- 1College of Fisheries, Ocean University of China, Qingdao, China
- 2School of Marine Sciences, University of Maine, Orono, ME, United States
- 3Laboratory for Marine Fisheries Science and Food Production Processes, Pilot National Laboratory for Marine Science and Technology (Qingdao), Qingdao, China
- 4Pacific Islands Fisheries Science Center, National Oceanic and Atmospheric Administration, Honolulu, HI, United States
- 5Washington, DC, United States
Single-species fisheries management (SSFM) is applied to many fisheries ecosystems around the world. The associated ecological impacts are usually not well understood due to the lack of considering trophic interactions among species in the ecosystem. This impedes the implementation of SSFM in an ecosystem context and reduces our ability to understand the possible ecological impacts of fishing activities. This study focuses on two economically important species in the Jiaozhou Bay, China: the short-lived, fast-growing, and relatively abundant Japanese mantis shrimp (Oratosquilla oratoria) and the long-lived, slow-growing, and less abundant Korean rockfish (Sebastes schlegelii). We evaluated how varying trophic interactions influenced O. oratoria and S. schlegelii (i.e., target-species) who were managed under constant fishing pressure. The increase of fishing pressure to other species (i.e., non-target species) was beneficial to O. oratoria and S. schlegelii. O. oratoria was more sensitive to the decrease of fishing pressure to other species. The predation mortality of age-0 O. oratoria increased with the increased fishing pressure to other species. The predation mortality of age-1 O. oratoria and age-0 S. schlegelii had negative relationships with the fishing pressure to other species. Age-1 S. schlegelii seemed not to be sensitive to the changes in trophic interactions. The predation mortality of O. oratoria and S. schlegelii had bigger changes than the starvation mortality after fishing changed. It suggested the prey-predator relationship had a bigger impact than the food competition. The increase of high-trophic-level fish Johnius belangerii fishery positively impacted O. oratoria, but negatively impacted S. schlegelii. S. schlegelii was more sensitive to the changes of the low-trophic-level fish Pholis fangi fishery. Given the complex dynamics of ecosystems, this study highlights the importance of species-specific responses of fishes to shifting trophic interactions in fisheries management.
Introduction
Ecosystem-based fisheries management (EBFM) is considered essential for achieving commercial growth and conserving fragile ecosystems in a changing marine environment (Trochta et al., 2018). Theories regarding holistic fishing patterns are increasingly put forward on managing marine ecosystems at an ecosystem level. For example, “balanced harvesting” calls for proportionally fishing all ecological components according to their productivity (Zhou et al., 2010; Garcia et al., 2012), while “protection of forage fish” aims at preserving the stability of food webs (Essington and Munch, 2014; Hilborn et al., 2017). With the increasing computational power over recent decades, various ecosystem models have been developed to help us understand ecosystem dynamics beneficial to optimizing decision-making processes (Travers et al., 2007). Ecosystem models are increasingly recognized as a helpful tool to facilitate the implementation of EBFM.
Although the benefits of EBFM have been demonstrated in research, few marine ecosystem currently employ any ecosystem-level management schemes. The applications of ecosystem models are often restricted by inadequate data, especially in developing countries (Costello et al., 2012; Hamel and Bryant, 2017). The high expense cost and requirement of complex trade-offs impede tactical applications of EBFM (Hilborn, 2011). Globally, single-species approaches are by far the most common tool used to inform fisheries management. Most traditional single-species approaches fail to account for trophic interactions (Skern-Mauritzen et al., 2016). It may increase the bias associated with model-based decision-making. Implementing single-species fisheries management (SSFM) with integrated trophic interactions is increasingly encouraged as an important step toward EBFM (Zhang et al., 2016).
While the total capture fishery production remains stable in China, many species of high commercial value have been replaced by species from lower trophic levels (Szuwalski et al., 2017). Such “fishing down the food webs” phenomena indicate the unsustainable exploitation (Pauly et al., 1998). Fisheries management agencies in China have installed a series of management measures to mitigate the impact of overfishing (Su et al., 2020). However, fishermen may change harvest strategies to catch non-target species as a way to offset the loss of landings from their primary managed stocks. Current regulations, such as marine protected areas (MPAs), a summer fishing moratorium, and “double control” system, are less efficient in restricting species-specific catches (Shen and Heino, 2014). Shifts in fishing effort can influence interspecies interactions (KC et al., 2017). We hypothesized that SSFM can be more efficient if trophic interactions were incorporated.
Jiaozhou Bay is a temperate bay located in the southeast Shandong Peninsula, China. The ecosystem structure of Jiaozhou Bay has altered over the last two decades due to human activities such as fishing, shoreline modifications, and water pollution. There is a decreasing trend of top predator biomass in recent years (Ma et al., 2018b). Controlling marine fishing vessels and their total engine power has been implemented to relieve fishing pressure on fisheries resources since 1987 in Chinese coastal waters (Huang and He, 2019). We focus on the management strategy that controls fishing effort on target species. Based on a developed end-to-end model OSMOSE-JZB (Object-oriented Simulator of Marine ecOSystEms; OSMOSE) (Xing et al., 2017), we evaluated the impacts of trophic interactions on the effectiveness of managing two commercial species (Oratosquilla oratoria and Sebastes schlegelii) characterized by different growth traits in the Jiaozhou Bay, China. Our analysis can improve our understanding of how varying trophic interaction influences the effectiveness of managing target species.
Materials and Methods
The Operational Model: OSMOSE-JZB
OSMOSE-JZB is a multispecies individual-based model developed to advise fisheries management in Jiaozhou Bay, China (Figure 1). The model simulates regional food webs from plankton to top predators, containing five low trophic level (LTL) groups and fourteen high trophic level (HTL) groups (Table 1). The two main trophic functional groups are modeled by the Finite Volume Coastal Ocean Model-North Pacific Ecosystem Model Used for Regional Oceanography (FVCOM-NEMURO; Chen et al., 2003; Aita et al., 2007) and OSMOSE model (Shin and Cury, 2001), respectively. In the FVCOM-NEMURO model, the hydrological characteristics are simulated by the FVCOM model. Coupling to the FVCOM model, the NEMURO model simulates the major chemical processes and dynamics of planktons in the Jiaozhou Bay. The OSMOSE model couples to the biogeochemical model based on a one-way coupling approach. The LTL groups are only available to HTL groups in predation processes. Detailed description of the FVCOM-NEMURO model can be found in Xing et al. (2017). Here, we briefly introduced the major processes and parameterization of the OSMOSE model and focused on variations of HTL groups.
