- 1The Nature Conservancy, Caribbean Division, Coral Gables, FL, United States
- 2Center for Global Discovery and Conservation Science, Arizona State University, Tempe, AZ, United States
- 3The Nature Conservancy, Hawai’i & Palmyra Programs, Honolulu, HI, United States
- 4School of Biological, Environmental, and Earth Sciences, The University of Southern Mississippi, Hattiesburg, MS, United States
Over the past decade, coral restoration efforts have increased as reefs continue to decline at unprecedented rates. Identifying suitable coral outplanting locations to maximize coral survival continues to be one of the biggest challenges for restoration practitioners. Here, we demonstrate methods of using derivatives from imaging spectroscopy from the Global Airborne Observatory (GAO) to identify suitable coral outplant sites and report on the survival rates of restored coral at those sites. Outplant sites for a community-based, citizen science outplant event in Bávaro, Dominican Republic, were identified using expert-defined criteria applied to a suitability model from data layers derived from airborne imagery. Photo quadrat analysis of the benthic community confirmed the accuracy of airborne remote sensing maps with live coral cover averaging 3.5–4% and mean algal cover (macro algae and turf) ranging from 28 to 32%. Coral outplant sites were selected at 3–7 m depth with maximized levels of habitat complexity (i.e., rugosity) and live coral cover and minimized levels of macroalgal cover, as predicted by the imaging spectrometer data. In November 2019, 1,722 Acropora cervicornis fragments (80–180 mm in length) were outplanted to these sites. Surveys conducted in January 2020 in four of these sites confirmed that 92% of outplants survived after 3 months. By October 2020 (11 months after outplanting), survivorship remained above 76%. These results demonstrate higher than average success rates for coral outplant survival for this species. An online tool was developed to enable replication and facilitate future selection of coral restoration sites. Our objective is to present a case study that uses GAO-derived map products within a suitability model framework to provide a quantitative and replicable method for selecting coral restoration sites with the goal of increasing outplant survival over time.
Introduction
Coral reefs provide tremendous economic benefits to coastal communities around the world, including fisheries production, tourism revenue, and coastal protection. Although they occupy less than 1% of the world’s ocean area, coral reefs are one of the most diverse ecosystems on the planet, providing essential habitat for one-quarter of all known marine species (Plaisance et al., 2011). Despite decades of continued effort to protect and restore coral reefs, these ecosystems continue to decline under a growing array of local and global threats, such as overfishing, pollution, and climate change-driven temperature extremes (Burke et al., 2011; Wear, 2016). The rapid decline of reef-building corals has led to a concomitant loss of structural complexity and biodiversity (Alvarez-Filip et al., 2009), reducing the ability of coral reefs to deliver critical ecosystem services that contribute to the well-being and economic livelihoods of millions of people (Hughes et al., 2010, 2017, Hoegh-Guldberg et al., 2019). While this trend has been increasingly recorded across the globe, Caribbean reefs have been among the most severely impacted during the past few decades, with decreases in coral cover by more than 50% since the 1970s (Jackson et al., 2014) and 90% of the region’s remaining reef systems being classified as threatened (Plaisance et al., 2011).
Given the ecological and economic importance of coral reefs, new approaches are needed to sustain their ecosystem function. Reef restoration aims to facilitate the recovery of damaged or degraded coral reef ecosystems that are unable to do so naturally (Hobbs and Cramer, 2008). While Marine Protected Areas are needed to support fish diversity and trophic structure, their establishment is not always sufficient to ensure coral reef recovery in the face of increasing threats and subsequent reef degradation (Cox et al., 2017). There is an urgent need for effective methods to strategically restore and augment the recovery of coral reefs and the ecosystem functions they provide (Boström-Einarsson et al., 2020). Coral restoration is a relatively new field and efforts in the Caribbean have focused on the recovery of endangered coral populations (e.g., Acropora palmata and A. cervicornis) and are increasingly expanding to restore the structure and function of coral communities and ecosystems (Boström-Einarsson et al., 2020; Bayraktarov et al., 2020). These restoration activities are driving an increased environmental stewardship awareness and community-based interest and action in protecting coral reefs (dela Cruz et al., 2015; Chamberland et al., 2017).
One significant challenge of coral restoration has been the selection of sites that will provide the best chance of restoration success (Foo and Asner, 2019). For years, the need for the selection of suitable outplant sites based on logistical, ecological, and physical factors that are conducive to coral survival has been acknowledged (Hernández-Delgado et al., 2014). Commonly, A. cervicornis restoration efforts in the Caribbean identify outplant sites by considering a wide array of factors, including logistical factors (e.g., distance from nursery and accessibility), ecological factors (coral cover, macroalgae cover, herbivore, and predator abundance), and physical factors (depth, water quality, temperature, and water flow) (Johnson et al., 2011; Hernández-Delgado et al., 2014, Mercado-Molina et al., 2015; Ladd et al., 2018). However, site selection has been traditionally accomplished via SCUBA-based surveys, which covers a limited portion of the potential habitat available to be restored and can be time and resource intensive. Additionally, there is an increasing call for coral reef restoration efforts to incorporate considerations of climate change and resilience characteristics, including indicators such as connectivity, biodiversity, and temperature variability (McClanahan et al., 2012; Shaver et al., 2020). Advancements in remote sensing provide important and novel opportunities for coral reef restoration site selection, including locating new sites, reducing in-water time to find sites, and the ability to incorporate key resilience indicators and climate change projections into restoration to target efforts that maximize coral success and the scalability of outplanting efforts (Foo and Asner, 2020).
Recent advances in coral science are providing exciting new methods to support reef restoration at broader scales (Boström-Einarsson et al., 2020). However, these methods and technologies must be integrated, tested, and applied at adequate scales to make a demonstrable impact on coral reef ecosystem recovery. Additionally, the rapid pace of reef degradation demands proactive collaboration and knowledge sharing among conservation organizations, scientists, governments, for-profit companies, and community stakeholders in the Caribbean and globally. The cost of coral restoration has been estimated from $1,717 up to $2,879,773 USD per hectare (Bayraktarov et al., 2016), and some research suggests that in certain contexts, current costs outweigh benefits (De Groot et al., 2013). Remotely sensed data can be economically incorporated into restoration activities because high spatial and spectral resolution data can be collected from airborne sensors over large areas (thousands to millions of hectares) with costs of ∼$0.01 USD per hectare at non-profit rates (Asner et al., 2014). Thus, the use of remote sensing for selecting suitable coral restoration outplant sites provides a cost-effective way to increase restoration success across large areas, particularly when multiple government agencies or other entities combine resources to map multiple project areas during a single field campaign.
With the purpose of integrating new remote sensing technologies into the strategic selection of coral reef restoration sites, The Nature Conservancy (TNC) partnered with the Asner Lab of Arizona State University to collect high resolution imaging spectrometer data over the southeast Dominican Republic in May 2017 using the Global Airborne Observatory (GAO) (Asner et al., 2012). The area mapped is part of the Santuario Marino Arrecifes del Sureste (SMASE), the second largest protected area in the Dominican Republic, where intensifying tourism activities increasingly threaten vulnerable marine ecosystems. SMASE has a co-management arrangement contained in a 25-year agreement with the Ministry of Environment and Natural Resources and a unique public-private partnership among local and international institutions, including Fundacion Grupo Puntacana (FGPC), Fundacion Dominicana para los Estudios Marinos (FUNDEMAR) and TNC. As part of the co-management of SMASE, these institutions, among other local partners, organize coral outplant events in which local stakeholders (NGOs, diving operators, tourism sector, and government agencies) engage in multi-day coral restoration efforts both within and around the sanctuary and in nearby reefs. Known as “Coral Manias,” these activities have facilitated the successful outplanting of thousands of A. cervicornis fragments onto degraded reefs throughout tens of square kilometers of coastal areas following repeated community events. Bávaro is a coastal community in northern Punta Cana, just 3 kilometers west of the SMASE boundary (Figure 1), that was identified as a high priority restoration site due to its degrading reefs and need for coastal protection. In the past, outplant sites for these events have been selected based on local expertise, without specific and/or standardized criteria. Our objective is to present a case study that uses GAO-derived map products within a suitability model framework to provide a quantitative and replicable method for selecting coral restoration sites with the goal of increasing outplant survival over time.
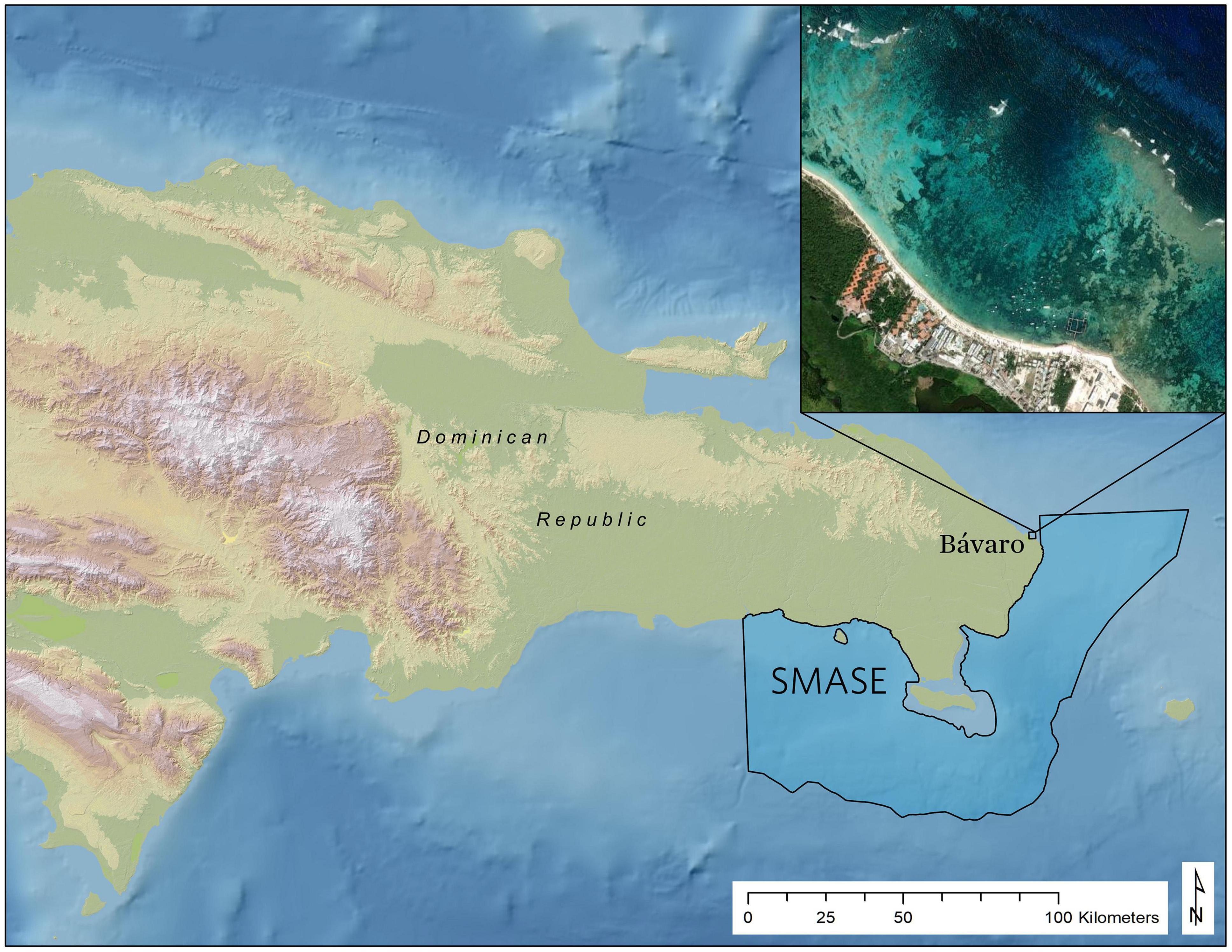
Figure 1. Reference map showing the location of the outplant locations in Bávaro near the South East Marine Sanctuary (SMASE: Santuario Marino Arrecifes del Sureste), the second largest protected area in the Dominican Republic where the Global Airborne Observatory (GAO) acquired imaging spectrometer data in May 2018.
