- 1Institute of Oceanography and Fisheries, Split, Croatia
- 2Department of Marine Studies, University of Split, Split, Croatia
- 3Laboratory for Analytical Chemistry, Croatian Veterinary Institute, Zagreb, Croatia
Fish farms, as artificial marine structures with a constant food supply, have a high capacity to attract surrounding wild fish. Different phenotypes of abundant gilthead seabream have been recorded in natural and aquaculture pressured environments in the eastern Adriatic Sea, where the influence of tuna farming on plastic traits associated with habitat use and foraging behaviour remains largely unknown. Several traits, such as body and otolith shape, external colouration, diet preference, and proximate tissue content, were analysed to examine habitat selection of the tuna farm-associated phenotype in comparison to wild and farmed phenotypes. Foraging behavioural shifts in prey selectivity, from hard-shelled bivalves towards soft textured baitfish feed, and local hydrodynamic conditions have initiated plastic responses in farm-associated seabream. Consequently, morphological traits connected with feeding and swimming performance and paler vs. vivid body colouration patterns differed between wild and farm-associated fish, highlighting the existence of resource polymorphism in gilthead seabream. While otolith shape proved to be a reliable phenotypic tracer in distinguishing farmed from wild and farm-associated fish, reduced sensitivity was found between individuals residing in the vicinity of tuna farms and wild ones. To fully understand the impact of fish farms on associated gilthead seabream and its plastic response with a distinctive morph outcome, the underlying molecular mechanisms involved in the regulation of alternative phenotypes needs to be investigated.
Introduction
Interaction and compatibility of aquaculture with the environment is one of the main debated issues linked to the sustainability of this food industry. Based on the expected trends in food consumption, development of farming technologies and their potential long-term ecological impacts on wild habitats, the European Union is anticipating to reach production of 109 million tonnes by 2030 (FAO, 2020). In the Mediterranean, mariculture has been accompanied by growing concerns about the potential impacts of escapees from cage installations on native populations through: (i) predation and competition for food; (ii) competition for space and breeding opportunities; (iii) the spread of parasites and diseases; and (iv) interbreeding with wild fish (Arechavala-Lopez et al., 2013b; Arechavala-Lopez et al., 2017). With an annual finfish production of 1.9 million tonnes (FAO, 2020) and over 21,000 finfish and tuna cages in the Mediterranean (Trujillo et al., 2012), farm leasehold areas also act as high-quality habitats that enhance the productivity of coastal fish assemblages (Sanchez-Jerez et al., 2011; Bayle-Sempere et al., 2013; Stagličić et al., 2017). Farming installations attract large fish aggregations by introducing structures and a constant food supply in form of uneaten feed into the pelagic environment, thereby enhancing the attractive effect on local populations by affecting their presence, abundance, residence time and diet (Dempster et al., 2002; Sanchez-Jerez et al., 2011; Šegvić-Bubić et al., 2011a; Stagličić et al., 2017). Due to eased foraging, wild fish assemblages have enrolled a new ecological role of mitigating the environmental impact of farms by consuming a large portion of the particulate waste generated from rearing activities (Vita et al., 2004; Ballester-Moltó et al., 2017). A recent world-wide assessment has documented up to 60 fish families with 170 species aggregating around sea-cage farms, where at least 17 species were recorded foraging on aquaculture feed (Sanchez-Jerez et al., 2011). A common feature emerging from Mediterranean studies is the consistent record of the family Sparidae around finfish and tuna farms, despite their discrepancies in attracted fish assemblages due to different site selection, i.e., coastal vs semi-offshore, and the proportion of uneaten and type of feed, i.e., pellets vs baitfish (Valle et al., 2007; Šegvić-Bubić et al., 2011a; Bacher et al., 2012; Stagličić et al., 2017).
Highly plastic responses to natural and/or aquaculture-induced environmental cues were observed in several studies of commercially important sparid species gilthead seabream, where significant phenotypic differences were noted between farmed fish and fish of wild origin (Rogdakis et al., 2011; Arechavala-Lopez et al., 2012; Šegvić-Bubić et al., 2014; Fragkoulis et al., 2016; Talijančić et al., 2019). Recently, the occurrence of a third phenotype was observed in the vicinity of tuna farms, where farm-associated individuals differed in morphological and ecophysiological traits compared to their wild counterparts (Talijančić et al., 2019), suggesting that plastic responses to novel environments tend to occur along phenotype components.
A significant increase of wild gilthead seabream has been documented in coastal areas of the eastern Adriatic Sea in recent years, mainly due to: (i) the introduction of domesticated individuals in wild populations through escape events (Šegvić-Bubić et al., 2011b, 2014; Glamuzina et al., 2014; Žužul et al., 2019); (ii) the ecological role of bluefin tuna (Thunnus thynnus) farms as populations sources for aggregated wild populations (Šegvić-Bubić et al., 2011a; Stagličić et al., 2017; Talijančić et al., 2019); and (iii) offspring spillover from tuna farm surroundings to natural nursery grounds of the species (Žužul et al., 2019). Despite the growing body of aquaculture impact research on phenotypic traits, it remains still relatively unknown how, and to what extent, the human activities associated with sea-cage farming influence the ecological attributes of seabream, such as habitat use, feeding habits, morphology, and life history traits. Thus, the aims of the present study were to reveal the factors that drive gilthead seabream adaptations within tuna farm-impacted areas, with reference to wild individuals in habitats with no cage farming, and to expand the base of distinguishable features between wild (WO), farm-associated (FA), and farmed (FO) fish. Since morphological structures require appropriate behaviours and physiological support for proper function, to meet the challenges presented by the environment (Norton et al., 1995), the specific objectives of this study were set to investigate: (i) how aspects of phenotype act to generate variability of body traits in wild and tuna farm-impacted habitats; (ii) applied foraging strategies of seabream in relation to length and disposable food resources; (iii) diet impact on muscle tissue quality composition, fatty acid profiles and skin colouration patterns and, finally, to explore (iv) otolith shape traits of WO, FO, and FA groups and their potential for fish origin classification.
Materials and Methods
Study Area and Sample Collection
Fish from four wild, two farm-associated, and two farm populations were sampled along the eastern Adriatic Sea during the spawning season, October through December, in two consecutive years, 2015 and 2016 (Supplementary Figure 1). Farm-associated seabream were collected below tuna farms (AK, AB) located in two large aquaculture areas in Croatia, where the majority of seabream, seabass, and tuna farming activities take place. In collaboration with local commercial fishing vessels, wild individuals were sampled in four fishing areas (WD, WN, WV, and WI), where no escapees were expected due to the distance of at least 50 km from a fish farming area. Farmed seabream were obtained from two large commercial grow-out farms on the southwest coast of the island of Brač (FS1) and on the southeast coast of the Istrian peninsula (FS2).
A total of 947 adult gilthead seabreams with mean total lengths (TL ± SD) of 26.85 ± 2.6 cm, 26.35 ± 5.4 cm, and 27 ± 1.9 cm were collected for WO (n = 334), FA (n = 320), and FO origin (n = 293), respectively (Table 1). All WO and FA specimens were photographed on the left lateral side of the body using a Canon EOS 600D digital camera (n = 654). From the same sample, an 84% success rate for otolith extraction was achieved (WO, n = 317; FA, n = 232), while a 100% extraction rate was achieved for FO fish (n = 293). A subsample of 99 individuals was used for diet content analysis (WO, n = 42; FA, n = 57). Finally, examination of external colour appearance (n = 149) was performed only on digital images of individuals sampled for diet content analysis, with an additional 50 images sampled from FO fish.
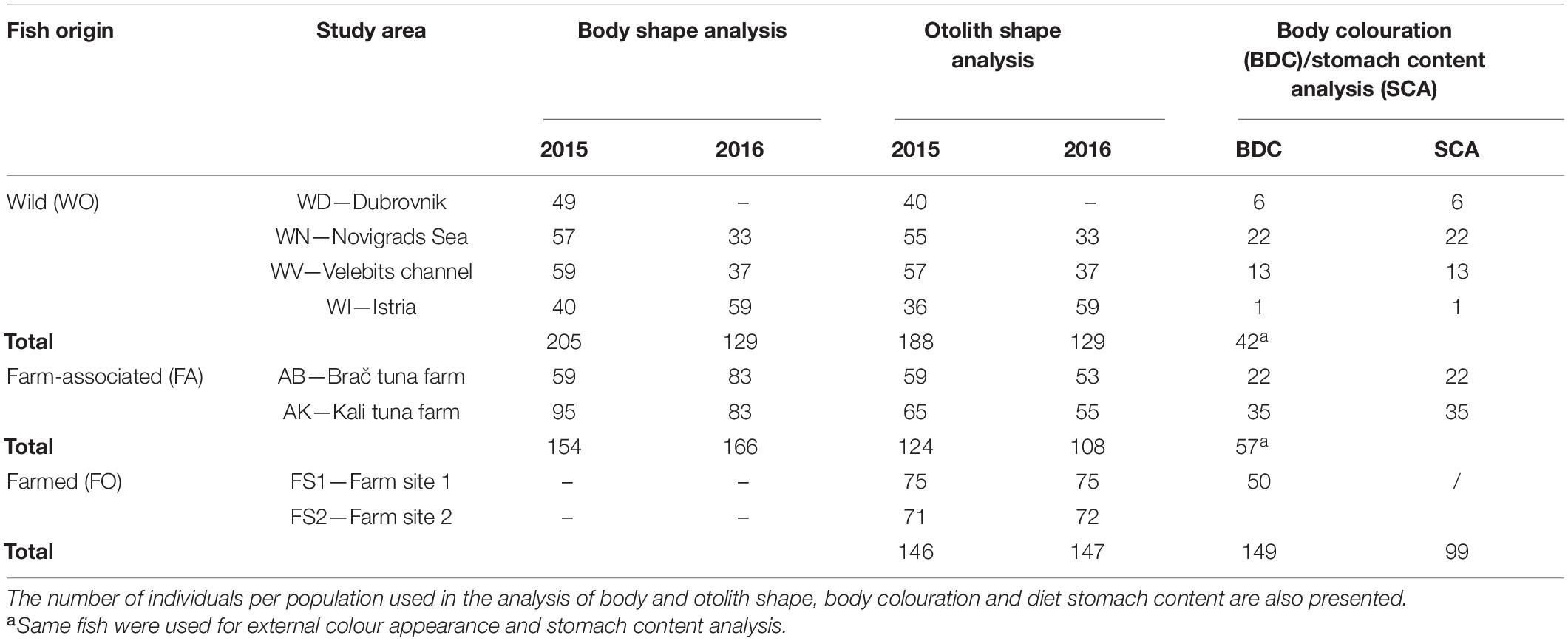
Table 1. Sampling location from the eastern Adriatic Sea, along with the origin and number of gilthead seabream Sparus aurata used in the present study.
Body Shape
Landmark-based geometric morphometrics (GM) was applied for body shape analysis on WO and FA dataset (n = 654), where a total of 38 points were digitised in tpsDig 2 (Rohlf, 2010), of these, 18 landmarks were collected as fixed, homologous points, and 19 semilandmarks as a curve outlining the head profile (Supplementary Figure 2). Semilandmarks were permitted to slide along their tangent directions using the Procrustes distance criterion. Configuration of points (x,y coordinates) were then subjected to a generalised Procrustes analysis (GPA) to retain shape variation by removing the effects of location, scale, and orientation with the gpagen function in the geomorph R package (Adams and Otarola-Castillo, 2013).
Prior to further analyses, allometric relationship of body shapes and log-transformed centroid size (CS) was studied using Procrustes coordinates. The function procD.allometry (geomorph R package) with group inclusion was used to quantify the relative amount of shape variation attributable to covariation with organism size. The homogeneity of slopes (HOS) test was performed to validate if the residuals of the multivariate regression (shape variables vs CS) could be used as size-free variables in subsequent statistical shape analyses. Furthermore, a multivariance comparison of variance within WO and FA origins was performed with vcvComp R package (Maître and Mitteroecker, 2019) in order to test interannual variability of body shapes before being pooled into a group.