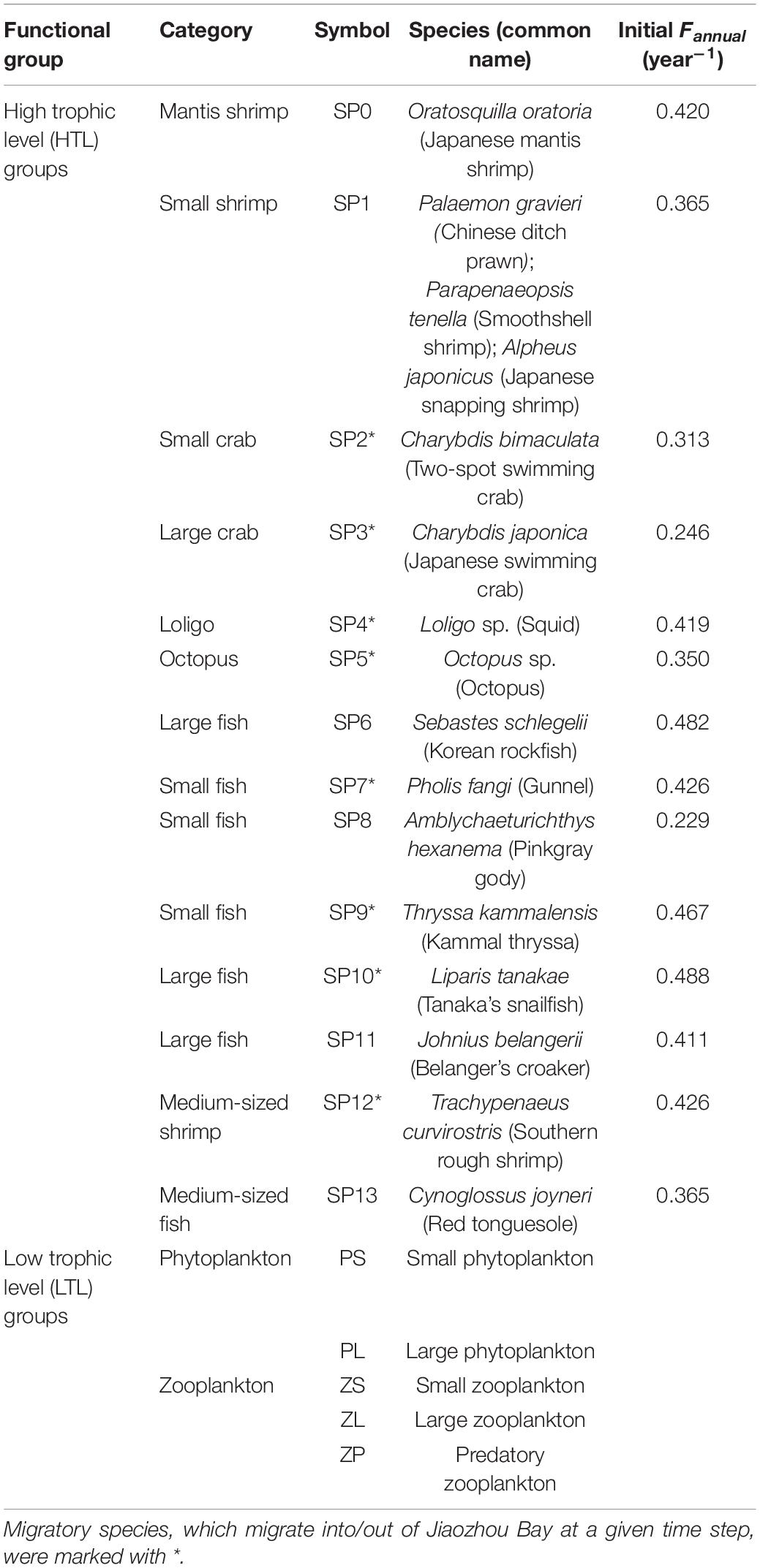
Table 1. High trophic level (HTL) and low trophic level (LTL) groups modeled in the initial OSMOSE-JZB built by Xing et al. (2017).
The OSMOSE model simulates dynamics of trophic interactions and full life cycle of HTL groups on a two-dimensional gridded map of Jiaozhou Bay which is divided into 1,435 cells (0.01° × 0.01°). The fish school (super-individual) is the basic modeling unit for carrying out biological processes. There are six processes occurring at each time step (half month), including foraging movement, predation processes, somatic growth, various mortalities, species-specific reproduction, and species migration. Foraging movement is mimicked by a random-walk that fish schools can move into the adjacent grid or stay in the current grid. The range of random-walk for each HTL group is set based on their swimming ability. As the water area of Jiaozhou Bay is small, fish schools are assumed to distribute over all the modeled water area. The predation process occurs on the condition that (1) the size of prey is within a suitable predator-prey size ratio and (2) prey and predator are in the same cell at a given time step. The individual growth is related to the prey biomass they obtain in the predation processes. If the ingested food is not enough to maintain the metabolic rate, individuals will stop growing or starve. The somatic growth is described by the von Bertalanffy growth model and weight-length relationship.
Except for mortalities derived from predation (Mpredation) and starvation (Mstarvation), there are three mortality sources considered in the OSMOSE-JZB model, including fishing mortality (Mfishing), additional natural mortality (Mnatural), and larval mortality (Mlarval). Fishing mortality is used to describe fishing impacts. The fishing mortality rate of each HTL group is assumed to be “knife-edge” that only individuals larger than minimum catch size can be harvested during the fishing season. Fishing mortality rates of all species evenly allocated during the fishing season (Xing et al., 2017). Additional natural mortality represents mortality sources which are not explicitly presented in the model. Larval mortality describes high mortality of non-fertilization and export of eggs and first feeding larvae. All mortalities are calculated based on the stochastic algorithm to ensure that mortality estimates are not biased. The HTL groups are categorized into resident species whose entire life cycle within the Jiaozhou Bay and migratory species that migrate into/out of simulated area at a given time step. The species-specific migration is characterized by age class, biomass, and migratory timing. The number of eggs spawned by individuals during the spawning season depends on matured female biomass and relative fecundity (Shin and Cury, 2004).
The OSMOSE-JZB was built based on the stratified random bottom trawl surveys conducted during February, May, August, and November of 2011 in Jiaozhou Bay. The maximum ingestion rate (r), which defines the superior limit of food intake, is set to 3.5 g of food per gram of body weight per year (Shin and Cury, 2001). The critical predation efficiency (ξcrit), which determines whether obtained food can support the growth of individuals, is set to 0.57. The minimum catch size of each HTL group is set at 5 cm in the model. The 3-month seasonal closure is considered in the model. The fishing mortality rate of each HTL group is set to zero when individuals migrate out of the simulated area or during the seasonal closure, and is averaged over other time periods. Some of the parameters for modeling processes are summarized in Supplementary Tables 1–3. Further details on the model parameterization and calibration are described in Xing et al. (2017).
Simulation Design
We simulated the SSFM that regulates fishing effort to managed species (i.e., target species). The HTL groups were further divided into two categories: (1) target species that were managed under stationary fishing mortality and (2) non-target species that were managed under different harvest strategies. Two economically important species in Jiaozhou Bay, China (the short-lived, fast-growing, and relatively abundant O. oratoria and the long-lived, slow-growing, and less abundant S. schlegeli) were selected as target species. A range of fishing mortality rates for each non-target species simulated the efficacy of managing target species when the fishing pressure to non-target species changed. The fishing mortality rate of target species was kept the same as the default OSMOSE-JZB configuration (i.e., the reference state). Harvest strategies implemented for the non-target species were imitated by changing their annual fishing mortality rates.
We considered five scenarios (i.e., S1 to S5) to simulate how changes in non-target species fisheries influence the efficacy of managing O. oratoria and S. schlegelii as target species, respectively (Figure 2):
(1) The scenario S1 described the response of O. oratoria (i.e., target species) to changes of fishing other species (i.e., non-target species). O. oratoria was managed under the stationary fishing mortality. The fishing mortality (F) of each non-target species changed by the same F-multiplier. Their fishing mortality rates were set from 0.7 to 1.3 times the initial value of the reference state with an incremental step of 0.05. The initial values of fishing mortality rate (Initial Fannual) were shown in Table 1;
(2) The scenario S2 described the response of S. schlegelii (i.e., target species) to changes of fishing other species (i.e., non-target species). The fishing mortality of S. schlegelii was unchanged. Similar to S1, the fishing mortality (F) of each non-target species was set from 0.7 to 1.3 times the initial value of the reference state with an incremental step of 0.05;
(3) In reality, the changes of fishing mortality are difficult to characterize after fishing effort changes. The scenario S3 investigated the consequences of uncertainty in non-target species fishing mortality. O. oratoria was managed as the target species under stationary fishing mortality. There were four sub-scenarios: fishing mortality rate of each non-target species was set randomly between 1 and 1.15 times the initial value (S3a), fishing mortality rate of each non-target species was set randomly between 0.85 and 1 times the initial value (S3b), fishing mortality rate of each non-target species was set randomly between 1.15 and 1.3 times the initial value (S3c), and fishing mortality rate of each non-target species was set randomly between 0.7 and 0.85 times the initial value (S3d);
(4) The fishing mortality of S. schlegelii was unchanged in the scenario S4. Similar to S3, the fishing mortality rates of other species changed at four levels: setting randomly between 1 and 1.15 times the initial value (S4a), setting randomly between 0.85 and 1 times the initial value (S4b), setting randomly between 1.15 and 1.3 times the initial value (S4c), and setting randomly between 0.7 and 0.85 times the initial value (S4d);
(5) The scenario S5 investigated the responses of O. oratoria and S. schlegelii to only changing fishing pressure to one of the other 12 HTL groups (i.e., “selected” species). There were 12 sub-scenarios that the mortality rate of each HTL group except for O. oratoria and S. schlegelii was set from 0.7 to 1.3 times the initial value with a step equal to 0.05, respectively.
