- 1Marine Biodiversity Group, Naturalis Biodiversity Center, Leiden, Netherlands
- 2Aquatic Ecology and Water Quality Management Group, Department of Environmental Sciences, Wageningen University, Wageningen, Netherlands
- 3Technical and Environmental Consulting Group, Servizi Sicurezza Lavoro, Siniscola, Italy
- 4National Research Council of Italy, Water Research Institute, Verbania, Italy
- 5Molecular Ecology and Evolution Group, School of Natural Sciences, Bangor University, Bangor, United Kingdom
- 6Centre of Molecular and Environmental Biology, University of Minho, Braga, Portugal
- 7Institute of Science and Innovation for Bio-Sustainability, University of Minho, Braga, Portugal
- 8Department of Ecosystem & Landscape Dynamics, Institute for Biodiversity & Ecosystem Dynamics, University of Amsterdam, Amsterdam, Netherlands
Meiofaunal animals, roughly between 0.045 and 1 mm in size, are ubiquitous and ecologically important inhabitants of benthic marine ecosystems. Their high species richness and rapid response to environmental change make them promising targets for ecological and biomonitoring studies. However, diversity patterns of benthic marine meiofauna remain poorly known due to challenges in species identification using classical morphological methods. DNA metabarcoding is a powerful tool to overcome this limitation. Here, we review DNA metabarcoding approaches used in studies on marine meiobenthos with the aim of facilitating researchers to make informed decisions for the implementation of DNA metabarcoding in meiofaunal biodiversity monitoring. We found that the applied methods vary greatly between researchers and studies, and concluded that further explicit comparisons of protocols are needed to apply DNA metabarcoding as a standard tool for assessing benthic meiofaunal community composition. Key aspects that require additional consideration include: (1) comparability of sample pre-treatment methods; (2) integration of different primers and molecular markers for both the mitochondrial cytochrome c oxidase subunit I (COI) and the nuclear 18S rRNA genes to maximize taxon recovery; (3) precise and standardized description of sampling methods to allow for comparison and replication; and (4) evaluation and testing of bioinformatic pipelines to enhance comparability between studies. By enhancing comparability between the various approaches currently used for the different aspects of the analyses, DNA metabarcoding will improve the long-term integrative potential for surveying and biomonitoring marine benthic meiofauna.
Introduction
Marine sediments harbor high, but understudied biodiversity (Snelgrove, 1999; Armonies et al., 2018; Hughes et al., 2021). They are inhabited by a highly diverse and abundant community (Giere, 2009; Balsamo et al., 2010; Appeltans et al., 2012; Sinniger et al., 2016), a substantial fraction of which is part of the benthic meiofauna. Meiofauna usually refers to organisms that pass through a 1 mm sieve but are retained on a sieve of approximately 45 μm mesh size, although no fixed size definition exists (Higgins and Thiel, 1988; Brannock and Halanych, 2015). The appropriateness of mesh sizes for meiofauna collection has been recently investigated, and an alternative minimum mesh size of 20 μm has been proposed (Ptatscheck et al., 2020). Furthermore, some authors refer to organisms with a great anatomical and physiological modification to interstitial life, thus covering a vast taxonomic variety (viz., Zeppilli et al., 2018). Indeed, meiobenthic species are found in at least 24 out of 35 described animal phyla (Giere, 2009; Balsamo et al., 2012) and are characterized by a high abundance of up to 106 individuals/m2, consisting of (amongst others) nematodes, copepods, gastrotrichs, and platyhelminthes (Higgins and Thiel, 1988; Giere, 2009). Meiobenthic animals affect nutrient cycling, microbial community structure, hydrodynamics, and are a link in food webs between microbial organisms and macrobenthos (Montagna, 1984; Coull, 1999; Schratzberger and Ingels, 2018). Meiofaunal species commonly have fast reproduction and turnover rates, allowing for rapid, but also heterogeneous responses to environmental changes. This makes them potentially valuable as bioindicators to evaluate the quality of marine ecosystems and perform environmental impact assessments, which is important in times of high anthropogenic pressure and climate change (Semprucci et al., 2015; Zeppilli et al., 2015).
Traditionally, meiofaunal communities are studied by morphological identification using light microscopy (Coull, 1999; Giere, 2009). However, light microscopy techniques are time consuming and often impeded by difficult identification of species, due to few (documented) distinctive morphological features and expert taxonomists (Fontaneto et al., 2009; Curini-Galletti et al., 2012). Recent advances in DNA-based methods allow for the evaluation of biodiversity at comparatively low costs, high speed, and on a larger scale compared to classic morphological studies (Bik et al., 2012a; Brannock and Halanych, 2015). In particular DNA metabarcoding (Taberlet et al., 2012a), meaning high-throughput amplicon sequencing targeting specific gene regions like the mitochondrial cytochrome c oxidase I (COI) or the ribosomal nuclear small subunit (nSSU) 18S gene, termed molecular barcodes, is increasingly used to explore meiofaunal community composition in a wide range of ecosystems (Guardiola et al., 2015; Fonseca et al., 2017; Wangensteen et al., 2018; Weigand and Macher, 2018; Müller et al., 2019; Fais et al., 2020a). In addition, meiofaunal community metabarcoding data can be applied in ecological monitoring, as has been done to assess ecosystem quality and recovery (Chariton et al., 2015; Cordier et al., 2017), short-term direct anthropogenic effects (Laroche et al., 2016; DiBattista et al., 2020; Martínez et al., 2020; He et al., 2021) and climate change (Leasi et al., 2021).
Although the potential of metabarcoding of meiofaunal communities for biodiversity and ecological studies has been demonstrated, currently used workflows differ among studies. Moreover, a consensus on an ideal metabarcoding approach for monitoring and assessing benthic meiofauna has not yet emerged. Each step in metabarcoding workflows is a potential source of bias in recovering community diversity and composition, as shown for example for the type of sample used for DNA extraction (i.e., whole sediment cores versus meiofauna isolated from sediment, Brannock and Halanych, 2015; Klunder et al., 2019; Pansu et al., 2021), sampling depth and design (Montagna et al., 2017; Nascimento et al., 2018; Fais et al., 2020b), target genes (Haenel et al., 2017; Cordier et al., 2019; Atienza et al., 2020; Laroche et al., 2020), primer pairs (Cowart et al., 2015; Fais et al., 2020b), and bioinformatic processing (Brannock and Halanych, 2015; Leasi et al., 2018; Antich et al., 2021). This implies that careful consideration and evaluation of the applied methods depending on the research question is crucial, and accurate reporting of adopted methodologies is important for better comparability between studies.
This review focuses on DNA metabarcoding of marine benthic meiofauna, which complements recently published reviews focusing on various types of bulk samples (van der Loos and Nijland, 2021) and freshwater meiofauna (Schenk and Fontaneto, 2020) by evaluating methods used specifically for marine meiofauna in bulk (isolated) as well as the sediment samples. Moreover, since the review on use of metagenetic tools for meiofauna by Carugati et al. (2015), a vast number of experimental studies has been published addressing the performance of specific steps of the meiofauna metabarcoding workflow, leading to new insight. The aim of this review is to assist researchers in selecting a DNA metabarcoding workflow for the study of marine benthic meiofauna by summarizing the currently used methodologies in different steps of the metabarcoding procedure, identifying limitations, and pointing out aspects of the metabarcoding workflow that should be carefully evaluated in future studies.
Methods
A search of scientific literature published between January 2010 and May 2021 was conducted within the Google Scholar database between March and May, 2021. Published articles were searched using terms that designate meiofaunal communities (i.e., “meiofauna,” “meiobenthos”) in marine benthic environments (i.e., “beach,” “sediment”) with or without the commonly used marker genes (i.e., “COI” and “18S”) in different combinations with the terms “marine” and “metabarcoding”. Search terms are disclosed in Supplementary Table 1.
Each paper was initially screened to confirm that the study included DNA metabarcoding of benthic meiofauna communities in marine environments (including estuarine and coastal areas). Publications on specific meiofaunal taxa (e.g., Nematoda) were excluded, as the applied methods (e.g., specimen extraction and taxa specific primer pairs) mostly cannot be applied to the study of whole meiofaunal communities. In studies on marine sediment environmental DNA (eDNA) or metabarcoding of complete benthic communities, the parts that focused on non-meiofaunal taxa were not further analyzed.
The information retrieved from each selected publication included: (1) the geographic area of the study; (2) the type of sampling sites; (3) the amount of sediment collected; (4) the number of replicates per sampling point; (5) the sampling instrument; (6) the depth of the layer of sampled sediment; (7) number of sliced layers; (8) the smallest sieve mesh size; (9) sample conservation method prior to DNA extraction; (10) the type of sample for DNA extraction; (11) the DNA extraction protocol, (12) amount of sediment for DNA extraction; (13) implementation of extraction controls; (14) number of extraction replicates; (14) the amplified marker gene(s) and gene region(s); (15) primer pairs; (16) number of PCR replicates; (17) implementation of PCR controls; (18) sequencing platform; (19) sequencing depth; (20) bioinformatic unit; (21) bioinformatic processing; and (22) reference database.
The geographic area was identified at the national level. The type of sampling site was identified according to four categories: estuary, intertidal, subtidal up to 200 m depth, or at depths of over 200 m. The amount of sediment collected per sampling site was reported in various units in different studies (volume, mass, and surface area). The amount of sediment was converted to mL by squaring the inner radius of the sediment core in cm and multiplying with π∗height, if reported. Amounts reported in other units (e.g., mass, surface area) were not converted as this could lead to imprecise estimates. The depth of the layer of sampled sediment was reported in cm starting from the surface sediment (0 cm depth). The type of the sample for DNA extraction was identified according to three categories: from sediment samples, from isolated meiofauna, or from extracellular DNA. Extraction of extracellular DNA was distinguished from extraction of sediment (total) DNA by the use of a saturated phosphate buffer protocol optimized for the recovery of extracellular DNA while avoiding organismal DNA (Taberlet et al., 2012b; Guardiola et al., 2015). The sieve mesh size was reported only for studies in which meiofauna was extracted from the sediment. As MoBio Laboratories was acquired by QIAGEN in 2015, data from identical DNA extraction kits that were first produced by MoBio was combined with QIAGEN data (e.g., MoBio PowerSoil kit and QIAGEN DNeasy PowerSoil kit). The bioinformatic unit was reported as the bins into which sequences are clustered based on their similarity (e.g., Operational Taxonomic Unit, Amplicon Sequence Variant). The bioinformatic processing was categorized according to the similarity threshold or pipeline used for delineation of the respective bioinformatic unit. We report sequencing depth as the total number of raw and quality filtered reads. All other variables were noted in the review as reported in the original paper, unless indicated otherwise. When authors did not mention certain methods, they were noted as “not reported”. Graphs were created using the ggplot2 package v3.3.3 (Wickham, 2016) in R v4.0.3 (R Core Team, 2020), Rstudio v1.3.1093 (RStudio Team, 2020).
