- 1Kansas Cooperative Fish and Wildlife Research Unit, Division of Biology, Kansas State University, Manhattan, KS, United States
- 2U. S. Geological Survey, Kansas Cooperative Fish and Wildlife Research Unit, Division of Biology, Kansas State University, Manhattan, KS, United States
- 3Fish Ecology Division, Northwest Fisheries Science Center, National Marine Fisheries Service, National Oceanic and Atmospheric Administration, Seattle, WA, United States
- 4Kentucky Department of Fish & Wildlife Resources, Bowling Green, KY, United States
Identifying patterns of organismal distribution can provide valuable insights for basic and applied marine and coastal ecology because understanding where animals are located is foundational to both research and science-based conservation. Understanding variation in distributional patterns can lead to a better assessment of ecological drivers and an improved ability to predict consequences of natural and altered relationships. Here, our purpose is to explore if quantifying coexisting groups of individual fish predators advances our understanding of field distribution patterns. Toward this end, we quantified locations of 59 acoustically tagged striped bass (Morone saxatilis) within a 26-stationary unit telemetry receiver array in Plum Island Estuary (PIE), MA, United States. We then used cluster analyses on spatial and temporal-spatial metrics from this dataset to (1) assess if distinct groups of individuals coexisted, (2) quantify group characteristics, and (3) test associations between groups and distribution (e.g., physical site type and region). Based on multiple lines of evidence, we identified four groups of striped bass with different space use patterns that persisted across seasons (summer and fall). Similar-sized striped bass clustered at spatial and temporal scales at which individuals within distinct groups could, and did, physically overlap. In addition, distributional groups were linked to components of physical site type and region suggesting that discrete groups of individuals can interact differently with the environment within the same ecological system. The identification of these distinct groups of individuals creates a baseline from which to explore further ecological implications of grouping behavior for research and conservation in geographically large, temporally dynamic, and spatially heterogeneous marine and coastal environments.
Introduction
Identifying spatial patterns of organismal distribution can provide valuable insights for marine and coastal science because understanding where animals are located is foundational for both ecological research and science-based conservation. As examples, different distributional patterns of individuals, complexities in population structure, and spatial diversification of fisheries can influence the most effective approaches for fisheries management (e.g., Goethel et al., 2011; Gonzalez-Mon et al., 2021). Consequently, understanding spatial patterns of organisms and the impacts that affect these organisms can lead to a better assessment of ecological drivers and an improved ability to predict consequences of natural and altered relationships (e.g., Mittermeier et al., 1998; Halpern et al., 2008; Su et al., 2021). Here our purpose is to assess if identifying discrete groups of individual fish that coexist within the same population can advance our understanding of fish distribution patterns.
Aquatic predators provide important goods and services to society (e.g., MacDonald et al., 2017; Hammerschlag et al., 2019), and can impact ecosystem function through trophic cascades (e.g., Frank et al., 2005; Estes et al., 2011; Altieri et al., 2012) and through energetic connections across communities (e.g., Casini et al., 2012; Mather et al., 2013). However, variation in spatial distributions of individual predators can reduce fish-related goods and services, change the magnitude of ecological impacts by and on predators, and influence relevant coastal and marine research, and management directions (e.g., Eero et al., 2012; Kessel et al., 2016; Shelton et al., 2020). In this research, we use individual-based acoustic telemetry of striped bass (a predator) to identify if quantifying behavioral groups can provide a more accurate understanding of the distribution of these fish in coastal seascapes.
Conceptual frameworks that explain how individuals can form groups within populations can be useful for science-based conservation (e.g., Merrick and Koprowski, 2017). As an example, fisheries professionals often use statistical models that assume that individuals are distributed randomly around a central tendency (mean, median) as predicted by a specific mathematical distribution (e.g., negative binomial, Poisson, and Gaussian; Bolker, 2008). However, coexisting groups of individuals within a population violate these assumptions, and, if these non-random associations are not considered, erroneous predictions can result. Behavioral syndromes and animal personalities are examples of conceptual frameworks that identify structure, formation, and consequences of groups of individuals within populations (e.g., Dall et al., 2012; Wolf and Weissing, 2012; Kelleher et al., 2018). Research related to the conceptual frameworks of behavioral syndromes and animal personalities show that organisms from an array of taxa can form discrete coexisting groups that vary in aggression, boldness, activity, exploration, and feeding (Supplementary Appendix Table 1). Coexisting groups of individuals within populations are difficult to detect in the field. If development, refinement, and testing of the above-described concepts continue to move beyond simplified environments, these frameworks that illustrate how groups of coexisting individuals may form and be maintained have great potential to explain highly variable distributional patterns in natural systems.
Links among groups of coexisting conspecific individuals, variation in distributional patterns, and ecological impacts of this variation are often unknown because of the difficulty of unpacking these multifaceted processes in field environments. For example, do some individuals of an estuarine fish species form groups that use primarily coastal riverine habitats within an estuary while other groups explore pelagic estuarine habitats? Can this use of specialized locations by groups of coexisting conspecific individuals result in differences in site fidelity and opportunistic exploration? Can these groups of individuals of the same species that use the same geographic location in predictable but diverse ways be linked to different patterns of diet, growth, survival, and reproduction? Researchers simply don’t know if differences in distributional metrics of interacting individuals of the same species using the same seascape can account for variation in the distribution of a population. Identifying if groups of individuals of the same species coexist and persist within the same field population is the first step in investigating this chain of connections.
Tracking seasonally resident, migratory striped bass (Morone saxatilis) within Plum Island Estuary (PIE), MA, United States, provides an ideal opportunity to examine groups within an interacting population of striped bass. Elsewhere, we determined that although geomorphic habitat features helped explain seascape distribution of these fish, organismal patterns were still highly variable (Taylor et al., 2019). Here we test three hypotheses that explore if quantifying groups of co-occurring individual organisms can explain variation in field distribution. Elsewhere, we have shown that these striped bass are in the feeding stage of their spring-fall coastal migration (Ferry and Mather, 2012; Mather et al., 2013). First, we hypothesize that individual subadult and small adult striped bass can form groups that coexist within the same foraging area. Distinct, space-based groups could potentially provide an advantage for individual predators if familiarity with local conditions increases fish foraging success. Second, if groups of individuals exist, we hypothesize that multiple metrics, rather than a single characteristic, will define these patterns. Third, we hypothesize that some, but not all, distributional groups could be linked to larger-scale habitat variables (e.g., physical features, ecosystem region).
Materials and Methods
Field Site
Plum Island Estuary is located on the northeastern coast of Massachusetts, United States (Figure 1A). Exits (or egress points) from our Plum Island Sound-Rowley River study area include the Plum Island River, Parker River, Ipswich River, and the ocean outlet at the south end of Plum Island Sound (Figure 1B). PIE has many tidal creeks that create confluences (i.e., where two water bodies join together). Examples of tidal creek confluences include West Creek, Rowley River, Third Creek, and Grape Island (Figure 1B). Plum Island Sound also contains many other sites that may or may not be associated with physical discontinuities such as channels, drop-offs, islands, or sand bars (Kennedy et al., 2016, 2017). PIE can be divided into four geographic regions (Upper Sound, Middle Sound, Lower Sound, and Rowley River; Figure 1B).