To stabilize the model, all simulations had an 80 years burn-in period under the same configuration of the initial OSMOSE-JZB model built in Xing et al. (2017). The following 40 years of each configuration were used for analysis. Every simulated sub-scenario in S1, S2, and S5 was repeated 100 times to account for model stochasticity. The scenario outputs were averaged over the simulation runs. In the scenarios S3 and S4, a Monte Carlo simulation approach was employed to randomly generate fishing mortality rates for each non-target species from uniform distributions with the given bounds. There were a total of 1000 simulations in each sub-scenario in S3 and S4. The initial OSMOSE-JZB model built by Xing et al. (2017) ran 120 years with 100 simulation runs. The reference state was represented by the predicted data of the initial OSMOSE-JZB model averaged over the last 40 years and 100 repetitions.
Measurement of Fisheries Status
For S1 and S2, the predicted species biomass was used to indicate annual variations of the fish population. In terms of predator pressure and food competition, the predation and starvation mortality of two age groups, which were below 1 year old (age-0) and between 1 and 2 years old (age-1), were used to explore how altered trophic interactions influenced different fish individuals. Interannual probability frequency distribution of species biomass was used for displaying results in scenarios S3 and S4.
In S5, we conducted the linear regression analysis. The linear regression model was calculated as:
where the response variable Bio denoted O. oratoria biomass or S. schlegelii biomass; the explanatory variable Multiplier corresponded the multipliers for the “selected” species fishing mortality rate; a and b were the slope and intercept in every linear regression model.
The slopes showed the increasing rate for the impact of fishing the “selected” species on O. oratoria and S. schlegelii (Halouani et al., 2019). The indicators used in S1 to S5 were summarized in Table 2.
Results
Changes at Population Level
O. oratoria and S. schlegelii had different responses to the changes in fishing pressure on other species (Figure 3). For scenario S1, the O. oratoria biomass had a downward trend when other species fishing mortality declined especially for fishing mortality rates below eighty percent of initial values. Alternatively, there were small variations in O. oratoria biomass when the fishing pressure to other species increased. In contrast to the performance of O. oratoria shown in the scenario S1, S. schlegelii had a marked upward trend along with imposing heavy fishing pressure to non-target species, and slightly declined under low fishing pressure to other species.
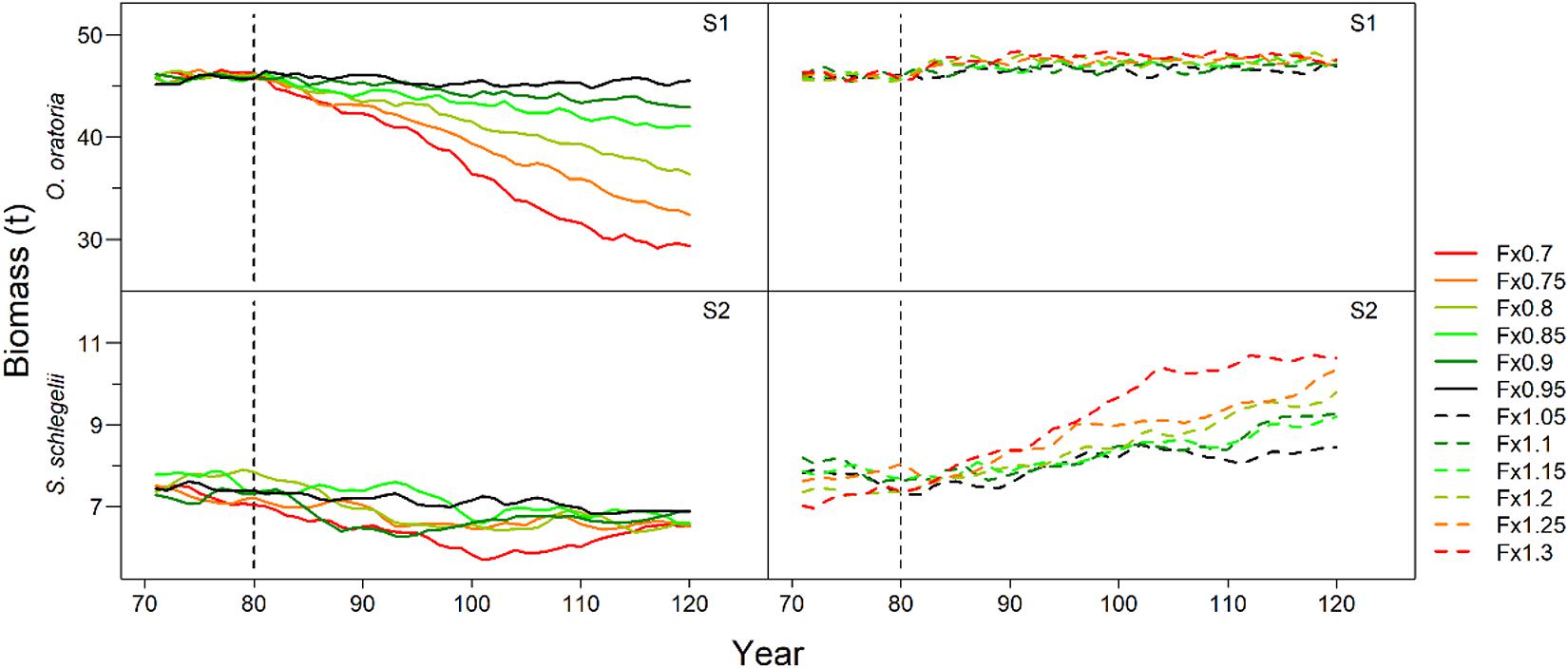
Figure 3. Interannual changes of target species biomass under different fishing scenarios. Scenarios S1 and S2 considered O. oratoria and S. schlegelii as target species, respectively. The fishing mortality rate of each non-target species was set from 0.7 (Fx0.7) to 1.3 (Fx1.3) times the initial value with a step equal to 0.05.
Changes at Individual Level
The predation mortality of O. oratoria had bigger changes than S. schlegelii (Figure 4). When the fishing pressure to other species increased, age-0 O. oratoria predation mortality increased (Figure 4A) while age-1 O. oratoria predation mortality declined (Figure 4C). By contrast, age-0 S. schlegelii predation mortality had a decrease trend with the increased fishing pressure to other species (Figure 4B). The age-1 S. schlegelii predation mortality seemed not to change under different fishing pressure levels (Figure 4D). It suggested S. schlegelii had a strong resistibility to changes in trophic interactions. There were smaller changes in the starvation mortality compared to the predation mortality (Figure 5). Both two age groups of O. oratoria experienced a lower starvation mortality after the fishing pressure to other species increased. The starvation mortality of age-1 O. oratoria had a bigger change than age-0 O. oratoria (Figures 5A,C). The changes in trophic interactions seemed not to influence the S. schlegelii starvation mortality (Figures 5B,D).
