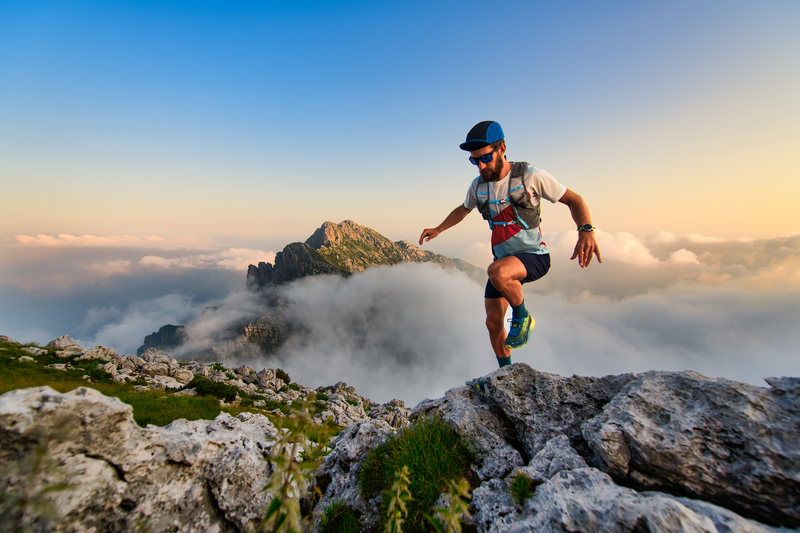
94% of researchers rate our articles as excellent or good
Learn more about the work of our research integrity team to safeguard the quality of each article we publish.
Find out more
ORIGINAL RESEARCH article
Front. Mar. Sci. , 17 August 2021
Sec. Marine Pollution
Volume 8 - 2021 | https://doi.org/10.3389/fmars.2021.697430
Understanding the physical mechanisms behind the transport and accumulation of floating objects in the ocean is crucial to efficiently tackle the issue of marine pollution. The main sinks of marine plastic are the coast and the bottom sediment. This study focuses on the former, investigating the timescales of dispersal from the ocean surface and onto coastal accumulation areas through a process called “beaching.” Previous studies found that the Stokes drift can reach the same magnitude as the Eulerian current speed and that it has a long-term effect on the trajectories of floating objects. Two particle tracking models (PTMs) are carried out and then compared, one with and one without Stokes drift, named PTM-SD and PTM-REF, respectively. Eulerian velocity and Stokes drift data from global reanalysis datasets are used for particle advection. Particles in the PTM-SD model are found to beach at a yearly rate that is double the rate observed in PTM-REF. The main coastal attractors are consistent with the direction of large-scale atmospheric circulation (Westerlies and Trade Winds). After 12 years (at the end of the run), the amount of beached particles is 20% larger in PTM-SD than in PTM-REF. Long-term predictions carried out with the aid of adjacency matrices found that after 100 years all particles have beached in PTM-SD, while 8% of the all seeded particles are still floating in PTM-REF. The results confirm the need to accurately represent the Stokes drift in particle models attempting to predict the behaviour of marine debris, in order to avoid overestimation of its residence time in the ocean and effectively guide policies toward prevention and removal.
Marine pollution is globally recognised as one of the great environmental issues our society is currently facing, with world-leading NGOs warning us that “plastic waste is flooding our oceans (Hancock, 2019)” and launching campaigns to fight “the ocean plastic crisis” (Weyler, 2017). Ocean waters have been contaminated in the last few decades by a range of man-made pollutants, from oil spills to rubber ducks (Ebbesmeyer and Ingraham, 1994). Among all these, plastic has received significant attention in the media. Most of it is non-biodegradable and can survive in the ocean for decades, dramatically affecting marine fauna through entanglement and ingestion (Onink et al., 2019). Plastic debris comes in a huge range of shapes, sizes, and materials, making it difficult to describe and predict its behaviour in the ocean in a reliable way. To prevent inconsistencies, plastic objects are often grouped by size: micro- (< 5mm), meso- (5−25mm), and macro-plastics (> 25mm) (Hinata et al., 2017). Plastic is generally highly buoyant and its maximum concentration in the water column is at the surface, where it is subject to the effect of winds, currents, and waves (Reisser et al., 2015). One particular wave effect that is prevalent close to the free surface is the Stokes drift. It is defined in Oceanography as the net drift that a particle moving in a fluid experiences in the direction of propagation of the fluid's wave field (Van den Bremer and Breivik, 2017). The Stokes drift velocity, , is also commonly described as the difference between the average Lagrangian velocity of the particle at a mean depth, , and the Eulerian velocity of the fluid at the same mean depth, (Röhrs et al., 2012; Breivik et al., 2016):
The Stokes current field generally has larger spatial scales than the Eulerian velocity field and it has been shown to affect the transport of floating objects such as oil droplets (Drivdal et al., 2014), pelagic eggs and larvae (Röhrs et al., 2014), and plastic (Onink et al., 2019).
The marine debris problem has been defined as a source, pathway and sink issue (Hardesty et al., 2017). Two major physical sinks of plastic pollution in the ocean are beaching at the coast and sinking to the bottom sediment (Kaandorp et al., 2020). This study aims at investigating the timescales of the beaching process of floating particles and the main accumulation zones in the presence of Stokes drift, building directly onto existing work (see e.g., Iwasaki et al., 2017; Onink et al., 2019). The hypothesis is that particles at the ocean surface will disperse and gather at the coast at a faster rate if Stokes drift is included in the model. Coastal attractors are also expected to arise at different locations as Stokes drift has been found to change the mean direction of transport, e.g., steering particles toward the poles (Onink et al., 2019). The hypothesis is tested by comparing two Lagrangian particle tracking models (PTMs): one where particles are advected by the Eulerian flow alone and one where Stokes drift is included. Reanalysis data from the HYCOM ocean model (Eulerian current) and the ERA-interim wave model (Stokes drift) are used to advect particles in the North Atlantic. The PTMs are two-dimensional (no vertical axis). To justify this choice, a theoretical background on the vertical scale of Stokes drift and particle concentration is given in section 2.1. The model runs and the methods used for data processing are described in sections 2.2 and 2.3. Section 3.1 presents an overview of the particle simulation output. The beaching process is analysed in terms of its timescales (section 3.2) and spatial patterns (section 3.3). The mean dispersal distance is calculated (section 3.4) and further forecasting of particle distribution over a longer time period is carried out (section 3.5). Finally, an interpretation of results and their implications on marine pollution is given in section 4.