Materials and Methods
Remote Sensing Data Collection
From May 1, 2018 to May 25, 2018, the GAO operated in the Dominican Republic, collecting aerial imaging spectrometer data using a high-fidelity visible-to-shortwave infrared (VSWIR) imaging spectrometer and a dual-beam light detection and ranging (LiDAR) scanner. Full descriptions of the aircraft, instrumentation, and data processing are provided by Asner et al. (2012, 2020a). A position and orientation system (POS) enabled the computation of aircraft position to within 5 cm (RMSE: root mean squared error) and aircraft orientation for the duration of all flights. GPS timing data were collected during flight by all three instruments, and this information, along with known position and instrument boresight offsets, allowed precise back-computation of position and orientation for the receiver of each instrument at all times during flight.
Collection location could change during each flight day and was actively managed based on need, cloud cover, and windspeeds to provide both the most efficient use of time and the best conditions for spectroscopic seafloor measurements. To maximize data consistency, airborne operations were performed from 0830 to 1100 local time. During flight, instrument settings were set for the planned nominal flight altitude of 1 km above the sea surface. Flight lines were spaced to achieve 50% overlap in VSWIR spectrometer coverage. Aircraft groundspeed was maintained between 130 and 140 kt. LiDAR pulse frequency was set to 200 kHz (100 kHz per laser), and scan frequency was 34 Hz with a field of view of 38°, allowing 2° of buffer on each side of the spectrometer field of view of 34°, and achieving a nominal pulse density (over land) of more than 8 pulses m–2.
Field Data Collection
A field campaign to measure and characterize the project area was conducted during the same time as the GAO data collection. In situ data collected for calibration and verification included 122 GPS-referenced underwater video transects, 37,400 transducer bathymetric points, and 2,477 benthic digital photos collected at sub-meter accuracy. High resolution satellite imagery converted to MBTile format and loaded into the Locus Pro application on a tablet was used to identify and navigate to survey point locations representing various benthic habitat types. A SeaViewer Sea-Drop 6000 HD (Tampa, FL, United States) underwater video camera with a 30 m vertical cable was used to record video transects at 1–2 m above the seafloor. A GPS reference point was collected at the start and end of each transect to allow for georeferencing of video surveys. A total of 152 km of bathymetric field measurements were collected using a Lowrance Elite7Ti® system with a xSonic P319 (50/200kHz) transducer and 10Hz GPS receiver that measured continuous depth readings at 3 pts/sec. In areas inaccessible by boat, snorkeler-based transect surveys were swam using a GoPro Hero 6 camera capturing video footage of various benthic habitat types. In addition, very high resolution (3 cm pixel) orthophoto mosaics and digital surface models were acquired for selected candidate coral outplant locations using a DGI Phantom 4 Pro Unmanned Aerial Vehicle (UAV).
To calibrate the imaging spectrometer benthic classification (e.g., percent live coral and algal cover), underwater photos were collected with coincident highly accurate positional data acquired with a survey grade Trimble Global Navigation Satellite System (GNSS) receiver. The GNSS receiver antenna was mounted to the top of a foam bodyboard and a Sony a6300 camera was positioned in a waterproof housing directly below this antenna on the underside of the board to collect vertical photos of the seafloor (Figure 2). The board was towed using a nylon tether rope. Cabling from the camera (both an HDMI and USB) and the GNSS was long enough to be included next to the tether. An operator on the boat was then able to view the camera’s viewfinder in real time using the video feed from the HDMI cable. The USB cable allowed for triggering of the camera shutter on an ad-hoc basis. At the same time, the operator could also trigger the GNSS system to record a point as that receiver was also onboard (in practice this was often done by a second operator). In addition, the GNSS was set to continuously log at 5 Hz (5 times per second).
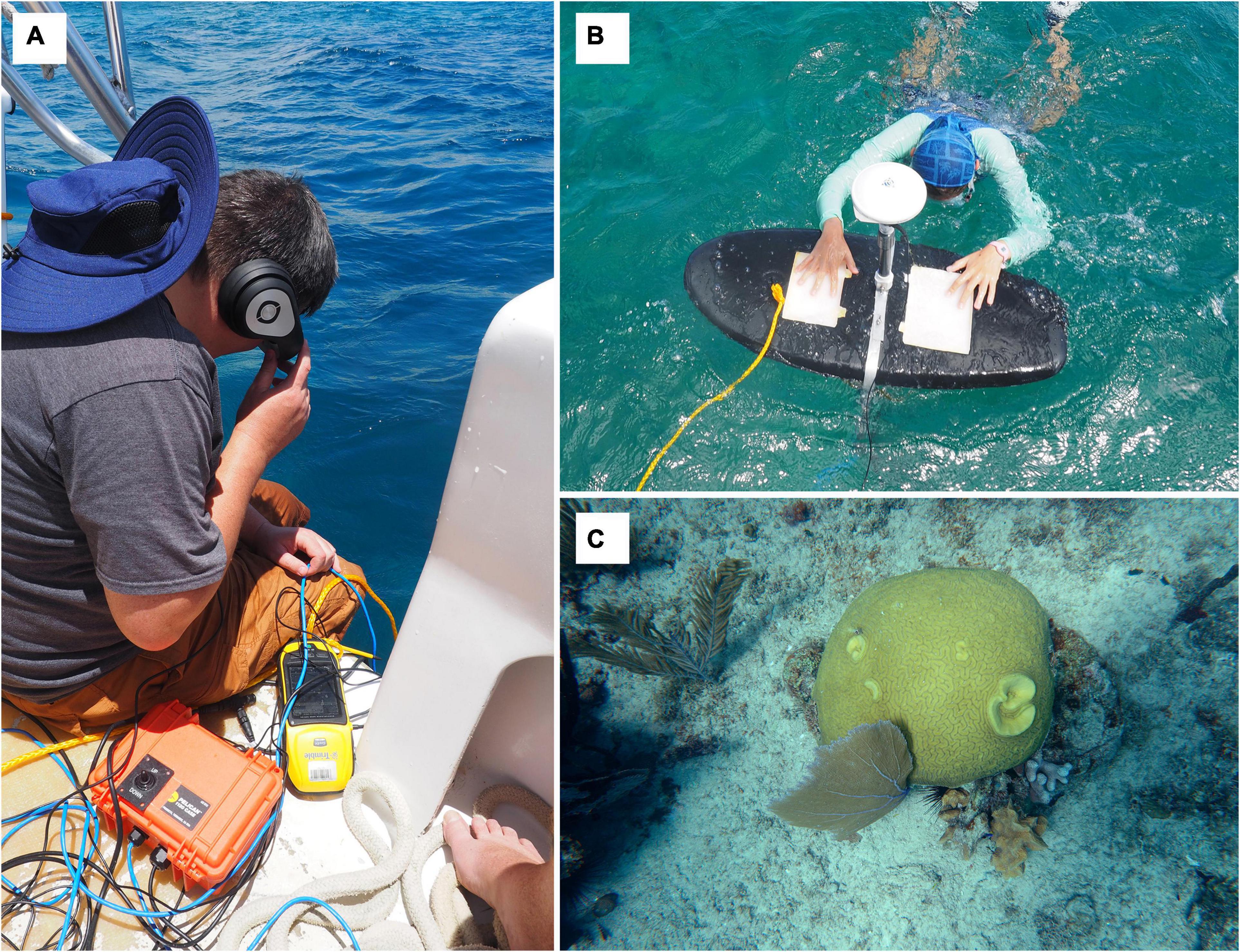
Figure 2. Field data collection used to calibrate the imaging spectrometer benthic classification: (A) an operator on the boat is able to view the video feed from the camera in real-time via HDMI cable; (B) the GNSS receiver antenna mounted to the top of a foam bodyboard and a Sony a6300 camera positioned in a waterproof housing directly below this antenna on the underside of the board to collect vertical photos of the seafloor; (C) an example of one of the 2,477 underwater photos collected with <1 m positional accuracy that was later classified by proportional cover in the following benthic classes: sand, live coral, algae, seagrass, and rubble.
Cellular or other internet connection on the boat was not reliable, thus the data were not corrected in real time. Therefore, it was necessary to post-process the GNSS locations and then match the images to those positions. Base station data from the Continuously Operating Reference Stations (CORS) sites, established by the National Geodetic Survey (NGS), and were used to correct the data using Post Processing Kinematic (PPK) techniques1. The Trimble Pathfinder Office software was used to post-process the corrections. Once the field-collected GNSS rover files are supplied to the software, the necessary base station files that match the specific time period in which the rover files were collected are downloaded, and the correction is performed. Trimble Pathfinder Office reported a final horizontal accuracy under 1 m for >98% of the locations with the majority being under 100 mm. Photos were assigned a location based on their timestamp to the closest corrected GNSS position in time. The few points with time differences over 1 s or with reported accuracies over 1 m were discarded. A total of 2,477 photos with a precise GNSS location (<1 m) were classified into their respective percent classes (sand, live coral, algae, seagrass, and rubble), and a point feature class with the assigned classification was created and used as ground reference for extracting these classes from the imaging spectroscopy data.