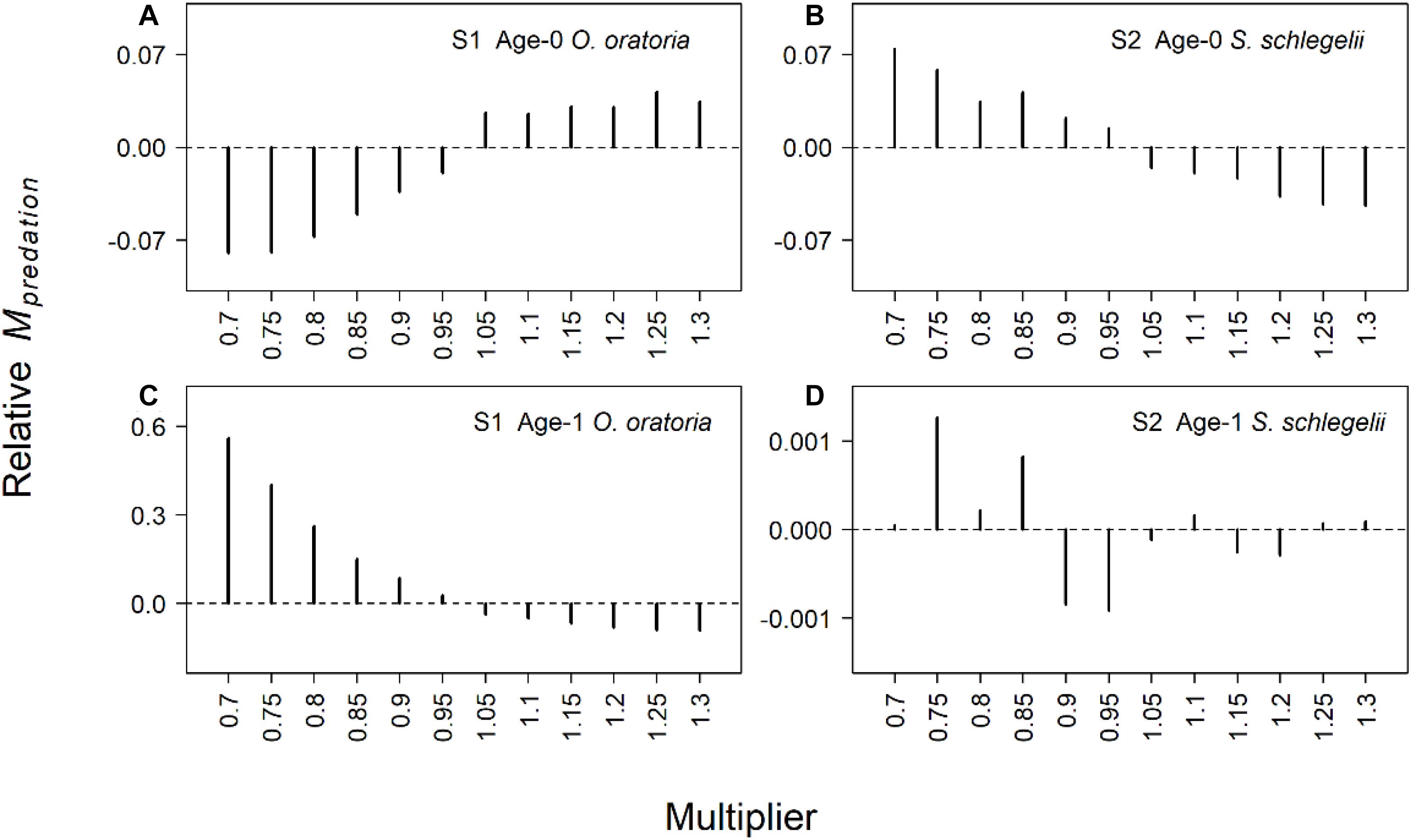
Figure 4. Relative changes of predation mortality rate for two age groups of target species under different fishing scenarios. The relative changes were the difference between scenarios S1–2 and reference state. Scenarios S1 and S2 considered O. oratoria and S. schlegelii as target species, respectively. The fishing mortality multipliers of each non-target species were set from 0.7 (Fx0.7) to 1.3 (Fx1.3) with a step equal to 0.05. (A) Relative predation mortality of age-0 O. oratoria in S1, (B) relative predation mortality of age-0 S. schlegelii in S2, (C) relative predation mortality of age-1 O. oratoria in S1, and (D) relative predation mortality of age-1 S. schlegelii in S2.
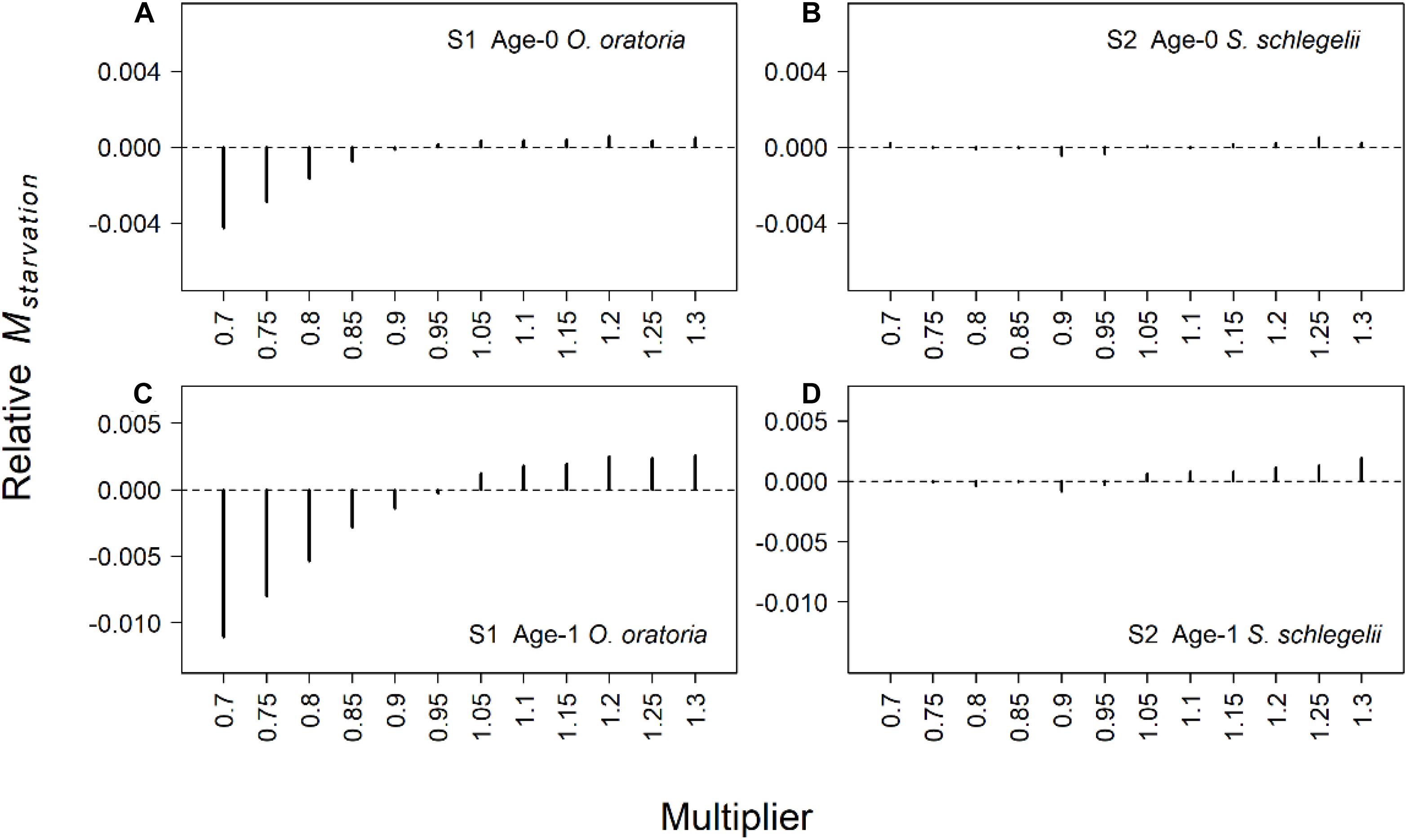
Figure 5. Relative changes of starvation mortality rate for two age groups of target species under different fishing scenarios. The relative changes were the difference between scenarios S1–2 and the reference state. Scenarios S1 and S2 considered O. oratoria and S. schlegelii as target species, respectively. The fishing mortality multipliers of each non-target species were set from 0.7 (Fx0.7) to 1.3 (Fx1.3) with a step equal to 0.05. (A) Relative starvation mortality of age-0 O. oratoria in S1, (B) relative starvation mortality of age-0 S. schlegelii in S2, (C) relative starvation mortality of age-1 O. oratoria in S1, and (D) relative starvation mortality of age-1 S. schlegelii in S2.