Results
A total of 67 papers matched the criteria and were included in this review (see Supplementary Table 2 for a list of publications and metadata). The sum of studies (n) for different steps of the metabarcoding workflow sometimes exceeds the total number of analyzed studies, as some studies applied multiple methods. The first study on DNA metabarcoding of benthic meiofauna was published in 2010 (Creer et al., 2010), and the number of meiofaunal community metabarcoding studies increased over the last decade (Supplementary Figure 1).
The reviewed papers covered samples taken from estuarine (n = 12), intertidal (n = 12), subtidal (n = 26), or deep (n = 12) sediments, or a combination of the previous (n = 5, Supplementary Figure 2). Samples were usually collected with a grab (n = 11), corer (n = 23), box corer (n = 7), multicorer (n = 10), or directly into tubes or jars (n = 9). Directly comparing studies regarding the amount of sediment collected was often impossible due to differences in reported units. However, it is apparent that the amount of sediment that was collected per replicate varied greatly amongst studies. Differences of over 1,000-fold were found, ranging from 0.035 to 5 kg and 0.003 to 4.5 L per replicate. Most studies implement 2–5 sampling replicates (n = 49), whereas more than five replicates (n = 5) or one replicate (n = 9) were used less frequently. Approximately a third of studies analyzed collected sediment from the upper sediment layer to a depth of 2 cm or less (n = 20), n = 18 studies collected sediment up to a depth of 3–5 cm, n = 17 to a depth of 10 cm, and sediment cores deeper than 10 cm were used in n = 5 studies. Collected sediment was mostly examined as a whole (n = 64) rather than separately for two (n = 1) or three (n = 3) different depth layers. After sample collection, samples were conserved predominantly frozen (n = 40), in ethanol (n = 14, varying concentrations and temperatures) or in DESS (n = 8).
DNA was most often extracted directly from sediment samples (n = 40), followed by DNA extracted from meiofaunal organisms separated from the sediment (n = 29), or from isolated extracellular DNA (n = 4). n = 27 studies used between 8 and 10.5 g or 10 mL of sediment or meiofauna isolate for DNA extraction, whereas extractions from relatively small amounts of material (0.2–0.35 g) are common as well (n = 11). Amounts were not clearly reported in n = 17 studies. Isolation of meiofauna from sediment was mostly done by sieving (n = 5), or through decantation over a sieve using Ludox solution (n = 10, separation based on density) or the anesthetic MgCl2 (n = 5, Supplementary Figure 3). Sieve mesh widths ranged from 20 to 500 μm, with 45 μm being used most frequently (n = 15, Supplementary Figure 4).
DNA from sediment samples was primarily extracted using the commercial QIAGEN (previously MoBio) PowerSoil (n = 17) and PowerMax (n = 23) kits (Figure 1). DNA from isolated meiofaunal samples was mostly extracted with these kits as well, with the PowerSoil used more frequently (n = 13) than the PowerMax Soil kit (n = 7). Kits or protocols not specifically designed for sediment samples were used in n = 9 studies (e.g., QIAGEN QIAamp DNA Blood Maxi kit, n = 5). Sediment extracellular DNA was extracted with a saturated phosphate buffer protocol (Taberlet et al., 2012b), in combination with either a commercial DNA extraction kit (n = 3) or chloroform and isoamyl alcohol (IAA) protocol (n = 1). n = 12 studies report on the number of extraction replicates used, varying from one to ten extraction replicates. Negative DNA extraction controls (field controls or extraction blanks) were reported to be included in n = 16 studies, while positive controls (including DNA spiking or mock samples) were reported in n = 8 studies.
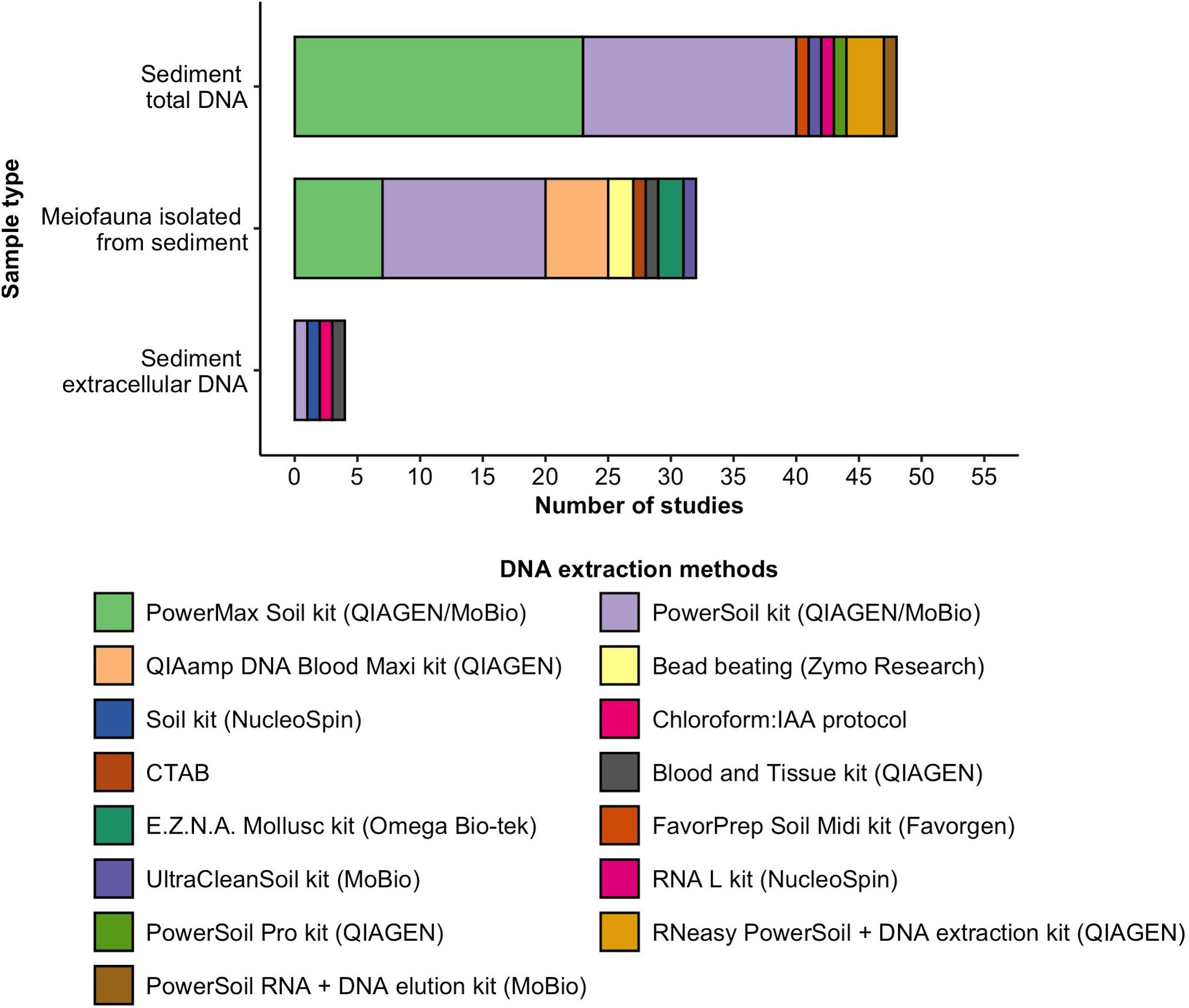
Figure 1. DNA extraction kits or protocols used in meiofaunal community metabarcoding studies for the extraction of DNA directly from sediment, isolated meiofauna, or sediment extracellular DNA.
In most studies, the researchers used only one target molecular region (n = 46, considering the different variable regions within the 18S rRNA gene as different targets), among which a region of the 18S rRNA gene was used in n = 45 studies and COI in only n = 1 study. Relatively fewer studies targeted two marker gene regions (n = 17), and the use of three marker gene regions (two variable regions of the 18S rRNA gene and COI) was rare (n = 4). Of the 18S rRNA variable regions, the V1–V2 region (n = 28) was the most frequently amplified metabarcoding fragment for meiobenthic communities, followed by V4 (n = 19), V9 (n = 16), V7 (n = 4), V4–V5 (n = 4), V1–V3 (n = 1), and V3–V4 (n = 1; Figure 2). All studies that targeted the 18S rRNA V1–V2 region used the primer pair SSU_F04 (forward) and SSU_R22 (reverse) either in its original form (n = 17, Blaxter et al., 1998; Fonseca et al., 2010) or with modifications in the forward or reverse primer (n = 11, Sinniger et al., 2016; Cordier et al., 2019; He et al., 2020). The V4 region was in most instances amplified with the TAReuk454FWD1 and TAReukREV3 primer combination (n = 12; Stoeck et al., 2010). The V9 region of the 18S rRNA gene was most commonly amplified with the primer combination 1380F + 1510R (n = 9, Amaral-Zettler et al., 2009), while the V7 region was amplified with the primer combination 18S_allshorts (n = 2, Guardiola et al., 2015) or nematode oriented NF1 and 18Sr2b (n = 2, Porazinska et al., 2009).
