- 1Swedish Geotechnical Institute, Linköping, Sweden
- 2Geological Survey of Sweden, Uppsala, Sweden
- 3Department of Aquatic Sciences and Assessment, Swedish University of Agricultural Sciences, Uppsala, Sweden
- 4Department of Earth Sciences, Uppsala University, Uppsala, Sweden
Unregulated discharges of wastewater from pulp and paper factories resulted in the formation of relatively thick organic (cellulose) rich sediments in shallow waters along the Swedish coast. These deposits are known as fiberbanks and are contaminated by persistent organic pollutants (POPs), metals and methylmercury, which can be dispersed by diffusion and advective processes coupled to propeller wash, high river discharges, strong wind waves and submarine landslides. Based on a case study of polychlorinated biphenyls (PCBs), one group of prevalent POPs in the fiberbanks, we present a probabilistic approach to estimate the potential risk of dispersion of fiberbank contaminants. The approach allows for estimation of the dispersal pathways that dominates the risk within a given time and provides more insight about the significance of various dispersion processes. We show that it is highly likely that chemical diffusion and advection triggered by ship-induced resuspension will disperse PCBs (sum of seven congeners; Σ7PCB) above a threshold level for environmental impact, while the likelihood of river and wind-wave generated resuspension dispersion pathways are lower (∼20%, respectively). We further show that there is approximately 5% likelihood that a submarine landslide will disperse Σ7PCB above the threshold level. The study implies that the governing parameters for risk assessment specifically should include reliable data on contaminant concentration, water depth above the fiberbank, estimation of concerned fiberbank areas, time duration of erosive fluid flows and measured diffusion. The approach provides insight into the importance of various dispersion processes. We suggest that it can be applied to support risk assessment, especially when there are limited available data and/or knowledge about the system under study.
Introduction
Historical and contemporary discharges of pollutants into water have caused sediments to become polluted with toxins, such as persistent organic pollutants (POPs), metals and methylmercury, which may pose a risk to exposed ecosystems (e.g., Apitz et al., 2006; Gerbersdorf et al., 2011; Dahlberg et al., 2021; USEPA, 2021). Globally, efforts are made to manage contaminated sediments, but many remediation measures are costly (Reible, 2014; Jersak et al., 2016a). Consequently, risk assessments are carried out to prioritize sites for remediation. Sediment can act both as a contaminant sink in calm accumulation areas and a contaminant source if the accumulated sediment is exposed to diffusive and advective dispersion processes (Förstner and Westrich, 2005), which can be continuous or intermittent. The sink or source perspective affects the risk scenario and the management options. Understanding and assessing the likelihood of dispersion thus becomes an important part of risk assessment (Reible, 2014).
Sediments have been (and are still) contaminated with metals and POPs by a range of point and diffuse sources. In Sweden, historically unregulated discharges of wastewater from pulp and paper factories resulted in the accumulation of sediments rich in cellulose fibers and wood chips along the Swedish coast (Apler et al., 2019, 2020; Dahlberg et al., 2020, 2021). Subaqueous, relatively thick cellulose rich deposits (further denoted as fiberbanks) have been found in shallow-water coastal areas close to the mills, and on nearby deeper seabed areas, fiber-rich sediments have formed. These coastally located fiberbanks may be a result of the relatively sheltered conditions that prevail in the Baltic Sea, which has no significant tides. Fiberbank deposits and fiber-rich sediments along the north-eastern coast of Sweden are estimated to cover an area of at least 2,500,000 m2 (estimated volume: 7,000,000 m3) and 26,500,000 m2 (estimated volume: 11,000,000 m3), respectively (Norrlin and Josefsson, 2017). Overall, little is known about the behavior of the fibrous material and the fibrous sediments that the pulp and paper industry have generated. The pulp and paper industry is an important industrial sector in the boreal forest region (Burton et al., 2003; Suhr et al., 2015). Occurrence of similar fibrous rich sediments has been reported from Canada (e.g., Poole et al., 1977; Krishnappan, 2000; Young and Smith, 2001; Hall, 2003; Hoffman et al., 2017), Finland (Kokko et al., 2018), Switzerland (Kienle et al., 2013), and Norway (Polovodova Asteman et al., 2015), albeit not reported using the terms “fiberbanks” or “fibrous sediments” and mostly occurring in riverine or lake environments.
In Sweden, the industrial discharge of suspended solids (e.g., cellulose fibers) ceased more than four decades ago. Yet, the offshore deposits remain, even in areas where hydrodynamics do not currently favor sedimentation, and deposition of less contaminated particles is generally low or non-existing (Norrlin and Josefsson, 2017). The fiberbanks differ from more natural, minerogenic sediments by their high fibrous organic content and low densities; some fiberbanks are almost buoyant due to inherent gas production from microbial degradation (Apler et al., 2019; Snowball et al., 2020). No organisms seem to thrive in the fiberbank deposits with the exception of some bacteria (Dahlberg et al., 2021) and the deposits are contaminated with POPs, such as polychlorinated dibenzo-p-dioxins (PCDDs), polychlorinated dibenzofurans (PCDFs), polychlorinated biphenyls (PCBs), dichlorodiphenyltrichloroethane (DDT) and polycyclic aromatic hydrocarbons (PAHs), and many metals, including mercury (Hg) (Norrlin and Josefsson, 2017; Apler et al., 2019; Dahlberg et al., 2020). Analyses of benthic biota from fiber-rich sediments show that organisms bioaccumulate the contaminants present in their habitat, thus acting as a vector for transfer to organisms at higher trophic levels (Dahlberg et al., 2021). Dispersion of larger amounts of cellulose fibers may occur and worsen the situation. For example, bathymetric surveys show clear traces of submarine landslides in fiberbank areas (Apler et al., 2014, 2019; Norrlin et al., 2016).
There is currently no national (Swedish) method for risk assessment of contaminated sediments. Common practice is to use the national guidelines for contaminated soils and international guidelines for contaminated sediments. From this viewpoint, our research was designed to assess: (i) the degree to which contaminants disperse from the fiberbanks to the surrounding water system, while considering the uncertainties; (ii) if dispersion becomes a risk to the environment; and (iii) the dispersion path(s) that determine(s) risk. Pathways for dispersion include diffusion and advection (e.g., Meddah et al., 2015). Differences in chemical potential between sediment and pore water, and concentration gradients between pore water and overlying bottom water drive lake and sea diffusion processes of POPs (e.g., Schwarzenbach et al., 1993; Parnish and Mackay, 2020). Chemical diffusion is a continuous process that disperses contaminants at a relatively slow rate, but it will persist until the chemical potential and concentration differences are equalized (Schwarzenbach et al., 1993; Parnish and Mackay, 2020). Advective processes generally disperse contaminants at a higher rate (e.g., Schnoor, 1996; Chanson, 2004), e.g., by resuspension of sediment particles due to ship traffic or during storms, high flow events and even submarine landslides. It is known that major flood events may re-mobilize contaminants from contaminated sediments (e.g., Haag et al., 2001; Westrich and Förstner, 2005; Roberts, 2012) and that propeller wash may re-suspend contaminated sediments (e.g., Michelsen et al., 1998; Superville et al., 2014; Prygiel et al., 2015). It has also been shown that extreme weather-events like hurricanes may increase dispersion of contaminants from contaminated sediments (Howell and Rifai, 2015; Dellapenna et al., 2020). Only a few studies have considered submarine landslides and other underwater mass movements as a pathway for contaminant dispersion, but they have been highlighted in a review paper by Kane and Clare (2019). Underwater mass movements are rare events and can be considered as more disastrous compared to other extreme discharge and storm events, not at least because of the potential to expose and redistribute large volumes of archived contaminants further downslope (e.g., Kane and Clare, 2019). Submarine landslides are driven by an imbalance between driving and resisting forces. Main driving forces are mass and shape of sediment formation (gravitation), excess pore water pressure, and the erosion at the toe of the slope, while the resistance is primarily determined by the strength of the materials involved. When the driving forces exceed the resisting forces, the slope gives way and a mass movement occurs.
The overall aim of this work was to develop a probabilistic method to estimate the risk for dispersion of POPs (here represented by Σ7PCB) from fiberbanks, considering several dispersion pathways, and to apply the method in a field case scenario. The method uses (mathematical) analytical solutions to estimate the likelihood of exceeding a level of pollution that is assumed to have a negative effect on the environment. The method is especially useful when available data and/or knowledge about a system under study are incomplete, because it considers several possible outcomes instead of one (e.g., UNDRR, 2021). A probabilistic approach can also help to identify parameters for which uncertainty needs to be reduced to improve assessments. Although the method is developed from a fiberbank perspective, it can be applied on contaminated sediments in general with the aim to consider slow continuous dispersion as well as extremely fast dispersion events.
Materials and Methods
A simplified probabilistic approach was used to assess the likelihood of contaminant dispersion from submerged anthropogenic fibrous pulp waste deposited on the near shore seabed outside an old pulp and paper factory (Figure 1). The approach can be used to discern the importance of possible dispersion pathways. Probable pathways for dispersion include chemical diffusion and advection caused by currents from propeller action, river discharge and wind-waves, and dispersion due to submarine landslides.
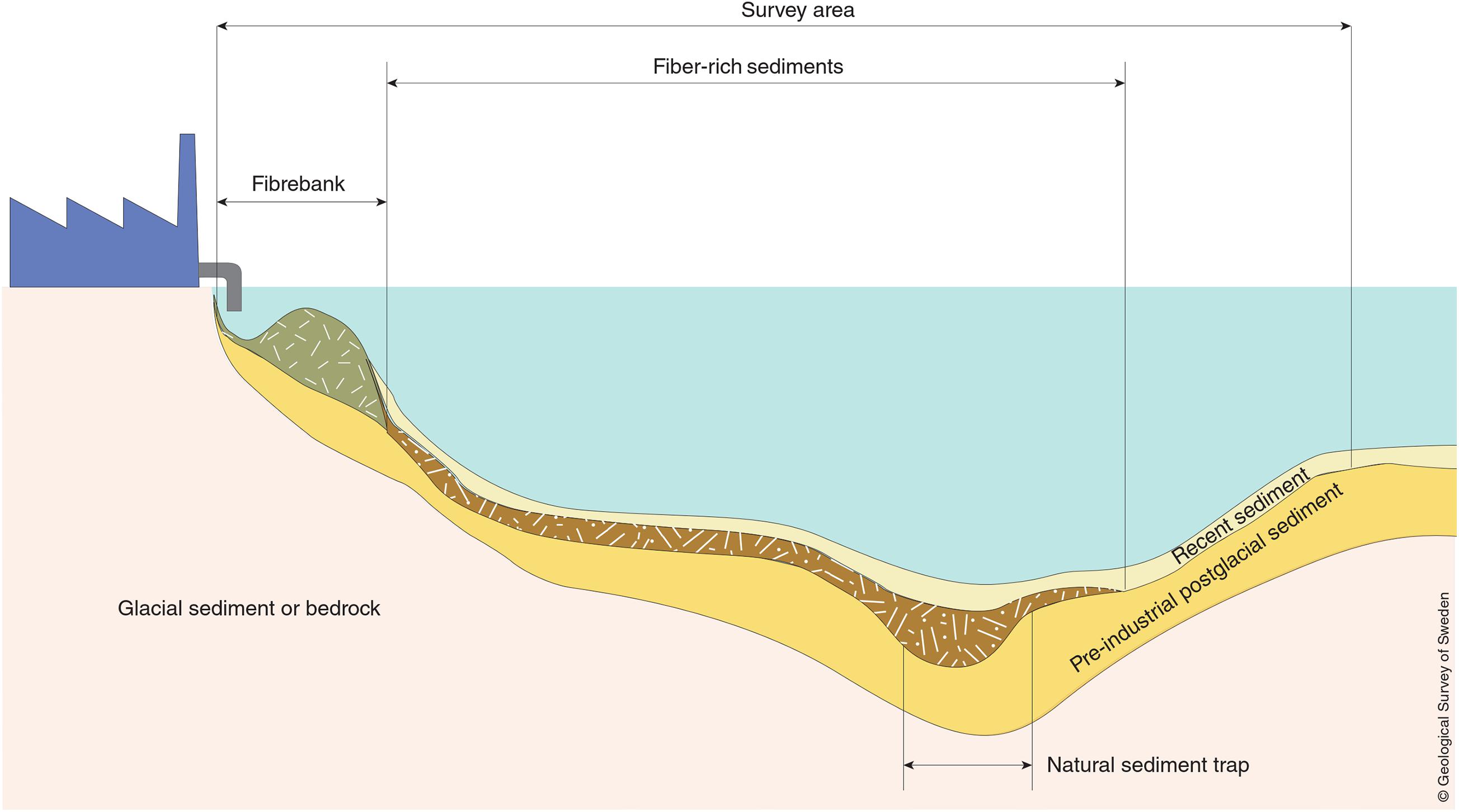
Figure 1. Schematic illustration of the situation with near shore fiberbank deposition. Illustration: Geological Survey of Sweden.