Data Processing
Airborne data from all three instruments were processed for orthorectification as well as radiometric and atmospheric correction. The raw LiDAR point cloud data were converted to a 50 cm resolution digital surface model (DSM) by interpolating between the first returns from each pulse using the GDAL writer functionality of the Point Data Abstraction Library (PDAL Contributors 2018). Regions missing from this surface map, due to specular reflection of the LiDAR beams off of the water surface, were filled in using inverse distance weighted interpolation (IDW) with power 2.
With the LiDAR DSM and known position, orientation, and camera lens model for each instrument, the 3-dimensional position of each spectrometer and digital camera pixel was ray-traced to the sea surface level. The raw spectrometer data collected onboard the GAO were first converted to radiance using laboratory calibration data collected before the campaign. The radiance data contain 427 spectral channels covering the wavelengths between 350 and 2500 nm in 5 nm increments. Using the LIDAR-derived observation angles and elevation as inputs, atmospheric correction was performed with a modified version of the ATREM model (Thompson et al., 2017).
The orthorectification for each flight line was adjusted for water refraction and depth. A neural network deep learning model was used to compute depth for each flight line (Asner et al., 2020a). Then for each spectrometer pixel, the at-surface view zenith angle, φa, was modified for refraction at the air-water interface to get below-surface zenith angle, φb, using Snell’s law and standard refractive indices of 1.33 and 1.00029 for water and air, respectively. Together, this resulted in the conversion equation: φb = sin−1(0.752098sinφa). From the original sea surface location, this angle was traced to the modeled ocean floor depth to get a new 3-dimensional position for each spectrometer pixel representing the pixel location on the seafloor.
With the location of each spectrometer pixel known, individual flight lines were mosaicked together using a strategy of minimum glint, where glint is defined as the average reflectance value for the five spectral bands covering the wavelengths 890–910 nm for each pixel. For each mosaic map pixel location, data from the flight line with the lowest glint at that location was kept.
Bottom Surface Reflectance
Water scatters higher energy wavelengths of light and absorbs lower energy wavelengths, making the shape and brightness of the spectrum observed at the surface of the water strongly dependent upon the depth of the water. To account for this in the spectrometer data, the bottom reflectance spectrum was estimated using an empirical approach with two assumptions: (1) sufficiently constant reflectance spectrum of sand patches within the region of interest, and (2) minimal change in inherent optical properties of water within the region of interest. The algorithm to back-compute bottom reflectance consisted of the following steps:
1. Identify individual pure sand patches within the region of interest, ensuring to select patches from the full range of depth in the region of interest.
2. Obtain a bottom reflectance spectrum that will represent pure sand, bsand*.
3. Collect surface reflectance spectra for all pixels within the identified sand patches, r*.
4. For each wavelength in the collected reflectance data, fit a loess model of power 1 (Cleveland and Devlin, 1988) to the brightness of that wavelength against depth and store this model.
5. To compute bottom reflectance for each pixel, get the predicted surface reflectance for sand, , at the given depth using the models fit above. Then bottom reflectance, , is computed as:
The Classification Model
Training data for the deep learning model were built from a combination of 2,477 GPS subsurface photos as well as 633 hand-selected pure class locations identified using the GAO 3-band color orthorectified surface reflectance imagery. Each of the subsurface photos were evaluated to estimate proportional cover in the following benthic classes: sand, live coral, algae, seagrass, and rubble. The hand-selected points were sand, seagrass, and “other” which was used as a catch-all class to help the model correctly remove pixels associated white water, unfiltered sun glint, dark shadows, and surface features such as floating sargassum. For each training sample (pixel), the 57-band modeled bottom surface reflectance was retained along with the modeled depth at that location.
The TensorFlow package in Python (Abadi et al., 2016) was used to train a feed-forward neural network (NN) model to predict the proportional class membership of each pixel using the 57-band estimated bottom reflectance spectrum as well as the matching GAO depth as input. Eighty percent of the samples were selected at random for use in model training, 10% for checking optimization stopping criteria, and 10% for validation. The model architecture included a 58-node input layer, along with four dense hidden layers of 500 nodes each. All dense hidden layers used a RELU activation function. Finally, a six-node output layer was used, one each for proportion of sand, live coral cover, algal cover, seagrass cover, rubble cover, and “other,” using a linear activation function. Here, algal cover is defined as a combination of strong light-absorbing turf algae and weaker light-absorbing macroalgal cover. Mean square error (MSE) was used as the loss function. The ADAM optimization algorithm (Kingma and Ba, 2014) was used to fit the network coefficients to the training data, with an automatic stop determined as no improvement in the validation set loss value in 30 epochs. The model required 103 epochs to reach this optimization criterion, with the overall unweighted MSE across all classes reaching 0.0275. The accuracy of these data and methods have been previously demonstrated in Asner et al. (2020b) where airborne estimates of live coral cover were found to be highly correlated with field-based estimates of live coral cover (R2 = 0.94).
Maps for Outplanting Strategy
With the trained classification model, maps of modeled proportion of sand, live coral, algae, and seagrass cover were produced for each 1 × 1 m pixel in the GAO coverage of the outplanting region with an estimated depth of <16 m. In addition to the proportional cover maps, two additional maps were prepared for assisting outplanting strategy. First, a map of benthic depth was built using the GAO depth model defined in Asner et al. (2020a). Second, habitat complexity was modeled from the benthic depth map using an algorithm for surface complexity defined in Jenness (2004), with a window size of 9 pixels × 9 pixels (9.0 m × 9.0 m). Because the distribution of raw complexity values is extremely skewed to the right, an empirical probability integral transformation was used to rescale the values to follow a uniform distribution and range from 0 to 1. For this procedure, the transformed complexity values are computed as the rank of the original complexity value divided by the number of pixels. Spatial boundaries for geomorphic zones (e.g., fore reef, reef crest, back reef, and lagoon) were manually digitized based on image interpretation of high resolution (1 m) satellite image base maps available in Google Earth, Microsoft Bing, and Esri and were used as selection criteria to supplement the GAO data layers.
Outplanting Criteria
Potential sites for outplanting in Bávaro were chosen based on two types of criteria: (1) logistical and (2) ecological. Logistical criteria aimed to facilitate outplanting by reducing time and costs in the field. Logistical criteria included distance from the nursery site (<1000 m) and wave exposure. Back reef habitats were targeted to avoid strong currents and surge, making the outplanting process safer for volunteers and potentially lowering the probability of coral fragments detaching from the substrate. Ecological criteria aimed to enhance the probability of survivorship of outplants were selected. Criteria ranges were based on what is known about the ecology and biology of A. cervicornis and the local and regional restoration efforts that have been conducted for the species in the past (Hernández-Delgado et al., 2014; Mercado-Molina et al., 2015; Ladd et al., 2018). Ideal ecological criteria were identified: (1) depth: 3–7 m; (2) maximize percentage of live coral: >2% (highest in this area was 10%); (3) minimize percentage of algal cover: <80%; and (4) maximize habitat complexity: >0.3 (Figure 3). These ranges were based on local conditions in the Bávaro area (i.e., <30% algal cover or higher live coral cover would be more ideal conditions but was not possible within the area of interest). The GAO-derived data layers were filtered to these ranges and subsequently intersected to identify areas that met all criteria. We also included information on the coastal protection and vulnerability reduction benefits of reef locations, as modeled using the InVEST (Integrated Valuation of Ecosystem Services and Tradeoffs) software, which uses geophysical and natural habitat characteristics of coastal landscapes to compare their exposure to erosion and flooding in severe weather (Sharp et al., 2018; Harris et al., 2018). When all criteria were considered, approximately 50,900 m2 of potential suitable area was selected for outplanting activities.
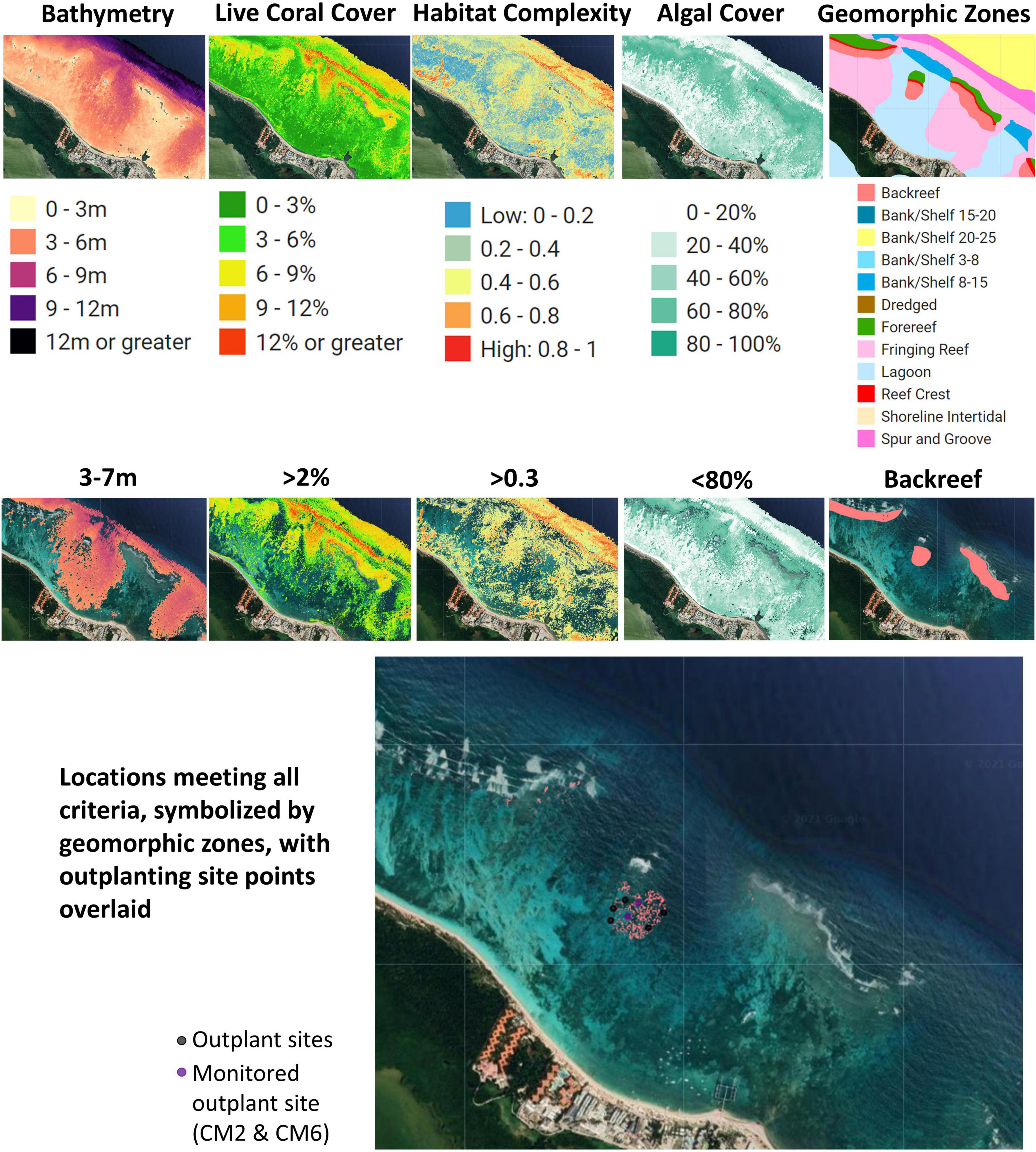
Figure 3. Data layers and criteria used to select suitable outplant sites in Bávaro, Dominican Republic. Sites were identified using the Coral Outplanting Siting Guide Google Earth Engine (GEE) app and used as the focal restoration area in the community-led outplanting activity. These criteria were based on key ecological factors of Acropora cervicornis that would promote the likelihood of outplant survivorship.