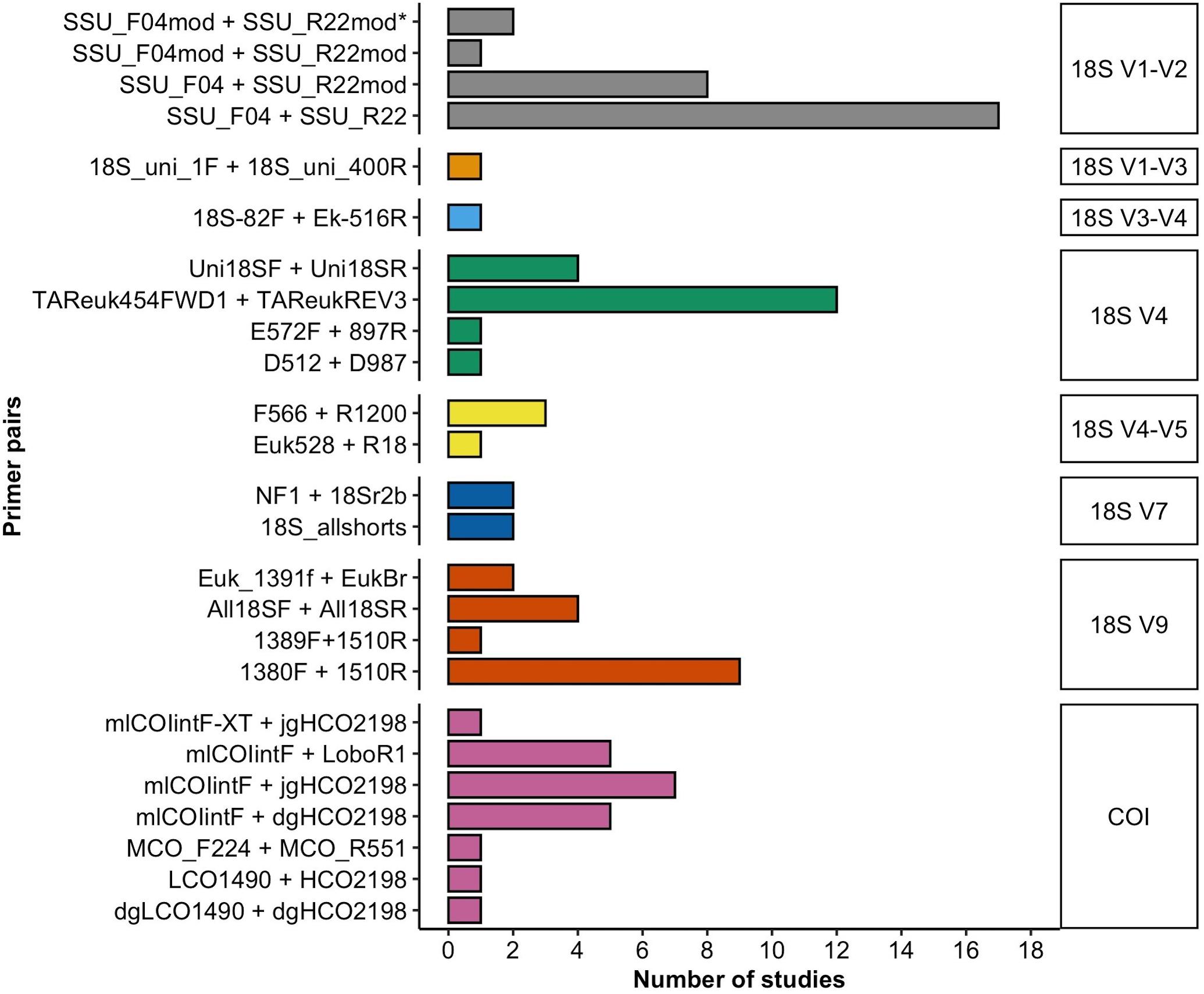
Figure 2. A total of 18 and 7 primer combinations were used that amplify the different variable regions of the 18S rRNA gene or partial segments of the 5′ end of the mitochondrial COI, respectively. Bars of primer pairs with a matching color target the identical molecular marker as indicated in the right margin of the figure.
Primers targeting the COI gene (n = 21) all amplify parts of the 5′ end of the gene, known as the Folmer region (Folmer et al., 1994), which is the standard molecular barcode for many animal taxa. A total of seven different primer combinations were used for the amplification of the COI fragment. In most cases, the mlCOIintF forward primer was combined with the reverse primer jgHCO2198 (n = 7, Leray et al., 2013), which amplifies the 313bp “mini-barcode region” in the 3′ of the Folmer fragment. The mlCOIintF primer in combination with the reverse primer LoboR1 (Lobo et al., 2013) was used less frequently (n = 5). Almost two thirds of studies used more than one replicate PCR (n = 40). Of these, PCRs were mostly performed in duplicates (n = 12) or triplicates (n = 25) to as many as eight replicates (n = 2). PCR negative controls (water only and no-template) were included in n = 34 studies.
Sequencing was mostly conducted on the Illumina MiSeq sequencing platform (n = 39), followed by Roche 454 pyrosequencing (n = 17; Supplementary Figure 5). Sequencing depth was found to be reported most commonly as the total number of raw reads obtained from sequencing, and the number of reads retained after quality filtering. As the total number of sequenced samples and replicates was not always reported, inferring sequencing depth per sample was often difficult. Therefore, we report the total number of reads per sample to illustrate the wide variation in sequencing depth. We found a range of approximately 125,000–61,000,000 total raw reads per marker, and 50,000–45,000,000 total quality filtered reads per marker. Subsequently, a variety of bioinformatic clustering methods and denoising tools were used to assign sequences into Operational Taxonomic Units (OTUs) or Amplicon Sequence Variants (ASVs; Supplementary Figure 6). OTU clustering (n = 52) was mostly performed using a cut-off similarity threshold (n = 45), with 97% genetic identity being the most commonly used threshold (n = 24), followed by 96% similarity (n = 7). Denoising and ASV formation was done using the DADA2 pipeline (n = 12, Callahan et al., 2016). In other studies, ASVs were subsequently clustered into OTUs using varying methods (n = 6) with a similarity threshold or with SWARM v2 (Mahé et al., 2015). Sequences were subsequently assigned to meiofaunal taxa using, for example, NCBI GenBank or nucleotide database (n = 22, Sayers et al., 2021), SILVA (n = 40, Pruesse et al., 2007), MIDORI (n = 6; Machida et al., 2017), or BOLD (n = 2, Ratnasingham and Hebert, 2007).
Discussion
We found a diverse array of methods in the metabarcoding workflow of meiobenthic community studies analyzed. Variance in metabarcoding procedures amongst studies is shared with other target communities, such as benthic macroinvertebrates (Duarte et al., 2021). Our results specifically identified discrepancies in pre-treatment of samples and DNA extraction techniques, selected marker genes and regions, preferred primer pairs, sequencing procedures, and bioinformatic processing of sequences. In the following sections, we discuss how bias may be potentially introduced within each of these steps, which conditions would favor the choice for a certain method over another, and indicate which aspects need careful consideration in future metabarcoding studies.
Sample Collection and Pre-treatment
Our results showed that the amount of sampled sediment varied greatly amongst studies and was often not reported clearly. The amount of sampled sediment may be important especially when DNA is extracted from isolated meiofaunal specimens or from a subsample of homogenized sediment and should thus be reported consistently in addition to the amount of sediment used for DNA extraction. In 92% of studies in which the sampling depth of the sediment layer was reported, the upper 10 cm of sediment or less was sampled, corresponding to the most biologically active part of the sediment in which meiofauna generally tends to concentrate (Thiel, 1983; Simpson and Batley, 2016).
Sample preservation is a critical step to avoid DNA degradation and was consistently reported in all but three studies. Our survey highlights that freezing or freeze-drying is the predominant method used, without fixatives such as ethanol or DESS. However, when a fixative was used this mostly comprised isolated meiofauna samples rather than sediment. Consistently, van der Loos and Nijland (2021) report that freezing is often used for sediment samples, and recommend the use of DESS over ethanol for bulk samples as it preserves both DNA and morphology with higher quality.
DNA extraction was most often performed directly from sediment samples compared to specimens isolated from sediment prior to DNA extraction. Compared to isolation of meiofauna, using sediment samples may be easier, it limits processing time, it avoids contamination, and it does not introduce any bias due to different methods of specimen isolation (Fonseca et al., 2018; Fais et al., 2020b). Our survey highlights that sediment samples are dominant in studies targeting environments hard to sample, such as the deep sea (e.g., Sinniger et al., 2016; Atienza et al., 2020; Kitahashi et al., 2020), and muddy and fine sediments difficult to elutriate (i.e., separating meiofauna from sediment by specific gravity using a liquid stream, Somerfield et al., 2005; viz., Lanzén et al., 2017; Faria et al., 2018; Salonen et al., 2019; Brandt et al., 2020). In addition, studies including a wider size range of benthic organisms such as micro- and macrofauna assessed through metabarcoding of sediment eDNA (e.g., Xie et al., 2017, 2018; Fais et al., 2020a,b; Klunder et al., 2020a,b) might have artificially raised the number of studies in which DNA was directly extracted from sediment samples. This method may lead to partially misleading results on the species composition present, as DNA in sediment can be recent or older extracellular DNA (Thomsen and Willerslev, 2015). This is especially relevant in deep sea environments, where extracellular DNA can constitute for 50–90% of the DNA, and ancient DNA can be very well conserved because of adsorption onto the sediment matrix (Torti et al., 2015). On the contrary, isolation of meiofaunal specimens from the sediment allows researchers to process a larger volume of sediment per sample, thereby possibly reducing sample heterogeneity and increasing recovery of rare species (Fonseca et al., 2010; Brannock and Halanych, 2015). Sieving reduces the dominance of large taxa (Cowart et al., 2015; Fonseca et al., 2018; He et al., 2020). Furthermore, elutriating or sieving samples was reported to result in a relatively high abundance of metazoan taxa while reducing the amplification of non-target bacterial or non-benthic taxa (Brannock and Halanych, 2015; He et al., 2020). When isolating meiofauna from the sediment, careful consideration is needed as soft-bodied organisms are easily damaged, whereas relatively heavy hard-bodied taxa such as mollusks may be retained in the sediment during elutriation, possibly leading to an underrepresentation of certain taxonomic groups (van der Loos and Nijland, 2021).
Recently, Castro et al. (2021) compared the recovered community composition from DNA extracted directly from sediment cores with two meiofauna extraction methods commonly used in metabarcoding studies, namely (1) separation of meiofaunal specimens from sediment by flotation and centrifugation with Ludox and (2) separation of meiofaunal specimens from sediment by decantation of specimens with MgCl2. The community composition recovered through direct DNA extraction from sediment differed strongly from that obtained with the isolation techniques (Castro et al., 2021). Isolation of specimens with Ludox or MgCl2 led to more taxa and meiofaunal species compared to DNA extraction directly from sediment (Castro et al., 2021). Differences were also found amongst isolation techniques, as some genera belonging to the phyla of platyhelminthes, annelids, mollusks, xenacoelomorpha, gastrotrichs, and nematodes were found in a lower abundance in samples obtained through MgCl2 decantation relative to Ludox flotation (Castro et al., 2021).
Hence, as sample type significantly affects both the OTU richness and taxonomic composition of meiofaunal communities (Brannock and Halanych, 2015; Haenel et al., 2017; Nascimento et al., 2018; Castro et al., 2021), a greater consideration should be given to the choice of sample and DNA extraction workflow. Indeed, any extraction method will lead to a bias toward taxonomic groups that are more easily isolated with the chosen technique. Therefore, if the goal is to maximize recovery of taxa, a combination of isolation methods is the best option (Castro et al., 2021).