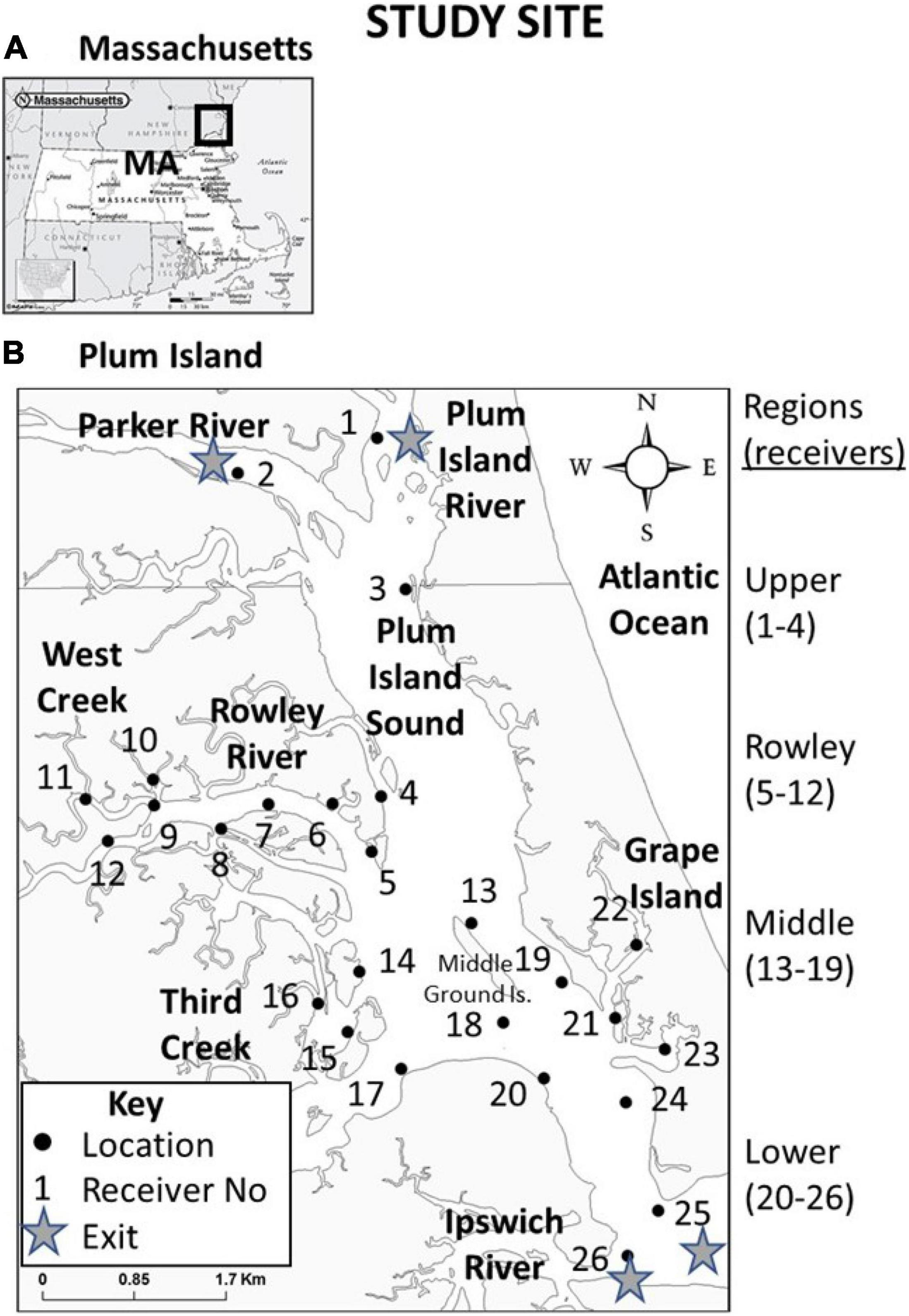
Figure 1. (A) Location of our study site in Plum Island Estuary (PIE) in northeastern MA, United States, and (B) study site characteristics including landmarks, confluences, geographic regions, and locations of receivers by number. Filled dots indicate stationary receiver locations. Receivers were placed to test fish use of three types of physical sites (exits, confluences, and non-confluences). Exit locations were at the four locations (receiver numbers 1, 2, 25, and 26). Confluences are locations where two smaller water bodies merge. To test confluences, we deployed three receivers each at Rowley River mouth (receivers 5, 6, and 8); West Creek (receivers 9, 10, and 11); Third Creek (receivers 14, 15, and 16); and Grape Island (receivers 21, 22, and 23). The non-confluence site type included six locations not directly adjacent to any river mouth (receiver numbers 3, 12, 13, 18, 19, and 20). A few receivers were deployed to detect fish in underrepresented areas and to provide greater spatial coverage (receivers 4, 7, 17, and 24). We deployed receivers in four regions (Upper region = receivers 1–4; Rowley River region = receivers 5–12; Middle region = receivers 13–19; and Lower region = receivers 20–26). All fish included in our analysis encountered all of the same receivers of an identical model deployed using an identical protocol.
Physical Habitat Types and Locations Sampled
We tracked acoustically tagged striped bass using a 26-unit stationary receiver array (Figure 1B). Receivers (VR2W 69 kHz) were intentionally placed in specific locations throughout the estuary to test fish use of three types of physical sites (exits, confluences, and non-confluences). Exit locations were at the four locations described earlier (receiver numbers 1, 2, 25, and 26; Figure 1B). To test how fish use confluences, we deployed three receivers each at Rowley River mouth (receivers 5, 6, and 8); West Creek (receivers 9, 10, and 11); Third Creek (receivers 14, 15, and 16); and Grape Island (receivers 21, 22, and 23; Figure 1B). The non-confluence site type included six locations not directly adjacent to any river mouth (receiver numbers 3, 12, 13, 18, 19, and 20; Figure 1B). A few additional receivers were deployed to provide greater general spatial coverage (receivers 4, 7, 17, and 24). These receivers spanned the four above-described regions (Upper Sound region = receivers 1–4; Rowley River region = receivers 5–12; Middle Sound region = 13–19; and Lower Sound region = 20–26; Figure 1B). Receiver range (500 m) was quantified as the average distance at which tags were detected at select test receivers. All tagged fish encountered the same set of receivers of an identical model deployed using the exact same protocol.
Fish Tagging
In 2015, 59 subadult and small adult striped bass (mean fish size = 524 mm TL, range = 434–623 mm TL, and SE = 5.85) were implanted with VEMCO V13 acoustic transmitters (weight in air: 11–13 g, weight in water: 6–6.5 g; and length: 36–48 mm) and released near the location where they were captured (Kennedy et al., 2016; Gerber et al., 2019). Tags transmitted at a frequency of 69 kHz using a 180-s nominal delay pulse-code-burst [random delay: 110–250 s; minimum delay: 110 s; maximum delay: 250 s; following the terminology of Loher et al. (2017)]. The size range that we tagged is substantially narrower than the size range of striped bass harvested in the 2017 Massachusetts fisheries (commercial: 863–1,143 mm TL, 6–15 yr; recreational: 686–1,143 mm TL, 4–15 yr; Nelson, 2018). We chose to tag similar-sized individuals, within this available size range, in order to tease apart the effect of body size from individual variation. Tags were less than 2% of the body weight (Bridger and Booth, 2003). Individual fish were anesthetized until they lost orientation (Aqui-S, 30 mg-L; mean = 2 min 18 s). Using surgical scalpels of size 12, a 15–30 mm lateral incision was made below the pectoral fin, about 3/4 of the way to the fin tip. The acoustic tag was sterilized (ethanol) and inserted into the body cavity. Then the incision was closed with 2–4 simple interrupted sutures (FS-2, 19 mm 3/8c, reverse cutting; Ethicon, Vicryl, 3-0; and mean surgery time = 2 min 31 s). Once fish regained orientation, they were released (mean recovery time = 6 min 15 s). This research was conducted under the auspices of Kansas State University IACUC Protocol #3576. Studies of variation across individuals vary in duration from within a season (Wat et al., 2020) to across years (Sabal et al., 2019). Here, we focus on specific space use patterns of tagged striped bass during their summer-fall residence (∼120 days) that represents the feeding component of their seasonal migration.
Residence Time Data
Detections of tagged fish by receivers within the 26 unit receiver array were modified into “residence time” (or amount of time that fish resided at each receiver) by summing the amount of time between detections until either tagged fish were not detected for 1 h or were detected by another receiver. Residence time was calculated at each receiver location for each fish using the VTrack package in Program R (R 2.15.2 software; R Core Team) (Campbell et al., 2012). Residence time was further simplified as “proportion of time” at each receiver by taking the amount of time a fish spent at a single receiver and dividing it by the total time a fish was detected across the entire array.
Fish Cluster Analysis
Grouping patterns were based on multiple methods: (a) exploratory data analyses that examined individual fish trajectory plots and seascape maps, (b) cluster analyses, (c) by-cluster metric plots, and (d) across-cluster metric statistical comparisons. To identify if distinct striped bass distributional groups existed, we ran sequential hierarchical cluster analyses using Ward’s linkage on Euclidean distance matrices (“cluster” package, R). To determine the optimal number of clusters, the average silhouette width was maximized (Kaufman and Rousseeuw, 1990). We examined all clusters with a Jaccard bootstrap mean values >0.5. Jaccard values between 0.60 and 75 were used to indicate cluster patterns (“clusterboot” function, “fpc” package, R; Hennig, 2008). Eight “short-timer” fish were detected briefly and sporadically at a limited number of receivers within PIE (i.e., detected in <3 weekly intervals within a 30 day period). Of these eight “short-timer” fish, six were detected in arrays outside of Plum Island. Because they provided limited information about PIE space use, these “short-timer” fish were manually removed from the dataset and excluded from subsequent analyses.