Community Outplant Event
Overall, the Bávaro outplanting area showed clear signs of old reef degradation, which is common across backreefs habitats in Bávaro. However, visual inspection of the pre-selected area from satellite imagery and field visits confirmed these sites were suitable for outplanting corals and met the selection criteria with high accuracy. More specifically, the area was composed of patchy hard bottom habitats with crevices, desired levels of rugosity, and sufficient percentages of live coral and algal cover (Figure 4). Selected sites were comprised of scattered pinnacles (5–10m depth) distributed across sandy bottoms, which is common for back reef habitats. Only a few coral species were observed, largely small-size brooders such as Agariciid (Agaricia tenuifolia and A. agaricites) and Poritids (Porites porites and P. astreoides), whereas large reef builders such as Orbicella annularis, O. faveolata, Colpophyllia natans and Pesudodiploria strigosa were less common. Algae clearly dominated the reefs, with species in the genera Dyctiota spp. and Lobophora spp. being the most prevalent.
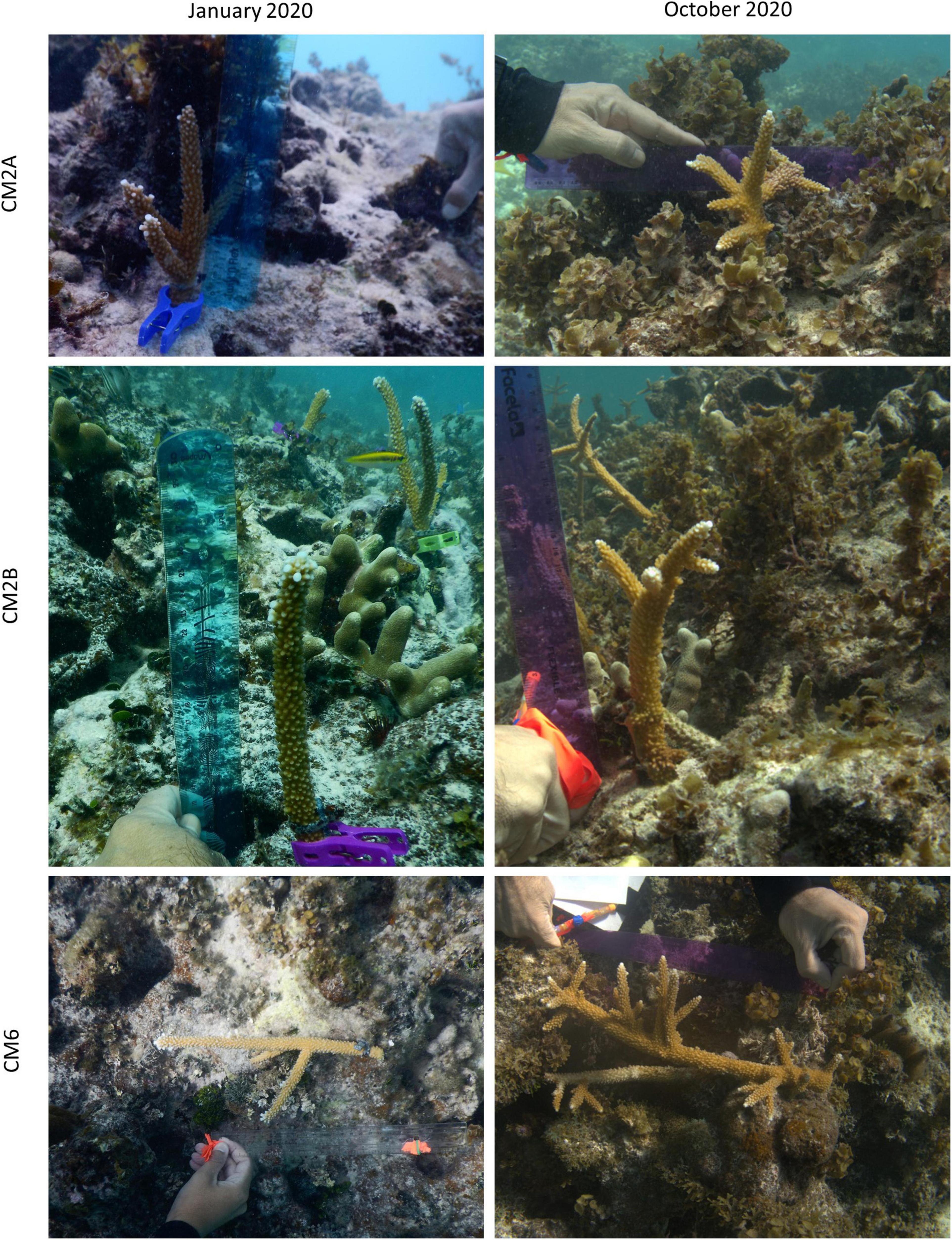
Figure 4. Coral monitoring photos comparing growth of outplants between January 2020 and October 2020.
Snorkelers field-checked the identified area of interest to verify the site met all relevant criteria and was suitable for coral outplanting. A total of 1,722 fragments of A. cervicornis ranging from 100 to 160 mm (mean 123 mm 32 SD) were outplanted at this location during a community-based citizen science outplant event, “Coral Mania,” hosted by Consorcio Dominicana de Restauracion Costera, Grupo Punta Cana Fundación, FUNDEMAR, and Counterpart International between November 17 and 21, 2019. More than 30 volunteer divers in three boats worked to outplant corals at nine plots: “Coral Mania 1” (CM1), CM2, CM3, CM4, CM5, CM6, CM7, CM8, and CM9 (Table 1). Prior to outplanting, divers enhanced the substrate by removing algae from the location where each coral fragment was to be attached. This process was minimal since the model had previously selected these sites based on lower levels of macroalgal cover. During the outplanting phase, divers outplanted in areas with low frequency of sessile organisms which compete with corals for substrate space (e.g., drilling and/or encrusting sponge, hydrocorals, etc.) and by the large numbers of crevices and overhangs that provide rugosity to the habitat.
Outplant Monitoring
Indicators of coral outplanting success include survivorship, growth rates, and community structure. Coral fragments and the associated benthic community structure were monitored at four randomly selected outplant sites (CM1, CM2, CM3, and CM4) during January 2020 before the COVID-19 pandemic and again in October 2020 when diving restrictions were lifted. During these surveys, 31–50 and 16–41 individual fragments were photographed in January and October 2020, respectively, using a 300 mm ruler as reference to determine the size of each fragment and the percent living tissue. Coral fragment size was estimated using measurements of maximum length and width of the individual coral colony using ImageJ software. Growth rates for the population of outplanted corals were calculated as the difference between the estimated mean of coral colonies in January and October 2020 and the estimated mean of the initial coral size at the time of outplanting (November 2019). Coral colony survivorship was measured as the change in the number of living coral colonies within the plots, while percent live coral tissue per colony was visually estimated to the nearest 5% using photos in ImageJ. While coral fragments were only monitored three times in 1 year, both survival and growth rates were measured with high precision from large sample sizes.
In addition, 7 to 9 photos of the benthic substrate were taken approximately 2m above the substrate in three out of four randomly selected sites to determine the percentage of live cover of selected major taxonomic groups [i.e., scleractinian corals, hydrocorals, octocorals, sponge, turf algae, macroalgae, and crustose coralline algae (CCA)] and abiotic substrates (i.e., bare substrate, dead coral, sand, and rubble). We analyzed 25 random points within each photo using the software photoQuad (Trygonis and Sini, 2012) following procedures outlined in the Global Coral Reef Monitoring Network protocol (Miyazawa et al., 2020). Benthic community changes were monitored in January 2020 but could not be assessed in October 2020 due to challenges caused by the COVID-19 pandemic.
Results
Survivorship and Growth
In January 2020, 2 months after the outplant event, survivorship among the four subplots over a random sample of 65 coral fragments was 91.6% ± 4.7 SD. By October 2020, almost a year after the outplant event, survivorship remained above 76% with an average of 83.5 ± 4.7 SD (Figure 5). During the first monitoring event in January 2020, the majority of outplants had 100% living tissue; however, by October 2020, the coral fragments had lost 26–84% live tissue, likely due to predation (Figures 6, 7). Moreover, results showed that the size of the coral fragments increased 0.5 to 3-fold with an average initial size 123 mm ± 32 SD and an average size after 3 months of 256 mm ± 15.3 SD. During the October 2020 revisit, continued growth was observed, but at a slower pace, with the exception of site CM1 where coral fragments doubled in size. Size frequency distributions, estimated in January 2020, indicate that 70–90% of the coral fragments had grown to reach sizes of 250–500 mm, further indicating rapid growth rates for the population of outplanted corals (20–40 mm per month). In October 2020, the size frequency distribution only changed at site CM1, suggesting that corals at this site continued to grow at similar rates that were observed after the first 3 months (Figure 8).