Case Study Site
The case study site constitutes the contaminated fiberbank outside of Väja pulp and paper factory. The fiberbank is situated in the inner part of the Ångermanälven river estuary, along the northern Bothnian Sea coast, Sweden (Figure 2). The estuary is shaped as a fjord-like basin that formed in a prolonged tectonic rift (Heinemo, 1999). The mean river discharge is ∼500 m3 s–1 at the river mouth. Between the years 1999 and 2015, a maximum of ∼2,300 m3 s–1 and a minimum of ∼90 m3 s–1 were recorded. The water in the estuary mostly contains 70–80% freshwater, but at discharges above 830 m3 s–1, the inflow of seawater is blocked and the proportion of freshwater temporarily becomes higher (Heinemo, 1999).
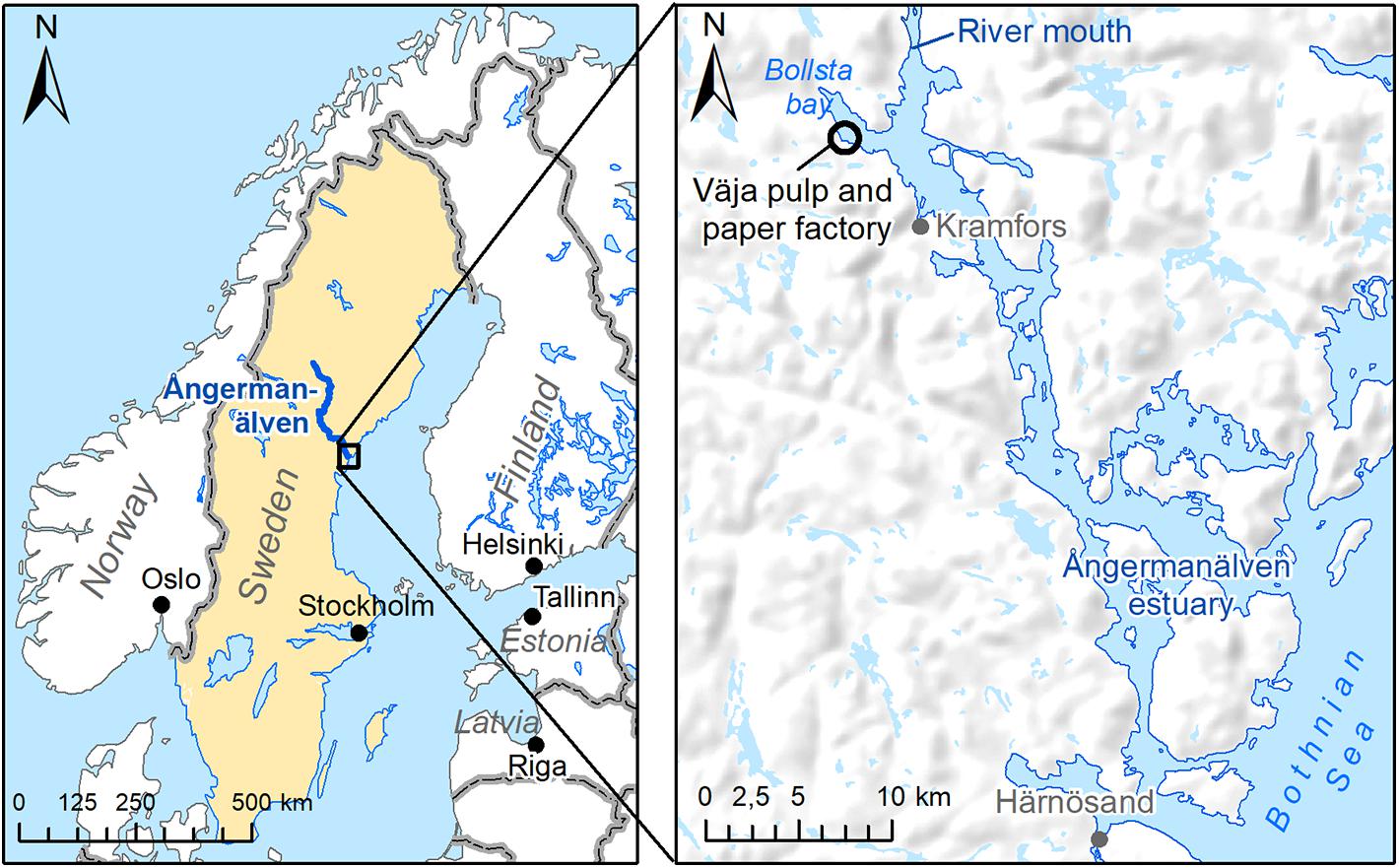
Figure 2. Location of the case study site. The contaminated fiberbank outside Väja pulp and paper factory is situated in the inner part of Ångermanälven river estuary, Sweden.
During the peak discharge of oxygen consuming organic fiber material from the industry in the post-war years of the 1950s, a clear expansion of anoxic seabed in the Ångermanälven river estuary was identified (Jerkeman and Norrström, 2018; Valeur, 2020). Many industries have closed down since then (Heinemo, 1999; Apler et al., 2020), and a recovery has been observed in some of the areas. However, several contaminated fiberbanks have been identified along the river estuary (Apler et al., 2014, 2019), and the mere presence of a fiberbank means that it has affected the natural sediment substrate and habitat at that location.
The studied contaminated sediment site is located adjacent to a sulfate pulp and paper factory (Figure 3) that has been in operation since the 1910s. Up to the 1980’s, oxygen consuming organic fiber material had accumulated on the seabed close to the factory’s water outlet. Although the discharge of suspended solids (including fibers) has essentially stopped, a large fiberbank, divided into two parts, remains, together covering an area of ∼70,000 m2 (Apler et al., 2019). The fiberbank is anoxic and consists mostly of cellulose fibers with a thickness exceeding 6 m in places. The fiberbank is resting upon natural minerogenic sediments some 20 m below the water surface and the surface of the deposit is located at ∼15 m water depth (Apler et al., 2019). The natural sediments successively slope about 10 degrees seaward down to a water depth of ca 50 m some 200 m out from the shoreline. The seabed next to the bank contains fiber-rich sediments that have been estimated to cover 800,000 m2, i.e., >10 times larger than the fiberbank itself. Several scars from submarine landslides are visible in 3D models of the seabed created from bathymetrical data (Figures 3, 4). The factory quay today receives about 40 vessels a year (personal communication with staff from Väja factory) for the handling of goods.
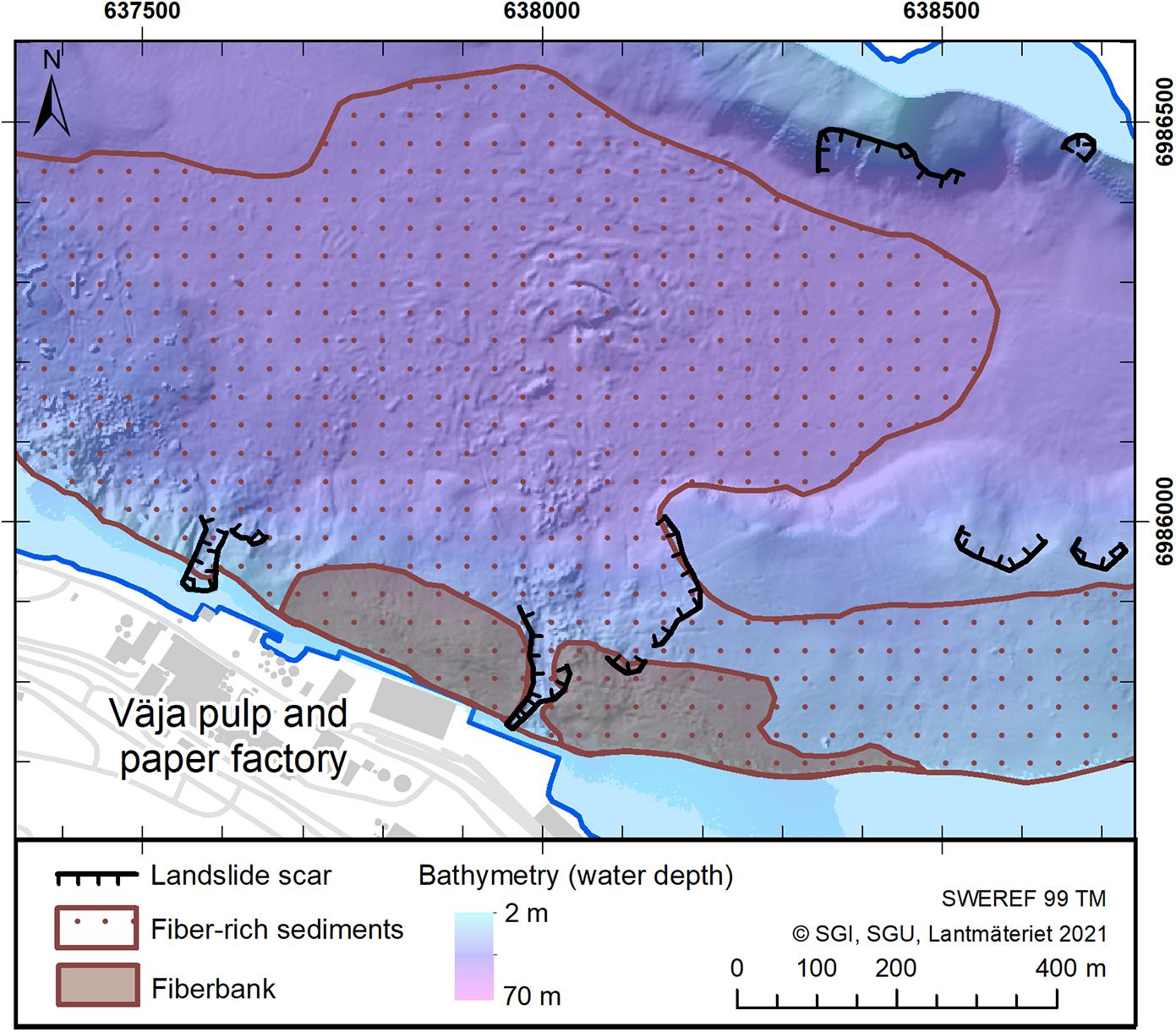
Figure 3. The fiberbank deposit and fiber-rich sediments outside Väja pulp factory, Ångermanälven river estuary, Sweden.