Examination of patterns followed a two-stage protocol that separated the effects of space alone from the effects of space combined with time. In the first stage of our cluster analysis, we used the metrics “proportion of time” at each of the 26 receivers to assign fish to a cohesive spatial cluster. In the second stage of our cluster analysis, we used four temporal-spatial metrics on the remaining fish. The first metric, total number of days in the Plum Island ecosystem, was measured as the days between an individual fish’s first detection and its last detection in the study system. We expected fish that stayed a short period of time to behave differently than seasonally resident fish. The second metric, total number of receivers visited, was a measure of mobility calculated by counting the number of unique receivers each fish visited throughout the season. A fish that visited many receivers was likely more active than a fish that stayed close to the same few receivers. The third metric, total number of movements, was another measure of between receiver activity that was calculated by summing the number of times fish moved from one receiver to another. The fourth metric, receiver allegiance, was a measure of site fidelity that was calculated as the proportion of time a fish spent at the single most visited receiver, based on residence time. A fish that stayed in the same place would have a high value of this metric compared to a fish that spent time at many different receivers throughout the estuary.
We examined our four metrics as boxplots to visualize similarities and differences across clusters. Differences in metrics across clusters were tested using a Kruskal–Wallis non-parametric ANOVA test (“kruskal.test” function, “stats” package, R) with post hoc Mann Whitney U multiple comparisons.
Distributional Groups, Physical Site Types, and Regions
We separately tested the effect of physical site types (exit, confluence, and non-confluence) then regions (Upper Sound Middle Sound, Lower Sound, and Rowley River) on residence time across the four distributional groups from the above-described cluster analyses (“anova” function, “stats” package, R). Residence time was summed for each fish at each receiver and individual tagged fish were treated as replicates. Data were log transformed to meet statistical assumptions. A post hoc Tukey test (“tukeyHSD” function, “stats” package, R) identified pairs of significantly different treatments. For these analyses, all fish were included (i.e., we retained fish with zero values at a receiver) to test how the fish behaved across the entire tagged population. A value of P < 0.05 was used to determine statistical significance.
Results
Overview
Most tagged fish were detected within PIE monthly (July: 50 fish; August: 48 fish) until they started to migrate south in the fall (September: 38 fish, October: 8 fish; data not shown). Of the 59 fish tagged in our study, 37 fish were detected in arrays outside PIE after they migrated. These high levels of frequent detection suggest limited (or no) post-tagging mortality.
We identified four clusters of individual striped bass in PIE using both spatial metrics (cluster 1; Figure 2A) and temporal-spatial metrics (clusters 2–4; Figure 2B). Spatial metrics identified the Rowley River group (Cluster 1, Figure 2A; Jaccard, 0.6). Temporal-spatial metrics identified clusters 2–4 (Figure 2B), which were the groups associated with Plum Island Sound (Cluster 2, Jaccard, 0.5), Exploratory (Cluster 3; Jaccard, 0.7), and Extreme Fidelity (Cluster 4; Jaccard, 0.6) groups. Exploratory data analysis plots of individual fish trajectories identified similar patterns for individual members of the same group. For each of our four clusters, we show one representative individual fish within each cluster to illustrate group trajectories (i.e., locations connected by movements) and distributional maps (Figures 3A–D). Then we use boxplots to show how mean metrics differed across the four clusters (Figures 4A–D).
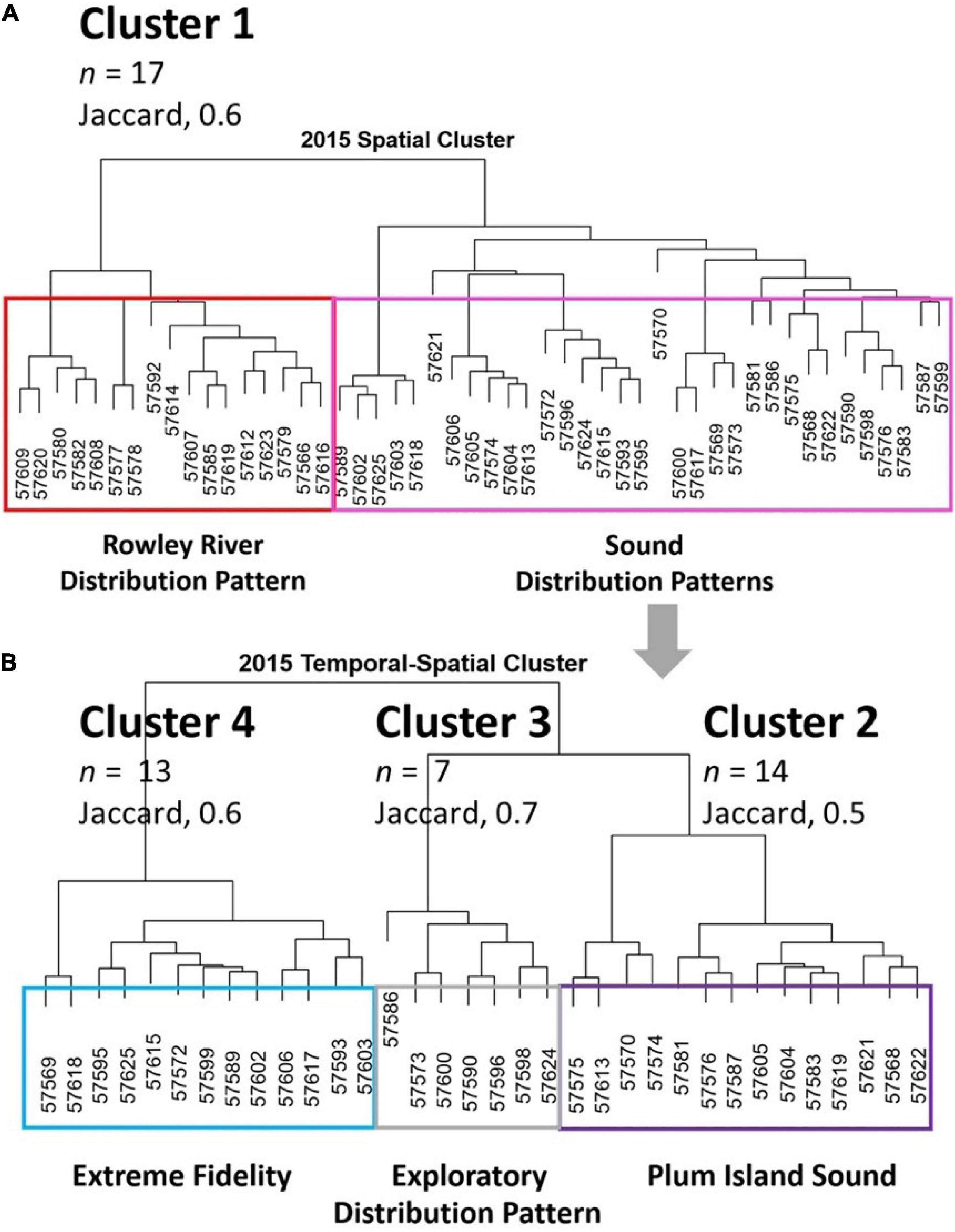
Figure 2. Four distributional cluster groups are shown. (A) Spatial cluster dendrogram based on proportion of time spent at each of 26 receivers that identifies the Rowley River group (Cluster 1, red box). (B) Temporal-spatial dendrogram that identified Plum Island Sound, Exploratory, and Extreme Fidelity distributional groups (Clusters 2–4).