DNA Extraction and PCR Procedures
We found that when DNA was extracted directly from sediment, researchers used kits specifically designed for soil samples, while these kits were used in only two-thirds of studies that extract DNA from meiofauna isolated from the sediment (Figure 1). Humic substances often present in sediments can be retained during DNA extraction and inhibit enzymes that amplify DNA during PCR, which can lead to false negatives (Matheson et al., 2010). Soil DNA extraction kits specifically remove these substances, thereby alleviating PCR biases caused by enzyme inhibition. Nonetheless, in earlier studies, clean isolated meiofauna samples and good amplification results were obtained using non-soil kits (e.g., Creer et al., 2010; Fonseca et al., 2010). More recently, it was recommended to use a soil-specific kit in samples that may contain remains of sediment to obtain high-purity DNA (van der Loos and Nijland, 2021), which also works well for marine and coastal sediments (Lekang et al., 2015; Taberlet et al., 2018; Fais et al., 2020b). The two most used kits in our analysis (i.e., MoBio/QIAGEN PowerSoil and PowerMax kit) differ in the amount of sediment that can be processed (a maximum of 0.25 and 10 g, respectively). Indeed, we found that in the analyzed studies DNA is mostly extracted from 5 to 10 g or 0.2 to 0.35 g, corresponding to the processing maxima of these kits. As some studies have shown that the number of meiofaunal taxa and OTUs increase with sample size where no elutriation steps are performed (Nascimento et al., 2018; Brandt et al., 2020; Fais et al., 2020b), 10 g of sediment is recommended for eDNA extraction. However, we point out that the QIAGEN PowerMax is costly (about 25€/USD per extraction), thereby limiting the number of samples that can be processed in many projects, as reflected in the number of extraction replicates found in this study. None of the studies that used the PowerMax kit used multiple extraction replicates, while these were reported using the PowerSoil kit (QIAGEN). Ultimately, several replicate DNA extractions and a lysis step are strongly suggested to improve OTU and taxa richness in studies on marine meiobenthos through DNA metabarcoding approaches (Lanzén et al., 2017). Additionally, it is recommended to perform at least three replicate PCR reactions to correct for the effects of PCR stochasticity and to potentially increase the number of OTUs and species detected (Leray and Knowlton, 2015; Bourlat et al., 2016; Alberdi et al., 2018), which was performed in less than half of the studies and not reported in 36% of studies analyzed here. Again, whether PCR replicates improve the results and outweigh the costs depends on the research goal (van der Loos and Nijland, 2021).
Even though negative extraction and PCR controls are essential control measures within metabarcoding, their use was reported in less than half of the studies analyzed here. Whether these controls were truly not implemented in the analyses, or this result only reflects a gap in reporting remains unknown, but either way this indicates an important point of improvement (van der Loos and Nijland, 2021). Field extraction controls (i.e., opening sample storing material at the sampling site without sample collection), no-template DNA extraction controls, no-template or water-only PCR controls all aim to identify potential contamination introduced in any step of the workflow and should therefore be processed and sequenced along the field samples and controlled for during bioinformatic quality filtering steps (e.g., Brandt et al., 2021a).
Choice of Marker Gene and Region
We found that the nuclear 18S rRNA molecular marker was the most commonly used, especially when only one marker region was amplified (n = 44 for 18S and n = 1 for COI). Most studies did not mention the reason behind marker region choice. Amongst the reasons for the choice of marker, sequencing length limitations of the Illumina platform was sometimes mentioned as a reason for selecting the 18S rRNA V9 region (Brannock et al., 2014, 2018; Brannock and Halanych, 2015). In other studies, 18S V4 was chosen over COI because this region is shorter and easier to PCR amplify and sequence using Illumina, and amplifies only eukaryotic sequences (Lejzerowicz et al., 2015; He et al., 2021). Furthermore, poor amplification results for marine nematodes with the COI Folmer primers in early barcoding studies (Derycke et al., 2010) have stimulated the use of 18S rRNA gene regions as genetic markers. The more preserved nature of 18S rRNA primer binding sites compared to COI allows for the amplification of a broader range of taxa (Schenk and Fontaneto, 2020). However, the relatively high conservation of 18S priming sites comes at the cost of resolution, often lacking the ability to discriminate at the species level (Tang et al., 2012).
Despite the extensive use of the 18S rRNA marker to study meiofauna, the mitochondrial COI gene is becoming more popular for meiofauna metabarcoding studies (28% of the studies analyzed in this review). Compared to 18S rRNA, the main advantage of COI is its high taxonomic resolution, often allowing for identification of species and even intraspecific variability (Tang et al., 2012; Cowart et al., 2015; Leray and Knowlton, 2016; Turon et al., 2020). Notwithstanding, meiofaunal species are often underrepresented in molecular reference databases (Sinniger et al., 2016; Curry et al., 2018; Wangensteen et al., 2018), leading to high percentages of uncharacterized OTUs. For example, 70.9% of the eukaryotic clusters remained unassignable at the phylum level in a deep-sea study by Atienza et al. (2020). However, it is important to consider that a high number of unassigned reads in COI datasets can be a result of non-target amplification of bacterial and other non-target species (Weigand and Macher, 2018). Therefore, both commonly used markers have their pitfalls and the choice of the most appropriate one depends on methodology, study system and research objective, as is true for all steps in the metabarcoding workflow. Using multiple molecular markers within a single study is advisable to maximize the recovery of meiofaunal taxa (Fais et al., 2020b and references therein), which is now implemented by less than a third of the studies analyzed. Furthermore, efforts should be undertaken to barcode individual species and place molecular references in barcode reference libraries to improve our ability to taxonomically assign metabarcoding data (Wangensteen et al., 2018; Weigand et al., 2019).
Selection of Primer Pairs
Optimal primer pairs for amplification of whole meiofaunal communities ideally amplify a broad range of meiofaunal animals while minimizing off-target amplification. Ideally, extensive reference databases containing sequences for this marker should be available to allow for high taxonomic resolution. Our data showed that a total of 22 different primer combinations were used in the studies analyzed in this review. This high number can be partly explained by studies that test multiple primer pairs, but it also reflects that a consensus on the optimal primers for amplification of marine meiofaunal communities has not been reached. Primer choice is important as primer bias due to mismatches affects the relative abundance of amplicons and species recovery (Elbrecht and Leese, 2015). Thus, conserved sequences facilitate universal primer design. As such, the SSU_F04 forward and SSU_R22 reverse primer set (Blaxter et al., 1998; Fonseca et al., 2010) targeting the 18S rDNA V1-V2 region is highly conserved and amplifies a broad range of meiofaunal organisms (Creer et al., 2010). In meiofaunal taxa, the V1–V2 region amplified by these primers is the most variable nSSU region and its length of 450 bp favors amplification of DNA from living organisms (Lallias et al., 2015), benefiting recovery of present meiofaunal communities. Further modifications, such as shortening and including an ambiguity (R) into the SSU_R22 reverse primer to accommodate the inclusion of more metazoans (called SSU_R22mod, Sinniger et al., 2016), can be used to target specific groups of taxa within meiofauna.
After 18S rRNA V1–V2, the V4 and V9 were commonly used to amplify eukaryotes. The 18S rRNA V4 region is the most variable region in eukaryotes (Nickrent and Sargent, 1991). Indeed, the 18S V4 primer pairs are designed to amplify a broad range of eukaryotic taxa [F566 and R1200 by Hadziavdic et al. (2014); Uni18S and Uni18R by Zhan et al. (2013); E572F and 897R by Comeau et al. (2011) and Hugerth et al. (2014)] or eukaryotic microbes [TAReuk454FWD1 and TAReukREV3 by Stoeck et al. (2010)] instead of meiofauna taxa specifically. The same goes for the eukaryotic primer set 1380F and 1510R targeting the V9 region (Amaral-Zettler et al., 2009). The short (100–110 bp) regions that are amplified using the 18S_allshorts primer set targeting 18S rRNA V7 are suitable for amplifying extracellular DNA due to its length, however limiting meiofaunal identification at species level (Guardiola et al., 2015, 2016).
The 658-bp fragment amplified by the original primer pair for the mitochondrial COI gene (LCO1490 and HCO2198, Folmer et al., 1994) is too long for commonly used Illumina sequencing platforms. The shorter mlCOIintF and dgHCO2198 (Meyer, 2003; Leray et al., 2013) and mlCOIintF and jgHCO2198 (Geller et al., 2013; Leray et al., 2013) are most commonly used for amplification of marine meiobenthos. However, the degenerate LoboR1 primer (Lobo et al., 2013) is a promising reverse primer in combination with the mlCOIintF forward primer, as it uncovered a higher diversity compared to the degenerate reverse primer dgHCO2198 (Haenel et al., 2017). This combination was used and recommended in several meiofaunal community studies (Haenel et al., 2017; Fais et al., 2020a,b; Castro et al., 2021). Additionally, inosine bases present in the widely used jgHCO2198 reverse primer may impair the performance of high-fidelity polymerases commonly used for PCR amplification in metabarcoding studies (Jungbluth et al., 2020). For analyses of meiobenthic communities extracted from the sediment, using highly degenerate primers that amplify the maximum number of taxa, such as the Leray and Lobo primers (Meyer, 2003; Leray et al., 2013; Lobo et al., 2013), may be beneficial. On the other hand, these primers can be suboptimal for amplification and sequencing of DNA extracted directly from sediment, as the primers can amplify a wide range of non-target microbial taxa abundantly present in the sediment preventing detection of target taxa (Alberdi et al., 2018; Weigand and Macher, 2018). Alternative methods such as elutriation or sieving, as suggested before, could reduce non-target amplification. Alternatively, less degenerate, more specific primers can be beneficial, at the cost of potentially losing some target taxa.
Consequently, no optimal primer pair is currently available for marine benthic meiofauna. To increase comparability between studies, researchers should aim to narrow down the amount of different primer combinations that are used in meiofaunal community studies, while considering the best primer pairs for their research objectives.
Sequencing
Primer pair selection eventually affects the choice of sequencing platform, and vice versa. Illumina MiSeq is currently the main sequencing platform used in meiofaunal community DNA metabarcoding studies and can produce 2 × 300 bp long reads, rendering the use of primer pairs that generate longer amplicons unfeasible. The 454 pyrosequencing platform was identified as the main sequencing platform in Carugati et al. (2015). In our review, we found that this platform was mainly used in older papers, as Roche discontinued 454 platform production in 2013 and support by 2016. It was recently shown that Illumina’s NovaSeq 6000 outcompetes the MiSeq platform in a seawater eDNA metabarcoding study, where NovaSeq detected a higher diversity at the same sequencing depth. Moreover, where the number of new sequence variants detected by the MiSeq platform leveled off at approximately one million reads, data from NovaSeq did not show such a plateau (Singer et al., 2019). At ten times the costs of MiSeq sequencing, however, the NovaSeq 6000 platform may be inaccessible for most studies (Singer et al., 2019) and was indeed not used in the studies analyzed in this review. However, costs might decrease and the platform, which can produce 2 × 250 bp long reads, might become a cost efficient option for future metabarcoding studies. In contrast, the popular Illumina MiSeq platform has relatively low costs per sequenced base pair. Sequencing techniques such as PacBio and Nanopore allow sequencing of longer amplicons (Callahan et al., 2019) and have been successfully used for metabarcoding of environmental samples (Heeger et al., 2018; Karst et al., 2018; Davidov et al., 2020; Okazaki et al., 2021). Even though trade-offs regarding the higher error rate and read coverage compared to Illumina short-read sequencing exist, longer reads comprising several genes may provide better taxonomic resolution due to the high informational content per read (Weirather et al., 2017; Baloğlu et al., 2021). To our knowledge, these sequencing platforms have not been used in marine meiofaunal metabarcoding studies and might thus be valuable options for future studies (Hebert et al., 2018).