The present experiment focuses on the upper meter at the ocean surface, where the concentration of buoyant particles C is at its maximum. For a steady state, C has a vertical distribution (Drivdal et al., 2014):
where C0 is the surface concentration, ν is a constant eddy diffusivity and wr is the particles' rising speed, i.e. the speed at which particles rise due to buoyancy forces. From Equation (2), the vertical decay scale of particle concentration δp is inversely proportional to their rising speed:
A typical value for eddy diffusivity in the upper ocean is ν = 10−2 m2s−1 (Talley, 2011), while wr varies significantly depending on the chemical and physical structure of the considered item. For instance, oil droplets have a typical rising speed of 400 m day−1 (Drivdal et al., 2014), yielding a depth scale of about 2 m, while Arctic cod eggs with would lead to δp = 8.5 m. Marine litter items, such as small wood pieces or plastic fragments with length scales of 1−10 cm, have an estimated upward terminal velocity of 0.1 to 1 ms−1 (Hinata et al., 2017). The particle depth scale δp would then range between 10 and 1 cm. The Stokes drift on a rotating plane is counteracted by a Eulerian return flow which dominates at depth, while the Stokes current is strongest at the surface (Van den Bremer and Breivik, 2017). For a single wave frequency, the Stokes drift decreases exponentially with depth. Its vertical scale for a full wave field, δSt, has been estimated to be around 1.8 m for basins larger than the gulf of Mexico (Clarke and Van Gorder, 2018). This depth is at least one order of magnitude smaller than the estimated Ekman depth, δEk, in a large basin. Given the magnitude of the three depth scales, the following approximation for the ocean surface can be made:
The Lagrangian velocity experienced by particles under these conditions is equal to the sum of the Ekman and Stokes currents (Clarke and Van Gorder, 2018) (see Appendix A for a more in depth derivation). PTM-REF then corresponds to the case where δp > δSt and, conversely, PTM-SD corresponds to δp ≤ δSt.
To investigate how the inclusion of Stokes drift affects particle transport in a Lagrangian simulation, numerical experiments are carried out with and without Stokes drift. For simplicity, the two experiments will be hereon referred to as PTM-REF and PTM-SD according to Table 1.
Daily means of eastward and northward water velocity from ocean model HYCOM are used to advect particles in PTM-REF, called uEu and vEu, respectively. The HYCOM model (for HYbrid Coordinate Ocean Model) is a data-assimilative hybrid isopycnal-sigma-pressure (generalised) coordinate ocean model (Chassignet et al., 2007). In PTM-SD, daily means of eastward and northward Stokes drift velocities, uSt and vSt, from wave model ERA-Interim (Dee et al., 2011) are added to the HYCOM velocity components. Both velocity datasets range from January 1997 to December 2012 and cover the global ocean from 80°S to 80°N. The domain is reduced to the North Atlantic ocean between 100°W and 60°E and from the Equator up to the Arctic at 80°N. Conclusions regarding the North Atlantic can be applied to the other ocean basins, where an equivalent structure in the accumulation of marine debris is observed (Maximenko et al., 2012). The initial condition consists of 2·104 particles distributed randomly over the spatial domain. This initial condition is repeated five times, by releasing a batch of 2·104 particles on January 1st of years 1997–2001, achieving a total of 105 particles. The five batches are then synced so that all particles begin drifting at the same time (t = 0) and a potential bias due to inter-annual variability is avoided. Seeding particles for the first 5 years is a trade-off between releasing particles for as long as possible while obtaining a drift period of at least 10 years for all particles. The OceanParcels v2.0 framework with its 4th order Runge-Kutta advection scheme is used to advect particles (Delandmeter and Van Sebille, 2019). The particle simulations produce daily outputs which are then processed in the following ways. Firstly, the North Atlantic domain is gridded in 2° × 2° boxes. Southern and northern boundaries are posed at 80°N and at the Equator, respectively. Finally, particles whose velocity drops to 10−7 ms−1 or less are considered beached and removed. This is based on the numerical assumption that such particle velocity values would only appear on land cells. The time-averaged Eulerian and Stokes drift velocities are shown in Figure 1 to gauge the main features of the two. The maximum time-averaged Eulerian flow is one order of magnitude faster than the time-averaged Stokes velocity, with peaks along the western boundaries given by the North Equatorial current and the Gulf stream, as well as the Caribbean and Guiana currents (Figure 1A). The North Atlantic subtropical and subpolar gyres are easily identifiable: the former revolving anticyclonically between 10 and 50°N, and the latter rotating cyclonically in the Northern sector of the domain. The direction of the Stokes drift velocity (Figure 1B) around the subtropical gyre is consistent with large-scale atmospheric circulation (Trade Winds and Westerlies). The effect of the Polar Easterlies is also visible between 60 and 80°N along the coast of Greenland.
Figure 1. Current and Stokes drift velocities in the North Atlantic from global reanalysis ocean and wave models, HYCOM (A) and ERA-Interim (B), averaged over years 1997–2012. Note the logarithmic colour-scale.
