumoto et al., 2014). However, the residence time is subject to a degree of variability depending on species and the region (Baidai et al., 2020). Using echosounder buoys data, Baidai et al. (2020) found that time span of tuna aggregations at FAD followed a time-independent process with short- and long-term residence modes, and FADs remained occupied for a large proportion of time after being colonized. Therefore, it is entirely possible that skipjack reside around FADs continuously or discontinuously for an adequate period of time that could affect their maturity.
Scientific studies have raised the concerns about the potential negative impacts of using FADs on the biology of tuna species (Ménard et al., 2000; Potier et al., 2001; Hallier and Gaertner, 2008; Jaquemet et al., 2011; Wang et al., 2012, 2014; Robert et al., 2014; Ashida et al., 2017). Our study also suggests that FADs could negatively affect the maturity of K. pelamis. From a management perspective, FADs may not only lead to growth overfishing but also reduce the population’s reproductive potential. Our results are consistent with previous studies. Ashida et al. (2017) compared the difference in reproductive traits of female K. pelamis between schools in the same area as this study. They found that the proportion of mature fish was significantly different between associated and unassociated schools. Although difference in size at maturity was not examined, they proposed that poorer food availability near FADs may impact oogenesis in K. pelamis. The difference in size at maturity may further confirm this speculation. They also concluded that inherent spawning characteristics (sex ratio, observed minimum size at first maturity, and spawning season) were not affected between schools. This is not surprising given that a FAD is more likely to affect food availability and consequently reduce reproduction.
Marine fisheries management is often based on biological reference points, which are predicted on an assumed relationship between stock reproductive potential and subsequent recruitment. The estimate of spawning stock biomass, which is based on maturity ogive, is often used to approximate reproductive potential. Therefore, imprecise or biased estimates of maturity ogive can lead to fishery management decisions based on mis-specified biological reference points. The findings of this study have important implications for skipjack tuna assessment and management. The estimated maturity ogive based on samples collected from either free school or associated school in isolation would likely to be biased. Our results also suggested that it is important to account for spatiotemporal variability when estimating the maturity ogive. Therefore, a comprehensive survey design that allows for representative samples to be collected is essential to estimate the maturity ogive for assessment and management use.
The ordinal regression approach proposed in this study fits maturity data obtained from both microscopic and macroscopic approaches naturally and can estimate the probability of being in each of the maturity stages. Thus, the maturity ogive can be derived by summing the probability across maturity stages that are considered mature. This allows for sensitivity analyses on maturity ogive in the assessments without refitting the regression model. Since the ordinal regression approach makes full use of the ordinality of the data, we hypothesize that this approach may be more robust to the misspecifications of maturity stages. However, this needs to be further tested. Therefore, future studies should evaluate the performance of ordinal regressions compared with conventional logistic regression on estimating maturity ogive, especially based on the data from a macroscopic method where misspecification errors are more likely to present, using a simulation approach. Finally, we envision that this ordinal regression approach could be applied more broadly in fisheries science when observations are taken as ordinal measurements, e.g., feeding levels.
The raw data supporting the conclusions of this article will be made available by the authors, without undue reservation.
The animal study was reviewed and approved by Academic Committee of Shanghai Ocean University.
JC and XW contributed to conception and design of the study. JC, XW, and CZ organized the database. JC performed the statistical analysis. JC, XW, and MD wrote the first draft of the manuscript. JC, XW, MD, and JZ wrote sections of the manuscript. All authors contributed to manuscript revision, read, and approved the submitted version.
This project was supported financially by the National key R&D Program of China (Project no. 2020YFD0901202) and the National Natural Science Foundation of China (No. 41506151).
The authors declare that the research was conducted in the absence of any commercial or financial relationships that could be construed as a potential conflict of interest.
All claims expressed in this article are solely those of the authors and do not necessarily represent those of their affiliated organizations, or those of the publisher, the editors and the reviewers. Any product that may be evaluated in this article, or claim that may be made by its manufacturer, is not guaranteed or endorsed by the publisher.
We would like to thank national data center for distant-water fisheries of China and captains and crew of tuna purse seiners “POHNPEI No.1,” “JINHUI No.6,” and “JINHUI No.7” for their help during the survey period.