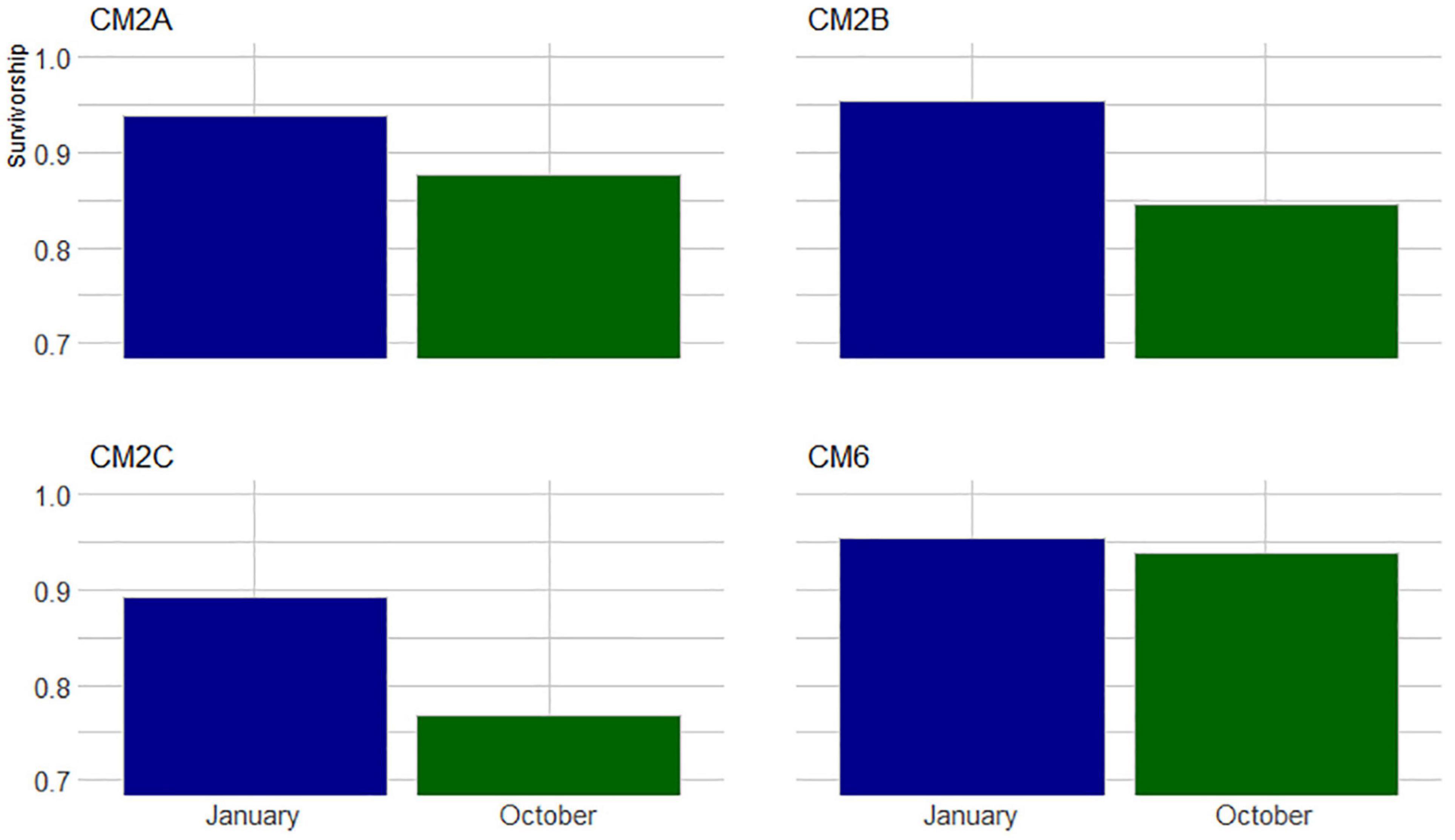
Figure 5. Proportion of colonies that survived between November 2019 to January 2020 and October 2020 across four monitored sites at Bávaro.
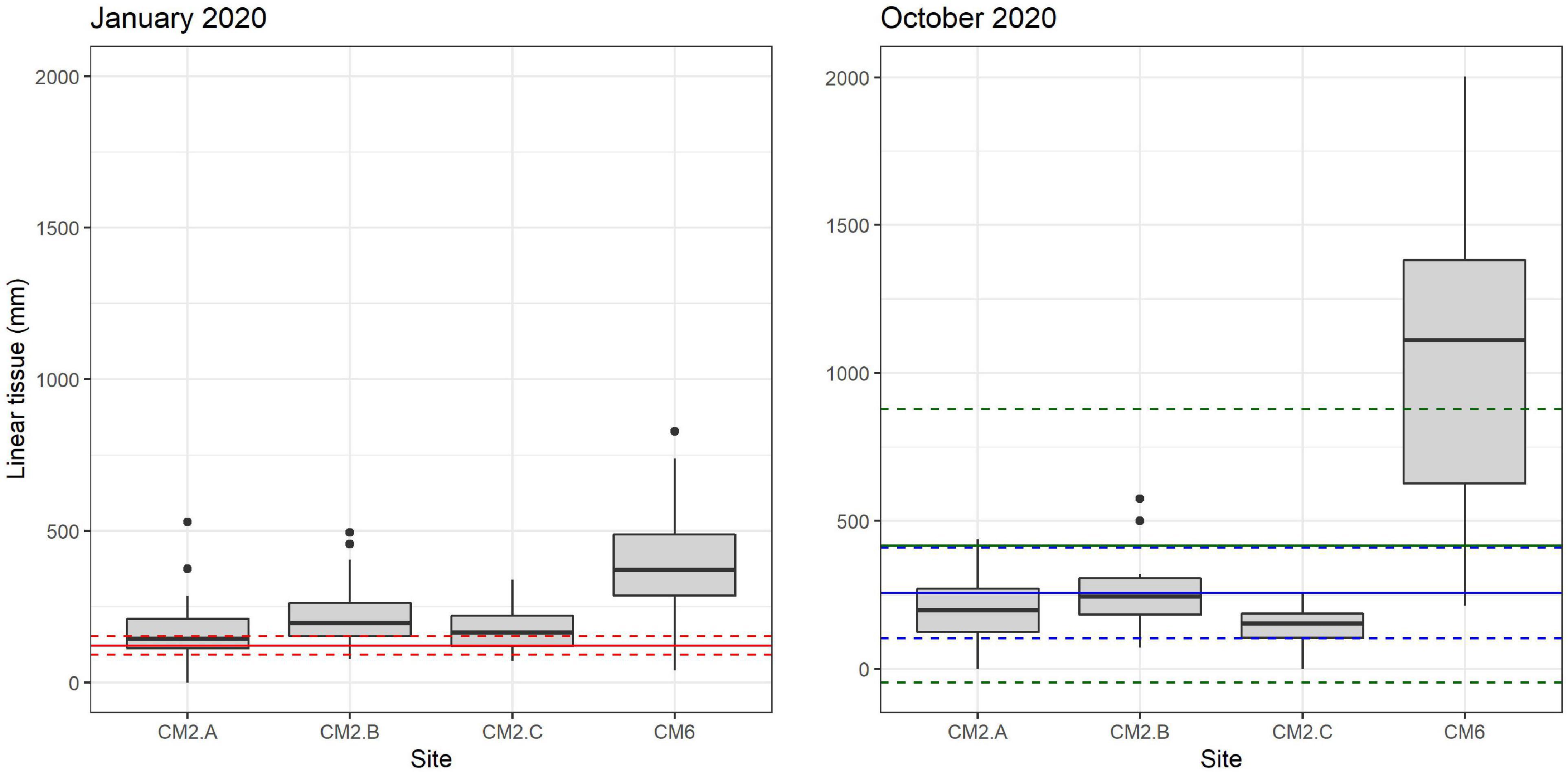
Figure 6. Linear growth box plot for colonies that survived between January 2020 and October 2020 across monitored sites. Solid and dashed red lines in panel January 2020 indicate the average and confidence interval (CI 95%) of initial sizes at the moment of outplanting (November 2019), respectively. Solid and blue dash lines in panel October 2020 indicate average and confidence interval (CI 95%) of growth recorded in January 2020. Solid and dashed green lines indicate mean and confidence interval (CI 95) recorded in October 2020, respectively. Black dots represent outliers.
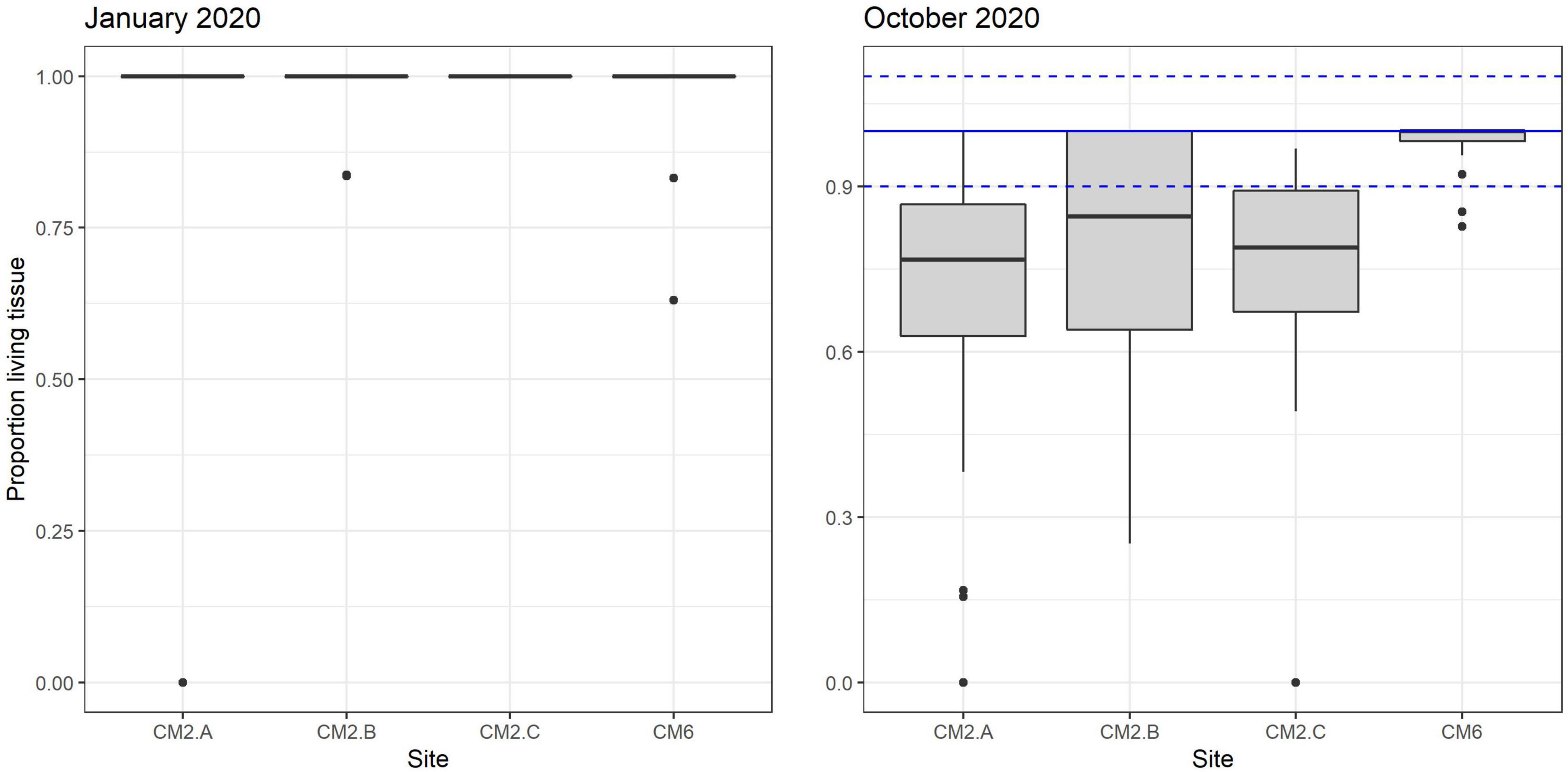
Figure 7. Proportion of living tissue box plot remaining on corals outplanted across sites between January and October 2020.