Uncertainty Analysis
Similar to S1 and S2 (Figure 4), the biomass of O. oratoria and S. schlegelii had positive relationships with the fishing pressure on other species (Figure 6). The biomass of O. oratoria and S. schlegelii had small variations when the fishing pressure to other species changed between 0.85 and 1.15 times the initial fishing mortality. The fluctuations of O. oratoria and S. schlegelii biomass were large when there were big changes in the fishing pressure on other species. The O. oratoria biomass markedly declined when the fishing pressure on other species changed between 0.7 and 0.85 times the initial fishing mortality.
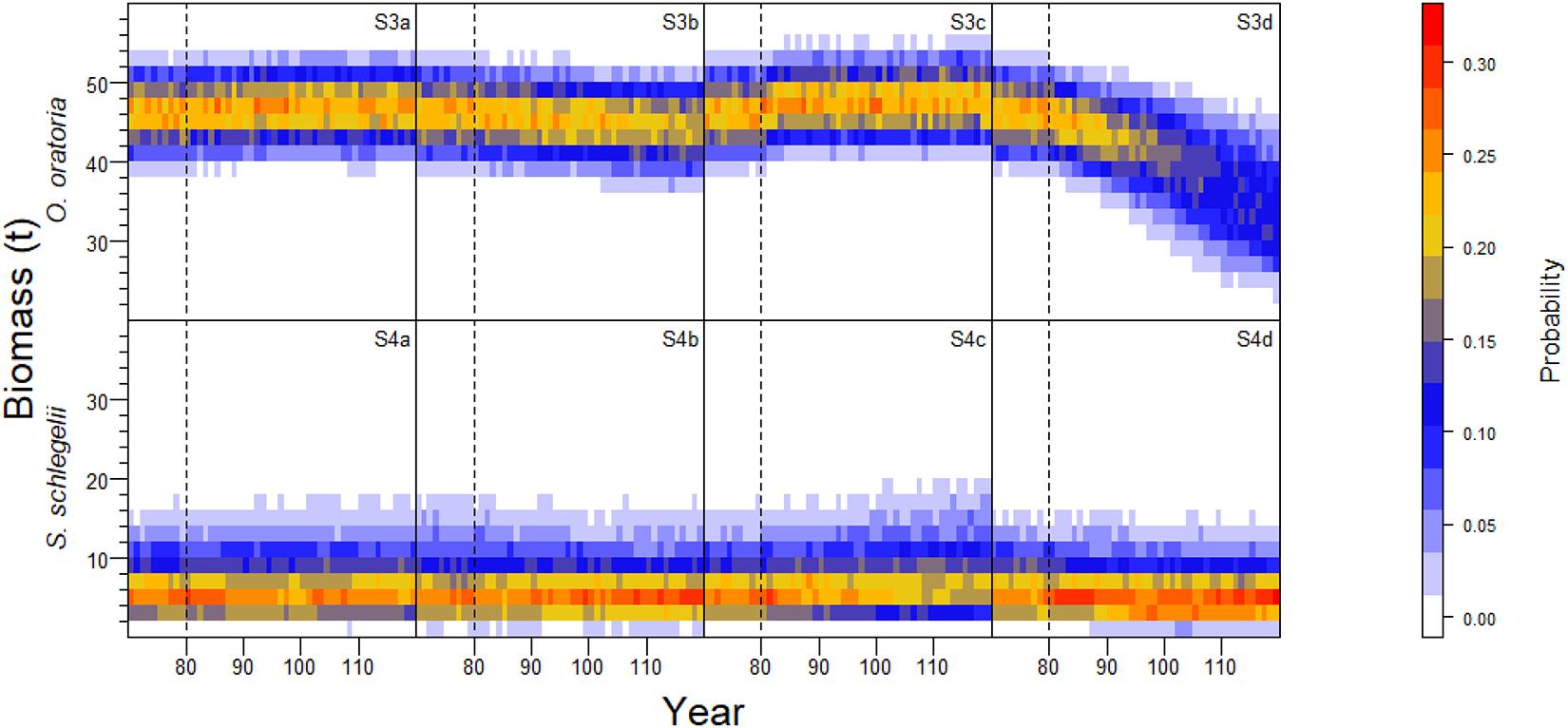
Figure 6. Interannual probability frequency distribution of population biomass of O. oratoria and S. schlegelii with 1000 Monte Carlo simulation runs at the uncertainty level of fishing mortality rates specified for non-target species in each sub-scenario.
Linear Regression Analysis
There were overall positive relationships between O. oratoria biomass and “selected” species fishing mortality rates except for the Charybdis bimaculata (SP2) and Octopus sp. (SP5). Small shrimp (SP1), Pholis fangi (SP7), Thryssa kammalensis (SP9), and Johnius belangerii (SP11) had significant linear relationships with O. oratoria (P-value < 0.05). The slope of O. oratoria was higher when the J. belangerii fishing mortality rates changed compared to other species. The increase of fishing pressure to J. belangerii was more beneficial to O. oratoria (Figure 7A). The S. schlegelii biomass had an upward trend with increasing the fishing pressure to small shrimp, Octopus sp., P. fangi, Amblychaeturichthys hexanema (SP8), T. kammalensis, and Cynoglossus joyneri (SP13). There were significant linear relationships between S. schlegelii biomass and P. fangi fishing mortality (P-value < 0.05). The slope of S. schlegelii was highest when the P. fangi fishing mortality rates changed. In contrast to O. oratoria, the decline of fishing pressure to J. belangerii was beneficial to S. schlegelii (Figure 7B).