Diversity detection increases with sequencing depth in metabarcoding studies, as shown for different target taxa (e.g., Smith and Peay, 2014; Lanzén et al., 2017; Alberdi et al., 2018). Sequencing depth increases the number of unique OTUs per PCR replicate, and thereby decreases similarity between PCR replicates (Alberdi et al., 2018). If a study aims to identify the whole meiofaunal diversity, a high sequencing depth is crucial. However, for general monitoring purposes deep sequencing depth may not be required. Nonetheless, a sufficient sequencing depth should be considered fitting the purpose of the study. Singer et al. (2019) inferred sequencing depth of 10 metabarcoding studies using DNA from various sources and found that an average sequencing depth of 55,000–60,000 reads per sample is common. Indeed, they also found that sequencing depth is often not reported clearly. Similar to the use of highly degenerate primers, detection of more species comes at the cost of an increasing number of artifactual or non-target sequences and should thus be considered during bioinformatic processing (Alberdi et al., 2018; Weigand and Macher, 2018).
Bioinformatic Approach
It has been shown by multiple studies that bioinformatic techniques affect recovered diversity (Brannock and Halanych, 2015; Leasi et al., 2018; Antich et al., 2021). We found a total of 23 different bioinformatic approaches used in studies on marine benthic meiofauna, which can potentially render comparison of results between studies unfeasible. The clustering methods (i.e., combining sequences into representative units such as OTUs or MOTUs) analyzed in this study are mostly based on either Bayesian methods (CROP; Hao et al., 2011), single linkage clustering (SWARM; Mahé et al., 2015), or similarity thresholds. The latter was most commonly applied in the reviewed studies, often with a cut-off value of 97% similarity. Denoising approaches without clustering (i.e., rectifying sequencing errors by merging sequences containing an error with the true sequence) as done in DADA2 (Callahan et al., 2016) or UNOISE (Edgar, 2016) pipelines, yields ASVs or zero-radius OTUs (ZOTUs), which allow studying intraspecific diversity. Similarly, but not widely implemented in meiofauna research, the Deblur pipeline (Amir et al., 2017) obtains single-nucleotide resolution and produces comparable weighted results to DADA2 and UNOISE2 (Nearing et al., 2018) and could be valuable for identification at (sub)species level.
Currently, the debate whether OTUs or ASVs are more appropriate for analyzing metabarcoding data is ongoing. It is argued that ASVs, due to their higher resolution and reusability across studies, should be the standard reporting unit (Callahan et al., 2017), but other researchers argue that clustering is crucial in more variable markers such as COI to conform to the biological species concept (Antich et al., 2021; Brandt et al., 2021b). Furthermore, denoising and clustering programs that were originally developed for rRNA (e.g., CROP, SWARM, DADA2, UNOISE) are now commonly used for the mitochondrial COI gene (Antich et al., 2021). Using these programs on sequences from variable markers such as COI demands for a critical consideration of parameter settings as done for SWARM2 (Turon et al., 2020; Brandt et al., 2021b) and CROP (Leray et al., 2013; Leray and Knowlton, 2015; Wangensteen et al., 2018). However, not all studies provide the used parameter settings, implying that the default settings were used without further consideration (Antich et al., 2021).
It has been pointed out in many studies that taxonomic assignment of recovered sequences is hampered by significant gaps in reference databases (Sinniger et al., 2016; Curry et al., 2018; Wangensteen et al., 2018; Weigand and Macher, 2018), leading to difficulties especially for the interpretation of metabarcoding data at lower taxonomic levels. The COI gene tends to be more variable than rRNA genes like 18S, meaning that for reliable identification on a low taxonomic level, representatives of the species or at least closely related species have to be present in a reference database (Leray and Knowlton, 2016). Moreover, databases are often limited to few genetic markers (e.g., SILVA, which contains rRNA sequences, BOLD, which is focused on COI), contain many sequences that are not openly available and may contain erroneous or wrongly assigned sequences (e.g., Radulovici et al., 2021). Combining multiple reference databases could therefore improve the number and accuracy of OTUs assigned within a study (Macher et al., 2017). However, this was only implemented by a limited number of studies analyzed here. Almost two-third of studies use the ribosomal RNA sequence database SILVA (Pruesse et al., 2007) for taxonomic assignments, corresponding to 18S being the most used marker in meiobenthic metabarcoding studies. Surprisingly, BOLD was only used in n = 2 studies. Studies targeting COI tend to rely more on GenBank, as some taxonomic groups, including annelids and mollusks, are better represented in GenBank compared to BOLD (Weigand et al., 2019).
Overall, implementation of a mock sample with known species composition could confirm the reliability and accuracy of the metabarcoding workflow, including bioinformatic processing, and can be used not only to verify the detection of mock species but also control for overestimation of OTUs. Of the studies reviewed, only n = 6 studies (e.g., Chariton et al., 2015; Klunder et al., 2019; Brandt et al., 2020) included a mock community, and routinely sequencing such a control sample might be beneficial for future studies.
Conclusion
We found that a consensus on optimal methods for metabarcoding of marine meiofaunal sediment communities has not yet emerged, making comparison of study results difficult or impossible. We identified several key aspects that should receive attention in future studies on marine meiobenthos: (1) comparison of sampling methods, i.e., direct extraction of DNA from sediment versus separation of specimens from the sediment, followed by DNA extraction; (2) comparison of different primers and multiple molecular marker regions to maximize taxon recovery; (3) all steps of the sampling and laboratory procedures should be accurately described to allow for replication and better comparability of experiments; and (4) bioinformatic approaches for processing data and assigning species names to sequences should be thoroughly compared and evaluated. Considering the vast number of improvements that have been made over the last decade, we believe metabarcoding will further develop into an accurate and efficient standard tool to monitor marine meiobenthos in the coming decade.
Author Contributions
RG designed and performed the research, analyzed data, and drafted the work. J-NM designed the research, interpreted data, and drafted and revised the work. MF, DF, WR, SC, and FC drafted and revised the work. All authors contributed to the article and approved the submitted version.
Funding
This work was funded by a BEN (Biodiversity-Ecology-Nature) grant (Number T0206/37197/2021/kg) of the Bauer-Hollmann foundation to J-NM.
Conflict of Interest
The authors declare that the research was conducted in the absence of any commercial or financial relationships that could be construed as a potential conflict of interest.
Publisher’s Note
All claims expressed in this article are solely those of the authors and do not necessarily represent those of their affiliated organizations, or those of the publisher, the editors and the reviewers. Any product that may be evaluated in this article, or claim that may be made by its manufacturer, is not guaranteed or endorsed by the publisher.
Acknowledgments
We thank the Naturalis Marine Biodiversity Group for support and helpful comments and two reviewers for their suggestions.
Supplementary Material
The Supplementary Material for this article can be found online at: https://www.frontiersin.org/articles/10.3389/fmars.2021.730063/full#supplementary-material
References
Alberdi, A., Aizpurua, O., Gilbert, M. T. P., and Bohmann, K. (2018). Scrutinizing key steps for reliable metabarcoding of environmental samples. Methods Ecol. Evol. 9, 134–147. doi: 10.1111/2041-210X.12849
Amaral-Zettler, L. A., McCliment, E. A., Ducklow, H. W., and Huse, S. M. (2009). A method for studying protistan diversity using massively parallel sequencing of V9 hypervariable regions of small-subunit ribosomal RNA genes. PLoS One 4:e6372. doi: 10.1371/journal.pone.0006372
Amir, A., McDonald, D., Navas-Molina, J. A., Kopylova, E., Morton, J. T., Xu, Z. Z., et al. (2017). Deblur rapidly resolves single-nucleotide community sequence patterns. mSystems 2:e191-16. doi: 10.1128/mSystems.00191-16
Antich, A., Palacin, C., Wangensteen, O. S., and Turon, X. (2021). To denoise or to cluster, that is not the question: optimizing pipelines for COI metabarcoding and metaphylogeography. BMC Bioinformatics 22:177. doi: 10.1186/s12859-021-04115-6
Appeltans, W., Ahyong, S. T., Anderson, G., Angel, M. V., Artois, T., Bailly, N., et al. (2012). The magnitude of global marine species diversity. Curr. Biol. 22, 2189–2202. doi: 10.1016/j.cub.2012.09.036
Armonies, W., Asmus, H., Buschbaum, C., Lackschewitz, D., Reise, K., and Rick, J. (2018). Microscopic species make the diversity: a checklist of marine flora and fauna around the Island of Sylt in the North Sea. Helgol. Mar. Res. 72:11. doi: 10.1186/s10152-018-0512-8
Atienza, S., Guardiola, M., Præbel, K., Antich, A., Turon, X., and Wangensteen, O. S. (2020). DNA metabarcoding of deep-sea sediment communities using COI: community assessment, spatio-temporal patterns and comparison with 18S rDNA. Diversity 12:123. doi: 10.3390/d12040123
Baloğlu, B., Chen, Z., Elbrecht, V., Braukmann, T., MacDonald, S., and Steinke, D. (2021). A workflow for accurate metabarcoding using nanopore MinION sequencing. Methods Ecol. Evol. 12, 794–804. doi: 10.1111/2041-210X.13561
Balsamo, M., Albertelli, G., Ceccherelli, V., Coccioni, R., Colangelo, M., Curini-Galletti, M., et al. (2010). Meiofauna of the adriatic sea: present knowledge and future perspectives. Chem. Ecol. 26, 45–63.
Balsamo, M., Semprucci, F., Frontalini, F., and Coccioni, R. (2012). “Meiofauna as a tool for marine ecosystem biomonitoring,” in Marine Ecosystems, ed. A. Cruzado (London: IntechOpen), 77–104.
Bik, H. M., Porazinska, D. L., Creer, S., Caporaso, J. G., Knight, R., and Thomas, W. K. (2012a). Sequencing our way towards understanding global eukaryotic biodiversity. Trends Ecol. Evol. 27, 233–243.
Blaxter, M. L., De Ley, P., Garey, J. R., Liu, L. X., Scheldeman, P., Vierstraete, A., et al. (1998). A molecular evolutionary framework for the phylum Nematoda. Nature 392, 71–75.
Bourlat, S. J., Haenel, Q., Finnman, J., and Leray, M. (2016). “Preparation of amplicon libraries for metabarcoding of marine eukaryotes using Illumina MiSeq: the dual-PCR method,” in Marine Genomics: Methods and Protocols, ed. S. J. Bourlat (New York, NY: Springer New York), 197–207.