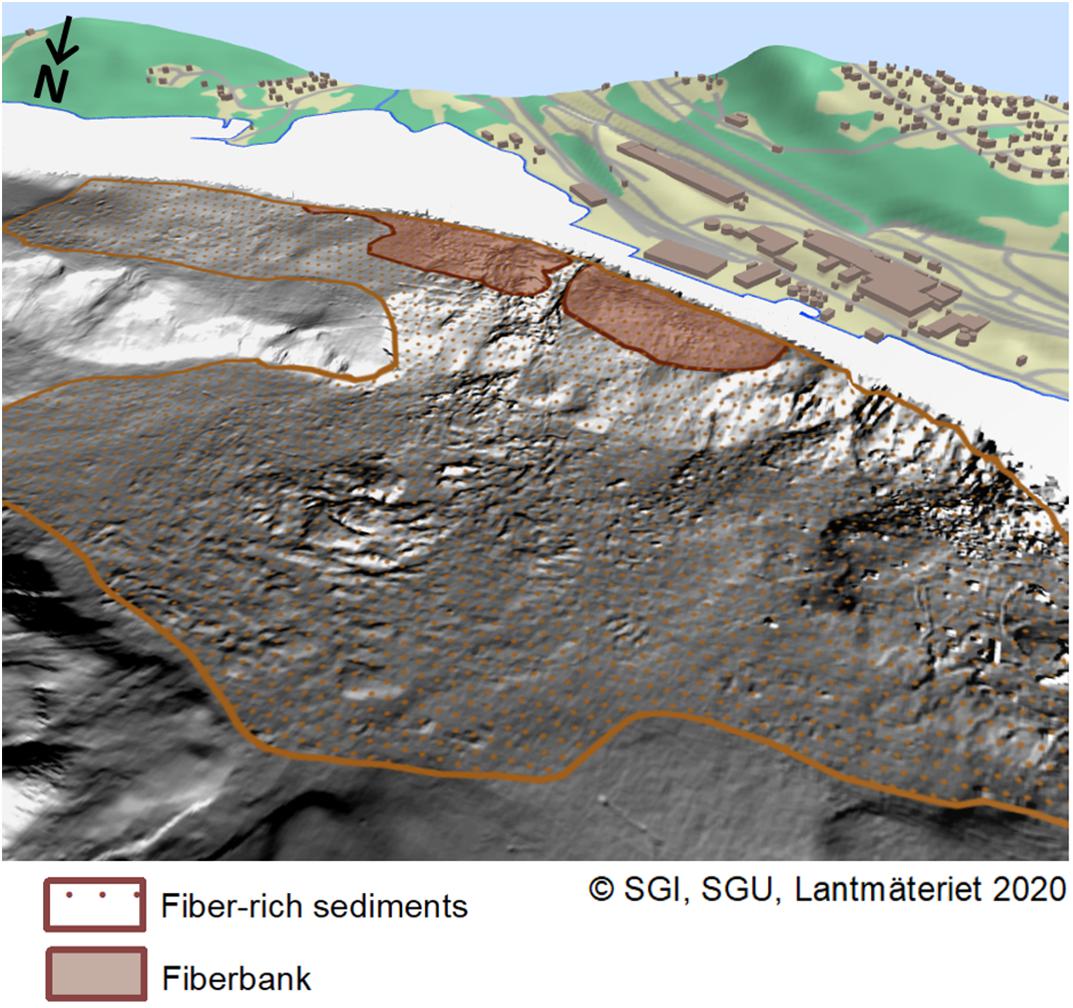
Figure 4. The same as Figure 3 but rotated to enhance the 3D image.
Field and Laboratory Measurements
All field measurements are presented in Apler et al.(2014, 2019, 2020), Dahlberg et al. (2020), Dahlberg et al. (2021), and Löfroth et al. (2021).
Geological and geotechnical surveys (2015 and 2017) show that the fiberbank is underlain by a thin layer of bark on top of a clayey silt, followed deeper down by laminated black-gray clayey silts that transition toward the base into glaciomarine varved clay (Löfroth et al., 2021). An interbedded coarse-grained layer was detected about 1–2 m down into the minerogenic sediment. The geotechnical investigation indicates that this layer has higher permeability and higher porewater pressure than the surrounding sediments (Löfroth et al., 2021).
Field samples were taken during a field study in Väja in August and September 2015. Subsequent laboratory studies of the samples showed that the fibers easily swirl during physical disturbance and that the fibers tend to tangle up and form small clumps (Lamparski, 2016). This observation shows that the resuspended fibers are thus of sufficiently low density to stay in suspension, disperse by currents and not settle until they reach calmer areas outside the fiberbank. Further, laboratory studies on particles obtained from two fiberbanks in the study area also indicate low settling velocities and indicate that the density of the pulp fibers is similar to the density of water (Löfroth et al., 2021). Figures 5, 6 show two photos of the fiberbank and fiberbank material at Väja.
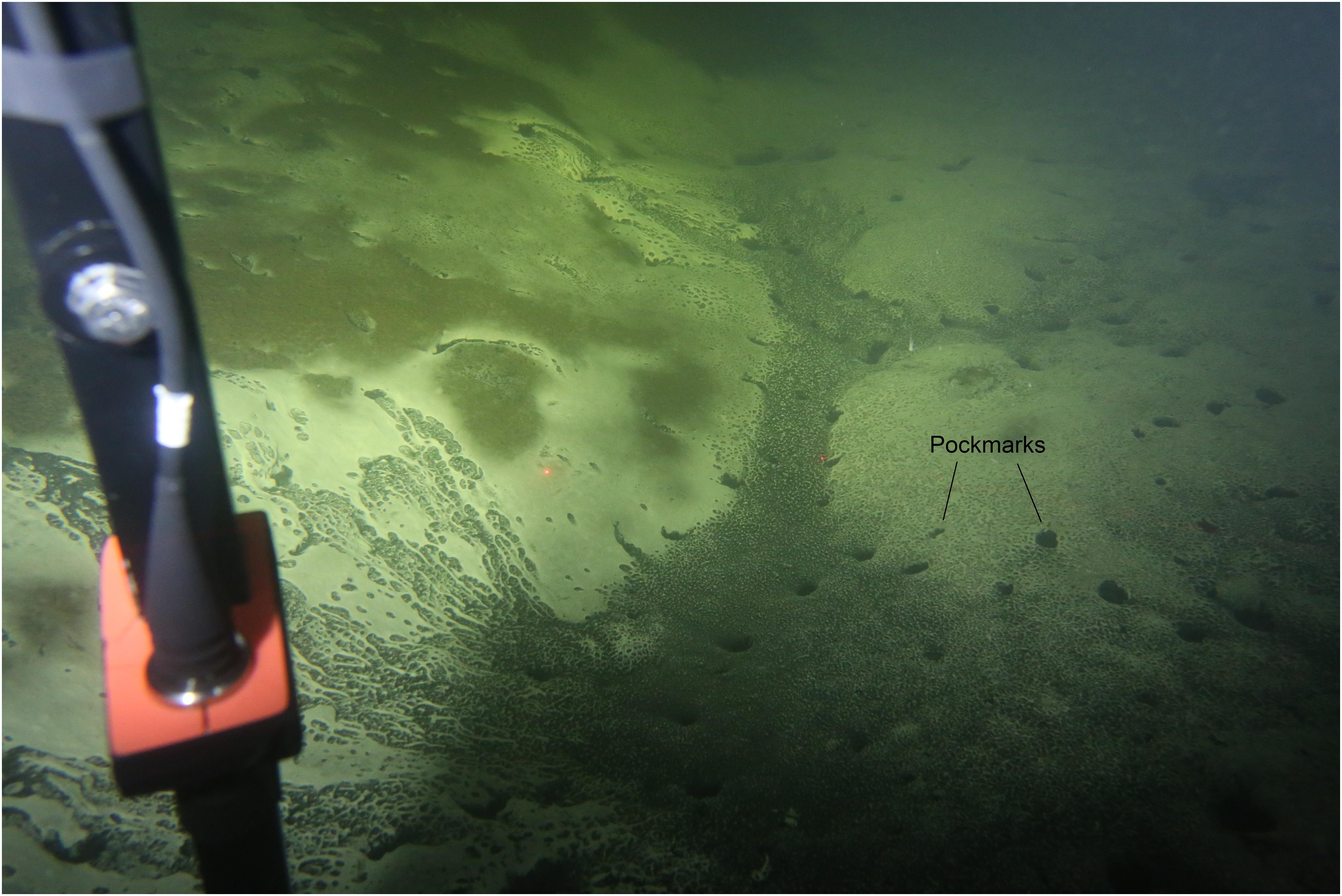
Figure 5. Underwater photo of the smooth surface of the fiberbank that is located in the shallow water outside Väja pulp and paper factory. Pockmarks are visible as round dark marks. The size of the pockmarks is ca 5 cm in diameter. Photo: Geological Survey of Sweden.
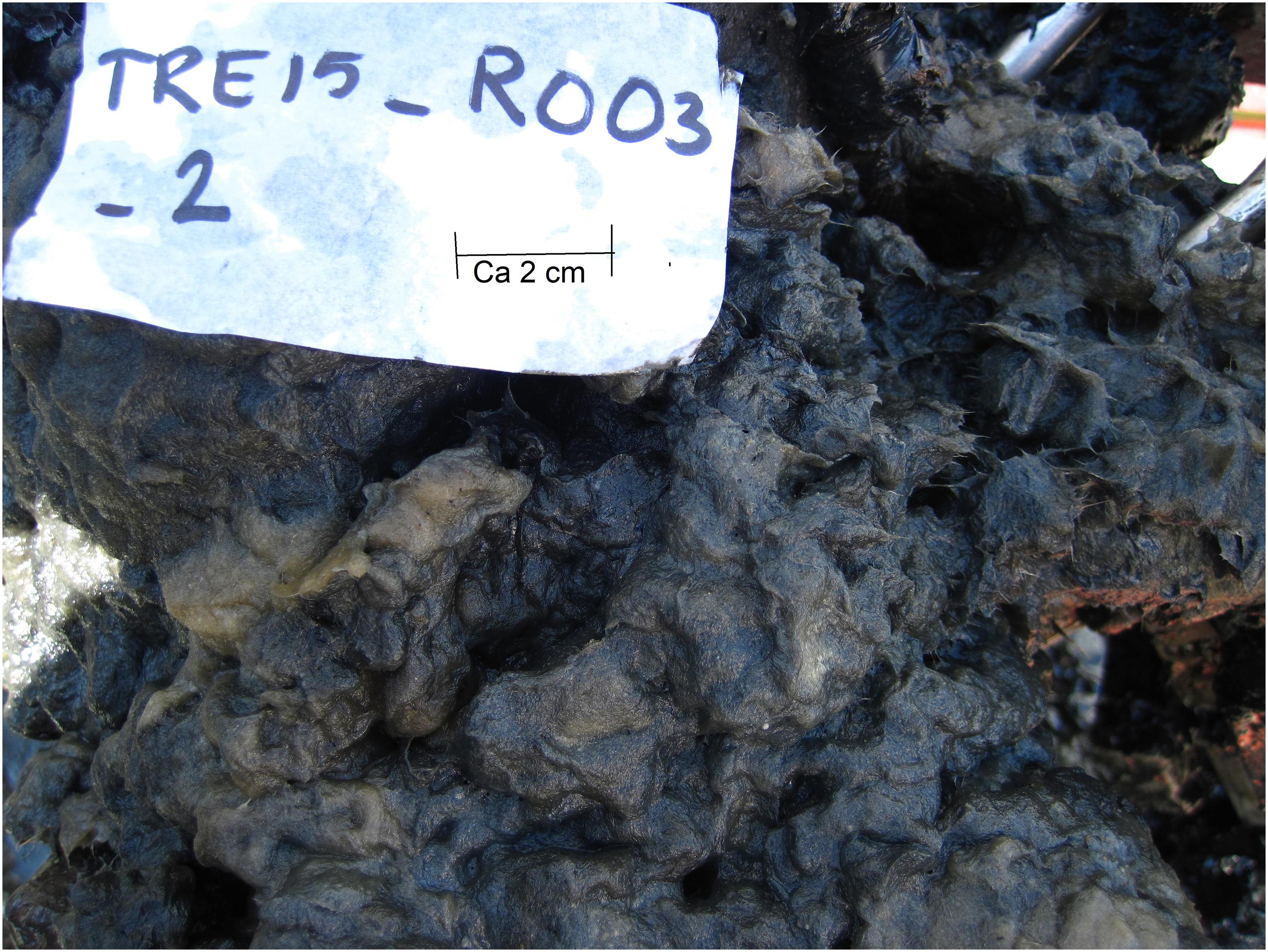
Figure 6. Photo of the thread-like cellulose fibers that makes up the fiberbank material looks like when it is taken up from the seabed. Photo: Geological Survey of Sweden.