Canonical variate analysis (CVA) was performed in MorphoJ software package (Klingenberg, 2011) to visualise body shape features that distinguish wild from farm-associated gilthead seabream. Procrustes shape coordinates were also extracted and used to predict group affiliation using PAST 3.0 software (Hammer and Harper, 2001) by applying the jack-knife cross-validation procedure.
Relative principal component analysis (relPCA) was performed with the vcvComp R package to identify body shape characters that exhibit maximal or minimal excess of variance between populations of WO and FA origin. Such an approach allowed for visualisation of morphological traits that exhibit a plastic response (i.e., higher variance) from those traits that show no response to environmental perturbations (i.e., lower variance). A likelihood ratio test of proportionality (ML) was used to test differences in variance patterns of populations, where a significant deviation from proportionality indicates the presence of divergent or stabilising selection regimes on body shape.
External Colour Appearance
A total of 149 seabream digital images were used for body colour pattern analysis of all three fish origins (Table 1). To obtain accurate colour data in red, green, and blue (RGB) colour space for subsequent variation analysis, the guideline of Stevens et al. (2007) was followed.
The comparison of colours and their patterns was performed with the colordistance (Weller and Westneat, 2019) and patternise (Van Belleghem et al., 2017) R packages. K-means clustering method was applied for the extraction of the dominant colour palette together with their pattern of spatial distribution. Due to the absence of distinct colour pattern boundaries in seabream, the number of clusters was defined manually through trial runs to distinguish the appropriate number of clusters that best assign pixels to a certain dominant colour pattern. The Colordistance R package was applied to quantitatively measure colour similarity among images by using the earth movers distance (EMD) metric to compare pixel colour clusters. Prior to examining whether the means of pairwise colour distance scores (CDS) differ between origins when performing the analysis of variance (ANOVA) comparison, the assumptions of homogeneity of variance and of normality were tested with the Levenes and Shapiro–Wilks tests. The Patternise R package was used to visualise variation in the extracted colour patterns along the outline of seabream body shape, where their alignment was performed with the intensity-based registration technique. For each cluster, the principal component analysis (PCA) and relative proportion of the colour pattern area was calculated in relation to the relative proportion of total body area, to differentiate and indicate possible overexpression of the extracted colour patterns in the observed origins. Statistical differences of pattern areas among the observed fish origins were tested by ANOVA and Tukey’s post hoc test.
Foraging Patterns
A total 99 seabream from WO and FA origin were included in food choice modelling (Table 1). Specimens were weighed to nearest 0.1 g and their total lengths (TL) measured to nearest mm. Stomachs were eviscerated, weighed (wet weight), and preserved in 4% formaldehyde for content examination under a dissecting microscope with reflected light. Quantitative diet analysis was presented by applying three standard indices: the percentage frequency of occurrence (%F = the number of stomachs containing prey item/total number of non-empty stomachs × 100); the percentage numerical abundance (%N = the number of prey items of a given prey category in all non-empty stomachs/total number of prey items in all stomachs × 100); the gravimetric percentage (%W = the weight of prey items of a given prey category in all non-empty stomachs/total weight of food items in all stomachs × 100) (Hyslop, 1980). The contribution of each prey to the diet was assessed using the Index Relative Importance (% IRI) (Hacunda, 1981) based on three previous indices [IRI = (%N + %W) × %F], and expressed as a percentage [(IRI/ΣIRI) × 100].
Based on the prey category found in greatest volume, each fish was additionally categorised in respect to its primary food choice (PFC), i.e., preference to fish, bivalves or invertebrates (for details on PFC see Delany et al., 1999; Supplementary Table S1). Multinomial logistic regression (MLR) was applied as a quantitative method for analysing PFC and used for the likelihood evaluation of food choice trends in seabream in the 22–31 cm TL interval. To estimate response probabilities of a categorically dependent PFC variable, log odds of the outcomes were modelled as a linear combination of the continuous “Total length” and the categorical “Origin” predictor variable. The regression model was developed by selecting bivalves as the baseline for calculating the odds of the fish and invertebrate food categories, since bivalves are known to be the main prey of wild gilthead seabream in the Mediterranean Sea (Kara and Quignard, 2019). Furthermore, the baseline for the “Origin” variable was set to wild, due to the prediction that FA populations exhibit different foraging behaviour than wild ones. The baseline-category logit model was expressed as:
where Yi is the response variable (food choice—PFC), β0 is the constant, β1,2,3 are regression parameters that quantifies the relationships between the predictor and response variables and j is the observed food category (fish, invertebrates). The likelihood ratio test was performed in the mlogit R package (Croissant, 2019) with the use of chi-square statistics for model fit evaluation. Wald statistics was computed to test if the regression coefficients significantly contributed to the prediction of the response food category outcome, whereas McFadden pseudo R2 was used as a proxy for the evaluation of model fitting to the data, where values from 0.2 to 0.4 indicate excellent model fit (McFadden, 1987).
Body Proximate Composition and Fatty Acid Determination
To test the impact of diet preferences in relation to seabream origins, nutritional flesh quality evaluation was performed on 12 individuals, i.e., 4 fish per origin (WO, FO, and FA). Upon catch or harvest, fish were eviscerated and fillets were immediately frozen on dry ice before transferring to storage at -80°C. The fillets were homogenised with a laboratory homogeniser (Grindomix GM 200, Retsch, Haam, Germany) to create a uniform mince. AOAC standard methods were applied for proximate composition and fatty acid analyses, following the protocols of Pleadin et al. (2017).
Otolith Shape Analysis
Left sagittal otoliths were extracted from 842 individuals following the open-the-hatch method (Stevenson and Campana, 1992). After extraction, otoliths were mechanically cleaned and air dried before being digitised using a microscope Dino-Lite Premier. Black and white silhouettes were generated from the images in tpsDig 2 software while outline extraction was performed using the R package Momocs (Bonhomme et al., 2014). The silhouettes were converted into a list of x and y coordinates for each pixel around the contour of a given shape and transformed into quantitative descriptors using Elliptic Fourier analysis (EFA) through harmonic related equations. Due to the irregularities of the otolith shape, the outlines were smoothed to reduce the noise of digitisation by 100 smoothing iterations. Since round and symmetric configurations, such as otoliths, tend to have poor alignments, the superimposition of the extracted outlines was performed by a full generalised Procrustes alignment before EFA (for details see Claude, 2008), without normalising the descriptors. Harmonics were added until at least 99% of the otolith shape variance was reconstructed and Fourier descriptors were obtained for further analysis.
A PCA was performed on the matrix of descriptors to observe shape variation among origins. The principal components (PCs), expressing shape variability, were analysed with multivariance comparison of covariance (MANCOVA) to test the effects of origin and otolith size on seabream otolith shape. The allometric relationship test was performed with maximum or Feret otolith length due to absence of intersample differences in preservation, shrinkage and distortion in comparison to fish length measurements (Campana and Casselman, 1993). As recommended, a descriptor with a significant size effect needs to be removed to achieve an unbiased comparison of shape types (Rodgveller et al., 2017). Prior to further analyses, MANOVA for all three fish origins was performed with the Momocs R package to test for interannual variability of otolith shapes. Linear discriminant analysis (LDA) was conducted to discriminate predefined groups (WO, FO, and FA) of all analysed individuals, followed by otolith shape comparison among origins with a pairwise MANOVA test. The classification success of individuals into their sampled origin was estimated using a leave-one-out cross-validation procedure. Outline areas that most contributed to the difference between origins were identified by comparisons of mean shapes extracted from the descriptors and visualised with Thin-Plate Spline (TPS) analysis.
Results
Body Shape
Size composition differed among populations, where a small yet significant (2%, p < 0.001) amount of shape variation was related to size. In addition, the HOS test recorded significant group allometries (p < 0.001), implying that a fit to a common regression line or to a common model for size correction of shape variables is not justified (Supplementary Table S2). Therefore, the effect of size on shape variables was not removed. The ML test displayed an absence of significant interannual variability of body shapes for WO (p = 0.22) and FA (p = 0.29) samples, allowing populations sampled in different years to be pooled into a single origin group.
The WO and FA origins differed in their average body shapes (p < 0.0001), but with small pair-wise Procrustes distance (0.0063). The main body shape differences were evident in head profile and the posterior part of the body, where FA fish were characterised with a slightly upwards position of the mouth on a smaller head profile and a fusiform body shape in comparison to their wild counterparts (Supplementary Figures 3, 4). The jack-knifed discriminant analysis correctly classified 85.17% individuals to their origins, where FA specimens exhibited a classification rate of 86.56%, as opposed to wild individuals that displayed 83.83% correct classification (Supplementary Table S3).
The multivariate comparison of variance was based on the first five PCs of the full Procrustes data, in order to avoid collinearities and to obtain a sufficient excess of cases over variables in the further analysis. The first three principal coordinates together accounted for 81.4% of the total variance (Figure 1). Differences in variance-covariance patterns between FA and WO populations were demonstrated by comparing farm-associated population (AB) with southern and norther wild populations (WD and WV), though other pairs of WO and FA population gave similar results. The ML test indicated that the covariance matrices of AB and WD (p = 0.038), and of AB and WV (p = 0.002), deviated significantly from proportionality. RelPCA analysis showed that the various features deviate in their variational properties across populations (Figure 2A) where the first relative PC was roughly twice as high in AB than in WD (first relative eigenvalue of 1.92) and WV (first relative eigenvalue of 2.13), whereas the variance of the last relative PC in AB was only the half of that in WD and WV (last eigenvalues of 0.53). The shape features captured by relative PC1 were head shape and the anatomical position of eyes, mouth and pectoral fins (Figure 2B). These morphological traits exhibited maximal excess of variance in FA populations, relative to WO or, in other words, they were maximally canalised in the wild individuals.