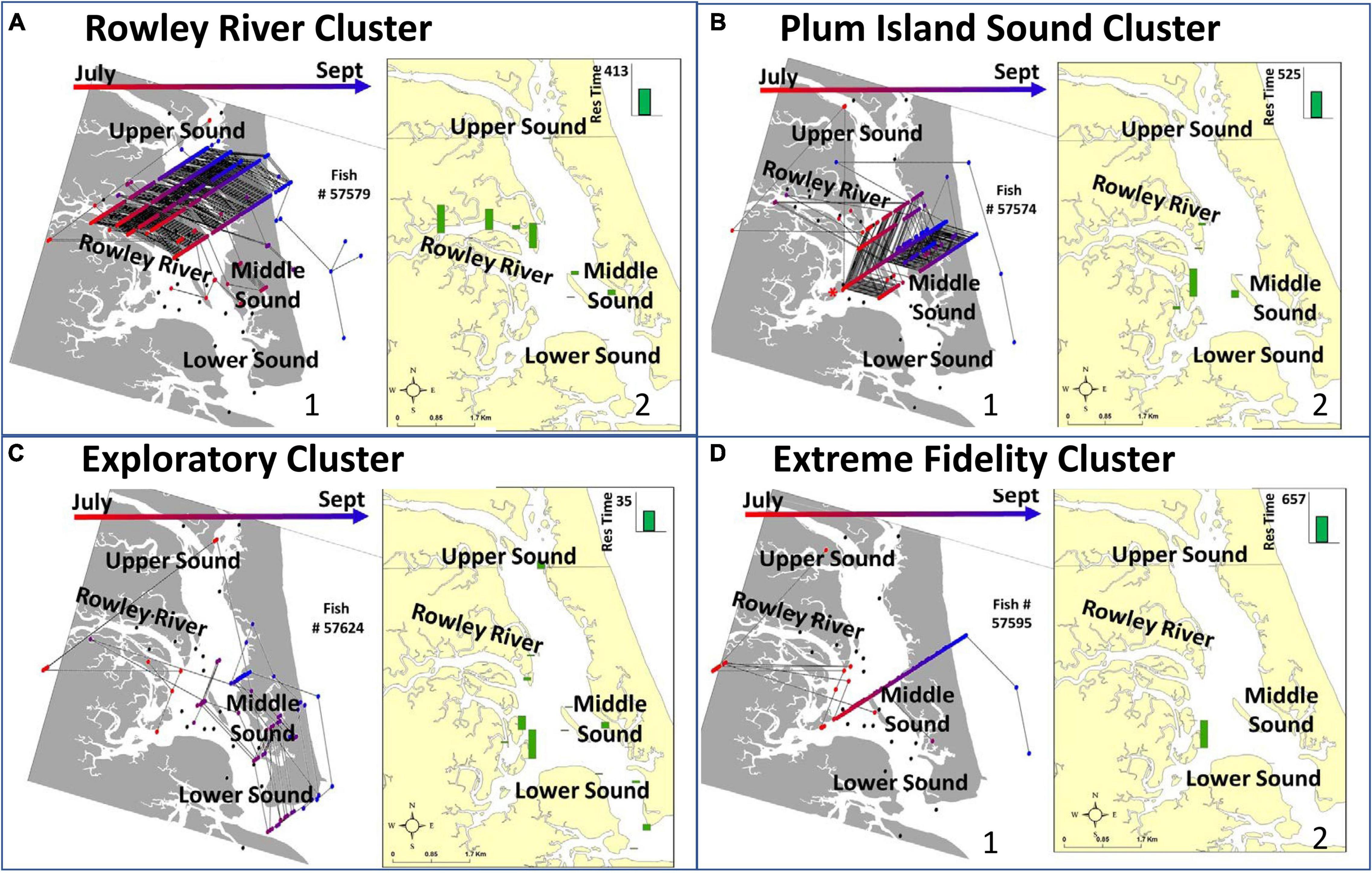
Figure 3. Representative examples of individual fish in each of the four clusters (A–D) including: trajectories displaying detections and movements among receivers over the entire study period (A-1,B-1,C-1,D-1) and maps of the average residence time that this individual fish spends at each receiver location (A-2,B-2,C-2,D-2). The arrows at the top and the corresponding dot colors in panels (A1–D1) show the direction that the fish moved through time. The green bars in panels (A2–D2) indicate receiver-specific residence time. When all detections (multicolor dots in A1–D1) are summed through time, the result is residence time (green bars in A2–D2). Both panels show the same data summarized in different ways. Both inform similarities within clusters and differences across clusters. Clusters include (A) Rowley River, (B) Plum Island Sound, (C) Exploratory, and (D) Extreme Fidelity groups. Trajectories are tracks of connected fish detections along the X, Y (latitude, longitude), and Z axis (time; July – bottom, September – top). The Z temporal data dimension comes out of the page.
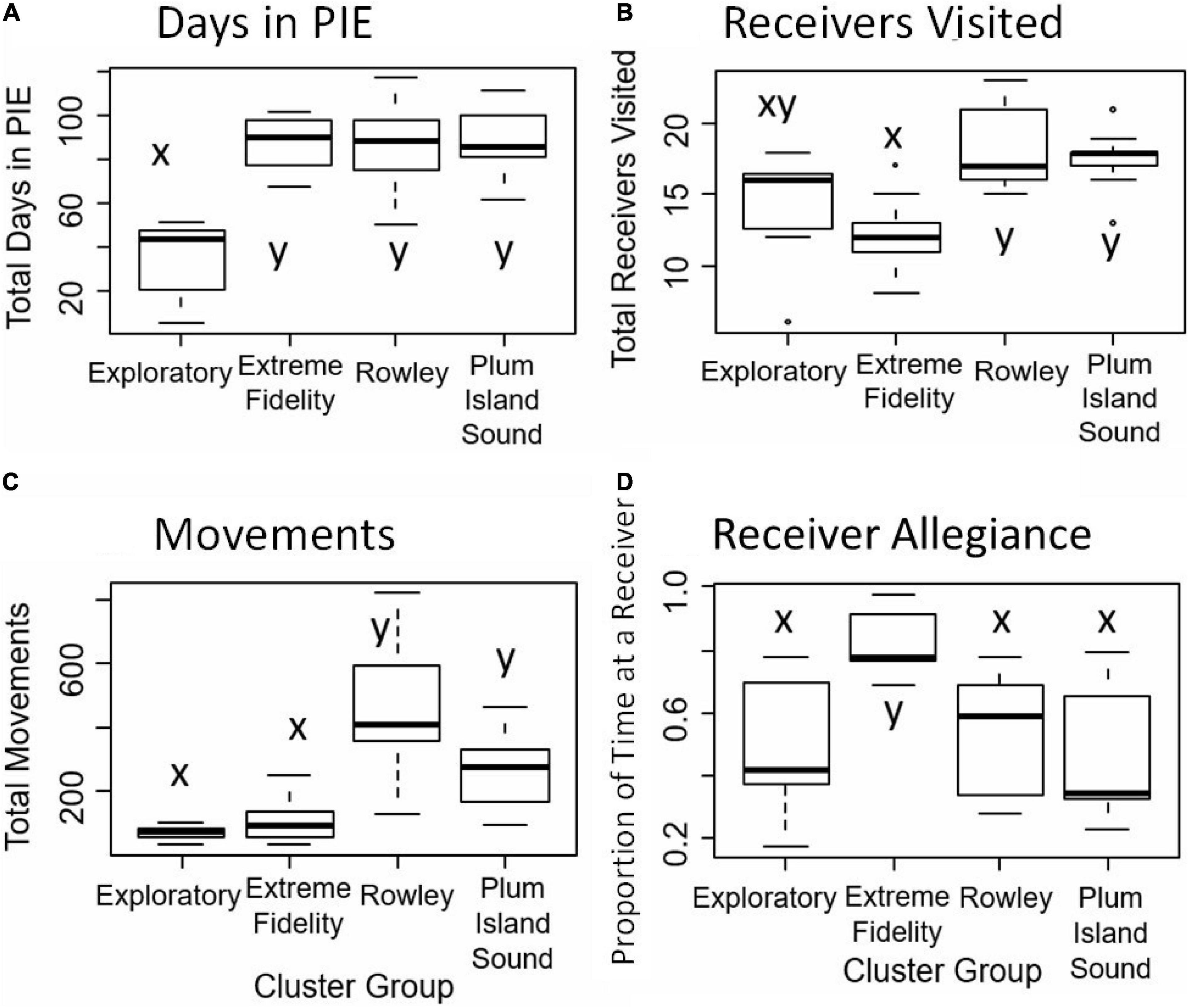
Figure 4. For the four clusters (Exploratory, Extreme Fidelity, Rowley, and Plum Island Sound; X axis), shown are the box plots of metrics used in the cluster analysis (Y axis). Metrics include: (A) total days in PIE, (B) total receivers visited, (C) total number of movements, (D) receiver allegiance. Receiver allegiance is the proportion of time a fish spent at the single most visited receiver. Letters indicate statistical differences.
Rowley River Group
Seventeen tagged individuals that were detected within PIE for 51–117 days formed the Rowley River group (cluster 1; Figure 2A). Trajectories and distributional maps showed that fish in this group remained primarily within the Rowley River (Figure 3A). The seasonally resident Rowley River group spent a high total number of days in PIE (average: 88.35 days, SE: 5.01; Figure 4A). This very mobile cluster of fish visited a high total number of receivers (average: 18.47, SE: 0.69; Figure 4B), and had a very high but variable total number of movements (average: 451.88, SE: 43.52; Figure 4C). However, this cluster exhibited medium high but variable receiver allegiance (average: 0.55, SE: 0.04; Figure 4D). Ecologically, individual fish in the Rowley River cluster moved many times across many receivers, but these residences and movements were primarily within a medium-scale, geographically localized subset of receivers.
Plum Island Sound Group
Fourteen tagged striped bass individuals that were detected within PIE for 72–111 days formed the Plum Island Sound group (cluster 2; Figure 2B). Trajectories for striped bass within this more variable group combined movements and residence events within both the Middle and Lower Sound (Figure 3B). Seasonally resident striped bass within the Plum Island Sound group spent a high total number of days in PIE (average: 89.08 days, SE: 4.32; Figure 4A). As a group, individual fish within the Plum Island Sound group visited a high total number of receivers (average: 17.62, SE: 0.53; Figure 4B), but were not often detected in the Rowley River. The Plum Island Sound group was neither as mobile nor as sedentary as other groups as reflected by an intermediate total number of movements (average: 270.00, SE: 32.40; Figure 4C). The Plum Island Sound group was more variable in spatial distribution than other groups because it included two sub-groups of individual fish that spent more time either around Middle Ground Island (Middle region) or at the southern end of the estuary (Lower region) resulting in a low median but highly variable receiver allegiance (average: 0.48, SE: 0.06; Figure 4D). Ecologically, the Plum Island Sound group resided at and moved among a preferred group of receivers within two subregions of the Sound.