Adjacency matrices are a powerful tool to extract statistics on the connectivity of different locations within the domain of a given problem (Jonsson et al., 2020). Here, weighted adjacency matrices are calculated for each of the five batches of particles. Each entry pij of such matrices represents the probability of a particle seeded in a bin i to be found in another bin j after a given time interval Δt. This is calculated as:
where ni→j is the number of particles seeded in bin i and found in bin j at time t1 = Δt and ni is the number of particles in bin i at time t0 = 0. Only active bins are considered, i.e., bins where at least one particle was seeded at time t0. Each active bin is identified by a unique number ranging from 0 to m−1, where m is the total number of active bins. The resulting five matrices are then averaged to produce the stochastic matrix Pij, also known as a dispersal matrix (Botsford et al., 2009). Seasonal variability is thus captured in the dispersal matrix by mixing statistics from different years. This computation is carried out for both PTM-REF and PTM-SD with Δt = 1 year, to compute mean dispersal distance (or “MDD”), and with Δt = 10 years to make long-term predictions of particle dispersal. The MDD is calculated as (Jonsson et al., 2020):
where Qij is a matrix of geographical distance between each bin i and all other bins j. The MDD computed as in Equation (6) indicates how far from their initial position particles are likely to be found after the time interval Δt. Long-term predictions of particle distribution are also computed using matrix multiplication (Botsford et al., 2009). In particular, the same initial condition used for PTM-REF is reshaped into a vector of size m, called here . This is the distribution of particles at time t0. Note that m is the number of 2° × 2° bins that make up the domain, as in section 2.2. As powers of a stochastic matrix are also stochastic matrices, it is possible to take to obtain predictions for the time interval nΔt. The particle distribution at time t1 = nΔt, called , is then given by:
Predictions obtained with the dispersal matrix are validated by comparison with the model output. Figure 2 shows the resulting anomaly. The matrix predictions overestimate concentrations in the subtropical accumulation zone compared to the model, especially in PTM-REF (dark red regions in Figures 2C–F). The maximum discrepancy for PTM-REF in the subtropical region reaches 0.4% of particles per grid-cell, 0.2% more than in PTM-SD. Underestimation occurs, on the other hand, in almost all coastal bins, indicated by the blue lining along the land boundary. In particular, the matrix prediction inaccurately represents the concentration of particles along the coast of Central and South America, especially in PTM-SD, as well as the western coast of Europe and the southern coast of west Africa (Figures 2E,F). The magnitude of the discrepancies increases over time as higher powers of the matrix are taken (low anomaly in Figures 2A,B). Nonetheless, the matrix and model outputs are overall in good agreement with each other, with a mean anomaly of O(0.04%) of particles per grid-cell for both PTM-REF and PTM-SD.
Figure 2. Anomaly between matrix and model predictions (= matrix - model). The adjacency matrix is calculated with a Δt of 1 year and predictions are calculated from the same initial condition as the PTMs. Colours represent the difference in particle concentration per 2° × 2° bin, in percentage, relative to the total number of particles. The anomaly is shown at snapshots of 2, 6, and 12 years for both PTM-REF (A,C,E) and PTM-SD (B,D,F).
Snapshots of the two particle tracking model runs are displayed in Figure 3 to gain a qualitative view of the particle's behaviour over time and highlight the differences that arise when Stokes drift is included. The results suggest that there are areas of particle convergence and areas of particle divergence. After 6 months (Figures 3A,B), all particles seeded North of the Equator have drifted away from this region. A similar divergence is also apparent at the Arctic boundary east of Greenland, as well as in the Gulf of Mexico and Caribbean Sea. After 2 years of run time (Figures 3C,D), the formation of a subtropical accumulation zone around 30°N becomes visible, as observed in previous studies (Maximenko et al., 2012). The area between this subtropical patch and the Equator has been entirely cleared of particles. A similar process appears after 6 years (Figures 3E,F), where the region North of the subtropical patch up to the Arctic shows 0 concentration everywhere. The maximum concentration in the subtropical patch decreases over time in PTM-SD while it remains stable in PTM-REF. At the end of the 12-year run (Figures 3G,H), all remaining particles (i.e., particles that have not beached) are in the accumulation zone. Overall, particle convergence/divergence occurs in the same areas in PTM-REF and PTM-SD. However, the timescales of dispersal from the subtropical accumulation zone differ. In particular, concentrations decrease over time at a faster rate in PTM-SD in this region. Just over 14% of particles are left in the domain by the end of the PTM-SD run, compared to almost 34% in PTM-REF.
Figure 3. Snapshots of model outputs after 6 months (A,B), 2 years (C,D), 6 years (E,F), and 12 years (G,H). Colours represent the amount of particles per 2° bin in percentage, relative to the number of seeded particles. Note the logarithmic scale in the colourbar. Beached particles are detected as in section 2.2 and removed. The text box indicates the amount of remaining particles, ptot.
Not enough clarity has yet been made on the residence time of plastic pollution in the ocean, with estimations ranging from a few years to millennia before it sinks to the bottom or it is washed ashore (Morét-Ferguson et al., 2010). The present experiment provides some insight on the timescales of particle dispersal from the oceanic accumulation zone. The beaching test described in section 2.2 is applied to the PTM-REF and PTM-SD cases. The resulting amount of beached particles is shown, in percentage, in Figure 4A, while Figure 4B depicts the concentration in the subtropical accumulation zone. The coastal accumulation increases rapidly within the first 2 years and it continues growing, albeit with a smaller gradient, until the end of the run. After 12 years, 86% of particles seeded in PTM-SD have beached; 20% more than in PTM-REF. New particles are seeded annually over the entire domain for the first 5 years. Some of these particles are located, at time 0, in those areas of divergence seen in Section. 3.1. The extreme increase in the number of beached particles in the Figure 4A reflects the quick removal of particles from divergence areas. As these regions empty out, the beaching process slows down. The average annual beaching rate (excluding the 5 years of seeding) is 1.6% in PTM-SD. This rate is halved in PTM-REF (0.8%). When particles are seeded, about 22% of particles are located in the offshore accumulation zone. The concentration in this subtropical patch increases within the first 4–5 years, partly due to the constant addition of particles during this time, reaching a maximum of 37% of particles in PTM-REF and 25% in PTM-SD. After the first 3–5 years, it then decreases steadily with a stronger gradient in PTM-SD. The anomaly between the two experiments increases over time, indicating that the subtropical gyre is a stronger attractor without the influence of Stokes drift (PTM-REF case). In particular, the subtropical box contains 32% of particles in PTM-REF at the end of the run, 20% more than the amount found in PTM-SD. The oscillation in Figure 4B appears as a result of seasonal variability.