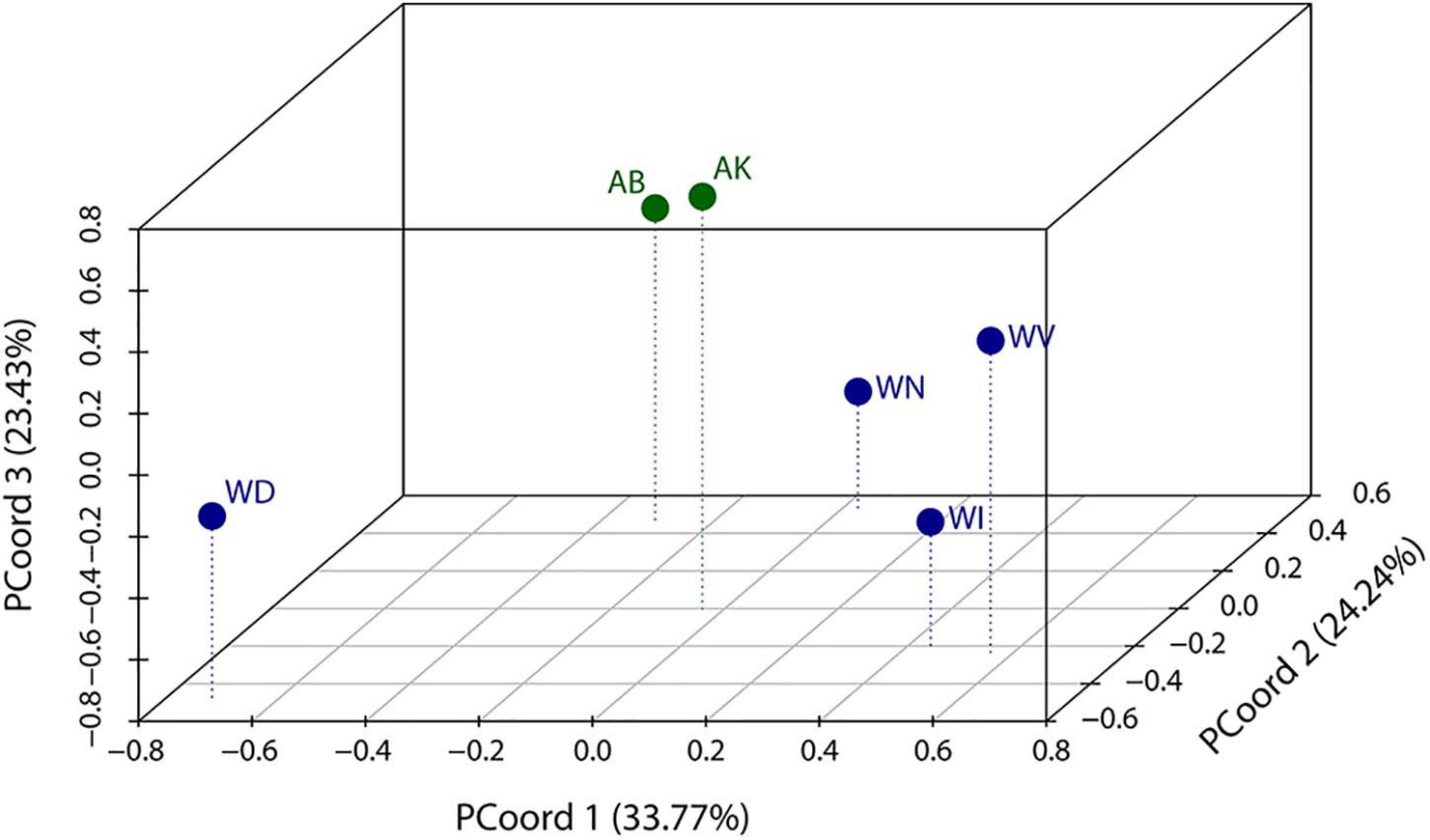
Figure 1. Scatterplot of the first three principal coordinates (PCoord) of the relative principal component analysis. Populations living in wild are shown in dark blue whereas farm-associated populations are displayed in green colour.
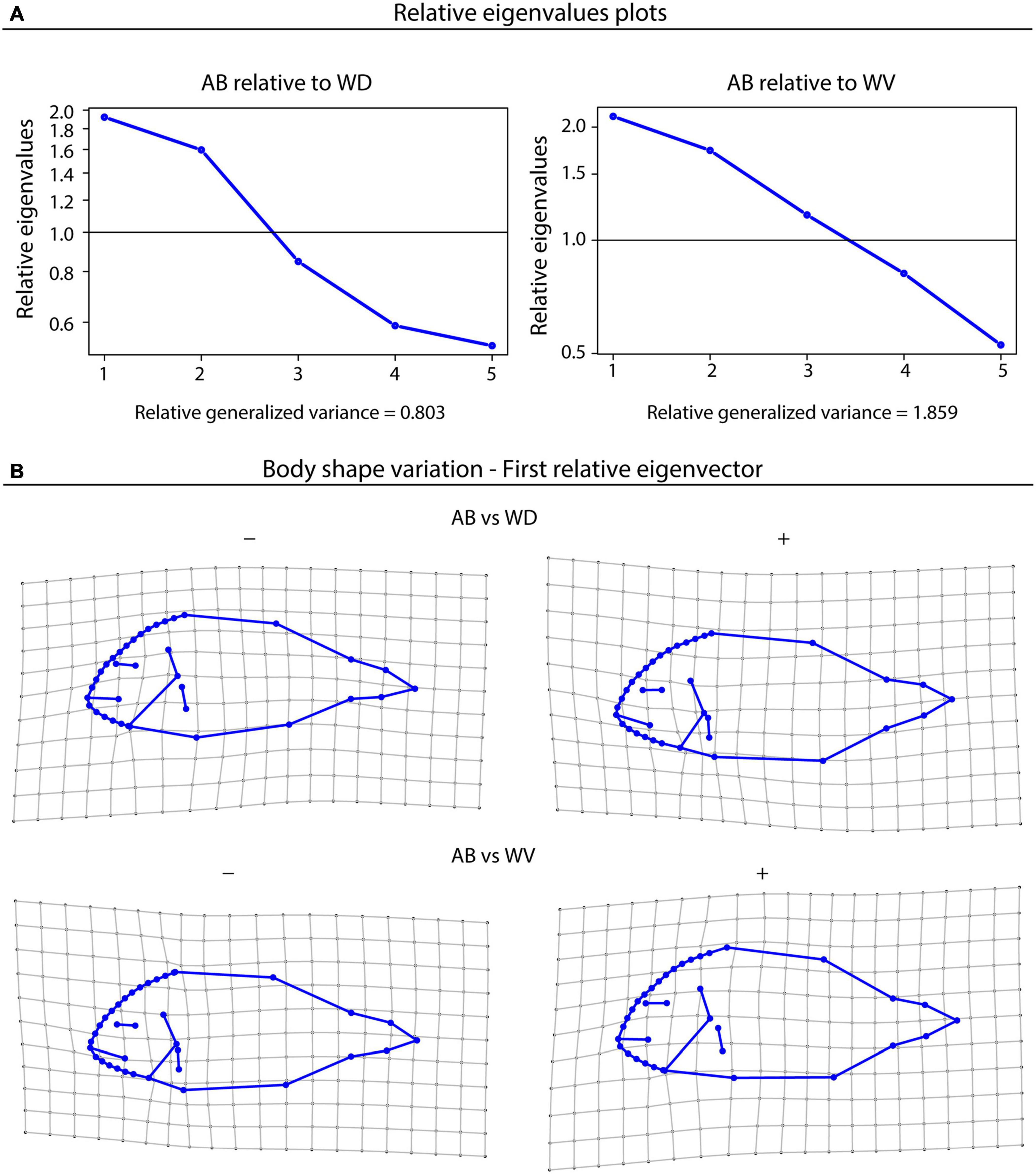
Figure 2. (A) Eigenanalysis of the population comparisons of AB vs WD and AB vs WV; (B) Thin-plate spline deformation grids visualisation of the shape pattern that corresponds to the first relative PC, displaying the maximal excess of variance in AB relative to WD and WV.
External Colour Appearance
From the dataset including all three fish origins (WO, FA, and FO), body colours were k-means clustered into four clusters, ranging from dark to light silvery-grey hues (Figure 3). The highest distribution uniformity of pairwise CDS was noted within the FO origin, whereas the other two origins demonstrated much wider distributions (Supplementary Figure 5). Levene’s and Shapiro–Wilks test indicated that the assumptions of homogeneity of variance (F = 125.06, p < 0.01) and normality (all origins—p < 0.01) were not met and, thus, Welch ANOVA for unequal variances was performed (Supplementary Table S4), together with the Games-Howell post hoc test. A statistically significant difference between origins was recorded (F = 895.85, p < 0.01) where approximately 42% (ω2 = 0.419) of the total CDS variance was attributable to origin (three levels). The post hoc test revealed that the pairwise comparisons WO vs FO and FA vs FO exhibited significant difference (p < 0.01), whereas the WO vs FA comparison displayed a non-significant difference (p = 0.96). Furthermore, EMD distance colour analysis recorded high within-origin similarity in FO, where 71.67% of individuals displayed the same grey hues in contrast to their wild conspecifics, which showed only 11.3% resemblance in the extracted colours. In the case of grey colour resemblance between origins, FA and FO showed a 9.24% similarity, as opposed to WO and FO, which displayed only 1.95% (Table 2).
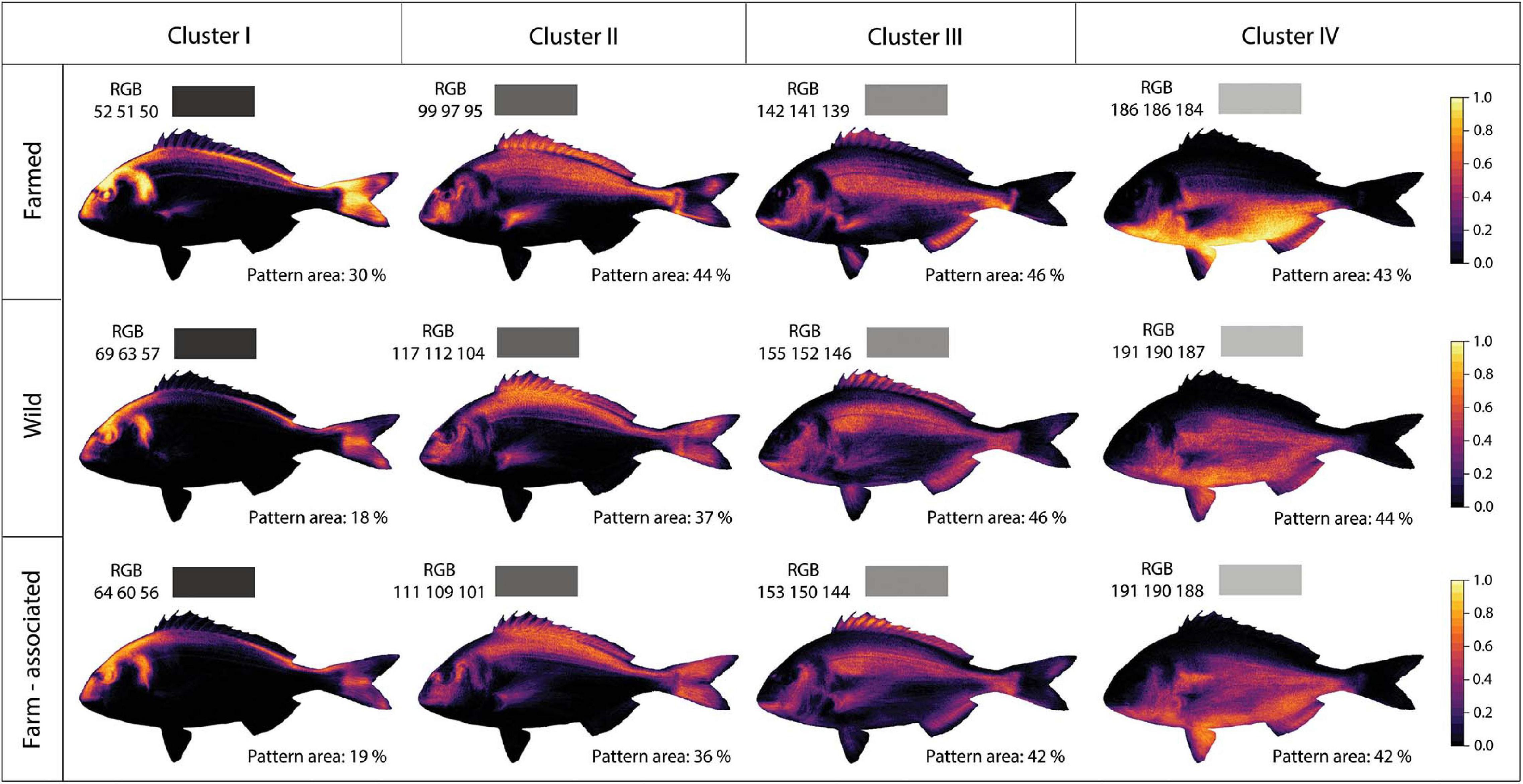
Figure 3. Image registration and k-means clustering of the colour patterns of gilthead seabream. For the wild, farmed, and farm-associated origins (rows) colours were k-means clustered into four groups (columns), ranging from dark to brighter silvery—grey colour hues with displayed average RBG values and coverage (%) of extracted colour pattern area along the body surface area.