Exploratory Group
Seven tagged fish that were detected at receivers within PIE for up to 52 days formed the Exploratory group (cluster 3; Figure 2B). Trajectories of individuals within this Exploratory group were characterized by residences and movements throughout the PIE study area (Figure 3C). Statistically, the Exploratory group spent a low total number of days in PIE (average: 34.28, SE: 6.79; Figure 4A), visited an intermediate total number of receivers (average: 14.00, SE: 1.56; Figure 4B), made a low total number of movements (average: 71.29, SE: 8.89; Figure 4C), and exhibited low but variable receiver allegiance (average: 0.50, SE: 0.08; Figure 4D). Ecologically, the Exploratory group was a roaming behavioral group that did not devote the majority of their time to any specific region or receiver.
Extreme Fidelity Group
Thirteen tagged fish that were detected within PIE for 68–102 days formed the Extreme Fidelity group (cluster 4; Figure 2B). Trajectories of the Extreme Fidelity group were characterized by a long and localized residence event (prolonged residence at 1–2 receivers) (Figure 3D). These fish exited the estuary in the fall as did all of our tagged fish. We emphasize egress and subsequent detections outside our study area for fish in this Extreme Fidelity cluster to demonstrate that these tagged fish (which did not move much within PIE) were alive and retained their tags. Seasonally resident individuals within the Extreme Fidelity group spent a high total number of days in PIE (average: 87.92 days, SE: 3.19; Figure 4A). As their name suggests, individuals of this behavioral group visited a low total number of receivers (average: 11.77, SE: 0.71; Figure 4B), made a low total number of movements (average: 109.08, SE: 20.12; Figure 4C), but had the highest receiver allegiance (average: 0.82, SE: 0.03; Figure 4D). Ecologically, individual fish within the Extreme Fidelity group spent the summer-fall period at limited, localized receiver locations from which they made few forays.
Differences in Group Metrics Across Clusters
Differences among groups existed for multiple metrics. For the total number of days metric, the Exploratory group spent a significantly lower number of days in PIE than the Extreme Fidelity, Rowley River, and Plum Island Sound groups (P < 0.05; Figure 4A). For the total number of receivers visited metric, the Rowley River and Plum Island Sound groups visited significantly more receivers than the Extreme Fidelity group (P < 0.05; Figure 4B). For the total number of movements metric, the Rowley River and Plum Island Sound groups had significantly more movements compared to the Exploratory and Extreme Fidelity groups (P < 0.05; Figure 4C). For the receiver allegiance metric, the Extreme Fidelity group spent a significantly higher proportion of time at a single receiver than all other groups (P < 0.05; Figure 4D).
Physical and Regional Drivers of Fish Distribution
Distributional groups provided additional information about physical and regional drivers for our tagged striped bass population. Residence times at exits were low for all four seasonally resident groups (Figure 5A). For non-confluence sites, mean residence time of the Extreme Fidelity group was very high, mean residence time of the Exploratory group was low, and use of non-confluence sites by the Rowley River and Plum Island Sound groups was intermediate (Figure 5A). High variability in use of non-confluence sites obscured any across-group differences in use of this site type. However, use of confluence sites differed across our four clusters. Striped bass in the Rowley River group had the highest residence time at confluences, the Plum Island Sound group had intermediate residence times at confluences, and the Extreme Fidelity and Exploratory groups spent little time at confluences (P < 0.05; Figure 5A).
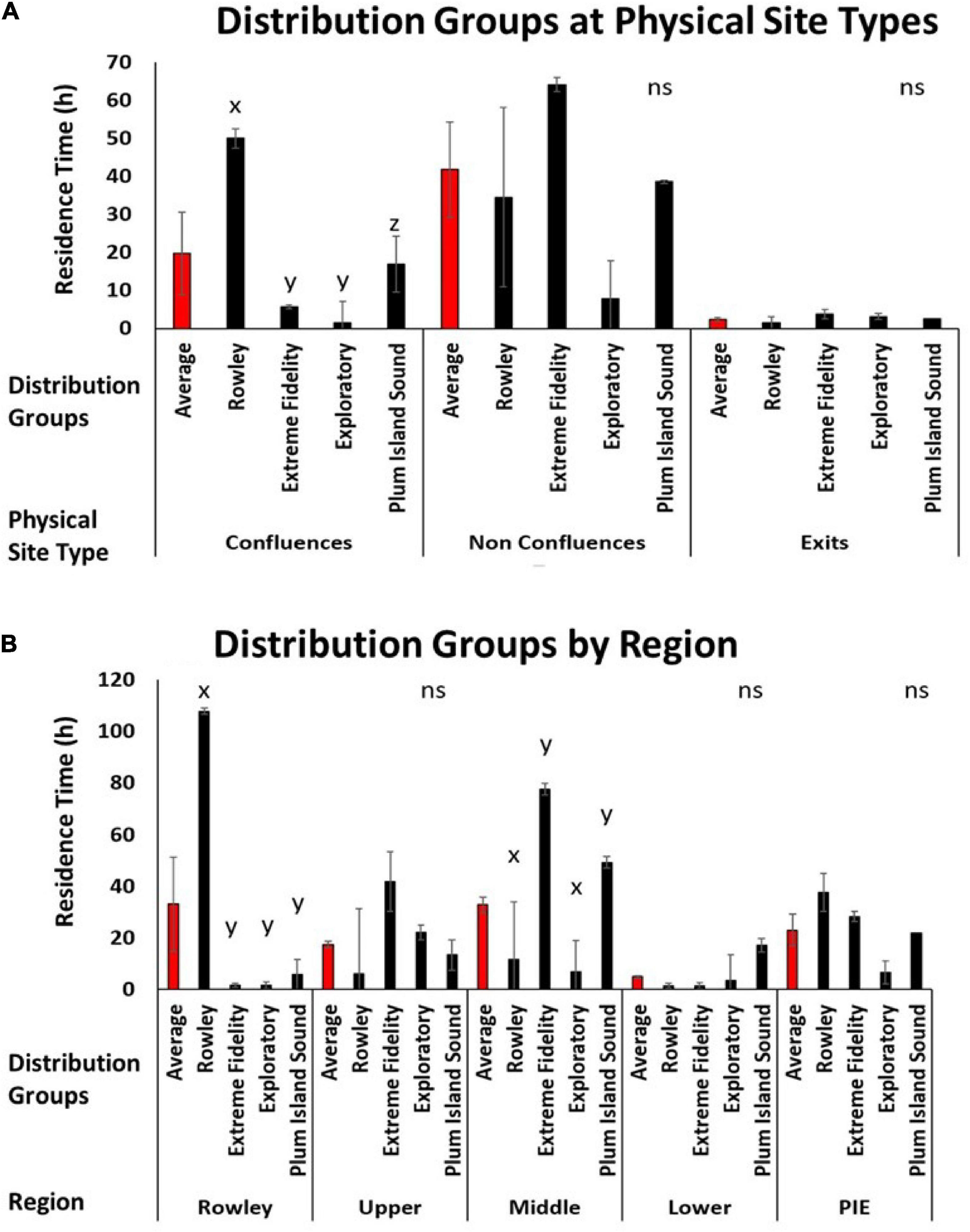
Figure 5. (A) Bar graphs depicting the average residence time (h) (Y axis) for each cluster group (Rowley, Extreme Fidelity, Exploratory, and Plum Island Sound; X axis) by physical site types (confluence, non-confluence, and exit; X axis). (B) Bar graphs depicting the average residence time (h) (Y axis) for each distribution group (X axis) by region (Rowley River, Upper Sound, Middle Sound, and Lower Sound) and the whole estuary average (PIE) (X axis). Letters indicate statistical differences.
By region, the Rowley River group spent significantly more time in the Rowley River region (P < 0.05; Figure 5B). No group, as a whole, spent much time in the Upper or Lower regions (Figure 5B). However, the Middle region was used more by the Extreme Fidelity and Plum Island Sound groups than the Exploratory or Rowley River groups (P < 0.05; Figure 5B).