Figure 4. Amount of beached particles over time (A) and of particles located within the subtropical accumulation zone (B). Quantities are shown in percentage relative to the total number of particles seeded. Beached particles are detected as described in section 2.2. The subtropical accumulation zone is defined as a box with longitude between 70 and 18°W and latitude between 21 and 42°N. Data points are taken every 30 days.
Discrepancies in the beaching process emerge not only in terms of timescales, but also as far as the main coastal areas attracting particles. It is thus worth looking at their distribution at the end of the run on each side of the domain, east and west (see Figure 5). Figures 5A,B show the meridional distribution of beached particles on each side of the domain as a sum in the longitudinal direction of the amount of particles per square degree. Figure 5C depicts the same distribution for all beached particles. Percentages are calculated from the total amount of particles. From the top panels, it is clear that PTM-REF (blue dashed line) and PTM-SD (red line) have a similar distribution in the meridional direction. In particular, they present maxima and minima at similar latitudes. No particles are found anywhere on the coast of the US between 33 and 45°N (Figure 5A) and on the coast of west Africa between 10 and 27°N (Figure 5B). Particles are located mainly North of 30°N in the east, while they appear split in half between north and south in the west. To gain a better understanding of the north-south distribution, the cumulative distribution function (CDF) is shown in the bottom panels (Figures 5D–F). It is calculated by integrating the meridional distribution of beached particles from south to north. The total number of beached particles is around 20% higher in PTM-SD (Figure 5F), in line with section 4. Half of the total beached particles are located in the northern region North of 55°N. This zonal threshold is not maintained when the CDF is calculated separately for the eastern and western coastal boundaries. In PTM-REF over 13% of particles end up South of 30°N on the western side of the North Atlantic (Figure 5D). This value reaches 25% in PTM-SD, meaning that a fourth of all particles beaches on the coast of Central America in this configuration. Another 14% of particles is found along the boundary North of 55°N in both PTMs. This covers the coasts of Canada, Greenland and Iceland. Conversely, <5% of particles wash up on the eastern coast South of 30°N (Figure 5E). This corresponds to a peak in the southern coast of West Africa. The vast majority of particles on the eastern side of the North Atlantic beaches on the European coast North of 35°N, from Gibraltar to Ireland and Scotland, up to the entire coast of Norway and Svalbard. This amounts to around 30% of all particles in PTM-REF and 40% in PTM-SD. The highest peak in concentration of beached particles is found in Northern Ireland in both experiments.
Figure 5. The top panels show the meridional distribution of beached particles at the end of the run on the western side of the domain (A), on the eastern side (B), as well as for all beached particles (C). Data points are in 1° bins and result from a sum in the longitudinal direction. The bottom panels depict the cumulative distribution function (or “CDF”) obtained by integrating the particle distribution from South to North. This is shown for particles beached on either side of the domain (D,E) and for all beached particles (F). Percentages are calculated out of the total number of particles seeded.
Mean dispersal distance (MDD) calculated as in section 2.3 is shown in Figure 6. Low MDD (close to 0 km) is found within the subtropical convergence zone at 30°N and along the coastal regions of West Africa, Northern Europe, and the Caribbean. A significant amount of particles was found to beach in these regions (see section 3.3). Particles seeded in any of these locations are likely to be in the same position after a year. The sector corresponding to the centre of the subtropical accumulation zone showing low MDD is larger in PTM-REF (Figure 6A) than in PTM-SD (Figure 6B), suggesting that particles are more likely to leave the gyre within a year if Stokes drift is included in the PTM. The highest values of MDD surround this area, following the anticyclonic circulation in the North Atlantic. From the anomaly plot (Figure 6C), it can be noted that MDD in the PTM-SD case exceeds PTM-REF almost everywhere, with the maximum difference found by the Canary islands (star marker). However, the mean dispersal distance in PTM-REF is larger along the strong Guiana and Caribbean currents. Stokes drift is weak here (see Figure 1) and pushes directly toward the coast, explaining why particles initialised here in PTM-SD are not likely to travel far. An area of negative anomaly is also found within the subpolar gyre. Here, Stokes drift is strongly directed toward the Norwegian coast, promoting beaching, while the Eulerian current points away from it. The maximum dispersal distance is located near the Equatorial current at 30°W in the PTM-REF case. In PTM-SD, however, it is observed right off the coast of west Africa at 20°N, coinciding with the Canary current. The maximum MDD in PTM-SD is well over a thousand kilometers larger than in PTM-REF. At 20°N, the distance between the African continent (20°W) and the islands in Central America (60°W) is around 4,200 km. This suggests that a particle seeded in this area may cross the North Atlantic ocean in less than a year's time if Stokes drift is taken into consideration.
Figure 6. Mean yearly dispersal distance, in km, for PTM-REF (A) and PTM-SD (B) calculated using Pij with a Δt of 1 year, as in section 2.3. The bottom panel (C) shows the anomaly between the two experiments (= MDDSD − MDDREF). The star markers represent the maximum value in each figure.
The calculation of an adjacency matrix allows for predictions that go beyond the 12 years of the model run and require minimal computational time. With this goal, matrix Pij is computed for PTM-REF and PTM-SD with Δt = 10 years. This allows to investigate particle distribution on a timescale of decades. It is worth pointing out that this calculation is valid for any initial condition. The calculation in Equation (7) is carried out using with n = 2, 5, 10 using the same initial condition as PTM-REF. The resulting distributions are shown in Figure 7. Previously made observations on the location of divergence and convergence zones still apply over a longer timescale. In particular, non-zero concentrations are observed only within the subtropical accumulation zone. The garbage patch at 30°N is present in both cases and in all snapshots, albeit with varying concentrations. After 20 years (Figures 7A,B), 28.3% of particles are still floating at the ocean surface in PTM-REF. In PTM-SD, on the other hand, only 8.4% of all particles remain in the ocean after 20 years: around 20% less than in the case without Stokes drift. This anomaly agrees with the findings in sections 3.2 and 3.3. Fifty years after initialisation (Figures 7C,D), the amount of active particles has decreased by over 10% in PTM-REF and by 7% in PTM-SD, reaching only 1.1%. All particles have beached after 100 years in PTM-SD, while almost 8% of the initial amount remain active in PTM-REF (Figures 7E,F).