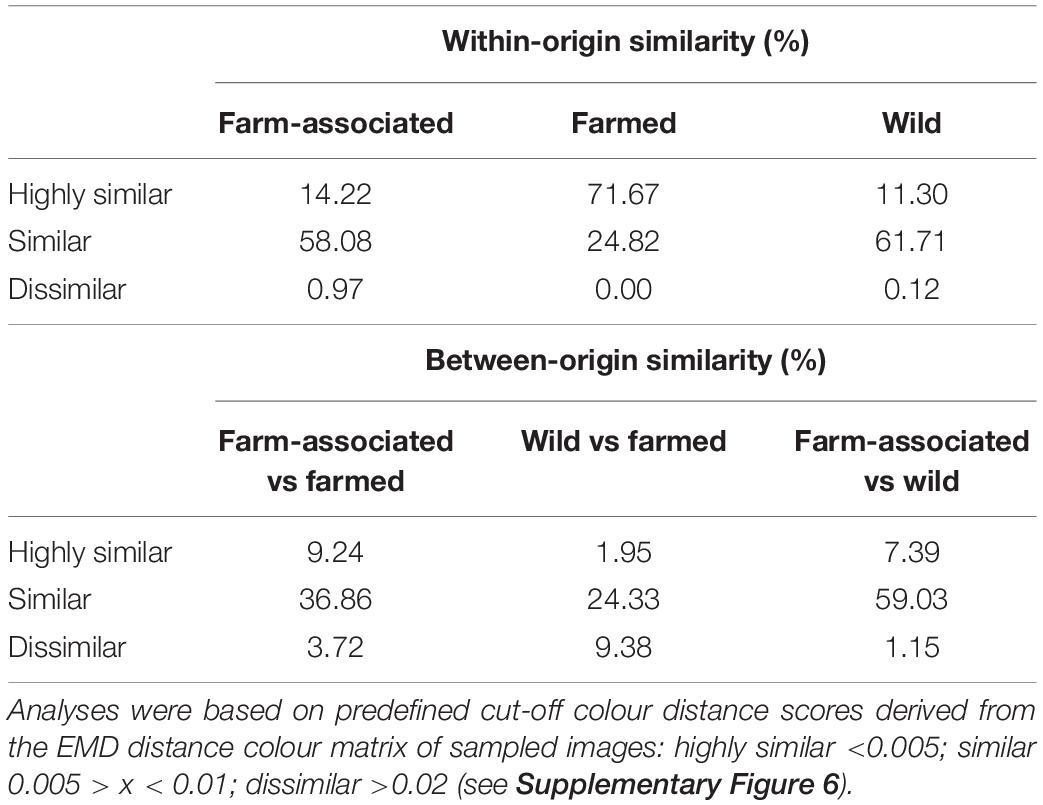
Table 2. Classification of within- and between-origin silvery-grey colour similarity in gilthead seabream.
The heatmap visualisation of colour patterns expression showed that FO had the dullest colouration, exhibiting darker grey colouration (lowest RGB values) in relation to the brighter silvery-grey colouration of the WO and FA populations. The first and fourth cluster of FO displayed the highest proportion rates, with prominent pattern distributions on the posterior part of the operculum, head profile, nape and the snout, dorsal and ventral body area, and caudal fin (Figure 3). The high proportion of the light grey colour cluster (IV) on the ventral body area more strongly emphasised the contrast with the dark grey dorsal areas, further enhancing the dull colouration of FO. The PCA analysis displayed a segregation between darker FO fish from WO and FA individuals (Figure 4). Similar RGB values and colour pattern coverage (%) areas were noted between WO and FA populations, indicating that they do not differ substantially in the extracted silvery grey colouration. A non-significant difference in the pattern areas of the colour clusters was observed only for the fourth cluster (p = 0.144; Supplementary Table S5). Furthermore, post hoc Tukey’s test on the first and second cluster revealed significant differences in the comparison of FO with WO and FA (p < 0.01), whereas for the third cluster significant differences were noted when comparing FA with FO and WO (p < 0.01).
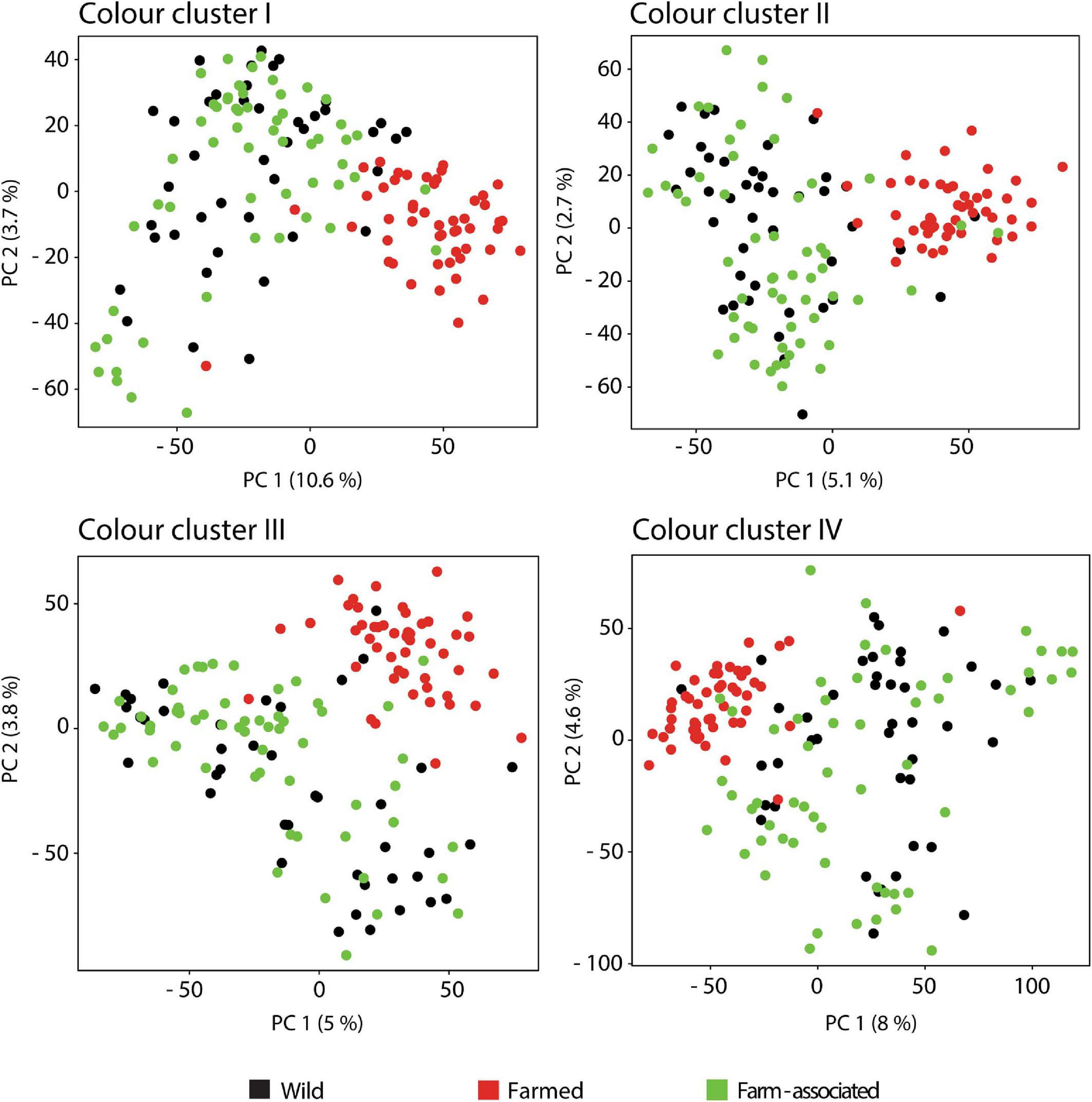
Figure 4. Principal component analysis of the pixel matrices obtained for extracted clusters of silvery—grey colour hues.
Foraging Patterns
The quantitative gut content analysis is presented in the overall list of determinable taxa (Supplementary Table S6). The MLR chi-square test revealed a statistical significance in the overall model fit (log-likelihood = −69.698, χ2 = 52.9, p < 0.001, McFadden R2 = 0.27). A significant interaction was found between the variables Total length and Origin (β = −0.94, Wald = −1.97, p < 0.05), revealing that FA individuals were less likely to choose fish over bivalves as food than their wild counterparts, who showed a higher probability of choosing fish over bivalves as they increased in size (Table 3 and Figure 5).
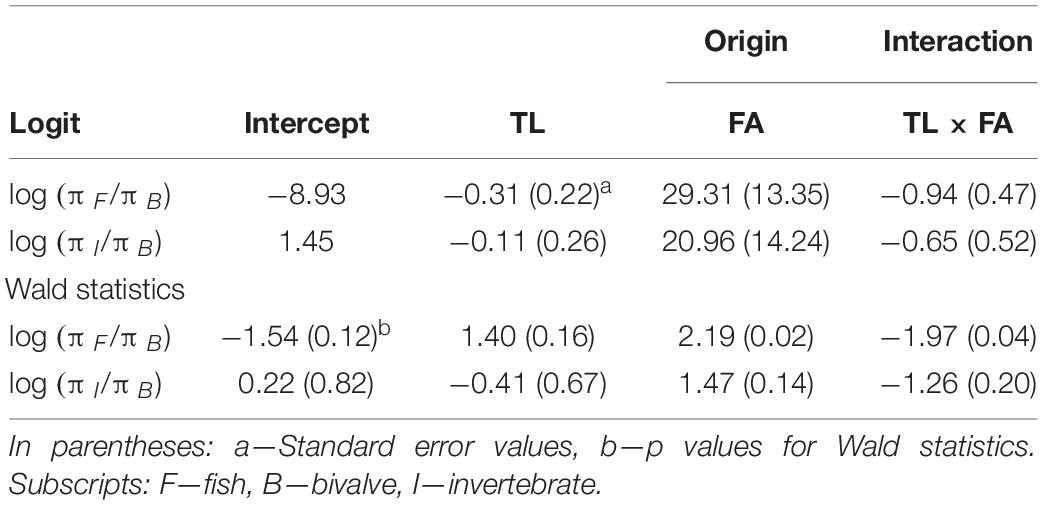
Table 3. Estimated parameters in baseline-category logit model for gilthead seabream dietary preference, based on the quantitative total length indicator variable for size.