Discussion
Take-Home Messages
Using multiple lines of evidence that emerged from fish telemetry data (trajectory plots, seascape maps, cluster analysis of metrics, by-cluster metric plots, and across-cluster metric statistical comparisons), we identified four groups of striped bass that persisted within PIE across two seasons (summer and fall). Our results show that individual fish were not using space in the same way, and that individual striped bass can form discrete distributional groups in complex field environments (hypothesis 1). As one example, the Rowley River group (1/3 of the tagged fish) used the river region for much of the summer and early fall (July-September; average residence time: 108 h); yet the rest of the tagged population spent little time in this nearby location (<6 h average time). Our results reinforced previous findings that mobility and distribution metrics can vary across individuals in an ecologically meaningful way (e.g., Frank et al., 2009; Zhang et al., 2015; Webber et al., 2020). Our results also confirmed previously identified trends that individual organisms can associate non-randomly with varying levels of specificity (e.g., Pautzke et al., 2010). However, general associations among individual organisms (e.g., Frank et al., 2011) are not the same as the distinct and persistent grouping of individuals that we identified here. In contrast to other telemetry studies in which organisms exhibit different distribution and mobility metrics across season, size, habitat, spawning condition, or stocking treatment (e.g., DeCelles and Cadrin, 2010; Sabal et al., 2019), our similar-sized, striped bass clustered into co-occurring groups at spatial and temporal scales at which individual organisms within different groups could, and did, physically overlap.
In our study, multiple metrics, cluster analyses, by-cluster plots, and associated cluster-related statistical analyses identified insights about fish distribution. Many multivariate statistical methods (e.g., cluster analysis, principal component analysis, and non-metric multidimensional scaling) exist to identify patterns in data. In exploratory data analysis, trajectory plots of raw data identified fish groups. We chose cluster analysis because this statistical tool provides an independent method for assigning groups. Of course, individual variation also occurred within our cluster groups. Examining both discrete and continuous patterns of variation are likely important for understanding distribution in most natural systems. The four metrics used in our two-stage cluster analysis addressed the need to use multiple integrated metrics in explaining space use (hypothesis 2). Specifically, the first stage of our cluster analysis identified that groups could form based on spatial components alone (i.e., Rowley River fish group). The second stage of our cluster analysis identified the interaction of multiple spatio-temporal metrics (e.g., Plum Island Sound fish groups). Other metrics can certainly add information here and in other studies. Likely complex distributional patterns that are driven by multiple causes will require carefully selected multiple metrics.
Our distributional groups were linked to physical site type and region suggesting that groups of individuals can interact differently with the same field environment (hypothesis 3). As one example, the Rowley River group used confluences more than other physical site types. Confluences are predicted to be ecological hotspots (Poole, 2002; Benda et al., 2004; Rice et al., 2006). In related research, these geomorphic hotspots were commonly used, but distribution patterns were highly variable (Taylor et al., 2019). Our present results add resolution to this previously reported, highly variable pattern by showing that the Rowley River cluster used confluences more than other fish groups. As a second example, our four seasonally resident clusters also had specific associations with estuary regions. Fish in the Extreme Fidelity and Plum Island Sound clusters dominated the use of the Middle Sound region. Finally, our study revealed that identifying groups through cluster analysis explained previously unknown spatial and temporal variation in the distribution of individual organisms. This clarification in distributional patterns can decrease the statistical error associated with high unexplained variation that impedes the quantification of scientific patterns, obscures the effects of ecological drivers, and limits effective, science-based conservation in natural ecosystems.
Potential Future Directions of Interest
How general and broadly relevant is the identification of behavioral groups for understanding spatial and temporal distribution patterns in natural habitats? Recent field tests report that organismal performance and animal personality groups may be linked (Villegas-Ríos et al., 2017; Wat et al., 2020), may not be linked (Lichtenstein et al., 2018; Kowalski et al., 2019), or both may and may not be linked depending on the metric used (Dhellemmes et al., 2021). As examples of positive links between animal groups and performance, contingents of sticklebacks make different shoaling choices (Harcourt et al., 2009) and fish groups can differ in their probability of crossing an instream barrier (Hirsch et al., 2017).
At this early stage of our understanding of the structure and function of co-occurring groups of organisms in natural environments, we simply don’t know how our groups of striped bass form or the wider ecological consequences of such groupings. An example of a potentially useful ecological application of the groups of striped bass that we identified in this study follows. Fishing can impact fish behavior (e.g., Uusi-Heikkilä et al., 2008; Goetze et al., 2017). Thus, the insights that we identify above can provide the basis for exploring behavioral links between fish distribution and commercial/recreational fishers. Striped bass are a popular commercial and recreational fish in coastal areas and have been historically imperiled and at times overfished (Richards and Rago, 1998; Shepherd et al., 2018). Coastal striped bass are composed of separate spawning populations (Wirgin et al., 2020) that have different distributions and movement patterns (e.g., Rulifson and Dadswell, 1995; Secor et al., 2020). These stock-specific movement patterns can vary across locations, seasons, and years (e.g., Waldman et al., 1990; Kneebone et al., 2014). In estuaries like PIE, known spawning stocks intermix to feed (Mather et al., 2010, 2013). Potentially, fishing could selectively remove fish with different behaviors and drive ecological change mediated through the clusters that we identify here. We hypothesize two intriguing links. First, if members of the behavioral cluster that frequent confluences are targeted and captured more often, fishing could drive evolutionary change away from confluence usage. Second, connections among energetic profitability, specialized forging tactics, and fishing pressure may vary across the behavioral clusters that we identify here. Striped bass in the Sound behavioral clusters could have a higher but more variable growth rate because they eat more pelagic fish prey through active foraging in open water habitats. Striped bass in the River behavioral cluster may use sit and wait tactics within the salt marsh habitat to capture a predictable invertebrate diet that produces slower growth and a smaller, less variable size distribution. If so, higher fishing pressure on the larger Sound fish may change links between foraging behavior, location, and diet. These links about the potential function of behavioral clusters can be tested in the future through focused field surveys and laboratory experiments.
What We Learned That We Didn’t Know Before and What We Still Need to Know
Our research adds to a growing body of literature that identifies discrete groups of individuals in field environments (Hertel et al., 2020; Wat et al., 2020; Dhellemmes et al., 2021). Others have suggested the important link between personalities and spatial ecology (Nilsson et al., 2014; Spriegel et al., 2016), but many gaps exist related to the frequency of co-occurring groups of individuals in field environments and the consequences of these grouping patterns. In our study, we don’t how long individuals remain within the same groups (>1 year, one generation), by what behavioral mechanism individual fish groups form, what is the role of learning, whether a genetic basis exists, or if there are ecological benefits to specific grouping patterns (e.g., growth, survival). Nevertheless, the space use of different groups of striped bass, identified here, provide a foundation on which to build a better understanding of organismal distribution in large complex estuarine, coastal, and marine seascapes.
Data Availability Statement
The raw data supporting the conclusions of this article will be made available by the authors, without undue reservation.
Ethics Statement
The animal study was reviewed and approved by this research was conducted under the auspices of Kansas State University IACUC Protocol #3576.
Author Contributions
RT and MM conceptualized the study design. RT, MM, and KB collected the data. RT, JS, and KB analyzed the data. All authors contributed to the interpretation of data, writing, and editing of the manuscript.
Funding
The Plum Island Ecosystems LTER program (OCE-0423565, OCE-1058747, and OCE-1238212) provided financial support, lodging and invaluable field, laboratory, and other assistance throughout the research. The project was administered by the Kansas Cooperative Fish and Wildlife Research Unit, a joint effort between Kansas State University, the U.S. Geological Survey, U.S. Fish and Wildlife Service, the Kansas Department of Wildlife, Parks, and Tourism (KDWPT), and the Wildlife Management Institute.
Conflict of Interest
The authors declare that the research was conducted in the absence of any commercial or financial relationships that could be construed as a potential conflict of interest.
Publisher’s Note
All claims expressed in this article are solely those of the authors and do not necessarily represent those of their affiliated organizations, or those of the publisher, the editors and the reviewers. Any product that may be evaluated in this article, or claim that may be made by its manufacturer, is not guaranteed or endorsed by the publisher.
Acknowledgments
We thank the Kansas State University Aquatic Group and PIE LTER scientific community for support and feedback. Jane Fencl, Sean Hitchman, Jennie Grill, Lindsay Arick, Linda Deegan, Anne Giblin, Barry Clemson, Sam Kelsey, and Cristina Kennedy provided field, laboratory, and editorial assistance. Walter Dodds, Anthony Overton, and Michi Tobler provided useful comments. Any use of trade, firm, or product names is for descriptive purposes only and does not imply endorsement by the U.S. Government.