Sediment-to-water fluxes of PCBs were measured in situ using benthic flux chambers (BFCs) equipped with semipermeable membrane devices (SPMDs) as passive samplers (see Eek et al. (2010) for detailed description of the BFCs used in this study). Seven indicator PCB congeners (CB-28, −52, −101, −118, −138, −153, −180) were measured and their sum concentrations (Σ7PCB) were used to illustrate the suggested methodology. The following matrices were analyzed with respect to levels of Σ7PCB: sediment (fiberbank, ng g–1 dw); porewater (pg L–1); diffusive flux (ng m–2 day–1) from SPMD samplers placed on the seabed; and dissolved fraction of the PCBs in sediment pore water (%). In addition, sediment (fiberbank) organic carbon content (% TOC), was determined. Detailed information on the chemical analyses is given in Dahlberg et al. (2020), Dahlberg et al. (2021).
River flow and wind data were obtained from SMHI. These data were used to theoretically calculate possible current conditions that may affect fiber particle mobility.
Assessing Likelihood of Dispersion
Dispersion pathways considered in this study were chemical diffusion and mechanical advection caused by current induced resuspension (propeller wash, wind-waves, river flow velocity) and submarine landslides (Figures 7A–E). The risk can be explained as a chain of events. In our study, the risk chain contains the following three events:
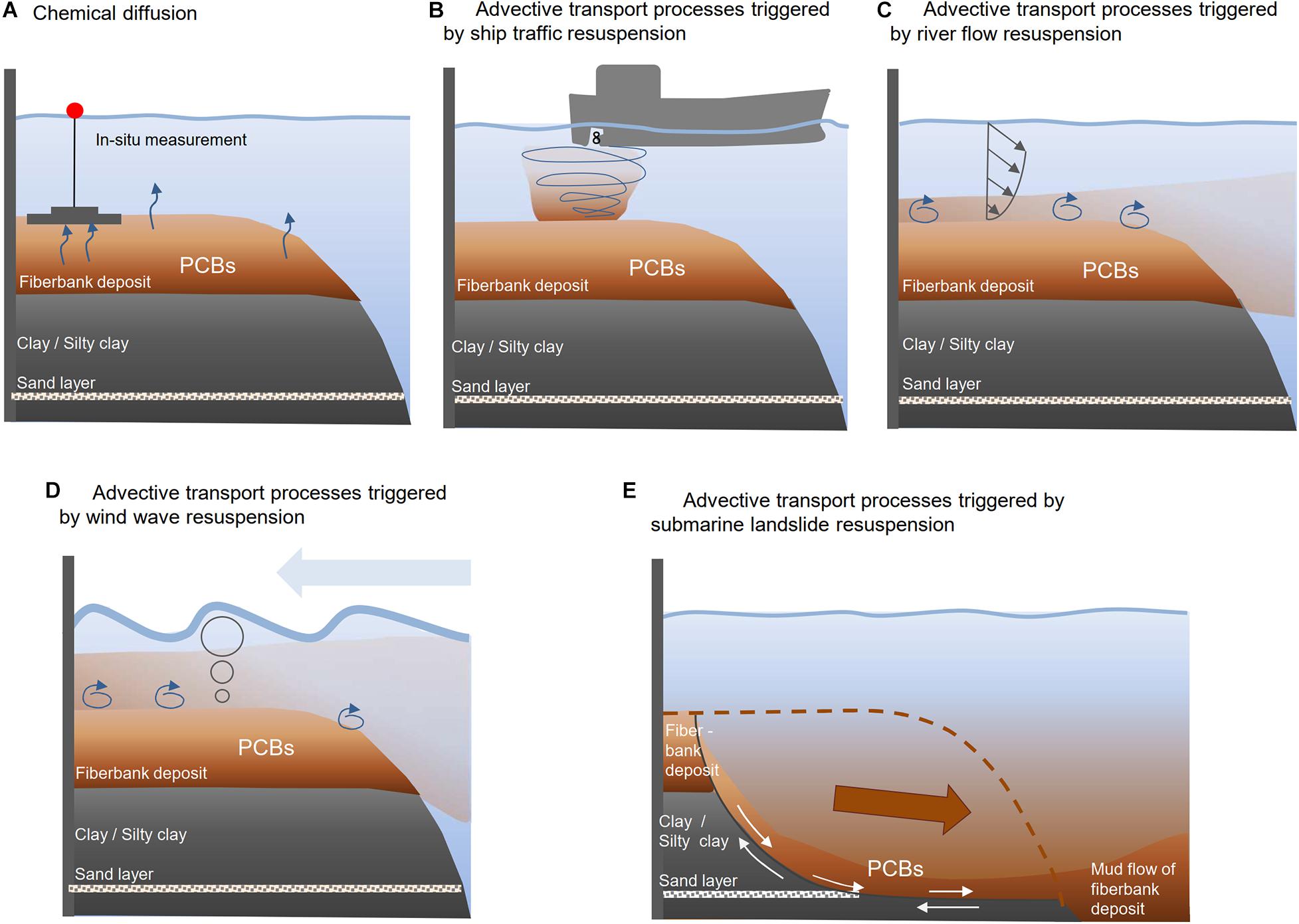
Figure 7. Schematic illustration of five different pathways for dispersion of contaminants and (contaminated) fiber particles from submerged fiberbank considered in our study: (A) chemical diffusion, (B) advection through ship (propeller) current induced resuspension, (C) advection through river flow current induced resuspension, (D) advection through wind-wave current induced resuspension, and (E) advection through submarine landslide induced resuspension. PCBs, polychlorinated biphenyls.
• Event 1 — the fiberbank is contaminated. (Note, however, that even if the fiber bank is not polluted, it poses an environmental problem due to the anaerobic and acidic conditions it creates.)
• Event 2 — the contaminants are dispersed from the fiberbank through one of the following dispersion pathways:
∘ Event 2A — chemical diffusion (Figure 7A)
∘ Event 2B — advection through ship (propeller) current induced resuspension (Figure 7B)
∘ Event 2C — advection through river flow current induced resuspension (Figure 7C)
∘ Event 2D — advection through wind-wave current induced resuspension (Figure 7D)
∘ Event 2E — advection through submarine landslide induced resuspension (Figure 7E)
• Event 3 — the dispersed contaminants in the water reach concentrations that exceed relevant contaminant impact thresholds.
If the probability of event 1 is equal to 1.0 (100%), the probability of exceeding a relevant contaminant threshold can be estimated by combining event 2 and 3. Here, event 2 concerns dispersion through five separate dispersion pathways and exceedance is estimated for each of them. Event 2 is expressed as PX in Eq. 1 and event 3 is expressed as P[FX > IT] in Eq. 1, further explained below.
Assessing the likelihood of contaminant dispersion thus consists of two parts: (1) assessing the likelihood that each of the five dispersion pathways in Figures 7A–E will occur within a given time; and (2) assessing the likelihood that one or several dispersion pathways will result in contaminant dispersal above a certain impact threshold considered likely to have negative impact on the environment. A general formula for assessing the probability of impact follows Göransson et al. (2014), Göransson et al. (2018) and is
where P is the probability, PI,X is the probability of impact (I) for a particular dispersion event (X), PX is the probability that this particular event will occur within a given time (here set to 1 year), FX is the flux of contaminants from such an event if it occurs, and IT is the impact threshold. For each of dispersion event, a method to calculate Px and Fx is determined. For the calculation of Fx, parameters are designated a probability distribution to account for uncertainties in the parameter values. Fluxes are presented in the unit of mg m–2 year–1.
In our study, we measured diffusion (Figure 7A) in the field using BFCs (Dahlberg et al., 2021). For other pathways (Figures 7B–E), we must rely on mathematical calculations based on sediment and contaminant characteristics measured in the field or using field samples, and empirical relationships found in the literature. For the specific dispersion pathway, X is here replaced by D for diffusion, S for ship (propeller) current induced resuspension, Q for river flow current induced resuspension, WC for wind-wave current induced resuspension, and L for submarine landslide-induced resuspension.
Chemical Diffusion (D)
The probability of diffusion, PD, is assumed equal to one because diffusion occurs more or less continuously (Miljødirektoratet, 2015). The flux FD can be calculated by using Fick’s law (see for example Schnoor, 1996; Miljødirektoratet, 2015), but in our case, FD was measured in the field using benthic flux chamber deployed at the surface of the fiberbank (Dahlberg et al., 2021). The contaminant flux was calculated as (i.e., Dahlberg et al., 2021),
where MSPMD is the amount (ng) of the target compound accumulated in the SPMD in the BFC and Ased is the sediment surface area (m2) covered by the chamber and tdeployment is the sampling time (days), then recalculated to represent the yearly flux and presented in the unit of mg m–2 year–1.
Ship (Propeller) Current Induced Resuspension (S)
According to Miljødirektoratet (2015), sediments located at water depths shallower than about 20 m can be dispersed as a result of propeller swirling. The water depth at possible ship impact area varies between 10 and 16 m. A conservative assumption is thus that all resuspended sediment will be redistributed outside the fiberbank and PS equals 1 for all calls. The formula by Miljødirektoratet (2015) was used to calculate the advective contaminant flux from resuspended fibers caused by the ship traffic (FS),
where FS is in mg m–2 year–1, 2Ns is the yearly number of vessel calls to the site where 2 accounts for the arrival and the departure, msed is the amount of resuspended fine fraction of sediment (here fibers) from each vessel call (kg), Csed is the contaminant concentration in the sediment (mg kg–1), fdiss is the dissolved fraction of the contaminant content in the sediment and that can become dissolved after resuspension (Miljødirektoratet, 2015), fsusp is the suspended fraction of the contaminant content in the sediment (i.e., proportion < 2 μm for minerogenic sediments; Miljødirektoratet, 2015), As is the sediment area (m2) affected by ship traffic. According to Miljødirektoratet (2015), msed can be calculated by,
where hs is the average water depth (m) at the ship impacted area, dp is the propeller depth (m), Bs is the ship width (m), ffine is the fraction fine sediment < 63 μm, and Tr is the ship trajectory length (m).
River Flow Current Induced Resuspension (Q)
PQ is the probability of erosive river flows (i.e., flow velocities) and it is calculated by estimating the probability that river flow will induce a bed shear stress greater than the critical shear stress for fiber particle mobility. Based on frequency analysis, recurrence interval for critical discharges was calculated according to (see for example Maidment, 1993),
that describes the probability that a certain event (X) will exceed a certain level (xT) once in N years, where T is the return period for the specific event (n) during the recorded time (t).
If we assume that the sedimentation rate is sufficiently low and that the resuspended fibers/fiber particles do not settle until they reach calm condition where the flow and wave action is very low (i.e. deposition flux occurs outside the fiberbank area), then the settling velocity above the deposit can be neglected. The advection flux from river current induced resuspension (FQ) can then be written,
where FQ is in mg m–2 year–1, E is the sediment erosion rate (kg m–2 s–1), or sediment erosion flux, generated by river flow, tQ is the yearly time duration with erosive flows, if such flows occur (s). The sediment erosion rate (E) can be written as Patheniades (1965), Kandiah (1974), and Ariathurai and Arulananda (1978),
where E0 is the erodibility coefficient (kg m–2 s–1), τ0 is the average shear stress (N m–2) created by the flowing fluid (water) and τc is the critical shear stress (N m–2) for particle mobility. Hanson and Simon (2001) suggested that E0 can be calculated by,
where τc is the critical sediment shear stress for mobility (N m–2).
Wind-Wave Current Induced Resuspension (WC)
Waves can disturb sediment and currents can transport it. Wavelength relative to undisturbed water depth determines situations of shallow water wave, intermediate wave, or deep-water wave induced sediment mobilization (Bridge and Demicco, 2008). For simplicity, we assume that linear wave theory can be applied, and that refraction and diffraction can be neglected. The surface of the contaminated deposit is assumed smooth (i.e., no ripples) which is consistent with our field observations. It is further assumed that the mean bed shear stress is in the direction of the combined velocity. Average bed shear stress from waves and currents was calculated using the derived formulas and procedure presented in Soulsby and Clarke (2005). The formulas include bed shear-stresses (τwc) for laminar, smooth-turbulent and rough-turbulent wave-plus-current flows. The flow regime was estimated based on the calculation of current Reynolds Number (Rec) and wave Reynolds Number (Re).