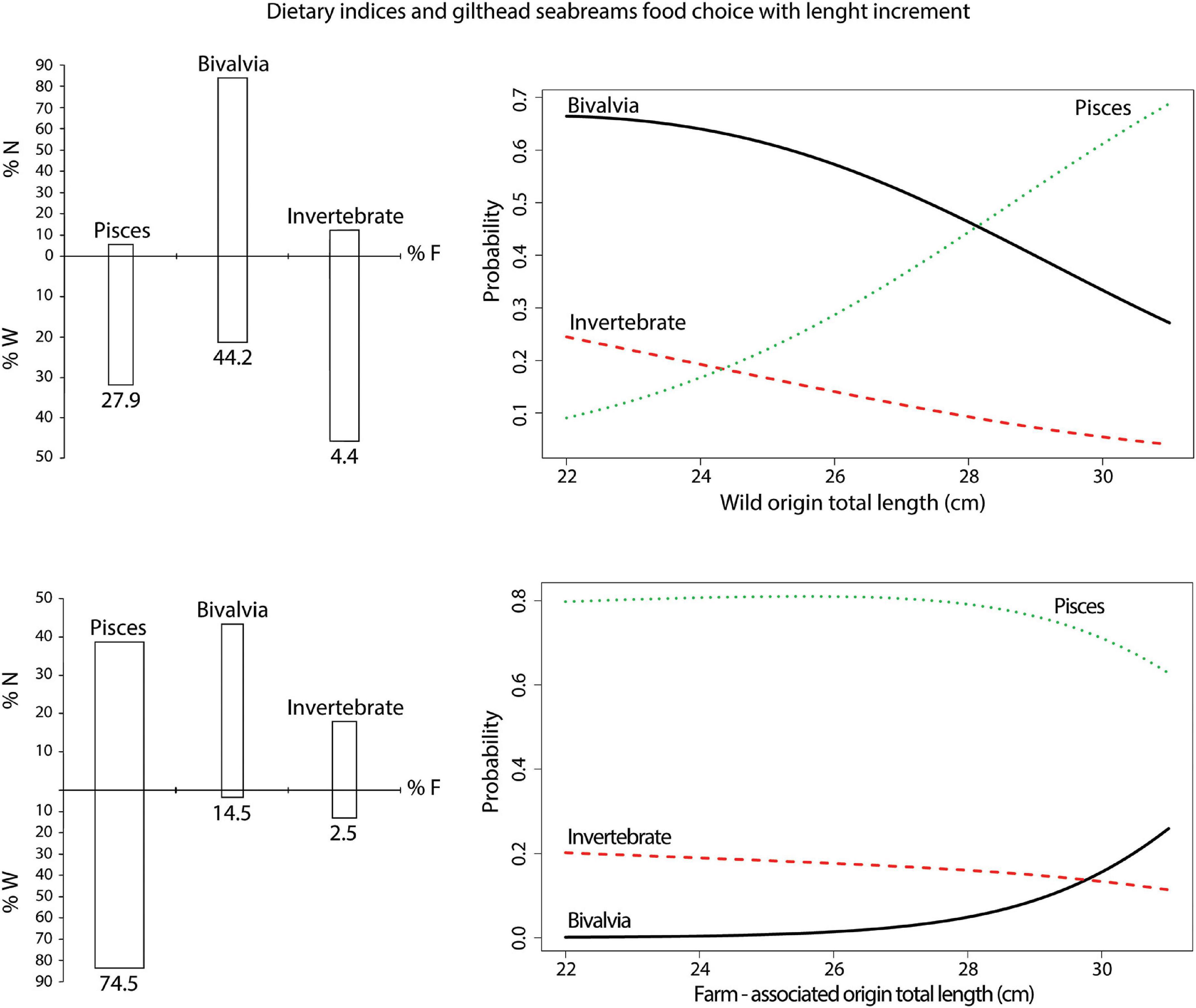
Figure 5. Estimated multinomial regression model for food choice data derived from interaction between “Total length” and “Origin” predictor variables, with corresponding dietary indices of frequency of occurrence (% F), precent number (% N), and weight (% W) for the major prey groups in the diet. The predicted probabilities are plotted against total length of the gilthead seabream specimens by origin for different categories of the food outcome variable.
Body Proximate Composition and Fatty Acid Profiles
The chemical and mineral compositions of gilthead seabream muscle tissue are shown in Supplementary Table S7. The FO group had a higher fat content in comparison to WO and FA groups, whereas protein component and mineral composition of fillets were in a similar range for all observed origins. Fatty acid composition showed substantial variation among fish origins (Supplementary Table S7). While the most abundant fatty acids, oleic and linoleic acids, were substantially more abundant in the FO group, the opposite was noted for palmitic and stearic acid, which were more abundant in the WO and FA groups. The highest proportions of monounsaturated (MUFAs), polyunsaturated fatty acids (PUFAs), total n-3 and n-6 fatty acids, and EPA/DHA ratio were recorded in the FO group, whereas the highest n-3/n-6 ratio was observed in FA fish.
Otolith Shape Analysis
The EFA analysis was able to describe otolith shape by applying 14 elliptical harmonics, extracting 56 individual descriptors. According to MANCOVA, which tested the allometric relationship of otolith length and shape, no significant differences were observed (p = 0.6) and, therefore, all Fourier descriptors were included in the subsequent analyses. The MANOVA displayed an absence of interannual variability of otolith shapes within WO (p = 0.28), FA (p = 0.31), and FO (p = 0.54) samples, allowing both years to be pooled into a single group for each origin. The PCA showed that the first two components account for 62.3% of the total variance. The first axis (PC1, 47.8%) indicated an elongation or suppression of the excisure area, while the variation of the second axis (PC2, 14.5%) was linked to general shape changes associated with a rounding of the otolith, particularly in the posterior region (Figure 6). Variability within the FO sample was considerable, due to the notched anterior region which emphasised the rostrum and antirostrum areas (Supplementary Figure 7), in comparison with the WO and FA shapes, which displayed an angled to peaked anterior region with wide excisura (without or with a shallow notch), and a short, broad and pointed rostrum.
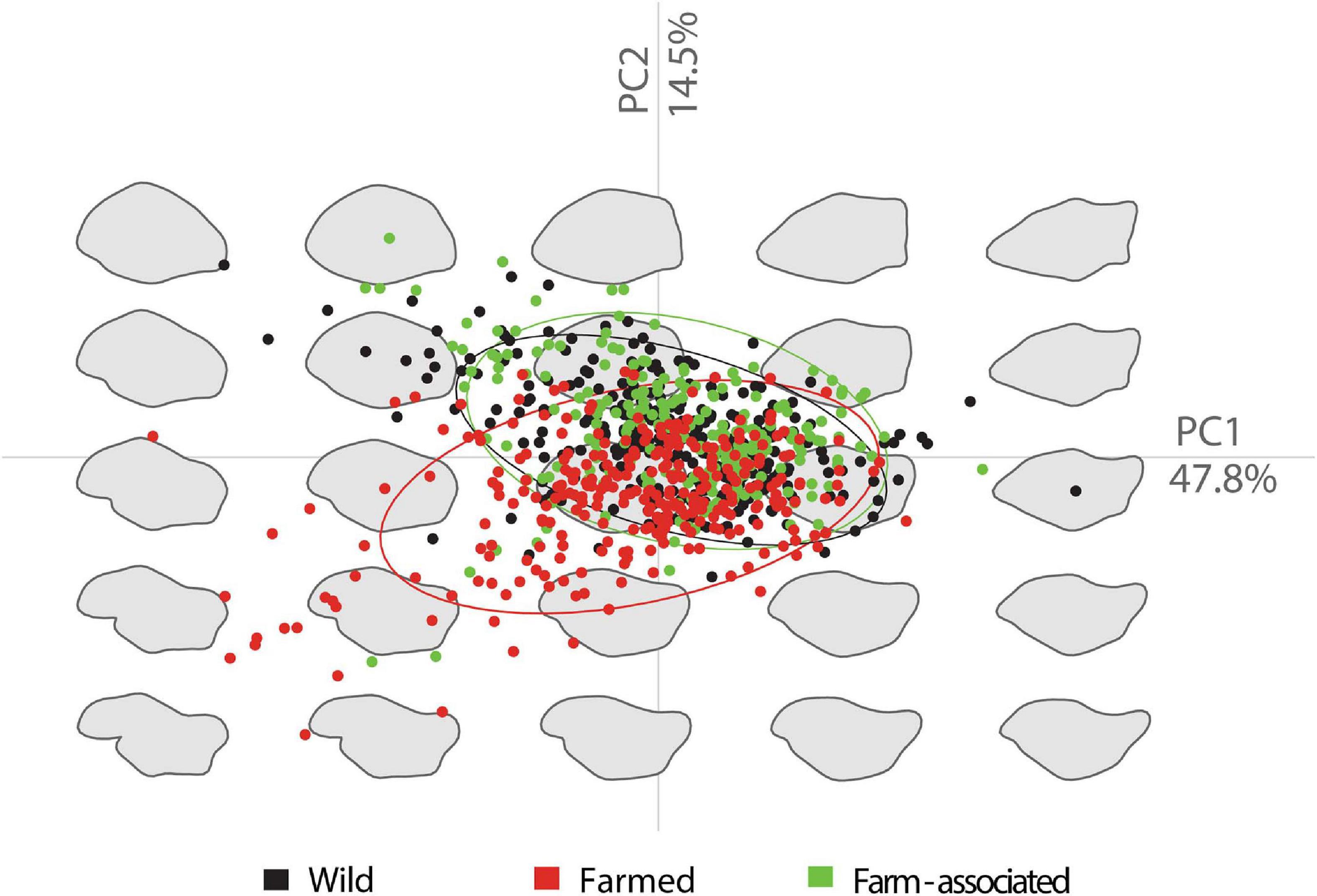
Figure 6. Principal component analysis plot with 95% confidence ellipses depicting the gilthead seabream otolith morphological variation based on elliptic Fourier descriptors.
When attempting to classify otoliths into a group, LDA recorded an overall correct classification rate of 65.8%. Otoliths from FO showed a clear segregation from the WO and FA groups with 87.37% correct classification, as opposed to a 70.98% correct classification rate for WO (Table 4). Due to the overlapping shape pattern among FA and WO, a high misclassification rate of 54.3% was recorded for the FA otoliths classified as WO, and 14.2% as FO. Pairwise MANOVA indicated a significant difference among all origins (p < 0.001). The mean morphological differentiations were related to the rostrum, antirostrum, ventral edge and postrostrum region (Figure 7). The FO fish displayed a significant indentation of the excisura area in relation to WO and FA, which mostly differed on the ventral edge and postrostrum part of the otolith.
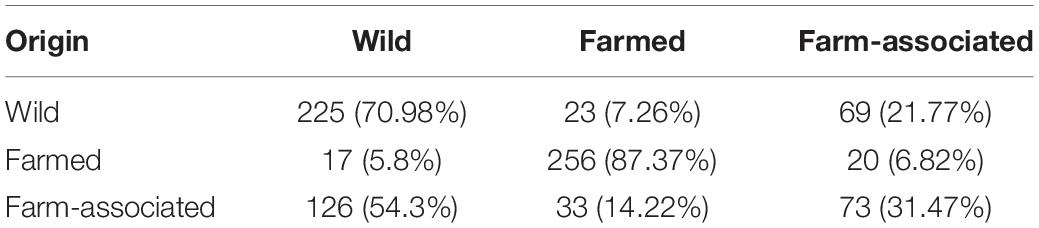
Table 4. Leave-one-out cross validation table of classified seabream according to fish origin, based on the otolith shape characteristics.
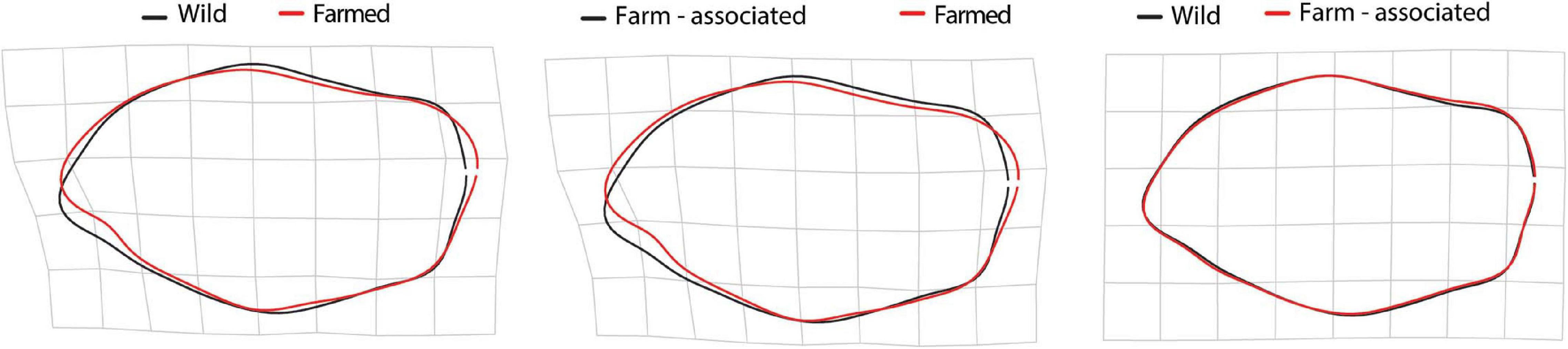
Figure 7. Otolith deformation grids obtained from thin plate splines (TPS) analysis between mean shapes of wild, farm-associated and farmed origin.