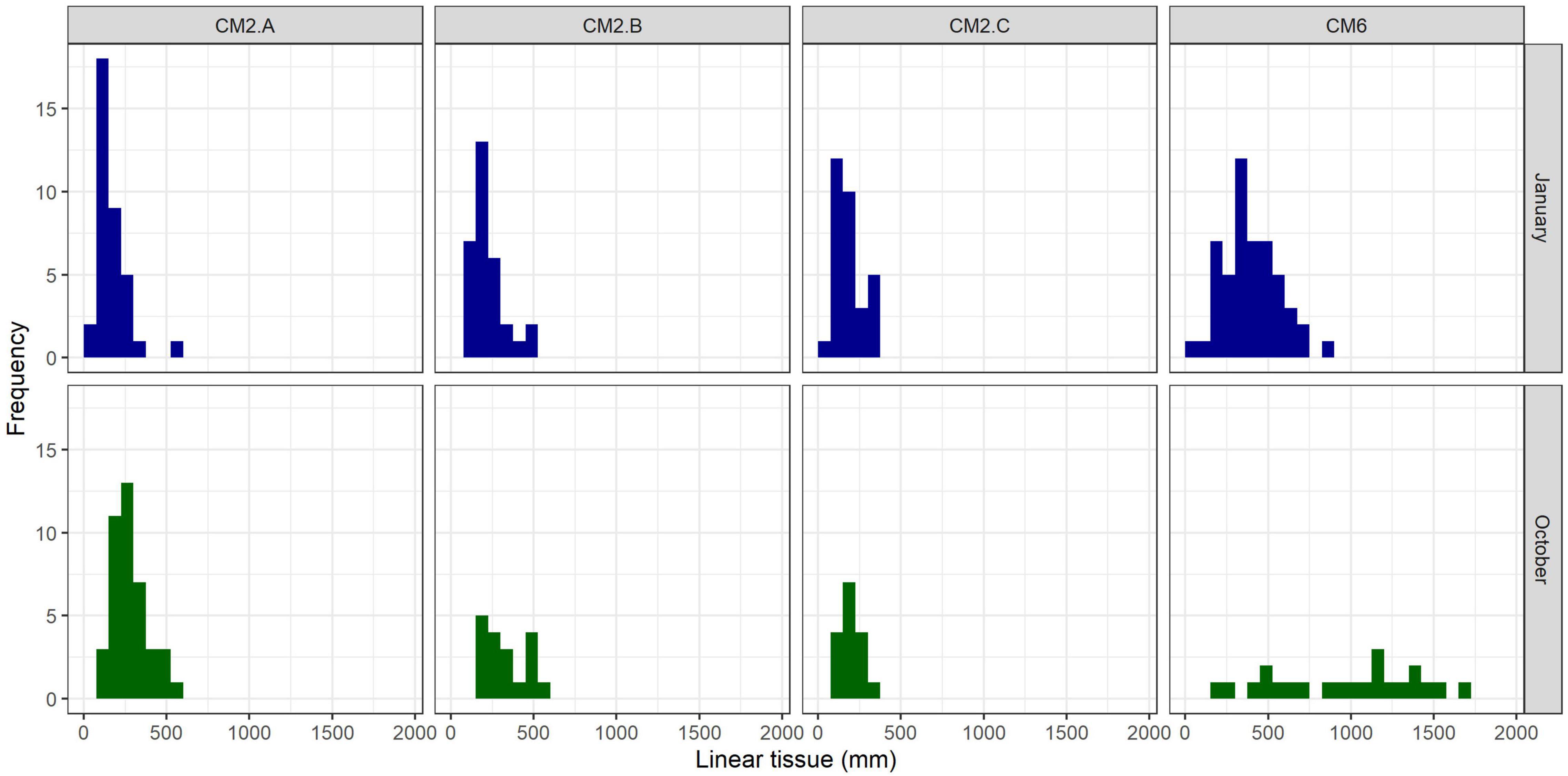
Figure 8. Size frequency distribution of colonies per monitoring site 3 months (January 2020) and 11 months (October 2020) after the outplant event (November 2019).
Benthic Community
A total of 3 months after outplanting the corals, the benthic community structure remained consistent with the findings observed in the site selection criteria model. Coral cover ranged from 3.5 to 4% with maximum values of 8–10% and an average value of 3.6% ± 0.4 SD. Turf algae was the predominant substrate, ranging from 34 to 46 % with an average of 40.9 % ± 5.9 SD, followed by the macroalgae, which varied from 27 to 33% with an average of 29.6 % ± 3.2 SD. CCA seldom exceeded 10%, varying from 4.7 to 6.4% with a mean of 5.4% ± 0.7 SD. Sponges and other substrate competitors were rare across the monitored sites, seldom exceeding 3% of cover (Table 2).
Discussion
During the past few decades, restoration efforts have increased significantly as coral reefs continue to decline (Boström-Einarsson et al., 2020). Coral restoration is a complex task which must consider multiple interactive factors (Hernández-Delgado et al., 2014) with the acknowledgment there are a variety of ecological and biophysical processes that cannot be detected using remotely sensed data. The reasons for coral decline vary from site to site and the costs and logistical constraints make it difficult to conduct restoration at large and ecologically relevant spatial scales. However, identifying strategies to increase the scale of restoration efforts is a priority for restoration practitioners and scientists (Boström-Einarsson et al., 2020). The development of science-based solutions for analyzing and optimizing the selection of suitable sites for outplanting corals across large reef sections is a necessary step for achieving this goal.
We address several ecological and logistical criteria for outplant site selection and demonstrate a proof-of-concept that remote sensing and suitability modeling can be used to increase the efficiency of coral outplant site selection. The approach we present is the first time this technology has been used to guide restoration practitioners in restoration site selection for a coral outplanting event implemented by community practitioners and citizen scientists. Our methods permit the screening of large areas (km2) for identifying a variety of suitable outplanting habitat locations across broad scales that otherwise would not be possible using traditional finer-scale site selection methods such as SCUBA–based surveys. The results of our coral survivorship surveys exceed 76% after 11 months, suggesting the technology and methodology we present provides an effective approach in identifying suitable sites where corals are more likely to persist. We suggest additional research is needed in a variety of coral reef conditions and locations to further test and refine this approach for broader use and application in the field of coral reef restoration.
Our methods are replicable and accessible via a web app where restoration practitioners can access the criteria modeling framework and datasets for pilot sites in the Dominican Republic and U.S. Virgin Islands. The Coral Outplanting Siting Guide, a Google Earth Engine (GEE) app, provides coral managers and restoration practitioners with the ability to repeat the suitability modeling process to dynamically select outplant locations based on site-specific or coral species-specific filters2 (Figure 9). Our approach provides seven criteria that can be used for filtering the map to select a specific outplant site: bathymetry (m), live coral cover (%), habitat complexity (rugosity), algal cover (%), geomorphic zone, seagrass cover (%), and sand cover (%). The user can input custom ranges (e.g., 3–7 m depth, 10–80% live coral cover) and results display only areas that meet those criteria. Alternatively, criteria values at existing successful outplant sites can be identified using the latitude and longitude of the site. Measurement tools can be used to select outplant sites based on logistical criteria including distance to a coral nursery lab or total area required for an outplant site.
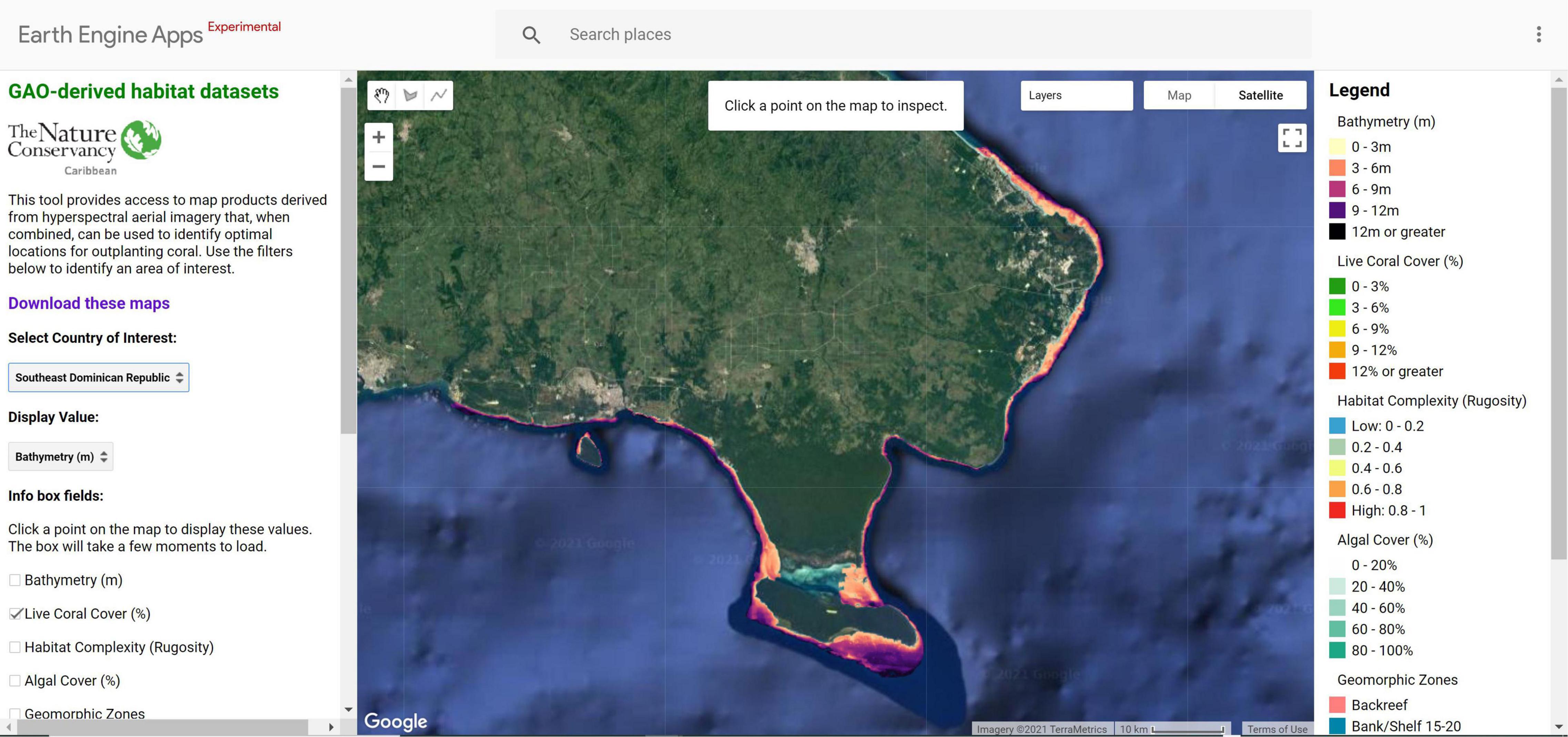
Figure 9. The Coral Outplanting Siting Guide, a Google Earth Engine (GEE) app, an open-source web browser-based tool that provides coral managers the ability to dynamically model and select outplanting locations based on site-specific or coral species-specific criteria. The tool allows users to interact with large spatial datasets without requiring software installation, technical knowledge, or data storage capacity.