Brandt, M. I., Trouche, B., Henry, N., Liautard-Haag, C., Maignien, L., de Vargas, C., et al. (2020). An assessment of environmental metabarcoding protocols aiming at favoring contemporary biodiversity in inventories of deep-sea communities. Front. Mar. Sci. 7:234. doi: 10.3389/fmars.2020.00234
Brandt, M. I., Pradillon, F., Trouche, B., Henry, N., Liautard-Haag, C., Cambon-Bonavita, M.-A., et al. (2021a). Evaluating sediment and water sampling methods for the estimation of deep-sea biodiversity using environmental DNA. Sci. Rep. 11:7856.
Brandt, M. I., Trouche, B., Quintric, L., Günther, B., Wincker, P., Poulain, J., et al. (2021b). Bioinformatic pipelines combining denoising and clustering tools allow for more comprehensive prokaryotic and eukaryotic metabarcoding. Mol. Ecol. Resour. 21, 1904–1921.
Brannock, P. M., and Halanych, K. M. (2015). Meiofaunal community analysis by high-throughput sequencing: comparison of extraction, quality filtering, and clustering methods. Mar. Genomics 23, 67–75.
Brannock, P. M., Learman, D. R., Mahon, A. R., Santos, S. R., and Halanych, K. M. (2018). Meiobenthic community composition and biodiversity along a 5500 km transect of Western Antarctica: a metabarcoding analysis. Mar. Ecol. Prog. Ser. 14, 47–60.
Brannock, P. M., Waits, D. S., Sharma, J., and Halanych, K. M. (2014). High-throughput sequencing characterizes intertidal meiofaunal communities in Northern Gulf of Mexico (Dauphin Island and Mobile Bay, Alabama). Biol. Bull. 227, 161–174. doi: 10.1086/BBLv227n2p161
Callahan, B. J., McMurdie, P. J., and Holmes, S. P. (2017). Exact sequence variants should replace operational taxonomic units in marker-gene data analysis. ISME J. 11, 2639–2643. doi: 10.1038/ismej.2017.119
Callahan, B. J., McMurdie, P. J., Rosen, M. J., Han, A. W., Johnson, A. J. A., and Holmes, S. P. (2016). DADA2: high-resolution sample inference from Illumina amplicon data. Nat. Methods 13, 581–583.
Callahan, B. J., Wong, J., Heiner, C., Oh, S., Theriot, C. M., Gulati, A. S., et al. (2019). High-throughput amplicon sequencing of the full-length 16S rRNA gene with single-nucleotide resolution. Nucleic Acids Res. 47:e103. doi: 10.1093/nar/gkz569
Carugati, L., Corinaldesi, C., Dell’Anno, A., and Danovaro, R. (2015). Metagenetic tools for the census of marine meiofaunal biodiversity: an overview. Mar. Genomics 24, 11–20. doi: 10.1016/j.margen.2015.04.010
Castro, L. R., Meyer, R. S., Shapiro, B., Shirazi, S., Cutler, S., Lagos, A. M., et al. (2021). Metabarcoding meiofauna biodiversity assessment in four beaches of Northern Colombia: effects of sampling protocols and primer choice. Hydrobiologia 848, 3407–3426. doi: 10.1007/s10750-021-04576-z
Chariton, A. A., Stephenson, S., Morgan, M. J., Steven, A. D. L., Colloff, M. J., Court, L. N., et al. (2015). Metabarcoding of benthic eukaryote communities predicts the ecological condition of estuaries. Environ. Pollut. 203, 165–174. doi: 10.1016/j.envpol.2015.03.047
Comeau, A. M., Li, W. K. W., Tremblay, J. -É, Carmack, E. C., and Lovejoy, C. (2011). Arctic ocean microbial community structure before and after the 2007 record sea ice minimum. PLoS One 6:e27492. doi: 10.1371/journal.pone.0027492
Cordier, T., Esling, P., Lejzerowicz, F., Visco, J., Ouadahi, A., Martins, C., et al. (2017). Predicting the ecological quality status of marine environments from eDNA metabarcoding data using supervised machine learning. Environ. Sci. Technol. 51, 9118–9126.
Cordier, T., Frontalini, F., Cermakova, K., Apothéloz-Perret-Gentil, L., Treglia, M., Scantamburlo, E., et al. (2019). Multi-marker eDNA metabarcoding survey to assess the environmental impact of three offshore gas platforms in the North Adriatic Sea (Italy). Mar. Environ. Res. 146, 24–34. doi: 10.1016/j.marenvres.2018.12.009
Coull, B. C. (1999). Role of meiofauna in estuarine soft-bottom habitats. Aust. J. Ecol. 24, 327–343.
Cowart, D. A., Pinheiro, M., Mouchel, O., Maguer, M., Grall, J., Miné, J., et al. (2015). Metabarcoding is powerful yet still blind: a comparative analysis of morphological and molecular surveys of seagrass communities. PLoS One 10:e0117562. doi: 10.1371/journal.pone.0117562
Creer, S., Fonseca, V. G., Porazinska, D. L., Giblin-Davis, R. M., Sung, W., Power, D. M., et al. (2010). Ultrasequencing of the meiofaunal biosphere: practice, pitfalls and promises. Mol. Ecol. 19, 4–20. doi: 10.1111/j.1365-294X.2009.04473.x
Curini-Galletti, M., Artois, T., Delogu, V., De Smet, W. H., Fontaneto, D., Jondelius, U., et al. (2012). Patterns of diversity in soft-bodied meiofauna: dispersal ability and body size matter. PLoS One 7:e33801. doi: 10.1371/journal.pone.0033801
Curry, C. J., Gibson, J. F., Shokralla, S., Hajibabaei, M., and Baird, D. J. (2018). Identifying North American freshwater invertebrates using DNA barcodes: are existing COI sequence libraries fit for purpose? Freshw. Sci. 37, 178–189.
Davidov, K., Iankelevich-Kounio, E., Yakovenko, I., Koucherov, Y., Rubin-Blum, M., and Oren, M. (2020). Identification of plastic-associated species in the Mediterranean Sea using DNA metabarcoding with Nanopore MinION. Sci. Rep. 10:17533. doi: 10.1038/s41598-020-74180-z
Derycke, S., Vanaverbeke, J., Rigaux, A., Backeljau, T., and Moens, T. (2010). Exploring the use of cytochrome oxidase c subunit 1 (COI) for DNA barcoding of free-living marine nematodes. PLoS One 5:e13716. doi: 10.1371/journal.pone.0013716
DiBattista, J. D., Reimer, J. D., Stat, M., Masucci, G. D., Biondi, P., De Brauwer, M., et al. (2020). Environmental DNA can act as a biodiversity barometer of anthropogenic pressures in coastal ecosystems. Sci. Rep. 10:8365. doi: 10.1038/s41598-020-64858-9
Duarte, S., Leite, B. R., Feio, M. J., Costa, F. O., and Filipe, A. F. (2021). Integration of DNA-based approaches in aquatic ecological assessment using benthic macroinvertebrates. Water 13:331.
Edgar, R. C. (2016). UNOISE2: improved error-correction for Illumina 16S and ITS amplicon sequencing. BioRxiv [Preprint]. doi: 10.1101/081257
Elbrecht, V., and Leese, F. (2015). Can DNA-based ecosystem assessments quantify species abundance? Testing primer bias and biomass—sequence relationships with an innovative metabarcoding protocol. PLoS One 10:e0130324. doi: 10.1371/journal.pone.0130324
Fais, M., Bellisario, B., Duarte, S., Vieira, P. E., Sousa, R., Canchaya, C., et al. (2020a). Meiofauna metabarcoding in Lima estuary (Portugal) suggests high taxon replacement within a background of network stability. Reg. Stud. Mar. Sci. 38:101341.
Fais, M., Duarte, S., Vieira, P. E., Sousa, R., Hajibabaei, M., Canchaya, C. A., et al. (2020b). Small-scale spatial variation of meiofaunal communities in Lima estuary (NW Portugal) assessed through metabarcoding. Estuar. Coast. Shelf Sci. 238:106683. doi: 10.1016/j.ecss.2020.106683
Faria, L. C. D., Di Domenico, M., Andrade, S. C. S., Santos, M. C. D., Fonseca, G., Zanol, J., et al. (2018). The use of metabarcoding for meiofauna ecological patterns assessment. Mar. Environ. Res. 140, 160–168. doi: 10.1016/j.marenvres.2018.06.013
Folmer, O., Black, M., Hoeh, W., Lutz, R., and Vrijenhoek, R. (1994). DNA primers for amplification of mitochondrial cytochrome c oxidase subunit I from diverse metazoan invertebrates. Mol. Mar. Biol. Biotechnol. 3, 294–299.
Fonseca, G., Fontaneto, D., and Di Domenico, M. (2018). Addressing biodiversity shortfall in meiofauna. J. Exp. Mar. Biol. Ecol. 502, 26–38. doi: 10.1016/j.jembe.2017.05.007
Fonseca, V. G., Carvalho, G. R., Sung, W., Johnson, H. F., Power, D. M., Neill, S. P., et al. (2010). Second-generation environmental sequencing unmasks marine metazoan biodiversity. Nat. Commun. 1:98.
Fonseca, V. G., Sinniger, F., Gaspar, J., Quince, C., Creer, S., Power, D. M., et al. (2017). Revealing higher than expected meiofaunal diversity in Antarctic sediments: a metabarcoding approach. Sci. Rep. 7:6094.
Fontaneto, D., Kaya, M., Herniou, E. A., and Barraclough, T. G. (2009). Extreme levels of hidden diversity in microscopic animals (Rotifera) revealed by DNA taxonomy. Mol. Phylogenet. Evol. 53, 182–189.
Geller, J., Meyer, C., Parker, M., and Hawk, H. (2013). Redesign of PCR primers for mitochondrial cytochrome c oxidase subunit I for marine invertebrates and application in all-taxa biotic surveys. Mol. Ecol. Resour. 13, 851–861. doi: 10.1111/1755-0998.12138
Giere, O. (2009). Meiobenthology: The Microscopic Motile Fauna of Aquatic Sediments. Heidelberg: Springer Science & Business Media.
Guardiola, M., Uriz, M. J., Taberlet, P., Coissac, E., Wangensteen, O. S., and Turon, X. (2015). Deep-sea, deep-sequencing: metabarcoding extracellular DNA from sediments of marine canyons. PLoS One 10:e0139633. doi: 10.1371/journal.pone.0139633
Guardiola, M., Wangensteen, O. S., Taberlet, P., Coissac, E., Uriz, M. J., and Turon, X. (2016). Spatio-temporal monitoring of deep-sea communities using metabarcoding of sediment DNA and RNA. PeerJ 4:e2807. doi: 10.7717/peerj.2807
Hadziavdic, K., Lekang, K., Lanzen, A., Jonassen, I., Thompson, E. M., and Troedsson, C. (2014). Characterization of the 18S rRNA Gene for Designing Universal Eukaryote Specific Primers. PLoS One 9:e87624. doi: 10.1371/journal.pone.0087624
Haenel, Q., Holovachov, O., Jondelius, U., Sundberg, P., and Bourlat, S. J. (2017). NGS-based biodiversity and community structure analysis of meiofaunal eukaryotes in shell sand from Hållö island, Smögen, and soft mud from Gullmarn Fjord, Sweden. Biodivers. Data J. 5:e12731. doi: 10.3897/BDJ.5.e12731
Hao, X., Jiang, R., and Chen, T. (2011). Clustering 16S rRNA for OTU prediction: a method of unsupervised bayesian clustering. Bioinformatics 27, 611–618.