Discussion
Phenotypic plasticity can be seen as the ability of individual to produce multiple phenotypes under different environmental conditions (Skúlason et al., 2019). As one of its aspects, trophic or resource-based polymorphism presents the occurrence of intraspecific morphs that show differences in feeding biology or habitat use (Smith and Skúlason, 1996). In particular, habitat diversity and resource niches play an important role in fostering the appearance of phenotypic variants within a population of a single species; these are known as morphs and may differ in morphology, colour, behaviour, or life history traits (Skúlason and Smith, 1995; Smith and Skúlason, 1996). The occurrence of several morphologically and genetically distinct gilthead seabream populations have been recognised in the eastern Adriatic Sea, and classified as being of farmed, wild or farm-associated origin (Šegvić-Bubić et al., 2014; Stagličić et al., 2017; Talijančić et al., 2019; Žužul et al., 2019). In the present study, a multidisciplinary approach was used to explore the impact of aquaculture altered habitats, such as tuna farm leasehold areas, on the occurrence of farm-associated fish phenotypes, through analyses of body and otoliths shape, external colouration and dietary preferences.
Fish Body Shape Characteristics and Its Variation According to Habitat and Trophic Resources
The body shape dataset analysed here described the farm-associated gilthead seabream as a fish with a slightly upwardly positioned mouth on a smaller head profile, where a blunter snout was noted due to diminished head curvature, and with a more fusiform body shape tapering towards the tail in comparison to their wild counterparts (see Supplementary Figure 3). These differences between fish origins may appear minor, though the classification test revealed a high number of correctly assigned individuals (85%), with only 13% of farm-associated fish of misclassified as being of wild origin (see Supplementary Table S3 and Supplementary Figure 4). Inclusion of head curvature shaped by 19 semilandmarks into the GM analysis generated an even higher correct classification score, in contrast to a study where only fixed landmarks were used on the same dataset, achieving a total correct classification score of 77% (Talijančić et al., 2019). Such an approach enabled better resolution of morphological trait variabilities within and among fish origins.
Namely, a two-fold higher variability of head shape, orientation of mouth and positions of eyes and paired fins was found within farm-associated populations than in wild populations (Figure 2B). This discrepancy in trait variability can be linked with resource variation of these environments, where the type, availability and abundance of resources all influence the overall fish morphology. In comparison to natural habitats, tuna farms provide additional structural complexity in the water column through sea cage infrastructures, food availability via baitfish loss and high hydrodynamic complexity, due to site selection criteria that require localities with high current velocities (Šegvić-Bubić et al., 2011a; Stagličić et al., 2017). The present study noted differences in dietary indexes (see Supplementary Table S6) where farm-associated individuals consumed fish prey (% IRI = 92) in contrast to the bivalve-based diet of wild fish (% IRI = 75), confirming the role of baitfish feed as the main trophic resource for wild aggregated fish populations (Fernandez-Jover et al., 2020). Thus, dietary and behavioural shifts, as reflected in the foraging position and type of prey consumed, were likely the prevailing factors responsible for initiating morphological plastic responses.
Farm-associated seabream exhibited a more fusiform body shape overall, due to the deeper/wider anterior body, tapering towards the tail region (see Supplementary Figure 3). This observed body shape enables better resource exploration and reflects morphological adaptation that provide an advantage under high hydrodynamic water conditions (Bracciali et al., 2016). High variation in fin position indicates the adjustment in locomotor repertoires in concordance with foraging behaviour where fish was the preferred dietary category. While durophagous wild seabream feeds mainly by grasing on prey in natural environments (Kara and Quignard, 2019), non-evasive and free-falling baitfish feed induces greater use of the paired fins to perform precise manoeuvres in any plane to catch the preferred prey (Webb, 1984). The correspondence of shape changes with the general pattern of dietary shifts, the transition from smaller and softer to larger and harder prey, has been noted previously in gilthead seabream, particularly in the head region and body profile (Russo et al., 2007, 2014). It can be speculated that adaptation of gilthead seabream to capture baitfish from the water column also triggered the development of a more upturned mouth, where the increased head shape variability reflects less frequent use of crushing force due to a monotonous, soft diet based on fish. A smaller head profile with blunter snout was also observed in farm-associated salema Sarpa salpa and bogue Boops boops residing around finfish sea cages (Abaad et al., 2016). Regardless of the species reared and feed used, it seems that farms elicit similar patterns of plastic responses in aggregated fish. It has been demonstrated that favourable ecological conditions promote the expression of plasticity in phenotypic traits (Van Buskirk and Steiner, 2009) and, in case of fish farms, the high resource availability generates greater variance in body shape traits. Consequentially, this initiated the process of morphological divergence between farm-associated fish and fish of wild origin. On the contrary, scarcer foraging conditions in natural niches promote a less plastic wild body shape by maintaining lower morphological variance and characteristics associated with the gilthead seabream durophagy eating behaviour.
Based on the quantitative model applied here that aimed to evaluate food choice trends of fish in accordance with origin and size, the farm-associated seabream showed a consistently higher probability of choosing fish as the main pray in relation to fish of wild origin, where a transition was noted from bivalves to fish prey as the preferred food type with increasing size (see Figure 5). The observed food choices reflected the optimal foraging behaviour concept on the one hand, and energy optimisation linked with reproduction success on the other (Townsend and Winfield, 1985). Namely, the predator maximises energy gains by evaluating prey handling time and its abundancy in the environment, i.e., the basic prey model (Gill, 2003). Due to an unlimited source of baitfish around farms (Šegvić-Bubić et al., 2011a; Stagličić et al., 2017; Fernandez-Jover et al., 2020), as a soft-textured prey rich in macro- and micronutrients that can be easily handled (Andrew et al., 2003), farm-associated seabream have favoured prey selectivity towards fish, despite the high quantities of biofouling communities on cage nets available as an alternative food source. For wild seabream, the diet is primarily based on bivalves, arthropods, and gastropods (Pita et al., 2002; Šegvić-Bubić et al., 2011a), and the modelled transition from bivalves to fish prey coincides with length sizes when gonad maturation and spawning of females occurs, explaining the shift in feeding behaviour towards a diet with a higher energy content that is required for successful reproduction (Lloret et al., 2014).
Impact of Diet on Flesh Quality Composition
In conjunction with the stomach content analysis, biochemical composition of tissue provided an additional insight into the trophic status of gilthead seabream and assisted in the detection of resource polymorphisms found in this dataset. Farmed seabream had a higher lipid and lower water content compared to the other two origins (see Supplementary Table S7), as a result of the high fat level intake and reduced activity in cultured conditions (Alasalvar et al., 2002). The increased levels of oleic and linoleic acid and a low n-3/n-6 ratio found in farmed seabream confirms their usefulness as indicators of pellet feed consumption, due to the inclusion of vegetable oils in fish feed. Namely, fatty acids of terrestrial origin are traceable due to their low natural presence in marine organisms (Arechavala-Lopez et al., 2013b). Also, higher values of EPA, DHA and n-3 fatty acids observed in farm-associated seabream in comparison to wild fish (see Supplementary Table S7) reflect the rich omega-3 fatty acid content of consumed baitfish (Mourente and Tocher, 2009), even though bivalves, as the primary prey of wild populations, are a good source of high quality lipids (Tan et al., 2020). These fatty acids are essential nutrients needed for optimal reproduction of gilthead seabream (Moretti et al., 1999). A transference of somatic energy for acquiring higher reproductive potential in populations associated with tuna farms has been reported, with a deviation from the known protandry sex reversal pattern, where functional females occurred at smaller body lengths than in wild individuals (Stagličić et al., 2017; Talijančić et al., 2019). Due to the complexity of fish reproduction, it is difficult to determine whether an increase in essential fatty acid content promotes the reproductive performance of resident populations. It appears that seabream possesses the ability to selectively forage those trophic resources that are beneficial for successful reproduction, especially during the spawning period when seabream continues to feed (Fernández-Palacios et al., 2011).
External Body Colour and Otolith Appearance Features
Differences in skin pigmentation between wild and farmed origins were recognised when image-based colour analysis was performed (see colour clusters I and II in Figure 3). Farmed fish exhibited a higher percentage of darker silvery-grey hues and their coverage on the (i) head region, particularly around the golden band between eyes; (ii) enlarged black notch at the origin of the lateral line; (iii) upper body area, where an increased number of dark longitudinal lines was recorded on the flanks, and on the (iv) dorsal, caudal and pectoral fins. To the best of our knowledge, this is the first study to describe the main colouration patterns along the body surface using automatic and non-invasive image analysis, and the results indicate it is a usable tool for wild and farmed fish stock discrimination. The results presented here are consistent with the findings where qualitative colour analyses were applied. Wild seabream were described as ones that exhibited vivid pigmentation, whereas farmed fish were reported as either with a pale greying or a darken skin description (Grigorakis et al., 2002; Rogdakis et al., 2011; Šimat et al., 2012). It seems that farming conditions, including high fish densities, daylight exposure and commercial feed deficient in natural sources of pigments, triggers an excessive production of melanin (Pavlidis et al., 2011). As a result, farmed fish show high colouration similarity and a typically dark farmed appearance, regardless of broodstock source and farming location, as observed in this study (see Table 2, Figures 4, and Supplementary Figure 5).
Interestingly, resident populations in the vicinity of tuna farms showed a greater colour similarity with the farmed group (46.09%) than with wild counterparts (26.28%), displaying the presence of a paler main body colour. It can be argued that due to foraging behaviour, where farm-associated individuals actively chose fish over other prey, the adequate carotenoid content needed for exhibiting iridescent and vivid colour hues is not achieved from the diet, as in case of wild fish having a carotenoid rich diet based on bivalves and other invertebrates. Inclusion of natural carotenoid sources in a low fishmeal diet can improve skin pigmentation of farmed seabream, especially with a mixture of dried marine microalgae and red swamp crayfish meal (Pulcini et al., 2020).
In combination with morphological body features, otolith shape is widely used as an effective tool for stock discrimination (Afanasyev et al., 2017), even for different origins growing under the same environmental conditions (Cardinale et al., 2004; Vignon and Moran, 2010). It has been recognised that otolith shape reflects an interplay of environmental, genetic and ontogenetic influences. In the case of gilthead seabream, this is an informative phenotypic tracer for farmed vs. wild fish recognition (Arechavala-Lopez et al., 2013b). In the present study, we observed an otolith specific shape response corresponding to fish origin when 56 Fourier descriptors were included, despite the high variability of the anterior–ventral notched part found in farmed fish that induced diversification of the rostrum and antirostrum structures (Figure 6, Supplementary Figure 7). Still, an indentation of the excisura area was found to be the main mean shape trait responsible for successful classification of individuals to their origin, when farmed (87%) and wild fish (71%) were considered, respectively. A similar farmed vs wild differentiation pattern in mean otolith shape of gilthead seabream has been reported elsewhere (Geladakis et al., 2020), suggesting that environmental conditions, including an altered diet, play the primary role in the formation of a farmed-specific otolith shape, while the genetic background was important to a lesser extent. Considering that the 70% level of successful classifications, this supports a hypothesis of separate fish stocks (Harbitz and Albert, 2015), and the applied image-based techniques and EFA methods in this study proved to be a reliable tool for wild-farmed origin delineation (see also Geladakis et al., 2020). Farm-associated individuals showed the lowest classification rate (31%) to its origin, with half of individuals classified as being of wild origin, as expected, due to limited shape variations between the origins. Only the ventral edge and postrostrum area varied, producing the rounder mean shape in FA individuals (Figures 6, 7). For several marine species, Mille et al. (2016) showed variations both in global shape, such as the degree of ellipticity, and in finer details, such as otolith crenation or the width of the excisura major, with intrapopulation differences in the taxonomic prey categories consumed. Authors suggested that protein composition in the saccular endolymph, which varies in accordance with the type of prey digested, contributes in the synthesis of otolith matrix proteins involved in the control of crystal structure. Thus, the dietary differences of farm-associated and wild fish (ensuing from a fish vs. bivalve-based diet) could explain the shape variations found in the present study.