Figure 7. Adjacency matrix predictions after 20 (A,B), 50 (C,D), and 100 years (E,F). Pij is calculated with Δt = 10 years to match the timescale of the prediction. Note the logarithmic scale in the colourbar.
The motivation behind the present study was the hypothesis that the inclusion of Stokes drift in models investigating trajectories of floating objects is of particular importance, as implied by previous analyses (Drivdal et al., 2014; Onink et al., 2019). This hypothesis is hereby confirmed as Stokes drift is seen to have an impact both on the timescales of particle dispersal and on the concentration in the main convergence zones. An accumulation zone centred at 30°N is observed within the first 2 years of run-time in both PTMs, covering the subtropical gyre. An equivalent aggregation of particles in the subtropics has been found in a variety of modelling experiments (Lebreton et al., 2012), as well as from trajectories of satellite-tracked Lagrangian drifters (Maximenko et al., 2012) and in-situ observations (Morét-Ferguson et al., 2010). Similar build-ups appear in the subtropics in all five ocean basins as a result of Ekman convergence (Onink et al., 2019). The distribution of beached particles at the end of the PTM runs reveals that the most affected coastal areas are northern Europe on the eastern side of the North Atlantic and Central/South America on the western side. This is in line with the direction of large-scale atmospheric circulation: particles are pushed southwest-ward by the Trade Winds and northeast-ward by the Westerlies. From the mean dispersal distance calculation (section 3.4), it can be inferred that particles released near the European coast are likely to beach locally. This is in agreement with previous studies where the densely populated north-east Atlantic region turned out to be highly impacted by beaching and coastal retention (Chenillat et al., 2021). On the other hand, small islands in Central America may be particularly affected by nonnative marine litter. These islands are located at the rim of the subtropical accumulation zone, from which they receive a constant leakage of particles which may be originating from the other side of the Atlantic and travelling across the ocean in less than a year (Lachmann et al., 2017). The increased mean dispersal distance in PTM-SD may occur as a result of the method chosen to include Stokes drift in the model. As the Stokes drift here is linearly added to the Eulerian flow, the velocity advecting particles in PTM-SD is always larger in magnitude than in PTM-REF, causing particles to travel faster. However, the consistency in direction of the Stokes drift and its predominantly zonal pattern suggest that such an increment in MDD is plausible. The adjacency matrix predictions compare well with the model results (Figure 2). Despite the coarse resolution of Pij, it succeeds in adequately representing the formation of the North Atlantic garbage patch and its decay over time. The long-term prediction (Figure 7) confirms that Stokes drift increases the rate of particle dispersal in the subtropical accumulation zone over time. The Stokes velocity measured by Lagrangian surface drifters has been shown to reach a comparable magnitude to the Eulerian current measured by ADCPs during strong wind events. However, under normal conditions, its magnitude decreases to only 20% of the Eulerian current on average (Röhrs et al., 2012). Despite this seemingly small contribution to the total current, the Stokes drift has a non-negligible effect on the rate of dispersal of floating particles. We find that the number of beached particles per year is doubled in PTM-SD compared to PTM-REF (section 3.2). Evidence of this was previously given in Onink et al. (2019), where Stokes drift was shown to counteract the formation of garbage patches resulting from Ekman convergence.
The present simulation succeeds in capturing the effects of large-scale phenomena acting on particle transport under the chosen conditions, in particular the formation of convergence and divergence zones. However, the model does not account for (1) the sinking of particles to the ocean bottom and (2) the possibility for particles that have washed ashore to drift back into the ocean, thus overestimating the amount of beached particles. The residence time at the surface before sinking due to biofouling is estimated in Chubarenko et al. (2016) for different types of micro-plastic particles. The study finds that polyethylene fibres spend 6–8 months within the upper layers, while spherical particles and plastic pieces remain at the surface for up to 10–15 years. In the latter case, the inclusion of sinking in the present model would not significantly affect the result, as the timescale of sinking is larger than the total run time and estimated residence time. However, in the case of polyethylene fibres or—more generally—of objects whose sinking rate is comparable to the beaching rate or faster, the inclusion of a sinking flux in the model would be of great importance. In future work, a more realistic budget of particle density should be employed with the inclusion of a flux transporting particles from the surface to the ocean bottom. In the framework of adjacency matrices, this can be represented by the probability psrf→btm of transitioning from the surface to the ocean bottom during a time interval Δt (Liubartseva et al., 2018):
where s is the rate of sedimentation, τage is the particle's age and Tsurf is its residence time at the ocean surface. Note that the probability of sinking increases exponentially with the particle's age, giving a realistic representation of the weathering of marine debris at the surface and the consequent buoyancy loss. In terms of beaching, a strict boundary condition at the coast was chosen: particles reaching a land-cell and a certain velocity (< 10−7ms−1) are considered beached and erased. Beaching is thus an irreversible process in the PTMs. This is however not necessarily realistic. Items of marine litter that have washed ashore may in fact drift back into the ocean as a consequence of e.g., a change in wind direction or strong wave action at the coast. With that in mind, a less rigid boundary condition was tested in previous runs of the PTMs, where beaching was reversible. In this configuration, particles whose velocity became smaller than 10−7 ms−1 were counted as “beached” but not erased, allowing them to drift back into the flow. The annual rate of dispersal from the subtropical accumulation zone and onto the coast remained doubled in PTM-SD compared to PTM-REF. A seasonal pattern was discovered in PTM-SD with a higher number of beached particles in summer and a decrease in winter. The same oscillation was not observed in PTM-REF and is likely due to a change in wind direction affecting the Stokes drift. However, there is no indication that the way the model represented this flux in and out of coastal bins is realistic and was thus neglected here. As a future improvement to the PTM, a horizontal diffusivity term such as the one used in e.g., Iwasaki et al. (2017) would have to be included to obtain a more realistic image of the beaching process.