The Supplementary Material for this article can be found online at: https://www.frontiersin.org/articles/10.3389/fmars.2021.736462/full#supplementary-material
Ashida, H., Tanabe, T., and Suzuki, N. (2017). Difference on reproductive trait of skipjack tuna Katsuonus pelamis female between schools (free vs FAD school) in the tropical western and central Pacific Ocean. Environ. Biol. Fishes 100, 935–945. doi: 10.1007/s10641-017-0621-2
Baidai, Y., Dagorn, L., Amandè, M. J., Gaertner, D., and Capello, M. (2020). Tuna aggregation dynamics at Drifting Fish Aggregating Devices: a view through the eyes of commercial echosounder buoys. ICES J. Mar. Sci. 77, 2960–2970. doi: 10.1093/icesjms/fsaa178
Bromhead, D. (2003). A Review of the Impact of Fish Aggregating Devices (FADs) on Tuna Fisheries. Canberra, ACT: Bureau of Rural Sciences.
Bürkner, P. C. (2017). brms: An R package for Bayesian multilevel models using Stan. J. Stat. Softw. 80, 1–28. doi: 10.18637/jss.v080.i01
Bürkner, P.-C., and Vuorre, M. (2019). Ordinal Regression Models in Psychology: A Tutorial. Adv. Methods Pract. Psychol. Sci. 2, 77–101. doi: 10.1177/2515245918823199
Dagorn, L., Holland, K. N., Restrepo, V., and Moreno, G. (2013). Is it good or bad to fish with FADs? What are the real impacts of the use of drifting FADs on pelagic marine ecosystems? Fish Fish. 14, 391–415. doi: 10.1111/j.1467-2979.2012.00478.x
Flores, A., Wiff, R., and Diáz, E. (2014). Using the gonadosomatic index to estimate the maturity ogive: Application to Chilean hake (Merluccius gayi gayi). ICES J. Mar. Sci. 72, 508–514. doi: 10.1093/icesjms/fsu155
Flores, A., Wiff, R., Ganias, K., and Marshall, C. T. (2019). Accuracy of gonadosomatic index in maturity classification and estimation of maturity ogive. Fish. Res. 210, 50–62. doi: 10.1016/j.fishres.2018.10.009
Fonteneau, A., Ariz, J., Gaertner, D., Nordstrom, V., and Pallares, P. (2000). Observed changes in the species composition of tuna schools in the Gulf of Guinea between 1981 and 1999, in relation with fish aggregating devices fishery. Aquat. Living Resour. 13, 253–257.
Glenn, H. D., Cowan, J. H., and Powers, J. E. (2017). A Comparison of Red Snapper Reproductive Potential in the Northwestern Gulf of Mexico: Natural versus Artificial Habitats. Mar. Coast. Fish. 9, 139–148. doi: 10.1080/19425120.2017.1282896
Grande, M., Murua, H., Zudaire, I., Arsenault-Pernet, E. J., Pernet, F., and Bodin, N. (2016). Energy allocation strategy of skipjack tuna Katsuwonus pelamis during their reproductive cycle. J. Fish Biol. 89, 2434–2448. doi: 10.1111/jfb.13125
Hallier, J., and Gaertner, D. (2008). Drifting fish aggregation devices could act as an ecological trap for tropical tuna species. Mar. Ecol. Prog. Ser. 353, 255–264. doi: 10.3354/meps07180
Hampton, J. (1993). Fishing for tunas associated with floating objects. Nouméa: South Pacific Commission.
Hedeker, D., and Gibbons, R. D. (1994). A Random-Effects Ordinal Regression Model for Multilevel Analysis. Biometrics 50:933. doi: 10.2307/2533433
Hilborn, R. (1991). Modeling the stability of fish schools: exchange of individual fish between schools of skipjack tuna (Katsuwonus pelamis). Can. J. Fish. Aquat. Sci. 48, 1081–1091. doi: 10.1139/f91-128
Hooten, M. B., and Hobbs, N. T. (2015). A guide to Bayesian model selection for ecologists. Ecol. Monogr. 85, 3–28. doi: 10.1890/14-0661.1
ISSF (2020). Status of the World Fisheries for Tuna. Nov. 2020. ISSF Technical Report 2020-16. Washington, DC: International Seafood Sustainability Foundation.