He, X., Gilmore, S. R., Sutherland, T. F., Hajibabaei, M., Miller, K. M., Westfall, K. M., et al. (2021). Biotic signals associated with benthic impacts of salmon farms from eDNA metabarcoding of sediments. Mol. Ecol. 30, 3158–3174. doi: 10.1111/mec.15814
He, X., Sutherland, T. F., and Abbott, C. L. (2020). Improved efficiency in eDNA metabarcoding of benthic metazoans by sieving sediments prior to DNA extraction. Environ. DNA 3, 716–726. doi: 10.1002/edn3.172
Hebert, P. D. N., Braukmann, T. W. A., Prosser, S. W. J., Ratnasingham, S., deWaard, J. R., Ivanova, N. V., et al. (2018). A sequel to sanger: amplicon sequencing that scales. BMC Genomics 19:219. doi: 10.1186/s12864-018-4611-3
Heeger, F., Bourne, E. C., Baschien, C., Yurkov, A., Bunk, B., Spröer, C., et al. (2018). Long-read DNA metabarcoding of ribosomal RNA in the analysis of fungi from aquatic environments. Mol. Ecol. Resour. 18, 1500–1514.
Higgins, R. P., and Thiel, H. (1988). Introduction to the Study of Meiofauna. Washington, DC: Smithsonian Institution Press, 1–488.
Hugerth, L. W., Muller, E. E. L., Hu, Y. O. O., Lebrun, L. A. M., Roume, H., Lundin, D., et al. (2014). Systematic design of 18S rRNA gene primers for determining eukaryotic diversity in microbial Consortia. PLoS One 9:e95567. doi: 10.1371/journal.pone.0095567
Hughes, A. C., Orr, M. C., Ma, K., Costello, M. J., Waller, J., Provoost, P., et al. (2021). Sampling biases shape our view of the natural world. Ecography 44, 1259–1269. doi: 10.1111/ecog.05926
Jungbluth, M. J., Burns, J., Grimaldo, L., Slaughter, A., Katla, A., and Kimmerer, W. (2020). Feeding habits and novel prey of larval fishes in the northern San Francisco Estuary. bioRxiv [Preprint]. doi: 10.1101/2020.10.18.344440
Karst, S. M., Dueholm, M. S., McIlroy, S. J., Kirkegaard, R. H., Nielsen, P. H., and Albertsen, M. (2018). Retrieval of a million high-quality, full-length microbial 16S and 18S rRNA gene sequences without primer bias. Nat. Biotechnol. 36, 190–195. doi: 10.1038/nbt.4045
Kitahashi, T., Sugime, S., Inomata, K., Nishijima, M., Kato, S., and Yamamoto, H. (2020). Meiofaunal diversity at a seamount in the Pacific Ocean: a comprehensive study using environmental DNA and RNA. Deep Sea Res. Part I Oceanogr. Res. Pap. 160:103253. doi: 10.1016/j.dsr.2020.103253
Klunder, L., Duineveld, G. C., Lavaleye, M. S., van der Veer, H. W., Palsbøll, P. J., and van Bleijswijk, J. D. (2019). Diversity of Wadden Sea macrofauna and meiofauna communities highest in DNA from extractions preceded by cell lysis. J. Sea Res. 152:101764.
Klunder, L., De Stigter, H., Lavaleye, M. S., Van Bleijswijk, J. D., Van Der Veer, H. W., Reichart, G.-J., et al. (2020a). A molecular approach to explore the background benthic fauna around a hydrothermal vent and their larvae: implications for future mining of deep-sea SMS deposits. Front. Mar. Sci. 7:134. doi: 10.3389/fmars.2020.00134
Klunder, L., Lavaleye, M. S. S., Filippidi, A., van Bleijswijk, J. D. L., Reichart, G.-J., van der Veer, H. W., et al. (2020b). Impact of an artificial structure on the benthic community composition in the southern North Sea: assessed by a morphological and molecular approach. ICES J. Mar. Sci. 77, 1167–1177. doi: 10.1093/icesjms/fsy114
Lallias, D., Hiddink, J. G., Fonseca, V. G., Gaspar, J. M., Sung, W., Neill, S. P., et al. (2015). Environmental metabarcoding reveals heterogeneous drivers of microbial eukaryote diversity in contrasting estuarine ecosystems. ISME J. 9, 1208–1221. doi: 10.1038/ismej.2014.213
Lanzén, A., Lekang, K., Jonassen, I., Thompson, E. M., and Troedsson, C. (2017). DNA extraction replicates improve diversity and compositional dissimilarity in metabarcoding of eukaryotes in marine sediments. PLoS One 12:e0179443. doi: 10.1371/journal.pone.0179443
Laroche, O., Kersten, O., Smith, C. R., and Goetze, E. (2020). Environmental DNA surveys detect distinct metazoan communities across abyssal plains and seamounts in the western Clarion Clipperton Zone. Mol. Ecol. 29, 4588–4604. doi: 10.1111/mec.15484
Laroche, O., Wood, S. A., Tremblay, L. A., Ellis, J. I., Lejzerowicz, F., Pawlowski, J., et al. (2016). First evaluation of foraminiferal metabarcoding for monitoring environmental impact from an offshore oil drilling site. Mar. Environ. Res. 120, 225–235.
Leasi, F., Sevigny, J. L., and Hassett, B. T. (2021). Meiofauna as a valuable bioindicator of climate change in the polar regions. Ecol. Indic. 121:107133. doi: 10.1016/j.ecolind.2020.107133
Leasi, F., Sevigny, J. L., Laflamme, E. M., Artois, T., Curini-Galletti, M., de Jesus Navarrete, A., et al. (2018). Biodiversity estimates and ecological interpretations of meiofaunal communities are biased by the taxonomic approach. Commun. Biol. 1:112. doi: 10.1038/s42003-018-0119-2
Lejzerowicz, F., Esling, P., Pillet, L., Wilding, T. A., Black, K. D., and Pawlowski, J. (2015). High-throughput sequencing and morphology perform equally well for benthic monitoring of marine ecosystems. Sci. Rep. 5:13932. doi: 10.1038/srep13932
Lekang, K., Thompson, E., and Troedsson, C. (2015). A comparison of DNA extraction methods for biodiversity studies of eukaryotes in marine sediments. Aquat. Microb. Ecol. 75, 15–25. doi: 10.3354/ame01741
Leray, M., and Knowlton, N. (2015). DNA barcoding and metabarcoding of standardized samples reveal patterns of marine benthic diversity. Proc. Natl. Acad. Sci. U.S.A. 112, 2076–2081. doi: 10.1073/pnas.1424997112
Leray, M., and Knowlton, N. (2016). Censusing marine eukaryotic diversity in the twenty-first century. Philos. Trans. R. Soc. B Biol. Sci. 371:20150331. doi: 10.1098/rstb.2015.0331
Leray, M., Yang, J. Y., Meyer, C. P., Mills, S. C., Agudelo, N., Ranwez, V., et al. (2013). A new versatile primer set targeting a short fragment of the mitochondrial COI region for metabarcoding metazoan diversity: application for characterizing coral reef fish gut contents. Front. Zool. 10:34. doi: 10.1186/1742-9994-10-34
Lobo, J., Costa, P. M., Teixeira, M. A., Ferreira, M. S., Costa, M. H., and Costa, F. O. (2013). Enhanced primers for amplification of DNA barcodes from a broad range of marine metazoans. BMC Ecol. 13:38. doi: 10.1186/1472-6785-13-34
Macher, J. N., Macher, T.-H., and Leese, F. (2017). Combining NCBI and BOLD databases for OTU assignment in metabarcoding and metagenomic datasets: the BOLD_NCBI _Merger. Metabarcod. Metagenom. 1:e22262.
Machida, R. J., Leray, M., Ho, S.-L., and Knowlton, N. (2017). Metazoan mitochondrial gene sequence reference datasets for taxonomic assignment of environmental samples. Sci. Data 4:170027. doi: 10.1038/sdata.2017.27
Mahé, F., Rognes, T., Quince, C., de Vargas, C., and Dunthorn, M. (2015). Swarm v2: highly-scalable and high-resolution amplicon clustering. PeerJ 3:e1420.
Martínez, A., Eckert, E. M., Artois, T., Careddu, G., Casu, M., Curini-Galletti, M., et al. (2020). Human access impacts biodiversity of microscopic animals in sandy beaches. Commun. Biol. 3:175. doi: 10.1038/s42003-020-0912-6
Matheson, C. D., Gurney, C., Esau, N., and Lehto, R. (2010). Assessing PCR inhibition from humic substances. Open Enzyme Inhib. J. 3, 38–45.
Meyer, C. P. (2003). Molecular systematics of cowries (Gastropoda: Cypraeidae) and diversification patterns in the tropics. Biol. J. Linn. Soc. 79, 401–459. doi: 10.1046/j.1095-8312.2003.00197.x
Montagna, P. A. (1984). In situ measurement of meiobenthic grazing rates on sediment bacteria and edaphic diatoms. Mar. Ecol. Prog. Ser. 18, 119–130.
Montagna, P. A., Baguley, J. G., Hsiang, C.-Y., and Reuscher, M. G. (2017). Comparison of sampling methods for deep-sea infauna. Limnol. Oceanogr. 15, 166–183. doi: 10.1002/lom3.10150
Müller, C. A., de Mattos Pereira, L., Lopes, C., Cares, J., dos Anjos Borges, L. G., Giongo, A., et al. (2019). Meiofaunal diversity in the Atlantic Forest soil: a quest for nematodes in a native reserve using eukaryotic metabarcoding analysis. For. Ecol. Manage. 453:117591.