Plastic debris in the ocean has two main sinks: the coast and the bottom sediment (Kaandorp et al., 2020). The buoyancy of an object highly influences its fate, determining which of the two sinks it will end up in. Buoyancy depends on chemical composition and physical properties, such as size and shape. Plastic objects at the ocean surface are subject to weathering due to UV radiation as well as temperature and salinity changes (Jahnke et al., 2017). Weathering leads to fragmentation, which is the process of larger objects breaking up into smaller pieces, leading to the formation of “secondary microplastic” (Jahnke et al., 2017). The rate at which microplastics sink to the bottom sediment is inversely proportional to their characteristic length-scale: given the same buoyancy, the smaller the object, the faster it sinks (Chubarenko et al., 2016). Sinking happens mainly as a consequence of biofouling, as the growth of biofilm around plastic objects is found to increase their density (Jahnke et al., 2017). This suggests that microplastics are more likely to settle within the water column and eventually sink, while larger—or in general, more buoyant—objects are more likely to be transported by winds and currents to one of the convergence zones, whether it be the coast or the subtropical garbage patch. As seen in section 2.1, the Stokes drift has its maximum at the ocean surface. The Lagrangian velocity of highly buoyant items at the ocean surface is equal to the sum of the Eulerian velocity and the Stokes drift velocity (Equation 5, Clarke and Van Gorder, 2018). If these objects are located just below the surface, where they are not directly affected by winds, their behaviour is then closely described by the PTM-SD configuration of the model. Nevertheless, the PTM-REF case is not unrealistic. As seen in section 2.1, the Stokes drift is counteracted by an Eulerian return flow which prevails below the Stokes depth δSt. The return flow is not present at the ocean surface and was thus not accounted for in PTM-SD (Equation 5). However, if the depth scale of particle concentration, δp, were larger than δSt, the effect of the Stokes drift on particle transport would be increasingly dampened by the Eulerian return flow as depth increases. This would lead to a scenario that is closer to PTM-REF. A similar result was derived in Iwasaki et al. (2017), where the contribution of Stokes drift to particle motion was found to depend on particle size, with buoyant large particles moving more rapidly at the surface.
This work presents a comparison between two different particle tracking models (PTMs) to shed light on the effect of Stokes drift on the dispersal of floating objects at the ocean surface. In the first experiment (PTM-REF) particles are advected by the Eulerian current alone, while in the second (PTM-SD) the Stokes drift is added linearly to the Eulerian current to transport particles. Global renalysis products HYCOM and ERA-interim are used for the Eulerian velocity and Stokes drift, respectively. Particles are seeded with a random geographical distribution over the North Atlantic and at different times to avert biases caused by inter-annual variability. The results reveal areas of particle convergence both within the basin and along the coast. An offshore accumulation of particles develops in the subtropics, around 30°N. This is observed in all five ocean basins and has been referred to in the literature as a “garbage patch.” The PTMs and adjacency matrix predictions show that the garbage patch disperses particles faster in PTM-SD, with an annual beaching rate that is doubled compared to the one in PTM-REF. The coastal environment is highly affected as it becomes a stronger attractor for floating particles than the open ocean. The model suggests Western Europe and Central America are the coastal regions most affected by beaching of particles. However, due to enormous difficulties in sampling plastic debris in the ocean, it is difficult to validate these results against independent information. It is shown by the mean dispersal distance calculation that particles beaching in Central America, which is located at the edge of the subtropical gyre, may originate from all the way across the ocean. Particles released off the coast of West Africa in PTM-SD, where the MDD is at its maximum, take under a year to be found almost 6,000 km from their starting point. On the other hand, particles found stuck on the European coast are likely domestic. Investigating the connectivity of different regions of an oceanic basin gives insight on the distances travelled by particles and their timescales. Such considerations can be useful in informing policy change on marine pollution prevention and clean-up. It is estimated that 60–80% of marine debris is comprised of plastic (Lebreton et al., 2012). The present results suggest that the idea that marine plastic may spend hundreds of years floating in the open ocean is likely a misconception. We highlight the need to take into account the Stokes drift velocity when modelling the transport of marine debris by showing that ignoring this wave effect may be causing an overestimation of the residence time of particles in the subtropical accumulation zones. This is of particular importance when modelling the behaviour of buoyant objects of marine litter that sit just below the surface, where the Stokes drift is at its maximum. On the other hand, for debris that tends to settle lower in the water column (such as certain items of microplastic, Hinata et al., 2017), the effect of Stokes drift on particle transport is dampened by the Eulerian return flow. Under these assumptions, both PTMs are deemed valid descriptors of two different limit cases: PTM-REF applies to the case where the depth scale of particle concentration is larger than the Stokes depth (δp > δSt), while PTM-SD better reflects the case where particles concentrate at the very surface of the ocean (δp ≤ δSt).
The raw data supporting the conclusions of this article will be made available by the authors, without undue reservation.
All authors contributed to conception and design of the study. SB performed the numerical simulation, statistical analysis, and wrote the manuscript, with support from GB and FR. GB and FR supervised the findings of this work. All authors contributed to manuscript revision, read, and approved the submitted version.
The authors declare that the research was conducted in the absence of any commercial or financial relationships that could be construed as a potential conflict of interest.
All claims expressed in this article are solely those of the authors and do not necessarily represent those of their affiliated organizations, or those of the publisher, the editors and the reviewers. Any product that may be evaluated in this article, or claim that may be made by its manufacturer, is not guaranteed or endorsed by the publisher.