We used the approach for river flow induced resuspension to calculate the recurrence interval for critical wind-waves. The advection flux from wind-wave induced erosion (FWC) can then be written (modified after Miljødirektoratet, 2015),
where FWC is in mg m–2 year–1, EWC is the erosion rate for wind-wave generated erosion (kg m–2 s–1), Csed is the sediment concentration (mg kg–1), fdiss is the dissolved fraction, fsusp is the suspended fraction and tWC is the yearly duration (s) with erosive wind-wave currents, if wind-wave driven currents occur. The yearly duration for on shore critical winds (critical direction and critical wind speed) was assessed using open data from the Swedish Meteorological and Hydrological Institute (SMHI). EWC can then be calculated by the same formula as in Eq. 7, but τ0 is the bed shear stress from waves and currents (N/m2), denoted τwc.
Submarine Landslide Induced Resuspension (L)
A specific landslide does not occur at the same place twice (within the timescale considered in this study) and, therefore, frequency analysis becomes difficult to apply. According to L’Heureux (2009) and L’Heureux et al. (2010), nearshore landslides often occur within a period of unfavorable stability such as during low tide (tides are not relevant for the Baltic Sea) or following a period of heavy rainfall that successively builds up a pore pressure in the soil and sediment. The sediment pore pressure thus depends on the precipitation that falls over land and infiltrates into the ground.
A method to calculate submarine landslide probability was developed based on Persson (2008), Berggren et al. (2011), and Göransson et al. (2014) and is briefly described here. First, landslide probability is determined for non-transient condition and only with respect to knowledge uncertainty. The stability of a slope can be calculated using SLOPE/W, or a similar tool, and the evaluation of the probability of failure using the point estimation method (see for example Rosenblueth, 1975, 1981; Odén et al., 2017). Knowledge uncertainty is then assessed for the most crucial/selected parameters, in this case undrained shear strength, friction angle, bulk density and level of permeable layer. Second, natural variations of high pore water pressures are evaluated. Here, we used a modification of the HBV-model (Persson, 2008) developed by the Swedish Meteorological and Hydrological Institute (SMHI), to analyze variation in groundwater levels (variation in precipitation as input to pore water pressures). Third, landslide probability is calculated including variations in pore water pressure. Fourth, annual landslide probability (PL), or for an arbitrary time, is determined (see further in Supplementary Material).
The advection flux from a submarine landslide (FL) can then be written as,
where FL is the contaminant flux triggered by landslide (mg m–2 year–1), Msed is the amount (kg) contaminated sediment involved in a landslide (volume⋅density). AL is the area (m2) involved in a slide. Msed can be estimated by assessing the surficial extent of a slide times the depth and density of the fiber bank deposit,
where AL is the area of contaminated sediment involved in a potential landslide/mud flow (m2), BL is the depth of the contaminated deposit that is involved in a potential landslide/mudflow (m), and ρFB is the bulk density (kg m–3). The surficial landslide extent (AL) can for example be estimated based on landslide scars detected by bathymetric surveys. Density was estimated based on laboratory testing.
Determination of Environmental Impact Threshold (IT)
In absence of guiding data for Σ7PCB fluxes from sediments, a reasonable impact threshold had to be selected. In this study, the impact threshold is based on measured fluxes from a reference site and normalized for the area. The reference site is located in Bollsta Bay near the study site: X-coordinate: 638505.00, Y-coordinate: 6986179.00 in the Swedish Reference Frame 1999 (Transverse Mercator). This location is the same as site FV3 in Dahlberg et al. (2021). Measured Σ7PCB flux amounted to 0.000013 mg m–2 year–1 (Dahlberg et al., 2021) and an impact threshold 10 times this value was initially chosen, i.e., IT = 0.00013 mg m–2 year–1. This threshold is an assumption and it is possible that that the background level harms the environment. We do not have data to be able to make a more rigorous assessment at this stage, but the chosen value can be seen as an approach to illustrate the methodology. We elaborate how this threshold value for flux relates to the Norwegian guideline values for the concentration of PCBs in surface waters in the discussion section.
Input Parameters and Probability Distributions
Assumptions made for the site-specific calculations of PX are shown in Supplementary Table 1. Input parameters and probability distributions for the calculation of FX (Eq.1) are shown in Supplementary Tables 2, 3. Monte Carlo simulations (10,000 trials per run) were used to calculate PX[Fx > IT] using the Crystal Ball add-in to Excel. The likelihood of exceeding the impact threshold was then calculated by multiplying PX with FX.
Possible Contribution to the Load
Once the above data are in place, the analysis can be developed to estimate the possible load of Σ7PCB and the amount of resuspended fiber particles from each of the dispersion pathways (Table 1).

Table 1. Calculation of possible Σ7PCB loads and amount resuspended fiberbank particles generated by each of the dispersion pathways.
Sensitivity Analysis
A sensitivity analysis provide information on which input parameters that influence the output the most. In Crystal Ball, sensitivity is calculated by computing Spearman’s rank correlation between every assumption and forecast, which is done simultaneously while the simulation is running. A sensitivity chart is produced for each forecast and it ranks the assumptions from the most important down to the least important in the model.
Results
The yearly probabilities of exceedance for the dispersion pathways, including both the probability of dispersion occurring and the probability of dispersion resulting in exceedance of the impact threshold, if it occurs, are shown in Table 2. Given the impact threshold, the yearly probability of exceedance is high for diffusion (100%) and advection of Σ7PCB fluxes triggered by ship-induced resuspension (100%). The yearly probabilities of failure of Σ7PCB fluxes through advection triggered by river flow and wind-wave currents are almost equal (18 and 19%, respectively), while submarine landslide poses the lowest risk (5%). If the time period increases from 1 to 10 years, the probability of dispersion rises to 86% for river flow, 89% for wind-wave currents, and 18% for submarine landslides, which also increases the overall probability of exceeding the impact threshold.
Given the dispersion, Monte Carlo simulated 50 and 90% percentiles, respectively, confidence levels, of Σ7PCB flux (mg m–2 year–1), Σ7PCB load (g year–1) and particle load from each dispersion pathways are shown in Table 3. These results indicate that, for example, 90% of the Σ7PCB flux from ship current induced resuspension is 0.60 mg m–2 year–1 or lower. However, there is a 90% confidence that the yearly Σ7PCB from ship current induced resuspension probably will lie somewhere between 0.11 and 0.74 mg m–2 year–1. The results (Table 3) further indicate that chemical diffusion will contribute least to the overall dispersion of Σ7PCB from the fiberbank deposit, followed by river flow, wind wave and ship current induced resuspension of Σ7PCB, in that order. A submarine landslide will release far most Σ7PCB and fiber particles. The estimated confidence intervals reflect the uncertainties in input parameters and hence, the amount that might be dispered in case of an event.
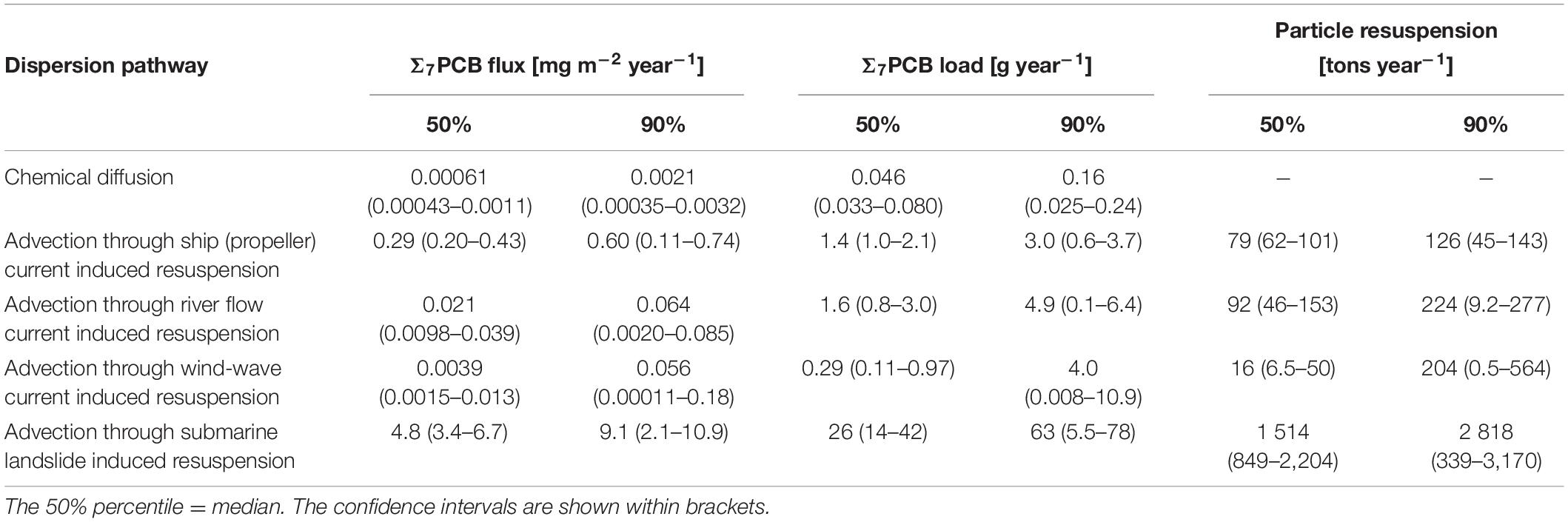
Table 3. Calculated 50 and 90% percentiles and the 50 and 90% confidence intervals for Σ7PCB flux, Σ7PCB load, and particle resuspension, given dispersion from the fiberbank.
The MC simulated fluxes and loads (Table 3) were compared to calculated fluxes and loads (Supplementary Table 4) for each of the dispersion pathways by using fixed mean and maximum data in Supplementary Table 2 (except for Manning’s M for which minimum value should be used to yield higher shear stress). Calculated results based on fixed data showed in principle a greater amount of dispersion than if uncertainties are taken into account.
The fiberbank may amount to about 19,000 tons of matter (Supplementary Table 4) or even more if the true values are closer to the maximum than the average. Given the fact that the fiberbank is still there 40 years after the discharges forming it had ceased, it is possible that parts of the resuspended fibers resettle on the fiberbank, except for submarine landslide induced resuspension. However, the formation of fiber-rich sediments outside the fiberbank indicates that resuspension and redeposition of fibers has occurred.
The result of the sensitivity analysis, with the most influential parameters for the outputs, is shown in Table 4. Positive coefficients indicate that an increase in the assumption is associated with an increase in the forecast. Negative coefficients imply the opposite situation. The larger the absolute value of the correlation coefficient, the greater the sensitivity. For example Σ7PCB fluxes from ship and landslide induced resuspension of fiber particles are governed by the Σ7PCB concentration in the sediment, which means that the higher the concentration in sediment, the higher the fluxes. Σ7PCB fluxes from river flow induced resuspension is governed by duration of erosive discharges, the more of then such discharges occur, the more Σ7PCB are dispersed. Water depth governs the Σ7PCB fluxes from wind wave induced resuspension and the deeper the water, the lesser the fluxes.
By reducing the uncertainties in the most influential parameters, the uncertainties in the output are consequently reduced. From Table 4, further surveys should primarily focus on reducing uncertainties for contaminant concentration in the sediments (Csed), water depths (h, hs), estimation of concerned fiberbank area potentially involved in a submarine landslide (AL), time duration of erosive currents (tQ, tWC), measured diffusion (FD) and trajectory length (Tr). Given the known uncertainties, the Monte Carlo simulations further indicate that the most influential parameters to the total yearly load of Σ7PCB (not shown in Table 4), if all dispersion pathways are used, are the fiberbank area (AL) involved in a potential submarine landslide (50%) and the contaminant concentration (Csed) in the fiberbank (40%).
The Monte Carlo computation also include fixed values for calculated basic parameters, such as critical shear stress and the erosion rate. The erosion rate depends on the erodibility coefficient, shear stresses and critical shear stress. The lower the erodibility coefficient, the lower the erosion rate. The more the shear stress exceeds the critical level, the greater the erosion. The magnitude of the impact of these parameters on the outcome is not apparent from the sensitivity analysis but are important for the output. For example, we needed to reduce the erosion coefficient to generate realistic results for fiber particle resuspension.