Conclusion
The multidisciplinary approach employed here revealed environmentally responsive plastic traits in populations of gilthead seabream, separating individuals of wild, farm-associated, and farmed origin when colouration, feeding behaviour and traits in body and otolith shape were considered. As farmed populations have been modified by their cultured environment and artificial selection, so have the farm-associated populations been shaped by their interactions with tuna farms, particularly with cues such as resource availability and local hydrodynamics. Still, to fully understand the different phenotypic outcomes of farm-associated gilthead seabream and whether this can be considered a distinctive morph stemming from resource polymorphism, the associated molecular mechanisms, both genetic and epigenetic, involved in the regulation of phenotypically plastic traits, should be studied. Whether these plastic traits are subject to selection and are ultimately manifested as an adaptive phenotype through quantitate genetic changes remains a challenge for future work. Concerning the applied methodologies, digital technology has proven useful in depicting the external appearance through images, for both body shape and colour analysis, respectively. Simultaneous acquisition of different traits increases the discriminating power and applicability of morphometric techniques as tools for controlling escape events within wild populations or fisheries landings. However, the degree to which phenotypic plasticity allows the farmed phenotype to (re)converge on a wild-type phenotype over time in the natural environment is still unknown, especially for the individuals escaping in early ontogenetic stages (Rogdakis et al., 2011; Arechavala-Lopez et al., 2013a). Thus, otolith shape presents a more useful phenotypic tracer due to its solid structure in comparison with environmentally plastic body features. Since escaped fish have an ecological and socioeconomic footprint on the environment and fisheries, these findings may be beneficial in allowing for more effective traceability of escapees in the wild.
Data Availability Statement
The raw data supporting the conclusions of this article will be made available by the authors, without undue reservation.
Ethics Statement
Ethical review and approval was not required for the animal study because fish samples were sourced from commercial fisheries.
Author Contributions
IT and TŠ-B conceived the study. IT, IŽ, LG, VK, and TŠ-B conducted the sampling. JŠ conducted stomach content analyses. JP proximated composition and fatty acid determination. IT performed the statistical data analyses. IT, TŠ-B, and IŽ wrote and revised the manuscript. TŠ-B obtained funding for the work. All authors have reviewed and approved the final manuscript.
Funding
This study was fully supported by the Croatian Science Foundation under project number IP-2014-09-9050.
Conflict of Interest
The authors declare that the research was conducted in the absence of any commercial or financial relationships that could be construed as a potential conflict of interest.
Acknowledgments
The work of Ph.D. candidate IT was fully supported by the “Young researchers’ career development project – training of doctoral students’ of the Croatian Science Foundation”, funded by the European Union from the European Social Fund under project number IP-2014-09-9050. The authors are grateful to local farmers for assistance with sample collections.
Supplementary Material
The Supplementary Material for this article can be found online at: https://www.frontiersin.org/articles/10.3389/fmars.2021.694627/full#supplementary-material
References
Abaad, M., Tuset, V. M., Montero, D., Lombarte, D., Otero-Ferrer, A. L., and Haroun, R. (2016). Phenotypic plasticity in wild marine fish associated with fish-cage aquaculture. Hydrobiologia 765, 343–358. doi: 10.1007/s10750-015-2428-5
Adams, D. C., and Otarola-Castillo, E. (2013). Geomorph: an R package for the collection and analysis of geometric morphometrics shape data. Methods Ecol. Evol. 4, 393–399. doi: 10.1111/2041-210X.12035
Afanasyev, P. K., Orlov, A. M., and Rolsky, A. Y. (2017). Otolith shape analysis of a tool for species identification and studying the population structure of different fish species. Biol. Bull. 44, 952–959. doi: 10.1134/S1062359017080027
Alasalvar, C., Taylor, K. D. A., Zubcov, E., Shahidi, F., and Alexis, M. (2002). Differentiation of cultured and wild sea bass (Dicentrarchus labrax): total lipid content fatty acid and trace mineral composition. Food Chem. 79, 145–150.
Andrew, J. E., Bégout Anras, M. L., Kadri, S., Holm, J., and Huntingford, F. A. (2003). Feeding responses of hatchery-reared gilthead seabream (Sparus aurata L.) to a commercial diet and natural prey items. Mar. Fresh. Behav. Physiol. 36, 77–86. doi: 10.1080/1023624031000109864
Arechavala-Lopez, P., Sanchez-Jerez, P., Izquierdo-Gomez, D., Toledo-Guedes, T., and Bayle-Sempere, J. T. (2013a). Does fin damage allow discrimination among wild, escaped and farmed Sparus aurata (L.) and Dicentrarchus labrax (L.)? J. Appl. Inctyol. 29, 352–357. doi: 10.1111/jai.12090
Arechavala-Lopez, P., Fernandez-Jover, D., Black, K. D., Ladoukakis, E., Bayle-Sempere, J. T., Sanchez-Jerez, P., et al. (2013b). Differentiating wild or farmed origin of Mediterranean fish: a review of tools for sea bream and sea bass. Rev. Aquacult. 4, 1–21.
Arechavala-Lopez, P., Sanchez-Jerez, P., Bayle-Sempere, J. T., Sfakianakis, D. G., and Somarakis, S. (2012). Morphological differences between wild and farmed Mediterranean fish. Hydrobiologia 679, 217–231. doi: 10.1007/s10750-011-0886-y
Arechavala-Lopez, P., Toledo-Guedes, K., Izquierdo-Gomez, D., Šegvić-Bubić, T., and Sanchez-Jerez, P. (2017). Implication of sea bream and sea bass escapes for sustainable aquaculture management: a review of interactions, risks and consequences. Rev. Fish. Sci. Aquac. 26, 214–234. doi: 10.1080/23308249.2017.1384789
Bacher, K., Gordoa, A., and Sagué, O. (2012). Spatial and temporal extension of wild fish aggregations at Sparus aurata and Thunnus thynnus farms in the north-western Mediterranean. Aquacult. Environ. Interact. 2, 239–252. doi: 10.3354/aei00042
Ballester-Moltó, M., Sanchez-Jerez, P., and Aguado-Giménez, F. (2017). Consumption of particulate wastes derived from cage fish farming by aggregated wild fish. An experimental approach. Mar. Environ. Res. 130, 166–173. doi: 10.1016/j.marenvres.2017.07.014
Bayle-Sempere, J. T., Arreguín-Sánchez, F., Sanchez-Jerez, P., Salcido-Guevara, L. A., Fernandez-Jover, D., and Zetina-Rejón, M. J. (2013). Trophic structure and energy fluxes around a Mediterranean fish farm. Ecol. Model. 248, 135–147. doi: 10.1016/j.ecolmodel.2012.08.028
Bonhomme, V., Picq, S., Gaucherel, C., and Claude, J. (2014). Momocs: outline analysis using R. J. Stat. Softw. 56:24. doi: 10.18637/jss.v056.i13
Bracciali, C., Guzzo, G., Giacoma, C., Dean, J. M., and Sará, G. (2016). Fish functional traits are affected by hydrodynamics at small spatial scale. Mar. Environ. Res. 113, 116–123. doi: 10.1016/j.marenvres.2015.12.002
Campana, S. E., and Casselman, J. M. (1993). Stock discrimination using otolith shape analysis. Can. J. Fish. Aquat. Sci. 50, 1062–1083. doi: 10.1139/f93-123
Cardinale, M., Doering-Arjes, P., Kastowsky, M., and Mosegaard, H. (2004). Effects of sex, stock and environment on the shape of known-age Atlantic cod (Gadus morhua) otoliths. Can. J. Fish. Aquat. Sci. 61, 158–167. doi: 10.1139/f03-151
Claude, J. (2008). Morphometrics With R. Use R! Series. Berlin: Springer, 316. doi: 10.1007/978-0-387-77789-4
Croissant, Y. (2019). Estimation of Multinomial Logit Models in R: The Mlogit Packages. Réunion: Universite de la Reunion.
Delany, M. F., Linda, S. B., and Moore, C. T. (1999). Diet and condition of American alligators in 4 Florida lakes. Proc. Annu. Conf. Southeast. Assoc. Fish and Wildl. Agencies 53, 375–389.
Dempster, T., Sanchez-Jerez, P., Bayle-Sempere, J. T., Giménez-Casalduero, F., and Valle, C. (2002). Attraction of wild fish to sea-cage fish farms in the south-western Mediterranean Sea: spatial and short-term temporal variability. Mar. Ecol. Prog. Ser. 242, 237–252. doi: 10.3354/meps242237
FAO, (2020). The State of World Fisheries and Aquaculture 2020. Sustainability in Action. Rome: FAO, doi: 10.4060/ca9229en
Fernandez-Jover, D., Mladineo, I., Grubišić, L., Lušić, J., and Sanchez-Jerez, P. (2020). Changes in trophic behaviour and trace metal concentrations in wild fish in a tuna-farming environment. The key role of a sound baitfish choice. Reg. Stud. Mar. Sci. 30:101357. doi: 10.1016/j.rsma.2020.101357
Fernández-Palacios, H., Izquierdo, M., Norberg, B., and Hamre, K. (2011). “Effects of broodstock diet on eggs and larvae,” in Larval Fish Nutrition, ed. G. J. Holt (Hoboken, NJ: Wiley-Blackwell), 153–182.
Fragkoulis, S., Christou, M., Karo, R., Ritas, C., Tzokas, C., Batargias, C., et al. (2016). Scaling of body-shape quality in reared gilthead seabream Sparus aurata L. Consumers preference assessment, wild standard and variability in reared phenotype. Aquac. Res. 48, 2402–2410. doi: 10.1111/are.13076
Geladakis, G., Somarakis, S., and Koumoundouros, G. (2020). Differences in otolith shape and fluctuating-asymmetry between reared and wild gilthead seabream (Sparus aurata Linnaeus, 1758). J. Fish. Biol. 98, 277–286. doi: 10.1111/jfb.14578
Gill, A. B. (2003). The dynamics of prey choice in fish: the importance of prey site and sationation. J. Fish. Biol. 63, 105–116. doi: 10.1046/j.1095-8649.2003.00214.x
Glamuzina, B., Pešić, A., Joksimović, A., Glamuzina, L., Matić-Skoko, S., Conides, A., et al. (2014). Observations on the increase of wild gilthead seabream, Sparus aurata abundance, in the eastern Adriatic Sea: problem and opportunities. Int. Aquat. Res. 6, 127–134.
Grigorakis, K., Alexis, M. N., Taylor, K. D. A., and Hole, M. (2002). Comparison of wild and cultured gilthead sea bream; composition, appearance and seasonal alterations. Int. J. Food Sci. Technol. 37, 477–484. doi: 10.1046/j.1365-2621.2002.00604.x
Hacunda, J. S. (1981). Trophic relationships among demersal fishes in a coastal area of the Gulf of Maine. Fish. Bull. 79, 775–788.
Hammer, Ø, and Harper, D. A. T. (2001). PAST: paleontological statistics software package for education and data analysis. Palaeontologia Electronica 4:9.
Harbitz, A., and Albert, O. T. (2015). Pitfalls in stock discrimination by shape analysis of otolith contours. ICES J. Mar. Sci. 72, 2090–2097. doi: 10.1093/icesjms/fsv048
Hyslop, E. J. (1980). Stomach content analysis - a review of methods and their application. J. Fish Biol. 17, 411–429. doi: 10.1111/j.1095-8649.1980.tb02775.x
Kara, M. H., and Quignard, J. P. (2019). Fisheries in Lagoons and Estuaries in the Mediterranean 3B. Migratory Fish. London: John Wiley & Sons, Inc.