Botsford, L., White, J. W., Coffroth, M.-A., Paris, C., Planes, S., Shearer, T., et al. (2009). Connectivity and resilience of coral reef metapopulations in marine protected areas: matching empirical efforts to predictive needs. Coral Reefs 28, 327–337. doi: 10.1007/s00338-009-0466-z
Breivik, Ø., Bidlot, J.-R., and Janssen, P. A. (2016). A stokes drift approximation based on the phillips spectrum. Ocean Model. 100, 49–56. doi: 10.1016/j.ocemod.2016.01.005
Chassignet, E. P., Hurlburt, H. E., Smedstad, O. M., Halliwell, G. R., Hogan, P. J., Wallcraft, A. J., et al. (2007). The hycom (hybrid coordinate ocean model) data assimilative system. J. Mar. Syst. 65, 60–83. doi: 10.1016/j.jmarsys.2005.09.016
Chenillat, F., Huck, T., Maes, C., Grima, N., and Blanke, B. (2021). Fate of floating plastic debris released along the coasts in a global ocean model. Mar. Pollut. Bull. 165:112116. doi: 10.1016/j.marpolbul.2021.112116
Chubarenko, I., Bagaev, A., Zobkov, M., and Esiukova, E. (2016). On some physical and dynamical properties of microplastic particles in marine environment. Mar. Pollut. Bull. 108, 105–112. doi: 10.1016/j.marpolbul.2016.04.048
Clarke, A. J., and Van Gorder, S. (2018). The relationship of near-surface flow, stokes drift and the wind stress. J. Geophys. Res. 123, 4680–4692. doi: 10.1029/2018JC014102
Dee, D. P., Uppala, S. M., Simmons, A., Berrisford, P., Poli, P., Kobayashi, S., et al. (2011). The era-interim reanalysis: Configuration and performance of the data assimilation system. Q. J. R. Meteorol. Soc. 137, 553–597. doi: 10.1002/qj.828
Delandmeter, P., and Van Sebille, E. (2019). The parcels v2.0 lagrangian framework: new field interpolation schemes. Geosci. Model Dev. 12, 3571–3584. doi: 10.5194/gmd-12-3571-2019
Drivdal, M., Broström, G., and Christensen, K. (2014). Wave-induced mixing and transport of buoyant particles: application to the statfjord a oil spill. Ocean Sci. 10:977. doi: 10.5194/os-10-977-2014
Ebbesmeyer, C. C., and Ingraham, W. J. Jr. (1994). Pacific toy spill fuels ocean current pathways research. Eos Trans. Am. Geophys. Union 75, 425–430. doi: 10.1029/94EO01056
Hancock, L. (2019). Plastic in the ocean. Available online at: https://www.worldwildlife.org/magazine/issues/fall-2019/articles/plastic-in-the-ocean (accessed August 2, 2021).
Hardesty, B. D., Harari, J., Isobe, A., Lebreton, L., Maximenko, N., Potemra, J., et al. (2017). Using numerical model simulations to improve the understanding of micro-plastic distribution and pathways in the marine environment. Front. Mar. Sci. 4:30. doi: 10.3389/fmars.2017.00030
Hinata, H., Mori, K., Ohno, K., Miyao, Y., and Kataoka, T. (2017). An estimation of the average residence times and onshore-offshore diffusivities of beached microplastics based on the population decay of tagged meso-and macrolitter. Mar. Pollut. Bull. 122, 17–26. doi: 10.1016/j.marpolbul.2017.05.012
Iwasaki, S., Isobe, A., Kako, S., Uchida, K., and Tokai, T. (2017). Fate of microplastics and mesoplastics carried by surface currents and wind waves: a numerical model approach in the sea of Japan. Mar. Pollut. Bull. 121, 85–96. doi: 10.1016/j.marpolbul.2017.05.057
Jahnke, A., Arp, H. P. H., Escher, B. I., Gewert, B., Gorokhova, E., Kühnel, D., et al. (2017). Reducing uncertainty and confronting ignorance about the possible impacts of weathering plastic in the marine environment. Environ. Sci. Technol. Lett. 4, 85–90. doi: 10.1021/acs.estlett.7b00008
Jonsson, P. R., Moksnes, P.-O., Corell, H., Bonsdorff, E., and Nilsson Jacobi, M. (2020). Ecological coherence of marine protected areas: new tools applied to the Baltic sea network. Aquat. Conserv. Mar. Freshw. Ecosyst. 30, 743–760. doi: 10.1002/aqc.3286
Kaandorp, M. L., Dijkstra, H. A., and van Sebille, E. (2020). Closing the mediterranean marine floating plastic mass budget: inverse modeling of sources and sinks. Environ. Sci. Technol. 54, 11980–11989. doi: 10.1021/acs.est.0c01984
Lachmann, F., Almroth, B. C., Baumann, H., Broström, G., Corvellec, H., Gipperth, L., et al. (2017). Marine plastic litter on small island developing states (SIDS): impacts and measures. Report No. 2017:4. Swedish Institute for the Marine Environment.
Lebreton, L.-M., Greer, S., and Borrero, J. C. (2012). Numerical modelling of floating debris in the world's oceans. Mar. Pollut. Bull. 64, 653–661. doi: 10.1016/j.marpolbul.2011.10.027
Liubartseva, S., Coppini, G., Lecci, R., and Clementi, E. (2018). Tracking plastics in the mediterranean: 2d lagrangian model. Mar. Pollut. Bull. 129, 151–162. doi: 10.1016/j.marpolbul.2018.02.019
Maximenko, N., Hafner, J., and Niiler, P. (2012). Pathways of marine debris derived from trajectories of lagrangian drifters. Mar. Pollut. Bull. 65, 51–62. doi: 10.1016/j.marpolbul.2011.04.016
Morét-Ferguson, S., Law, K. L., Proskurowski, G., Murphy, E. K., Peacock, E. E., and Reddy, C. M. (2010). The size, mass, and composition of plastic debris in the western North Atlantic ocean. Mar. Pollut. Bull. 60, 1873–1878. doi: 10.1016/j.marpolbul.2010.07.020
Onink, V., Wichmann, D., Delandmeter, P., and Van Sebille, E. (2019). The role of Ekman currents, geostrophy, and stokes drift in the accumulation of floating microplastic. J. Geophys. Res. 124, 1474–1490. doi: 10.1029/2018JC014547
Reisser, J., Slat, B., Noble, K., Du Plessis, K., Epp, M., Proietti, M., et al. (2015). The vertical distribution of buoyant plastics at sea: an observational study in the North Atlantic gyre. Biogeosciences 12, 1249–1256. doi: 10.5194/bg-12-1249-2015
Röhrs, J., Christensen, K. H., Hole, L. R., Broström, G., Drivdal, M., and Sundby, S. (2012). Observation-based evaluation of surface wave effects on currents and trajectory forecasts. Ocean Dyn. 62, 1519–1533. doi: 10.1007/s10236-012-0576-y
Röhrs, J., Christensen, K. H., Vikebø, F., Sundby, S., Saetra, Ø., and Broström, G. (2014). Wave-induced transport and vertical mixing of pelagic eggs and larvae. Limnol. Oceanogr. 59, 1213–1227. doi: 10.4319/lo.2014.59.4.1213
Talley, L. D. (2011). Descriptive Physical Oceanography: An Introduction. London, UK: Academic Press. doi: 10.1016/B978-0-7506-4552-2.10001-0
Vallis, G. K. (2017). Atmospheric and Oceanic Fluid Dynamics. Cambridge, UK: Cambridge University Press. doi: 10.1017/9781107588417
Van den Bremer, T., and Breivik, Ø. (2017). Stokes drift. Philos. Trans. R. Soc. A Math. Phys. Eng. Sci. 376:20170104. doi: 10.1098/rsta.2017.0104
Weyler, R. (2017). The ocean plastic crisis: plastic debris appears in every ocean of the world. Available online at: https://www.greenpeace.org/usa/the-ocean-plastic-crisis/ (accessed August 1, 2021).