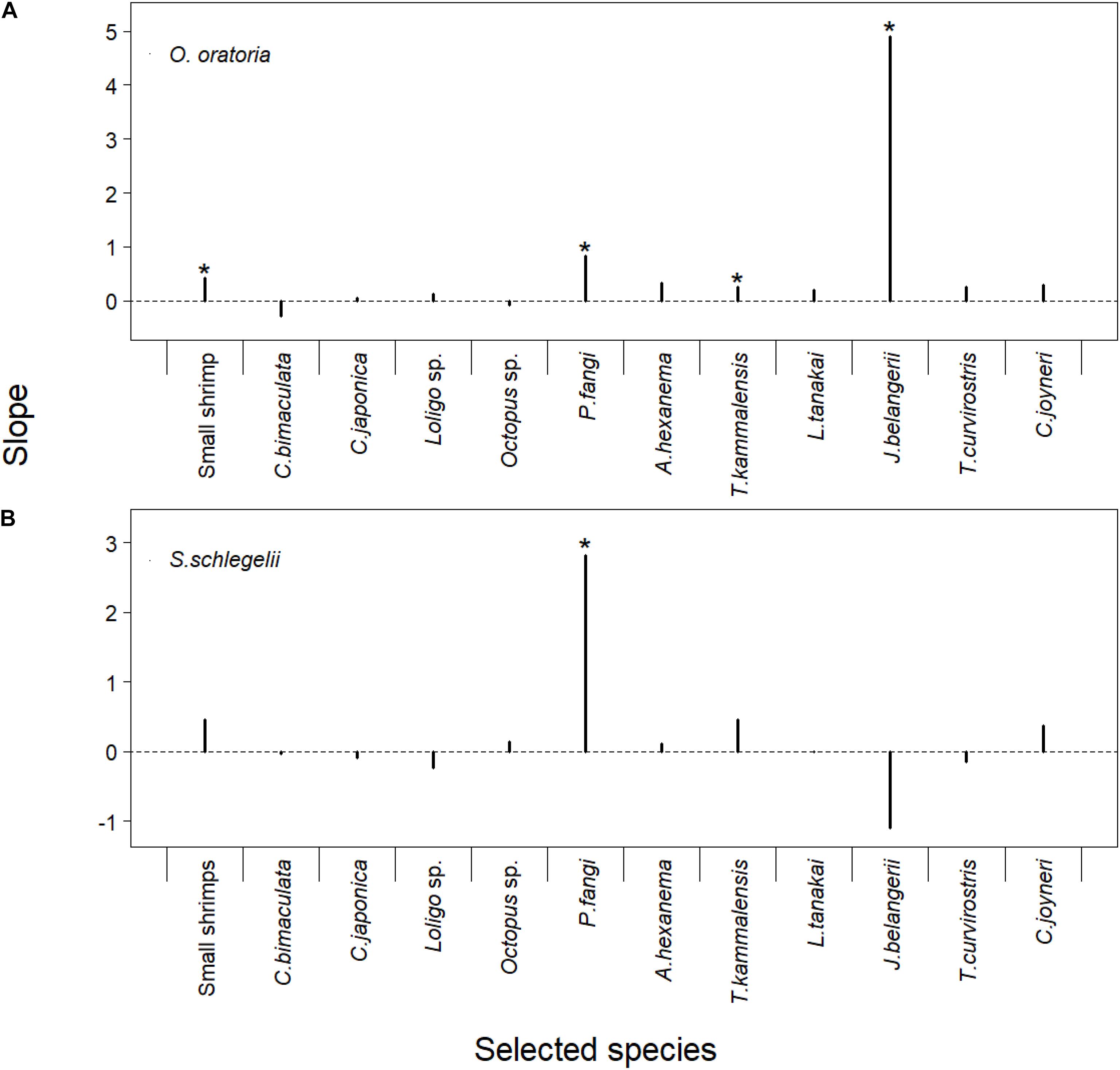
Figure 7. Linear regression model of corresponding multipliers of fishing mortality rates for the “selected” species and biomass of O. oratoria (A) or S. schlegelii (B). The detailed results presented in Supplementary Table 4. The “selected species” showed in the X-axis. The regression models whose P-values were lower than 0.05 were marked with *.
Discussion
Trophic interactions play an important role in influencing the fish population (Fu et al., 2020; Xing et al., 2020a). The impacts of fishing other species on the low-trophic-level mantis shrimp O. oratoria were complex. On the one hand, the proportion of large fishes in fish community would increase with the reduced fishing effort (Xing et al., 2021). The increase of large fish biomass was beneficial to age-0 O. oratoria via influencing small fishes who fed on age-0 O. oratoria (Figure 4A). On the other hand, the increased biomass of predators resulted in the decline of age-1 O. oratoria biomass (Figure 4C). As the top predator in the Jiaozhou Bay (Han et al., 2017), age-1 S. schlegelii had a strong resistibility to changes of trophic interactions (Figure 4D). The changes of other species fisheries were more likely to influence age-0 S. schlegelii (Figure 4B). The food condition influences the growth of fish population especially for low-trophic-level species (Fu et al., 2020). There was a positive relationship between O. oratoria biomass and starvation mortality. The starvation mortality of S. schlegelii seemed not to change when the S. schlegelii biomass increased (Figure 5). It was mainly attributed to (1) S. schlegelii population was much smaller than O. oratoria population and (2) S. schlegelii fed on more species in the Jiaozhou Bay (Ma et al., 2018a). The changes in predation mortality of O. oratoria and S. schlegelii were bigger than starvation mortality. It implied the impacts of prey-predator processes on O. oratoria and S. schlegelii were bigger than the food competition.
Han et al. (2017) reported the negative relationship between demersal fishes (e.g., J. belangerii) and O. oratoria. The linear regression analysis showed the increase of J. belangerii fishing mortality had a bigger positive impact on O. oratoria compared to other species fisheries (Figure 7A). Small fishes can influence large juvenile fish via trophic interactions (Vergnon et al., 2008). S. schlegelii was more sensitive to the change of P. fangi fishery (Figure 7B). The modeled spawning season of S. schlegelii was set from May to June based on the study of Lin (2013). The P. fangi biomass markedly increased since March and peaked in June (Xing et al., 2017). A large number of P. fangi in May and June certainly had a negative impact on the recruitment of S. schlegelii stock. There was an overlap of trophic niches between S. schlegelii and J. belangerii (Ma et al., 2015). Interestingly, S. schlegelii gained benefits from the decline of fishing pressure to J. belangerii. The low-trophic-level species have been dominant in the Jiaozhou Bay as a long-term overfishing. The food competition between S. schlegelii and J. belangerii was low as abundant prey biomass and their small population size (Xing et al., 2017). Moreover, the increase of J. belangerii biomass could reduce the impact of small fish on age-0 S. schlegelii via predator-controlled processes.
It is a consensus of implementing fisheries management in an ecosystem context (Herrón et al., 2019). Successful SSFM is built on the understanding of ecological dynamics as well as fishing (Hilborn, 2011). Stock assessment may be biased without considering possible ecological processes (Fu et al., 2018). Our study highlighted identifying fisheries management strategies should incorporate a broader consideration of species-specific characteristics. The “fishing less” and “fishing more” are potential adaptive harvest strategies in response to the decline of natural resources (Cinner et al., 2011). Catch reduction and high labor cost will result in the decline of fishing effort. Han et al. (2018) reported the high-trophic-level species biomass increased and low-trophic-level species declined with removing fishing. The fast growth and high reproduction supported O. oratoria to recover quickly from heavy fishing. However, O. oratoria would be at a disadvantage with competing with other species when the fishing effort on other species is reduced. This might result in the further decline of O. oratoria stock. Thus, the fishery management may be less effective in the case of simply emphasizing the direct fishing impact on O. oratoria. S. schlegelii have a stronger resistibility to the increase of other species biomass. S. schlegelii fishery management should pay more attention to the direct fishing impact. Growing market demand and subsidy policy encourage fishermen to catch more high-trophic-level species with a high commercial value. It will be a risk to S. schlegelii fishery when J. belangerii fishery increased and P. fangi fishery decreased.
Complex and hierarchical model structure inevitably introduces uncertainty into modeling processes that weaken the confidence of stakeholders and hampers their applications in tactical management (Moffit et al., 2016). We quantified uncertainty in fishing efforts to solidify our findings. Similar to findings in S1 and S2, the results of S3 and S4 suggested that intensifying the extent of fishing efforts exposed to other species could result in a larger variation on target species biomass. But the understanding of the impacts of core parameters on model outputs is still needed (Xing et al., 2020b). Except for overfishing, climate change threatens the sustainable development of fisheries. Moullec et al. (2019) report the sea warming will result in a decline in the proportion of large fishes. Climate change can influence fishes by changing the primary productivity (Fu et al., 2020). The changes in fishing and environmental factors will likely influence the population processes such as fish growth and recruitment (Szuwalski and Hollowed, 2016; Martino et al., 2019). Fisheries management can benefit from consideration of both interspecies interactions and climate change (Travers-Trolet et al., 2014). The future application of our model should further investigate the impact of changed trophic interactions resulting from fishing impacts and environment variability.