Klingenberg, C. P. (2011). MoprhoJ: an integrated software package for geometric morphometrics. Mol. Ecol. Resour. 11, 353–357. doi: 10.1111/j.1755-0998.2010.02924.x
Lloret, J., Shulman, G., and Love, M. R. (2014). Condition and Health Indicators of Exploited Marine Fishes. Oxford: John Wiley & Sons.
Maître, A., and Mitteroecker, P. (2019). Multivariate comparison of variance in R. Methods Ecol. Evol. 10, 1380–1392. doi: 10.1111/2041-210X.13253
McFadden, D. (1987). Regression-based specification tests for the multinomial logit model. J. Econom. 34, 63–82. doi: 10.1016/0304-4076(87)90067-4
Mille, T., Mahé, K., Cachera, M., Villanueva, M. C., Pontual, H., and Ernande, B. (2016). Diet is correlated with otolith shape in marine fish. Mar. Ecol. Prog. Ser. 555, 167–184. doi: 10.3354/meps11784
Moretti, A., Fernandez-Criado, M., Cittolin, G., and Guidastri, R. (1999). Manual on Hatchery Production of Seabass and Gilthead Seabream, Vol. 1. Rome: FAO.
Mourente, G., and Tocher, D. R. (2009). Tuna nutrition and feeds: current status and future perspectives. Rev. Fish. Sci. 17, 373–390. doi: 10.1080/10641260902752207
Norton, S. F., Luczkovich, J. J., and Motta, P. J. (1995). The role of ecomorphological traits in the comparative biology of fishes. Environ. Biol. Fish. 44, 287–304.
Pavlidis, M. A., Chatzifotis, S., and Adachi, K. (2011). “Pigmentation physiology and discoloration problem,” in Sparidae: Biology and Aquaculture of Gilthead Sea Bream and Other Species, eds M. Pavlidis and M. C. Mylonas (Oxford: Wiley-Blackwell), 295–321.
Pita, C., Gamito, S., and Erzini, K. (2002). Feeding habits of the gilthead seabream (Sparus aurata) from the Ria Formosa (southern Portugal) as compared to the black seabream (Spondyliosoma cantharus) and the annular seabream (Diplodus annularis). J. Appl. Ichthyol. 18, 81–86. doi: 10.1046/j.1439-0426.2002.00336.x
Pleadin, J., Lešić, T., Krešić, G., Barić, R., Bogdanović, T., Oraić, D. V., et al. (2017). Nutritional quality of different fish species farmed in the Adriatic Sea. Ita. J. Food. Sci. 29, 537–543. doi: 10.14674/IJFS-706
Pulcini, D., Capoccioni, F., Franceschini, S., Martinoli, M., and Tibaldi, E. (2020). Skin pigmentation in gilthead seabream (Sparus aurata L.) fed conventional and novel protein sources in diets deprived of fish meal. Animals 10:2138. doi: 10.3390/ani10112138
Rodgveller, C. J., Hutchinson, C. E., Harris, J. P., Vulstek, S. C., and Guthrie, C. M. (2017). Otolith shape variability and associated body growth differences in giant grenadier. Albatrossia pectoralis. PLoS One 12:e0180020. doi: 10.1371/journal.pone.0180020
Rogdakis, Y. G., Koukou, K. K., Ramfos, A., Dimitriou, E., and Katselis, G. N. (2011). Comparative morphology of wild, farmed and hatchery-released gilthead sea bream (Sparus aurata) in western Greece. Int. J. Fish. Aquac. 3, 1–9. doi: 10.5897/IJFA.9000006
Rohlf, F. J. (2010). TPS Dig 2.16 Software. New York NY: State University of New York at Stony Brook.
Russo, T., Costa, C., and Cataudella, S. (2007). Correspondence between shape and feeding habit changes throughour ontogeny of gilthead seabream Sparus aurata L., 1758. J. Fish Biol. 71, 629–656. doi: 10.1111/j.1095-8649.2007.01528.x
Russo, T., Scardi, M., and Cataudella, S. (2014). Application of self-organizing maps for ecomorphological investigation throught early ontogeny of fish. PLoS One 9:e86646. doi: 10.1371/journal.pone.0086646
Sanchez-Jerez, P., Fernandez-Jover, D., Uglem, I., Arechavala-Lopez, P., Dempster, T., Bayle-Sempere, J. T., et al. (2011). “Coastal fish farms as fish aggregation devices (FADs),” in Artificial Reefs in Fisheries Management, eds S. A. Bortone, F. P. Brandini, G. Fabi, and S. Otake (Boca Raton, FL: CRC Press), 187–208.
Šegvić-Bubić, T., Grubišić, L., Tičina, V., and Katavić, I. (2011a). Temporal and spatial variability of pelagic wild fish assemblages around Atlantic bluefin tuna Thunnus thynnus farms in the eastern Adriatic Sea. J. Fish. Biol. 78, 78–97. doi: 10.1111/j.1095-8649.2010.02837.x
Šegvić-Bubić, T., Lepen, I., Trumbić, Ž, Ljubković, J., Sutlović, D., Matić-Skoko, S., et al. (2011b). Population genetic structure of reared and wild gilthead sea bream (Sparus aurata) in the Adriatic Sea inferred with microsatellite loci. Aquaculture 318, 309–315. doi: 10.1016/j.aquaculture.2011.06.007
Šegvić-Bubić, T., Talijančić, I., Grubišić, L., Izquierdo-Gomez, D., and Katavić, I. (2014). Morphological and molecular differentiation of wild and farmed gilthead seabream Sparus aurata: implications for management. Aquacult Environ Interact 6, 43–54.
Šimat, V., Bogdanović, T., Krželj, M., Soldo, A., and Maršić-Lučić, J. (2012). Differences in chemical, physical and sensory properties during shelf life assessment of wild and farmed gilthead sea bream (Sparus aurata, L.). J. Appl. Ichthyol. 28, 95–101. doi: 10.1111/j.1439-0426.2011.01883.x
Skúlason, S., Person, K. J., Svanbäck, R., Räsänen, K., Ferguson, M. M., Adams, C. E., et al. (2019). A way forward with eco evo devo: an extendent theory of resource polymorphism with postglacial fishes as model systems. Biol. Rev. 94, 1786–1808. doi: 10.1111/brv.12534
Skúlason, S., and Smith, T. B. (1995). Resource polymorphisms in vertebrates. Tree 10, 366–370. doi: 10.1016/S0169-5347(00)89135-1
Smith, T. B., and Skúlason, S. (1996). Evolutionary significance of resource polymorphisms in fishes, amphibians, and birds. Annu. Rev. Evol. Syst. 27, 111–133. doi: 10.1146/annurev.ecolsys.27.1.111
Stagličić, N., Šegvić-Bubić, T., Ugarković, P., Talijančić, I., Žužul, I., Tičina, V., et al. (2017). Ecological role of bluefin tuna (Thunnus thynnus) fish farms for associated wild fish assemblages in the Mediterranean Sea. Mar. Environ. Res. 132, 79–93. doi: 10.1016/j.marenvres.2017.10.015
Stevens, M., Párraga, C. A., Cuthill, I. C., Partridge, J., and Troscianko, T. S. (2007). Using digital photography to study animal coloration. Biol. J. Lin. Soc. 90, 211–237. doi: 10.1111/j.1095-8312.2007.00725.x
Stevenson, D. K., and Campana, S. E. (1992). Otolith microstructure examination and analysis. Can. Spec. Publ. Fish. Aquat.Sci 177:126. doi: 10.13140/RG.2.2.22258.61127
Talijančić, I., Šegvić-Bubić, T., Žužul, I., Džoić, T., Maršić-Lučić, J., and Grubišić, L. (2019). Morphological and ecophysiological adaptations of wild gilthead seabream Sparus aurata associated with tuna farms. Aquacult. Environ. Interact. 11, 97–110. doi: 10.3354/aei00111
Tan, K., Ma, H., Li, S., and Zheng, H. (2020). Bivalvia as future source of sustainable natural omega-3 polyunsaturated fatty acids. Food Chem. 311:125907. doi: 10.1016/j.foodchem.2019.125907
Townsend, C. R., and Winfield, I. J. (1985). “’The application of optimal foraging theory to feeding behaviour in fish,” in Fish Energetics, eds P. Tytler and P. Calow (Dordrecht: Springer Science Business Media), 67–98.
Trujillo, P., Piroddi, C., and Jacquet, J. (2012). Fish farms at sea: the ground truth from Google Earth. PLoS One. 7:6. doi: 10.1371/journal.pone.0030546
Valle, C., Bayle-Sempere, J. T., Dempster, T., Sanchez-Jerez, P., and Giménez-Casalduero, F. (2007). Temporal variability of wild fish assemblages associated with a sea-cage fish farm in the south-western Mediterranean Sea. Estuar. Coast. Shelf. S. 72, 299–307. doi: 10.1016/j.ecss.2006.10.019
Van Belleghem, S. M., Papa, R., Ortiz-Zuazaga, H., Hendrickx, F., Jiggins, C. D., McMillan, W. O., et al. (2017). patternize: an R package for quantifying colour pattern variation. Methods Ecol. Evol. 9, 390–398. doi: 10.1111/2041-210X.12853
Van Buskirk, J., and Steiner, U. K. (2009). The fitness cost of developmental canalization and plasticity. J. Evol. Biol. 22, 852–860. doi: 10.1111/j.1420-9101.2009.01685.x
Vignon, M., and Moran, F. (2010). Environmental and genetic determinant of otolith shape revealed by a non-indigenous tropical fish. Mar. Ecol. Prog. Ser. 411, 231–241. doi: 10.3354/meps08651
Vita, R., Marin, A., Madrid, J. A., Jiménez-Brinquis, B., Cesar, A., and Marin-Guirao, L. (2004). Effects of wild fishes of waste exportation from a Mediterranean fish farm. Mar. Ecol. Prog. Ser. 277, 253–261. doi: 10.3354/meps277253
Webb, P. W. (1984). Body form, locomotion and foraging in aquatic vertebrates. Amer. Zool. 24, 107–120.
Weller, H. I., and Westneat, M. W. (2019). Quantitative color profiling of digital images with earth mover’s distance using R package colordistance. PeerJ 7, e6398. doi: 10.7717/peerj.6398
Žužul, I., Šegvić-Bubić, T., Talijančić, I., Džoić, T., Lepen Pleić, I., Beg Paklar, G., et al. (2019). Spatial connectivity pattern of expanding gilthead seabream populations and its interactions with aquaculture sites: a combined population genetic and physical modelling approach. Sci. Rep. 9:14718. doi: 10.1038/s41598-019-51256-z
Keywords: aquaculture, gilthead seabream, phenotype, morphology, foraging, proximate composition, colour, otolith shape
Citation: Talijančić I, Žužul I, Kiridžija V, Šiljić J, Pleadin J, Grubišić L and Šegvić-Bubić T (2021) Plastic Responses of Gilthead Seabream Sparus aurata to Wild and Aquaculture Pressured Environments. Front. Mar. Sci. 8:694627. doi: 10.3389/fmars.2021.694627
Received: 13 April 2021; Accepted: 07 June 2021;
Published: 02 July 2021.
Edited by:
Pedro M. Costa, New University of Lisbon, PortugalReviewed by:
Tommaso Russo, University of Rome Tor Vergata, ItalyPablo Sanchez-Jerez, University of Alicante, Spain
Copyright © 2021 Talijančić, Žužul, Kiridžija, Šiljić, Pleadin, Grubišić and Šegvić-Bubić. This is an open-access article distributed under the terms of the Creative Commons Attribution License (CC BY). The use, distribution or reproduction in other forums is permitted, provided the original author(s) and the copyright owner(s) are credited and that the original publication in this journal is cited, in accordance with accepted academic practice. No use, distribution or reproduction is permitted which does not comply with these terms.
*Correspondence: Tanja Šegvić-Bubić, dGFuamEuc2VndmljQGl6b3IuaHI=