Jaquemet, S., Potier, M., and Ménard, F. (2011). Do drifting and anchored Fish Aggregating Devices (FADs) similarly influence tuna feeding habits? A case study from the western Indian Ocean. Fish. Res. 107, 283–290. doi: 10.1016/j.fishres.2010.11.011
King, J. R., and McFarlane, G. A. (2003). Marine fish life history strategies: applications to fishery management. Fish. Manag. Ecol. 10, 249–264. doi: 10.1046/j.1365-2400.2003.00359.x
Leroy, B., Phillips, J. S., Nicol, S., Pilling, G. M., Harley, S., Bromhead, D., et al. (2013). Aquatic Living Resources Review A critique of the ecosystem impacts of drifting and anchored FADs use by purse-seine tuna fisheries in the Western and Central Pacific Ocean. Aquat. Living Resour. 26, 49–61. doi: 10.1051/alr/2012033
Liu, X., O’Connell, A. A., and Koirala, H. (2011). Ordinal regression analysis: Predicting mathematics proficiency using the continuation ratio model. J. Mod. Appl. Stat. Methods 10, 513–527. doi: 10.22237/jmasm/1320120600
Margulies, D., Suter, J. M., Hunt, S. L., Olson, R. J., Scholey, V. P., Wexler, J. B., et al. (2007). Spawning and early development of captive yellowfin tuna (Thunnus albacares). Fish. Bull. 105, 249–266.
Marsac, F., Fonteneau, A., and Ménard, F. (2000). “Drifting FADs used in tuna fisheries: an ecological trap?,” in Pêche Thonière et Dispositifs de Concentration de Poisons, Vol. 28, eds J. Y. Le Gall, P. Cayré, and M. Taquet (Brest: Actes Colloques-IFREMER), 537–552.
Matsumoto, T., Satoh, K., and Toyonaga, M. (2014). Behavior of skipjack tuna (Katsuwonus pelamis) associated with a drifting FAD monitored with ultrasonic transmitters in the equatorial central Pacific Ocean. Fish. Res. 157, 78–85. doi: 10.1016/j.fishres.2014.03.023
Ménard, F., Stéquert, B., Rubin, A., Herrera, M., and Marchal, É (2000). Food consumption of tuna in the Equatorial Atlantic ocean: FAD-associated versus unassociated schools. Aquat. Living Resour. 13, 233–240. doi: 10.1016/S0990-7440(00)01066-4
Núñez, L. A., Hallfredsson, E. H., and Falk-Petersen, I. B. (2015). Different maturity scales affect estimations of fecundity, TEP and spawning stock size of Greenland halibut, Reinhardtius hippoglossoides (Walbaum, 1792). Mar. Biol. Res. 11, 824–833. doi: 10.1080/17451000.2015.1031797
Olson, R. J., and Boggs, C. H. (1986). Apex Predation by Yellowfïn Tuna (Thunnus albacares): Independent Estimates from Gastric Evacuation and Stomach Contents, Bioenergetics, and Cesium Concentrations. Can. J. Fish. Aquat. Sci. 43, 1760–1775. doi: 10.1139/f86-220
Potier, M., Romanov, E., Cherel, Y., Sabatié, R., Zamorov, V., and Ménard, F. (2008). Spatial distribution of Cubiceps pauciradiatus (Perciformes: Nomeidae) in the tropical Indian Ocean and its importance in the diet of large pelagic fishes. Aquat. Living Resour. 21, 123–134. doi: 10.1051/alr:2008026
Potier, M., Sabatié, R., and Ménard, F. (2001). Preliminary results of tuna diet studies in the West Equatorial Indian Ocean. IOTC Proc. 2001, 273–278.
Robert, M., Dagorn, L., Bodin, N., Pernet, F., Arsenault-Pernet, E.-J., and Deneubourg, J. L. (2014). Comparison of condition factors of skipjack tuna (Katsuwonus pelamis) associated or not with floating objects in an area known to be naturally enriched with logs. Can. J. Fish. Aquat. Sci. 71, 472–478. doi: 10.1139/cjfas-2013-0389
Roger, C. (1994). The plankton of the tropical western Indian ocean as a biomass indirectly supporting surface tunas (yellowfin, Thunnus albacares and skipjack, Katsuwonus pelamis). Environ. Biol. Fishes 39, 161–172. doi: 10.1007/BF00004934
Sardenne, F., Bodin, N., Chassot, E., Amiel, A., Fouché, E., Degroote, M., et al. (2016). Trophic niches of sympatric tropical tuna in the Western Indian Ocean inferred by stable isotopes and neutral fatty acids. Prog. Oceanogr. 146, 75–88. doi: 10.1016/j.pocean.2016.06.001
Schaefer, K. M., and Fuller, D. W. (2013). Simultaneous behavior of skipjack (Katsuwonus pelamis), bigeye (Thunnus obsesus), and yellowfin (T. albacares) tunas, within large multi-species aggregations associated with drifting fish aggregating devices (FADs) in the equatorial eastern Pacific Ocean. Mar. Biol. 160, 3005–3014. doi: 10.1007/s00227-013-2290-9
Schott, F. A., Dengler, M., and Schoenefeldt, R. (2002). The shallow overturning circulation of the Indian Ocean. Prog. Oceanogr. 53, 57–103. doi: 10.1016/S0079-6611(02)00039-3
Suzuki, Z., Miyabe, N., Ogura, M., Shono, S., and Uozumi, Y. (2003). Some important factors in controlling fishing capacity in tuna fisheries. Rome: FAO.