While site selection is key for success and is extremely valuable for scaling up these efforts (Foo and Asner, 2019), a more complete understanding of coral species biology, and ecology is fundamental for successful coral restoration. Coral outplant survivorship not only depends on habitat suitability and the features of the physical environment (Shaver and Silliman, 2017, Ladd et al., 2018), but is also determined by a series of biological process such as predation (Glynn, 1962; Baums et al., 2003; Miller et al., 2014), herbivory (Burkepile and Hay, 2010; Shaver and Silliman., 2017; Ladd et al., 2018; Lefcheck et al., 2019; Cano et al., 2021), disease (Hernández-Delgado et al., 2014), and genetic identity of coral outplants (Drury et al., 2017). These types of variables can vary greatly across space and time and are undetectable in remotely sensed images. Thus, we recommend outplant site selection should be evaluated in combination with remotely sensed data and field-based surveys where ecological processes and site conditions can be fully assessed.
Our results indicate that even in suitable sites, predation marks likely produced by the fireworm Hermodice caraculata and the corallivorous snail Coralliophila gala were present in 10–15% of A. cervicornis fragments surveyed. We also recorded loss of 14–26% of living tissues from January to October 2020, further suggesting this polychaetae hampers the survivorship of fragments. Similar results have been recorded in many restoration programs across the Caribbean (Hernández-Delgado et al., 2014). In addition to predation, macroalgae cover could be a significant factor influencing the success of coral outplants. In most cases, coral reef macroalgal cover near Bávaro has been reported > 40%. It was observed within our outplant sites, brown algae often smothered coral fragments, particularly those shorter than 120 mm in length. Thus, in areas where macroalgal cover is naturally high (60–70%), outplanting larger fragments (e.g., 150–250 mm) may be needed to increase survival success.
One of the biggest challenges to restoration success is the inadequate attention or control of threats causing reef decline and the need for restoration in the first place (Hein et al., 2020). Though we did consult with local experts on the influence of threats within our restoration site (i.e., temperature and history of coral bleaching, presence of predators/grazers, disease, turbidity/water quality, tourism activity, and land-based pollution), spatial data on these threats were not available and could not be incorporated into the modeling process. Thus, future research could more systematically take local anthropogenic and climate change-related threats into account in the site selection process relying on in-water surveys, expert guidance, and/or further modeling (Forsman et al., 2015). Given the influence of local and climate threats on corals and the success of restoration efforts, more research into how threats can be incorporated into restoration site selection processes is warranted.
As suggested by Foo and Asner (2019), remote sensing, in particular hyperspectral sensing techniques, are currently underutilized and represent a key system in moving toward a more methodical way to identify reef restoration sites that have a heightened chance of surviving projected change. Remote sensing can provide information on abiotic conditions (e.g., water quality, sedimentation, and temperature) across much broader spatial and temporal scales when compared to diver surveys. When coupled with appropriate field surveys and spectral libraries, these technologies have the potential to detect between healthy and diseased corals, identify colonies at the species level, and are ideal approaches for monitoring restoration success. Additional research is needed to identify the ideal size, extent and position of an outplant area that will most likely facilitate the recovery of adjacent reefs and how outplanting influences reef connectivity and larval seeding (Foo and Asner, 2019). As remote sensing technologies are advancing rapidly, costs are becoming more affordable as new sensors and platforms evolve. When considering costs, remote sensing for restoration is more cost-effective if implemented through strategic partnerships between multiple agencies and organizations that are interested in collecting imagery over large areas which can greatly reduce the final cost of data collection and processing per hectare.
Conclusion
Advances in aerial mapping technologies have allowed mapping of broad reef ecosystems and the collection of multiple proxies of ecosystem health (e.g., coral and algal cover, rugosity, and structural complexity). Our research demonstrates for the first time the novel application of these tools to guide restoration efforts and locate sites that are suitable for outplanted corals. However, these technologies alone fail to quantify all of the important ecological, physical, and social processes and factors that are important for coral survivorship which require in situ assessments. A combination of broad-scale and fine-scale restoration technologies, conservation, and monitoring techniques represent the best approach. While we provide a proof-of-concept that broad-scale remote sensing is useful for selecting suitable sites for restoration, local restoration expertise is critical for identifying local threats, designing and implementing feasible conservation plans, and monitoring restoration success.
Data Availability Statement
The raw data supporting the conclusions of this article will be made available by the authors, without undue reservation.
Author Contributions
GA collected the data. NV processed that data into data layers. SS and GR collected field data to validate the remote sensing products. SS, FP, AC, XE-F, and VM interpreted the data and worked with partners to select outplant sites. AC validated the data in the field, helped to define criteria for outplant sites, conducted monitoring and evaluation of the outplants, and analyzed monitoring data. VM developed the app. AC, VM, and SS generated the figures and tables. All authors designed the study, and drafted and edited the manuscript.
Funding
This research was funded by Daniel C. Chung, The Kowalski Family Foundation, The Tiffany & Co. Foundation (Grant No. 12171), The Paul G. Allen Family Foundation (Grant No. 12809), The Community Foundation of the Virgin Islands, The J.A. Woollam Foundation, and The John D. and Catherine T. MacArthur Foundation (Grant No. 96061-0). The Global Airborne Observatory is made possible by support provided by private foundations, visionary individuals, and Arizona State University.
Conflict of Interest
The authors declare that the research was conducted in the absence of any commercial or financial relationships that could be construed as a potential conflict of interest.
Publisher’s Note
All claims expressed in this article are solely those of the authors and do not necessarily represent those of their affiliated organizations, or those of the publisher, the editors and the reviewers. Any product that may be evaluated in this article, or claim that may be made by its manufacturer, is not guaranteed or endorsed by the publisher.
Acknowledgments
We would like to acknowledge the support of Carlos Garcia, Francisco Núñez, Giselle Hall, Jessica Wiseman, Lisa Price, Luis Solorzano, Marci Eggers, Montserrat Acosta-Morel, Robyn McGuinness, Robert Brumbaugh, and Zach Horton. We would also like to acknowledge local partners leading the Coral Mania including the Dominican Republic Coral Restoration Consortium (DR – CRC), Jake Kheel and Susanne Leib from Grupo Puntacana Foundation, Rita Sellares and Maria Villalpando from FUNDEMAR, Someira Zambrano from Red Arrecifal Dominicana, Emile Mariotti from Sea Pro Divers, and Victor Galvan.
Footnotes
References
Abadi, M., Agarwal, A., Barham, P., Brevdo, E., Chen, Z., Citro, C., et al. (2016). TensorFlow: large-scale machine learning on heterogeneous distributed systems. arXiv [Preprint] CoRR abs/1603.04467,
Alvarez-Filip, L., Dulvy, N. K., Gill, J. A., Côté, I. M., and Watkinson, A. R. (2009). Flattening of Caribbean coral reefs: region-wide declines in architectural complexity. Proc. R. Soc. Lond. B Biol. Sci. 276, 3019–3025. doi: 10.1098/rspb.2009.0339
Asner, G. P., Knapp, D. E., Boardman, J., Green, R. O., Kennedy-Bowdoin, T., Eastwood, M., et al. (2012). Carnegie airborne observatory-2 : increasing science data dimensionality via high-fidelity multi-sensor fusion. Remote Sens. Environ. 124, 454–465. doi: 10.1016/j.rse.2012.06.012
Asner, G. P., Knapp, D. E., Martin, R. E., Tupayachi, R., Anderson, C. B., Mascaro, J., et al. (2014). Targeted carbon conservation at national scales with high-resolution monitoring. Proc. Natl. Acad. Sci. U. S. A. 111, E5016–E5022.
Asner, G. P., Vaughn, N. R., Balzotti, C., Brodrick, P. G., and Heckler, J. (2020a). High-resolution reef bathymetry and coral habitat complexity from airborne imaging spectroscopy. Remote Sens. 12:310. doi: 10.3390/rs12020310
Asner, G. P., Vaughn, N. R., Heckler, J., Knapp, D. E., Balzotti, C., Shafron, E., et al. (2020b). Large-scale mapping of live corals to guide reef conservation. Proc. Natl. Acad. Sci. U. S. A. 117, 33711–33718. doi: 10.1073/pnas.2017628117
Baums, I. B., Miller, M. W., and Szmant, A. M. (2003). Ecology of a corallivorous gastropod, Coralliophila abbreviata, on two scleractinian hosts. II. feeding, respiration and growth. Mar. Biol. 142, 1093–1101. doi: 10.1007/s00227-003-1053-4
Bayraktarov, E., Banaszak, A. T., Montoya Maya, P., Kleypas, J., Arias-González, J. E., Blanco, M., et al. (2020). Coral reef restoration efforts in Latin American countries and territories. PloS One 15:e0228477. doi: 10.1371/journal.pone.0228477
Bayraktarov, E., Saunders, M. I., Abdullah, S., Mills, M., Beher, J., Possingham, H. P., et al. (2016). The cost and feasibility of marine coastal restoration. Ecol. Appl. 26, 1055–1074. doi: 10.1890/15-1077
Boström-Einarsson, L., Babcock, R. C., Bayraktarov, E., Ceccarelli, D., Cook, N., Ferse, S. C., et al. (2020). Coral restoration – a systematic review of current methods, successes, failures and future directions. PLoS One 15:e0226631. doi: 10.1371/journal.pone.0226631
Burke, L., Reytar, K., Spalding, M., and Perry, A. (2011). Reefs at Risk Revisited. Washington, DC: World Resources Institute.