Discussion
It should be emphasized that the data base for each parameter is extremely small, from single datum points to a few datum points. This means a very limited statistical basis and that the uncertainties about the “true” values are great. We could have calculated the dispersion solely on the basis of known data, but in order to make the uncertainties visible and how these uncertainties can affect the outcome, we chose a probability-based method. The choice of method can of course be discussed, but an advantage is that the results can be used to justify why further investigations and modeling is needed and what these should be focused on. By making the prevailing uncertainties visible, more robust decisions can be made.
There are still many uncertainties in the flux of contaminants at the sediment/water interface and the processes that affect its variation in space and time. Uncertainties refer to knowledge uncertainty, or epistemic uncertainty, and uncertainty in the natural variation, so called aleatory uncertainty or genuine uncertainty. For example, Howell and Rifai (2015) showed a variation in PCB fluxes due to storm surges and flooding, and Dahlberg et al. (2021) showed that particle resuspension may occur even at gentle disturbance of the sediment surface. For a better understanding, measurements and analyzes performed under different environmental, seasonal and hydrological conditions are still needed.
The Applied Method
The method presented in this paper has been applied on contaminated, organic (cellulose) rich sediments of anthropogenic origin but can also be applied to contaminated sediments in general. The method aims to support risk assessment by estimating the risk of dispersion, considering, but not limited to, dispersion through chemical diffusion and advection induced by particle resuspension. We have chosen to use a probabilistic approach, as a complement to deterministic risk assessment, to consider systematic and statistical uncertainties with the aim to assess the likelihood of pollution spread and its likelihood for environmental impact. However, one difficulty with such an approach is the quantification of the overall uncertainty due to a forward propagation of uncertainties, as well as model and parameter uncertainties (e.g., Gouldby et al., 2010). On the other hand, a probabilistic method may reveal uncertainties that otherwise can be hidden if only a deterministic analysis is applied (Arabi et al., 2007; Qu et al., 2016). As such, a probabilistic method may be more rigorous, for example by displaying percentiles and confidence intervals that are more precise (Qu et al., 2016) and considering different environmental conditions, as shown by Shojaeezadeh et al. (2020). Although the confidence intervals here can be considered large, they give an understanding of possible best- and worst-case scenario for the different continuous, periodic or rare occurring dispersion pathways based on the available knowledge. For example, by comparing the Monte Carlo simulated fluxes and loads (Table 3) with calculated fluxes and loads for each of the dispersion pathways by using fixed mean and maximum values (except for Manning’s M for which the minimum value should be used to yield higher shear stress) (Supplementary Table 4), we found a discrepancy for river flow current, wind wave current, and submarine landslide induced dispersion. In the case of limited data, calculations based on individual values may therefor give misleading results.
Using a probabilistic approach helps to understand where the uncertainties lie and identify parameters for which uncertainty needs to be reduced to better estimate the risk. Such information can form a basis for the design of supplementary field studies and laboratory analyzes.
The method presented in this study provides information on whether or not dispersion occurs, but does not predict where the dispersed contaminants and particles will end up. For such an assessment, a numerical model is needed. Toxicological screening and modeling could also be included to assess the impact on the ecosystem being exposed. Thus, the accuracy to which the risk can be estimated or assessed with the suggested method depends on how well the threshold level for environmental impact can be described. Nevertheless, a probabilistic method can be used to confirm the validity of a deterministic risk assessment, if such exists (Shojaeezadeh et al., 2020).
In our study, the computational model was set up in a spread sheet tool, supported by Monte Carlo simulation, which makes it easy to update the (mathematical) analytical solutions when new knowledge become available, if governing equations need to be changed, and if additional dispersion pathways need to be added. Also, Monte Carlos simulations assume variables to be independent. However, some of the uncertain independent variables may not be truly independent. For example, diffusion depends on concentration gradient, wavelength depends on wind speed and fetch, near bed flow velocity depends on discharge and bed geometry. When more data are available, one could choose assumptions to correlate in a correlation matrix with correlation coefficients as input to the sensitivity analysis.
The Case Study
Based on the results, it can be concluded that Σ7PCB are dispersed and that fiberbank particles are resuspended from the studied fiberbank. The results clearly show that the rare occurrence of extreme events will have a relatively large impact on the amount dispersed, which indicates that the fiberbank cannot be considered stable with respect to contaminant and particle dispersion. The results further indicate that the fiberbank will likely continue to negatively affect the surrounding ecosystem if nothing is done to prevent dispersion. This outcome is probably also true for many other fiberbank deposits, and for contaminated sediments located in shallow waters (e.g., Butcher and Garvey, 2004; Davis et al., 2007; Howell and Rifai, 2015). Even archived contaminants may become exposed to dispersion because of sediment scouring during extreme weather-events like hurricanes (Dellapenna et al., 2020). Our study also includes the potential for contaminant dispersion from submarine landslides, and the results indicate that a submarine landslide will disperse most contaminants per unit area (i.e., flux), followed by advection through ship induced resuspension, river flow resuspension, wind-wave current resuspension, and least by chemical diffusion.
We applied a threshold level for Σ7PCB sediment-to-water flux to estimate the probability for environmental impact. Such an approach was also applied by Shojaeezadeh et al. (2020). However, in our study, this threshold is related to measured Σ7PCB fluxes at a reference site because no Swedish environmental quality standard exists for PCBs in water. The threshold level thus simply reflects what the situation is in a less contaminated part of the area, but it does not give information on potential consequences to the environment or the recipient, which would have been the better threshold level. The Norwegian Miljødirektoratet has set a guideline value for a yearly average of Σ7PCB in coastal waters to 2.4E-06 μg L–1 (Miljødirektoratet, 2016). Sediment-to-water fluxes can be recalculated to an approximate water concentration if the representative water volume and the water residence time is known. For the Bollsta Bay, the water volume is about 0.11 km3 (SMHI, 2021a) and the residence time is roughly 1 year, depending on water depth (Heinemo, 1999). In our case study, we chose a threshold level of 0.00013 mg m–2 year–1, which roughly would correspond to 4.9E-06 μg L–1. Thus, the suggested threshold level is about two times higher than the Norwegian guideline, but within the same order of magnitude, which means that the selected threshold value is not unreasonable. The water volume above Väja fiberbank, not the entire Bollsta Bay, is roughly 1 million m3 and with the same residence time, the measured yearly fluxes of chemical diffusion from the fiberbank (0.0011 mg m–2 year–1) would roughly correspond to a concentration of 7.7E-05 μg L–1, which is about 30 times higher than the Norwegian guideline value. Calculated fluxes from the other dispersion pathways are even higher than that. To conclude, this means that the estimated risk for our case study is an underestimation rather than an overestimation, even considering the lower levels of the 90% confidence intervals. It also confirms the importance of taking into account advective dispersion pathways in risk assessments (e.g., Erickson et al., 2005; Davis et al., 2007; Reible, 2014; Howell and Rifai, 2015; Dellapenna et al., 2020).
The uncertainty in input parameter values can be reduced with more precise laboratory and field measurements. We used benthic flux chambers (presented in Dahlberg et al., 2021) to measure chemical diffusion, however, we cannot be sure that we only measure diffusion with the BFCs because the advection can also be influenced by e.g., bioturbating benthic organisms. We did not have the possibility to measure all the parameters needed for the calculations, partly because existing field and laboratory equipment are not designed for the atypical material that the fiberbanks consist of. Thus, several parameter values had to be estimated based on literature and the knowledge gained so far about this anthropogenic sediment. There are some parameters that were more challenging to assess. For example, the high organic cellulose fiber content and their characteristics made it difficult to assess parameters like critical shear stresses, grain size distribution and bed surface roughness, which are parameters commonly included in formulas for sediment transport. It was also challenging to conduct proper geotechnical measurements of the fiberbank, for example to collect undisturbed core samples of the soft unconsolidated fibrous sediment and the underlying strata (Löfroth et al., 2021). The varves (thin silt and clay layers) in the underlying soft clay made trimming of specimens for laboratory tests challenging (Löfroth et al., 2021). Flow and current velocity profiles would have provided better input to calculate shear stresses. The optimal would have been to have access to measurement data showing current directions and current velocities, calibrated against prevailing flows, winds and ocean currents, and then to establish a hydrodynamic model across different scenarios.
It has also been shown that resuspension of fiberbank materials and fiber-rich sediments may be induced by only gentle disturbance at the site (Dahlberg et al., 2021), which should be considered in future work. Gas production (methane gas from anaerobic decomposition of organic matter) can also be high in fiberbanks (Lehoux et al., 2021), and gas ebullition may perhaps enhance contaminant dispersal in fiberbanks through increased sediment-pore water exchange (Klein, 2006) and through resuspension of contaminated particles (Yuan et al., 2007; Viana et al., 2012). Gas formation can cause parts of the fiberbank to be boyant and float away. Furthermore, the geological setting at a site may provide conditions for advection through upwelling submarine groundwater. In our case study, the upwelling of submarine groundwater was not assumed an issue because the permeable thin silt layer is not in contact with the contaminated sediment layers. The observed pockmarks can indicate submarine groundwater seepage but are more likely caused by gas ebullition.
In the middle and northern parts of Sweden, the effect of climate change includes increased precipitation and milder winters, which in turn will cause more precipitation to fall as rain instead of snow. A climate analysis for the county shows that the run-off to the rivers in the area increases as the climate changes (SMHI, 2021b). Thus, the flow in the Ångermanälven river is likely to increase, which may affect the flow-induced resuspension of fiber particles. However, the Ångermanälven river discharge is strongly regulated by several hydropower stations, and an ongoing landslide risk investigation of the Ångermanälven river valley conducted by SGI has shown that the flow control obscures the effect of climate change on river flow. Nevertheless, increased rainfall will affect pore water pressure and hence landslide probability. It is not currently known whether climate change will cause stronger and/or more frequent storms in the area, but if these occur, wind-wave currents will pose a greater problem than today for the dispersion of contaminants and fiber particles. Other studies have also shown that sediment-to-water dispersion of PCBs increases during hurricanes (Howell and Rifai, 2015; Dellapenna et al., 2020).
The case study site is located in a part of Sweden that currently experiences isostatic rebound of the earth’s crust of 8 mm year–1 in response to the last glaciation more than 10,000 years ago, thus resulting in a gradual seaward displacement of the shoreline. This means that the water depth over the fiberbank is slowly decreasing, eventually exposing the fiberbank more to currents from both vessels, discharge and waves. However, this situation may change over time due to sea level rise, and it is possible that the rate of sea level rise may exceed the isostatic rebound rate in about 100 years (personal communication, L. Johansson, SMHI), but by then, the sediments may already have been redistributed over the seabed. In addition, isostatic rebound and other tectonic movements of the earth’s crust cause minor earthquakes, but so far the highest measured magnitude in the area is 3.1 on the Richter scale1 and it has been considered that these magnitudes are not enough to trigger a landslide in the area (Löfroth et al., 2021).
The scientific knowledge on the characteristics of the cellulose fibers and wood chips deposits in the environment is in its infancy. The fiberbank deposits will be difficult and costly to remediate due mainly to high organic content, high water content, very low density, and the large volumes (Jersak et al., 2016a,b). It may also be difficult to determine who is legally responsible for the costs and implementation of the action in accordance with the Polluter Pays Principle (PPP). Because not all sites can be remediated at once, we need to understand the impact from these deposits on the ecosystem in order to prioritize sites for remediation. We need to understand more about the different dispersion pathways from the fiberbanks to the surrounding recipient and about their different significance for the spread of these pollutants. This can lead to an understanding of the fiberbanks’ impact on the ecosystem and in turn how we address fiberbanks to minimize their environmental impact. The approach presented here takes uncertainties into account and can be used as an initial assessment of whether dispersion can be a problem, which dispersion pathway probably contributes most, and in the dispersions order of magnitude.