Vehtari, A., Gabry, J., Magnusson, M., Yao, Y., Bürkner, P., Paananen, T., et al. (2020). loo: Efficient Leave-One-Out Cross-Validation and WAIC for Bayesian Models. R Package Version 2.4.1. Available online at: https://mc-stan.org/loo/
Vehtari, A., Gelman, A., and Gabry, J. (2017). Practical Bayesian model evaluation using leave-one-out cross-validation and WAIC. Stat. Comput. 27, 1413–1432. doi: 10.1007/s11222-016-9696-4
Wagner, T., Hayes, D. B., and Bremigan, M. T. (2011). Accounting for Multilevel Data Structures in Fisheries Data using Mixed Models. Hoboken: Wiley.
Wang, X., Chen, Y., Truesdell, S., Xu, L., Cao, J., and Guan, W. (2014). The Large-Scale Deployment of Fish Aggregation Devices Alters Environmentally-Based Migratory Behavior of Skipjack Tuna in the Western Pacific Ocean. PLoS One 9:e98226. doi: 10.1371/journal.pone.0098226
Wang, X., Xu, L., Chen, Y., Zhu, G., Tian, S., and Zhu, J. (2012). Impacts of fish aggregation devices on size structures of skipjack tuna Katsuwonus pelamis. Aquat. Ecol. 46, 343–352. doi: 10.1007/s10452-012-9405-0
WCPFC (2019). “Overview of tuna fisheries in the western and central Pacific Ocean, including economic-2018,” in Proceedings of the 15th Regular Session of the Scientific Committee 12-20 August 2019, (Pohnpei: Western and Central Pacific Fisheries Commission).
Zudaire, I., Murua, H., Grande, M., Goñi, N., Potier, M., Ménard, F., et al. (2015). Variations in the diet and stable isotope ratios during the ovarian development of female yellowfin tuna (Thunnus albacares) in the Western Indian Ocean. Mar. Biol. 162, 2363–2377. doi: 10.1007/s00227-015-2763-0
Keywords: maturity ogive, Bayesian multilevel ordinal regression, drifting fish aggregation devices (FADs), Katsuonus pelamis, ordinal measurement
Citation: Cao J, Wang X, Damiano MD, Zhou C and Zhu J (2021) A Bayesian Multilevel Ordinal Regression Model for Fish Maturity Data: Difference in Maturity Ogives of Skipjack Tuna (Katsuwonus pelamis) Between Schools in the Western and Central Pacific Ocean. Front. Mar. Sci. 8:736462. doi: 10.3389/fmars.2021.736462
Received: 05 July 2021; Accepted: 13 October 2021;
Published: 01 December 2021.
Edited by:
Nancy Louise Shackell, Bedford Institute of Oceanography (BIO), CanadaReviewed by:
Jin Gao, Memorial University of Newfoundland, CanadaCopyright © 2021 Cao, Wang, Damiano, Zhou and Zhu. This is an open-access article distributed under the terms of the Creative Commons Attribution License (CC BY). The use, distribution or reproduction in other forums is permitted, provided the original author(s) and the copyright owner(s) are credited and that the original publication in this journal is cited, in accordance with accepted academic practice. No use, distribution or reproduction is permitted which does not comply with these terms.
*Correspondence: Xuefang Wang, eGZ3YW5nQHNob3UuZWR1LmNu
Disclaimer: All claims expressed in this article are solely those of the authors and do not necessarily represent those of their affiliated organizations, or those of the publisher, the editors and the reviewers. Any product that may be evaluated in this article or claim that may be made by its manufacturer is not guaranteed or endorsed by the publisher.
Research integrity at Frontiers
Learn more about the work of our research integrity team to safeguard the quality of each article we publish.