Conclusion
Single-species approaches may perform inefficiently for ecosystem-level management because of ignoring comprehensive fishing impacts on the ecosystem (Jacobsen et al., 2017). End-to-end modeling approaches like the one used in this study can be used to investigate the impact of changed trophic interactions. As the specificity of ecosystem and complex interspecies interactions, the understanding of trophic dynamics in a particular ecosystem is important for an effective decision-making process. The simulations demonstrate fisheries management should be considered in a more comprehensive perspective instead of only emphasizing direct fishing impacts on managed fish stocks. Admittedly, some of our conclusions and consequences are similar to the studies in other fisheries ecosystems. The study presents an ecosystem approach aiming to facilitate the implementation of SSFM in an ecosystem context, and is an attempt in narrowing the gap in using the ecosystem modeling approach to advise fisheries management.
Data Availability Statement
The raw data supporting the conclusions of this article will be made available by the authors, without undue reservation.
Author Contributions
LX: conceptualization, data curation, formal analysis, methodology, software, and writing – original draft. YC: conceptualization, methodology, supervision, and writing – review and editing. BL, KT, and RB: methodology and writing – review and editing. YR: data curation, supervision, and writing – review and editing. BX: data curation, supervision, and funding acquisition. All authors fully participated in the project, accepted the responsibility for the work, and agreed to the submission.
Funding
This study was supported by the National Key R&D Program of China (2018YFD0900904 and 2018YFD0900906). The senior author’s study at Chen’s lab at the University of Maine was supported by the China Scholarship Council, Ocean University of China, and University of Maine. All sources of funding are acknowledged in the manuscript.
Conflict of Interest
The authors declare that the research was conducted in the absence of any commercial or financial relationships that could be construed as a potential conflict of interest.
Publisher’s Note
All claims expressed in this article are solely those of the authors and do not necessarily represent those of their affiliated organizations, or those of the publisher, the editors and the reviewers. Any product that may be evaluated in this article, or claim that may be made by its manufacturer, is not guaranteed or endorsed by the publisher.
Acknowledgments
The authors are grateful to the editor and two peer reviewers for helpful comments and edits.
Supplementary Material
The Supplementary Material for this article can be found online at: https://www.frontiersin.org/articles/10.3389/fmars.2021.698991/full#supplementary-material
Partial equations and parameter values for major processes modeled in the initial OSMOSE-JZB model.
Supplementary Table 1 | Summary of major processes modeled in the OSMOSE-JZB model (Shin and Cury, 2004; Xing et al., 2017).
Supplementary Table 2 | Summary of partial input parameters for foraging, migration, and suitable predator-prey size ratio in the OSMOSE-JZB model (Xing et al., 2017).
Supplementary Table 3 | Summary of partial input parameters for growth, reproduction, and mortality rate in the OSMOSE-JZB model (Xing et al., 2017).
Supplementary Table 4 | Linear regression of species biomass and corresponding multipliers for fishing mortality rates.
References
Aita, M. N., Yamanaka, Y., and Kishi, M. J. (2007). Interdecadal variation of the lower trophic ecosystem in the northern Pacific between 1948 and 2002, in a 3-D implementation of the NEMURO model. Ecol. Model. 202, 81–94. doi: 10.1016/j.ecolmodel.2006.07.045
Chen, C., Liu, H., and Beardsley, R. C. (2003). An unstructured grid, finite-volume, three-dimensional, primitive equations ocean model: application to coastal ocean and estuaries. J. Atmos. Ocean. Technol. 20, 159–186. doi: 10.1175/1520-04262003020<0159:AUGFVT<2.0.CO;2
Cinner, J. E., Folke, C., Daw, T., and Hicks, C. C. (2011). Responding to change: using scenarios to understand how socioeconomic factors may influence amplifying or dampening exploitation feedbacks among Tanzanian fishers. Glob. Environ. Change 21, 7–12. doi: 10.1016/j.gloenvcha.2010.09.001
Costello, C., Ovando, D., Hilborn, R., Gaines, S. D., Deschenes, O., and Lester, S. E. (2012). Status and solutions for the world’s unassessed fisheries. Science 338, 517–520. doi: 10.1126/science.1223389
Essington, T. E., and Munch, S. B. (2014). Trade-offs between supportive and provisioning ecosystem services of forage species in marine food webs. Ecol. Appl. 24, 1543–1557. doi: 10.1890/13-1403.1
Fu, C., Travers-Trolet, M., Velez, L., Grüss, A., Bundy, A., Shannon, L. J., et al. (2018). Risky business: the combined effects of fishing and changes in primary productivity on fish communities. Ecol. Model. 368, 265–276. doi: 10.1016/j.ecolmodel.2017.12.003
Fu, C., Xu, Y., Guo, C., Olsen, N., Grüss, A., Liu, H., et al. (2020). The cumulative effects of fishing, plankton productivity, and marine mammal consumption in a marine ecosystem. Front. Mar. Sci. 7:565699. doi: 10.3389/fmars.2020.565699
Garcia, S. M., Kolding, J., Rice, J., Rochet, M. J., Zhou, S., Arimoto, T., et al. (2012). Reconsidering the consequences of selective fisheries. Science 335, 1045–1047. doi: 10.1126/science.1214594
Halouani, G., Le Loc’h, F., Shin, Y. J., Velez, L., Hattab, T., Romdhane, M. S., et al. (2019). An end-to-end model to evaluate the sensitivity of ecosystem indicators to track fishing impacts. Ecol. Indic. 98, 121–130. doi: 10.1016/j.ecolind.2018.10.061
Hamel, P., and Bryant, B. P. (2017). Uncertainty assessment in ecosystem services analyses: seven challenges and practical responses. Ecosyst. Serv. 24, 1–15. doi: 10.1016/j.ecoser.2016.12.008
Han, D., Chen, Y., Zhang, C., Ren, Y., Xu, B., and Xue, Y. (2018). Evaluation of effects of shellfish aquaculture and capture fishery on a semi-closed bay ecosystem. Estuar. Coast. Shelf Sci. 207, 175–182. doi: 10.1016/j.ecss.2018.04.005
Han, D., Xue, Y., Zhang, C., and Ren, Y. (2017). A mass balanced model of trophic structure and energy flows of a semi-closed marine ecosystem. Acta Oceanol. Sin. 36, 60–69. doi: 10.1007/s13131-017-1071-6
Herrón, P., Castellanos-Galindo, G. A., Stäbler, M., Díaz, J. M., and Wolff, M. (2019). Toward ecosystem-based assessment and management of small-scale and multi-gear fisheries: insights from the tropical eastern Pacific. Front. Mar. Sci. 6:127. doi: 10.3389/fmars.2019.00127
Hilborn, R. (2011). Future directions in ecosystem based fisheries management: a personal perspective. Fish. Res. 108, 235–239. doi: 10.1016/j.fishres.2010.12.030
Hilborn, R., Amoroso, R. O., Bogazzi, E., Jensen, O. P., Parma, A. M., Szuwalski, C., et al. (2017). When does fishing forage species affect their predators? Fish. Res. 191, 211–221. doi: 10.1016/j.fishres.2017.01.008
Huang, S., and He, Y. (2019). Management of China’s capture fisheries: review and prospect. Aquac. Fish. 4, 173–182. doi: 10.1016/j.aaf.2019.05.004
Jacobsen, N. S., Burgess, M. G., and Andersen, K. H. (2017). Efficiency of fisheries is increasing at the ecosystem level. Fish Fish. 18, 199–211. doi: 10.1111/faf.12171
KC, K. B., Bond, N., Fraser, E. D. G., Elliott, V., Farrell, T., McCann, K., et al. (2017). Exploring tropical fisheries through fishers’ perceptions: fishing down the food web in the Tonlé Sap, Cambodia. Fish. Manag. Ecol. 24, 452–459. doi: 10.1111/fme.12246
Lin, Y. (2013). Reproductive Physiological and Developmental Biology Research in Sebastes Schlegeli Hilgendorf. Ph.D. thesis. Qingdao: Ocean University of China.