Wijffels, S., Firing, E., and Bryden, H. (1994). Direct observations of the ekman balance at 10 n in the pacific. J. Phys. Oceanogr. 24, 1666–1679. doi: 10.1175/1520-0485(1994)024<1666:DOOTEB>2.0.CO;2
For a single wave frequency ω of a surface gravity wave in a deep ocean, the Stokes drift has the following vertical profile (Van den Bremer and Breivik, 2017):
where c is the phase speed of the wave, a its amplitude, k the wave number, z the fluid depth, negative downwards with z = 0 at the surface, and the unit vector in the direction of wave propagation. The Stokes vertical scale for a single wave frequency δSt is then proportional to wavelength: long waves induce a deeper Stokes drift. Using the dispersion relation for deep water surface gravity waves, ω2 = gk (Vallis, 2017), the vertical decay scale becomes:
In a realistic ocean, the wave field is composed of a sum of waves with different frequencies. To obtain a more truthful picture of the vertical scale of the Stokes drift, Equation (1) must therefore be integrated over the whole wave spectrum S(ω). The Stokes depth δSt calculated from buoy measurements in different locations in the Gulf of Mexico and North Pacific ocean is found to range between 59 cm and 2 m. (Clarke and Van Gorder, 2018).
It is important to understand how the Stokes drift affects the equations of motion at the ocean surface. To do this, the classical Ekman problem (Vallis, 2017) can be solved with the inclusion of the Coriolis-Stokes force: (Van den Bremer and Breivik, 2017). This force arises in the presence of Stokes drift in a rotating ocean. In the Northern Hemisphere, it is at right angles with the direction of wave propagation and further deflects the Eulerian current (Drivdal et al., 2014). During strong wind and high wave events it has a comparable magnitude to that of the standard Coriolis force (Röhrs et al., 2012). For a steady state and in a deep ocean with no horizontal pressure gradients, the equation of motion becomes:
where is the constant Coriolis parameter f multiplied by the vertically directed unit vector and ν is a constant eddy viscosity. Note that, in the absence of friction or mixing, this equation simply yields . This implies that Stokes drift has an induced counter current at a steady state in a rotating ocean. Recalling from standard Ekman theory that the Ekman depth scale is:
and, using the vertical profile of for a single frequency given above, Equation (3) can be solved analytically, giving the following complex solution:
where ū = u+iv is the complex notation for and the non-dimensional number ε has been introduced as the ratio between the Stokes and the Ekman depths, . The first term in Equation (5) is the standard Ekman flow, obtained when there is no surface Stokes drift. The second term represents what is known as the Stokes-induced Eulerian return flow. The mass transport due to Stokes drift is divergent on the scale of the wave group and acts to “pump” water from the waves' trailing edge to their leading edge (Van den Bremer and Breivik, 2017). It therefore generates an opposite Eulerian flow to balance it out and maintain conservation of (vertically integrated) mass and momentum in the water column. For a deep enough ocean, the Stokes drift prevails at the surface above the e-folding depth, while the return flow, which has a slower vertical decay, dominates far below the free surface (Breivik et al., 2016). The magnitude of uSt was found to range between 3 and 13 cm s−1 (Clarke and Van Gorder, 2018), while the Ekman (ageostrophic) current velocity near the surface has been estimated to average 4 ms−1 in the Atlantic ocean (Wijffels et al., 1994).
Keywords: marine pollution, Stokes drift, beaching, plastic, Lagrangian, Eulerian, residence time, garbage patch
Citation: Bosi S, Broström G and Roquet F (2021) The Role of Stokes Drift in the Dispersal of North Atlantic Surface Marine Debris. Front. Mar. Sci. 8:697430. doi: 10.3389/fmars.2021.697430
Received: 19 April 2021; Accepted: 26 July 2021;
Published: 17 August 2021.
Edited by:
Britta Denise Hardesty, Commonwealth Scientific and Industrial Research Organisation (CSIRO), AustraliaReviewed by:
Joseph Harari, University of São Paulo, BrazilCopyright © 2021 Bosi, Broström and Roquet. This is an open-access article distributed under the terms of the Creative Commons Attribution License (CC BY). The use, distribution or reproduction in other forums is permitted, provided the original author(s) and the copyright owner(s) are credited and that the original publication in this journal is cited, in accordance with accepted academic practice. No use, distribution or reproduction is permitted which does not comply with these terms.
*Correspondence: Sofia Bosi, Z3VzYm9zaXNvQHN0dWRlbnQuZ3Uuc2U=
Disclaimer: All claims expressed in this article are solely those of the authors and do not necessarily represent those of their affiliated organizations, or those of the publisher, the editors and the reviewers. Any product that may be evaluated in this article or claim that may be made by its manufacturer is not guaranteed or endorsed by the publisher.
Research integrity at Frontiers
Learn more about the work of our research integrity team to safeguard the quality of each article we publish.