Nascimento, F. J. A., Lallias, D., Bik, H. M., and Creer, S. (2018). Sample size effects on the assessment of eukaryotic diversity and community structure in aquatic sediments using high-throughput sequencing. Sci. Rep. 8:11737. doi: 10.1038/s41598-018-30179-1
Nearing, J. T., Douglas, G. M., Comeau, A. M., and Langille, M. G. (2018). Denoising the denoisers: an independent evaluation of microbiome sequence error-correction approaches. PeerJ 6:e5364. doi: 10.7717/peerj.5364
Nickrent, D. L., and Sargent, M. L. (1991). An overview of the secondary structure of the V4 region of eukaryotic small-subunit ribosomal RNA. Nucleic Acids Res. 19, 227–235. doi: 10.1093/nar/19.2.227
Okazaki, Y., Fujinaga, S., Salcher, M. M., Callieri, C., Tanaka, A., Kohzu, A., et al. (2021). Microdiversity and phylogeographic diversification of bacterioplankton in pelagic freshwater systems revealed through long-read amplicon sequencing. Microbiome 9:24. doi: 10.1186/s40168-020-00974-y
Pansu, J., Chapman, M. B., Hose, G. C., and Chariton, A. A. (2021). Comparison of an extracellular v. total DNA extraction approach for environmental DNA-based monitoring of sediment biota. Mar. Freshw. Res. doi: 10.1071/MF20269
Porazinska, D. L., Giblin-Davis, R. M., Faller, L., Farmerie, W., Kanzaki, N., Morris, K., et al. (2009). Evaluating high-throughput sequencing as a method for metagenomic analysis of nematode diversity. Mol. Ecol. Resour. 9, 1439–1450.
Pruesse, E., Quast, C., Knittel, K., Fuchs, B. M., Ludwig, W., Peplies, J., et al. (2007). SILVA: a comprehensive online resource for quality checked and aligned ribosomal RNA sequence data compatible with ARB. Nucleic Acids Res. 35, 7188–7196.
Ptatscheck, C., Gehner, S., and Traunspurger, W. (2020). Should we redefine meiofaunal organisms? The impact of mesh size on collection of meiofauna with special regard to nematodes. Aquat. Ecol. 54, 1135–1143.
R Core Team (2020). R: A Language and Environment for Statistical Computing. Vienna: R Foundation for Statistical Computing.
Radulovici, A. E., Vieira, P. E., Duarte, S., Teixeira, M. A. L., Borges, L. M. S., Deagle, B., et al. (2021). Revision and annotation of DNA barcode records for marine invertebrates: report of the 8th iBOL conference hackathon. bioRxiv [Preprint]. doi: 10.1101/2021.03.07.434272
Ratnasingham, S., and Hebert, P. D. (2007). BOLD: the barcode of life data system (http://www. barcodinglife. org). Mol. Ecol. Notes 7, 355–364.
Salonen, I., Chronopoulou, P., Leskinen, E., and Koho, K. (2019). Metabarcoding successfully tracks temporal changes in eukaryotic communities in coastal sediments. FEMS Microbiol. Ecol. 95:fiy226.
Sayers, E. W., Cavanaugh, M., Clark, K., Pruitt, K. D., Schoch, C. L., Sherry, S. T., et al. (2021). GenBank. Nucleic Acids Res. 49, D92–D96.
Schenk, J., and Fontaneto, D. (2020). Biodiversity analyses in freshwater meiofauna through DNA sequence data. Hydrobiologia 847, 2597–2611.
Schratzberger, M., and Ingels, J. (2018). Meiofauna matters: the roles of meiofauna in benthic ecosystems. J. Exp. Mar. Biol. Ecol. 502, 12–25.
Semprucci, F., Sbrocca, C., Rocchi, M., and Balsamo, M. (2015). Temporal changes of the meiofaunal assemblage as a tool for the assessment of the ecological quality status. J. Mar. Biol. Assoc. U. K. 95, 247–254. doi: 10.1017/S0025315414001271
Simpson, S., and Batley, G. (2016). Sediment Quality Assessment: A Practical Guide. Victoria: Csiro Publishing.
Singer, G. A. C., Fahner, N. A., Barnes, J. G., McCarthy, A., and Hajibabaei, M. (2019). Comprehensive biodiversity analysis via ultra-deep patterned flow cell technology: a case study of eDNA metabarcoding seawater. Sci. Rep. 9:5991. doi: 10.1038/s41598-019-42455-9
Sinniger, F., Pawlowski, J., Harii, S., Gooday, A. J., Yamamoto, H., Chevaldonné, P., et al. (2016). Worldwide analysis of sedimentary DNA reveals major gaps in taxonomic knowledge of deep-sea benthos. Front. Mar. Sci. 3:92. doi: 10.3389/fmars.2016.00092
Smith, D. P., and Peay, K. G. (2014). Sequence depth, not PCR replication, improves ecological inference from next generation DNA sequencing. PLoS One 9:e90234. doi: 10.1371/journal.pone.0090234
Snelgrove, P. V. R. (1999). Getting to the bottom of marine biodiversity: sedimentary habitats: ocean bottoms are the most widespread habitat on Earth and support high biodiversity and key ecosystem services. BioScience 49, 129–138. doi: 10.2307/1313538
Somerfield, P. J., Warwick, R. M., and Moens, T. (2005). “Meiofauna techniques,” in Methods for the Study of Marine Benthos, 3rd Edn, eds A. Eleftheriou and A. McIntyre (Oxford: Blackwell Science), 229–272.
Stoeck, T., Bass, D., Nebel, M., Christen, R., Jones, M. D. M., Breiner, H.-W., et al. (2010). Multiple marker parallel tag environmental DNA sequencing reveals a highly complex eukaryotic community in marine anoxic water. Mol. Ecol. 19, 21–31. doi: 10.1111/j.1365-294X.2009.04480.x
Taberlet, P., Bonin, A., Zinger, L., and Coissac, E. (2018). Environmental DNA: For Biodiversity Research and Monitoring. Oxford: Oxford University Press.
Taberlet, P., Coissac, E., Pompanon, F., Brochmann, C., and Willerslev, E. (2012a). Towards next-generation biodiversity assessment using DNA metabarcoding. Mol. Ecol. 21, 2045–2050.
Taberlet, P., Prud’homme, S. M., Campione, E., Roy, J., Miquel, C., Shehzad, W., et al. (2012b). Soil sampling and isolation of extracellular DNA from large amount of starting material suitable for metabarcoding studies. Mol. Ecol. 21, 1816–1820.
Tang, C. Q., Leasi, F., Obertegger, U., Kieneke, A., Barraclough, T. G., and Fontaneto, D. (2012). The widely used small subunit 18S rDNA molecule greatly underestimates true diversity in biodiversity surveys of the meiofauna. Proc. Natl. Acad. Sci. U.S.A. 109, 16208–16212.
Thiel, H. (1983). “Meiobenthos and nanobenthos of the deep sea,” in Deep-sea Biology, The Sea, Vol. 8, ed. G. T. Rowe (New York, NY: John Wiley and Sons), 167–230.
Thomsen, P. F., and Willerslev, E. (2015). Environmental DNA–An emerging tool in conservation for monitoring past and present biodiversity. Biol. Conserv. 183, 4–18.
Torti, A., Lever, M. A., and Jørgensen, B. B. (2015). Origin, dynamics, and implications of extracellular DNA pools in marine sediments. Mar. Genomics 24, 185–196.
Turon, X., Antich, A., Palacín, C., Præbel, K., and Wangensteen, O. S. (2020). From metabarcoding to metaphylogeography: separating the wheat from the chaff. Ecol. Appl. 30:e02036. doi: 10.1002/eap.2036
van der Loos, L. M., and Nijland, R. (2021). Biases in bulk: DNA metabarcoding of marine communities and the methodology involved. Mol. Ecol. 30, 3270–3288. doi: 10.1111/mec.15592
Wangensteen, O. S., Palacín, C., Guardiola, M., and Turon, X. (2018). DNA metabarcoding of littoral hard-bottom communities: high diversity and database gaps revealed by two molecular markers. PeerJ 6:e4705. doi: 10.7717/peerj.4705
Weigand, A. M., and Macher, J. N. (2018). A DNA metabarcoding protocol for hyporheic freshwater meiofauna: evaluating highly degenerate COI primers and replication strategy. Metabarcoding Metagenom. 2:e26869. doi: 10.3897/mbmg.2.26869
Weigand, H., Beermann, A. J., Čiampor, F., Costa, F. O., Csabai, Z., Duarte, S., et al. (2019). DNA barcode reference libraries for the monitoring of aquatic biota in Europe: gap-analysis and recommendations for future work. Sci. Total Environ. 678, 499–524. doi: 10.1016/j.scitotenv.2019.04.247
Weirather, J. L., de Cesare, M., Wang, Y., Piazza, P., Sebastiano, V., Wang, X.-J., et al. (2017). Comprehensive comparison of pacific biosciences and oxford nanopore technologies and their applications to transcriptome analysis. F1000Res. 6:100.
Xie, Y., Hong, S., Kim, S., Zhang, X., Yang, J., Giesy, J. P., et al. (2017). Ecogenomic responses of benthic communities under multiple stressors along the marine and adjacent riverine areas of northern Bohai Sea, China. Chemosphere 172, 166–174. doi: 10.1016/j.chemosphere.2016.12.121
Xie, Y., Zhang, X., Yang, J., Kim, S., Hong, S., Giesy, J. P., et al. (2018). eDNA-based bioassessment of coastal sediments impacted by an oil spill. Environ. Pollut. 238, 739–748.
Zeppilli, D., Leduc, D., Fontanier, C., Fontaneto, D., Fuchs, S., Gooday, A. J., et al. (2018). Characteristics of meiofauna in extreme marine ecosystems: a review. Mar. Biodivers. 48, 35–71. doi: 10.1007/s12526-017-0815-z
Zeppilli, D., Sarrazin, J., Leduc, D., Arbizu, P. M., Fontaneto, D., Fontanier, C., et al. (2015). Is the meiofauna a good indicator for climate change and anthropogenic impacts? Mar. Biodivers. 45, 505–535. doi: 10.1007/s12526-015-0359-z
Keywords: meiofauna, DNA metabarcoding, marine ecosystem, biomonitoring, meiobenthos, molecular methods, sampling techniques
Citation: Gielings R, Fais M, Fontaneto D, Creer S, Costa FO, Renema W and Macher J-N (2021) DNA Metabarcoding Methods for the Study of Marine Benthic Meiofauna: A Review. Front. Mar. Sci. 8:730063. doi: 10.3389/fmars.2021.730063
Received: 24 June 2021; Accepted: 13 September 2021;
Published: 30 September 2021.
Edited by:
Ylenia Carotenuto, Stazione Zoologica Anton Dohrn, ItalyReviewed by:
Laura Carugati, University of Cagliari, ItalyCory Douglas Bishop, St. Francis Xavier University, Canada
Copyright © 2021 Gielings, Fais, Fontaneto, Creer, Costa, Renema and Macher. This is an open-access article distributed under the terms of the Creative Commons Attribution License (CC BY). The use, distribution or reproduction in other forums is permitted, provided the original author(s) and the copyright owner(s) are credited and that the original publication in this journal is cited, in accordance with accepted academic practice. No use, distribution or reproduction is permitted which does not comply with these terms.
*Correspondence: Jan-Niklas Macher, jan.macher@naturalis.nl