Conclusion
We have proposed a probabilistic approach to estimate the probability of dispersion of pollutants from contaminated sediment and to estimate the likelihood that such dispersal may have negative environmental impact. The approach takes use of uncertainties and is meant to be part of a risk assessment. The approach was tested for a case study consisting of contaminated cellulose fibrous sediment originating from unregulated discharge of pulp waste from a pulp factory (referred to as fiberbanks). Although the data set is extensive, within this context it has limitations. Despite, the approach allowed for the assessment of possible contaminant dispersion from this type of contaminated sediments and for the estimation whether dispersion may pose a risk to the environment or not. The approach enabled the assessment of dispersion paths that may govern the risk.
It can be concluded that the fiberbank studied is not stable with respect to the dispersion of contaminants as well as contaminated sediment particles. The result indicates that there is a 100% probability that ship induced resuspension and chemical diffusion will disperse Σ7PCB above a limit of failure, respectively. The probability is just below 20% for river flow, respectively, wind-wave current induced dispersion, and about 5% for landslide induced dispersion. These probabilities are governed by the probability for dispersion. If dispersion occurs, then all dispersion paths will generate a release of Σ7PCB above the threshold level that was set to assess negative environmental impact. With regards to the specific case study, it can also be concluded that as long as the thick cellulose rich and contaminated deposit is left unmanaged, it is likely that several of the global goals for sustainable development (specifically SDGs 3, 14 and 15) will not be met in the area.
Data Availability Statement
The raw data supporting the conclusions of this article will be made available by the authors, without undue reservation.
Author Contributions
GG was the main author and developed the conceptual and probabilistic approach. AA contributed to writing and organized the overall field works. A-KD contributed to writing and performed the chemical analyses of PCBs. HL contributed to writing, performed geotechnical investigations and analyses, and development of a method for landslide probability. SJ contributed to writing and field measurement of PCBs. KW contributed to the choice of contaminant threshold values and writing. PF-K contributed to writing and field work. PN performed the landslide probability calculations. JH contributed with geospatial data and GIS. IS contributed to writing and had the overall responsibility for the TREASURE project that this study contributed to. All authors contributed to conceptualization, manuscript revision, reading, revising, and approving the submitted version.
Funding
The study was part of the TREASURE (Targeting Emerging Contaminated Sediments Along the Uplifting Northern Baltic Coast of Sweden for Remediation) research project that was run between 2014 and 2018. TREASURE was financed within the National Development Program TUFFO by the Swedish Geotechnical Institute and The Swedish Research Council for Environment, Agricultural Sciences and Spatial Planning (FORMAS, grant number 214-2014-63). The TREASURE research project was a collaboration between Uppsala University (lead), the Swedish Geotechnical Institute (SGI), the Geological Survey of Sweden (SGU), the Swedish University of Agricultural Sciences (SLU), Stockholm University, Lund University and the Center for Marine Environmental Sciences at the University of Bremen, Germany. TREASURE was co-funded by all project members.
Conflict of Interest
The authors declare that the research was conducted in the absence of any commercial or financial relationships that could be construed as a potential conflict of interest.
Publisher’s Note
All claims expressed in this article are solely those of the authors and do not necessarily represent those of their affiliated organizations, or those of the publisher, the editors and the reviewers. Any product that may be evaluated in this article, or claim that may be made by its manufacturer, is not guaranteed or endorsed by the publisher.
Acknowledgments
We would like to thank all funders. We also would like to thank Espen Eek at the Norwegian Geotechnical Institute (NGI) for providing the benthic flux chambers (BFCs), and local resident Rolf Nilsson for helping us to retrieve the BFCs after measurements were completed. We also thank Emeritus Claes Alén, Chalmers University of Technology, that helped us develop a model to calculate submarine landslide probability. Finally, we would like to thank the reviewers who helped improve the article.
Supplementary Material
The Supplementary Material for this article can be found online at: https://www.frontiersin.org/articles/10.3389/fmars.2021.729243/full#supplementary-material
Footnotes
References
Apitz, A., Elliott, M., Fountain, M., and Galloway, T. (2006). European environmental management: moving to an ecosystem approach. Int. Environ. Asses. Manag. 2, 80–85. doi: 10.1002/ieam.5630020114
Apler, A., Nyberg, J., Jönsson, K., Hedlund, I., Heinemo, S. -Å, and Kjellin, B. (2014). Kartläggning Av Fiberhaltiga Sediment Längs Västernorrlands Kust. SGU-Repport 2014:16. Geological Survey of Sweden.
Apler, A., Snowball, I., Frogner-kockum, P., and Josefsson, S. (2019). Distribution and dispersal of met-als in contaminated fibrous sediments of industrial origin. Chemosphere 215, 470–481. doi: 10.1016/j.chemosphere.2018.10.010
Apler, A., Snowball, I., and Josefsson, S. (2020). Dispersal of cellulose fibers and metals from contami-nated sediments of industrial origin in an estuary. Environ. Pollut. 266:115182. doi: 10.1016/j.envpol.2020.115182
Arabi, M., Govindaraju, R. S., and Hantush, M. M. (2007). A probabilistic approach for analysis of un-certainty in the evaluation of watershed management practices. J. Hydrol. 333, 459–471. doi: 10.1016/j.jhydrol.2006.09.012
Ariathurai, R., and Arulananda, K. (1978). Erosion rates of cohesive soils. J. Hydr. Div. 104, 279–283. doi: 10.1061/jyceaj.0004937
Berggren, B., Alén, C., Bengtsson, P. E., and Falemo, S. (2011). Methodology for Assessment of Land-Slides Probability: Quantitative Model. Linköping: Swedish Geotechnical Institute.
Bridge, J., and Demicco, R. (2008). Earth Surface Processes, Landforms and Sediment Deposits. Cambridge: Cambridge University Press.
Burton, P. J., Messier, C., and Smith, D. W. (2003). Towards Sustainable Management of the Boreal Forest. Ottawa: NRC Research Press, 1039.
Butcher, J. B., and Garvey, E. A. (2004). PCB loading from sediment in the hudson river: congener signature analysis of pathways. Environ. Sci. Technol. 38, 3232–3238. doi: 10.1021/es035453t
Dahlberg, A.-K., Apler, A., Frogner-Kockum, P., Göransson, G., Snowball, I., Wiberg, K., et al. (2021). Dispersal of persistent organic pollutants from fiber-contaminated sediments: biotic and abiotic pathways. J. Soils Sediments 21, 1852–1865. doi: 10.1007/s11368-020-02871-1
Dahlberg, A.-K., Apler, A., Vogel, L., Wiberg, K., and Josefsson, S. (2020). Persistent organic pollu-tants in wood fiber-contaminated sediments from the Baltic Sea. J. Soils Sediments 20, 2471–2483. doi: 10.1007/s11368-020-02610-6
Davis, J. A., Hetzel, F., Oram, J. J., and McKee, L. J. (2007). Polychlorinated biphenyls (PCBs) in san francisco bay. Environ. Res. 105, 67–86. doi: 10.1016/j.envres.2007.01.013
Dellapenna, T., Hoelscher, C., Hill, L., Al Mukaimi, M., and Knap, A. (2020). How tropical cyclone flooding caused erosion and dispersal of mercury-contaminated sediment in an urban estuary: the impact of hurricane harvey on buffalo bayou and the san jacinto estuary, galveston bay. Sci. Total Environ. 748:141226. doi: 10.1016/j.scitotenv.2020.141226
Eek, E., Cornelissen, G., and Breedveld, G. D. (2010). Field measurement of diffusional mass transfer of HOCs at the sediment-water interface. Environ. Sci. Technol. 44, 6752–6759. doi: 10.1021/es100818w
Erickson, M. J., Turner, C. L., and Thibodeaux, L. J. (2005). Field observation and modeling of dissolved fraction sediment-water exchange coefficients for PCBs in the hudson river. Environ. Sci. Technol. 39, 549–556. doi: 10.1021/es034520g
Förstner, U., and Westrich, B. (2005). BMBF coordinated research project SEDYMO (2002-2006)sediment dynamics and pollutant mobility in river basins. J. Soils Sediments 5, 134–138. doi: 10.1065/jss2005.08.002
Gerbersdorf, S. U., Hollert, H., Brinkmann, M., Wieprecht, S., Schüttrumpf, H., and Manz, W. (2011). Anthropogenic pollutants affect ecosystem services of freshwater sediments: the need for a “triad plus x” approach. J. Soils Sediments 11, 1099–1114. doi: 10.1007/s11368-011-0373-0
Gouldby, B. P., Syers, P. B., Panzeri, M. C., and Lanyon, J. E. (2010). Development and application of efficient methods for the forward propagation of epistemic uncertainty and sensitivity analysis within complex broad-scale flood risk system models. Can. J. Civil Eng. 37, 955–967. doi: 10.1139/L09-173
Göransson, G., Norrman, J., and Larson, M. (2018). Contaminated landslide runout deposits in riversmethod for estimating long-term ecological risks. Sci. Total Environ. 642, 553–566. doi: 10.1016/j.scitotenv.2018.06.078
Göransson, G., Norrman, J., Larson, M., Alén, C., and Rosén, L. (2014). A methodology for estimating risks associated with landslide of contaminated soil into rivers. Sci. Total Environ. 472, 481–495. doi: 10.1016/j.scitotenv.2013.11.013
Haag, I., Kern, U., and Westrich, B. (2001). Erosion investigation and sediment quality measurements for a comprehensive risk assessment of contaminated aquatic sediments. Sci. Total Environ. 266, 249–257. doi: 10.1016/S0048-9697(00)00753-1
Hall, E. R. (2003). “Forest industry aqueous effluents and the aquatic environment,” in Towards Sustainable Management of the Bo-real Forest, eds P. J. Burtons, C. Messier, D. W. Smith, and W. L. Adamowicz (Ottawa: NRC Research Press), 669–712. doi: 10.1139/9780660187624
Hanson, G. J., and Simon, A. (2001). Erodibility of cohesive streambeds in the loess area of the mid-western USA. Hydrol. Proc. 15, 23–38. doi: 10.1002/hyp.149
Heinemo, S. -Å (1999). Kan Ångermanfjorden Ta Tillbaka Livet På Bottnarna? Havet.nu. Available online at https://www.havet.nu/dokument/Bv1999angermanfjorden.pdf. (accessed April 25, 2020)
Hoffman, E., Guernsey, J. R., Walker, T. R., Kim, J. S., Sherren, K., and Andreou, P. (2017). Pilot study investigating ambient air toxics emissions near a Canadian kraft pulp and paper facility in pictou county, nova scotia. Environ. Sci. Pollut. Res. 24, 20685–20698. doi: 10.1007/s11356-017-9719-5
Howell, N., and Rifai, H. (2015). Longitudinal estimates of sediment-water diffusive flux of PCB con-geners in the houston ship channel. Estuarine Coastal Shelf Sci. 164, 19–27. doi: 10.1016/j.ecss.2015.06.024
Jerkeman, P., and Norrström, H. (2018). Historien Om Skogsindustrins Miljöarbete - Vägen Mot Hållbar-Het. Skogsindustrierna. ISBN 9789198360134.
Jersak, J., Göransson, G., Ohlsson, Y., Larsson, L., Flyhammar, P., and Lindh, P. (2016a). In-Situ Capping of Contaminated Sediments. Sediment Remediation Technologies: A General Overview. SGI Publication 30-3E. Swedish Geotechnical Institute, Linköping. Available online at https://www.sgi.se/globalassets/publikationer/sgi-publikation/sgi-30-3e.pdf (accessed March 10, 2010).
Jersak, J., Göransson, G., Ohlsson, Y., Larsson, L., Flyhammar, P., and Lindh, P. (2016b). In-Situ Capping of Contaminated Sediments. In-Situ Capping of Sweden’s Fiberbank Sediments: A Unique Challenge. SGI Publication 30-5E, Swedish Geotechnical Institute, Linköping. Available onlin at https://www.sgi.se/globalassets/publikationer/sgi-publikation/sgi-p30-5e.pdf (accessed March 10, 2010).
Kandiah, A. (1974). Fundamental Aspects of Surface Erosion of Cohesive Soils, Ph. D, Thesis. California: University of California.