Burkepile, D. E., and Hay, M. E. (2010). Impact of herbivore identity on algal succession and coral growth on a Caribbean reef. PloS One 5:e8963. doi: 10.1371/journal.pone.0008963
Cano, I., Sellares-Blasco, R. I., Lefcheck, J. S., Villalpando, M. F., and Croquer, A. (2021). Effects of herbivory by the urchin Diadema antillarum on early restoration success of the coral Acropora cervicornis in the central Caribbean. J. Exp. Mar. Biol. Ecol. 539:151541.
Chamberland, V. F., Petersen, D., Guest, J. R., Petersen, U., Brittsan, M., and Vermeij, M. J. (2017). New seeding approach reduces costs and time to outplant sexually propagated corals for reef restoration. Sci. Rep. 7, 1–12.
Cleveland, W. S., and Devlin, S. J. (1988). Locally weighted regression: an approach to regression analysis by local fitting. J. Am. Stat. Assoc. 83, 596–610. doi: 10.1080/01621459.1988.10478639
Cox, C., Valdivia, A., McField, M., Castillo, K., and Bruno, J. F. (2017). Establishment of marine protected areas alone does not restore coral reef communities in Belize. Mar. Ecol. Prog. Ser. 563, 65–79. doi: 10.3354/meps11984
De Groot, R. S., Blignaut, J., Van Der Ploeg, S., Aronson, J., Elmqvist, T., and Farley, J. (2013). Benefits of investing in ecosystem restoration. Conserv. Biol. 27, 1286–1293. doi: 10.1111/cobi.12158
dela Cruz, D. W., Rinkevich, B., Gomez, E. D., and Yap, H. T. (2015). Assessing an abridged nursery phase for slow growing corals used in coral restoration. Ecol. Eng. 84, 408–415. doi: 10.1016/j.ecoleng.2015.09.042
Drury, C., Manzello, D., and Lirman, D. (2017). Genotype and local environment dynamically influence growth, disturbance response and survivorship in the threatened coral, Acropora cervicornis. PLoS One 12:e0174000. doi: 10.1371/journal.pone.0174000
Foo, S. A., and Asner, G. P. (2019). Scaling up coral reef restoration using remote sensing technology. Front. Mar. Sci. 6:79. doi: 10.3389/fmars.2019.00079
Foo, S. A., and Asner, G. P. (2020). Sea surface temperature in coral reef restoration outcomes. Environ. Res. Lett. 15:074045. doi: 10.1088/1748-9326/ab7dfa
Forsman, Z. H., Page, C. A., Toonen, R. J., and Vaughan, D. (2015). Growing coral larger and faster: micro-colony-fusion as a strategy for accelerating coral cover. PeerJ 3:e1313. doi: 10.7717/peerj.1313
Glynn, P. W. (1962). Hermodice carunculata and Mithraculus sculpttts, two hermatypic coral predators. Proc. Assoc. Isl. Mar. Lab. Caribb. 4, 16–17.
Harris, D. L., Rovere, A., Casella, E., Power, H., Canavesio, R., Collin, A., et al. (2018). Coral reef structural complexity provides important coastal protection from waves under rising sea levels. Sci. Adv. 4:eaao4350. doi: 10.1126/sciadv.aao4350
Hein, M. Y., McLeod, I. M., Shaver, E. C., Vardi, T., Pioch, S., Boström-Einarsson, L., et al. (2020). Coral Reef Restoration as a Strategy to Improve Ecosystem Services – A Guide to Coral Restoration Methods. Nairobi: United Nations Environment Program.
Hernández-Delgado, E. A., Mercado-Molina, A. E., Alejandro-Camis, P. J., Candelas-Sánchez, F., Fonseca-Miranda, J. S., González-Ramos, C. M., et al. (2014). Community-based coral reef rehabilitation in a changing climate: lessons learned from hurricanes, extreme rainfall, and changing land use impacts. Open J. Ecol. 4:918. doi: 10.4236/oje.2014.414077
Hobbs, R. J., and Cramer, V. A. (2008). Restoration ecology: interventionist approaches for restoring and maintaining ecosystem function in the face of rapid environmental change. Annu. Rev. Environ. Resour. 33, 39–61. doi: 10.1146/annurev.environ.33.020107.113631
Hoegh-Guldberg, O., Pendleton, L., and Kaup, A. (2019). People and the changing nature of coral reefs. Reg. Stud. Mar. Sci. 30:100699. doi: 10.1016/j.rsma.2019.100699
Hughes, T. P., Graham, N. A., Jackson, J. B., Mumby, P. J., and Steneck, R. S. (2010). Rising to the challenge of sustaining coral reef resilience. Trends Ecol. Evol. 25, 633–642. doi: 10.1016/j.tree.2010.07.011
Hughes, T. P., Kerry, J. T., Álvarez-Noriega, M., Álvarez-Romero, J. G., Anderson, K. D., Baird, A. H., et al. (2017). Global warming and recurrent mass bleaching of corals. Nature 543, 373–377.
Jackson, J. B. C., Donovan, M. K., Cramer, K. L., and Lam, V. V. (2014). Status and Trends of Caribbean Coral Reefs: 1970-2012. Gland: Global Coral Reef Monitoring Network, IUCN.
Jenness, J. S. (2004). Calculating landscape surface area from digital elevation models. Wildl. Soc. Bull. 32, 829–839. doi: 10.2193/0091-7648(2004)032[0829:clsafd]2.0.co;2
Johnson, M. E., Lustic, C., Bartels, E., Baums, I. B., Gilliam, D. S., Larson, E. A., et al. (2011). Caribbean Acropora Restoration Guide: Best Practices for Propagation and Population Enhancement. New York, NY: The Nature Conservancy.
Kingma, D. P., and Ba, J. (2014). Adam: a method for stochastic optimization. arXiv [Preprint] Available online at: https://arxiv.org/pdf/1412.6980.pdf (accessed February 19, 2021).,
Ladd, M. C., Miller, M. W., Hunt, J. H., Sharp, W. C., and Burkepile, D. E. (2018). Harnessing ecological processes to facilitate coral restoration. Front. Ecol. Environ. 16:239–247. doi: 10.1002/fee.1792
Lefcheck, J. S., Innes-Gold, A. A., Brandl, S. J., Steneck, R. S., Torres, R. E., and Rasher, D. B. (2019). Tropical fish diversity enhances coral reef functioning across multiple scales. Sci. Adv. 5:eaav6420. doi: 10.1126/sciadv.aav6420
McClanahan, T. R., Donner, S. D., Maynard, J. A., MacNeil, M. A., Graham, N. A., Maina, J., et al. (2012). Prioritizing key resilience indicators to support coral reef management in a changing climate. PloS One 7:e42884. doi: 10.1371/journal.pone.0042884
Mercado-Molina, A. E., Ruiz-Diaz, C. P., and Sabat, A. M. (2015). Demographics and dynamics of two restored populations of the threatened reef-building coral Acropora cervicornis. J. Nat. Conserv. 24, 17–23. doi: 10.1016/j.jnc.2015.01.001
Miller, M. W., Marmet, C., Cameron, C. M., and Williams, D. E. (2014). Prevalence, consequences, and mitigation of fireworm predation on endangered staghorn coral. Mar. Ecol. Prog. Ser. 516, 187–194. doi: 10.3354/meps10996
Miyazawa, E., Montilla, L. M., Agudo-Adriani, E. A., Ascanio, A., Mariño-Briceño, G., and Croquer, A. (2020). On the importance of spatial scales on beta diversity of coral assemblages: a study from Venezuelan coral reefs. PeerJ 8:e9082. doi: 10.7717/peerj.9082
Plaisance, L., Caley, M. J., Brainard, R. E., and Knowlton, N. (2011). The diversity of coral reefs: what are we missing? PLoS One 6:e25026. doi: 10.1371/journal.pone.0025026
Sharp, R., Tallis, H. T., Ricketts, T., Guerry, A. D., Wood, S. A., Chaplin-Kramer, R., et al. (2018). InVEST 3.4. 4 User’s Guide. Palo Alto, CA: The Natural Capital Project.
Shaver, E. C., and Silliman, B. R. (2017). Time to cash in on positive interactions for coral restoration. PeerJ 5:e3499. doi: 10.7717/peerj.3499
Shaver, E. C., Courtney, C. A., West, J. M., Maynard, J., Hein, M., Wagner, C., et al. (2020). A Manager’s Guide to Coral Reef Restoration Planning and Design. NOAA Coral Reef Conservation Program. NOAA Technical Memorandum CRCP 36. Arlington, VA: The Nature Conservancy.
Thompson, D. R., Hochberg, E. J., Asner, G. P., Green, R. O., Knapp, D. E., Gao, B. C., et al. (2017). Airborne mapping of benthic reflectance spectra with Bayesian linear mixtures. Remote Sens. Environ. 200, 18–30. doi: 10.1016/j.rse.2017.07.030
Trygonis, V., and Sini, M. (2012). photoQuad: a dedicated seabed image processing software, and a comparative error analysis of four photoquadrat methods. J. Exp. Mar. Biol. Ecol. 424, 99–108. doi: 10.1016/j.jembe.2012.04.018
Keywords: reef restoration, Caribbean, remote sensing, coral survival, imaging spectroscopy, suitability modeling, coral outplanting
Citation: Schill SR, Asner GP, McNulty VP, Pollock FJ, Croquer A, Vaughn NR, Escovar-Fadul X, Raber G and Shaver E (2021) Site Selection for Coral Reef Restoration Using Airborne Imaging Spectroscopy. Front. Mar. Sci. 8:698004. doi: 10.3389/fmars.2021.698004
Received: 20 April 2021; Accepted: 06 July 2021;
Published: 29 July 2021.
Edited by:
Baruch Rinkevich, National Institute of Oceanography, Israel Oceanographic and Limnological Research, IsraelReviewed by:
Rodolfo Rioja-Nieto, Universidad Nacional Autónoma de México, MexicoAmilcar Leví Cupul-Magaña, University of Guadalajara, Mexico
Copyright © 2021 Schill, Asner, McNulty, Pollock, Croquer, Vaughn, Escovar-Fadul, Raber and Shaver. This is an open-access article distributed under the terms of the Creative Commons Attribution License (CC BY). The use, distribution or reproduction in other forums is permitted, provided the original author(s) and the copyright owner(s) are credited and that the original publication in this journal is cited, in accordance with accepted academic practice. No use, distribution or reproduction is permitted which does not comply with these terms.
*Correspondence: Steven R. Schill, c3NjaGlsbEB0bmMub3Jn