Supplementary Material
The Supplementary Material for this article can be found online at: https://www.frontiersin.org/articles/10.3389/fmars.2021.723025/full#supplementary-material
References
Altieri, A. H., Bertness, M. D., Coverdale, T. C., Hermann, N. C., and Angelini, C. (2012). A trophic cascade triggers collapse of a salt-marsh ecosystem with intensive recreational fishing. Ecology 93, 1402–1410. doi: 10.1890/11-1314.1
Benda, L., Poff, N. L., Miller, D., Dunne, T., Reeves, G., Pess, G., et al. (2004). The network dynamics hypothesis: how channel networks structure riverine habitats. Bioscience 54, 413–427. doi: 10.1641/0006-3568(2004)054[0413:tndhhc]2.0.co;2
Bolker, B. M. (2008). “Probability and stochastic distributions for ecological modeling. Chapter 4,” in Ecological Models and Data in R, ed. B. M. Bolker (Princeton, NJ: Princeton University Press), 103–146. doi: 10.1515/9781400840908-005
Bridger, C. J., and Booth, R. K. (2003). The effects of biotelemetry transmitter presence and attachment procedures on fish physiology and behavior. Rev. Fish. Sci. 11, 13–34. doi: 10.1080/16226510390856510
Campbell, H. A., Watts, M. E., Dwyer, R. G., and Franklin, C. E. (2012). V-Track: software for analysing and visualising animal movement from acoustic telemetry detections. Mar. Freshw. Res. 63, 815–820. doi: 10.1071/mf12194
Casini, M., Blenckner, T., Mollmann, C., Gardmark, A., Lindegren, M., Llope, M., et al. (2012). Predator transitory spillover induces trophic cascades in ecological sinks. Proc. Natl. Acad. Sci. U.S.A. 109:8185. doi: 10.1073/pnas.1113286109
Dall, S. R. X., Bell, A. M., Bolnick, D. I., and Ratnieks, F. L. W. (2012). An evolutionary ecology of individual differences. Ecol. Lett. 15, 1189–1198.
DeCelles, G. R., and Cadrin, S. X. (2010). Movement groups of Winter Flounder (Pseudopleuronectes americanus) in the southern Gulf of Maine: observations with the use of passive acoustic telemetry. Fish. Bull. 108, 408–419.
Dhellemmes, F., Finger, J. S., Smukall, M. J., Gruber, S. J. Guttridge, T. L., Lasskowski, K. L., et al. (2021). Personality-driven life history trade-offs differ in two subpopulations of free-ranging predators. J. Anim. Ecol. 90, 260–272. doi: 10.1111/1365-2656.13283
Eero, M., Vinther, M., Haslob, H., Huwer, B., Casini, M., Storr-Paulsen, M., et al. (2012). Spatial management of marine resources can enhance the recovery of predators and avoid local depletion of forage fish. Conserv. Lett. 5, 486–492. doi: 10.1111/j.1755-263X.2012.00266.x
Estes, J. A., Terborgh, J., Brashares, J. S., Power, M. E., Berger, J., Bond, W. J., et al. (2011). Trophic downgrading of planet earth. Science 333, 301–306.
Ferry, K. H., and Mather, M. E. (2012). Spatial and temporal diet groups of subadult and small adult striped bass in Massachusetts estuaries: data, a synthesis, and trends across scales. Mar. Coast. Fish. Dyn. Manage. Ecosyst. Sci. 4, 30–45. doi: 10.1080/19425120.2011.642747
Frank, H. J., Mather, M. E., Smith, J. M., Muth, R. M., and Finn, J. T. (2011). Role of origin and release location in pre-spawning movements of anadromous alewives. Fish. Manage. Ecol. 18, 12–24. doi: 10.1111/j.1365-2400.2010.00759.x
Frank, H. J., Mather, M. E., Smith, J. M., Muth, R. M., Finn, J. T., and McCormick, S. D. (2009). What is ‘fallback’?: metrics needed to assess telemetry tag effects on anadromous fish behavior. Hydrbiologia 35, 237–249. doi: 10.1007/s10750-009-9917-3
Frank, K. T., Petrie, B., Choi, J. S., and Leggett, W. C. (2005). Trophic cascades in a formerly cod-dominated ecosystem. Science 308, 1621–1623. doi: 10.1126/science.1113075
Gerber, K. M., Mather, M. E., Smith, J. M., and Peterson, Z. (2019). Evaluation of a field protocol for internally-tagging fish predators using difficult-to-tag ictalurid catfish as examples. Fish. Res. 209, 58–66. doi: 10.1016/j.fishres.2018.09.003
Goethel, D. R., Quinn, T. J. I. I., and Cadrin, S. X. (2011). Incorporating spatial structure in stock assessment: movement modeling in marine fish population dynamics. Rev. Fish. Sci. 19, 119–136. doi: 10.1080/10641262.2011.557451
Goetze, J. S., Januchowski-Hartley, F. A., Claudet, J., Langlois, T. J., Wilson, S. K., and Jupiter, S. D. (2017). Fish wariness is a more sensitive indicator to changes in fishing pressure than abundance, length or biomass. Ecol. Appl. 27, 1178–1189. doi: 10.1002/eap.1511
Gonzalez-Mon, B., Bodin, O., Lindkvist, E., Frawley, T. H., Giron-Nava, A., Basurto, X., et al. (2021). Spatial diversification as a mechanism to adapt to environmental changes in small-scale fisheries. Environ. Sci. Policy 116, 246–257. doi: 10.1016/j.envsci.2020.11.006
Halpern, B. S., Walbridge, S., Selkoe, K. A., Kappel, C. V., Micheli, F., D’Agrosa, C., et al. (2008). A global map of human impact on marine ecosystems. Science 319, 948–952.
Hammerschlag, N., Schmitz, O. J., Flecker, A. S., Lafferty, K. D., Atwood, T. B., Gallagher, A. J., et al. (2019). Ecosystem function and services of aquatic predators in the Anthropocene. Trends Ecol. Evol. 34, 369–383. doi: 10.1016/j.tree.2019.01.005
Harcourt, J. L., Sweetman, G., Johnstone, R. A., and Manica, A. (2009). Personality counts: the effect of boldness on shoal choice in threespined sticklebacks. Anim. Behav. 77, 1501–1505. doi: 10.1016/j.anbehav.2009.03.004
Hennig, C. (2008). Dissolution point and isolation robustness: robustness criteria for general cluster analysis methods. J. Multiv. Anal. 99, 1154–1176. doi: 10.1016/j.jmva.2007.07.002
Hertel, A. G., Niemela, P. T., Dingemanse, N. J., and Mueller, T. (2020). A guide for studying among-individual behavioral variation from movement data in the wild. Mov. Ecol. 8:30. doi: 10.1186/s40462-020-00216-
Hirsch, P. E., Thorlacius, M., Brodin, T., and Burkhardt-Holm, P. (2017). An approach to incorporate individual personality in modeling fish dispersal across in-stream barriers. Ecol. Evol. 7, 720–732. doi: 10.1002/ece3.2629
Kaufman, L., and Rousseeuw, P. J (eds). (1990). “Partitioning around medoids (program pam),” in Finding Groups in Data: An Introduction to Cluster Analysis (Hoboken, NJ: John Wiley & Sons, Inc), 68–125. doi: 10.1002/9780470316801.ch2
Kelleher, S. R., Silla, A. J., and Byrne, P. G. (2018). Animal personality and behavioral syndromes in amphibians: a review of the evidence, experimental approaches, and implications for conservation. Behav. Ecol. Sociobiol. 72:79. doi: 10.1007/s00265-018-2493-7
Kennedy, C. G., Mather, M. E., and Smith, J. M. (2017). Quantifying integrated, spatially-explicit, ecologically-relevant, physical heterogeneity within an estuarine seascape. Estuaries Coasts 40, 1385–1397. doi: 10.1007/s12237-016-0207-9
Kennedy, C. G., Mather, M. E., Smith, J. M., Finn, J. T., and Deegan, L. A. (2016). Discontinuities concentrate mobile predators: quantifying organism-environment interactions at a seascape scale. Ecosphere 7:e01226. doi: 10.1002/ecs2.1226
Kessel, S. T., Hussey, N. E., Crawford, R. E., and Yurkowski, D. (2016). Distinct groups of Arctic cod (Boreogadus saida) presence and absence in a shallow high Arctic embayment, revealed across open-water and ice-covered periods through acoustic telemetry. Polar Biol. 39, 1057–1068. doi: 10.1007/s00300-015-1723-y
Kneebone, J., Hoffman, W. S., Dean, M. J., Fox, D. A., and Armstrong, M. P. (2014). Movement patterns and stock composition of adult striped bass tagged in Massachusetts coastal waters. Trans. Am. Fish. Soc. 143, 1115–1129. doi: 10.1080/00028487.2014.889752
Kowalski, G. J., Grimm, V., Herde, A., Guenther, A., and Eccard, J. A. (2019). Does animal personality affect movement in habitat corridors? experiments with common voles (Microtus arvalis) using different corridor widths. Animals 9:291. doi: 10.3390/ani9060291
Lichtenstein, J. L. L., Rice, H. K., and Pruitt, J. N. (2018). Personality variation in two predator species does not impact prey species survival or plant damage in staged mesocosms. Behav. Ecol. Sociobiol. 72:70. doi: 10.1007/s00265-018-2487-5
Loher, T., Webster, R. A., and Carlile, D. (2017). A test of the detection range of acoustic transmitters and receivers deployed in deep waters of Southeast Alaska, USA. Anim. Biotele. 5:27.