Ma, M., Chen, Z., Xu, Y., Zhang, K., Yuan, W., and Xu, S. (2018a). Analysis of structure and energy flow in Jiaozhou Bay ecosystem based on Ecopath model. Chin. J. Ecol. (in Chinese) 37, 462–470. doi: 10.13292/j.1000-4890.201802.020
Ma, M., Xu, S., Xu, Y., Zhang, K., Yuan, W., and Chen, Z. (2018b). Comparative study of Jiaozhou Bay ecosystem based on an Ecopath model. J. Fish. Sci. China (in Chinese) 25, 413–422. doi: 10.3724/SP.J.1118.2018.17335
Ma, Q., Han, D., Liu, H., Xue, Y., Ji, Y., and Ren, Y. (2015). Construction of a continuous trophic spectrum for the food web in jiaozhou bay using stable isotope analyses. Acta Ecol. Sin. (in Chinese) 35, 7207–7218. doi: 10.5846/stxb201310312632
Martino, J. C., Fowler, A. J., Doubleday, Z. A., Grammer, G. L., and Gillanders, B. M. (2019). Using otolith chronologies to understand long-term trends and extrinsic drivers of growth in fisheries. Ecosphere 10:e02553. doi: 10.1002/ecs2.2553
Moffit, E. A., Punt, A. E., Holsman, K., Aydin, K. Y., Ianelli, J. N., and Ortiz, I. (2016). Moving towards ecosystem-based fisheries management: options for parameterizing multi-species biological reference points. Deep Sea Res 2 Top Stud. Oceanogr. 134, 350–359. doi: 10.1016/j.dsr2.2015.08.002
Moullec, F., Barrier, N., Drira, S., Guilhaumon, F., Marsaleix, P., Somot, S., et al. (2019). An end-to-end model reveals losers and winners in a warming mediterranean sea. Front. Mar. Sci. 6:345. doi: 10.3389/fmars.2019.00345
Pauly, D., Christensen, V., Dalsgaard, J., Froese, R., and Torres, F. (1998). Fishing down marine food webs. Nature 279, 860–863. doi: 10.1126/science.279.5352.860
Shen, G., and Heino, M. (2014). An overview of marine fisheries management in China. Mar. Policy 44, 265–272. doi: 10.1016/j.marpol.2013.09.012
Shin, Y. J., and Cury, P. (2001). Exploring fish community dynamics through size-dependent trophic interactions using a spatialized individual-based model. Aquat. Living Resour. 14, 65–80. doi: 10.1016/S0990-7440(01)01106-8
Shin, Y. J., and Cury, P. (2004). Using an individual-based model of fish assemblages to study the response of size spectra to changes in fishing. Can. J. Fish. Aquat. Sci. 61, 414–431. doi: 10.1139/f03-154
Skern-Mauritzen, M., Ottersen, G., Handegard, N. O., Huse, G., Dingsør, G. E., Stenseth, N. C., et al. (2016). Ecosystem processes are rarely included in tactical fisheries management. Fish Fish. 17, 165–175. doi: 10.1111/faf.12111
Su, S., Tang, Y., Chang, B., Zhu, W., and Chen, Y. (2020). Evolution of marine fisheries management in China from 1949 to 2019: how did China get here and where does China go next? Fish Fish. 21, 435–452. doi: 10.1111/faf.12439
Szuwalski, C. S., Burgess, M. G., Costello, C., and Gaines, S. D. (2017). High fishery catches through trophic cascades in China. P. Natl. Acad. Sci. U.S.A. 114, 717–721. doi: 10.1073/pnas.1612722114
Szuwalski, C. S., and Hollowed, A. B. (2016). Climate change and non-stationary population processes in fisheries management. ICES J. Mar. Sci. 73, 1297–1305. doi: 10.1093/icesjms/fsv229
Travers, M., Shin, Y. J., Jennings, S., and Cury, P. (2007). Towards end-to-end models for investigating the effects of climate and fishing in marine ecosystems. Prog. Oceanogr. 75, 751–770. doi: 10.1016/j.pocean.2007.08.001
Travers-Trolet, M., Shin, Y. J., Shannon, L. J., Moloney, C. L., and Field, J. G. (2014). Combined fishing and climate forcing in the southern benguela upwelling ecosystem: an end-to-end modelling approach reveals dampened effects. PLoS One 9:e94286. doi: 10.1371/journal.pone.0094286
Trochta, J. T., Pons, M., Rudd, M. B., Krigbaum, M., Tanz, A., and Hilborn, R. (2018). Ecosystem-based fisheries management: perception on definitions, implementations, and aspirations. PLoS One 13:e0190467. doi: 10.1371/journal.pone.0190467
Vergnon, R., Shin, Y. J., and Cury, P. (2008). Cultivation, allee effect and resilience of large demersal fish populations. Aquat. Living Resour. 21, 287–295. doi: 10.1051/alr:2008042
Xing, L., Chen, Y., Boenish, R., Tanaka, K. R., Barrier, N., and Ren, Y. (2021). Evaluating the impacts of fishing and migratory species in a temperate bay of China using the ecosystem model OSMOSE-JZB. Fish. Res. 243:106051. doi: 10.1016/j.fishres.2021.106051
Xing, L., Chen, Y., Zhang, C., Li, B., Shin, Y. J., and Ren, Y. (2020a). Evaluating impacts of pulse fishing on the effectiveness of seasonal closure. Acta. Oceanol. Sin. 39, 89–99. doi: 10.1007/s13131-020-1536-x
Xing, L., Chen, Y., Zhang, C., Li, B., Tanaka, K. R., Boenish, R., et al. (2020b). Evaluating impacts of imprecise parameters on the performance of an ecosystem model OSMOSE-JZB. Ecol. Model. 419:108923. doi: 10.1016/j.ecolmodel.2019.108923
Xing, L., Zhang, C., Chen, Y., Shin, Y. J., Verley, P., Yu, H., et al. (2017). An individual-based model for simulating the ecosystem dynamics of Jiaozhou Bay. China. Ecol. Model. 360, 120–131. doi: 10.1016/j.ecolmodel.2017.06.010
Zhang, C., Chen, Y., and Ren, Y. (2016). An evaluation of implementing long-term MSY in ecosystem-based fisheries management: incorporating trophic interaction, bycatch and uncertainty. Fish. Res. 174, 179–189. doi: 10.1016/j.fishres.2015.10.007
Keywords: OSMOSE, single-species fisheries management, prey-predator process, food competition, Jiaozhou Bay
Citation: Xing L, Chen Y, Li B, Tanaka KR, Boenish R, Ren Y and Xu B (2021) Evaluating Impacts of Trophic Interactions on the Effectiveness of Single-Species Fisheries Management. Front. Mar. Sci. 8:698991. doi: 10.3389/fmars.2021.698991
Received: 22 April 2021; Accepted: 21 June 2021;
Published: 23 July 2021.
Edited by:
Michael Phillips, World Fish, MalaysiaReviewed by:
Biju Kumar Kumar, University of Kerala, IndiaYuan Li, Third Institute of Oceanography, State Oceanic Administration, China
Copyright © 2021 Xing, Chen, Li, Tanaka, Boenish, Ren and Xu. This is an open-access article distributed under the terms of the Creative Commons Attribution License (CC BY). The use, distribution or reproduction in other forums is permitted, provided the original author(s) and the copyright owner(s) are credited and that the original publication in this journal is cited, in accordance with accepted academic practice. No use, distribution or reproduction is permitted which does not comply with these terms.
*Correspondence: Binduo Xu, YmR4dUBvdWMuZWR1LmNu