Kane, I. A., and Clare, M. A. (2019). Dispersion, accumulation, and the ultimate fate of microplastics in deep-marine environments: a review and future directions. Front. Earth Sci. 7:80. doi: 10.3389/feart.2019.00080
Kienle, C., Langer-Jaesrich, M., Baumberger, D., Hohmann, D., Santiago, S., Köhler, H.-R., et al. (2013). Integrated toxicity evaluation of a pulp deposit using organisms of different trophic levels. J. Soils Sediments 13, 1611–1625. doi: 10.1007/s11368-013-0733-z
Klein, S. (2006). Sediment porewater exchange and solute release during ebullition. Mari. Chem. 102, 60–71. doi: 10.1016/j.marchem.2005.09.014
Kokko, M., Koskue, V., and Rintala, J. (2018). Anaerobic digestion of 30-100-year-old boreal lake sedimented fibre from the pulp industry: extrapolating methane production potential to a practical scale. Water Res. 133, 218–226. doi: 10.1016/j.watres.2018.01.041
Krishnappan, B. G. (2000). In situ size distribution of suspended particles in the fraser river. J. Hydraul. Eng. 126, 561–569. doi: 10.1061/(ASCE)0733-94292000126:8(561)
Lamparski, A. (2016). Geotechnical Study of Fiber-Bank Core Samples From the Bothnian Sea. Ph. D, Thesis. Germany: Geosciences of the University of Bremen.
Lehoux, A., Isidorova, A., Colling, F., Koestel, J., Snowball, I., and Dahlberg, A.-K. (2021). Extreme gas production in anthropogenic fibrous sediments: an overlooked biogenic source of greenhouse gas emissions. Sci. Total Environ. 781:146772. doi: 10.1016/j.scitotenv.2021.146772
L’Heureux, J. S. (2009). Multidisciplinary Study of Shoreline Landslides: From Geological Develop-Ment to Geohazard Assessment in the Bay of Trondheim, Mid-Norway. Ph. D, Thesis. Trondheim: Norwegian University of Science and Technology, NTNU.
L’Heureux, J. S., Hansen, L., Longva, O., Emdal, A., and Grande, L. O. (2010). A multidisciplinary study of submarine landslides at the Nidelva fjord delta, Central Norway-implications for geohazard assessment. Norwegian J. Geol. 90, 1–20.
Löfroth, H., O’Regan, M., Snowball, I., Holmén, M., Kopf, A., Göransson, G., et al. (2021). Challenges in slope stability assessment of contaminated fibrous sediments along the northern Baltic coast of Sweden. Eng. Geol. 289:106190. doi: 10.1016/j.enggeo.2021.106190
Michelsen, T. C., Boatman, C. D., Norton, D., Ebbesmeyer, C. C., and Francisco, M. D. (1998). Transport of contaminants along the seattle waterfront: effects of vessel traffic and waterfront construction activities. Water Sci. Technol. 37, 9–15. doi: 10.1016/S0273-1223(98)00176-0
Parnish, M. J., and Mackay, D. (2020). Multimedia Environmental Models, 3rd Edn. Boca Raton: Imprint CRC Press.
Meddah, S., Saidane, A., Hadjel, M., and Hireche, O. (2015). Pollutant dispersion modeling in natural streams using the transmission line matrix method. Water 7, 4932–4950. doi: 10.3390/w7094932
Miljødirektoratet (2015). Guidelines for Risk Assessment of Contaminated Sediments. Available onlin at: https://www.miljodirektoratet.no/publikasjoner/2016/september-2016/veileder-for-risikovurdering-av-forurenset-sediment/ (accessed November 24, 2020).
Miljødirektoratet (2016). Grenseverdier for Klassifisering Av Vann, Sediment Og Biota - Quality Standards For Water, Sediment and Biota. Veileder. Available online at https://www.miljodirektoratet.no/globalassets/publikasjoner/M608/M608.pdf, (accessed January. 15, 2020)
Norrlin, J., Josefsson, S., Larsson, O., and Gottby, L. (2016). Kartläggning Och Riskklassning Av Fiberbankar i Norrland. SGU-Repport 2016:21. Geological Survey of Sweden.
Norrlin, J., and Josefsson, S. (2017). Förorenade Fibersediment i Svenska Hav Och Sjöar. SGU-Repport 2017-07. Geological Survey of Sweden.
Odén, K., Bergdahl, K., Löfroth, H., Göransson, G., Jönsson, Å, Kiilsgaard, R., et al. (2017). “Mapping of landslide risks in a changing climate-development of simplified methodology,” in Advances in Natural and Technological Hazards Research, eds V. Thakur, J. S. L’Heureux, and A. Locat (Springer), 571–580. doi: 10.1007/978-3-319-56487-6_49
Patheniades, E. (1965). Erosion and deposition of cohesive soils. J. Hydr. Div. Am. Soc. Civ. Eng. 91, 105–139. doi: 10.1061/jyceaj.0001165
Persson, H. (2008). Estimation of pore pressure levels in slope stability calculations: analyses and modelling of groundwater level fluctuations in confined aquifers along the Swedish west coast. Lic Department Civil Environ. Eng. Chalmers Univer. Technol. 2008:11.
Polovodova Asteman, I., Hanslik, D., and Nordberg, K. (2015). An almost completed pollution-recovery cycle reflected by sediment geochemistry and benthic foraminiferal assemblages in a Swedish–Norwegian Skagerrak fjord. Mar. Pollut. Bull. 95, 126–140. doi: 10.1016/j.marpolbul.2015.04.031
Poole, N. J., Parkes, R. J., and Wildish, D. J. (1977). Reaction of estuarine ecosystems to effluent from pulp and paper industry. Helgoländer Wissenschaftliche Meeresunter Suchungen 30, 622–632. doi: 10.1007/BF02207866
Prygiel, E., Superville, P.-J., Dumoulin, D., Lizon, F., Prygiel, J., and Billon, G. (2015). On biogeochemistry and water quality of river canals in Northern France subject to daily sediment resuspension due to intense boating activities. Environ. Pollut. 197, 295–308. doi: 10.1016/j.envpol.2014.11.022
Qu, C., Li, B., Wu, H., Wang, S., and Li, F. (2016). Probabilistic ecological risk assessment of heavy metals in sediments from China’s major aquatic bodies. Stoch. Environ. Res. Risk. Assess. 30, 271–282. doi: 10.1007/s00477-015-1087-4
Reible D. (ed.) (2014). “Processes, assessment and remediation of contaminated sediments,” in Serdp Estcp Environmental Remediation Technology, (Springer).
Roberts, D. A. (2012). Causes and ecological effects of resuspended contaminated sediments (RCS) in marine environments. Environ. Int. 40, 230–243. doi: 10.1016/j.envint.2011.11.013
Rosenblueth, E. (1975). Point estimation for probability moments. Proc. Natl. Acad. Sci. U.S.A. 72, 3812–3814. doi: 10.1073/pnas.72.10.3812
Schwarzenbach, R. P., Gschwend, P. M., and Imboden, D. M. (1993). Environmental Organic Chemistry. New York: John Wiley & sons, INC.
Schnoor, J. (1996). Environmental Modeling. Fate and Transport of Pollutants in Water, Air and Soil. A Wiley-Interscience Publication. ISBN 0-471-12436-2.
Shojaeezadeh, S. A., Nikoo, M. R., Mirchi, A., Mallakpour, I., Kouchak, A. A., and Sadegh, M. (2020). Probabilistic hazard assessment of contaminated sediment in rivers. Sci. Total Environ. 703:134875. doi: 10.1016/j.scitotenv.2019.134875
SMHI (2021a). Swedish Meteorological and Hydrological Institute’s Water Webb. Available online at: https://vattenwebb.smhi.se/modelarea/ (accessed February 18, 2021).
SMHI (2021b). Swedish Meteorological and Hydrological Institute. County-Specific Climate ana-Lyzes. Available online at: https://www.smhi.se/klimat/framtidens-klimat/lansanalyser/vasternorrland/local-100-year-inflow (accessed February 18, 2021).
Snowball, I., Apler, A., Dahlberg, A.-K., Frogner-Kockum, P., Göransson, G., Hedfors, J., et al. (2020). TREASURE: Targeting Emerging Contaminated Sediments Along the Uplifting Northern Baltic Coast of Sweden for Remediation. Linköping: Swedish Geotechnical Institute, SGI.
Soulsby, R., and Clarke, S. (2005). Bed Shear-Stresses Under Combined Waves and Currents on Smooth and Rough Beds. Report TR 137.
Suhr, M., Klein, G., Kourti, I., Gonzalo, M., Santonja, G., Roudier, S., et al. (2015). Best Available Techniques (BAT)Reference Document on Best Available Techniques in the Pulp and Paper Industry, JRC Science and Policy Reports. Luxemburg: Institute for Prospective Technological Studies, 906. doi: 10.2791/370629
Superville, P.-J., Prygiel, E., Magnier, A., Lesven, L., Gao, Y., Baeyens, W., et al. (2014). Daily variations of Zn and Pb concentrations in the deûle River in relation to the resuspension of heavily polluted sediments. Sci. Total Environ. 470–471, 600–607. doi: 10.1016/j.scitotenv.2013.10.015
UNDRR (2021). Prevention Web. The Knowledge Platform for Disaster Risk Reduction. Available online at: https://www.preventionweb.net/disaster-risk/concepts/deterministic-probabilistic/, (accessed February. 16, 2021)
USEPA (2021). Contaminated Site Clean-Up InformationSediments. U.S. Environmental Protec-tion Agency. Available online at: https://clu-in.org/issues/default.focus/sec/Sediments/cat/Risk_Assessment/.(accessed February. 10, 2021).
Valeur, C. (2020). Papper Och Massa i Ångermanland - Från Handpappersbruk Till Processindustri. Skogsindustriernas Historiska Utskotts Skriftserie, 295 pp. Skogsindustrierna. ISBN 9789197125253.
Viana, Z. P., Yin, K., and Rockne, J. K. (2012). Field measurements and modeling of ebullition-facilitated flux of heavy metals and polycyclic aromatic hydrocarbons from sediments to the water column. Environ. Sci. Technol. 46, 12046–12054. doi: 10.1021/es302579e
Westrich, B., and Förstner, U. (2005). Sediment dynamics and pollutant mobility in rivers (SEDYMO): assessing catchment-wide emission-immission relationships from sediment studies. BMBF coordinated research project SEDYMO (2002–2006). J. Soils Sediments 5, 197–200. doi: 10.1065/jss2005.11.002
Yuan, Q., Valsaraj, T. K., Reible, D. D., and Willson, S. C. (2007). A laboratory study of sediment and contaminant release during gas ebullition. J. Air Waste Manag. Assoc. 57, 1103–1111. doi: 10.3155/1047-3289.57.9.1103
Keywords: contaminated sediments, extreme events, PCBs, risk assessment, submarine landslide
Citation: Göransson G, Apler A, Dahlberg A-K, Löfroth H, Josefsson S, Wiberg K, Frogner-Kockum P, Nylander P, Hedfors J and Snowball I (2021) Assessing the Risk of Contaminant Dispersion From Fibrous Sediments of Industrial Origin. Front. Mar. Sci. 8:729243. doi: 10.3389/fmars.2021.729243
Received: 22 June 2021; Accepted: 20 August 2021;
Published: 08 September 2021.
Edited by:
Julian Blasco, Consejo Superior de Investigaciones Científicas (CSIC), SpainReviewed by:
Cindy Lee, Clemson University, United StatesJenny Norrman, Chalmers University of Technology, Sweden
Xiaolei Liu, Ocean University of China, China
Copyright © 2021 Göransson, Apler, Dahlberg, Löfroth, Josefsson, Wiberg, Frogner-Kockum, Nylander, Hedfors and Snowball. This is an open-access article distributed under the terms of the Creative Commons Attribution License (CC BY). The use, distribution or reproduction in other forums is permitted, provided the original author(s) and the copyright owner(s) are credited and that the original publication in this journal is cited, in accordance with accepted academic practice. No use, distribution or reproduction is permitted which does not comply with these terms.
*Correspondence: Gunnel Göransson, gunnel.goransson@sgi.se