MacDonald, C., Gallagher, A. J., Barnett, A., Brunnschweiler, J., Shiffman, D. S., and Hammerschlag, N. (2017). Conservation potential of apex predator tourism. Biol. Conserv. 215, 132–141. doi: 10.1016/j.biocon.2017.07.013
Mather, M. E., Finn, J. T., Kennedy, C. G., Deegan, L. A., and Smith, J. M. (2013). What happens in an estuary does not stay there: patterns of biotic connectivity resulting from long term ecological research. Oceanography 26, 168–179. doi: 10.5670/oceanog.2013.60
Mather, M. E., Finn, J. T., Pautzke, S. M., Fox, D., Savoy, T., and Brundage, H. M. III, et al. (2010). Destinations, routes, and timing of adult striped bass on their southward fall migration: implications for coastal movements. J. Fish Biol. 77, 2326–2337. doi: 10.1111/j.1095-8649.2010.02811.x
Merrick, M. J., and Koprowski, J. L. (2017). Should we consider individual behavior differences in applied wildlife conservation studies? Biol. Conserv. 209, 34–44. doi: 10.1016/j.biocon.2017.01.021
Mittermeier, R. A., Myers, N., Thomsen, J. B., Da Fonseca, G. A. B., and Olivieri, S. (1998). Biodiversity hotspots and major tropical wilderness areas: approaches to setting conservation priorities. Conserv. Biol. 12, 516–520. doi: 10.1046/j.1523-1739.1998.012003516.x
Nelson, G. (2018). Massachusetts Striped Bass Monitoring Report for 2017. Massachusetts Division of Marine Fisheries Technical Report TR-68. Boston, MA: Massachusetts Division of Marine Fisheries.
Nilsson, J., Bronmark, C., Hansson, L., and Chapman, B. B. (2014). Individuality in Movement: the Role of Animal Personality in Animal Movement Across Scales, eds L. Hansson and S. Åkesson (Oxford: Oxford University Press).
Pautzke, S. M., Mather, M. E., Finn, J. T., Deegan, L. A., and Muth, R. M. (2010). Seasonal use of a New England estuary by foraging contingents of migratory striped bass. Trans. Am. Fish. Soc. 139, 257–269. doi: 10.1577/t08-222.1
Poole, G. C. (2002). Fluvial landscape ecology: addressing uniqueness within the river discontinuum. Freshw. Biol. 47, 641–660. doi: 10.1046/j.1365-2427.2002.00922.x
Rice, S. P., Ferguson, R. I., and Hoey, T. B. (2006). Tributary control of physical heterogeneity and biological diversity at river confluences. Can. J. Fish. Aquatic Sci. 63, 2553–2566. doi: 10.1139/f06-145
Richards, A. R., and Rago, P. J. (1998). A Case history of effective fishery management: Chesapeake Bay striped bass. North Am. J. Fish. Manage. 19, 356–375. doi: 10.1577/1548-8675(1999)019<0356:ACHOEF>2.0.CO;2
Rulifson, R. A., and Dadswell, M. J. (1995). Life history and population characteristics of striped bass in Atlantic Canada. Trans. Am. Fish. Soc. 124, 477–507. doi: 10.1577/1548-8659(1995)124<0477:lhapco>2.3.co;2
Sabal, M. C., Michel, C. J., Smith, J. M., Hampton, A., and Hayes, S. A. (2019). Seasonal movement patterns of striped bass (Morone saxatilis) in their nonnative range. Estuaries Coasts 42, 567–579. doi: 10.1007/s12237-018-0467-7
Secor, D. H., O’Brien, M. H. P., Gahagan, B. I., Fox, D. A., Higgs, A. L., and Best, J. E. (2020). Multiple spawning run contingents and population consequences in migratory striped bass Morone saxatilis. PLoS One 15:e0242797. doi: 10.1371/journal.pone.0242797
Shelton, A. O., Sullaway, G. H., Ward, E. J., Feist, B. E., Somers, K. A., Tuttle, V. J., et al. (2020). Redistribution of salmon populations in the northeast Pacific ocean in response to climate. Fish Fish. 22, 503–517. doi: 10.1111/faf.12530
Shepherd, G. R., Nelson, G. A., Rago, P., Richards, A., Boreman, J., and Goodyear, C. P. (2018). A Chronicle of Striped Bass Population Restoration and Conservation in the Northwest Atlantic, 1979 - 2016. NOAA Technical Memorandum NMFS NE; 246. Washington, DC: U.S. Department of Commerce.
Spriegel, O., Leu, S. T., Michael Bull, C., and Sih, A. (2016). What’s your move? Movement as a link between personality and spatial dynamics in animal populations. Ecol. Lett. 20, 3–18. doi: 10.1111/ele.12708
Su, G., Logez, M., Xu, J., Tao, S., Villeger, S., and Brosse, S. (2021). Human impacts on global freshwater fish biodiversity. Science 371, 835–838. doi: 10.1126/science.abd3369
Taylor, R. B., Mather, M. E., Smith, J. M., and Gerber, K. M. (2019). Confluences function as ecological hotspots: geomorphic and regional drivers can identify patterns of fish predator distribution within a seascape. Mar. Ecol. Prog. Ser. 629, 133–148. doi: 10.3354/meps13088
Uusi-Heikkilä, S., Wolter, C., Klefoth, T., and Arlinghaus, R. (2008). A behavioral perspective on fishing-induced evolution. Trends Ecol. Evol. 23, 419–421. doi: 10.1016/j.tree.2008.04.006
Villegas-Ríos, D., Réale, D., Freitas, C., Moland, E., and Olsen, E. M. (2017). Individual level consistency and correlations of fish spatial behaviour assessed from aquatic animal telemetry. Anim. Behav. 2017, 83–94. doi: 10.1016/j.anbehav.2016.12.002
Waldman, J., Dunning, D. J., Ross, Q. E., and Mattson, M. T. (1990). Range dynamics of Hudson River Striped Bass along the Atlantic Coast. Trans. Am. Fish. Soc. 143, 1115–1129.
Wat, K., Banks, P., and McArthur, C. (2020). Linking animal personality to problem-solving performance in urban common brushtail possums. Anim. Behav. 162, 35–45. doi: 10.1016/j.anbehav.2020.01.013
Webber, Q. M. R., Laforge, M. P., Bonar, M., Robitaille, A. L., Hart, C., Zabihi-Seissan, S., et al. (2020). The ecology of individual differences empirically applied to space-use and movement tactics. Am. Nat. 196, E1–E15. doi: 10.1086/7087219
Wirgin, I., Maceda, L., Tozer, M., Stabile, J., and Waldman, J. (2020). Atlantic coastwide population structure of striped bass Morone saxatilis using microsatellite DNA analysis. Fish. Res. 226:105506. doi: 10.1016/j.fishres.2020.105506
Wolf, M., and Weissing, F. J. (2012). Animal personalities: consequences for ecology and evolution. Trends Ecol. Evol. 27, 452–461.
Keywords: striped bass, discrete groups, distribution, confluence, telemetry, cluster analysis
Citation: Taylor RB, Mather ME, Smith JM and Boles KM (2021) Can Identifying Discrete Behavioral Groups With Individual-Based Acoustic Telemetry Advance the Understanding of Fish Distribution Patterns? Front. Mar. Sci. 8:723025. doi: 10.3389/fmars.2021.723025
Received: 09 June 2021; Accepted: 04 October 2021;
Published: 25 November 2021.
Edited by:
José M. Riascos, University of Antioquia, ColombiaReviewed by:
Michael Armstrong, Massachusetts Division of Marine Fisheries, United StatesChristopher Henderson, University of the Sunshine Coast, Australia
Danielle Haulsee, Stanford University, United States
Copyright © 2021 Taylor, Mather, Smith and Boles. This is an open-access article distributed under the terms of the Creative Commons Attribution License (CC BY). The use, distribution or reproduction in other forums is permitted, provided the original author(s) and the copyright owner(s) are credited and that the original publication in this journal is cited, in accordance with accepted academic practice. No use, distribution or reproduction is permitted which does not comply with these terms.
*Correspondence: Martha E. Mather, bW1hdGhlckBrc3UuZWR1